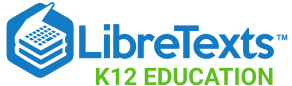
- school Campus Bookshelves
- menu_book Bookshelves
- perm_media Learning Objects
- login Login
- how_to_reg Request Instructor Account
- hub Instructor Commons
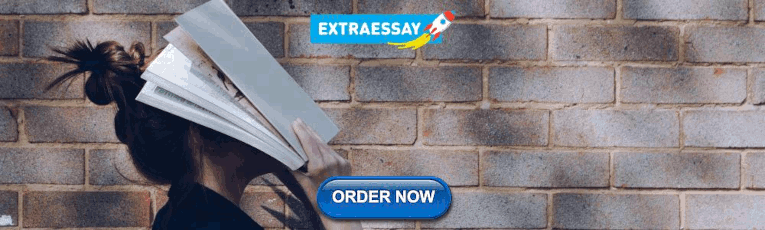
Margin Size
- Download Page (PDF)
- Download Full Book (PDF)
- Periodic Table
- Physics Constants
- Scientific Calculator
- Reference & Cite
- Tools expand_more
- Readability
selected template will load here
This action is not available.
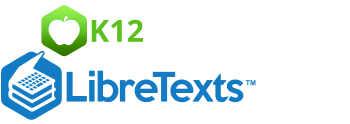
9.7: Dependent and Independent Samples
- Last updated
- Save as PDF
- Page ID 5789
\( \newcommand{\vecs}[1]{\overset { \scriptstyle \rightharpoonup} {\mathbf{#1}} } \)
\( \newcommand{\vecd}[1]{\overset{-\!-\!\rightharpoonup}{\vphantom{a}\smash {#1}}} \)
\( \newcommand{\id}{\mathrm{id}}\) \( \newcommand{\Span}{\mathrm{span}}\)
( \newcommand{\kernel}{\mathrm{null}\,}\) \( \newcommand{\range}{\mathrm{range}\,}\)
\( \newcommand{\RealPart}{\mathrm{Re}}\) \( \newcommand{\ImaginaryPart}{\mathrm{Im}}\)
\( \newcommand{\Argument}{\mathrm{Arg}}\) \( \newcommand{\norm}[1]{\| #1 \|}\)
\( \newcommand{\inner}[2]{\langle #1, #2 \rangle}\)
\( \newcommand{\Span}{\mathrm{span}}\)
\( \newcommand{\id}{\mathrm{id}}\)
\( \newcommand{\kernel}{\mathrm{null}\,}\)
\( \newcommand{\range}{\mathrm{range}\,}\)
\( \newcommand{\RealPart}{\mathrm{Re}}\)
\( \newcommand{\ImaginaryPart}{\mathrm{Im}}\)
\( \newcommand{\Argument}{\mathrm{Arg}}\)
\( \newcommand{\norm}[1]{\| #1 \|}\)
\( \newcommand{\Span}{\mathrm{span}}\) \( \newcommand{\AA}{\unicode[.8,0]{x212B}}\)
\( \newcommand{\vectorA}[1]{\vec{#1}} % arrow\)
\( \newcommand{\vectorAt}[1]{\vec{\text{#1}}} % arrow\)
\( \newcommand{\vectorB}[1]{\overset { \scriptstyle \rightharpoonup} {\mathbf{#1}} } \)
\( \newcommand{\vectorC}[1]{\textbf{#1}} \)
\( \newcommand{\vectorD}[1]{\overrightarrow{#1}} \)
\( \newcommand{\vectorDt}[1]{\overrightarrow{\text{#1}}} \)
\( \newcommand{\vectE}[1]{\overset{-\!-\!\rightharpoonup}{\vphantom{a}\smash{\mathbf {#1}}}} \)
Testing a Hypothesis for Dependent and Independent Samples
Hypothesis testing for dependent and independent samples.
We have learned about hypothesis testing for proportion and means with both large and small samples. However, in the examples in those lessons only one sample was involved. In this lesson we will apply the principals of hypothesis testing to situations involving two samples. There are many situations in everyday life where we would perform statistical analysis involving two samples. For example, suppose that we wanted to test a hypothesis about the effect of two medications on curing an illness. Or we may want to test the difference between the means of males and females on the SAT. In both of these cases, we would analyze both samples and the hypothesis would address the difference between two sample means.
In this Concept, we will identify situations with different types of samples, learn to calculate the test statistic, calculate the estimate for population variance for both samples and calculate the test statistic to test hypotheses about the difference of proportions or means between samples.
Dependent and Independent Samples
When we are working with one sample, we know that we need to select a random sample from the population, measure that sample statistic and then make hypothesis about the population based on that sample. When we work with two independent samples we assume that if the samples are selected at random (or, in the case of medical research, the subjects are randomly assigned to a group), the two samples will vary only by chance and the difference will not be statistically significant. In short, when we have independent samples we assume that the scores of one sample do not affect the other.
Independent samples can occur in two scenarios.
Testing the difference of the means between two fixed populations we test the differences between samples from each population. When both samples are randomly selected, we can make inferences about the populations.
When working with subjects (people, pets, etc.), if we select a random sample and then randomly assign half of the subjects to one group and half to another we can make inferences about the populations.
Dependent samples are a bit different. Two samples of data are dependent when each score in one sample is paired with a specific score in the other sample. In short, these types of samples are related to each other. Dependent samples can occur in two scenarios. In one, a group may be measured twice such as in a pretest-posttest situation (scores on a test before and after the lesson). The other scenario is one in which an observation in one sample is matched with an observation in the second sample.
To distinguish between tests of hypotheses for independent and dependent samples, we use a different symbol for hypotheses with dependent samples. For dependent sample hypotheses, we use the delta symbol δ to symbolize the difference between the two samples. Therefore, in our null hypothesis we state that the difference of scores across the two measurements is equal to 0;δ=0 or:
H 0 :δ=μ 1 −μ 2
Calculating the Pooled Estimate of Population Variance
When testing a hypothesis about two independent samples, we follow a similar process as when testing one random sample. However, when computing the test statistic, we need to calculate the estimated standard error of the difference between sample means,
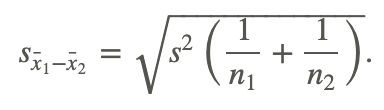
Calculating s2 Suppose we have two independent samples of student reading scores.
The data are as follows:
From this sample, we can calculate a number of descriptive statistics that will help us solve for the pooled estimate of variance:
Using the formula for the pooled estimate of variance, we find that
We will use this information to calculate the test statistic needed to evaluate the hypotheses.
Testing Hypotheses with Independent Samples
When testing hypotheses with two independent samples, we follow similar steps as when testing one random sample:
- State the null and alternative hypotheses.
- Choose α
- Set the criterion (critical values) for rejecting the null hypothesis.
- Compute the test statistic.
- Make a decision: reject or fail to reject the null hypothesis.
- Interpret the decision within the context of the problem.
When stating the null hypothesis, we assume there is no difference between the means of the two independent samples. Therefore, our null hypothesis in this case would be:
H 0 :μ 1 =μ 2 or H 0 :μ 1 −μ 2 =0
Similar to the one-sample test, the critical values that we set to evaluate these hypotheses depend on our alpha level and our decision regarding the null hypothesis is carried out in the same manner. However, since we have two samples, we calculate the test statistic a bit differently and use the formula:
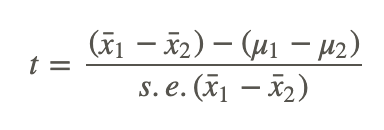
x̄ 1 −x̄ 2 is the difference between the sample means
μ1−μ 2 is the difference between the hypothesized population means
s.e.(x̄ 1 −x̄ 2 ) is the standard error of the difference between sample means
Evaluating the Difference Between Two Samples
The head of the English department is interested in the difference in writing scores between remedial freshman English students who are taught by different teachers. The incoming freshmen needing remedial services are randomly assigned to one of two English teachers and are given a standardized writing test after the first semester. We take a sample of eight students from one class and nine from the other. Is there a difference in achievement on the writing test between the two classes? Use a 0.05 significance level.
First, we would generate our hypotheses based on the two samples.
H 0 :μ 1 =μ 2
H a :μ 1 ≠μ 2
This is a two tailed test. For this example, we have two independent samples from the population and have a total of 17 students that we are examining. Since our sample size is so low, we use the t−distribution. In this example, we have 15 degrees of freedom (number in the samples minus 2) and with a .05 significance level and the t distribution, we find that our critical values are 2.131 standard scores above and below the mean.
To calculate the test statistic, we first need to find the pooled estimate of variance from our sample. The data from the two groups are as follows:
From this sample, we can calculate several descriptive statistics that will help us solve for the pooled estimate of variance:
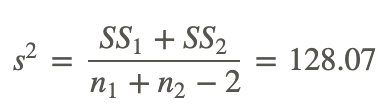
and the standard error of the difference of the sample means is:

Using this information, we can finally solve for the test statistic:

Since -3.53 is less than the critical value of 2.13, we decide to reject the null hypothesis and conclude there is a significant difference in the achievement of the students assigned to different teachers.
Testing Hypotheses about the Difference in Proportions between Two Independent Samples
Suppose we want to test if there is a difference between proportions of two independent samples. As discussed in the previous lesson, proportions are used extensively in polling and surveys, especially by people trying to predict election results. It is possible to test a hypothesis about the proportions of two independent samples by using a similar method as described above. We might perform these hypotheses tests in the following scenarios:
- When examining the proportion of children living in poverty in two different towns.
- When investigating the proportions of freshman and sophomore students who report test anxiety.
- When testing if the proportion of high school boys and girls who smoke cigarettes is equal.
In testing hypotheses about the difference in proportions of two independent samples, we state the hypotheses and set the criterion for rejecting the null hypothesis in similar ways as the other hypotheses tests. In these types of tests we set the proportions of the samples equal to each other in the null hypothesis H 0 :p 1 =p 2 and use the appropriate standard table to determine the critical values (remember, for small samples we generally use the t distribution and for samples over 30 we generally use the z−distribution).
When solving for the test statistic in large samples, we use the formula:
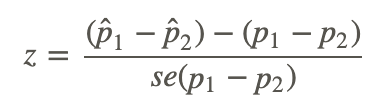
p̂ 1 ,p̂ 2 are the observed sample proportions
p 1 ,p 2 are the population proportions under the null hypothesis
se(p 1 −p 2 ) is the standard error of the difference between independent proportions
Similar to the standard error of the difference between independent samples, we need to do a bit of work to calculate the standard error of the difference between independent proportions. To find the standard error under the null hypothesis we assume that p 1 =p 2 =p and we use all the data to estimate p.
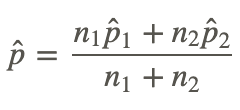
Determining Statistical Difference
Suppose that we are interested in finding out which particular city is more is more satisfied with the services provided by the city government. We take a survey and find the following results:
Is there a statistical difference in the proportions of citizens that are satisfied with the services provided by the city government? Use a 0.05 level of significance.
First, we establish the null and alternative hypotheses:
H 0 :p 1 =p 2
H a :p 1 ≠p 2
Since we have a large sample size we will use the z−distribution. At a .05 level of significance, our critical values are ±1.96. To solve for the test statistic, we must first solve for the standard error of the difference between proportions.
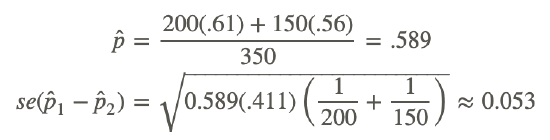
Therefore, the test statistic is:
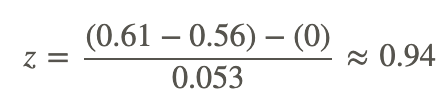
Since 0.94 does not exceed the critical value 1.96, the null hypothesis is not rejected. Therefore, we can conclude that the difference in the probabilities could have occurred by chance and that there is no difference in the level of satisfaction between citizens of the two cities.
Testing Hypotheses with Dependent Samples
When testing a hypothesis about two dependent samples, we follow the same process as when testing one random sample or two independent samples:
- Choose the level of significance
- Make a decision, reject or fail to reject the null hypothesis
- Interpret our results.

s 2 d is the sample variance
d is the difference between corresponding pairs within the sample
n is the number in the sample
sd is the standard deviation
With the standard deviation, we can calculate the standard error using the following formula:
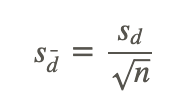
After we calculate the standard error, we can use the general formula for the test statistic:
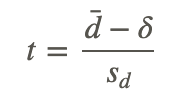
Evaluating the Relationship Between Two Samples
The math teacher wants to determine the effectiveness of her statistics lesson and gives a pre-test and a post-test to 9 students in her class. Our hypothesis is that there is no difference between the means of the two samples and our alternative hypothesis is that the two means of the samples are not equal. In other words, we are testing whether or not these two samples are related or:
H 0 :δ=μ 1 −μ 2 =0
H a :δ=μ1−μ 2 ≠0
The results for the pre-and post-tests are below:
Using the information from the table above, first solve for the standard deviation of the two samples, then the standard error of the two samples and finally the test statistic.
Standard Deviation:
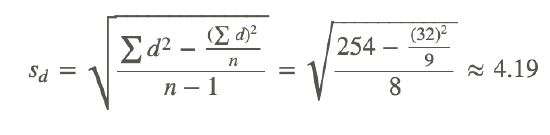
Standard Error of the Difference:
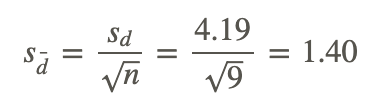
Test Statistic (t−Test)
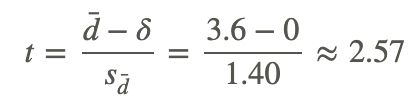
With 8 degrees of freedom (number of observations - 1) and a significance level of .05, we find our critical values to be ±2.306. Since our test statistic exceeds this critical value, we can reject the null hypothesis that the two samples are equal and conclude that the lesson had an effect on student achievement.
You have obtained the number of years of education from one random sample of 38 police officers from City A and the number of years of education from a second random sample of 30 police officers from City B. The average years of education for the sample from City A is 15 years with a standard deviation of 2 years. The average years of education for the sample from City B is 14 years with a standard deviation of 2.5 years. Is there a statistically significant difference between the education levels of police officers in City A and City B?
First, find the test statistic:
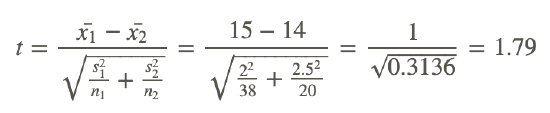
This is a t – statistic with 66 degrees of freedom. This is a two-sided test, with the p-value = 0.07. Since this is greater than .05 we fail to reject the null hypothesis. This means that we believe there is no statistically significant difference between the education levels of police officers in the two different cities.
- In hypothesis testing, we have scenarios that have both dependent and independent samples. Give an example of an experiment with (1) dependent samples and (2) independent samples.
- True or False: When we test the difference between the means of males and females on the SAT, we are using independent samples.
- A study is conducted on the effectiveness of a drug on the hyperactivity of laboratory rats. Two random samples of rats are used for the study and one group is given Drug A and the other group is given Drug B and the number of times that they push a lever is recorded. The following results for this test were calculated:
(a) Does this scenario involve dependent or independent samples? Explain.
(b) What would the hypotheses be for this scenario?
(c) Compute the pooled estimate for population variance.
(d) Calculate the estimated standard error for this scenario.
(e) What is the test statistic and at an alpha level of .05 what conclusions would you make about the null hypothesis?
- A survey is conducted on attitudes towards drinking. A random sample of eight married couples is selected, and the husbands and wives respond to an attitude-toward-drinking scale. The scores are as follows:
(a) What would be the hypotheses for this scenario?
(b) Calculate the estimated standard deviation for this scenario.
(c) Compute the standard error of the difference for these samples.
(d) What is the test statistic and at an alpha level of .05 what conclusions would you make about the null hypothesis?
- In a random sample of 160 couples, the difference between the husband and wife’s ages had a mean of 2.24 years and a standard deviation of 4.1 years. Test the hypothesis that men are significantly older than their wives, on average.
- The weights of marathon runners were taken before and after a run to test if runners lose dangerous levels of fluid.
- Do levels of knowledge about current events differ between freshmen and juniors in college?
- x̄ 1 =35,s 1 =10,n 1 =100,x̄ 2 =33,s 2 =9,n 2 =81
- The difference between the sample means is 52, the standard error of the difference between the sample means is 24.
- Consider the following data. Assume the data comes from appropriate random samples: Data set A: 188.5 183 194.5 185 214. 205.5 187 183.5 Data set B: 188 185.5 207 188.5 196.5 204.5 180 187 Test the hypothesis that the means of the two populations are equal versus that they are not equal.
- A sociologist is interested in determining of the life expectancy of people in Asia is greater than the life expectancy of people in Africa. In a sample of 42 Asians the mean life expectancy was 65.2 years with a standard deviation of 9.3 years. In the sample of 53 Africans the mean life expectancy was 55.3 years with a standard deviation of 8.1 years. Test the hypothesis at the .01 level of significance.
- H 0 :μ 1 −μ 2 =0,t=2.33,df=8,p−value=0.048
- H 0 :μ 1 −μ 2 =0,t=−2.33,df=8,p−value=0.024
- H 0 :μ 1 −μ 2 =0,t=−2.33,df=8,p−value=0.976
- A manufacturer is testing two different designs for an air tank. This involves observing how much pressure the tank can withstand before it bursts. For design A, four tanks are sampled and the average pressure to failure was 1500 psi with a standard deviation 250 psi. For design B, six tanks were sampled and had an average pressure to failure of 1610 psi with a standard deviation of 240 psi. Test for a difference in mean pressure to failure for the two designs at the 10% level of significance. Assume the two populations are normally distributed and have the same variance.
- Researchers were studying whether the administration of a growth hormone affects weight gain in pregnant rats. For 6 rats receivng the growth hormone the mean weight gain was 60.8 with a standard deviation of 16.4. For the 6 control rats the weight gain was 41.8 with a standard deviation of 7.6. Is the weight gain for rats receiving the hormone significantly higher than the weight gain in the control group? (source: V.T. Sara, Science 186)
- Do two types of music, type-I and type-II, have different effects upon the ability of college students to perform a series of mental tasks requiring concentration? Thirty college students were randomly divided into two groups of 15 students each. They were asked to perform a series of mental tasks under conditions that are identical in every respect except one: namely, that group A has music of type-I playing in the background, while group B has music of type-II. Following are the results showing how many of the 40 components the students were able to complete.
Complete the hypothesis test to determine if the two types of music have different effects upon the ability of college students to perform a series of mental tasks requiring concentration. (source: Vassar College)
- The campus bookstore asked a random sample of sophomores and juniors how much they spent on textbooks. The bookstore believes the two groups spend the same amount on textbooks. Fifty sophomores had a mean expenditure of $40 with a sample variance of $500 and the 70 juniors sampled had a mean expenditure of $45 with a sample variance of $800. Based on this information is the bookstore’s belief accurate?
- In 1988 Wood, et al, did a study. Eighty-nine sedentary men were given one of two treatments. Forty-two of the men were placed on a diet while forty-seven of them were put on an exercise program. The group on the diet lost an average of 7.2 kg, with a standard deviation of 3.7 kg. The men who exercised lost an average of 4 kg, with a standard deviation of 3.9 kg. Test the hypothesis that the mean weight loss would be different under the two different programs.
- Do the minutes spent exercising in a week differ between men and women in college? To answer this question a random sample of students was taken and the time each spent exercising for a week was recorded. Following is the data that was collected: Women: 65 243 0 365 455 210 100 72 24 60 64 370 190 3 100 280 Men: 190 310 70 490 0 95 310 176 203 701 300 250 Conduct a test to determine if the mean amount of exercise differs for men and women.
Review (Answers)
To view the Review answers, open this PDF file and look for section 8.6.
Additional Resources
Video: t-Test Two Sample for Means Hypothesis Test
Practice: Dependent and Independent Samples
Dependent and independent samples
What is the difference between a dependent sample and an independent sample? And why is it important to know the difference? Whether the data at hand are from a dependent or an independent sample determines which hypothesis test is used.
If your data are independent, for example, an independent samples t-test or an ANOVA without repeated measures is calculated. If your data are dependent, a t-test for dependent samples or an ANOVA with repeated measures is calculated.
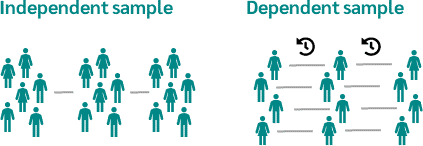
Example independent and dependent variable
Let's say you want to find out whether holidays have an effect on people's stress levels. To find out, you have created a small online survey on datatab.net that allows you to measure people's stress levels. In the survey, you ask people about their stress levels before and after their holiday. You now have two options:

