Thank you for visiting nature.com. You are using a browser version with limited support for CSS. To obtain the best experience, we recommend you use a more up to date browser (or turn off compatibility mode in Internet Explorer). In the meantime, to ensure continued support, we are displaying the site without styles and JavaScript.
- View all journals
- My Account Login
- Explore content
- About the journal
- Publish with us
- Sign up for alerts
- Open access
- Published: 14 January 2022
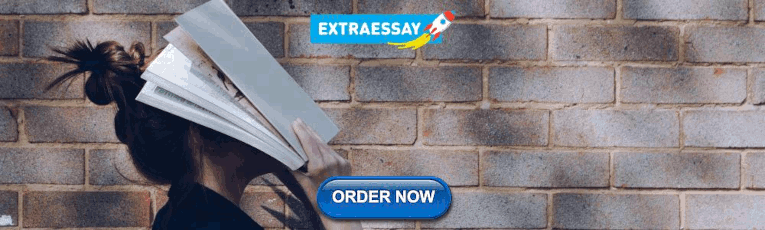
Deep learning-based algorithm for lung cancer detection on chest radiographs using the segmentation method
- Akitoshi Shimazaki 1 ,
- Daiju Ueda 1 , 2 ,
- Antoine Choppin 3 ,
- Akira Yamamoto 1 ,
- Takashi Honjo 1 ,
- Yuki Shimahara 3 &
- Yukio Miki 1
Scientific Reports volume 12 , Article number: 727 ( 2022 ) Cite this article
21k Accesses
50 Citations
7 Altmetric
Metrics details
- Lung cancer
- Radiography
We developed and validated a deep learning (DL)-based model using the segmentation method and assessed its ability to detect lung cancer on chest radiographs. Chest radiographs for use as a training dataset and a test dataset were collected separately from January 2006 to June 2018 at our hospital. The training dataset was used to train and validate the DL-based model with five-fold cross-validation. The model sensitivity and mean false positive indications per image (mFPI) were assessed with the independent test dataset. The training dataset included 629 radiographs with 652 nodules/masses and the test dataset included 151 radiographs with 159 nodules/masses. The DL-based model had a sensitivity of 0.73 with 0.13 mFPI in the test dataset. Sensitivity was lower in lung cancers that overlapped with blind spots such as pulmonary apices, pulmonary hila, chest wall, heart, and sub-diaphragmatic space (0.50–0.64) compared with those in non-overlapped locations (0.87). The dice coefficient for the 159 malignant lesions was on average 0.52. The DL-based model was able to detect lung cancers on chest radiographs, with low mFPI.
Similar content being viewed by others
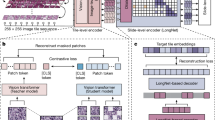
A whole-slide foundation model for digital pathology from real-world data
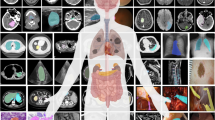
Segment anything in medical images
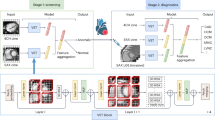
Screening and diagnosis of cardiovascular disease using artificial intelligence-enabled cardiac magnetic resonance imaging
Introduction.
Lung cancer is the primary cause of cancer death worldwide, with 2.09 million new cases and 1.76 million people dying from lung cancer in 2018 1 . Four case-controlled studies from Japan reported in the early 2000s that the combined use of chest radiographs and sputum cytology in screening was effective for reducing lung cancer mortality 2 . In contrast, two randomized controlled trials conducted from 1980 to 1990 concluded that screening with chest radiographs was not effective in reducing mortality in lung cancer 3 , 4 . Although the efficacy of chest radiographs in lung cancer screening remains controversial, chest radiographs are more cost-effective, easier to access, and deliver lower radiation dose compared with low-dose computed tomography (CT). A further disadvantage of chest CT is excessive false positive (FP) results. It has been reported that 96% of nodules detected by low-dose CT screening are FPs, which commonly leads to unnecessary follow-up and invasive examinations 5 . Chest radiography is inferior to chest CT in terms of sensitivity but superior in terms of specificity. Taking these characteristics into consideration, the development of a computer-aided diagnosis (CAD) model for chest radiograph would have value by improving sensitivity while maintaining low FP results.
The recent application of convolutional neural networks (CNN), a field of deep learning (DL) 6 , 7 , has led to dramatic, state-of-the-art improvements in radiology 8 . DL-based models have also shown promise for nodule/mass detection on chest radiographs 9 , 10 , 11 , 12 , 13 , which have reported sensitivities in the range of 0.51–0.84 and mean number of FP indications per image (mFPI) of 0.02–0.34. In addition, radiologist performance for detecting nodules was better with these CAD models than without them 9 . In clinical practice, it is often challenging for radiologists to detect nodules and to differentiate between benign and malignant nodules. Normal anatomical structures often appear as if they are nodules, which is why radiologists must pay careful attention to the shape and marginal properties of nodules. As these problems are caused by the conditions rather than the ability of the radiologist, even skillful radiologists can misdiagnose 14 , 15 .
There are two main methods for detecting lesions using DL: detection and segmentation. The detection method is a region-level classification, whereas the segmentation method is a pixel-level classification. The segmentation method can provide more detailed information than the detection method. In clinical practice, classifying the size of a lesion at the pixel-level increases the likelihood of making a correct diagnosis. Pixel-level classification also makes it easier to follow up on changes in lesion size and shape, since the shape can be used as a reference during detection. It also makes it possible to consider not only the long and short diameters but also the area of the lesion when determining the effect of treatment 16 . However, to our knowledge, there are no studies using the segmentation method to detect pathologically proven lung cancer on chest radiographs.
The purpose of this study was to train and validate a DL-based model capable of detecting lung cancer on chest radiographs using the segmentation method, and to evaluate the characteristics of this DL-based model to improve sensitivity while maintaining low FP results.
The following points summarize the contributions of this article:
This study developed a deep learning-based model for detection and segmentation of lung cancer on chest radiographs.
Our dataset is high quality because all the nodules/masses were pathologically proven lung cancers, and these lesions were pixel-level annotated by two radiologists.
The segmentation method was more informative than the classification or detection methods, which is useful not only for the detection of lung cancer but also for follow-up and treatment efficacy.
Materials and methods
Study design.
We retrospectively collected consecutive chest radiographs from patients who had been pathologically diagnosed with lung cancer at our hospital. Radiologists annotated the lung cancer lesions on these chest radiographs. A DL-based model for detecting lung cancer on radiographs was trained and validated with the annotated radiographs. The model was then tested with an independent dataset for detecting lung cancers. The protocol for this study was comprehensively reviewed and approved by the Ethical Committee of Osaka City University Graduate School of Medicine (No. 4349). Because the radiographs had been acquired during daily clinical practice and informed consent for their use in research had been obtained from patients, the Ethical Committee of Osaka City University Graduate School of Medicine waived the need for further informed consent. All methods were performed in accordance with the relevant guidelines and regulations.
Eligibility and ground truth labelling
Two datasets were used to train and test the DL-based model, a training dataset and a test dataset. We retrospectively collected consecutive chest radiographs from patients pathologically diagnosed with lung cancer at our hospital. The training dataset was comprised of chest radiographs obtained between January 2006 and June 2017, and the test dataset contained those obtained between July 2017 and June 2018. The inclusion criteria were as follows: (a) pathologically proven lung cancer in a surgical specimen; (b) age > 40 years at the time of the preoperative chest radiograph; (c) chest CT performed within 1 month of the preoperative chest radiograph. If the patient had multiple chest radiographs that matched the above criteria, the latest radiograph was selected. Most of these chest radiographs were taken as per routine before hospitalization and were not intended to detect lung cancer. Chest radiographs on which radiologists could not identify the lesion, even with reference to CT, were excluded from analysis. For eligible radiographs, the lesions were annotated by two general radiologists (A.S. and D.U.), with 6 and 7 years of experience in chest radiography, using ITK-SNAP version 3.6.0 ( http://www.itksnap.org/ ) . These annotations were defined as ground truths. The radiologists had access to the chest CT and surgical reports and evaluated the lesion characteristics including size, location, and edge. If > 50% of the edge of the nodule was traceable, the nodule was considered to have a “traceable edge”; if not, it was termed an “untraceable edge”.
Model development
We adopted the CNN architecture using segmentation method. The segmentation method outputs more information than the detection method (which present a bounding box) or the classification method (which determine the malignancy from a single image). Maximal diameter of the tumor is particularly important in clinical practice. Since the largest diameter of the tumor often coincides with an oblique direction, not the horizontal nor the vertical direction, it is difficult to measure with detection methods which present a bounding box. Our CNN architecture was based on the encoder-decoder architecture to output segmentation 17 . The encoder-decoder architecture has a bottleneck structure, which reduces the resolution of the feature map and improves the model robustness to noise and overfitting 18 .
In addition, one characteristic of this DL-based model is that it used both a normal chest radiograph and a black-and-white inversion of a chest radiograph. This is an augmentation that makes use of the experience of radiologists 19 . It is known that black-and-white inversion makes it easier to confirm the presence of lung lesions overlapping blind spots. We considered that this augmentation could be effective for this model as well, so we applied a CNN architecture to each of the normal and inverted images and then an ensemble model using these two architectures 20 . Supplementary Fig. S1 online shows detailed information of the model.
Using chest radiographs from the training dataset, the model was trained and validated from scratch, utilizing five-fold cross-validation. The model when the value of the loss function was the smallest within 100 epochs using Adam (learning rate = 0.001, beta_1 = 0.9, beta_2 = 0.999, epsilon = 0.00000001, decay = 0.0) was adopted as the best-performing.
Model assessment
A detection performance test was performed on a per-lesion basis using the test dataset to evaluate whether the model could identify malignant lesions on radiographs. The model calculated the probability of malignancy in a lesion detected on chest radiographs as an integer between 0 and 255. If the center of output generated by the model was within the ground truth, it was considered true positive (TP). All other outputs were FPs. When two or more TPs were proposed by the model for one ground truth, they were considered as one TP. If there was no output from the model for one ground truth, it was one FN. Two radiologists (A.S. and D.U.) retrospectively referred to the radiograph and CT to evaluate what structures were detected by the FP output. The dice coefficient was also used to evaluate segmentation performance.
Statistical analysis
In the detection performance test, metrics were evaluated on a per-lesion basis. We used the free-response receiver-operating characteristic (FROC) curve to evaluate whether the bounding boxes proposed by the model accurately identified malignant cancers in radiographs 21 . The vertical axis of the FROC curve is sensitivity and the horizontal axis is mFPI. Sensitivity is the number of TPs that the model was able to identify divided by the number of ground truths. The mFPI is the number of FPs that the model mistakenly presented divided by the number of radiographs in the dataset. Thus, the FROC curve shows sensitivity as a function of the number of FPs shown on the image.
One of the authors (D.U.) performed all analyses, using R version 3.6.0 ( https://www.r-project.org/ ). The FROC curves were plotted by R software. All statistical inferences were performed with two-sided 5% significance level.
Figure 1 shows a flowchart of the eligibility criteria for the chest radiographs. For the training dataset, 629 radiographs with 652 nodules/masses were collected from 629 patients (age range 40–91 years, mean age 70 ± 9.0 years, 221 women). For the test dataset, 151 radiographs with 159 nodules/masses were collected from 151 patients (age range 43–84 years, mean age 70 ± 9.0 years, 57 women) (Table 1 ).
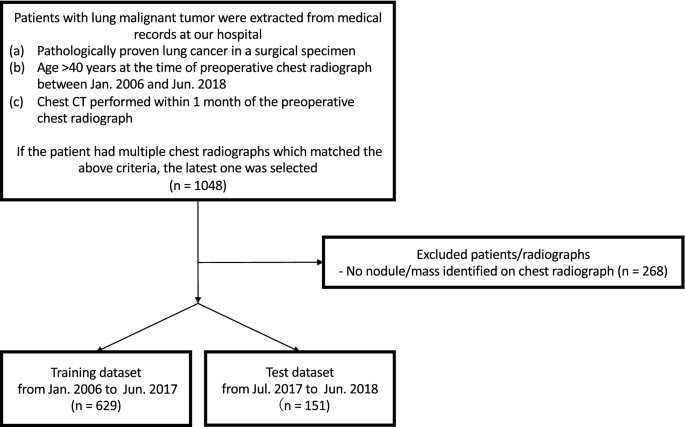
Flowchart of dataset selection.
The DL-based model had sensitivity of 0.73 with 0.13 mFPI in the test dataset (Table 2 ). The FROC curve is shown in Fig. 2 . The highest sensitivity the model attained was 1.00 for cancers with a diameter of 31–50 mm, and the second highest sensitivity was 0.85 for those with a diameter > 50 mm. For lung cancers that overlapped with blind spots such as the pulmonary apices, pulmonary hila, chest wall, heart, or sub-diaphragmatic space, sensitivity was 0.52, 0.64, 0.52, 0.56, and 0.50, respectively. The sensitivity of lesions with traceable edges on radiographs was 0.87, and that for untraceable edges was 0.21. Detailed results are shown in Table 2 .
The dice coefficient for all 159 lesions was on average 0.52 ± 0.37 (standard deviation, SD). For 116 lesions detected by the model, the dice coefficient was on average 0.71 ± 0.24 (SD). The dice coefficient for all 71 lesions overlapping blind spots was 0.34 ± 0.38 (SD). For 39 lesions detected by the model that overlapped with blind spots, the dice coefficient was 0.62 ± 0.29 (SD).
Of the 20 FPs, 19 could be identified as some kind of structure on the chest radiograph by radiologists (Table 3 ). In these 20 FPs, 13 overlapped with blind spots. There were 43 FNs, ranging in size from 9 to 72 mm (mean 21 ± 15 mm), 32 of which overlapped with blind spots (Table 4 ). There were four FNs > 50 mm, all of which overlapped with blind spots. Figure 3 shows representative cases of our model. Figure 4 shows overlapping of a FP output with normal anatomical structures and Fig. 5 shows a FN lung cancer that overlapped with a blind spot. Supplementary Fig. S2 online shows visualized images of the first and last layers. An ablation study to use black-and-white inversion images is shown in Supplementary Data online.
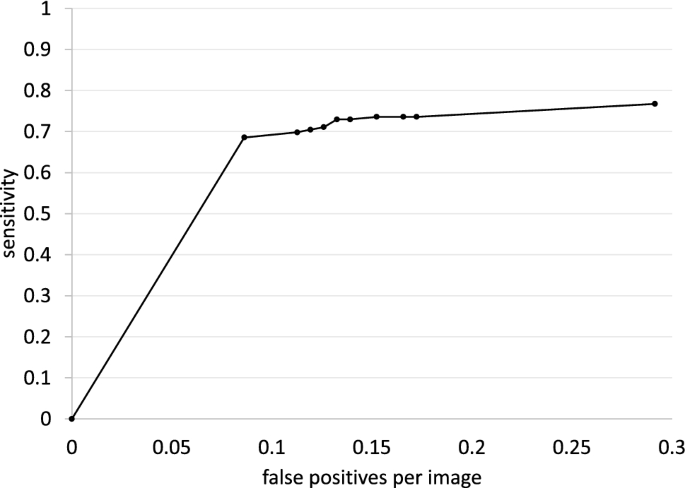
Free-response receiver-operating characteristic curve for the test dataset.
