
Government of Nepal
Ministry of Health and Population
Department of Health Services
Epidemiology and Disease Control Division
- IEC Materials & Factsheets
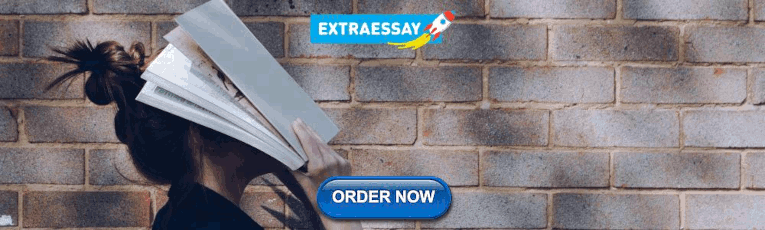
COVID-19 Presentation in Nepali

- Epidemiology & Outbreak Management Section
News & Update
2024_07_08_Dengue Situation Update

सर्ने संक्रामक रोगहरुको सुचीमध्ये प्राथमिकीकरण गरीएका रोगहरुको सुची (सं १७, ३-२०)

2024_07_03_Dengue Situation Update

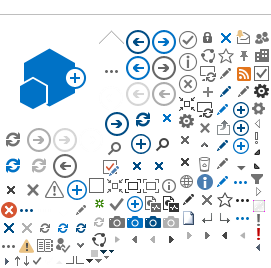
- Emergency information
- Increase Font Size
- Decrease Font Size
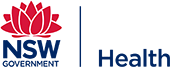
Nepali (नेपाली)
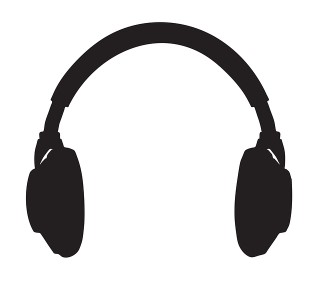
ORIGINAL RESEARCH article
Assessment of covid-19 pandemic in nepal: a lockdown scenario analysis.

- 1 Research and Innovation Department, Science Hub, Kathmandu, Nepal
- 2 Department of Research and Training, Public Health Perspective Nepal, Pokhara, Nepal
- 3 Faculty of Health and Applied Sciences, University of the West of England, Bristol, United Kingdom
- 4 Department of Civil Engineering, University of Bristol, Bristol, United Kingdom
The Government of Nepal issued a nationwide lockdown from 24 March to 21 July 2020, prohibiting domestic and international travels, closure of the border and non-essential services. There were only two confirmed cases from 610 Reverse Transcription Polymerase Chain Reaction (RT-PCR) tests and no fatalities when the government introduced nationwide lockdown. This study aimed to explore the overall scenario of COVID-19 including spatial distribution of cases; government efforts, and impact on public health, socio-economy, and education during the lockdown in Nepal. We collated and analyzed data using official figures from the Nepalese Ministry of Health and Population. Nepal had performed 7,791 RT-PCR tests for COVID-19, the highest number of tests during the lockdown. It has recorded its highest daily rise in coronavirus infections with a total of 740 new cases from the total of 4,483 RT-PCR tests performed on a single day. Nepal had reported a total of 17,994 positive cases and 40 deaths at the end of lockdown. The spatial distribution clearly shows that the cases were rapidly spreading from the southern part of the country where most points of entry and exit from India are located. To contain the spread of the virus, the government has also initiated various preventive measures and strategies during the lockdown. The Government of Nepal needs to allocate more resources, increase its capacity to test and trace, establish dedicated isolation and quarantine facility and impose local restrictions such as a local lockdown based on risk assessment rather than a nationwide lockdown.
Introduction
Coronavirus disease (COVID-19) outbreak originating from Wuhan, China in late 2019 has spread worldwide claiming more than 2.5 million lives all over the world as of 01 March 2021 ( 1 ). On 11 March 2020, the World Health Organization (WHO) declared it as a pandemic ( 1 ). Since the outbreak of the disease WHO through its guidelines has prioritized the actions for responding to the virus; urged the government to maintain health facilities, raise public awareness, and stock up on medical supplies ( 2 ).