In the left case you would have an independent sample, because the people you interviewed before the holiday have nothing to do with the people you interviewed after the holiday.
In the right case you would have a dependent sample, you would interview people before the holiday and interview the same people after the holiday, so the measures are always available in pairs. In this case, this is the preferred solution for this research question!
Dependent sample
In a dependent sample, the measures are related. For example, if you take a sample of people who have had a knee operation and interview them before and after the operation, this is a dependent sample. This is because the same person was interviewed at two different times.
Of course, there does not necessarily need to be a before-and-after relationship to be studied.
For example, if you want to investigate whether a new baseball bat has an effect on batting performance, and the same people play once with the old bat and once with the new one, then you have a dependent sample. In this case, the measurements are also available in pairs, each player has used both bats, so there are two measurements for each player.
And it does not have to be the same person. For example, if you wanted to find out whether, in a relationship between men and women, women do more gardening than men, you would also have a dependent sample. You would have two measures that always go together in pairs, always one woman and one man.
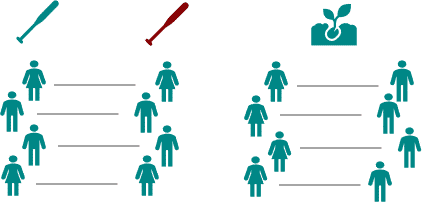
Independent sample
In independent samples, the values come from two or more different groups. For example, if the men's group and the women's group are asked about their income, independent samples exist. In this case, a person from one sample cannot be assigned to a person from the other sample.
More than two dependent or independent samples
Of course, in the case of independent and dependent sampling, there can be more than two samples. The important thing is that in the case of independent sampling, the individual groups or samples have nothing to do with each other, and in the case of dependent sampling, a respondent appears in all groups.
Hypothesis testing for dependent and independent samples
In general, there is always a hypothesis test for independent samples and a counterpart for dependent samples. Instead of the term dependent and independent , paired and unpaired are often used in the case of analysis of variance with and without repeated measures, as well as in the case of the t-test.
In DATAtab you can choose with one click whether you want to calculate the respective hypothesis test for dependent or independent samples.
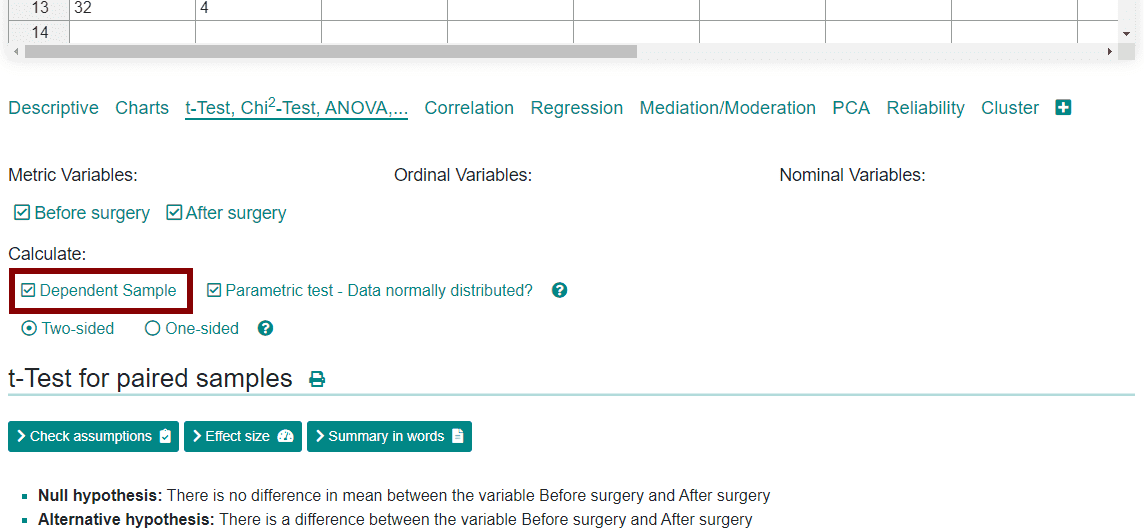
Depending on the format in which you insert your data, a variant is pre-selected. Usually, a series is a respondent or, more generally, a case. Therefore, metric values that are in a series are initially considered dependent.
If a metric and a categorical variable are clicked, the respective independent test is automatically selected.
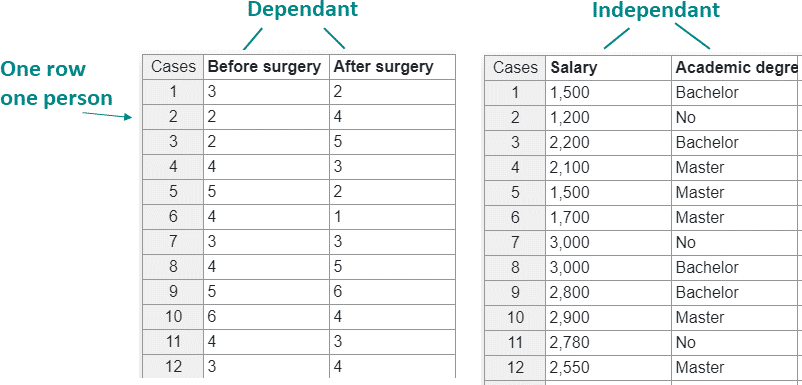
Statistics made easy
- many illustrative examples
- ideal for exams and theses
- statistics made easy on 412 pages
- 5rd revised edition (April 2024)
- Only 7.99 €
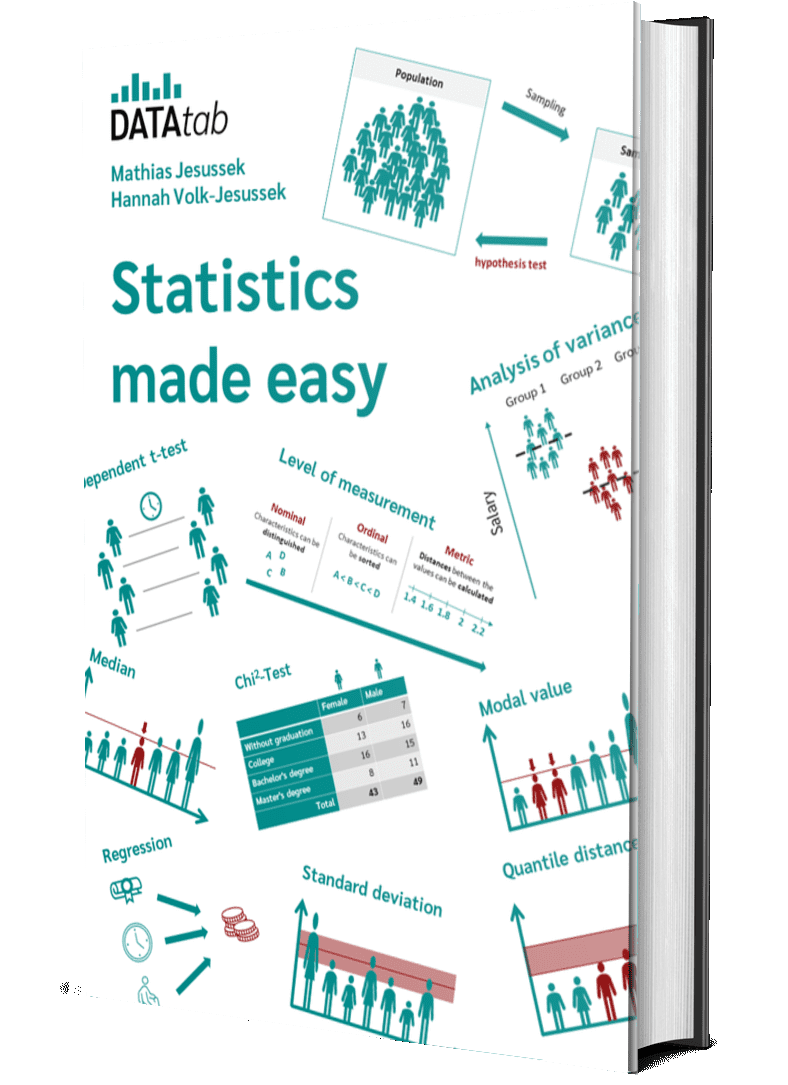
"Super simple written"
"It could not be simpler"
"So many helpful examples"
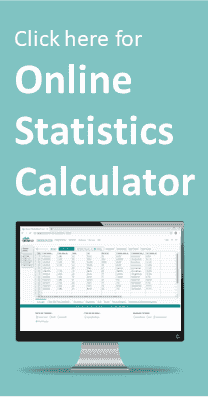
Cite DATAtab: DATAtab Team (2024). DATAtab: Online Statistics Calculator. DATAtab e.U. Graz, Austria. URL https://datatab.net
Have a language expert improve your writing
Run a free plagiarism check in 10 minutes, automatically generate references for free.
- Knowledge Base
- Methodology
- How to Write a Strong Hypothesis | Guide & Examples
How to Write a Strong Hypothesis | Guide & Examples
Published on 6 May 2022 by Shona McCombes .
A hypothesis is a statement that can be tested by scientific research. If you want to test a relationship between two or more variables, you need to write hypotheses before you start your experiment or data collection.
Table of contents
What is a hypothesis, developing a hypothesis (with example), hypothesis examples, frequently asked questions about writing hypotheses.
A hypothesis states your predictions about what your research will find. It is a tentative answer to your research question that has not yet been tested. For some research projects, you might have to write several hypotheses that address different aspects of your research question.
A hypothesis is not just a guess – it should be based on existing theories and knowledge. It also has to be testable, which means you can support or refute it through scientific research methods (such as experiments, observations, and statistical analysis of data).
Variables in hypotheses
Hypotheses propose a relationship between two or more variables . An independent variable is something the researcher changes or controls. A dependent variable is something the researcher observes and measures.
In this example, the independent variable is exposure to the sun – the assumed cause . The dependent variable is the level of happiness – the assumed effect .
Prevent plagiarism, run a free check.
Step 1: ask a question.
Writing a hypothesis begins with a research question that you want to answer. The question should be focused, specific, and researchable within the constraints of your project.
Step 2: Do some preliminary research
Your initial answer to the question should be based on what is already known about the topic. Look for theories and previous studies to help you form educated assumptions about what your research will find.
At this stage, you might construct a conceptual framework to identify which variables you will study and what you think the relationships are between them. Sometimes, you’ll have to operationalise more complex constructs.
Step 3: Formulate your hypothesis
Now you should have some idea of what you expect to find. Write your initial answer to the question in a clear, concise sentence.
Step 4: Refine your hypothesis
You need to make sure your hypothesis is specific and testable. There are various ways of phrasing a hypothesis, but all the terms you use should have clear definitions, and the hypothesis should contain:
- The relevant variables
- The specific group being studied
- The predicted outcome of the experiment or analysis
Step 5: Phrase your hypothesis in three ways
To identify the variables, you can write a simple prediction in if … then form. The first part of the sentence states the independent variable and the second part states the dependent variable.
In academic research, hypotheses are more commonly phrased in terms of correlations or effects, where you directly state the predicted relationship between variables.
If you are comparing two groups, the hypothesis can state what difference you expect to find between them.
Step 6. Write a null hypothesis
If your research involves statistical hypothesis testing , you will also have to write a null hypothesis. The null hypothesis is the default position that there is no association between the variables. The null hypothesis is written as H 0 , while the alternative hypothesis is H 1 or H a .
Hypothesis testing is a formal procedure for investigating our ideas about the world using statistics. It is used by scientists to test specific predictions, called hypotheses , by calculating how likely it is that a pattern or relationship between variables could have arisen by chance.
A hypothesis is not just a guess. It should be based on existing theories and knowledge. It also has to be testable, which means you can support or refute it through scientific research methods (such as experiments, observations, and statistical analysis of data).
A research hypothesis is your proposed answer to your research question. The research hypothesis usually includes an explanation (‘ x affects y because …’).
A statistical hypothesis, on the other hand, is a mathematical statement about a population parameter. Statistical hypotheses always come in pairs: the null and alternative hypotheses. In a well-designed study , the statistical hypotheses correspond logically to the research hypothesis.
Cite this Scribbr article
If you want to cite this source, you can copy and paste the citation or click the ‘Cite this Scribbr article’ button to automatically add the citation to our free Reference Generator.
McCombes, S. (2022, May 06). How to Write a Strong Hypothesis | Guide & Examples. Scribbr. Retrieved 7 June 2024, from https://www.scribbr.co.uk/research-methods/hypothesis-writing/
Is this article helpful?
Shona McCombes
Other students also liked, operationalisation | a guide with examples, pros & cons, what is a conceptual framework | tips & examples, a quick guide to experimental design | 5 steps & examples.
Dependent t-test for paired samples (cont...)
What hypothesis is being tested.
The dependent t-test is testing the null hypothesis that there are no differences between the means of the two related groups. If we get a statistically significant result, we can reject the null hypothesis that there are no differences between the means in the population and accept the alternative hypothesis that there are differences between the means in the population. We can express this as follows:
H 0 : µ 1 = µ 2
H A : µ 1 ≠ µ 2
What is the advantage of a dependent t-test over an independent t-test?
Before we answer this question, we need to point out that you cannot choose one test over the other unless your study design allows it. What we are discussing here is whether it is advantageous to design a study that uses one set of participants whom are measured twice or two separate groups of participants measured once each. The major advantage of choosing a repeated-measures design (and therefore, running a dependent t-test) is that you get to eliminate the individual differences that occur between participants – the concept that no two people are the same – and this increases the power of the test. What this means is that if you are more likely to detect a (statistically significant) difference, if one does exist, using the dependent t-test versus the independent t-test.
Can the dependent t-test be used to compare different participants?
Yes, but this does not happen very often. You can use the dependent t-test instead of using the usual independent t-test when each participant in one of the independent groups is closely related to another participant in the other group on many individual characteristics. This approach is called a "matched-pairs" design. The reason we might want to do this is that the major advantage of running a within-subject (repeated-measures) design is that you get to eliminate between-groups variation from the equation (each individual is unique and will react slightly differently than someone else), thereby increasing the power of the test. Hence, the reason why we use the same participants – we expect them to react in the same way as they are, after all, the same person. The most obvious case of when a "matched-pairs" design might be implemented is when using identical twins. Effectively, you are choosing parameters to match your participants on, which you believe will result in each pair of participants reacting in a similar way.
How do I report the result of a dependent t-test?
You need to report the test as follows:

where df is N – 1, where N = number of participants.
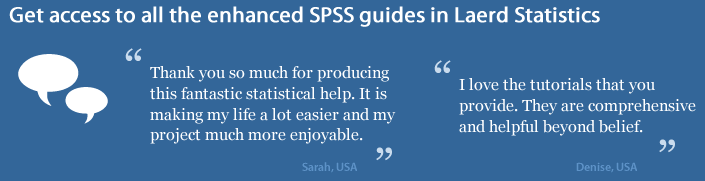
Should I report confidence levels?
Confidence intervals (CI) are a useful statistic to include because they indicate the direction and size of a result. It is common to report 95% confidence intervals, which you will most often see reported as 95% CI. Programmes such as SPSS Statistics will automatically calculate these confidence intervals for you; otherwise, you need to calculate them by hand. You will want to report the mean and 95% confidence interval for the difference between the two related groups.
If you wish to run a dependent t-test in SPSS Statistics, you can find out how to do this in our Dependent T-Test guide.