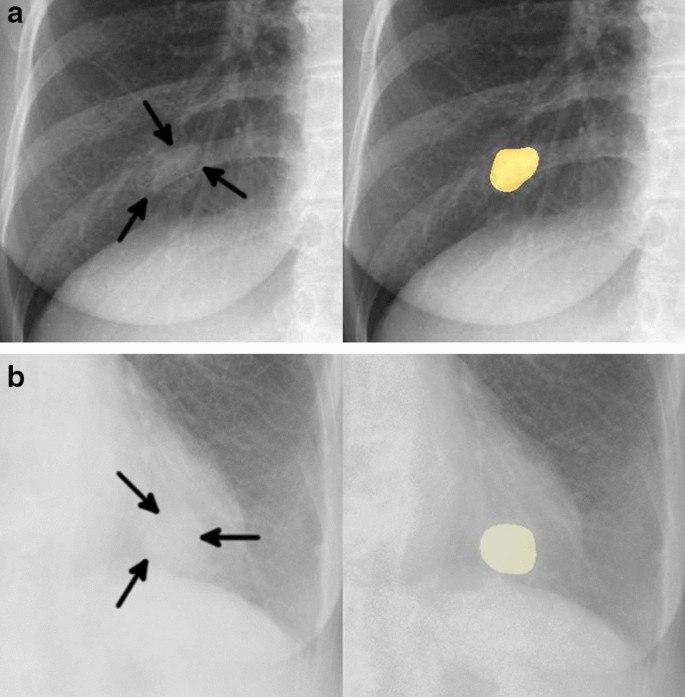
Two representative true positive cases. The images on the left are original images, and those on the right are images output by our model. ( a ) A 48-year-old woman with a nodule in the right lower lobe that was diagnosed as adenocarcinoma. The nodule was confused with rib and vessels (arrows). The model detected the nodule in the right middle lung field. ( b ) A 74-year-old woman with a nodule in the left lower lobe that was diagnosed as squamous cell carcinoma. The nodule overlapped with the heart (arrows). The lesion was identifiable by the model because its edges were traceable.
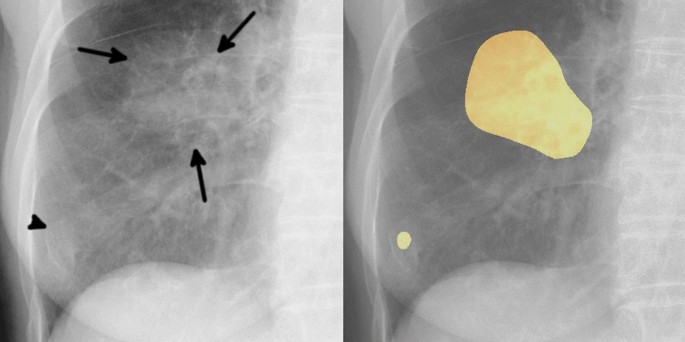
Example of one false positive case. The image on the left is an original image, and the image on the right is an image output by our model. An 81-year-old woman with a mass in the right lower lobe that was diagnosed as squamous cell carcinoma. The mass in the right middle lung field (arrows) was carcinoma. Our model detected this lesion, and also detected a slightly calcified nodule in the right lower lung field (arrowhead). This nodule was an old fracture of the right tenth rib, but was misidentified as a malignant lesion because its shape was obscured by overlap with the right eighth rib and breast.
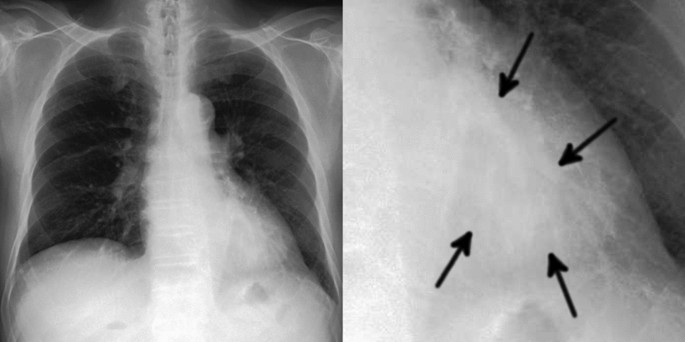
Example of one false negative case. The image on the left is a gross image, and the image on the right is an enlarged image of the lesion. A 68-year-old man with a mass in the left lower lobe that was diagnosed as adenocarcinoma. This lesion overlapped with the heart and is only faintly visible (arrows). Our model failed to detect the mass.
In this study, we developed a model for detecting lung cancer on chest radiographs and evaluated its performance. Adding pixel-level classification of lesions in the proposed DL-based model resulted in sensitivity of 0.73 with 0.13 mFPI in the test dataset.
To our knowledge, ours is the first study to use the segmentation method to detect pathologically proven lung cancer on chest radiographs. We found several studies that used classification or detection methods to detect lung cancer on chest radiographs, but not the segmentation method. Since the segmentation method has more information about the detected lesions than the classification or detection methods, it has advantages not only in the detection of lung cancer but also in follow-up and treatment efficacy. We achieved performance as high as that in similar previous studies 9 , 10 , 11 , 12 , 13 using DL-based lung nodule detection models, with fewer training data. It is particularly noteworthy that the present method achieved low mFPI. In previous studies, sensitivity and mFPI were 0.51–0.84 and 0.02–0.34, respectively, and used 3,500–13,326 radiographs with nodules or masses as the training data, compared with the 629 radiographs used in the present study. Although comparisons to these studies are difficult because the test datasets were different, our accuracy was similar to that of the detection models employed in most of the previous studies. We performed pixel-level classification of the lesions based on the segmentation method and included for analysis only lesions that were pathologically proven to be malignant, based on examination of surgically resected specimens. All previous studies 9 , 10 , 11 , 12 , 13 have included potentially benign lesions, clinically malignant lesions, or pathologically malignant lesions by biopsy in their training data. Therefore, our model may be able to analyze the features of the malignant lesions in more detail. In regard with the CNN, we created this model based on Inception-ResNet-v2 17 , which combines the Inception structure and the Residual connection. In the Inception-ResNet block, convolutional filters of multiple sizes are combined with residual connections. The use of residual connections not only avoids the degradation problem caused by deep structures but also reduces the training time. In theory, the combination of these features further improves the recognition accuracy and learning efficiency 17 . By using this model with combining normal and black-white-inversion images, our results achieved comparable or better performance with fewer training data than previous studies. In regard with the robustness of the model, we consider this model to be relatively robust against imaging conditions or body shape because we consecutively collected the dataset and did not set any exclusion criteria based on imaging conditions or body shape.
The dice coefficient for 159 malignant lesions was on average 0.52. On the other hand, for the 116 lesions detected by the model, the dice coefficient was on average 0.71. These values provide a benchmark for the segmentation performance of lung cancer on chest radiograph. The 71 lesions which overlapped with blind spots tended to have a low dice coefficient with an average of 0.34, but for 39 lesions detected by the model that overlapped with blind spots, the average dice coefficient was 0.62. This means that lesions overlapping blind spots were not only difficult to detect, but also had low accuracy in segmentation. On the other hand, the segmentation accuracy was relatively high for lesions that were detected by the model even if they overlapped with the blind spots.
Two interesting tendencies were found after retrospectively examining the characteristics of FP outputs. First, 95% (19/20) FPs could be visually recognized on chest radiographs as nodule/mass-like structures. The model identified some nodule-like structures (FPs), which overlapped with vascular shadows and ribs. This is also the case for radiologists in daily practice. Second, nodules with calcification overlapped with normal anatomical structures tended to be misdiagnosed by the model (FPs). Five FPs were non-malignant calcified lung nodules on CT and also overlapped with the heart, clavicle or ribs. As the model was trained only on malignant nodules without calcification in the training dataset, calcified nodules should not be identified in theory. Most calcified nodules are actually not identified by the model, however, this was not the case for calcified nodules that overlapped with normal anatomical structures. In other word, there is a possibility that the model could misidentify the lesion as a malignant if the features of calcification that should signal a benign lesion are masked by normal anatomical structures.
When we investigated FNs, we found that nodules in blind spots and metastatic nodules tended to be FNs. With regard to blind spots, our model showed a decrease in sensitivity for lesions that overlapped with normal anatomical structures. It was difficult for the model to identify lung cancers that overlapped with blind spots even when the tumor size was large (Fig. 5 ). In all FNs larger than 50 mm, there was wide overlap with normal anatomical structures, for the possible reason that it becomes difficult for the model to detect subtle density differences in lesions that overlapped with large structures such as the heart. With regard to metastatic nodules, 33% (14/43) metastatic lung cancers were FNs. These metastatic nodules ranged in size from 10 to 20 mm (mean 14 ± 3.8 mm) and were difficult to visually identify on radiographs, even with reference to CT. In fact, the radiologists had overlooked most of the small metastatic nodules at first and could only identify them retrospectively, with knowledge of the type of lung cancer and their locations.
There are some limitations of this study. The model was developed using a dataset collected from a single hospital. Although our model achieved high sensitivity with low FPs, the number of FPs may be higher in a screening cohort and the impact of this should be considered. Furthermore, an observer’s performance study is needed to evaluate the clinical utility of the model. In this study, we included only chest radiographs containing malignant nodules/masses. The fact that we used only pathologically proven lung cancers and pixel-level annotations by two radiologists in our dataset is a strength of our study, on the other hand, it may reduce the detection rate of benign nodules/masses. This is often not a problem in clinical practice. Technically, all areas other than the malignant nodules/masses could be trained as normal areas. However, normal images should be mixed in and tested to evaluate the model for detailed examination in clinical practice.
In conclusion, a DL-based model developed using the segmentation method showed high performance in the detection of lung cancer on chest radiographs. Compared with CT, chest radiographs have advantages in terms of accessibility, cost effectiveness, and low radiation dose. However, the known effectiveness of the model for lung cancer detection is limited. We believe that a CAD model with higher performance can support clinical detection and interpretation of malignant lesions on chest radiographs and offers additive value in lung cancer detection.
Bray, F. et al. Global cancer statistics 2018: GLOBOCAN estimates of incidence and mortality worldwide for 36 cancers in 185 countries. CA Cancer J. Clin. 68 , 394–424. https://doi.org/10.3322/caac.21492 (2018).
Article PubMed Google Scholar
Sagawa, M. et al. The efficacy of lung cancer screening conducted in 1990s: Four case–control studies in Japan. Lung Cancer 41 , 29–36. https://doi.org/10.1016/s0169-5002(03)00197-1 (2003).
Fontana, R. S. et al. Lung cancer screening: The Mayo program. J. Occup. Med. 28 , 746–750. https://doi.org/10.1097/00043764-198608000-00038 (1986).
Article CAS PubMed Google Scholar
Kubik, A. et al. Lack of benefit from semi-annual screening for cancer of the lung: Follow-up report of a randomized controlled trial on a population of high-risk males in Czechoslovakia. Int. J. Cancer 45 , 26–33. https://doi.org/10.1002/ijc.2910450107 (1990).
Raghu, V. K. et al. Feasibility of lung cancer prediction from low-dose CT scan and smoking factors using causal models. Thorax 74 , 643–649. https://doi.org/10.1136/thoraxjnl-2018-212638 (2019).
Hinton, G. Deep learning—a technology with the potential to transform health care. JAMA 320 , 1101–1102. https://doi.org/10.1001/jama.2018.11100 (2018).
LeCun, Y., Bengio, Y. & Hinton, G. Deep learning. Nature 521 , 436–444. https://doi.org/10.1038/nature14539 (2015).
Article ADS CAS PubMed Google Scholar
Ueda, D., Shimazaki, A. & Miki, Y. Technical and clinical overview of deep learning in radiology. Jpn. J. Radiol. 37 , 15–33. https://doi.org/10.1007/s11604-018-0795-3 (2019).
Nam, J. G. et al. Development and validation of deep learning–based automatic detection algorithm for malignant pulmonary nodules on chest radiographs. Radiology 290 , 218–228. https://doi.org/10.1148/radiol.2018180237 (2019).
Park, S. et al. Deep learning-based detection system for multiclass lesions on chest radiographs: Comparison with observer readings. Eur. Radiol. 30 , 1359–1368. https://doi.org/10.1007/s00330-019-06532-x (2020).
Yoo, H., Kim, K. H., Singh, R., Digumarthy, S. R. & Kalra, M. K. Validation of a deep learning algorithm for the detection of malignant pulmonary nodules in chest radiographs. JAMA Netw. Open 3 , e2017135. https://doi.org/10.1001/jamanetworkopen.2020.17135 (2020).
Article PubMed PubMed Central Google Scholar
Sim, Y. et al. Deep convolutional neural network–based software improves radiologist detection of malignant lung nodules on chest radiographs. Radiology 294 , 199–209. https://doi.org/10.1148/radiol.2019182465 (2020).
Hwang, E. J. et al. Development and validation of a deep learning-based automated detection algorithm for major thoracic diseases on chest radiographs. JAMA Netw. Open 2 , e191095. https://doi.org/10.1001/jamanetworkopen.2019.1095 (2019).
Manser, R. et al. Screening for lung cancer. Cochrane Database Syst Rev CD001991. https://doi.org/10.1002/14651858.CD001991.pub3 (2013).
Berlin, L. Radiologic errors, past, present and future. Diagnosis (Berl) 1 , 79–84. https://doi.org/10.1515/dx-2013-0012 (2014).
Article Google Scholar
From the RECIST committee. Schwartz, L.H. et al. RECIST 1.1-Update and clarification. Eur. J. Cancer. 62 , 132–137. https://doi.org/10.1016/j.ejca.2016.03.081 (2016).
Szegedy, C., Ioffe, S., Vanhoucke, V. & Alemi, A. A. Inception-v4, Inception-ResNet and the Impact of Residual Connections on Learning . In Proceedings of the Thirty-First AAAI Conference on Artificial Intelligence , 4278–4284 (AAAI Press, San Francisco, California, USA, 2017).
Matějka P. et al. Neural Network Bottleneck Features for Language Identification. In Proceedings of Odyssey 2014. vol. 2014. International Speech Communication Association , 299–304 (2014).
Sheline, M. E. et al. The diagnosis of pulmonary nodules: Comparison between standard and inverse digitized images and conventional chest radiographs. Am. J. Roentgenol. 152 (2), 261–263. https://doi.org/10.2214/ajr.152.2.261 (1989).
Article CAS Google Scholar
Wang, G., Hao, J., Ma, J. & Jiang, H. A comparative assessment of ensemble learning for credit scoring. Expert. Syst. Appl. 38 (1), 223–230 (2011).
Bunch, P., Hamilton, J., Sanderson, G. & Simmons, A. A free response approach to the measurement and characterization of radiographic observer performance. Proc. SPIE 127 , 124–135. https://doi.org/10.1117/12.955926 (1977).
Article ADS Google Scholar
Download references
Acknowledgements
We are grateful to LPIXEL Inc. for joining this study.
Author information
Authors and affiliations.