Several modeling studies have been conducted during the early phases of the outbreak to predict the epidemic and effectiveness of multiple population-wide strategies, including lockdown, social distancing, quarantine, testing and contact tracing, and media-related awareness among others to mitigate the spread of COVID-19 ( 3 – 9 ). The strict lockdown was enforced to limit the spread of COVID-19 in countries such as Italy, Spain, France, the UK after the steady rise in cases whereas Nepal introduced lockdown during the early phase of the pandemic ( 10 ). Lockdown is the blanket approach that buys time to prepare the healthcare system (active case finding through testing and tracing, case management, for example, quarantine, isolation and treatment, and availability of protective equipment) to confine the virus and its spread. The Government of Nepal issued a nationwide lockdown from 24 March to 21 July 2020, prohibiting domestic and international travels, closure of border and non-essential services in the first stage, which was later eased on 11 June 2020.
The basic reproduction number (R0) which measures the potential transmission of an infectious disease is a fundamental metric to determine if an outbreak is expected to continue. In general, the disease is expected to spread and become epidemic if R0 is more than one and to decline and ultimately end if R0 is <1. The R0 value of the COVID-19 outbreak was not available for Nepal when the government was preparing for the lockdown. However, in neighboring countries, the estimated R0 value of coronavirus was above 2.0 in India ( 4 ) and between 2.2 to 3.5 in China ( 11 ), indicating a potential to cause an outbreak. There were only two confirmed cases from 610 Reverse Transcription Polymerase Chain Reaction (RT-PCR) tests and no fatalities before lockdown ( 12 ). The indexed case was found on 23 January 2020 in Kathmandu on a person who had traveled from Wuhan, China ( 13 ). The second case was confirmed 2 months later on 23 March who had traveled to Nepal from France via Qatar ( 14 ).
This study aimed to assess the overall scenario of COVID-19 during the lockdown (positive cases, RT-PCR test performed, recoveries, total active and deaths cases including case fatality ratio) including spatial distribution of the cases, government measures to manage the pandemic; and impacts on public health, socio-economy, and education. Finally, we offer helpful suggestions to address the challenges brought by the impact of COVID-19.
Study Design
This was a descriptive study to assess the multiple scenarios of the COVID-19 pandemic in Nepal during the lockdown period. Statistical and spatial presentations of data, government efforts, impacts on public health, socio-economy, and education were discussed to summarize the situation of Nepal during the lockdown period. The main focus of the study was for the lockdown period, however, data was updated and discussed to reflect the post lockdown scenario.
Nepal is a lower-middle-income country in South Asia between India (in the south, east, and west) and China (in the North). It has a population of around 30 million. The new constitution promulgated on 20 September 2015 made Nepal a federal democratic republic and is now divided into seven provinces, 77 districts, and 753 local units (municipalities and rural municipalities). It is divided into three physiographic regions: Mountain region (Great Himalayan Range in the northern part), Hilly region, and Terai region (low land region at the Indian border in the southern part).
Data Collection
We analyzed results from the COVID-19 situation reports prepared by the Nepalese Ministry of Health and Population (MOHP). For this study, we downloaded the COVID-19 situation reports from the openly available online resources on the web portal (covid19.mohp.gov.np). We collated the data for the lockdown period (from 24 March to 21 July 2020). The MOHP has defined a confirmed COVID-19 case as any person with laboratory confirmation of COVID-19 infection, irrespective of clinical signs and symptoms, that is, person who had RT-PCR tested positive for Severe Acute Respiratory Syndrome Coronavirus-2 (SARS-CoV-2). Municipal level data has been taken from the data prepared for COVIRA , a COVID-19 risk assessment tool ( 15 ).