Want to create or adapt books like this? Learn more about how Pressbooks supports open publishing practices.
8.1 Inference for Two Dependent Samples (Matched Pairs)
Learning Objectives
By the end of this chapter, the student should be able to:
- Classify hypothesis tests by type
- Conduct and interpret hypothesis tests for two population means, population standard deviations known
- Conduct and interpret hypothesis tests for two population means, population standard deviations unknown
- Conduct and interpret hypothesis tests for matched or paired samples
- Conduct and interpret hypothesis tests for two population proportions
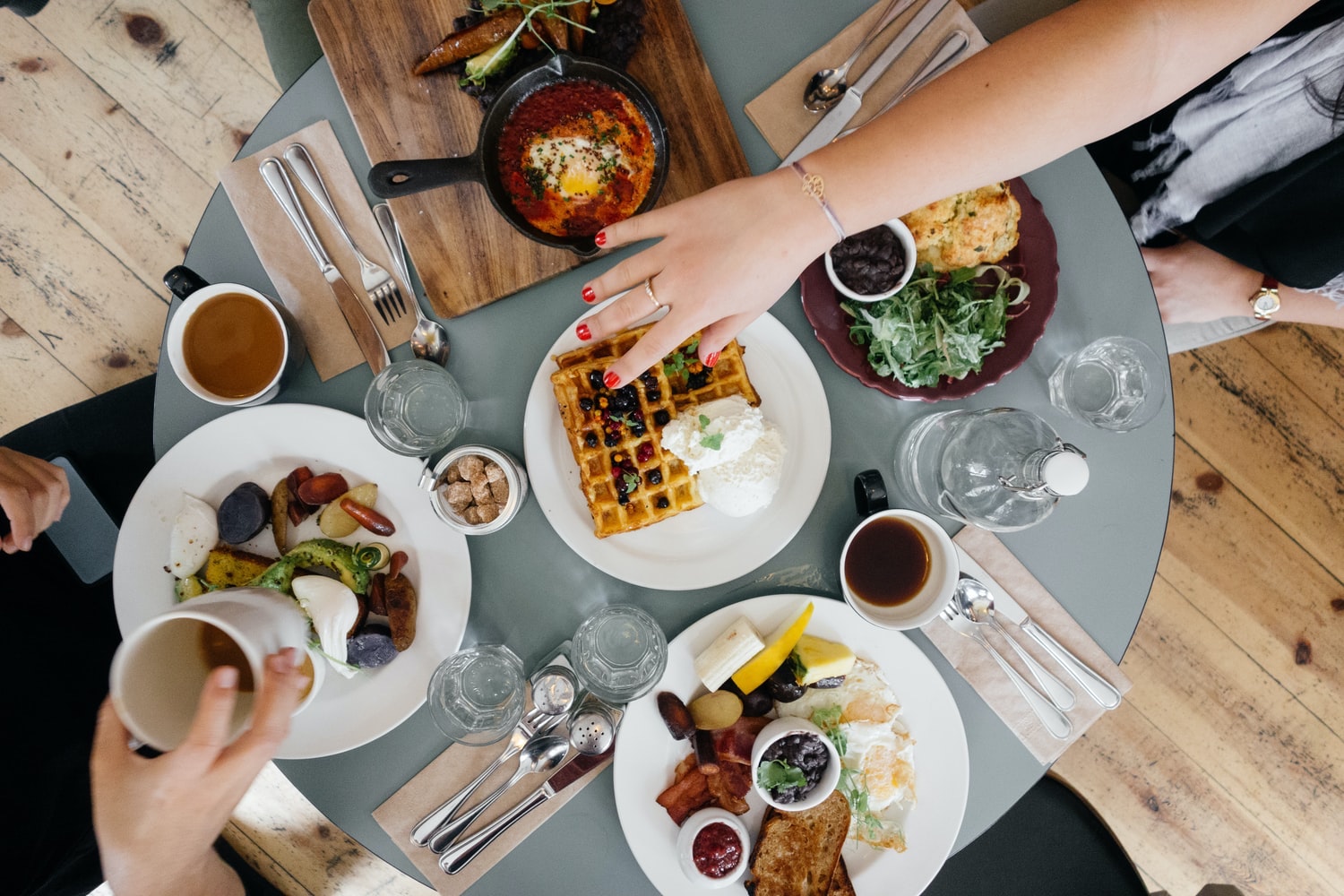
Studies often compare two groups. For example, maybe researchers are interested in the effect aspirin has in preventing heart attacks. One group is given aspirin and the other a placebo , and the heart attack rate is studied over several years. Other studies may compare various diet and exercise programs. Politicians compare the proportion of individuals from different income brackets who might vote for them. Students are interested in whether SAT or GRE preparatory courses really help raise their scores.
You have learned to conduct inference on single means and single proportions . We know that the first step is deciding what type of data we are working with. For quantitative data we are focused on means, while for categorical we are focused on proportions. In this chapter we will compare two means or two proportions to each other. The general procedure is still the same, just expanded. With two sample analysis it is good to know what the formulas look like and where they come from, however you will probably lean heavily on technology in preforming the calculations.
To compare two means we are obviously working with two groups, but first we need to think about the relationship between them. The groups are classified either as independent or dependent. I ndependent samples consist of two samples that have no relationship, that is, sample values selected from one population are not related in any way to sample values selected from the other population. Dependent samples consist of two groups that have some sort of identifiable relationship.
Two Dependent Samples (Matched Pairs)
Two samples that are dependent typically come from a matched pairs experimental design. The parameter tested using matched pairs is the population mean difference . When using inference techniques for matched or paired samples, the following characteristics should be present:
- Simple random sampling is used.
- Sample sizes are often small.
- Two measurements (samples) are drawn from the same pair of (or two extremely similar) individuals or objects.
- Differences are calculated from the matched or paired samples.
- The differences form the sample that is used for analysis.

Confidence intervals may be calculated on their own for two samples but often, especially in the case of matched pairs, we first want to formally check to see if a difference exists with a hypothesis test. If we do find a statistically significant difference then we may estimate it with a CI after the fact.
Hypothesis Tests for the Mean difference
In a hypothesis test for matched or paired samples, subjects are matched in pairs and differences are calculated, and the population mean difference, μ d , is our parameter of interest. Although it is possible to test for a certain magnitude of effect, we are most often just looking for a general effect. Our hypothesis would then look like:
H o : μ d =0
H a : μ d (<, >, ≠) 0
The steps are the same as we are familiar with, but it is tested using a Student’s-t test for a single population mean with n – 1 degrees of freedom, with the test statistic:

A study was conducted to investigate the effectiveness of hypnotism in reducing pain. Results for randomly selected subjects are shown in the figure below. A lower score indicates less pain. The “before” value is matched to an “after” value and the differences are calculated. The differences have a normal distribution. Are the sensory measurements, on average, lower after hypnotism? Test at a 5% significance level.
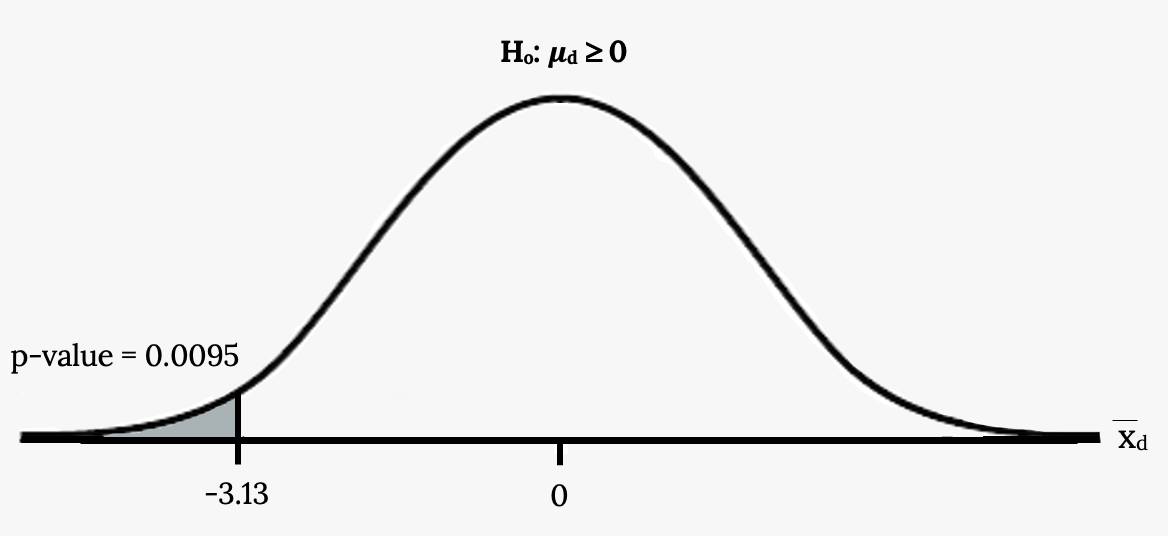
A study was conducted to investigate how effective a new diet was in lowering cholesterol. Results for the randomly selected subjects are shown in the table. The differences have a normal distribution. Are the subjects’ cholesterol levels lower on average after the diet? Test at the 5% level.
Confidence Intervals for the Mean difference

If we are using the t distribution, the error bound for the population mean difference is:
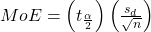
- use df = n – 1 degrees of freedom, where n is the number of pairs
- s d = standard deviation of the differences.
A college football coach was interested in whether the college’s strength development class increased his players’ maximum lift (in pounds) on the bench press exercise. He asked four of his players to participate in a study. The amount of weight they could each lift was recorded before they took the strength development class. After completing the class, the amount of weight they could each lift was again measured. The data are as follows:
The coach wants to know if the strength development class makes his players stronger, on average.
Using the differences data, calculate the sample mean and the sample standard deviation.
Using the difference data, this becomes a test of a single __________ (fill in the blank).

Calculate the p -value:
What is the conclusion?
A new prep class was designed to improve SAT test scores. Five students were selected at random. Their scores on two practice exams were recorded, one before the class and one after. The data recorded in the figure below. Are the scores, on average, higher after the class? Test at a 5% level.
Image Credits
Figure 8.1: Ali Inay (2015). “Brunching with Friends.” Public domain. Retrieved from https://unsplash.com/photos/y3aP9oo9Pjc
Figure 8.3: Kindred Grey via Virginia Tech (2020). “Figure 8.3” CC BY-SA 4.0. Retrieved from https://commons.wikimedia.org/wiki/File:Figure_8.3.png . Adaptation of Figure 5.39 from OpenStax Introductory Statistics (2013) (CC BY 4.0). Retrieved from https://openstax.org/books/statistics/pages/5-practice
Figure 8.6: Kindred Grey via Virginia Tech (2020). “Figure 8.6” CC BY-SA 4.0. Retrieved from https://commons.wikimedia.org/wiki/File:Figure_8.6.png . Adaptation of Figure 5.39 from OpenStax Introductory Statistics (2013) (CC BY 4.0). Retrieved from https://openstax.org/books/statistics/pages/5-practice
An inactive treatment that has no real effect on the explanatory variable
The facet of statistics dealing with using a sample to generalize (or infer) about the population
The arithmetic mean, or average of a population
The number of individuals that have a characteristic we are interested in divided by the total number in the population
Numerical data with a mathematical context
Data that describes qualities, or puts individuals into categories
The occurrence of one event has no effect on the probability of the occurrence of another event
Very similar individuals (or even the same individual) receive two different two treatments (or treatment vs. control) then the difference in results are compared
The mean of the differences in a matched pairs design
The probability distribution of a statistic at a given sample size
The value that is calculated from a sample used to estimate an unknown population parameter
Significant Statistics Copyright © 2020 by John Morgan Russell, OpenStaxCollege, OpenIntro is licensed under a Creative Commons Attribution-ShareAlike 4.0 International License , except where otherwise noted.
Share This Book

Statistics Resources
- Excel - Tutorials
- Basic Probability Rules
- Single Event Probability
- Complement Rule
- Intersections & Unions
- Compound Events
- Levels of Measurement
- Independent and Dependent Variables
- Entering Data
- Central Tendency
- Data and Tests
- Displaying Data
- Discussing Statistics In-text
- SEM and Confidence Intervals
- Two-Way Frequency Tables
- Empirical Rule
- Finding Probability
- Accessing SPSS
- Chart and Graphs
- Frequency Table and Distribution
- Descriptive Statistics
- Converting Raw Scores to Z-Scores
- Converting Z-scores to t-scores
- Split File/Split Output
- Partial Eta Squared
- Downloading and Installing G*Power: Windows/PC
- Correlation
- Testing Parametric Assumptions
- One-Way ANOVA
- Two-Way ANOVA
- Repeated Measures ANOVA
- Goodness-of-Fit
- Test of Association
- Pearson's r
- Point Biserial
- Mediation and Moderation
- Simple Linear Regression
- Multiple Linear Regression
- Binomial Logistic Regression
- Multinomial Logistic Regression
- Independent Samples T-test
Dependent Samples T-test
- Testing Assumptions
- T-tests using SPSS
- T-Test Practice
- Predictive Analytics This link opens in a new window
- Quantitative Research Questions
- Null & Alternative Hypotheses
- One-Tail vs. Two-Tail
- Alpha & Beta
- Associated Probability
- Decision Rule
- Statement of Conclusion
- Statistics Group Sessions
The dependent samples t-test is used to compare the sample means from two related groups. This means that the scores for both groups being compared come from the same people. The purpose of this test is to determine if there is a change from one measurement (group) to the other.
Basic Hypotheses
Null: The mean difference between the two groups is not different from 0. Alternative: The mean difference between the two groups is different from 0.
Real-World Examples
- Is there an improvement in reading scores after participating in the Read Like a Pro course?
- Do people recall more words after learning a memorization strategy?
- Do people perform better when given praise or punishment?
- Is there a difference in how many miles a car can be driven when using AC versus having the windows down?
Reporting Results in APA Style
When reporting the results of the dependent-samples t-test, APA Style has very specific requirements on what information should be included. Below is the key information required for reporting the results of the. You want to replace the red text with the appropriate values from your output.
t (degrees of freedom) = the t statistic, p = p value.
Example : A dependent-samples t-test was run to determine if long-term recall improved with the introduction of the Say it Again memorization technique. The results showed that the average number of words recalled without this technique ( M = 13.5, SD = 2.4) was significantly less than the average number of words recalled with this technique ( M = 16.2, SD = 2.7), ( t (52) = 4.8, p < .001).
- When reporting the p-value, there are two ways to approach it. One is when the results are not significant. In that case, you want to report the p-value exactly: p = .24. The other is when the results are significant. In this case, you can report the p-value as being less than the level of significance: p < .05.
- The t statistic should be reported to two decimal places without a 0 before the decimal point: .36
- Degrees of freedom for this test are n - 1, where " n " represents the number of pairs in the sample. n can be found in the SPSS output.
Additional Resources
Laerd Statistics - Dependent T-test for Paired Samples guide Statistics Solutions - Paired Samples T-test
Was this resource helpful?
- << Previous: Independent Samples T-test
- Next: Testing Assumptions >>
- Last Updated: May 29, 2024 9:48 AM
- URL: https://resources.nu.edu/statsresources