Department of Diagnostic and Interventional Radiology, Graduate School of Medicine, Osaka City University, Osaka, Japan
Akitoshi Shimazaki, Daiju Ueda, Akira Yamamoto, Takashi Honjo & Yukio Miki
Smart Life Science Lab, Center for Health Science Innovation, Osaka City University, Osaka, Japan
LPIXEL Inc, Tokyo, Japan
Antoine Choppin & Yuki Shimahara
You can also search for this author in PubMed Google Scholar
Contributions
All authors contributed to the study conception and design. Material preparation, data collection and analysis were performed by A.S., D.U., A.Y. and T.H. Model development was performed by A.C. and Y.S. The first draft of the manuscript was written by A.S. and all authors commented on previous versions of the manuscript. All authors read and approved the final manuscript.
Corresponding author
Correspondence to Daiju Ueda .
Ethics declarations
Competing interests.
Akitoshi Shimazaki has no relevant relationships to disclose. Daiju Ueda has no relevant relationships to disclose. Antoine Choppin is an employee of LPIXEL Inc. Akira Yamamoto has no relevant relationships to disclose. Takashi Honjo has no relevant relationships to disclose. Yuki Shimahara is the CEO of LPIXEL Inc. Yukio Miki has no relevant relationships to disclose.
Additional information
Publisher's note.
Springer Nature remains neutral with regard to jurisdictional claims in published maps and institutional affiliations.
Supplementary Information
Supplementary information., rights and permissions.
Open Access This article is licensed under a Creative Commons Attribution 4.0 International License, which permits use, sharing, adaptation, distribution and reproduction in any medium or format, as long as you give appropriate credit to the original author(s) and the source, provide a link to the Creative Commons licence, and indicate if changes were made. The images or other third party material in this article are included in the article's Creative Commons licence, unless indicated otherwise in a credit line to the material. If material is not included in the article's Creative Commons licence and your intended use is not permitted by statutory regulation or exceeds the permitted use, you will need to obtain permission directly from the copyright holder. To view a copy of this licence, visit http://creativecommons.org/licenses/by/4.0/ .
Reprints and permissions
About this article
Cite this article.
Shimazaki, A., Ueda, D., Choppin, A. et al. Deep learning-based algorithm for lung cancer detection on chest radiographs using the segmentation method. Sci Rep 12 , 727 (2022). https://doi.org/10.1038/s41598-021-04667-w
Download citation
Received : 04 August 2021
Accepted : 29 December 2021
Published : 14 January 2022
DOI : https://doi.org/10.1038/s41598-021-04667-w
Share this article
Anyone you share the following link with will be able to read this content:
Sorry, a shareable link is not currently available for this article.
Provided by the Springer Nature SharedIt content-sharing initiative
This article is cited by
Deep volcanic residual u-net for nodal metastasis (nmet) identification from lung cancer.
- M. Ramkumar
- K. Kalirajan
Biomedical Engineering Letters (2024)
ELCD-NSC2: a novel early lung cancer detection and non-small cell classification framework
- Hadeer A. Helaly
- Mahmoud Badawy
- Amira Y. Haikal
Neural Computing and Applications (2024)
Fairness of artificial intelligence in healthcare: review and recommendations
- Taichi Kakinuma
- Shinji Naganawa
Japanese Journal of Radiology (2024)
Lung tumor analysis using a thrice novelty block classification approach
- S. L. Soniya
- T. Ajith Bosco Raj
Signal, Image and Video Processing (2023)
Radon transform-based improved single seeded region growing segmentation for lung cancer detection using AMPWSVM classification approach
- K. Vijila Rani
- M. Eugine Prince
By submitting a comment you agree to abide by our Terms and Community Guidelines . If you find something abusive or that does not comply with our terms or guidelines please flag it as inappropriate.
Quick links
- Explore articles by subject
- Guide to authors
- Editorial policies
Sign up for the Nature Briefing: Cancer newsletter — what matters in cancer research, free to your inbox weekly.

Risk Prediction Models for Lung Cancer: A Systematic Review
Affiliations.
- 1 Department of School of Health and Related Research, University of Sheffield, Sheffield, United Kingdom. Electronic address: [email protected].
- 2 Department of School of Health and Related Research, University of Sheffield, Sheffield, United Kingdom.
- PMID: 26712102
- DOI: 10.1016/j.cllc.2015.11.007
Many lung cancer risk prediction models have been published but there has been no systematic review or comprehensive assessment of these models to assess how they could be used in screening. We performed a systematic review of lung cancer prediction models and identified 31 articles that related to 25 distinct models, of which 11 considered epidemiological factors only and did not require a clinical input. Another 11 articles focused on models that required a clinical assessment such as a blood test or scan, and 8 articles considered the 2-stage clonal expansion model. More of the epidemiological models had been externally validated than the more recent clinical assessment models. There was varying discrimination, the ability of a model to distinguish between cases and controls, with an area under the curve between 0.57 and 0.879 and calibration, the model's ability to assign an accurate probability to an individual. In our review we found that further validation studies need to be considered; especially for the Prostate, Lung, Colorectal, and Ovarian (PLCO) Cancer Screening Trial 2012 Model Version (PLCOM2012) and Hoggart models, which recorded the best overall performance. Future studies will need to focus on prediction rules, such as optimal risk thresholds, for models for selective screening trials. Only 3 validation studies considered prediction rules when validating the models and overall the models were validated using varied tests in distinct populations, which made direct comparisons difficult. To improve this, multiple models need to be tested on the same data set with considerations for sensitivity, specificity, model accuracy, and positive predictive values at the optimal risk thresholds.
Keywords: Clinical utility; Prediction models; Prediction models' design; Prediction models' discrimination; Validation results.
Copyright © 2016 Elsevier Inc. All rights reserved.
Publication types
- Research Support, Non-U.S. Gov't
- Systematic Review
- Decision Support Techniques*
- Early Detection of Cancer / methods
- Lung Neoplasms / diagnosis*
- Lung Neoplasms / epidemiology
- Models, Statistical
- Predictive Value of Tests
- Risk Assessment
- Risk Factors
- Sensitivity and Specificity
- Introduction
- Conclusions
- Article Information
eAppendix 1. Participant Eligibility and Survey Questions
eAppendix 2. Lung Screening Interview Guide
eAppendix 3. Lung Screening Focus Group Guide
Data Sharing Statement
- Shifting the Lens on Lung Cancer Screening Inequities JAMA Network Open Invited Commentary May 31, 2024 Lisa Carter-Bawa, PhD, MPH, APRN, ANP-C
See More About
Sign up for emails based on your interests, select your interests.
Customize your JAMA Network experience by selecting one or more topics from the list below.
- Academic Medicine
- Acid Base, Electrolytes, Fluids
- Allergy and Clinical Immunology
- American Indian or Alaska Natives
- Anesthesiology
- Anticoagulation
- Art and Images in Psychiatry
- Artificial Intelligence
- Assisted Reproduction
- Bleeding and Transfusion
- Caring for the Critically Ill Patient
- Challenges in Clinical Electrocardiography
- Climate and Health
- Climate Change
- Clinical Challenge
- Clinical Decision Support
- Clinical Implications of Basic Neuroscience
- Clinical Pharmacy and Pharmacology
- Complementary and Alternative Medicine
- Consensus Statements
- Coronavirus (COVID-19)
- Critical Care Medicine
- Cultural Competency
- Dental Medicine
- Dermatology
- Diabetes and Endocrinology
- Diagnostic Test Interpretation
- Drug Development
- Electronic Health Records
- Emergency Medicine
- End of Life, Hospice, Palliative Care
- Environmental Health
- Equity, Diversity, and Inclusion
- Facial Plastic Surgery
- Gastroenterology and Hepatology
- Genetics and Genomics
- Genomics and Precision Health
- Global Health
- Guide to Statistics and Methods
- Hair Disorders
- Health Care Delivery Models
- Health Care Economics, Insurance, Payment
- Health Care Quality
- Health Care Reform
- Health Care Safety
- Health Care Workforce
- Health Disparities
- Health Inequities
- Health Policy
- Health Systems Science
- History of Medicine
- Hypertension
- Images in Neurology
- Implementation Science
- Infectious Diseases
- Innovations in Health Care Delivery
- JAMA Infographic
- Law and Medicine
- Leading Change
- Less is More
- LGBTQIA Medicine
- Lifestyle Behaviors
- Medical Coding
- Medical Devices and Equipment
- Medical Education
- Medical Education and Training
- Medical Journals and Publishing
- Mobile Health and Telemedicine
- Narrative Medicine
- Neuroscience and Psychiatry
- Notable Notes
- Nutrition, Obesity, Exercise
- Obstetrics and Gynecology
- Occupational Health
- Ophthalmology
- Orthopedics
- Otolaryngology
- Pain Medicine
- Palliative Care
- Pathology and Laboratory Medicine
- Patient Care
- Patient Information
- Performance Improvement
- Performance Measures
- Perioperative Care and Consultation
- Pharmacoeconomics
- Pharmacoepidemiology
- Pharmacogenetics
- Pharmacy and Clinical Pharmacology
- Physical Medicine and Rehabilitation
- Physical Therapy
- Physician Leadership
- Population Health
- Primary Care
- Professional Well-being
- Professionalism
- Psychiatry and Behavioral Health
- Public Health
- Pulmonary Medicine
- Regulatory Agencies
- Reproductive Health
- Research, Methods, Statistics
- Resuscitation
- Rheumatology
- Risk Management
- Scientific Discovery and the Future of Medicine
- Shared Decision Making and Communication
- Sleep Medicine
- Sports Medicine
- Stem Cell Transplantation
- Substance Use and Addiction Medicine
- Surgical Innovation
- Surgical Pearls
- Teachable Moment
- Technology and Finance
- The Art of JAMA
- The Arts and Medicine
- The Rational Clinical Examination
- Tobacco and e-Cigarettes
- Translational Medicine
- Trauma and Injury
- Treatment Adherence
- Ultrasonography
- Users' Guide to the Medical Literature
- Vaccination
- Venous Thromboembolism
- Veterans Health
- Women's Health
- Workflow and Process
- Wound Care, Infection, Healing
Get the latest research based on your areas of interest.
Others also liked.
- Download PDF
- X Facebook More LinkedIn
Richmond J , Fernandez JR , Bonnet K, et al. Patient Lung Cancer Screening Decisions and Environmental and Psychosocial Factors. JAMA Netw Open. 2024;7(5):e2412880. doi:10.1001/jamanetworkopen.2024.12880
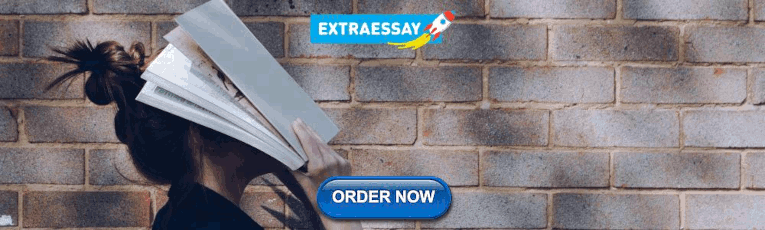
Manage citations:
© 2024
- Permissions
Patient Lung Cancer Screening Decisions and Environmental and Psychosocial Factors
- 1 Division of Public Health Sciences, Department of Social Sciences and Health Policy, Wake Forest University School of Medicine, Winston-Salem, North Carolina
- 2 Division of Intramural Research, National Institute on Minority Health and Health Disparities, National Institutes of Health, Bethesda, Maryland
- 3 Department of Psychology, Vanderbilt University, Nashville, Tennessee
- 4 Qualitative Research Core, Vanderbilt University Medical Center, Nashville, Tennessee
- 5 Division of Geriatric Medicine, Department of Medicine, Vanderbilt University Medical Center, Nashville, Tennessee
- 6 Division of Genetic Medicine, Department of Medicine, Vanderbilt University Medical Center, Nashville, Tennessee
- 7 Department of Biomedical Informatics, Vanderbilt University Medical Center, Nashville, Tennessee
- 8 Department of Thoracic Surgery, Vanderbilt University Medical Center, Nashville, Tennessee
- 9 NORC at the University of Chicago, Bethesda, Maryland
- Invited Commentary Shifting the Lens on Lung Cancer Screening Inequities Lisa Carter-Bawa, PhD, MPH, APRN, ANP-C JAMA Network Open
Question Do environmental, psychosocial, and modifying factors influence patient decisions to undergo screening for lung cancer?
Findings In this qualitative study consisting of interviews and focus groups with 34 individuals eligible for lung cancer screening, participants emphasized historical and present-day racism as a critical factor contributing to mistrust of health care practitioners and avoidance of cancer screening. Participants also identified key psychosocial and modifying factors involved in lung cancer screening decisions, such as health insurance coverage and fear of a cancer diagnosis.
Meaning The findings suggest that systems-level interventions are needed to address environmental, psychosocial, and modifying barriers to effective lung cancer screening decision-making.
Importance Screening for lung cancer using low-dose computed tomography is associated with reduced lung cancer–specific mortality, but uptake is low in the US; understanding how patients make decisions to engage with lung cancer screening is critical for increasing uptake. Prior research has focused on individual-level psychosocial factors, but environmental factors (eg, historical contexts that include experiencing racism) and modifying factors—those that can be changed to make it easier or harder to undergo screening—also likely affect screening decisions.
Objective To investigate environmental, psychosocial, and modifying factors influencing lung cancer screening decision-making and develop a conceptual framework depicting relationships between these factors.
Design, Setting, and Participants This multimethod qualitative study was conducted from December 2021 to June 2022 using virtual semistructured interviews and 4 focus groups (3-4 participants per group). All participants met US Preventive Services Task Force eligibility criteria for lung cancer screening (ie, age 50-80 years, at least a 20 pack-year smoking history, and either currently smoke or quit within the past 15 years). Screening-eligible US participants were recruited using an online panel.
Main Outcomes and Measures Key factors influencing screening decisions (eg, knowledge, beliefs, barriers, and facilitators) were the main outcome. A theory-informed, iterative inductive-deductive approach was applied to analyze data and develop a conceptual framework summarizing results.
Results Among 34 total participants (interviews, 20 [59%]; focus groups, 14 [41%]), mean (SD) age was 59.1 (4.8) years and 20 (59%) identified as female. Half had a household income below $20 000 (17 [50%]). Participants emphasized historical and present-day racism as critical factors contributing to mistrust of health care practitioners and avoidance of medical procedures like screening. Participants reported that other factors, such as public transportation availability, also influenced decisions. Additionally, participants described psychosocial processes involved in decisions, such as perceived screening benefits, lung cancer risk appraisal, and fear of a cancer diagnosis or harmful encounters with practitioners. In addition, participants identified modifying factors (eg, insurance coverage) that could make receiving screening easier or harder.
Conclusions and Relevance In this qualitative study of patient lung cancer screening decisions, environmental, psychosocial, and modifying factors influenced screening decisions. The findings suggest that systems-level interventions, such as those that help practitioners understand and discuss patients’ prior negative health care experiences, are needed to promote effective screening decision-making.