Statistical Analysis
Daily positive cases reported in local administrative units (municipality or rural municipality) were analyzed along with total daily data for the country. Data were analyzed descriptively in a Microsoft Excel 2019 Version 16.0 (Microsoft Corporation, Redmond, Washington, USA). The number of COVID-19 cases, daily RT-PCR tests performed and the number of recoveries were used to assess the COVID-19 pandemic situation. The number of deaths and Case Fatality Rate (CFR) was presented by different age groups. CFR was calculated as the proportion of confirmed deaths among identified confirmed cases. Everyday data of COVID-19 positive cases were presented in local level units. The maps used to show the spatial distribution of COVID-19 cases in Nepal were created using the QGIS Version 3.14 ( www.qgis.org ).
Figure 1 shows the daily and weekly average of tests and positive cases during the lockdown period. On 29 June, Nepal had performed 7,791 tests for COVID-19, the highest number of tests during the lockdown. It has recorded its highest daily rise in coronavirus infections with a total of 740 new cases from the total of 4,483 test performed on a single day on 3 July. The number of daily cases was decreasing after its peak on 3 July but subsequently, the number of tests was also decreasing.
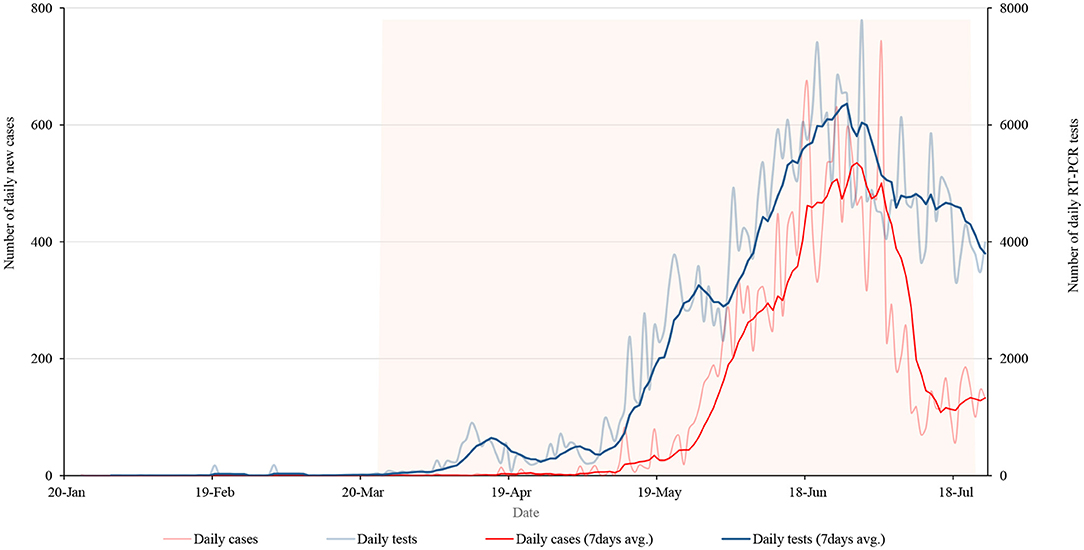
Figure 1 . Daily new cases and RT-PCR tests along with 7 days average of cases and tests during the lockdown period.
Figure 2 shows COVID-19 cumulative cases, recoveries, and active cases during the lockdown. As per the figure, the number of people recovered from the coronavirus in Nepal increased during the lockdown. However, the number of active cases decreased. On 1 July there were 10,390 active cases in the country which was continuously declining till the end of the lockdown period. Lack of proper management of quarantine and isolation centers may have caused a significant increase in cases 2-months after lockdown started as most of the cases were imported from India, and were later transmitted to the community. Data show that the bending of the curve had started before the government decided to lift the nationwide lockdown however the risks of further transmission in the community were prevailing.