- Bipolar Disorder
- Therapy Center
- When To See a Therapist
- Types of Therapy
- Best Online Therapy
- Best Couples Therapy
- Best Family Therapy
- Managing Stress
- Sleep and Dreaming
- Understanding Emotions
- Self-Improvement
- Healthy Relationships
- Student Resources
- Personality Types
- Guided Meditations
- Verywell Mind Insights
- 2024 Verywell Mind 25
- Mental Health in the Classroom
- Editorial Process
- Meet Our Review Board
- Crisis Support
How to Write a Great Hypothesis
Hypothesis Definition, Format, Examples, and Tips
Kendra Cherry, MS, is a psychosocial rehabilitation specialist, psychology educator, and author of the "Everything Psychology Book."
:max_bytes(150000):strip_icc():format(webp)/IMG_9791-89504ab694d54b66bbd72cb84ffb860e.jpg)
Amy Morin, LCSW, is a psychotherapist and international bestselling author. Her books, including "13 Things Mentally Strong People Don't Do," have been translated into more than 40 languages. Her TEDx talk, "The Secret of Becoming Mentally Strong," is one of the most viewed talks of all time.
:max_bytes(150000):strip_icc():format(webp)/VW-MIND-Amy-2b338105f1ee493f94d7e333e410fa76.jpg)
Verywell / Alex Dos Diaz
- The Scientific Method
Hypothesis Format
Falsifiability of a hypothesis.
- Operationalization
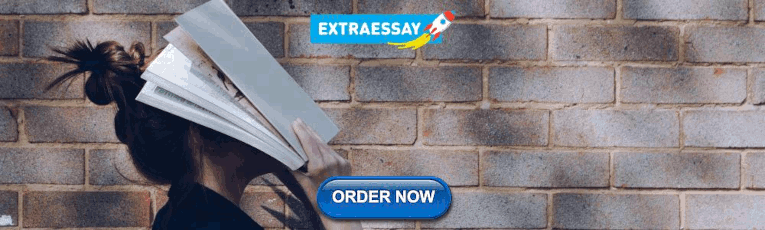
Hypothesis Types
Hypotheses examples.
- Collecting Data
A hypothesis is a tentative statement about the relationship between two or more variables. It is a specific, testable prediction about what you expect to happen in a study. It is a preliminary answer to your question that helps guide the research process.
Consider a study designed to examine the relationship between sleep deprivation and test performance. The hypothesis might be: "This study is designed to assess the hypothesis that sleep-deprived people will perform worse on a test than individuals who are not sleep-deprived."
At a Glance
A hypothesis is crucial to scientific research because it offers a clear direction for what the researchers are looking to find. This allows them to design experiments to test their predictions and add to our scientific knowledge about the world. This article explores how a hypothesis is used in psychology research, how to write a good hypothesis, and the different types of hypotheses you might use.
The Hypothesis in the Scientific Method
In the scientific method , whether it involves research in psychology, biology, or some other area, a hypothesis represents what the researchers think will happen in an experiment. The scientific method involves the following steps:
- Forming a question
- Performing background research
- Creating a hypothesis
- Designing an experiment
- Collecting data
- Analyzing the results
- Drawing conclusions
- Communicating the results
The hypothesis is a prediction, but it involves more than a guess. Most of the time, the hypothesis begins with a question which is then explored through background research. At this point, researchers then begin to develop a testable hypothesis.
Unless you are creating an exploratory study, your hypothesis should always explain what you expect to happen.
In a study exploring the effects of a particular drug, the hypothesis might be that researchers expect the drug to have some type of effect on the symptoms of a specific illness. In psychology, the hypothesis might focus on how a certain aspect of the environment might influence a particular behavior.
Remember, a hypothesis does not have to be correct. While the hypothesis predicts what the researchers expect to see, the goal of the research is to determine whether this guess is right or wrong. When conducting an experiment, researchers might explore numerous factors to determine which ones might contribute to the ultimate outcome.
In many cases, researchers may find that the results of an experiment do not support the original hypothesis. When writing up these results, the researchers might suggest other options that should be explored in future studies.
In many cases, researchers might draw a hypothesis from a specific theory or build on previous research. For example, prior research has shown that stress can impact the immune system. So a researcher might hypothesize: "People with high-stress levels will be more likely to contract a common cold after being exposed to the virus than people who have low-stress levels."
In other instances, researchers might look at commonly held beliefs or folk wisdom. "Birds of a feather flock together" is one example of folk adage that a psychologist might try to investigate. The researcher might pose a specific hypothesis that "People tend to select romantic partners who are similar to them in interests and educational level."
Elements of a Good Hypothesis
So how do you write a good hypothesis? When trying to come up with a hypothesis for your research or experiments, ask yourself the following questions:
- Is your hypothesis based on your research on a topic?
- Can your hypothesis be tested?
- Does your hypothesis include independent and dependent variables?
Before you come up with a specific hypothesis, spend some time doing background research. Once you have completed a literature review, start thinking about potential questions you still have. Pay attention to the discussion section in the journal articles you read . Many authors will suggest questions that still need to be explored.
How to Formulate a Good Hypothesis
To form a hypothesis, you should take these steps:
- Collect as many observations about a topic or problem as you can.
- Evaluate these observations and look for possible causes of the problem.
- Create a list of possible explanations that you might want to explore.
- After you have developed some possible hypotheses, think of ways that you could confirm or disprove each hypothesis through experimentation. This is known as falsifiability.
In the scientific method , falsifiability is an important part of any valid hypothesis. In order to test a claim scientifically, it must be possible that the claim could be proven false.
Students sometimes confuse the idea of falsifiability with the idea that it means that something is false, which is not the case. What falsifiability means is that if something was false, then it is possible to demonstrate that it is false.
One of the hallmarks of pseudoscience is that it makes claims that cannot be refuted or proven false.
The Importance of Operational Definitions
A variable is a factor or element that can be changed and manipulated in ways that are observable and measurable. However, the researcher must also define how the variable will be manipulated and measured in the study.
Operational definitions are specific definitions for all relevant factors in a study. This process helps make vague or ambiguous concepts detailed and measurable.
For example, a researcher might operationally define the variable " test anxiety " as the results of a self-report measure of anxiety experienced during an exam. A "study habits" variable might be defined by the amount of studying that actually occurs as measured by time.
These precise descriptions are important because many things can be measured in various ways. Clearly defining these variables and how they are measured helps ensure that other researchers can replicate your results.
Replicability
One of the basic principles of any type of scientific research is that the results must be replicable.
Replication means repeating an experiment in the same way to produce the same results. By clearly detailing the specifics of how the variables were measured and manipulated, other researchers can better understand the results and repeat the study if needed.
Some variables are more difficult than others to define. For example, how would you operationally define a variable such as aggression ? For obvious ethical reasons, researchers cannot create a situation in which a person behaves aggressively toward others.
To measure this variable, the researcher must devise a measurement that assesses aggressive behavior without harming others. The researcher might utilize a simulated task to measure aggressiveness in this situation.
Hypothesis Checklist
- Does your hypothesis focus on something that you can actually test?
- Does your hypothesis include both an independent and dependent variable?
- Can you manipulate the variables?
- Can your hypothesis be tested without violating ethical standards?
The hypothesis you use will depend on what you are investigating and hoping to find. Some of the main types of hypotheses that you might use include:
- Simple hypothesis : This type of hypothesis suggests there is a relationship between one independent variable and one dependent variable.
- Complex hypothesis : This type suggests a relationship between three or more variables, such as two independent and dependent variables.
- Null hypothesis : This hypothesis suggests no relationship exists between two or more variables.
- Alternative hypothesis : This hypothesis states the opposite of the null hypothesis.
- Statistical hypothesis : This hypothesis uses statistical analysis to evaluate a representative population sample and then generalizes the findings to the larger group.
- Logical hypothesis : This hypothesis assumes a relationship between variables without collecting data or evidence.
A hypothesis often follows a basic format of "If {this happens} then {this will happen}." One way to structure your hypothesis is to describe what will happen to the dependent variable if you change the independent variable .
The basic format might be: "If {these changes are made to a certain independent variable}, then we will observe {a change in a specific dependent variable}."
A few examples of simple hypotheses:
- "Students who eat breakfast will perform better on a math exam than students who do not eat breakfast."
- "Students who experience test anxiety before an English exam will get lower scores than students who do not experience test anxiety."
- "Motorists who talk on the phone while driving will be more likely to make errors on a driving course than those who do not talk on the phone."
- "Children who receive a new reading intervention will have higher reading scores than students who do not receive the intervention."
Examples of a complex hypothesis include:
- "People with high-sugar diets and sedentary activity levels are more likely to develop depression."
- "Younger people who are regularly exposed to green, outdoor areas have better subjective well-being than older adults who have limited exposure to green spaces."
Examples of a null hypothesis include:
- "There is no difference in anxiety levels between people who take St. John's wort supplements and those who do not."
- "There is no difference in scores on a memory recall task between children and adults."
- "There is no difference in aggression levels between children who play first-person shooter games and those who do not."
Examples of an alternative hypothesis:
- "People who take St. John's wort supplements will have less anxiety than those who do not."
- "Adults will perform better on a memory task than children."
- "Children who play first-person shooter games will show higher levels of aggression than children who do not."
Collecting Data on Your Hypothesis
Once a researcher has formed a testable hypothesis, the next step is to select a research design and start collecting data. The research method depends largely on exactly what they are studying. There are two basic types of research methods: descriptive research and experimental research.
Descriptive Research Methods
Descriptive research such as case studies , naturalistic observations , and surveys are often used when conducting an experiment is difficult or impossible. These methods are best used to describe different aspects of a behavior or psychological phenomenon.
Once a researcher has collected data using descriptive methods, a correlational study can examine how the variables are related. This research method might be used to investigate a hypothesis that is difficult to test experimentally.
Experimental Research Methods
Experimental methods are used to demonstrate causal relationships between variables. In an experiment, the researcher systematically manipulates a variable of interest (known as the independent variable) and measures the effect on another variable (known as the dependent variable).
Unlike correlational studies, which can only be used to determine if there is a relationship between two variables, experimental methods can be used to determine the actual nature of the relationship—whether changes in one variable actually cause another to change.
The hypothesis is a critical part of any scientific exploration. It represents what researchers expect to find in a study or experiment. In situations where the hypothesis is unsupported by the research, the research still has value. Such research helps us better understand how different aspects of the natural world relate to one another. It also helps us develop new hypotheses that can then be tested in the future.
Thompson WH, Skau S. On the scope of scientific hypotheses . R Soc Open Sci . 2023;10(8):230607. doi:10.1098/rsos.230607
Taran S, Adhikari NKJ, Fan E. Falsifiability in medicine: what clinicians can learn from Karl Popper [published correction appears in Intensive Care Med. 2021 Jun 17;:]. Intensive Care Med . 2021;47(9):1054-1056. doi:10.1007/s00134-021-06432-z
Eyler AA. Research Methods for Public Health . 1st ed. Springer Publishing Company; 2020. doi:10.1891/9780826182067.0004
Nosek BA, Errington TM. What is replication ? PLoS Biol . 2020;18(3):e3000691. doi:10.1371/journal.pbio.3000691
Aggarwal R, Ranganathan P. Study designs: Part 2 - Descriptive studies . Perspect Clin Res . 2019;10(1):34-36. doi:10.4103/picr.PICR_154_18
Nevid J. Psychology: Concepts and Applications. Wadworth, 2013.
By Kendra Cherry, MSEd Kendra Cherry, MS, is a psychosocial rehabilitation specialist, psychology educator, and author of the "Everything Psychology Book."

Module 7 - Comparing Continuous Outcomes
- Page:
- 1
- | 2
- | 3
- | 4
- | 5
- | 6
- | 7
- | 8
- | 9

T-test for Two Dependent Samples (Paired or Matched Design)
T-test of two dependent (paired) samples using r.

The third application of a t-test that we will consider is for two dependent (paired or matched) samples. This can be applied in either of two types of comparisons.
- Pre-post Comparisons: One sample of subjects is measure twice under two different conditions, e.g., before and after receiving a drug.
- Comparison of Matched Samples: Two samples of pair-matched subjects, e.g., siblings or twins, or subjects matched by age and hospital ward
Example 1: Does Intervention Increase HIV Knowledge?
Early in the HIV epidemic, there was poor knowledge of HIV transmission risks among health care staff. A short training was developed to improve knowledge and attitudes around HIV disease. Was the training effective in improving knowledge?
Table - Mean ( ± SD) knowledge scores, pre- and post-intervention, n=15
The raw data for this comparison is shown in the next table.
The strategy is to calculate the pre-/post- difference in knowledge score for each person and determine whether the mean difference=0.
First, establish the null and alternative hypotheses.
- H 0 : μ d = 0
- H 1 : μ d ≠ 0
Then compute the test statistic for paired or matched samples
For the example above we can compute the p-value using R. First, we compute the means.
> mean(Kscore1 ) [1] 18.33333
> mean(Kscore2) [1] 21.86667
Then we perform the t-test for two dependent samples.
> t.test(Kscore2,Kscore1,paired=TRUE)
Paired t-test
data: Kscore2 and Kscore1 t = 3.4861, df = 14, p-value = 0.003634 alternative hypothesis: true difference in means is not equal to 0 95 percent confidence interval: 1.359466 5.707201 sample estimates: mean of th e differences 3.533333
The null hypothesis is that the mean change in knowledge scores from before to after is 0. However, the analyis shows that the mean difference is 3.53 with a 95% confidence interval that ranges from 1.36 to 5.7. Since the confidence interval does not include the null value of 0, the p-value must be < 0.05, and in fact it is 0.003634.
return to top | previous page | next page
Content ©2019. Some Rights Reserved. Date last modified: May 16, 2019. Wayne W. LaMorte, MD, PhD, MPH


- Calculators
- Descriptive Statistics
- Merchandise
- Which Statistics Test?
T Test Calculator for 2 Dependent Means
The t -test for dependent means (also called a repeated-measures t -test, paired samples t -test, matched pairs t -test and matched samples t -test) is used to compare the means of two sets of scores that are directly related to each other. So, for example, it could be used to test whether subjects' galvanic skin responses are different under two conditions - first, on exposure to a photograph of a beach scene; second, on exposure to a photograph of a spider.
Requirements
- The data is normally distributed
- Scale of measurement should be interval or ratio
- The two sets of scores are paired or matched in some way
Null Hypothesis
H 0 : U D = U 1 - U 2 = 0, where U D equals the mean of the population of difference scores across the two measurements.

A computational model for sample dependence in hypothesis testing of genome data
- Original Paper - Cross-Disciplinary Physics and Related Areas of Science and Technology
- Published: 30 May 2024
Cite this article
- Sunhee Kim ORCID: orcid.org/0000-0002-9525-3179 1 &
- Chang-Yong Lee ORCID: orcid.org/0000-0003-1778-6532 1
15 Accesses
Explore all metrics
Statistical hypothesis testing assumes that the samples being analyzed are statistically independent, meaning that the occurrence of one sample does not affect the probability of the occurrence of another. In reality, however, this assumption may not always hold. When samples are not independent, it is important to consider their interdependence when interpreting the results of the hypothesis test. In this study, we address the issue of sample dependence in hypothesis testing by introducing the concept of adjusted sample size. This adjusted sample size provides additional information about the test results, which is particularly useful when samples exhibit dependence. To determine the adjusted sample size, we use the theory of networks to quantify sample dependence and model the variance of network density as a function of sample size. Our approach involves estimating the adjusted sample size by analyzing the variance of the network density, which reflects the degree of sample dependence. Through simulations, we demonstrate that dependent samples yield a higher variance in network density compared to independent samples, validating our method for estimating the adjusted sample size. Furthermore, we apply our proposed method to genomic datasets, estimating the adjusted sample size to effectively account for sample dependence in hypothesis testing. This guides interpreting test results and ensures more accurate data analysis.
This is a preview of subscription content, log in via an institution to check access.
Access this article
Price includes VAT (Russian Federation)
Instant access to the full article PDF.
Rent this article via DeepDyve
Institutional subscriptions
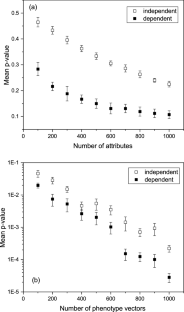
Similar content being viewed by others
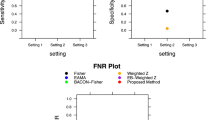
Robust meta-analysis for large-scale genomic experiments based on an empirical approach
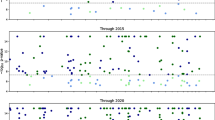
Priors, population sizes, and power in genome-wide hypothesis tests
Control procedures and estimators of the false discovery rate and their application in low-dimensional settings: an empirical investigation, code and script availability.
We have released our analysis tool, a detailed script of the sampling procedure, input files, and output files so that anyone can reproduce the results. These are available at https://github.com/infoLab204/adj_size .
W. Finch, J. Bolin, K. Kelley, Multilevel Modeling Using R (Chapman and Hall/CRC, 2014)
Google Scholar
E. Gómez-de-Mariscal, V. Guerrero, A. Sneider et al., Sci. Rep. 11 , 20942 (2021). https://doi.org/10.1038/s41598-021-00199-5
Article ADS Google Scholar
K. Stewart, Encyclopedia of Quality of Life and Well-Being Research (Springer, New York, 2014), pp.6462–6464
Book Google Scholar
I. Park, H. Lee, Stat. Can. 30 , 183 (2004)
L. Kish, Survey Sampling (John Wiley, New York, 1965)
M. Lin, H. Lucas Jr., G. Shmueli, Inf. Syst. Res. 24 , 906 (2013). https://doi.org/10.1287/isre.2013.0480
Article Google Scholar
A. Barabási, Network Science (Cambridge University Press, Cambridge, 2016)
S. Kim, J. Yun, J. Korean Phys. Soc. 81 , 697 (2022). https://doi.org/10.1007/s40042-022-00590-z
H. Jo, J. Korean Phys. Soc. 82 , 430 (2023). https://doi.org/10.1007/s40042-022-00675-9
R. Nelsen, An Introduction to Copulas (Springer, New York, 1999)
D. Tjøstheim, H. Otneim, B. Støve, Statistical Modeling Using Local Gaussian Approximation (Academic Press, Cambridge, 2021), pp.135–159
D. Lewandowski, D. Kurowicka, H. Joe, J. Multivar. Anal. 100 , 1989 (2009). https://doi.org/10.1016/j.jmva.2009.04.008
K. Zhao, C. Tung et al., Nat. Commun. 13 , 467 (2011). https://doi.org/10.1038/ncomms1467
K. Kim, B. Nawade et al., Front. Plant Sci. 13 , 1036177 (2022). https://doi.org/10.3389/fpls.2022.1036177
K. Zhao, 44K SNP set. (Rice Diversity), http://ricediversity.org/data/index.cfm. Accessed 13 Apr 2024
K. Kim, Data Sheet 1.xlsx (850K_KNU data), https://www.frontiersin.org/articles/10.3389/fpls.2022.1036177/full#supplementary-material. Accessed 13 Apr 2024
W. Qiu, H. Joe, rcorrmatrix (cluster generation) https://rdrr.io/cran/clusterGeneration/man/rcorrmatrix.html. Accessed 13 Apr 2024
W. Press, S. Teukolsky, W. Vetterling, B. Flannery, Numerical Recipes in C: The Art of Scientific Computing , 2nd edn. (Cambridge University Press, Cambridge, 1992), pp.699–706
S. Besenbacher, T. Mailund, M. Schierup, Genetics 181 , 747 (2009). https://doi.org/10.1534/genetics.108.092643
A. Edwards, Genetics 179 , 1143 (2008). https://doi.org/10.1534/genetics.104.92940
G. Di Leo, F. Sardanelli, Eur. Radiol. Exp. 4 , 18 (2020). https://doi.org/10.1186/s41747-020-0145-y
C. Lee, Comput. Biol. Chem. 64 , 94 (2016). https://doi.org/10.1016/j.compbiolchem.2016.06.003
Article MathSciNet Google Scholar
C. Kelley, Iterative Methods for Linear and Nonlinear Equations (SIAM, Philadelphia, 1995)
J. Myers, A. Well, Research Design and Statistical Analysis , 2nd edn. (Lawrence Erlbaum, Mahwah, 2003)
A. Brophy, Behav. Res. Methods 18 , 45 (1986). https://doi.org/10.3758/BF03200993
R-core, cor.test.R (stats package) https://www.rdocumentation.org/packages/stats/versions/3.6.2/topics/cor.test. Accessed 13 Apr 2024
S. Stigler, Ann. Statist. 9 , 465 (1981). https://doi.org/10.1214/aos/1176345451
R. Burden, J. Faires, Numerical Analysis , 9th edn. (Brooks/cole, Pacific Grove, 2010)
Download references
Acknowledgements
We are very grateful to Prof. Yong-Jin Park and Dr. Sang-Ho Chu for providing us with the 580K_KNU datasets. This work was supported by the National Research Foundation of Korea(NRF) grant funded by the Korean government(MSIT) (No. 2022R1A4A1030348), (No. 2021R1I1A3044289), and by the research grant of the Kongju National University in 2021.
Author information
Authors and affiliations.
The Department of Industrial Engineering, Kongju National University, Cheonan, 31080, Republic of Korea
Sunhee Kim & Chang-Yong Lee
You can also search for this author in PubMed Google Scholar
Corresponding author
Correspondence to Chang-Yong Lee .
Additional information
Publisher's note.
Springer Nature remains neutral with regard to jurisdictional claims in published maps and institutional affiliations.
Supplementary Information
Below is the link to the electronic supplementary material.
Supplementary file1 (PDF 217 KB)
Rights and permissions.
Springer Nature or its licensor (e.g. a society or other partner) holds exclusive rights to this article under a publishing agreement with the author(s) or other rightsholder(s); author self-archiving of the accepted manuscript version of this article is solely governed by the terms of such publishing agreement and applicable law.
Reprints and permissions
About this article
Kim, S., Lee, CY. A computational model for sample dependence in hypothesis testing of genome data. J. Korean Phys. Soc. (2024). https://doi.org/10.1007/s40042-024-01100-z
Download citation
Received : 15 April 2024
Revised : 07 May 2024
Accepted : 16 May 2024
Published : 30 May 2024
DOI : https://doi.org/10.1007/s40042-024-01100-z
Share this article
Anyone you share the following link with will be able to read this content:
Sorry, a shareable link is not currently available for this article.
Provided by the Springer Nature SharedIt content-sharing initiative
- Sample dependence
- Hypothesis testing
- Adjusted sample size
- Find a journal
- Publish with us
- Track your research
Independent and Dependent Variables
Saul Mcleod, PhD
Editor-in-Chief for Simply Psychology
BSc (Hons) Psychology, MRes, PhD, University of Manchester
Saul Mcleod, PhD., is a qualified psychology teacher with over 18 years of experience in further and higher education. He has been published in peer-reviewed journals, including the Journal of Clinical Psychology.
Learn about our Editorial Process
Olivia Guy-Evans, MSc
Associate Editor for Simply Psychology
BSc (Hons) Psychology, MSc Psychology of Education
Olivia Guy-Evans is a writer and associate editor for Simply Psychology. She has previously worked in healthcare and educational sectors.
On This Page:
In research, a variable is any characteristic, number, or quantity that can be measured or counted in experimental investigations . One is called the dependent variable, and the other is the independent variable.
In research, the independent variable is manipulated to observe its effect, while the dependent variable is the measured outcome. Essentially, the independent variable is the presumed cause, and the dependent variable is the observed effect.
Variables provide the foundation for examining relationships, drawing conclusions, and making predictions in research studies.