Lung cancer is the leading cause of cancer death in the US. 1 Screening for lung cancer using low-dose computed tomography (LDCT) of the chest is associated with reduced lung cancer–specific mortality because it improves the chances of an early-stage diagnosis. 2 , 3 The US Preventive Services Task Force (USPSTF) recommends annual lung cancer screening for adults aged 50 to 80 years with at least a 20 pack-year smoking history and who currently smoke or have quit smoking within the past 15 years. 4 Pack-years are calculated by multiplying the number of cigarette packs that someone has smoked per day by the number of years they have smoked. 5 Lung cancer screening uptake is low, ranging from 5% to 21% among eligible individuals in the US. 6 - 9 Screening receipt is even lower among US populations that bear a disproportionate burden of lung cancer, such as Black individuals. 10 - 15 In the US, Black individuals are 16% less likely than White individuals to survive 5 years after a lung cancer diagnosis but are also less likely than White individuals to receive LDCT. 13 , 16 , 17
Understanding the lung cancer screening decision-making process is a necessary component of increasing uptake. This process is especially important for lung cancer screening because the Centers for Medicare & Medicaid Services requires that Medicare beneficiaries have a documented shared decision-making visit before undergoing LDCT to understand potential benefits and harms. 18 Therefore, it is vital that health care practitioners understand the factors that patients consider when making screening decisions so they can offer appropriate information during shared decision-making visits.
Prior research has focused on individual-level psychosocial factors involved in LDCT decisions (eg, perceived lung cancer risk). 19 - 21 Yet, environmental factors, such as availability of public transportation, may also impact cancer screening decisions. 19 , 22 Furthermore, the US history of racism, such as unethical medical experimentation on Black people, has also affected engagement with the health care system. 23 - 25 Discussions of racism are largely absent from the lung cancer screening literature, though. 19 - 21 , 26 , 27 One notable study created a conceptual model of lung cancer screening participation that highlights contributing social and environmental factors. 26 That model focuses on media exposure and influences of family and friends as social or environmental factors, creating opportunities to investigate racism and other environmental factors. 26 , 28 Finally, modifying factors—factors that can be changed to make it easier or harder to receive screening—are understudied in screening decision-making. To address these knowledge gaps, we examined environmental, psychosocial, and modifying factors influencing lung cancer screening decision-making and developed a conceptual framework to summarize relationships between these factors.
This multimethod qualitative study consisted of virtual semistructured interviews and 4 focus groups (3-4 participants per group) with participants throughout the US. We conducted both interviews and focus groups to diversify the information elicited (eg, information on a wide range of participant experiences from focus groups and detailed information on these experiences from interviews). 29 The sample size was informed by recommendations for conducting online qualitative research and the number of interviews or focus groups needed to reach saturation. 30 - 33 The Vanderbilt University institutional review board deemed this study exempt per 45 CFR §46. 34 Participants provided written informed consent. This study followed the Consolidated Criteria for Reporting Qualitative Research ( COREQ ) reporting guideline.
We used a purposive sampling approach to identify participants with insights relevant to lung cancer screening. 35 Participants were eligible if they met USPSTF eligibility criteria for lung cancer screening (age 50 to 80 years, at least a 20 pack-year smoking history, and either currently smoke or quit within the past 15 years). We also recruited a study population in which about half of participants self-identified as Black, a group that has been disproportionately affected by lung cancer. 1 The race and ethnicity categories included in the study were Asian, Black or African American, Black and American Indian, Black and White, Hispanic or Latino, and White and were ascertained by self-report.
We partnered with CloudResearch, an organization specializing in using online platforms for research recruitment, to identify participants for a 1-time interview or focus group. 36 CloudResearch used its Amazon Mechanical Turk (MTurk) Approved List and Prime Panels platforms to facilitate recruitment. 37 - 41 CloudResearch’s MTurk Approved List is a list of MTurk participants who meet quality metrics (eg, no history of using technology to automatically complete surveys). 41 MTurk has a smaller pool of participants than Prime Panels, but unlike Prime Panels, it supports real-time recruitment, by which researchers post a study and eligible participants can join the interview within minutes. We used MTurk to recruit in real time until we were unable to reach additional participants (after 9 interviews). We used Prime Panels to recruit for the remaining interviews and focus groups. CloudResearch had access to participant demographic information, which was leveraged to purposively recruit a sample in which about half of participants self-identified as Black.
To assess study eligibility, potential participants completed an online REDCap survey (eAppendix 1 in Supplement 1 ). 42 This survey included questions about demographic characteristics, smoking history, and prior screening receipt. Participants who met eligibility criteria received additional study information and reviewed an informed consent form, which included permission to record sessions and publish deidentified findings. Because MTurk supports real-time recruitment, these participants received the link to join the video study after consenting and were permitted to immediately begin the study. Prime Panels has a lag between study posting and when the study is visible to participants. Therefore, Prime Panels participants received information about upcoming interview or focus group times and, after consenting, signed up for a session. No participants dropped out of the study after consenting. All data were stored on secure servers.
We collaborated with a local community advisory board 43 composed of 8 community members with various cancer diagnoses, tobacco use experiences, and racial and ethnic backgrounds and a history of collaborating with the study team (J.R., M.C.A.) on lung cancer research to develop semistructured interview and focus group guides (presented in eAppendixes 2 and 3 in Supplement 1 ). The guides included questions about factors influencing lung cancer screening decisions (eg, knowledge, beliefs, and environmental barriers). Staff with qualitative methods expertise and training at the Vanderbilt University Medical Center Qualitative Research Core 44 (K.B.) conducted interviews and focus groups using a videoconferencing platform. The principal investigator (J.R.), who has doctoral-level training in qualitative methods and has conducted qualitative research for over a decade, observed all sessions, presented the study purpose to participants, and answered questions as needed. No participants had existing relationships with the researchers. The interviewer and principal investigator wrote field notes and regularly debriefed after interviews and focus groups to record insights emerging from the data and to reflexively consider how context (eg, the interviewer’s and principal investigator’s backgrounds) influenced the research. 45 Furthermore, the principal investigator regularly reflected to examine how her background, experiences with cancer, and identity as a multiracial Black woman motivated her to pursue this study.
Interviews lasted approximately 30 to 60 minutes, and focus groups lasted approximately 90 minutes. Interviews and focus groups were audiorecorded and professionally transcribed. Transcripts and study data were not returned to study participants for comment to avoid creating identifiable links between participants and their data. During data collection and analysis, we reviewed field notes and transcripts to examine codes arising in the data and assess saturation. 46 After 20 interviews and 4 focus groups, no new insights were emerging from the data. All sessions occurred between December 2021 and June 2022.
The COREQ guideline informed data coding and analysis. 47 We developed an initial hierarchical coding system and codebook and iteratively refined it by using the interview and focus group guides and a preliminary review of transcripts. 48 Examples of major coding categories include health and social history, emotions and feelings, attitudes and beliefs, coping, encounters with health care practitioners, LDCT decision-making, screening barriers and facilitators, and societal factors relevant to screening.
To establish intercoder reliability, 2 experienced coders independently coded 1 interview and 1 focus group transcript. We compared coding and resolved all discrepancies. After reaching consensus in use of the coding system, the 2 coders divided and independently coded the remaining transcripts. Microsoft 365 Excel, version 2402, and SPSS, version 28.0 (IBM Corp), were used to manage data analysis (eg, to sort files by code and run cross-tabulations to examine code co-occurrences). 49 , 50
We used an iterative inductive-deductive approach to identify higher-order themes. 51 , 52 The goal of this inductive-deductive approach was to develop a conceptual framework deductively informed by established theories while inductively integrating details from the qualitative data. Deductively, the analysis was informed by Social Cognitive Theory, which posits that behavior is influenced by interactions of personal, environmental, and behavioral factors. 53 , 54 We were also guided by the Health Belief Model, a theory positing that health decisions are informed by several factors, such as perceived benefits and risks. 55 These theories have been widely used for decades to explain health behavior and, when combined, may elucidate the influence of psychosocial and environmental factors on health behaviors. 55 - 57 Inductively, the framework’s content was derived from the qualitative data.
A total of 34 individuals participated in this study (20 [59%] in interviews and 14 [41%] in focus groups). Mean (SD) age was 59.1 (4.8) years, and mean (SD) smoking history was 39.3 (21.2) pack-years. Twenty participants (59%) identified as female and 14 (41%) as male. Overall, 2 (6%) identified as Asian, 16 (47%) as Black or African American, 1 (3%) as Black and American Indian, 1 (3%) as Black and White, 1 (3%) as Hispanic or Latino, and 13 (38%) as White. Half of participants (17 [50%]) had an annual household income of less than $20 000. Most participants had health insurance (31 [91%]), lived in the southern US census region (22 [65%]), and had never been screened for lung cancer (27 [79%]). Table 1 presents participant sociodemographic characteristics.
The Figure presents the conceptual framework resulting from our analysis. On the left side of the framework, circles representing psychosocial processes (eg, risk appraisal) interact with aspects of the environment (eg, historical context) to influence screening decisions. The middle of the framework presents arrows representing modifying factors (eg, insurance coverage) that can make it easier or harder to receive screening. In the following sections, we describe each element of the framework. Table 2 presents illustrative participant quotes from each framework element.
Knowledge of and beliefs about the mistreatment of historically and contemporarily marginalized populations in the US influenced several participants’ decisions to seek lung cancer screening. Several participants, most of whom identified as Black, described how experiences of racism cause mistrust and health care avoidance, particularly in Black communities. One participant explained, “In the Black community..., because of slavery, there’s a mistrust of police officers. There’s a mistrust of doctors... And a lot of that distrust comes from racism and it trickles down.” Another participant elaborated that, “there is mistrust among the Black community when it comes to medical care... Because of previous experience, many feel that the best medical care will not be provided to us.” Participants also described community knowledge of health care atrocities that cause avoidance of medical care: “They call it blue ball...that’s just something that Black people call it... And so they just inject them with so much morphine that they pass away.”
Participants also described community cultures in which personal health is not discussed with others, medical visits are reserved for emergencies, and preventive care is avoided. For example, 1 participant explained, “I guess it’s an older generation thing. You didn’t talk about stuff.” Another participant elaborated that in their community, “if you don’t have a gunshot wound or you’re missing a limb, they’re not going to the doctor.” To avoid medical care, some participants described using local healing traditions that had been passed down in their communities.
Participants identified key resources required to engage in lung cancer screening. Most frequently, these resources included income stability and access to transportation. Participants expressed frustration with the US health care system, “because it’s all about money. It’s not about the individual.” Accordingly, participants described how lung cancer screening is inaccessible to those who cannot afford or access it.
Most commonly, participants described screening as an important tool to catch disease early and improve treatment outcomes. Yet, others struggled to describe any benefits and questioned whether screening would benefit them. These participants often explained that they would prefer not to know if they had cancer. One participant noted, “What’s the point [of screening]? If they find it [lung cancer], it’s not good for me. If they don’t, then I’m going to continue to do what I’m doing, even though I’m going to stop smoking for one day.”
Participants largely described the importance of health care practitioner rapport and communication to facilitate trust, which could motivate screening decisions. However, participants frequently described negative, harmful practitioner experiences that diminished their willingness to seek future care. These participants described misdiagnoses, being dismissed when describing pain, and being rushed out of medical offices. One participant described extreme pain that practitioners ignored during a cesarean delivery: “I told them that I was feeling it and they didn’t believe me when they were cutting me... So they ended up just strapping me down, because I told them, ‘I feel this. You’re hurting me.’ They just strapped me down and continued.” Participants, particularly those who had Medicaid or no health insurance at some time in their lives, also described being dismissed due to their insurance status.
Participants often had complex health histories and were diagnosed with comorbidities that impacted screening decisions. These participants expressed exhaustion with frequent medical procedures and explained that other health conditions often took precedence and made it hard to determine screening benefits. One participant described that, “I have vascular disease and I think that’s going to get me before any cancer does.” Conversely, awareness of their health status motivated other participants to engage in preventive care before their health worsened.
Participants had differing perceptions of their personal lung cancer risk. Most participants noted the health risks associated with smoking as a factor that could motivate decisions to undergo screening. One participant explained, “I’m a smoker, so I know I’m at risk... That’s why you [get screened].” Other participants expressed that health-promoting habits (eg, physical activity) might mitigate their lung cancer risk: “I’m still smoking 10-plus cigarettes a day, and I know the health risk, but it doesn’t bother me... Maybe because I’m very active.”
Participants identified “fear of actually discovering [lung cancer]” as a major barrier to screening. Participants also described fear arising from hearing stories about people who were healthy before a routine health care practitioner visit rapidly led to a cancer diagnosis and death. For example, 1 participant described knowing a healthy man who was diagnosed with cancer after undergoing a routine physical examination: “But I think that if he had...not had that physical, I think he would’ve just kept on living. It looked like the moment they told him he had it, he just started going downhill that day.” Participants who mistrusted practitioners also reported fearing future harm from the health care system. To cope with these fears, participants described avoiding medical practitioners.
Participants raised several questions about screening elements, reflecting how screening knowledge and awareness could affect decisions. The most common question was whether LDCT is invasive or painful. Other participants wondered how much time the procedure would take, how far they would have to travel, and how accurate the test is. Many participants also wondered whether they would need someone to pick them up after the procedure.
Participants often expressed interest in being screened but wanted assurance that it would be covered by health insurance. Without insurance coverage, screening was described as inaccessible due to out-of-pocket costs. Although most participants currently had health insurance, they still expressed uncertainty about costs and worried their insurance carrier would not “deem it medically necessary” to avoid paying for screening.
For participants who had received lung cancer screening, direct practitioner communication was a key facilitator motivating their decision. One of these participants described how their doctor “explained it to the T and he said, ‘Now, you go and evaluate. Is this something that you think you want to do?... Because if so, then we’ll catch it early.’” Conversely, participants who had not been screened explained that their practitioners never mentioned it, which was often frustrating to participants with long-term relationships with primary care practitioners. One participant explained that this lack of communication “doesn’t feel good at all because it’s like they’re not concerned about me, my doctors.” At times, participants also expressed worry that screening discussions could lead to uncomfortable conversations in which practitioners shame them for smoking.
We applied a qualitative approach to develop a conceptual framework depicting environmental, psychosocial, and modifying factors influencing lung cancer screening decisions. Study participants emphasized that community cultures influenced screening decisions, mirroring previous research suggesting that social influence is associated with screening participation. 26 , 28 Our study also expands prior work 26 , 28 by eliciting new insights about how aspects of the environment and society (eg, historical context) influence lung cancer screening decision-making. Participants described racism as a key factor contributing to mistrust of practitioners and avoidance of medical procedures. These findings mirror prior evidence suggesting that historical atrocities and harm, especially in Black communities, contribute to present-day mistrust of health care. 58 , 59 Importantly, participants cited examples of harm they experienced in recent history (eg, having pain ignored). Previous research also found that mistrust of medical practitioners and systems arose not only from historical atrocities but also from present-day racism and negative health care experiences. 58 , 60 - 63 To our knowledge, these findings provide some of the first evidence highlighting connections between racism and lung cancer screening decisions. Moving forward, participants noted that practitioners can increase trust by communicating effectively with patients. Such communication efforts should aim to avoid stigmatizing smoking behaviors, an issue raised by participants in our study and found in previous research. 64 , 65 Our study also suggests that trust-building should entail efforts to acknowledge, understand, and reduce the present-day incidence of harmful patient experiences with practitioners. Additionally, future efforts to build trust and promote screening should meaningfully engage with communities and community-based organizations. For example, researchers, patients, and other community members might colead interventions that bring free or low-cost screening into communities through mobile units instead of relying on patients to initiate care from medical spaces that previously harmed them.