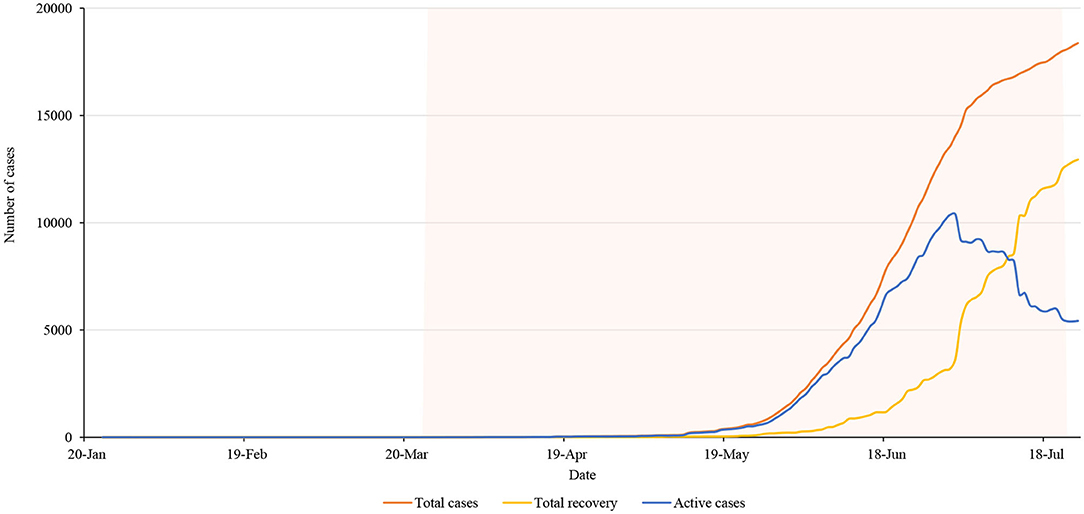
Figure 2 . Total cases, recovery and active cases in Nepal during the lockdown period.
Death and Case Fatality Ratio
Nepal had reported a total of 17,994 positive cases and 40 deaths on the last date of nationwide lockdown. Figures 3A,B shows the distribution of cases and a death toll, respectively, in age and gender groups. It clearly shows that the younger population of age group 21–30 years is more infected, and most importantly the fraction of female who got infected is relatively smaller. The main reason behind such distribution is most of the cases were imported by young immigrants who went abroad for work. The number of deaths and the corresponding overall death rate is very small (0.22%) however the relative death rate curve tells a different story ( Figure 4 ).
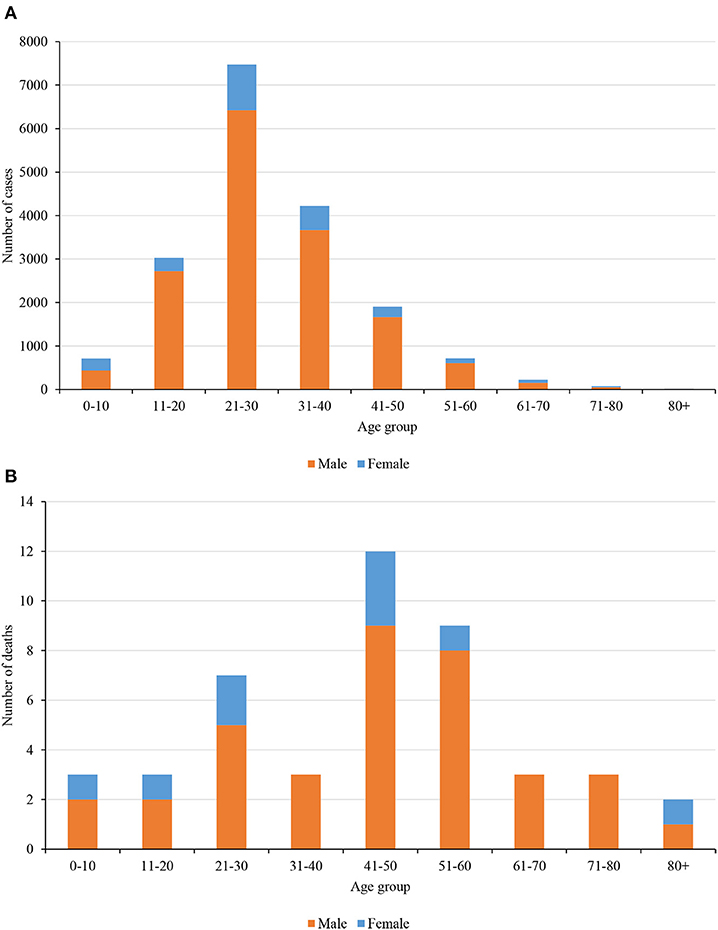
Figure 3. (A) COVID-19 cases distribution among age and gender groups during the lockdown period. (B) . COVID-19 deaths distribution among age and gender groups during the lockdown period.