Independent Variable
In psychology, the independent variable is the variable the experimenter manipulates or changes and is assumed to directly affect the dependent variable.
It’s considered the cause or factor that drives change, allowing psychologists to observe how it influences behavior, emotions, or other dependent variables in an experimental setting. Essentially, it’s the presumed cause in cause-and-effect relationships being studied.
For example, allocating participants to drug or placebo conditions (independent variable) to measure any changes in the intensity of their anxiety (dependent variable).
In a well-designed experimental study , the independent variable is the only important difference between the experimental (e.g., treatment) and control (e.g., placebo) groups.
By changing the independent variable and holding other factors constant, psychologists aim to determine if it causes a change in another variable, called the dependent variable.
For example, in a study investigating the effects of sleep on memory, the amount of sleep (e.g., 4 hours, 8 hours, 12 hours) would be the independent variable, as the researcher might manipulate or categorize it to see its impact on memory recall, which would be the dependent variable.
Dependent Variable
In psychology, the dependent variable is the variable being tested and measured in an experiment and is “dependent” on the independent variable.
In psychology, a dependent variable represents the outcome or results and can change based on the manipulations of the independent variable. Essentially, it’s the presumed effect in a cause-and-effect relationship being studied.
An example of a dependent variable is depression symptoms, which depend on the independent variable (type of therapy).
In an experiment, the researcher looks for the possible effect on the dependent variable that might be caused by changing the independent variable.
For instance, in a study examining the effects of a new study technique on exam performance, the technique would be the independent variable (as it is being introduced or manipulated), while the exam scores would be the dependent variable (as they represent the outcome of interest that’s being measured).
Examples in Research Studies
For example, we might change the type of information (e.g., organized or random) given to participants to see how this might affect the amount of information remembered.
In this example, the type of information is the independent variable (because it changes), and the amount of information remembered is the dependent variable (because this is being measured).
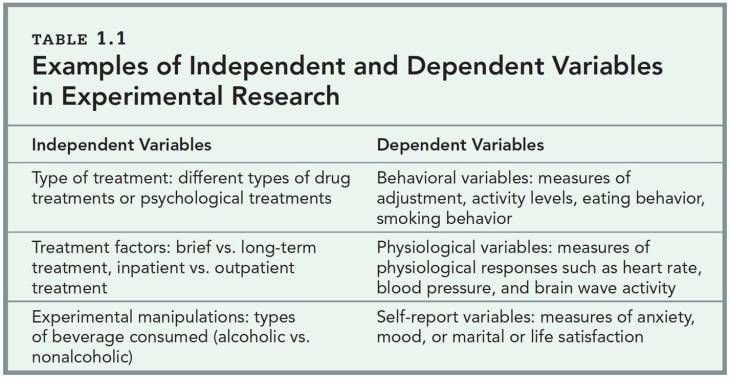
For the following hypotheses, name the IV and the DV.
1. Lack of sleep significantly affects learning in 10-year-old boys.
IV……………………………………………………
DV…………………………………………………..
2. Social class has a significant effect on IQ scores.
DV……………………………………………….…
3. Stressful experiences significantly increase the likelihood of headaches.
4. Time of day has a significant effect on alertness.
Operationalizing Variables
To ensure cause and effect are established, it is important that we identify exactly how the independent and dependent variables will be measured; this is known as operationalizing the variables.
Operational variables (or operationalizing definitions) refer to how you will define and measure a specific variable as it is used in your study. This enables another psychologist to replicate your research and is essential in establishing reliability (achieving consistency in the results).
For example, if we are concerned with the effect of media violence on aggression, then we need to be very clear about what we mean by the different terms. In this case, we must state what we mean by the terms “media violence” and “aggression” as we will study them.
Therefore, you could state that “media violence” is operationally defined (in your experiment) as ‘exposure to a 15-minute film showing scenes of physical assault’; “aggression” is operationally defined as ‘levels of electrical shocks administered to a second ‘participant’ in another room.
In another example, the hypothesis “Young participants will have significantly better memories than older participants” is not operationalized. How do we define “young,” “old,” or “memory”? “Participants aged between 16 – 30 will recall significantly more nouns from a list of twenty than participants aged between 55 – 70” is operationalized.
The key point here is that we have clarified what we mean by the terms as they were studied and measured in our experiment.
If we didn’t do this, it would be very difficult (if not impossible) to compare the findings of different studies to the same behavior.
Operationalization has the advantage of generally providing a clear and objective definition of even complex variables. It also makes it easier for other researchers to replicate a study and check for reliability .
For the following hypotheses, name the IV and the DV and operationalize both variables.
1. Women are more attracted to men without earrings than men with earrings.
I.V._____________________________________________________________
D.V. ____________________________________________________________
Operational definitions:
I.V. ____________________________________________________________
2. People learn more when they study in a quiet versus noisy place.
I.V. _________________________________________________________
D.V. ___________________________________________________________
3. People who exercise regularly sleep better at night.
Can there be more than one independent or dependent variable in a study?
Yes, it is possible to have more than one independent or dependent variable in a study.
In some studies, researchers may want to explore how multiple factors affect the outcome, so they include more than one independent variable.
Similarly, they may measure multiple things to see how they are influenced, resulting in multiple dependent variables. This allows for a more comprehensive understanding of the topic being studied.
What are some ethical considerations related to independent and dependent variables?
Ethical considerations related to independent and dependent variables involve treating participants fairly and protecting their rights.
Researchers must ensure that participants provide informed consent and that their privacy and confidentiality are respected. Additionally, it is important to avoid manipulating independent variables in ways that could cause harm or discomfort to participants.
Researchers should also consider the potential impact of their study on vulnerable populations and ensure that their methods are unbiased and free from discrimination.
Ethical guidelines help ensure that research is conducted responsibly and with respect for the well-being of the participants involved.
Can qualitative data have independent and dependent variables?
Yes, both quantitative and qualitative data can have independent and dependent variables.
In quantitative research, independent variables are usually measured numerically and manipulated to understand their impact on the dependent variable. In qualitative research, independent variables can be qualitative in nature, such as individual experiences, cultural factors, or social contexts, influencing the phenomenon of interest.
The dependent variable, in both cases, is what is being observed or studied to see how it changes in response to the independent variable.
So, regardless of the type of data, researchers analyze the relationship between independent and dependent variables to gain insights into their research questions.
Can the same variable be independent in one study and dependent in another?
Yes, the same variable can be independent in one study and dependent in another.
The classification of a variable as independent or dependent depends on how it is used within a specific study. In one study, a variable might be manipulated or controlled to see its effect on another variable, making it independent.
However, in a different study, that same variable might be the one being measured or observed to understand its relationship with another variable, making it dependent.
The role of a variable as independent or dependent can vary depending on the research question and study design.