Consistent with prior literature, 21 , 66 - 68 participants also highlighted psychosocial factors affecting screening decisions (eg, fear). Furthermore, participants had varying perceptions regarding their personal lung cancer risk and the importance of undergoing screening. These results point to opportunities for shared decision-making approaches that support psychosocial decision-making processes. For example, practitioners might offer information about a patient’s unique lung cancer risk along with potential benefits and screening outcomes. Additionally, shared decision-making discussions might benefit from conversations about community cultures and patient concerns regarding how their ancestors, family, and friends have historically been treated by the health care system. These discussions offer opportunities to understand concerns that would not ordinarily come up during a medical visit. For example, a practitioner might gain a new understanding of how a patient’s family member experienced harm after a cancer diagnosis and initiate conversations on ways to reduce the likelihood of similar harms occurring if the patient is diagnosed with lung cancer. Yet, prior research suggests the quality and occurrence of shared decision-making about lung cancer screening varies widely (often due to practitioner time constraints) and that these discussions often do not elicit patient values. 69 - 75 Accordingly, future interventions are needed to promote tailored shared decision-making discussions.
Interestingly, participants identified a concern consistent with a limitation of the overall scientific evidence base: understanding whether patients who have complex health histories benefit from screening. 76 - 78 Current USPSTF guidelines do not provide concrete guidance about LDCT for patients with comorbidities but instead note that screening should stop if the patient develops a health problem that substantially limits life expectancy or the ability or willingness to receive surgical treatment. 4 In these situations, shared decision-making is often recommended, but more evidence is needed about how comorbidities should be incorporated into decisions.
Similar to other studies, health insurance coverage and cost were critical factors influencing decisions, even for participants with health insurance. 21 Although Medicare and most private insurers cover LDCT in the US, Medicaid does not cover screening in all states, and patients may encounter costs for follow-up procedures. 79 , 80 Participants wanted to know all potential costs up front, showcasing the need for policies that support LDCT insurance coverage and cost transparency. Participants also wanted their practitioners to have information about these costs and general screening elements (eg, screening accuracy). Yet, there is widespread variation in practitioner knowledge about lung cancer screening. 81 - 84 Without such knowledge, patient-practitioner communication (a key modifying factor) may disintegrate. Novel interventions are needed to promote practitioner and patient knowledge of and meaningful communication about lung cancer screening.
This study has important limitations. We purposively recruited participants online, which may have excluded participants with unreliable or no internet access. Participants also self-reported information required to determine lung cancer screening eligibility (eg, smoking history), and results may have been affected by recall and other self-report biases. Additionally, most participants currently smoked, and decisions about lung cancer screening may vary among people who formerly smoked. Lastly, future community-engaged research is needed to apply and increase the transferability of our conceptual framework to larger populations.
In this qualitative study of patient lung cancer screening decision-making, decisions involved interactions among environmental, psychosocial, and modifying factors. Some environmental and psychosocial factors (eg, experiencing racism and fear of a cancer diagnosis) hindered screening decisions, but intervening on modifying factors like patient-practitioner communication may offer future opportunities to facilitate screening uptake.
Accepted for Publication: March 17, 2024.
Published: May 31, 2024. doi:10.1001/jamanetworkopen.2024.12880
Open Access: This is an open access article distributed under the terms of the CC-BY License . © 2024 Richmond J et al. JAMA Network Open .
Corresponding Author: Jennifer Richmond, PhD, MSPH, Division of Public Health Sciences, Department of Social Sciences and Health Policy, Wake Forest University School of Medicine, 525 Vine St, Winston-Salem, NC 27101 ( [email protected] ).
Author Contributions: Dr Richmond had full access to all of the data in the study and takes responsibility for the integrity of the data and the accuracy of the data analysis.
Concept and design: Richmond, Fernandez, Wilkins, Aldrich.
Acquisition, analysis, or interpretation of data: All authors.
Drafting of the manuscript: Richmond, Fernandez, Bonnet, Sellers.
Critical review of the manuscript for important intellectual content: All authors.
Statistical analysis: Richmond, Bonnet, Sellers.
Obtained funding: Richmond.
Administrative, technical, or material support: Richmond, Fernandez, Sellers, Wilkins.
Supervision: Richmond.
Conflict of Interest Disclosures: Dr Fernandez reported receiving support from the Division of Intramural Research of the National Institute on Minority Health and Health Disparities (NIMHD). Dr Schlundt reported receiving payment from Vanderbilt University Medical Center for qualitative work during the conduct of the study. Dr Forde reported being an employee of the NIMHD and receiving support from the Division of Intramural Research of the NIHMD. Dr Wilkins reported receiving grants from the National Institutes of Health during the conduct of the study. Dr Aldrich reported receiving grants from the National Cancer Institute (NCI) and personal fees from Guardant Health outside the submitted work. No other disclosures were reported.
Funding/Support: This work was supported by grant T32HS026122 from the Agency for Healthcare Research and Quality. The study used the REDCap platform, which is supported by grant UL1TR000445 from the National Center for Advancing Translational Sciences. The study was also supported by grants K99CA277366, R00CA277366, and L60CA264691 (Dr Richmond) and R01CA251758 (Dr Aldrich) from the NCI and a Geographic Management of Cancer Health Disparities Program Region 1–South Cancer Health Disparities Professional Development and Research Stimulus Award (Dr Richmond).
Role of the Funder/Sponsor: The funders had no role in the design and conduct of the study; collection, management, analysis, and interpretation of the data; preparation, review, or approval of the manuscript; and decision to submit the manuscript for publication.
Disclaimer: The content is solely the responsibility of the authors and does not necessarily represent the official views of the funding organizations.
Data Sharing Statement: See Supplement 2 .
Additional Contributions: We thank members of the Meharry Medical College/Vanderbilt–Ingram Cancer Center/Tennessee State University Cancer Partnership Community Advisory Board Lung Cancer Subcommittee for their valuable perspectives.
- Register for email alerts with links to free full-text articles
- Access PDFs of free articles
- Manage your interests
- Save searches and receive search alerts
The role of imaging in the diagnosis of lung cancer in primary care
--> Bradley, Stephen (2022) The role of imaging in the diagnosis of lung cancer in primary care. PhD thesis, University of Leeds.
Background Lung cancer is the leading cause of cancer death worldwide. The UK relies more heavily upon chest x-ray than many other high income countries. Little is known about the performance of the test, the risk of cancer following negative test, consequences of ‘false negative’ results and the factors that affect how frequently chest x-ray is used. Aims 1. Determine sensitivity and specificity of chest x-ray. 2. Determine if there are differences in outcomes between patients with ‘true positive’ versus ‘false negative’ chest x-rays. 3. Determine the risk of lung cancer following a negative chest x-ray with respect to symptoms. 4. Quantify the volume of chest x-rays undertaken by English general practices and understand the extent to which variations in chest x-ray frequency are due to differences in patient populations and the practices themselves. Methods 1. Systematic review on sensitivity of chest x-ray 2. Observational study to determine sensitivity and compare stage and survival between those with ‘true positive’ versus ‘false negative’ results. 3. Cohort study to determine chest x-ray specificity and lung cancer risk following negative chest x-ray. 4. Retrospective study to quantify general practices’ chest x-rays with respect to characteristics of their patient populations and the practices. Results 1. Sensitivity was 77-80% (systematic review) and 82% (observational study). Specificity was 90%. 2. ‘False negative’ chest x-rays were not associated with adverse outcomes, although given the retrospective methodology this cannot be excluded. 3. Lung cancer risk following negative chest x-ray was <1% for all symptoms except haemoptysis (3%). 4. There was substantial variation in chest x-ray utilisation (median 34/1000 patients, IQR 26-43), with 18% of variance accounted for by recorded characteristics. Conclusions Chest x-ray does not identify ~20% of lung cancers but it continues to have a useful role. The substantial variation in rates of investigation suggest that it may be underutilised in many practices.
--> Final eThesis - complete (pdf) -->
Filename: Thesis 04 05 22unmarked.pdf

Embargo Date:
You do not need to contact us to get a copy of this thesis. Please use the 'Download' link(s) above to get a copy. You can contact us about this thesis . If you need to make a general enquiry, please see the Contact us page.

Cessation services provided to individuals before their lung cancer screening exam, and the development of a Patient-Reported Outcome Measure for use in lung cancer screening
Add to collection, downloadable content.
- Affiliation: Gillings School of Global Public Health, Department of Health Behavior
- Introduction: Lung Cancer Screening (LCS) is an important timepoint for individuals at high risk of developing lung cancer. Before screening, current smokers must be provided with cessation services, and providers must assess eligibility and engage individuals in a discussion about risks and benefits of screening. This dissertation focused on the requirements of this visit, exploring predictors of cessation services and the symptoms that should be assessed. Methods: In Aim 1, a sample of individuals who smoked at the time of their LCS exam was identified from two hospital systems in North Carolina. Cessation services, individual-level, provider-level, and hospital-level details were abstracted from electronic health records and a questionnaire. Logistic regression models were run for each outcome: receipt of cessation advice and receipt of intensive cessation services (i.e., pharmacotherapy or cessation counseling). In Aim 2, we identified symptom domains to develop a conceptual model for a patient-reported outcome measure (PROM) for individuals receiving LCS. First, we reviewed measures from disease-areas common in the LCS-eligible population to identify potential domains. These were then assessed through qualitative interviews with individuals who received LCS and physicians. Results: Among the 840 individuals in Aim 1, 77% received cessation advice and 38% received intensive services. Referring department was significantly associated with both outcomes. In both regression models, individuals referred from pulmonology were more likely to receive cessation services than individuals referred from family medicine, internal medicine, or other clinics. In Aim 2, potential symptom domains were identified including breathlessness, cough, fatigue, and physical functioning. Stakeholders noted the importance of respiratory domains and the value of assessing generic domains in a respiratory-focused way. Providers reported differing interpretations of how symptoms impact LCS, what symptoms are important to assess and stressed the importance of red-flag symptoms. Conclusions: In both aims, differences in how LCS components are implemented or interpreted emerged by provider departments. In Aim 1, we found significant variation in the cessation services delivered by referring department. Aim 2 identified symptom domains that should be assessed during LCS, though there were differences by provider specialty in what symptoms were perceived as important and how symptoms impact LCS.
- Public health
- Smoking Cessation
- Patient-Reported Outcomes
- Lung Cancer Screening
- https://doi.org/10.17615/yq4y-zm41
- Dissertation
- In Copyright - Educational Use Permitted
- Ribisl, Kurt M
- Stover, Angela M
- Henderson, Louise
- Mills, Sarah D
- Gilkey, Melissa
- Doctor of Public Health
- University of North Carolina at Chapel Hill Graduate School
This work has no parents.
Select type of work
Master's papers.
Deposit your masters paper, project or other capstone work. Theses will be sent to the CDR automatically via ProQuest and do not need to be deposited.
Scholarly Articles and Book Chapters
Deposit a peer-reviewed article or book chapter. If you would like to deposit a poster, presentation, conference paper or white paper, use the “Scholarly Works” deposit form.
Undergraduate Honors Theses
Deposit your senior honors thesis.
Scholarly Journal, Newsletter or Book
Deposit a complete issue of a scholarly journal, newsletter or book. If you would like to deposit an article or book chapter, use the “Scholarly Articles and Book Chapters” deposit option.
Deposit your dataset. Datasets may be associated with an article or deposited separately.
Deposit your 3D objects, audio, images or video.
Poster, Presentation, Protocol or Paper
Deposit scholarly works such as posters, presentations, research protocols, conference papers or white papers. If you would like to deposit a peer-reviewed article or book chapter, use the “Scholarly Articles and Book Chapters” deposit option.

An official website of the United States government
The .gov means it’s official. Federal government websites often end in .gov or .mil. Before sharing sensitive information, make sure you’re on a federal government site.
The site is secure. The https:// ensures that you are connecting to the official website and that any information you provide is encrypted and transmitted securely.
- Publications
- Account settings
Preview improvements coming to the PMC website in October 2024. Learn More or Try it out now .
- Advanced Search
- Journal List
- HHS Author Manuscripts

COMPLICATED GRIEF SYMPTOMS IN CAREGIVERS OF PERSONS WITH LUNG CANCER: THE ROLE OF FAMILY CONFLICT, INTRAPSYCHIC STRAINS, AND HOSPICE UTILIZATION *
Guided by a stress process conceptual model, this study examines social and psychological determinants of complicated grief symptoms focusing on family conflict, intrapsychic strains, and the potential moderating effect of care quality and hospice utilization. Relying on data from 152 spouse and adult child lung cancer caregiver survey respondents, drawn from an ancillary study of the Assessment of Cancer CarE and SatiSfaction (ACCESS) in Wisconsin, hierarchical multiple regression analysis was used to examine determinants of complicated grief. After controlling for contextual factors and time since death, complicated grief symptoms were higher among care-givers with less education, among families with lower prior conflict but higher conflict at the end-of-life, who had family members who had difficulty accepting the illness, and who were caring for patients with greater fear of death. Additionally, hospice utilization moderated the effect of fear of death on complicated grief. Findings suggest that family conflict, intrapsychic strains, and hospice utilization may help to explain the variability found in complicated grief symptoms among bereaved caregivers. Implications for enhancing complicated grief assessment tools and preventative interventions across the continuum of cancer care are highlighted.
INTRODUCTION
Every year approximately 160,000 persons, primarily elders, die from lung cancer; the leading cause of cancer mortality in the United States ( American Cancer Society, 2009 ). Given the rapid deteriorating course of illness, prevalence of uncontrolled symptoms ( Griffin, Koch, Nelson, & Cooley, 2007 ), and stigma associated with lung cancer that is reported by smokers and non-smokers ( Chapple, Ziebland, & McPherson, 2004 ), it is not surprising that these families report high levels of distress during the dying process ( Cameron, Franche, Cheung, & Stewart, 2002 ). Compared to the general population, significant others of lung cancer patients score lower on psychological well-being and experience a decline in family functioning over the course of illness that continues after the death ( Persson, Östlund, Wennman-Larsen, Wengström, & Gustavsson, 2008 ). Little research has been done on how family conflict and experiences during treatment and at the end-of-life may influence the bereavement outcomes of family caregivers.
There is tremendous variability in how individuals adapt following the death of a family member; the vast majority of whom are adequately supported, and do not benefit from intervention ( Jordan & Neimeyer, 2003 ). Given the high risk of suicide and other adverse mental and physical health outcomes ( Latham & Prigerson, 2004 ), understanding what contributes to complicated grief symptomatology is necessary to identify caregivers at risk and to develop relevant interventions. Preliminary evidence suggests that family conflict and intrapsychic strains at the end-of-life that are commonly experienced by family members of persons with lung cancer ( Cameron et al., 2002 ), may contribute to bereavement adjustment difficulties ( Kramer, Boelk, & Auer, 2006 ). The purpose of this article was to examine the predictors of complicated grief symptoms focusing on the role of family conflict, intrapsychic strains, and the potential moderating effect of care quality and hospice utilization.
Theoretical Framework
Stress process theories have been influential in informing an extensive body of research on the impact of family caregiving ( Kramer & Vitaliano, 1994 ), and more recently caregiving and bereavement outcomes ( Burton, Haley, Small, Finley, Dillinger-Vasille, & Schonwetter, 2008 ). Aside from background and contextual considerations, the stress process model is made up of four primary domains: primary stressors, secondary stressors, moderators, and outcomes ( Aneshensel, Pearlin, Mullan, Zarit, & Whitlatch, 1995 ; Pearlin, Mullan, Semple, & Skaff, 1990 ).