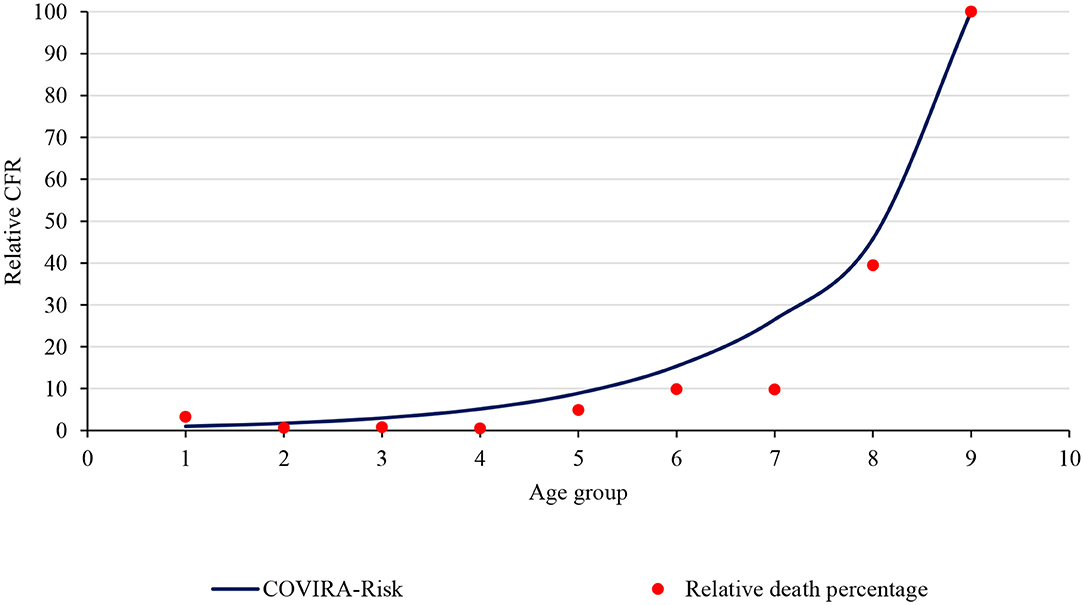
Figure 4 . Relative case fatality ratio and comparison with the COVIRA risk model.
CFR in Nepal shows a similar trend to the COVIRA risk model ( 15 ) which is the risk model based on early-stage pandemic data. Figure 4 shows the comparison of the COVIRA risk model and Nepal data (relative percentage of CFR). The correlation between CFR in age groups and the COVIRA model is 0.986 which shows very good agreement of data. The trend of CFR by the age group shows an exponential relation with an r-square value of 0.714. Hence, one of the alarming points for Nepal is, if the infection spread in the community and the older people get infected, the death toll would rapidly increase.
Spatial Distribution
Figure 5 shows the spatial distribution of total cases on the first and the 15th days of the months over a lockdown period. These maps clearly show that the cases were rapidly spreading from the southern part of the country where most points of entry and exit from India are located.