Related Articles
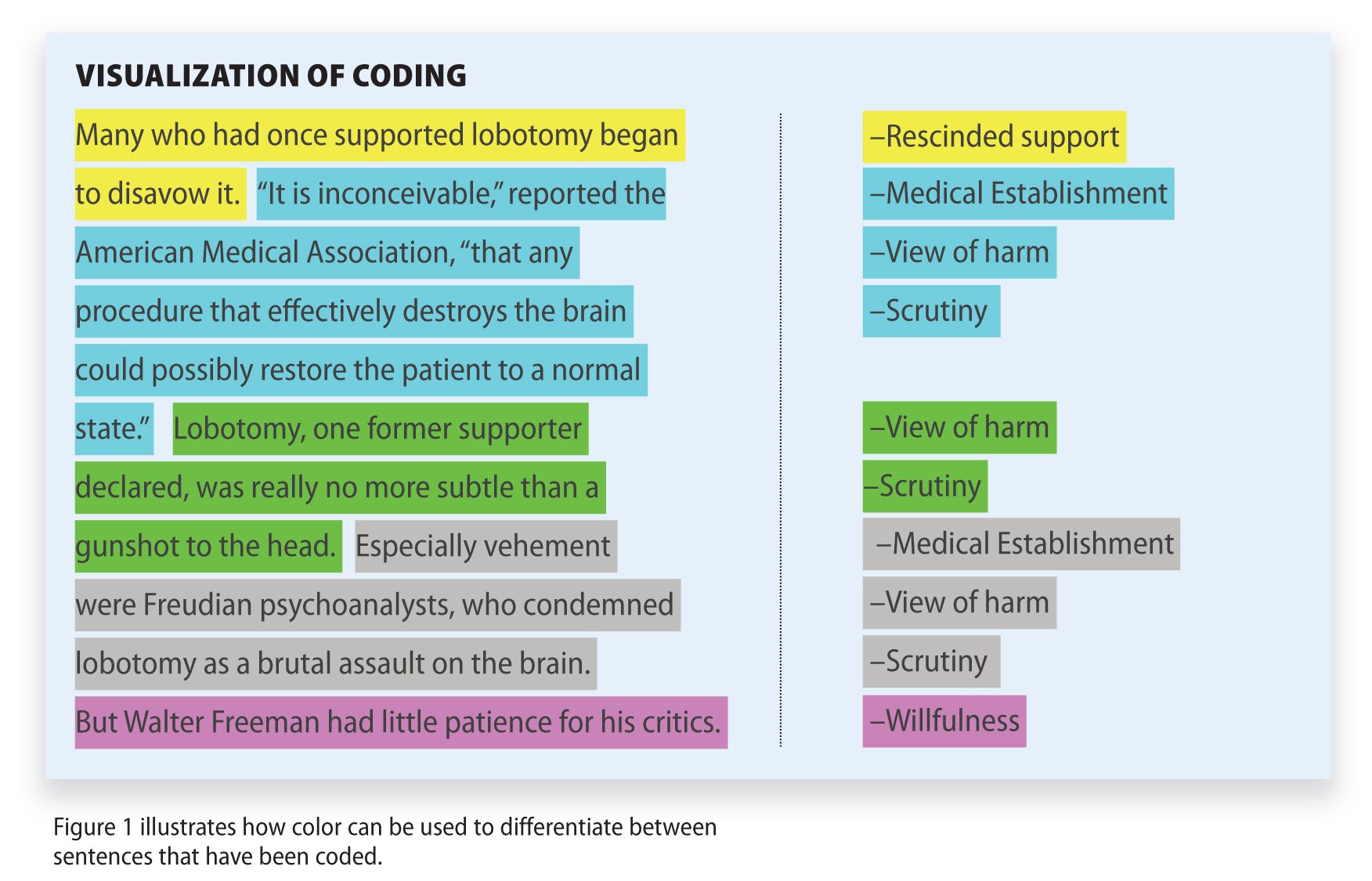
Research Methodology
Qualitative Data Coding
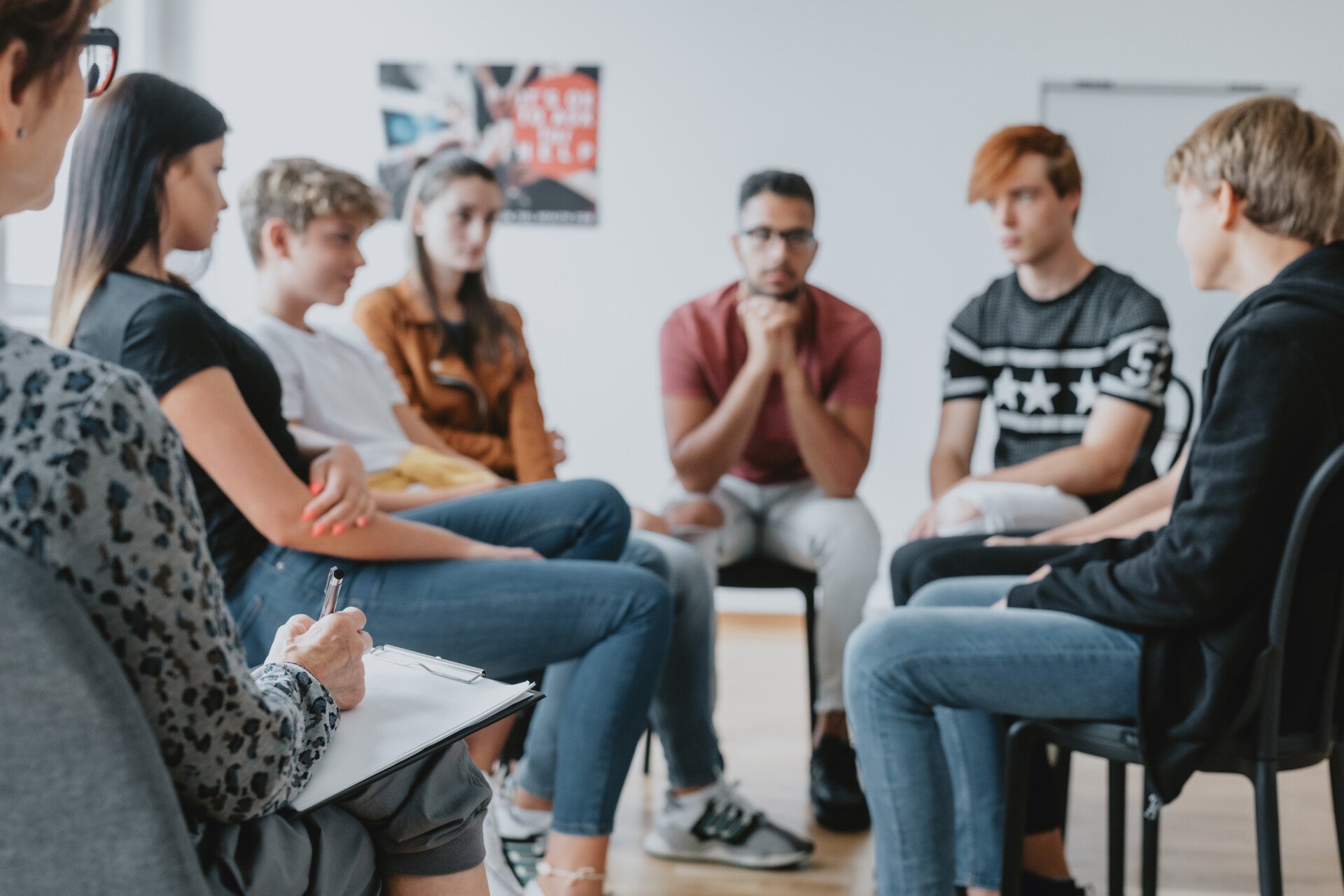
What Is a Focus Group?
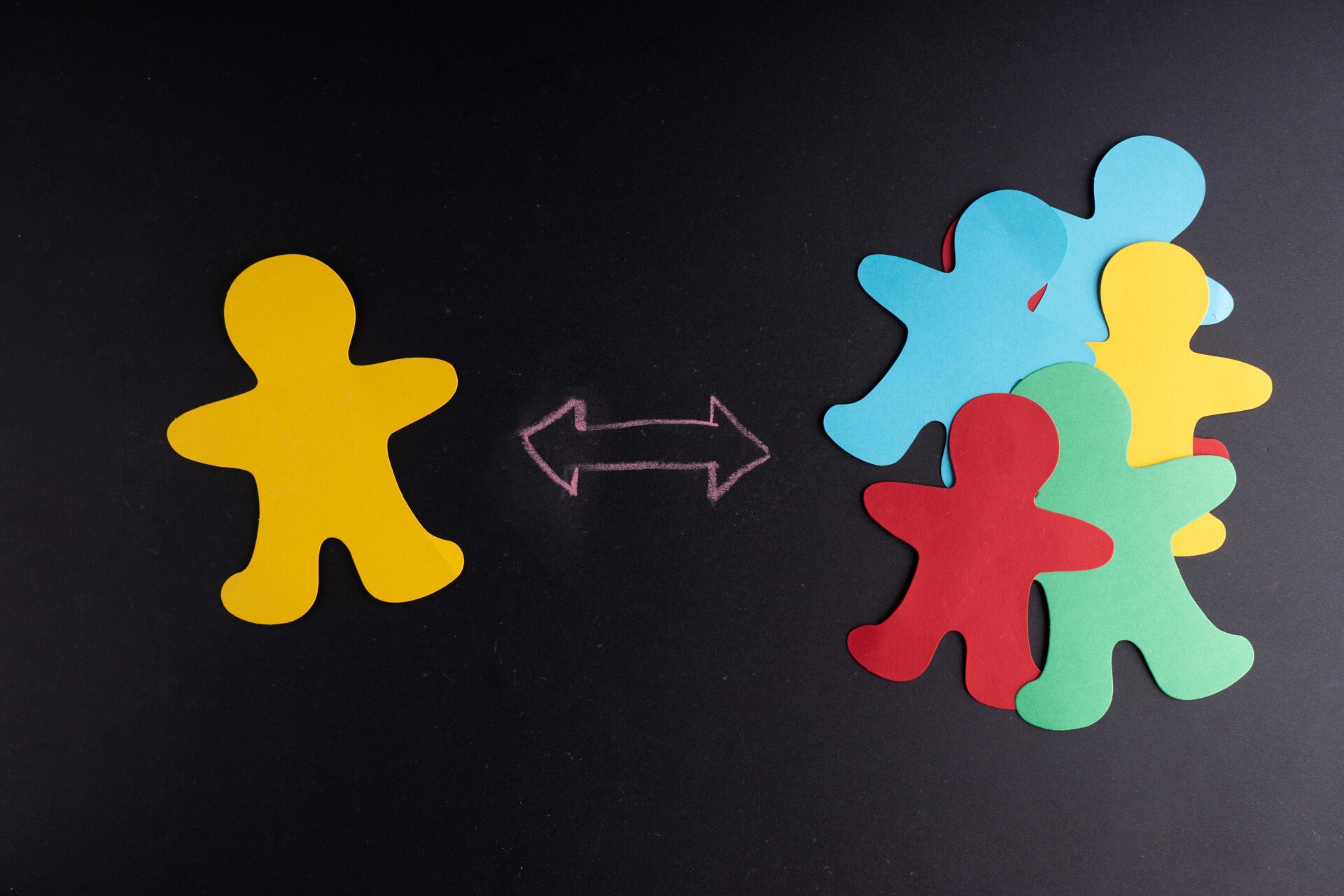
Cross-Cultural Research Methodology In Psychology
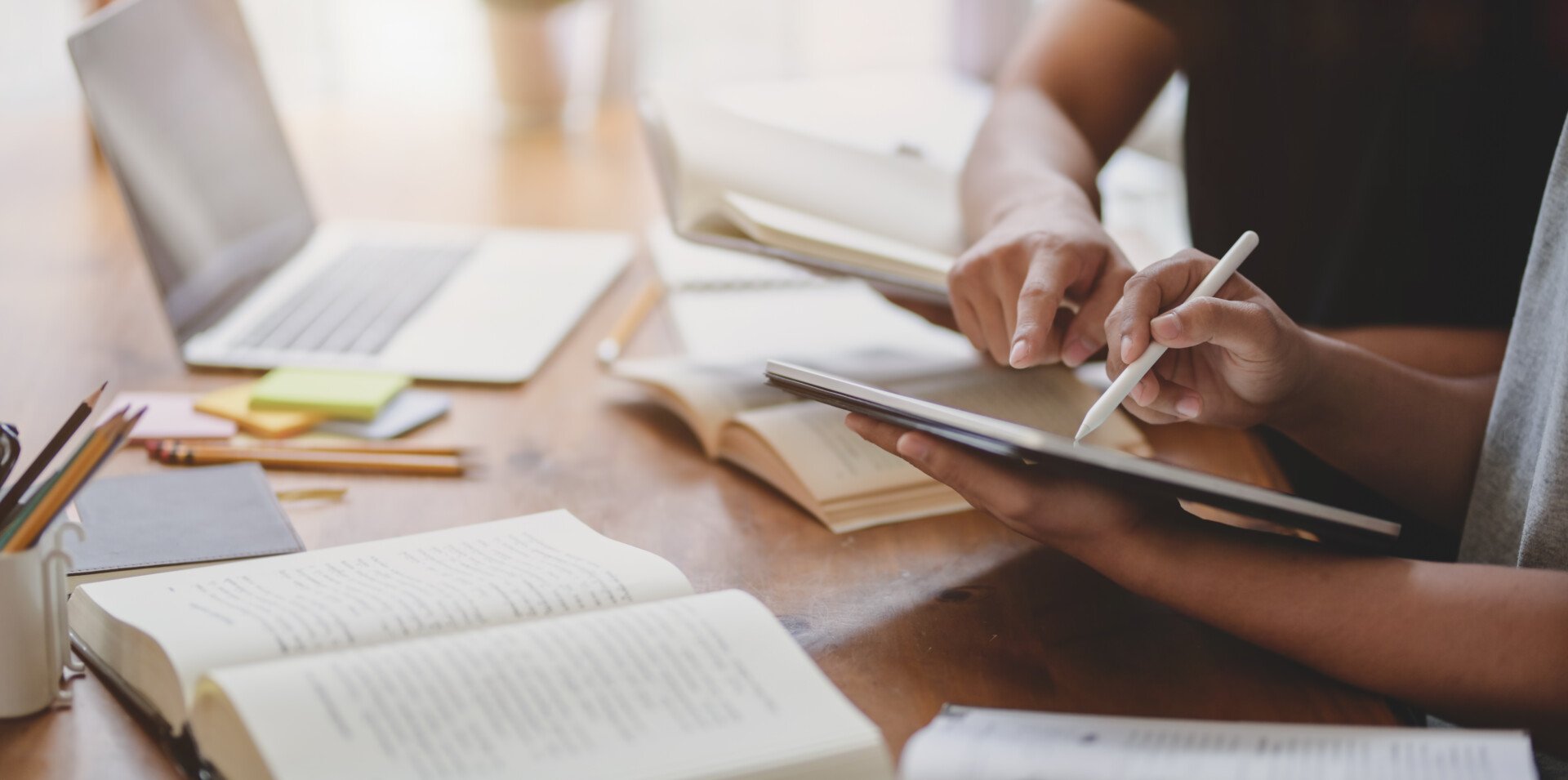
What Is Internal Validity In Research?
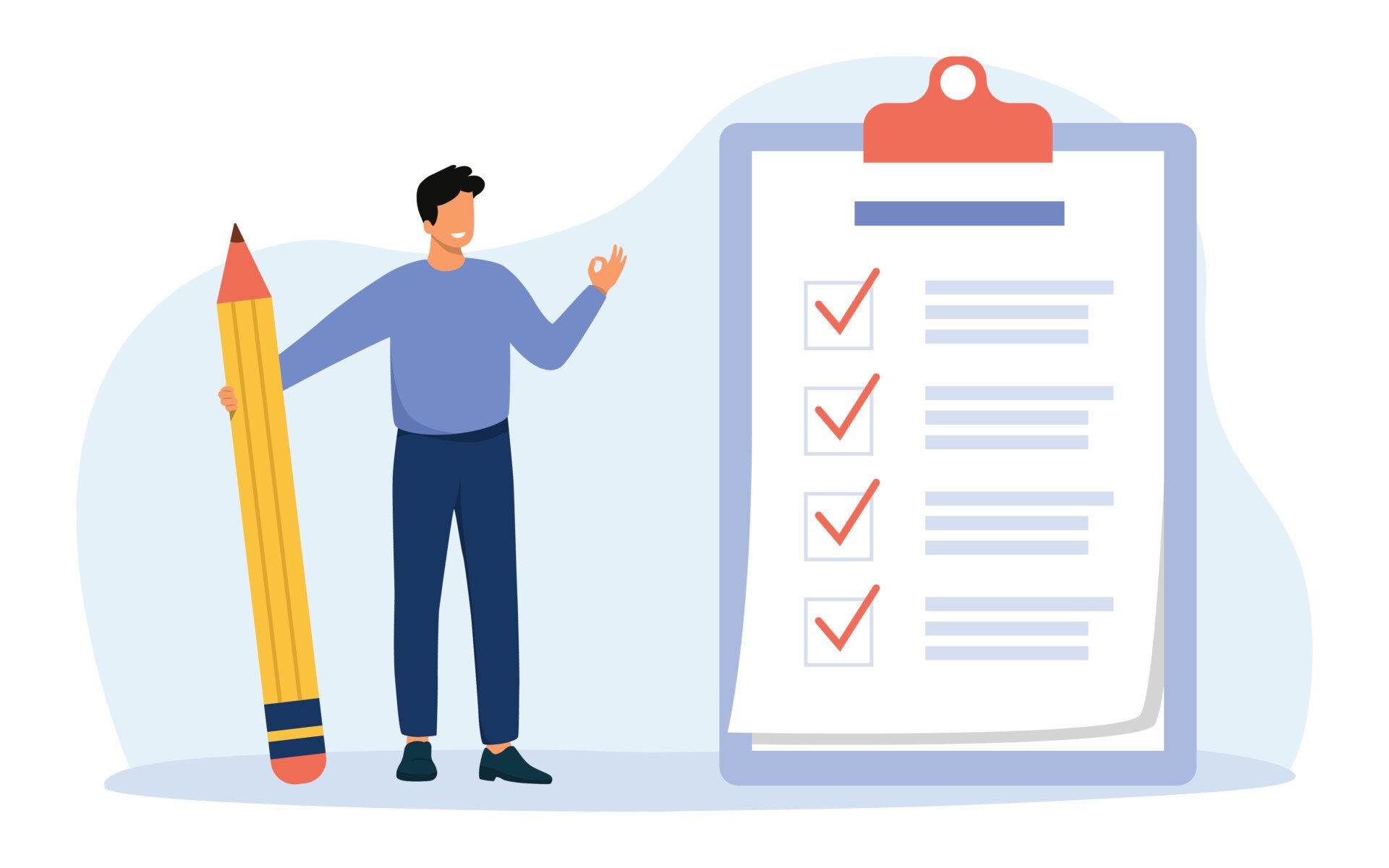
Research Methodology , Statistics
What Is Face Validity In Research? Importance & How To Measure
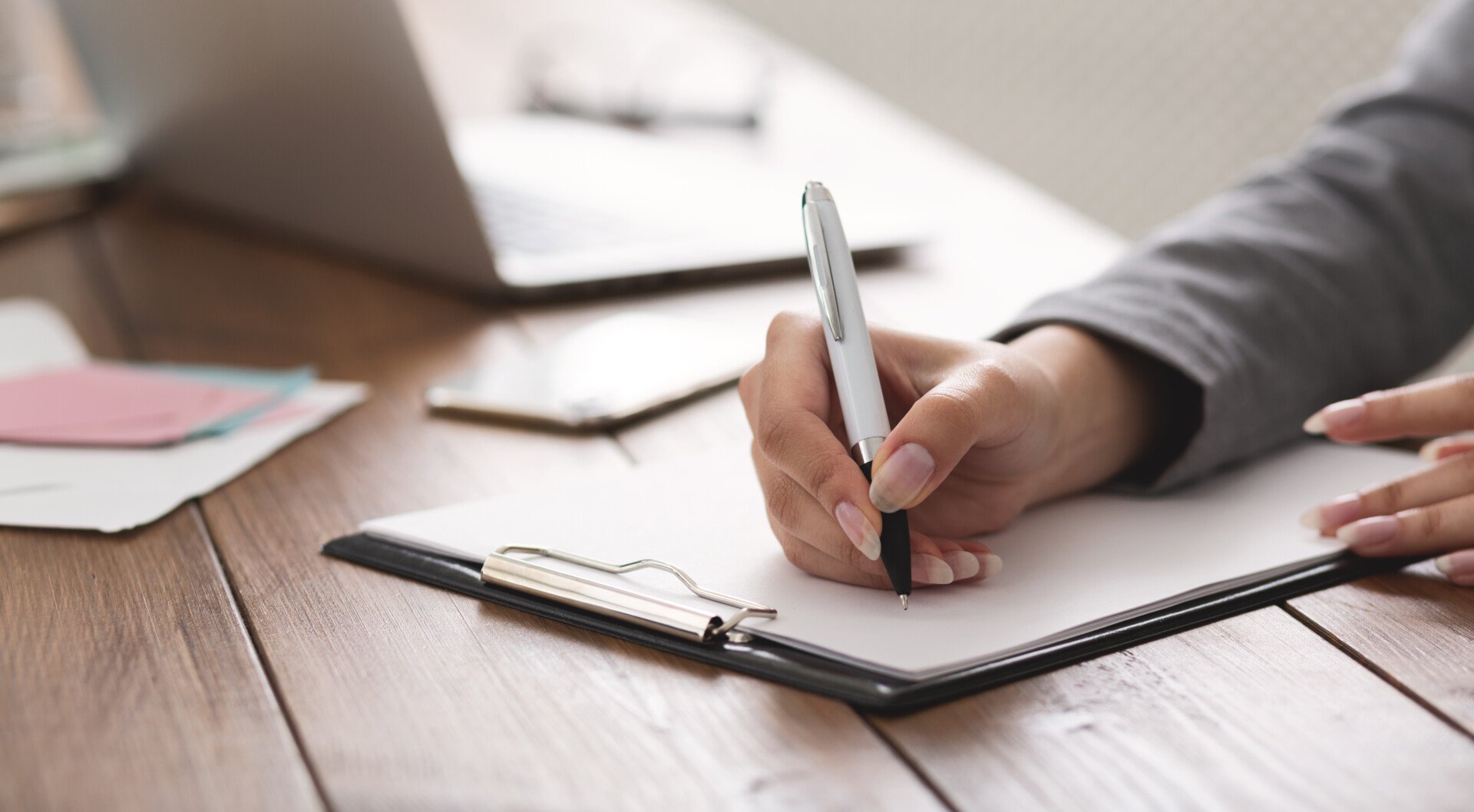
Criterion Validity: Definition & Examples

An official website of the United States government
The .gov means it’s official. Federal government websites often end in .gov or .mil. Before sharing sensitive information, make sure you’re on a federal government site.
The site is secure. The https:// ensures that you are connecting to the official website and that any information you provide is encrypted and transmitted securely.
- Publications
- Account settings
Preview improvements coming to the PMC website in October 2024. Learn More or Try it out now .
- Advanced Search
- Journal List
- Cell Mol Life Sci
- v.70(19); 2013 Oct
- PMC11113768