Primary Stressors
Patient clinical care needs.
Primary stressors are the difficult circumstances experienced by family care-givers that stem from the patient’s care needs ( Pearlin et al., 1990 ). Lung cancer patients have a high prevalence of uncontrolled distressing physical symptoms; the most common are pain, shortness of breath, nausea or vomiting, and fatigue ( Griffin et al., 2007 ). These symptoms exacerbate co-occurring psychological symptoms such as patient depression and anxiety ( Portenoy, Payne, & Jacobson, 1999 ), and family distress ( McMillan & Small, 2007 ). Caregivers experience better bereavement adjustment when these symptoms are minimized and quality of life is enhanced ( Grande, Farquhar, & Barclay, 2004 ).
Secondary Stressors
Role strains.
“Secondary stressors are the ways that primary stressors influence and disrupt other areas of the caregiver’s life” ( Gustavsson & Dal Santo, 2008 , p. 292), and are associated with worse bereavement outcomes ( Bernard & Guarnaccia, 2003 ). Pearlin and colleagues (1990) identified family conflict as a central role strain that may arise in caregiving families. Based on prior research, suggesting that greater bereavement distress among family caregivers is more likely among families in conflict ( Kramer et al., 2006 ) and who have poorer family functioning ( Kissane, Bloch, Miach, Smith, Seddon, & Keks, 1997 ), it is hypothesized that family conflict will be associated with more complicated grief symptoms.
Intrapsychic Strains
Intrapsychic strains are psychological difficulties occurring within the mind that may be influenced by primary stressors. Two intrapsychic strains that may be particularly relevant to lung cancer caregivers are difficulty accepting a highly stigmatized illness and the patient’s fear of death, that is distressing to witness ( Chapple et al., 2004 ). “Acceptance” has been put forth as an essential component of illness adjustment, and a growing body of literature has focused on the correlates of acceptance among patients with acute and chronic heath conditions ( Stuifbergen, Becker, Blozis, & Beal, 2008 ). Grieving may be hampered when one has difficulty accepting illness and related feelings of loss ( Prigerson & Maciejewski, 2008 ). Although an extensive body of literature has developed investigating the predictors and outcomes of death anxiety among elders, the seriously ill and professional caregivers ( Neimeyer, Wittkowski, & Moser, 2004 ), and personal fear of death as associated with bereavement outcomes ( Barr & Cacciatore, 2008 ), little attention has been given to how the patient’s fear of death might influence caregiver bereavement. Compared to other cancer patient populations, lung cancer patients exhibit the highest rates of psychological disorders, and existential distress is elevated closer to death ( Lichtenthal, Nilsson, Zhang, Trice, Kissane, & Prigerson, 2009 ). Emotional distress is higher among caregivers of patients perceived to have death anxiety ( Morita, Akechi, Ikenaga, Inoue, Kohara, Matsubara, et al., 2007 ). It is hypothesized that difficulty accepting the illness and patient fear of death will contribute to complicated grief symptoms.
Resources are the various supports that one has to manage stressful experiences and these are thought to moderate the relationship between stressors and outcomes ( Pearlin et al., 1990 ). Two resources that are particularly salient to the needs of lung cancer patients and families are quality of care and hospice utilization. Better care quality is associated with higher quality of life for care-givers of patients with lung cancer ( Ryan, Howell, Jones, & Hardy, 2008 ), and bereaved family members who are adequately supported by health care providers experience lower levels of psychiatric illness following hospital deaths ( Siegel, Hayes, Vanderwerker, Loseth, & Prigerson, 2008 ). Support received prior to death, is a stronger predictor of bereavement adjustment than support received after death ( Bass, Bowman, & Noelker, 1991 ), and perceptions of care quality are associated with better bereavement adjustment ( Grande et al., 2004 ).
Hospice programs are specifically designed to provide end-of-life care in order to manage distressing symptoms, maximize patient quality of life, address needs of family, and is touted as the optimal care model for patients with advanced lung cancer ( Griffin, Koch, Ackerman, & Cole, 2003 ). Family members report greater satisfaction with hospice care than care in other settings ( Yancey, Greger, & Coburn, 1990 ), and improved family well-being and functioning ( Godkin, Krant, & Doster, 1983–84 ). Findings regarding hospice utilization and the influence on bereavement outcomes are mixed. Some find utilization to have a positive influence ( Christakis & Iwashyna, 2003 ; Steele, 1990 ), but others report no relationship ( Grande et al., 2004 ; Yancey, Greger, & Coburn, 1990 ). According to the stress process model, we hypothesize that the impact of family conflict and intrapsychic strains on complicated grief symptoms will be intensified when resources are low and diminished when they are plentiful.
Complicated Grief Symptoms
Salient outcomes of the stress process include psychological well-being indicators related to the individual’s “ability to sustain themselves in their social roles” ( Pearlin et al., 1990 , p. 590). Complicated grief symptoms interfere with daily life and the fulfillment of social roles ( Prigerson, Maciejewski, Reynolds, Bierhals, Newsom, Fasiczka, et al., 1995 ) and are experienced by 10%–20% of the population ( Prigerson & Jacobs, 2001 ). Although there are important features that distinguish it from bereavement related depression, anxiety and Posttraumatic Stress Disorder (PTSD) ( Zhang, El-Jawahri, & Prigerson, 2006 ), one study reports as many as 75% of complicated grievers also meet criteria for PTSD ( Tolstikova, Fleming, & Chartier, 2005 ). Given that complicated grievers experience intense, persistent emotional and functional impairment ( Schulz, Boerner, Shear, Zhang, & Gitlin, 2006 ), identifying the contributing factors of this particular outcome is an important goal of bereavement research.
Background and Context—Control Variables
Pearlin et al. (1990 , p. 585) propose that the effects of “ascribed statuses, such as age, gender, ethnicity, along with education … and economic attainments are expected to be threaded throughout the entire stress process,” influence the primary and secondary stressors individuals are exposed to, the resources they have access to, and the outcomes they experience. As such it is important to control for these and other contextual variables. Other contextual variables include advanced care planning and the family context. Among several forms of advanced care planning, discussions with significant others is considered an essential component of the planning process ( Hammes & Rooney, 1998 ). Stress and post-death depression and anxiety among relatives may be reduced when caregivers know the patient’s preferences for care ( Detering, Hancock, Reade, & Silvester, 2010 ; Tilden, Tolle, Garland, & Nelson, 1995 ), and evidence suggests that “end-of-life discussions may have cascading benefits for patients and their caregivers” including better caregiver bereavement adjustment ( Wright, Zhang, Ray, Mack, Trice, Balboni, et al., 2008 , p. 1670). Although little attention has been given to the family context, higher bereavement risk was found among those with young children at home ( Beckwith, Beckwith, Gray, & Micsko, 1990 ) and a history of conflict was associated with guilt following death which negatively effects bereavement adaptation ( Mullan, 1992 ). In addition, a history of conflict is a strong predictor of family conflict at the end-of-life ( Kramer, Kavanaugh, Trentham-Dietz, Walsh, & Yonker, 2009 ), and needs to be taken into consideration in order to understand how conflict at the end-of-life might influence bereavement outcomes.
This study will identify and control for the background and contextual variables that are significantly associated with complicated grief among care-givers of deceased lung cancer patients and will test the following two hypotheses, which were generated from this review of the literature and the stress process model.
Hypothesis 1: After controlling for time since death, history of family conflict, and other contextual and primary stressor variables, secondary stressors (i.e., family conflict at the end-of-life, family member difficulty accepting the illness, and patient fear of death) will significantly predict complicated grief symptoms.
Hypothesis 2: The quality of care provided to the patient and family and hospice utilization will moderate the effect of secondary stressors on complicated grief symptoms.
Data Sources
The results reported here are drawn from an ancillary mail survey of family members of persons who died with lung cancer, drawn from the larger Assessment of Cancer CarE and SatiSfaction (ACCESS) study conducted in the state of Wisconsin. Sampling and survey methods from the ACCESS and ancillary lung cancer family study are described in detail by Trentham-Dietz and Walsh (2008) and Kramer et al. (2009) respectively. In brief, of 358 primary family caregivers of persons who died with lung cancer, who were identified in a statewide registry and who had contact information available, 205 (57%) agreed to receive the study questionnaire, and 169 returned surveys (82% of those who received the survey; 47% of total contacted). Consistent with the national profile of informal end-of-life caregivers ( Wolff, Dy, Frick, & Kasper, 2007 ), the majority, 158 (93%) of respondents were spouses ( n = 141) or adult children ( n = 17). The analytic sample is confined to 152 of these respondents. These included 148 who answered all of the complicated grief items, and four others who had completed 50% or more of the items. In these cases we used the person mean substitution method ( Downey & King, 1998 ) found to be the optimal technique for imputing missing data when half of the items are present ( Hawthorne & Elliott, 2005 ). The time between death and completion of the surveys averaged 19 months ( SD = 7.37), and ranged from .9 to 34 months.
Dependent Variable—Complicated Grief
In 1995 the Inventory of Complicated Grief (ICG) was introduced as the first measure to assess maladaptive grief symptoms ( Prigerson et al., 1995 ), and has since been well validated and widely used. The ICG-revised (ICG-R) is a more contemporary version of the ICG further refined by an expert consensus panel ( Prigerson & Jacobs, 2001 ). Using 5-point scales to represent increasing levels of symptom severity respondents rated nine items addressing symptoms of yearning and longing for the deceased, trouble accepting the death, difficulty trusting people, bitterness over the death, inability to move forward, sense of emotional numbness, sense of edginess, feeling the future holds no prospect for fulfillment without the deceased, and feeling life is empty or meaningless without the deceased. This measure is highly correlated with clinical assessments of complicated grief and distinguishes complicated grief from typical grief symptoms in terms of the prediction of morbidity, persistence and intensity ( Prigerson & Maciejewski, 2005 ). A mean score was calculated. Cronbach’s α was .90.
Two measures of patient clinical care needs were assessed. An index of four common physical symptoms associated with lung cancer was assessed by asking: “Did your family member have: a) pain; b) shortness of breath; c) nausea or vomiting; or d) severe tiredness or fatigue during or after any of his/her cancer treatments?” Cronbach’s α was .66. A psychological symptoms index was assessed by asking “Did your family member experience: a) loss of hope or depression; and/or b) anxiety during or after any of his/her cancer treatments?” Cronbach’s α was .57.
Role strains
A four-item Family Conflict at the End-of Life (FC-EOL) scale was developed for this study, based on findings from a prior qualitative study ( Kramer et al., 2006 ), a literature review, and prior clinical experience. Respondents were asked to use a 5-point scale (1 = not at all to 5 = very much ) to answer the following four questions “As you think about the decisions that your family member and the rest of the family faced near the end-of-life, how much did any family members: Disagree or argue with one another? Feel resentment toward one another? Feel anger toward one another? Insult or yell at one another?” A mean score for family conflict was calculated. Cronbach’s α was .93; item to total correlations ranged from .72 to .88.
Intrapsychic strains
Family difficulty accepting the illness was assessed with response to the question “How much did any family members find it hard to accept the illness?” using a 5-point continuum to indicate the degree of difficulty (0 = not at all to 4 = very much ). Patient fear of death was measured by a 5-point rating of agreement (1 = strongly disagree to 5 = strongly agree ) with the statement “After his/her lung cancer diagnosis, my family member was afraid to die.”
Moderators—Resources
Patient quality of care was assessed using nine items adapted from the Toolkit of Instruments to Measure End-of-Life Care ( Teno, 2004 ). Using a 5-point response set (0 = not at all to 5 = very much ), respondents rated the extent to which the patient was treated with kindness and respect, and their physical, psychological, spiritual, and informational needs were adequately addressed. Cronbach’s α was .86. Family quality of care was assessed using five items adapted from the Toolkit of Instruments ( Teno, 2004 ) to rate family care quality. Respondents rated the extent to which the treatment staff included them in decisions, were sensitive to their feelings, paid attention to their wishes for care, and were helpful in explaining the patient’s condition, using a 5-point response set (0 = not at all to 5 = very much ). Cronbach’s α was .84. Hospice utilization was determined with the question “Was hospice involved in the medical care of your family member?” (0 = no ; 1 = yes ).
Background and Context
Demographic characteristics included sociodemographic variables such as age (continuous variable that was dichotomized; 0 = under 65 ; 1 = 65 or over ), gender (0 = male ; 1 = female ), race (0 = Non-Hispanic White ; 1 = Black ), income (four income categories included less than $12,000, $15,000–$29,999, $30,000–$49,999, and $50,000+), education (two categories that include high school or less, and some college or advanced degree), and relationship to the deceased (0 = adult child ; 1 = spouse ). Care preferences specified was ascertained by asking: “Did your family member have specific wishes or plans about the types of medical treatment he/she wanted while dying?” (0 = no ; 1 = yes ). Regarding family context we assessed presence of children in the home (0 = no ; 1 = yes ), and history of family conflict prior to illness with a two-item scale developed by (first author). Respondents were asked “Prior to [the decedent’s] illness, how much did any of your family members: ‘have serious arguments with one another;’ and ‘insult or yell at one another’?” Response categories range from 0 = not at all to 4 = very much . Cronbach’s α was .94.
Analytic Plan
Details regarding strategies for handling missing data are reported elsewhere ( Kramer et al., 2009 ). The analysis has four parts. First, we present descriptive statistics and the results of the two-tailed t tests and F tests comparing the means for complicated grief on all values of the categorical background and contextual variables. Second, we present the descriptives and bivariate correlations for the analytic variables. Third, to test the first hypothesis, we used hierarchical multiple regression analysis to control for the time since death, contextual variables, and primary stressors. Given the limited sample size and the number of independent variables, aside from time since death and history of family conflict, only the variables that were significantly correlated with complicated grief were chosen for the analysis to allow for a more parsimonious model. Omitted contextual variables were analyzed as covariates in an exploratory regression analysis, and their presence did not alter appreciably the relationships between conflict, intra-psychic strains, and complicated grief. Finally, to test hypothesis 2 and examine the extent to which resources would have a stress-buffering effect, interaction terms were computed by multiplying each of the selected secondary stressor variables by each of the three resources variables, which were centered before forming the multiplicative terms ( Jaccard, Turrisi, & Wan, 1990 ). Separate regression analyses were run to identify any significant interactions. One interaction was a significant predictor of complicated grief and as such, the regression analysis included two additional blocks to control for resources and this interaction effect respectively. The more conservative two-tailed tests of significance were used to interpret the results.
Sample Characteristics and Contextual Correlates of Complicated Grief
Sample characteristics and complicated grief means for categorical background and contextual variables are described in Table 1 . The majority of respondents were female, Non-Hispanic White, spouses, with less than a college education. The sample fell fairly evenly into the under and over age 65 groupings. Consistent with the national profile of end-of-life caregivers, the mean age was 63 for family members ( Wolff et al., 2007 ), and 66 for patients ( SD = 8.82; range = 43–80), indicating that this was primarily an older sample. Less than half of the patients had expressed specific wishes for end-of-life care (39%) and the majority (68%) received hospice care. Complicated grief symptom were greater among those who were younger ( t = 2.96, p ≤ .01), less educated ( t = 2.65, p ≤ .01), and who had more children in the home ( t = 2.49, p ≤ .01). No differences were found by gender, race, income, or relationship, and grief was higher among those caring for patients who had expressed their end-of-life care wishes ( t = 2.52, p ≤ .01).