Marked by association: techniques for proximity-dependent labeling of proteins in eukaryotic cells
Kyle j. roux.
1 Children’s Health Research Center, Sanford Research/USD, North 60th St. East, Sioux Falls, SD 57104 USA
2 Department of Pediatrics, University of South Dakota School of Medicine, Sioux Falls, SD 57105 USA
Various methods have been established for the purpose of identifying and characterizing protein–protein interactions (PPIs). This diverse toolbox provides researchers with options to overcome challenges specific to the nature of the proteins under investigation. Among these techniques is a category based on proximity-dependent labeling of proteins in living cells. These can be further partitioned into either hypothesis-based or unbiased screening methods, each with its own advantages and limitations. Approaches in which proteins of interest are fused to either modifying enzymes or receptor sequences allow for hypothesis-based testing of protein proximity. Protein crosslinking and BioID (proximity-dependent biotin identification) permit unbiased screening of protein proximity for a protein of interest. Here, we evaluate these approaches and their applications in living eukaryotic cells.
Introduction
In the wake of the genome revolution, we are faced with the daunting task of revealing not just the identity but also the function of all those genes, or more specifically the function of the proteins they encode. Far more often than not these functions remain partially or completely unknown. One common approach to provide clues as to the function of a protein is to identify which other proteins it associates with. With this knowledge of PPIs in hand, one can often make testable hypotheses as to the nature of the protein under study. To take this relatively simple paradigm further, it is clear that proteins do not exist solely in simple complexes but in vast interconnected networks, which we are only beginning to uncover. It is the field of systems biology that is most in need of a confident understanding of PPIs. Unfortunately, we are far from reaching this goal given the vast complexity of PPIs that vary dramatically with variables including cell type, proliferation state, environmental influences or age. Thankfully, there have been considerable advances in the variety and sophistication of methods to study protein associations. With more sensitive mass spectrometry combined with computational advances, it has become commonplace to identify tens or even hundreds of protein candidates from single experiments. And approaches such as protein-fragment complementation (e.g., split-GFP or split-luciferase) and forster (or fluorescence) resonance energy transfer (FRET) have enabled real-time observation of protein proximity in live cells. No one single method reigns supreme, but each contributes unique advantages to the growing toolbox available to the study of PPIs and, ultimately, protein function.
There are a variety of fundamental approaches to investigate PPIs. Some rely on the in vitro maintenance of direct physical protein interactions that have occurred in vivo. Such methods range from simple co-immunoprecipitation, where antibodies are utilized to isolate protein complexes, to sophisticated tandem affinity complex purification in which fusion proteins containing dual affinity tags are utilized to isolate intact protein complexes. These approaches often take advantage of mass spectrometry to identify and even quantify the composition of protein complexes [ 1 ]. Other methods rely on the observation of events that are triggered in vivo by protein interactions or proximity. Yeast-2-hybrid (Y2H) is the most widely utilized of these methods and utilizes the expression of selectable reporter genes in response to protein proximity [ 2 ]. Other examples include split-luciferase or GFP assays that rely on the generation of functional reporter proteins from non-functional fragments fused to proteins that reside in close proximity. Proximity ligation assay combines fluorescent antibody-based protein labeling, PCR amplification and fluorescent in situ hybridization to assess the proximity of two proteins or epitopes in fixed cells [ 3 ].
Why proximity-dependent protein labeling?
Another approach to investigate PPIs whose methods have expanded in recent years is based on proximity-dependent labeling of proteins in living cells. Proximity-dependent protein labeling refers to any general method to investigate protein proximity by specifically labeling proteins based on their proximity to each other. For the purpose of this discussion, labeling refers to a covalent modification of the protein. Intrinsic to these approaches is a fundamental limitation, namely that most by their very nature do not directly test for physical interactions between proteins. Instead, the presence of this labeling implies a spatial proximity that can be used to provide candidate protein interactors. These candidate interactions should be tested with other more direct methods if they are to be designated as genuine physical PPIs. Detection of PPIs is not the only useful application of these methods. The ability to observe protein proximity can provide valuable information about protein dynamics, allow the monitoring of post-translation modifications within a subpopulation of proteins, and can be used to reveal the constituency of discrete cellular structures. Some of these methods can also be applied to investigate intra-molecular proximity. To derive physiologically relevant information, techniques in proximity-dependent labeling are most useful when applied in living cells. Therefore, this review focuses on applications in living eukaryotic cells.
Variations on a theme: hypothesis-driven pursuit of protein interactions
Hypothesis-based analysis of PPIs occurs when specific pre-defined PPIs are investigated. The identities of these proteins must already be known a priori, and thus necessitates a pre-existing rationale to suspect potential interaction. Examples of such methods include common techniques such as FRET and protein-fragment complementation assays for which genetically engineered fusion proteins must be generated to test the interaction. Of course, these same technical principles can also be applied to non-hypothesis-based screens that use the power of genetics, for instance the protein-fragment complementation-based Y2H, to test large numbers of interactions. Advantages of the hypothesis-based approach, as compared to screening (often called ‘fishing’), include a wide selection of specific methods to choose from and the flexibility to test them in a variety of conditions, since the experiments are traditionally far simpler to perform than a large screen. Disadvantages include the obvious limitation of testing often binary interactions where the identity of the proteins must be known and the bias that this generates for the investigator to generate a positive interaction.
Among hypothesis-based methods of proximity-dependent protein labeling, there are a handful of examples. Most of these are variants of the BirA/BioTag system that harnesses BirA, the biotin ligase from E. coli . BirA is a dual function protein that in E. coli serves both to transcriptionally regulate the biotin synthetic operon as well as to biotinylate a subunit of the acetyl-coA carboxylase [ 4 ]. It is the latter function that has proven methodologically useful for protein isolation and analysis. Small biotin acceptor tags (BATs) have been designed such that they contain a lysine specifically biotinylated by BirA [ 5 , 6 ]. Fusion proteins containing these BATs are co-expressed with BirA, often in eukaryotic cells, leading to the specific biotinylation of the fusion protein. As biotinylation is a relatively rare protein modification and is amenable to high-affinity capture with avidin/streptavidin, this system permits robust enrichment of the fusion protein for protein complex purification or chromatin immunoprecipitation. To accomplish hypothesis-based proximity-dependent protein labeling, the Ting group pioneered the use of a simple modification of the BioTag system in which BirA was fused to one protein of interest and co-expressed with a BAT-fusion protein [ 7 ]. Thus, biotinylation can only occur if both proteins are in close physical proximity (Fig. 1 ). Modifications to the BAT tag were made to reduce its affinity to BirA to avoid stabilizing the interaction or generation of an artificial interaction. This approach is conducive to monitoring interactions by both fluorescence microscopy and western blot analysis of protein biotinylation. In proof-of-principle experiments, which utilized the rapamycin-mediated interaction of FRB and FKBP as well as the interaction between Cdc25C and 14.3.3ε as positive controls, labeling was induced by cellular incubation with excess biotin for periods as short as 1 min. A variant of this system, named PUB-MS (proximity utilizing biotinylation and mass spectrometry) and developed by the Ogryzko group, used modified BAT sequences to facilitate mass spectrometry-based analysis [ 8 ]. Several PPI models were used to test PUB-MS including self-oligomerizing proteins such as TAP54α and HP1γ as well as the characterized binary interaction of KAP1 and HP1γ. Advantages of PUB-MS include an ability to utilize variable BAT sequences that theoretically permit evaluation of multiplex interactions. The Ogryzko group also utilized a pulse-chase approach, capitalizing on the permanence of the biotinylation, to monitor the fate of labeled proteins over a period of time [ 8 ]. This advantageous application likely extends to any of the proximity-dependent methods that permanently modify proteins in living cells.

Hypothesis-based methods for proximity dependent protein labeling. These methods rely on the co-expression of two fusion proteins, one fused to a ligase (Protein A) and the other to an acceptor peptide (Protein B). To test for an interaction between Protein A and Protein B the fusion proteins are co-expressed and labeling of protein B is evaluated. Ligases include BirA and LplA that specifically label an acceptor peptide sequence. The label is either biotin for BirA or a coumarin fluorophor for LplA. When the BirA, BAT combination is applied to cell surface proteins expressed in distinct cell populations for intercellular labeling this method is termed BLINC. The BirA/BAT, PUB-MS and BLINC methods utilize the BirA/BAT combination, whereas ID-PRIME uses the LplA/LAP combination. Both ID-PRIME and BLINC permit imaging of protein proximity in populations of live cells
A fundamentally similar approach also generated by the Ting group is termed BLINC (biotin labeling of intercellular contacts) [ 9 ]. BLINC is designed to monitor protein interactions at the cell surface and can be applied intercellularly (Fig. 1 ). Specifically, these experiments used the BirA and BAT system fused to cell surface receptors neurexin and neuroligin found at synaptic junctions of rat hippocampal neurons. One cell population expressed the BirA-fusion protein and the other the BAT-fusion protein. Upon cell contact and in the presence of biotinoyl-AMP, or biotin and ATP, the BAT-fusion protein can be covalently labeled with biotin permitting visual identification of the interaction with the BirA-fusion protein on the other cell. BLINC does not trap interactions and permits spatiotemporal assessment of protein interactions on the surface of live cells. Drawbacks to the method include the irreversibility of the biotin visualization due to the high affinity of biotin to streptavidin and the time required to visualize the biotinylation with streptavidin may be slower than the internalization rate of the biotinylated proteins. This means that not all of the biotinylated proteins may be observed and not all of the proteins that are biotinylated are actively interacting with the partner protein. However, BLINC provides a powerful tool to monitor dynamic protein interactions at the cell surface in live cells.
The Ogryzko group recently reported a modified version of PUB-MS called PUB-NChIP (proximity utilizing biotinylation with native chromatin immuno-precipitation; ChIP) in which a nuclear protein of interest is fused to BirA and co-expressed with a BAT-tagged histone [ 10 ]. As initially applied, the BAT was fused to specific histones and the ligase to Rad18, an E3 ubiquitin protein ligase associated with DNA repair. The net effect of PUB-NChIP is the biotinylation of specific histones associated with the protein of interest. This labeling permits the isolation of DNA associated with the subpopulation of histones proximate to the protein of interest. This method, similar to native ChIP [ 11 ], avoids the crosslinking necessary for conventional ChIP since core histones are relatively tightly associated with DNA. This preserves the ability to analyze posttranslational modifications on the histones since lysines are the most frequent targets of crosslinking with ChIP and also common sites of posttranslational modification. Other advantages of PUB-NChIP include the ability to utilize histone variants associated with specific functional states (e.g., active or repressed chromatin), the potential to observe post-translational modification of the labeled population and the capacity to perform pulse-chase experiments to monitor protein turnover. It is worth noting that these last two advantages extend beyond the simple purpose of detecting PPIs and may apply to other methods of proximity-dependent labeling such as BioID. One potential caveat with PUB-NChIP is the potential presence of naturally biotinylated histones [ 12 ]; however, these appear to exist in such extremely low abundance that they are unlikely to significantly impact the results and can easily be controlled for.
A technique called ID-PRIME (interaction-dependent probe incorporation mediated by enzymes), also developed by the Ting group, was developed to permit imaging of protein–protein interactions inside living cells [ 13 ]. ID-PRIME capitalizes on a mutant form of the E. coli lipoic acid ligase, LplA W37V , to specifically attach a coumarin fluorophore to an LplA acceptor peptide (LAP). In principle, this approach is similar to the BirA-BAT system in that the ligase and acceptor peptide are independently fused to proteins chosen in a hypothesis-based manner and these are co-expressed in cells. Where ID-PRIME differs is by providing a method to visualize evidence of past or current interactions in living cells. Since the cells are loaded for a period of ~10 min and then unloaded to clear the unattached fluorophore for 30–50 min, what is observed is evidence of past interactions that may or may not remain. This system was tested with a multiple positive controls, including rapamycin-mediated interaction of FRB and FKBP and the leucine zipper domains of Jun and Fos. Although not a real-time observation of protein proximity, as is possible with FRET for example, ID-PRIME does permit a modest temporospatial analysis of protein proximity that suggests the evidence of a PPI.
These methods all share a need to express at least two fusion proteins, one containing a modifying enzyme and the other with the receptor for that modification. As compared to the size of GFP, a commonly accepted fusion protein that permits fluorescence-based imaging, both BirA and LplA are larger. At their largest dimension, GFP is ~4.6 nm (27 kDa) [ 14 ], BirA is ~7.0 nm (35 kDa) [ 15 ], and LplA is ~7.5 nm (38 kDa) [ 16 ]. This added bulk may impart undesired targeting or stability issues and, depending on placement in the fusion protein, may interfere with the normal protein function and interactions due to steric hindrance. An additional complication is that all of these methods rely on the proximity of the enzyme with the acceptor protein. The spatial configuration needed for successful labeling remains unclear, but is likely to vary on a case-by-case basis and will depend on factors such as the relative orientation of proteins to each other, accessibility of the acceptor sequences, and the presence, length and flexibility of any linker sequences between the enzyme or receptor and the bait. It is not difficult to imagine situations where sizeable proteins may interact, but, depending on the placement of the enzyme and acceptor tag, no labeling would occur. Such false negatives must always be considered when using these approaches. The main advantage of these approaches is that, by the covalent modification of one of the candidates, they can generate a permanent mark of protein proximity in live cells. This mark can be used to monitor distribution, natural post-translational modifications, and fate of these proteins.
Fishing for friends with proximity-dependent labeling
Often, researchers cannot rely on the hypothesis-based method to test PPIs. Sometimes, there is a need for methods to detect interactions that are not already known or surmised. These fall into the broad category known as fishing or screening, in which the bait is defined but the identities of the protein interactors (prey) are unknown. In some instances, such as Y2H, there are large libraries of prey that are screened for an interaction with the bait. Other methods attempt to probe PPIs that have occurred with endogenous prey under physiological conditions. The most common of these approaches is affinity complex purification. In this situation, prey are identified by bottom-up mass spectrometry. It is advances in bottom-up mass spectrometry that have most dramatically facilitated these PPI fishing expeditions. There are two fundamentally different methods to fish for candidate interactions in vivo that both rely on proximity-dependent labeling, protein crosslinking, and BioID.
Protein crosslinking: proximity-dependent tethering
Crosslinking is by far the most utilized method of proximity-dependent protein labeling and a detailed evaluation is beyond the scope of this review, but has been well covered elsewhere [ 17 – 24 ]. For the purposes of detecting PPIs, crosslinking is essentially a variant of affinity complex purification that seeks to overcome the loss of interactions during solubilization of the protein complexes. This is accomplished by the covalent crosslinking of proximate proteins (or other molecules) while the cells are intact and essentially alive (Fig. 2 ). A variety of reagents can be used to obtain this physical crosslinking, but all are based on the use of molecules with at least two reactive groups that serve to tether proteins to their neighbors. Following this crosslinking procedure, the approach follows that of affinity complex purification in which a protein is isolated via some specific property (e.g., an affinity purification domain or epitope) and the proteins to which it is physically tethered can be identified by mass spectrometry. Goals of chemical crosslinking in proteomics are twofold: the most common one is identification of constituents in protein complexes while the other is to map interface sites between or within proteins. It is this latter goal that makes chemical crosslinking unique in its potential to step beyond the simple identification of protein complex constituents. This relies on the detection of specific sites where inter- or intra-molecular links have occurred. These linked peptides signify a site where those sequences are in close proximity and can be used to identify actual interfaces of PPIs or potentially intra-molecular interfaces within a protein. This application of crosslinking is more comprehensively covered elsewhere [ 25 – 30 ].

Overview of protein crosslinking to identify PPIs and/or map protein interfaces. Live cells are treated with a crosslinking reagent to covalently connect proximate proteins. These fixed cells are lysed and crosslinked protein complexes are released into solution for affinity purification of the desired complex(es). The purified complex(es) is digested into peptide fragments which are analyzed by mass spectrometry to identify the constituents of the complex and/or by using inter- or intra-crosslinked peptides to determine the structure and/or interfaces of the proteins in the complex
There are numerous reagents spanning distances ranging from <3–40 Å that can be utilized for chemical crosslinking. These include formaldehyde, NHS esters, carbodiimides and benzophenones. Reactions can occur through a variety of chemistries to sites within proteins such as side chains or the N-termini. Some of these reagents can be photoactivated to permit the user to control the timing of the reaction by exposing samples to UV light. More sophisticated approaches include the incorporation of unnatural amino acids with photo-reactive side chains to covalently trap proximate proteins [ 31 ]. Another such method uses protein interaction reporters (PIR) that contain an affinity motif and can be cleaved in a two-step process by low-energy MS [ 32 ]. The first step is to identify the ions with crosslinks and their nature (intra- or inter-molecular) and the second is to release the individual peptides for identification.
TRAP-crosslinking (targeted releasable affinity probe) is a notable method that limits protein crosslinking to only to one specific protein of interest [ 33 ]. This approach generated by the Mayer laboratory is based on the fusion of a cell permeable photo-activatable benzophenone crosslinker to a fluorescent biarsenical probe (the TRAP probe) that binds to engineered tetracysteine motifs within the protein of interest. A fusion protein is expressed in vivo that contains one or more of these short tetracysteine motifs. The TRAP probe can be added to the cells to fluorescently label the proteins and, when photo-activated, to crosslink proximate proteins. Finally, with the use of dithiols to break the association with the tetracysteine tag, the fluorescent probe can be released from the protein of interest where it remains on the interacting protein to facilitate identification of the proteins, and potentially the specific binding site, by mass spectrometry. TRAP was validated in vitro and in vivo in a prokaryotic system and applied to cultured myocytes to identify an interaction between phospholamban and fibronectin. Clearly, the main advantage of TRAP is its ability to focus only on the protein of interest among the complex mixture of proteins found in living cells. Advantages include ability to release the biarsenical fluorophore from the protein of interest with high concentrations of dithiols. Potential limitations include palmitoylation and oxidation of the tetracysteine motif [ 34 ], and uncertainty of consequences to normal protein behavior during the period of photoactivation (~2 h). In principle, this approach has the potential to provide a powerful tool to fish for proximate proteins in a physiological setting while avoiding many of the drawbacks associated with conventional crosslinking.
For the purposes of proximity-dependent labeling, protein crosslinking typically relies on affinity capture of the crosslinked proteins. Highly mobile proteins that engage in transient interactions would require different crosslinking conditions than immobile proteins in a large complex or protein matrix. If the protein of interest has subpopulations with variable properties, it is difficult to imagine optimizing the crosslinking conditions to capture relevant interactions that span all subpopulations. Successful crosslinking is a delicate balance, with concentrations of crosslinking reagents and duration of crosslinking affecting the outcome. Too much crosslinking can lead to protein ‘loss’ due to fixation in large insoluble complexes, whereas too little reduces the capture of weak and transient interactions. There also exists the possibility of masking epitopes or otherwise interfering with affinity complex purification. Ideally, the net effect of such crosslinking is akin to taking a snapshot of interactions at the time of fixation. As such, crosslinking excels at the identification and potentially the structural characterization of relatively discrete and stable protein complexes. Where it faces limitations is with transient interactions, especially those that occur in low abundance. For example, if only 1 % of a kinase (the bait) interacts with a specific substrate (the prey) at any one point in time it may be difficult to identify that kinase–substrate interaction with conventional crosslinking, especially if the bait is a protein of relatively low abundance.
BioID: following the protein footprints
Another method of screening or ‘fishing’ for protein interactions has recently been reported [ 35 ]. Called BioID (proximity dependent biotin identification), this approach capitalizes on a mutant prokaryotic biotin ligase capable of promiscuous biotinylation [ 36 , 37 ]. When expressed in cells, the BioID fusion protein biotinylates proximate proteins, permitting their selective isolation with avidin/streptavidin-based biotin affinity capture (Fig. 3 ). These proteins can be identified by immunoblot analysis if hypothesis-based interactions are being investigated or by mass spectrometry if ‘fishing’ for candidates. BioID generates a history of protein footprints that occurred over a period of time in a relatively natural cellular setting. In this way, BioID is very different from crosslinking approaches that capture the snapshot of protein proximity. In theory, transient interactions will accumulate biotin labeling over time leading to an increased chance of identification with BioID as compared to crosslinking.