Sample Characteristics and Complicated Grief Means for Categorical Background and Contextual Variables ( N = 152)
Table 2 presents the means and standard deviations of the analytic variables as well as the zero-order correlations among them. Complicated grief symptoms were not correlated with a history of family conflict, but were significantly correlated with physical ( r = .28, p ≤ .001) and psychological symptoms of the patient ( r = .34, p ≤ .001).
Correlations and Descriptives for Analytic Variables
Secondary Stressors and Complicated Grief: Hypothesis 1
We hypothesized that after controlling for time since death and a history of family conflict, and other contextual and primary stressor variables, that higher levels of family conflict at the end-of-life, family member difficulty accepting the illness and patient fear of death will contribute to complicated grief symptoms. Table 3 presents the results of the regression analysis for complicated grief. As shown in Step 3, after controlling for contextual and primary stressor variables, secondary stressors uniquely explained 15% of the variance in complicated grief symptoms, the most important two being family conflict at the end-of-life ( b = .23, p ≤ .01) and family member difficulty accepting the illness ( b = .34, p ≤ .001).
Hierarchical Regression Analysis for Complicated Grief Symptoms
Moderators of Complicated Grief Symptoms: Hypothesis 2
We hypothesized that the quality of care provided to the patient and family and hospice utilization will buffer the effect of secondary stressors on complicated grief symptoms. As shown in the final model of Table 3 , net of the other predictors, an interaction between patient fear of death and hospice care utilization significantly predicted complicated grief symptoms ( b = −.16, p ≤ .05). We graphed this interaction using the estimated regression coefficients for combinations of low and high patient fear of death by hospice or no hospice status; all other variables evaluated at their mean (see Figure 1 for a portrayal of this interaction effect). Patient fear of death contributed to complicated grief when hospice care was not utilized, and supports the stress-buffering hypothesis.

Interaction between patient fear of death and hospice utilization on complicated grief.
The regression equation explained 45% of the total variance in complicated grief symptoms. In the final model, in addition to the moderator variable (i.e., patient fear of death by hospice utilization), significantly more complicated grief symptoms were found among caregivers without college education ( b = −.25, p ≤ .001), who were caring for patients who had specified their end-of-life care preferences ( b = .13, p ≤ .10), and had more psychological symptoms ( b = .15, p ≤ .10), and fear of dying ( b = .15, p ≤ .05), who had family members who expressed difficulty accepting the illness ( b = .33, p ≤ .001), and who had low levels of a history of family conflict ( b = −.21, p ≤ .05), but higher levels of family conflict near the end-of-life ( b = .21, p ≤ .05).
The most compelling findings from this study concern the importance of secondary stressors in predicting complicated grief symptoms and hospice utilization in moderating the stress process. Secondary stressors uniquely explained 15% of the variance in complicated grief symptoms and both family conflict and the two intrapsychic strains were significant predictors in the final model. Kissane and colleagues ( Kissane & Bloch, 2002 ; Kissane, McKenzie, Bloch, Moskowitz, McKenzie, & O’Neill, 2006 ) have well documented a relationship between “maladaptive family functioning” and bereavement outcomes. Our results suggest that although not very common, as evidenced by the strong correlation between prior conflict and conflict at the end-of-life (i.e., r = .62), that families with lower levels of prior conflict and higher levels of conflict associated with difficult decisions at the end-of-life may be at most risk. Developing and testing interventions to meet the needs of families at risk of experiencing conflict at the end-of-life (see Kramer et al., 2009 ), to better support families during critical junctures in decision making, and in the aftermath of unresolved conflict across the continuum of cancer care are important goals for future research and practice.
National guidelines and preferred practices for palliative care have been established that emphasize the importance of assessing and treating social and psychological responses to illness that often contribute to patient and family suffering ( Hultman, Keene-Reder, & Dahlin, 2008 ). Our findings suggest that assessment and treatment of these needs should not be relegated to palliative care only. Family difficulty accepting illness and patient fear of death both represent psychological needs that may be present very soon after the diagnosis. Oncologists, family physicians, and other interdisciplinary care providers need to be mindful of the intrapsychic strains that are stimulated by a lung cancer diagnosis and consider psychotherapeutic interventions or referrals to address them. Cognitive-existential group therapy has been used successfully to address death anxiety among cancer patients ( Kissane et al., 1997 ). Similar models might be employed to support lung cancer patients and family members to address fear of death and difficulty accepting the illness.
Although no direct bivariate relationship was found between hospice utilization and complicated grief, hospice enrollment did serve to buffer the effect of patient fear of death and the outcome. The decedent’s fear of death, as reported by the survivor, did not lead to complicated grief when hospice was used, but did have a negative effect on bereavement outcomes for those not enrolled. Hospice professionals are found to have less death anxiety and more death related experience than other health care professionals ( Carr & Merriman, 1995–1996 ), and may have greater competency to address fear of death among patients. Of course this is an empirical question, but may help to explain our findings. Hospice care is recommended as the optimal care model of patients with advanced lung cancer ( Griffin et al., 2003 ), but is typically underutilized by lung cancer patients ( McCarthy, Burns, Davis, & Phillips, 2003 ). In addition to encouraging efforts to promote timely hospice referral, other initiatives to increase the knowledge, skills, and competencies of oncology and other health care providers for routinely assessing and treating patient fear of death may help prevent complicated bereavement.
Not surprisingly, bivariate analysis revealed strong relationships between lower quality of care of the patient and family and higher levels of complicated grief. Given the documented concerns regarding care quality for lung cancer patients and their families ( Krishnasamy, Wilkie, & Haviland, 2001 ), this is an important finding. The relationship between care quality and complicated grief was no longer statistically significant in the multivariate model when contextual and stressor variables were included and care quality did not moderate the effect of stressors on grief. Routine care may not sufficiently address the distinctive problems associated with secondary stressors. More intensive and targeted interventions to meet these distinctive needs may be required.
Several limitations should be acknowledged when interpreting these results. The cross-sectional design and reliance on retrospective reports of care and conflict at the end-of-life are important limitations. Current affective states may affect retrospective appraisals of subjective measures. Prospective studies are necessary to evaluate the direction of relationships and capture experiences as they occur, but do present ethical and methodological challenges of their own ( Casarett, Knebel, & Helmers, 2003 ). Although the interaction between hospice utilization and patient fear of death was interpretable, it may be a chance finding given the multiple exploratory tests used to evaluate possible interaction effects. Other studies are needed to test for replication. Some of our measures are limited such as the clinical care needs of the patient that measured the number of symptoms rather than symptom severity that may underestimate the relationship between symptoms and complicated grief. In addition, we did not collect any data on the end-of-life care treatment preferences that were expressed by patients or the extent to which they were carried out, which seriously limits our ability to interpret the unanticipated positive correlation between care preferences specified and grief symptoms. It is possible that the patient’s expressed end-of-life care wishes were not fulfilled or were a source of disagreement among family members. Finally, the nonrepresentative nature of the sample, seriously limits the generalizability of study findings. Although the ACCESS study sought a representative statewide sample and over sampled by race, less than half of the eligible families completed the survey, and the sample was primarily older White female spouses. In addition, the hospice utilization rate of our sample is higher than that of lung cancer Medicare beneficiaries (i.e., 68% compared to 27%; McCarthy et al., 2003 ), and the overall rates of family conflict were fairly modest ( Kramer et al., 2009 ).
Despite its limitations, this study was guided by a theoretical framework to provide a more comprehensive understanding of the complex determinants of the bereavement process with attention to domains not previously considered in research. Results provide substantial support documenting a relationship between family conflict, intrapsychic strains and complicated grief, and a moderating influence of hospice utilization. Risk assessment tools used to identify persons at risk for complicated grief ( Melliar-Smith, 2002 ) could be expanded to include assessment of patient symptoms, intrapsychic strains, prior and end-of-life conflict, and caregiver education. Future research to replicate these findings and to illuminate understanding of the primary and secondary stressors and resources that might influence the bereavement process, as well as development and testing of interventions to address these are warranted.
Acknowledgments
The authors gratefully acknowledge the bereaved family members for their participation in this study, and the contributions of our colleagues who participated in the design of the ACCESS survey.
* This study used data from the Assessment of Cancer CarE and SatiSfaction (ACCESS), survey, a project of the Wisconsin Comprehensive Cancer Control Program. Funding for the WI CCC Program is provided by The Centers for Disease Control & Prevention, The Wisconsin Department of Health Services, The Wisconsin Partnership Fund for a Healthy Future, and the UW-Paul P. Carbone Comprehensive Cancer Center. This article was prepared with support from the National Institute of Mental Health (T32 MH65185 to Jan. Greenberg).
- American Cancer Society. Cancer facts and figures 2009. Atlanta, GA: American Cancer Society; 2009. [ Google Scholar ]
- Aneshensel CS, Pearlin LI, Mullan JT, Zarit SH, Whitlatch CJ. Profiles in caregiving: The unexpected career. New York: Academic Press; 1995. [ Google Scholar ]
- Barr P, Cacciatore JE. Personal fear of death and grief in bereaved mothers. Death Studies. 2008; 32 :445–460. [ PubMed ] [ Google Scholar ]
- Bass DM, Bowman K, Noelker LS. The influence of caregiving and bereavement support on adjusting to an older relative’s death. The Gerontologist. 1991; 31 :32–42. [ PubMed ] [ Google Scholar ]
- Beckwith BE, Beckwith SK, Gray TL, Micsko MM. Identification of spouses at high risk during bereavement: A preliminary assessment of Parkes and Weiss’ Risk Index. Hospice Journal. 1990; 6 (3):35–46. [ PubMed ] [ Google Scholar ]
- Bernard LL, Guarnaccia CA. Two models of caregiver strain and bereavement adjustment: A comparison of husband and daughter caregivers of breast cancer hospice patients. The Gerontologist. 2003; 43 :808–816. [ PubMed ] [ Google Scholar ]
- Burton AM, Haley WE, Small BJ, Finley MR, Dillinger-Vasille M, Schonwetter R. Predictors of well-being in bereaved former hospice care-givers: The role of caregiving stressors, appraisals, and social resources. Palliative and Supportive Care. 2008; 6 :149–158. [ PubMed ] [ Google Scholar ]
- Cameron J, Franche R, Cheung AM, Stewart DE. Lifestyle interference and emotional distress in family caregivers of advanced cancer patients. Cancer. 2002; 94 :521–527. [ PubMed ] [ Google Scholar ]
- Carr M, Merriman MP. Comparison of death attitudes among hospice workers and health care professionals in other settings. Omega. 1995–1996; 32 :287–301. [ Google Scholar ]
- Casarett D, Knebel A, Helmers K. Ethical challenges of palliative care research. Journal of Pain and Symptom Management. 2003; 25 (4):S3–S5. [ PubMed ] [ Google Scholar ]
- Chapple A, Ziebland S, McPherson A. Stigma, shame, and blame experienced by patients with lung cancer: Qualitative study. British Medical Journal. 2004; 328 (7454):1470–1474. [ PMC free article ] [ PubMed ] [ Google Scholar ]
- Christakis NA, Iwashyna TJ. The health impact of health care on families: A matched cohort study of hospice use by decedents and mortality outcomes in surviving, widowed spouses. Social Science & Medicine. 2003; 57 :465–475. [ PubMed ] [ Google Scholar ]
- Detering KM, Hancock AD, Reade MC, Silvester W. The impact of advance care planning on end of life care in elderly patients: A randomised controlled trial. British Medical Journal. 2010; 340 doi: 10.1136/bmj.c1345. [ PMC free article ] [ PubMed ] [ CrossRef ] [ Google Scholar ]
- Downey RG, King C. Missing data in Likert ratings: A comparison of replacement methods. Journal of General Psychology. 1998; 125 :175–191. [ PubMed ] [ Google Scholar ]
- Godkin MA, Krant MJ, Doster NJ. The impact of hospice care on families. International Journal of Psychiatry in Medicine. 1983–84; 13 (2):153–165. [ PubMed ] [ Google Scholar ]
- Grande GE, Farquhar MC, Barclay SIG. Caregiver bereavement outcome: Relationship with hospice at home, satisfaction with care, and home death. Journal of Palliative Care. 2004; 20 (2):69–77. [ PubMed ] [ Google Scholar ]
- Griffin JP, Koch KA, Ackerman TF, Cole FH., Jr End-of-life care in patients with lung cancer. CHEST: Supplement. 2003; 123 :312S–331S. [ PubMed ] [ Google Scholar ]
- Griffin JP, Koch KA, Nelson JE, Cooley ME. Palliative care consultation, quality-of-life measurements, and bereavement for end-of-life care in patients with lung cancer. CHEST: Supplement. 2007; 132 :404S–422S. [ PubMed ] [ Google Scholar ]
- Gustavson K, Dal Santo TS. Caregiver service use: A complex story of care at the end of life. Journal of Social Work in End-of-Life & Palliative Care. 2008; 4 :286–311. [ Google Scholar ]
- Hammes BJ, Rooney BL. Death and end-of-life planning in one midwestern community. Archives of Internal Medicine. 1998; 158 :383–390. [ PubMed ] [ Google Scholar ]
- Hawthorne G, Elliott P. Imputing cross-sectional missing data: Comparison of common techniques. Australian and New Zealand Journal of Psychiatry. 2005; 39 :583–590. [ PubMed ] [ Google Scholar ]
- Hultman T, Keene Reder EA, Dahlin CM. Improving psychological and psychiatric aspects of palliative care: The national consensus project and the national quality forum preferred practices for palliative and hospice care. Omega. 2008; 57 :323–339. [ PubMed ] [ Google Scholar ]
- Jaccard J, Turrisi R, Wan CK. Interaction effects in multiple regression. Newbury Park, CA: Sage; 1990. [ PubMed ] [ Google Scholar ]
- Jordan JR, Neimeyer RA. Does grief counseling work? Death Studies. 2003; 27 :765–786. [ PubMed ] [ Google Scholar ]