Outline of the BioID method. A BioID-fusion protein, consisting of a bait protein fused to a promiscuous biotin ligase, is expressed in cells. Biotin is added to the cells for a period of labeling, during which time proteins proximate to the BioID-fusion protein are biotinylated. The cells are subsequently lysed, all of the proteins are denatured and the biotinylated proteins are selectively isolated for identification by mass spectrometry. The identified proteins are those proteins that were proximate to the bait during the labeling period and thus represent candidates PPIs
Since biotinylation is a covalent modification, biotin–avidin interactions are nearly covalent [ 38 ] and there is no need to maintain protein–protein interactions, the proteins can be solubilized and affinity captured under extremely stringent conditions. An obvious benefit of this nearly covalent affinity capture is the ability to apply extremely stringent wash conditions (including buffers containing 2 % SDS or 500 mM NaCl) that remove many contaminants typically detected with affinity complex purification. Another advantage of BioID is its applicability to insoluble proteins (e.g., intermediate filaments or integral membrane proteins) that are traditionally difficult to study by conventional affinity complex purification or Y2H.
Supplemental biotin in the cell media is necessary for robust biotinylation by the BioID fusion protein, perhaps due to a reduced affinity of the mutant ligase to biotin and/or the limited levels founds in tissue culture media. This requirement for excess biotin provides a mechanism to temporally regulate the biotinylation process to meet the experimental needs. Based on the initial reported use of BioID, it appears that biotinylation is saturated prior to 24 h, whereas biotinylation becomes noticeably detected above background within 1 h [ 35 ]. These times may be sufficient for temporally restricted experiments such as selective observation of cell-cycle-specific protein behavior.
So far, there have only been two published applications of BioID. In the first, a constituent of the nuclear lamina was fused to BioID (BioID-lamin A) and expressed in human cells [ 35 ]. Over 100 protein candidates were identified and ranked according to abundance. The majority of those candidates fit with what is known about the protein constituency of the nuclear lamina. Also identified were several uncharacterized proteins, the most abundant of which was shown to predominantly reside at the nuclear envelope. This protein, named SLAP75, represents one of a very few non-lamin proteins known to specifically localize to the nuclear envelope despite the lack of a transmembrane domain. This may reflect the predicted strength of BioID to identify weak and/or transient PPIs often missed by other methods. The second application of BioID was in the unicellular eukaryote, Typanosoma brucei [ 39 ]. To identify novel constituents of the discrete insoluble cytoskeletal structure called the bilobe, Morriswood et al. fused BioID to TbMORN1, one of the few known bilobe proteins. By analyzing insoluble proteins biotinylated by BioID–TbMORN1, seven novel bilobe proteins and two novel flagellar attachment proteins were identified. These results demonstrate the utility of BioID in identifying protein constituents of discrete intractable cellular structures and its versatility in application within divergent model systems.
BioID is not without its drawbacks and limitations [ 40 ]. There is the obvious need to express at least low levels of an exogenous fusion protein. As mentioned above, the BirA enzyme does add to the size of the protein and could compromise its targeting and/or function. During the biotinylation process, the irreversible covalent modification of primary amines may impact the function of labeled proteins, at least by blocking biotinylated sites (which include lysines) from alternative modifications. Another limitation of BioID relates to how the results can be interpreted. In theory, false negatives could arise from proteins without proximate reactive primary amines. Positive candidates do not prove direct interaction with the BioID fusion protein. Labeled candidates may instead reside in close proximity to the fusion protein, but not physically interact. This is the result of BioID’s mechanism of promiscuous biotinylation. The wild-type BirA uses biotin and ATP to generate biotinoyl-AMP [ 41 ]. BirA holds on to that reactive biotin molecule until it is covalently attached to a very specific substrate. The mutant BirA (R118G) used in BioID has reduced affinity to biotinoyl-AMP [ 42 ] and is thought to prematurely release the reactive biotin molecule [ 36 , 37 ]. It is this reactive biotin that labels proximate proteins. What remains unclear is the extent to which the reactive biotin can diffuse away from the ligase before reacting with a protein or being otherwise hydrolyzed. In vitro, a similar adenylate is reported to be relatively stable (nonenzymatic hydrolysis rate ~0.7 × 10 −3 per second) implying a potential for distal labeling [ 43 ]. The hydrolysis rate of biotinoyl-AMP in vivo remains unknown; however, it is likely to be rapid due in part to the high concentration of reactive sites (e.g., reactive primary amines on proteins) in a biological setting. By interpreting the proteomic data from BioID-LaA, a labeling distance of <20 nm has been proposed [ 35 ]; however, this is based on only one report and may vary with each application and cellular environment. Future studies will be needed to resolve the practical range of biotinylation by BioID. To facilitate successful application of BioID, a detailed protocol that includes extensive analysis of its strengths and limitations has been published [ 40 ].
Conclusions
Techniques involving proximity-dependent labeling do not currently represent commonly utilized ‘front-line’ approaches to detect and monitor PPIs. With the exception of protein crosslinking, none have been used more than once or twice in the literature, and are often presented in a proof-of-principle format. It remains to be seen whether any of the hypothesis-based techniques reach utilization levels similar to the more widespread approaches such as FRET or protein-fragment complementation. And although certainly more established, techniques in protein crosslinking face challenges in widespread acceptance, simplicity of use, and data interpretation, especially for the more sophisticated variants. As the newest member of this group, the fate of BioID remains unclear. Further studies are needed to resolve issues surrounding the spatial dimensions of labeling that impact data interpretation and provide a clearer picture as to the strengths and limitation of BioID. Hopefully, the same vision and creativity that led to the inception of these methods will ultimately lead to their appropriate adoption and creative use by the broader scientific community.
Acknowledgment
This work was supported by Sanford Research.
Non-Standard Abbreviations
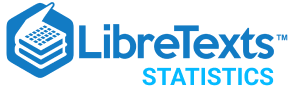
- school Campus Bookshelves
- menu_book Bookshelves
- perm_media Learning Objects
- login Login
- how_to_reg Request Instructor Account
- hub Instructor Commons
Margin Size
- Download Page (PDF)
- Download Full Book (PDF)
- Periodic Table
- Physics Constants
Scientific Calculator
- Reference & Cite
- Tools expand_more
- Readability
selected template will load here
This action is not available.
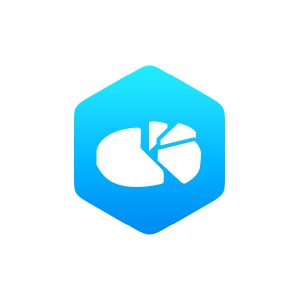
34: Hypothesis Test and Confidence Interval Calculator for Two Dependent Samples
- Last updated
- Save as PDF
- Page ID 8622
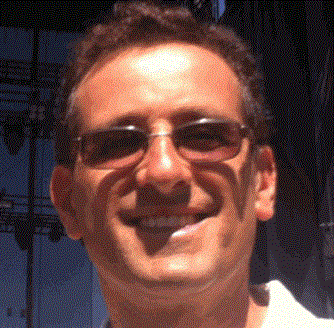
- Larry Green
- Lake Tahoe Community College
\( \newcommand{\vecs}[1]{\overset { \scriptstyle \rightharpoonup} {\mathbf{#1}} } \)
\( \newcommand{\vecd}[1]{\overset{-\!-\!\rightharpoonup}{\vphantom{a}\smash {#1}}} \)
\( \newcommand{\id}{\mathrm{id}}\) \( \newcommand{\Span}{\mathrm{span}}\)
( \newcommand{\kernel}{\mathrm{null}\,}\) \( \newcommand{\range}{\mathrm{range}\,}\)
\( \newcommand{\RealPart}{\mathrm{Re}}\) \( \newcommand{\ImaginaryPart}{\mathrm{Im}}\)
\( \newcommand{\Argument}{\mathrm{Arg}}\) \( \newcommand{\norm}[1]{\| #1 \|}\)
\( \newcommand{\inner}[2]{\langle #1, #2 \rangle}\)
\( \newcommand{\Span}{\mathrm{span}}\)
\( \newcommand{\id}{\mathrm{id}}\)
\( \newcommand{\kernel}{\mathrm{null}\,}\)
\( \newcommand{\range}{\mathrm{range}\,}\)
\( \newcommand{\RealPart}{\mathrm{Re}}\)
\( \newcommand{\ImaginaryPart}{\mathrm{Im}}\)
\( \newcommand{\Argument}{\mathrm{Arg}}\)
\( \newcommand{\norm}[1]{\| #1 \|}\)
\( \newcommand{\Span}{\mathrm{span}}\) \( \newcommand{\AA}{\unicode[.8,0]{x212B}}\)
\( \newcommand{\vectorA}[1]{\vec{#1}} % arrow\)
\( \newcommand{\vectorAt}[1]{\vec{\text{#1}}} % arrow\)
\( \newcommand{\vectorB}[1]{\overset { \scriptstyle \rightharpoonup} {\mathbf{#1}} } \)
\( \newcommand{\vectorC}[1]{\textbf{#1}} \)
\( \newcommand{\vectorD}[1]{\overrightarrow{#1}} \)
\( \newcommand{\vectorDt}[1]{\overrightarrow{\text{#1}}} \)
\( \newcommand{\vectE}[1]{\overset{-\!-\!\rightharpoonup}{\vphantom{a}\smash{\mathbf {#1}}}} \)
Two dependent Samples with data Calculator
Type in the values from the two data sets separated by commas, for example, 2,4,5,8,11,2. Then enter the tail type and the confidence level and hit Calculate and the test statistic, t, the p-value, p, the confidence interval's lower bound, LB, the upper bound, UB, and the data set of the differences will be shown. Be sure to enter the confidence level as a decimal, e.g., 95% has a CL of 0.95.
Back to the Calculator Menu
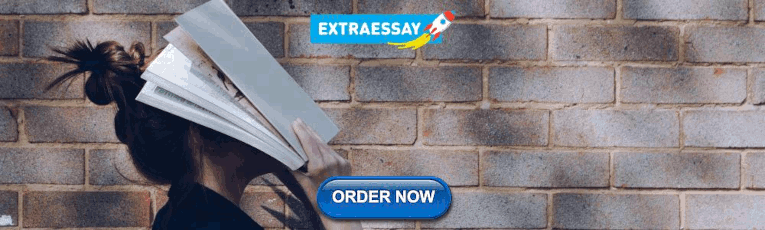
IMAGES
VIDEO
COMMENTS
Independent Samples vs. Dependent Samples. Hypothesis tests and statistical modeling that compare groups have assumptions about the nature of those groups. Choosing the correct test or model depends on knowing which type of groups your experiment has. Additionally, when designing your study, selecting the best type can help you tailor the ...
2.4: Dependent Samples t-test 2.4.2: Dependent Sample t-test Calculations ... As with our other hypotheses, we express the hypothesis for paired samples \(t\)-tests in both words and mathematical notation. The exact wording of the written-out version should be changed to match whatever research question we are addressing (e.g. " There mean at ...
Developing a hypothesis (with example) Step 1. Ask a question. Writing a hypothesis begins with a research question that you want to answer. The question should be focused, specific, and researchable within the constraints of your project. Example: Research question.
Testing Hypotheses with Dependent Samples. When testing a hypothesis about two dependent samples, we follow the same process as when testing one random sample or two independent samples: State the null and alternative hypotheses. Choose the level of significance; Set the criterion (critical values) for rejecting the null hypothesis.
Dependent and Independent Samples Calculations for two samples of data (both dependent or both independent) necessary to reject or accept the null hypothesis Progress
In DATAtab you can choose with one click whether you want to calculate the respective hypothesis test for dependent or independent samples. Depending on the format in which you insert your data, a variant is pre-selected. Usually, a series is a respondent or, more generally, a case. Therefore, metric values that are in a series are initially ...
Table of contents. Step 1: State your null and alternate hypothesis. Step 2: Collect data. Step 3: Perform a statistical test. Step 4: Decide whether to reject or fail to reject your null hypothesis. Step 5: Present your findings. Other interesting articles. Frequently asked questions about hypothesis testing.
Step 5: Phrase your hypothesis in three ways. To identify the variables, you can write a simple prediction in if … then form. The first part of the sentence states the independent variable and the second part states the dependent variable. If a first-year student starts attending more lectures, then their exam scores will improve.
D = XT2 −XT1 D = X T 2 − X T 1. Where D D is the difference score, XT1 X T 1 is the score on the variable at Time 1 (Before), and XT2 X T 2 is the score on the variable at Time 2 (After). Notice that we start with Time 2 so that positive scores show improvement, and negative scores show that skills decreased.
The dependent t-test is testing the null hypothesis that there are no differences between the means of the two related groups. If we get a statistically significant result, we can reject the null hypothesis that there are no differences between the means in the population and accept the alternative hypothesis that there are differences between ...
Two Dependent Samples (Matched Pairs) Two samples that are dependent typically come from a matched pairs experimental design. The parameter tested using matched pairs is the population mean difference. When using inference techniques for matched or paired samples, the following characteristics should be present: Simple random sampling is used.
Dependent Samples T-test. The dependent samples t-test is used to compare the sample means from two related groups. This means that the scores for both groups being compared come from the same people. The purpose of this test is to determine if there is a change from one measurement (group) to the other. Basic Hypotheses
A hypothesis is a tentative statement about the relationship between two or more variables. It is a specific, testable prediction about what you expect to happen in a study. It is a preliminary answer to your question that helps guide the research process. Consider a study designed to examine the relationship between sleep deprivation and test ...
The t-test for dependent samples is a statistical test for comparing the means from two dependent populations (or the difference between the means from two populations). The t-test is used when the differences are normally distributed. The samples also must be dependent. The formula for the t-test statistic is: t = D¯−μD (SD n√) t = D ...
First, we compute the means. Then we perform the t-test for two dependent samples. > t.test (Kscore2,Kscore1,paired=TRUE) Paired t-test. The null hypothesis is that the mean change in knowledge scores from before to after is 0. However, the analyis shows that the mean difference is 3.53 with a 95% confidence interval that ranges from 1.36 to 5.7.
Hypothesis Testing for Dependent and Independent Samples. We have learned about hypothesis testing for proportion and means with both large and small samples. However, in the examples in those lessons only one sample was involved. In this lesson we will apply the principals of hypothesis testing to situations involving two samples.
T Test Calculator for 2 Dependent Means. The t-test for dependent means (also called a repeated-measures t-test, paired samples t-test, matched pairs t-test and matched samples t-test) is used to compare the means of two sets of scores that are directly related to each other.So, for example, it could be used to test whether subjects' galvanic skin responses are different under two conditions ...
The independent variable is the cause. Its value is independent of other variables in your study. The dependent variable is the effect. Its value depends on changes in the independent variable. Example: Independent and dependent variables. You design a study to test whether changes in room temperature have an effect on math test scores.
Estimation of the adjusted sample size indicates the need for careful consideration when interpreting the results of hypothesis tests involving dependent samples, particularly about the p value. Because the hypothesis test assumes sample independence, relying solely on the number of dependent samples as the sample size may prove inadequate.
In a paired samples t-test, that takes the form of 'no change'. There is no improvement in scores or decrease in symptoms. Thus, our null hypothesis is: Null Hypothesis: The means of Time 1 and Time 2 will be similar; there is no change or difference. Symbols: ¯ XT1 = ¯ XT2.
Calculations for two samples of data (both dependent or both independent) necessary to reject or accept the null hypothesis % Progress . MEMORY METER. This indicates how strong in your memory this concept is. Practice. Preview; Assign Practice; Preview. Progress % Practice Now.
A hypothesis test can help determine if a difference in the estimated proportions reflects a difference in the population proportions. 10.5: Matched or Paired Samples When using a hypothesis test for matched or paired samples, the following characteristics should be present: Simple random sampling is used. Sample sizes are often small.
In research, a variable is any characteristic, number, or quantity that can be measured or counted in experimental investigations. One is called the dependent variable, and the other is the independent variable. In research, the independent variable is manipulated to observe its effect, while the dependent variable is the measured outcome.
Hypothesis-based methods for proximity dependent protein labeling. These methods rely on the co-expression of two fusion proteins, one fused to a ligase (Protein A) and the other to an acceptor peptide (Protein B). To test for an interaction between Protein A and Protein B the fusion proteins are co-expressed and labeling of protein B is evaluated.
Two dependent Samples with data Calculator. Type in the values from the two data sets separated by commas, for example, 2,4,5,8,11,2. Then enter the tail type and the confidence level and hit Calculate and the test statistic, t, the p-value, p, the confidence interval's lower bound, LB, the upper bound, UB, and the data set of the differences will be shown.