- Kissane DW, Bloch S. Family focused grief therapy: A model of family-centered care during palliative care and bereavement. Philadelphia, PA: Open University Press; 2002. [ Google Scholar ]
- Kissane DW, Bloch S, Miach P, Smith GC, Seddon A, Keks N. Cognitive-existential group therapy for patients with primary breast cancer—Techniques and themes. Psycho-Oncology. 1997; 6 :25–33. [ PubMed ] [ Google Scholar ]
- Kissane DW, McKenzie M, Bloch S, Moskowitz C, McKenzie DP, O’Neill J. Family focused grief therapy: A randomized, controlled trial in palliative care and bereavement. American Journal of Psychiatry. 2006; 163 (7):1208–1218. [ PubMed ] [ Google Scholar ]
- Kramer BJ, Boelk A, Auer C. Family conflict at the end-of-life: Lessons learned in a model program for vulnerable older adults. Journal of Palliative Medicine. 2006; 9 :791–801. [ PubMed ] [ Google Scholar ]
- Kramer BJ, Kavanaugh M, Trentham-Dietz A, Walsh M, Yonker JA. Predictors of family conflict at the end of life: The experience of spouses and adult children of persons with lung cancer. The Gerontologist. 2009 doi: 10.1093/geront/gnp 121. [ PMC free article ] [ PubMed ] [ CrossRef ] [ Google Scholar ]
- Kramer BJ, Vitaliano PP. Coping: A review of the theoretical frameworks and the measures used among caregivers of individuals with dementia. Journal of Gerontological Social Work. 1994; 23 (1/2):151–174. [ Google Scholar ]
- Krishnasamy M, Wilkie E, Haviland J. Lung cancer health care needs assessment: Patients’ and informal carers’ responses to a national mail questionnaire survey. Palliative Medicine. 2001; 15 :213–227. [ PubMed ] [ Google Scholar ]
- Latham AE, Prigerson HG. Suicidality and bereavement: Complicated grief as psychiatric disorder presenting greatest risk for suicidality. Suicide and Life-Threatening Behavior. 2004; 34 :350–362. [ PMC free article ] [ PubMed ] [ Google Scholar ]
- Lichtenthal WG, Nilsson M, Zhang B, Trice ED, Kissane DW, Prigerson HG. Do rates of mental disorders and existential distress among advanced stage cancer patients increase as death approaches. Psycho-Oncology. 2009; 18 :50–61. [ PMC free article ] [ PubMed ] [ Google Scholar ]
- McCarthy EP, Burns RB, Davis RB, Phillips RS. Barriers to hospice care among older patients dying with lung and colorectal cancer. Journal of Clinical Oncology. 2003; 21 :728–735. [ PubMed ] [ Google Scholar ]
- McMillan SC, Small BJ. Using the COPE intervention for family caregivers to improve symptoms of hospice homecare patients: A clinical trial. Oncology Nursing Forum. 2007; 34 :313–321. [ PubMed ] [ Google Scholar ]
- Melliar-Smith C. The risk assessment of bereavement in a palliative care setting. International Journal of Palliative Nursing. 2002; 8 :281–287. [ PubMed ] [ Google Scholar ]
- Morita T, Akechi T, Ikenaga M, Inoue S, Kohara H, Matsubara T, et al. Terminal decline: Recommendations from bereaved families’ experiences. Journal of Pain and Symptom Management. 2007; 34 :579–589. [ PubMed ] [ Google Scholar ]
- Mullan JT. The bereaved caregiver: A prospective study of changes in well-being. The Gerontologist. 1992; 32 :673–683. [ PubMed ] [ Google Scholar ]
- Neimeyer RA, Wittkowski J, Moser RP. Psychological research on death attitudes: An overview and evaluation. Death Studies. 2004; 28 :309–340. [ PubMed ] [ Google Scholar ]
- Pearlin L, Mullan J, Semple M, Skaff M. Caregiving and the stress process: An overview of concepts and their measures. The Gerontologist. 1990; 30 (5):583–594. [ PubMed ] [ Google Scholar ]
- Persson C, Östlund U, Wennman-Larsen A, Wengström Y, Gustavsson P. Health-related quality of life in significant others of patients dying from lung cancer. Palliative Medicine. 2008; 22 :239–247. [ PubMed ] [ Google Scholar ]
- Portenoy R, Payne D, Jacobson P. Breakthrough pain: Characteristics and impacts in patients with cancer pain. Pain. 1999; 81 :129–134. [ PubMed ] [ Google Scholar ]
- Prigerson HG, Jacobs SC. Traumatic grief as a distinct disorder: A rationale, consensus criteria, and a preliminary empirical test. In: Stroebe MS, Hansson RO, Stroebe W, Schut H, editors. Handbook of bereavement research: Consequences, coping and care. Washington, DC: American Psychological Association; 2001. pp. 613–637. [ Google Scholar ]
- Prigerson HG, Maciejewski PK. A call for sound empirical testing and evaluation of criteria for complicated grief proposed for DSM-V. Omega. 2005; 52 :9. [ Google Scholar ]
- Prigerson HG, Maciejewski PK. Grief and acceptance as opposite sides of the same coin: Setting a research agenda to study peaceful acceptance of loss. British Journal of Psychiatry. 2008; 193 :435–437. [ PubMed ] [ Google Scholar ]
- Prigerson HG, Maciejewski PK, Reynolds CF, Bierhals AJ, Newsom JT, Fasiczka A, et al. Inventory of complicated grief: A scale to measure maladaptive symptoms of loss. Psychiatry Research. 1995; 59 :65–79. [ PubMed ] [ Google Scholar ]
- Ryan RP, Howell V, Jones J, Hardy EJ. Lung cancer, caring for the caregivers. A qualitative study of providing pro-active social support targeted to the carers of patients with lung cancer. Palliative Medicine. 2008; 22 :233–238. [ PubMed ] [ Google Scholar ]
- Schulz R, Boerner K, Shear K, Zhang S, Gitlin LN. Predictors of complicated grief among dementia caregivers: A prospective study of bereavement. American Journal of Geriatric Psychiatry. 2006; 14 :650–656. [ PubMed ] [ Google Scholar ]
- Siegel MD, Hayes E, Vanderwerker LC, Loseth DB, Prigerson HG. Psychiatric illness in the next of kin in patients who die in the intensive care unit. Critical Care Medicine. 2008; 36 :1722–1728. [ PubMed ] [ Google Scholar ]
- Steele LL. The death surround: Factors influencing the grief experience of survivors. Oncology Nursing Forum. 1990; 17 :235–241. [ PubMed ] [ Google Scholar ]
- Stuifbergen A, Becker H, Blozis S, Beal C. Conceptualization and development of the acceptance of chronic health conditions scale. Issues in Mental Health Nursing. 2008; 29 :101–114. [ PubMed ] [ Google Scholar ]
- Teno JM. TIME: Toolkit of instruments to measure end of life care. 2004 Retrieved August 1, 2000 from http://www.chcr.brown.edu/pcoc/linkstoinstrumhtm.htm .
- Tilden VP, Tolle SW, Garland MJ, Nelson CA. Decisions about life-sustaining treatments. Archives of Internal Medicine. 1995; 155 :633–638. [ PubMed ] [ Google Scholar ]
- Tolstikova K, Fleming S, Chartier B. Grief, complicated grief, and trauma: The role of the search for meaning, impaired self-reference, and death anxiety. Illness, Crisis & Loss. 2005; 18 :293–313. [ Google Scholar ]
- Trentham-Dietz A, Walsh MC. Summary of results. Madison, WI: University of Wisconsin Paul P. Carbone Comprehensive Cancer Center; 2008. ACCESS: Assessment of Cancer CarE and SatiSfaction. [ Google Scholar ]
- Wolff JL, Dy SM, Frick KD, Kasper JD. End-of-life care: Findings from a national survey of informal caregivers. Annals of Internal Medicine. 2007; 167 :40–46. [ PubMed ] [ Google Scholar ]
- Wright AA, Zhang B, Ray A, Mack JW, Trice E, Balboni T, et al. Associations between end-of-life discussions, patient mental health, medical care near death, and caregiver bereavement adjustment. Journal of the American Medical Association. 2008; 300 :1665–1673. [ PMC free article ] [ PubMed ] [ Google Scholar ]
- Yancey D, Greger HA, Coburn P. Determinants of grief resolution in cancer death. Journal of Palliative Care. 1990; 6 (4):24–31. [ PubMed ] [ Google Scholar ]
- Zhang A, El-Jawahri A, Prigerson HG. Update on bereavement research: Evidence-based guidelines for the diagnosis and treatment of complicated bereavement. Journal of Palliative Medicine. 2006; 9 :1188–1203. [ PubMed ] [ Google Scholar ]
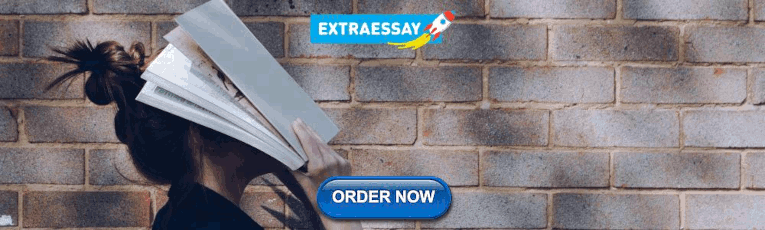
IMAGES
VIDEO
COMMENTS
Lung cancer is one of the most frequently diagnosed cancers and the leading cause of cancer-related deaths worldwide with an estimated 2 million new cases and 1·76 million deaths per year. Substantial improvements in our understanding of disease biology, application of predictive biomarkers, and refinements in treatment have led to remarkable ...
The 100 most cited articles in lung cancer are listed in Table 1, arranged in descending order according to the number of times cited. The number of citations ranged from 7751 to 889, and the mean number of citations per article was 1879.82 ±1264.78 (range: 7751-889). We found that the most cited article (times cited: 7751) on lung cancer ...
Lung cancer remains a devastating diagnosis to receive. The goal of this thesis was to better understand the impact of lung cancer and study how to integrate palliative and supportive care.
Lung Cancer Screening Program Revenue April 2016 verses 2017. A 2012 study found lung cancer screening to be one of the most cost-effective. screening tests available at about $11,000 per life-year saved, compared with $18,000 for. colon cancer screening, $31,000 for breast cancer screening, and $50,000 for cervical.
Lung cancers are classified into SCLC or NSCLC (A), which are subdivided into squamous and non-squamous histology (B). (C) The frequencies of common oncogenic driver mutations in NSCLC. Based on a cohort of 4064 patients with metastatic NSCLC by Singal and colleagues.19 NSCLC=non-small-cell lung cancer. SCLC=small-cell lung cancer.
both limited-stage small cell lung cancer patients and extensive-stage small cell lung cancer patients throughout the study period. Lu et al. (2019) demonstrated that the average incidence of lung cancer was 59.0/100,000 people for the years from 1973 to 2015. Specifically, the incidence rate was at an apex in 1992 and then constantly declined.
Therefore, in this thesis, to improve the efficiency of lung cancer screening and to provide personalized treatment strategies for patients, we focused on the development of deep learning algorithms for automatic pulmonary nodule detection and overall survival prediction of lung cancer. Early detection of lung cancer is a fundamental step for ...
Lung cancer has four common types: lung nodules, non-small cell lung cancer (NSCLC), small cell lung cancer (SCLC), and mesothelioma; the most common of which is non-small cell lung cancer [6]. It is important that research and modeling is done on lung cancer in an attempt to better understand and counteract this disease. 1.1 A549 Cells One ...
University of San Diego
QUESTIONNAIRE FOR ADVANCED LUNG CANCER PATIENTS by ... A Thesis Submitted to the Faculty of Purdue University In Partial Fulfillment of the Requirements for the degree of Master of Science Department of Psychology at IUPUI Indianapolis, Indiana May 2020 . 2 THE PURDUE UNIVERSITY GRADUATE SCHOOL STATEMENT OF COMMITTEE APPROVAL Dr. Catherine ...
This thesis will be defended in public on Wednesday 24 February 2016 at 11:00 hrs by Ali Saber Hosseinabadi born on 11 September 1981 in Rasht, Iran . Supervisors ... Lung cancer is a result of multiple genetic alterations that accumulate during life21. In 1982, a human gene with transforming activity was identified in a lung ...
DeanF.Davies,M.D.,Ph.D. TheAmericanCancerSocietyentered activelyintothewaragainstlungcancer afternationwidepublicityhadbeengiven toclinicalreportsofanassociationbe
Lung cancer is a disease of uncontrolled cell growth in tissues of the lung. This growth may lead to metastasis, which is the invasion of adjacent tissue and infiltration beyond the lungs. The ...
represented over 25% of all cancer fatalities.1. The National Cancer Database (NCDB) is a hospital-based outcomes data resource that is. estimated to capture 70% of newly diagnosed lung cancers in the United States. The. NCDB is jointly sponsored by the American College of Surgeons and the American.
This study demonstrates accurate lung cancer classification and prediction using technologies enabled by machine learning and image processing. To begin, photographs must be collected. Following that, the images are preprocessed using a geometric mean filter. This eventually leads to an increase in image quality.
Radon gas is a primary cause of lung cancer among nonsmokers and the second leading cause among smokers. 32,33 Radon, a radioactive gas prone to indoor accumulation, arises naturally as a decay product of uranium-238. Indoor radon has been a group 1 carcinogen since 1988, responsible for 3%-14% of lung cancer cases. 33 Emitting alpha-ionizing radiation, radon is linked to a wide variety of ...
approximately 2.09 million new cases and 1.76 million lung cancer-related deaths [2]. Lung cancer cases and deaths have increased significantly globally [2]. Approximately 85-88% of lung cancer cases are non-small cell lung carcinoma (NSCLS), and about 12-15% of lung cancer cases are small cell lung cancer (SCLC) [3]. Early lung cancer ...
Lung cancer is the primary cause of cancer death worldwide, with 2.09 million new cases and 1.76 million people dying from lung cancer in 2018 1.Four case-controlled studies from Japan reported in ...
Abstract. Many lung cancer risk prediction models have been published but there has been no systematic review or comprehensive assessment of these models to assess how they could be used in screening. We performed a systematic review of lung cancer prediction models and identified 31 articles that related to 25 distinct models, of which 11 ...
Importance Screening for lung cancer using low-dose computed tomography is associated with reduced lung cancer-specific mortality, but uptake is low in the US; understanding how patients make decisions to engage with lung cancer screening is critical for increasing uptake. Prior research has focused on individual-level psychosocial factors, but environmental factors (eg, historical contexts ...
TRIPLE POINT THESIS STATEMENT You can include your major supporting points in your thesis statement. This is called a TRIPLE POINT THESIS. MAJOR POINTS: improved lung function, decreased risk of heart disease, less risk of lung cancer TRIPLE POINT THESIS STATEMENT: Quitting smoking is good for your health because it will improve lung
Methods 1. Systematic review on sensitivity of chest x-ray 2. Observational study to determine sensitivity and compare stage and survival between those with 'true positive' versus 'false negative' results. 3. Cohort study to determine chest x-ray specificity and lung cancer risk following negative chest x-ray. 4.
people with lung cancer die within one year of being diagnosed. Only 16 percent of patients diagnosed with lung cancer are still alive after five years, compared with over 90 percent of breast and prostate cancer patients. 7 The low survival rate contributes to perceptions that this disease is a "death sentence." This results in a very
Cessation services provided to individuals before their lung cancer screening exam, and the development of a Patient-Reported Outcome Measure for use in lung cancer screening
Patient Clinical Care Needs . Primary stressors are the difficult circumstances experienced by family care-givers that stem from the patient's care needs (Pearlin et al., 1990).Lung cancer patients have a high prevalence of uncontrolled distressing physical symptoms; the most common are pain, shortness of breath, nausea or vomiting, and fatigue (Griffin et al., 2007).
Metastasis is responsible for 90% of all cancer related death[3], but our knowledge on the subject is still lacking. It is not known why some cells are metastatic and invasive while others are not, and wether this property is in fact reversible[4] . The projects to be presented in this thesis concern flow dynamics in confluent mono-
Lung cancer CT screening has been shown to reduce both lung cancer and overall mortality in smokers, while also providing an opportune moment for smoking cessation interventions. All participants had a long and heavy smoking history (≥15 cigarettes per day for at least 25 years or ≥10 cigarettes per day for at least 30 years).