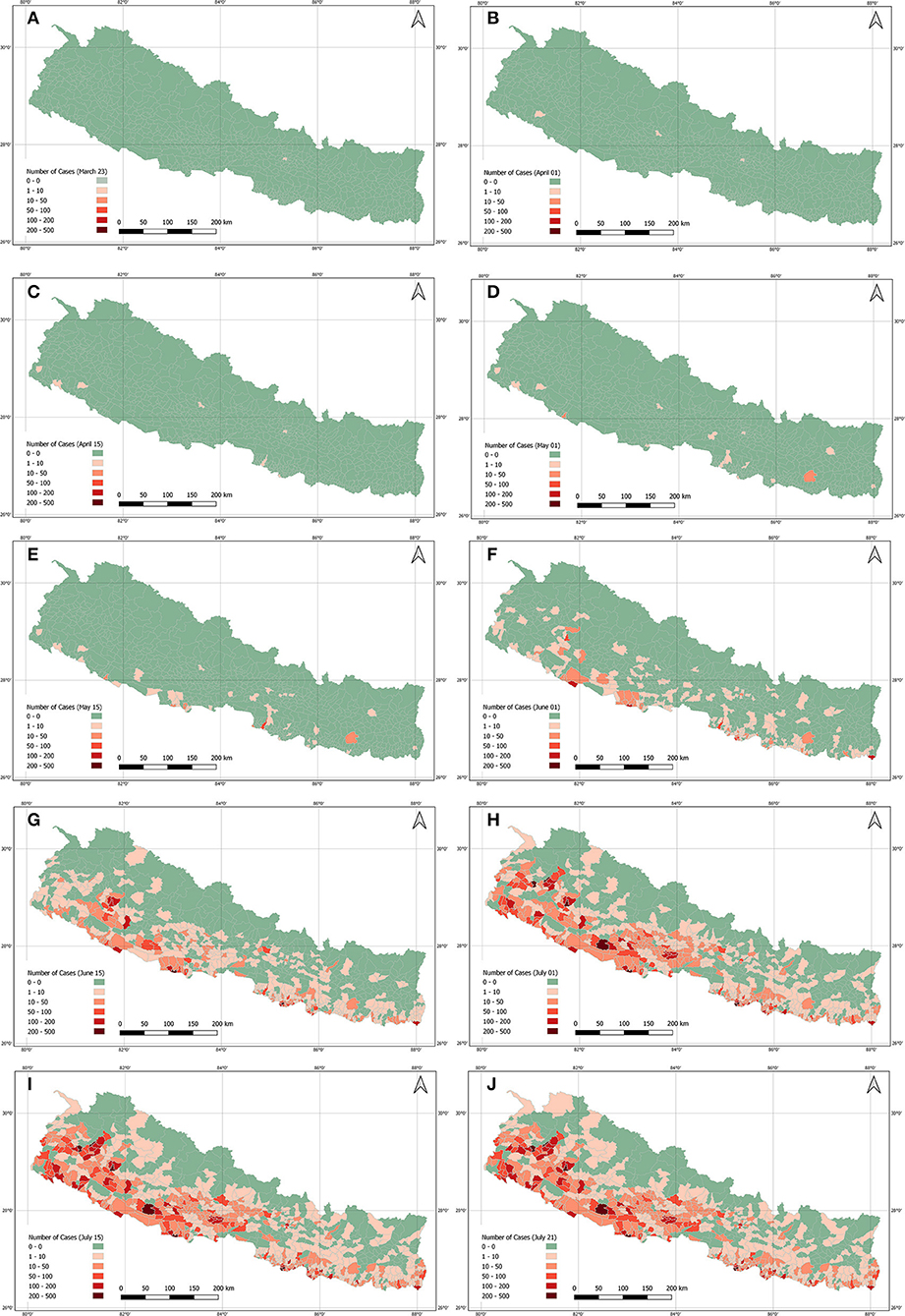
Figure 5 . Total positive COVID-19 cases across the country by date during lockdown period. (A) : March 23. (B) : April 01. (C) : April 15. (D) : May 01. (E) : May 15. (F) : June 1. (G) : June 15. (H) : July 1. (I) : July 15. (J) : July 21.
The government lifted the lockdown when the curve of daily cases was flattening, however, the situation in the country was not totally under control. There was a high possibility of spreading the virus if an infected person was exposed to others until around 2 weeks of infection. Mapping the report of last active cases across the country was important to look at the prevailing risk zones. Figure 6 shows the number of days since the last case was reported on the day when the lockdown was lifted.
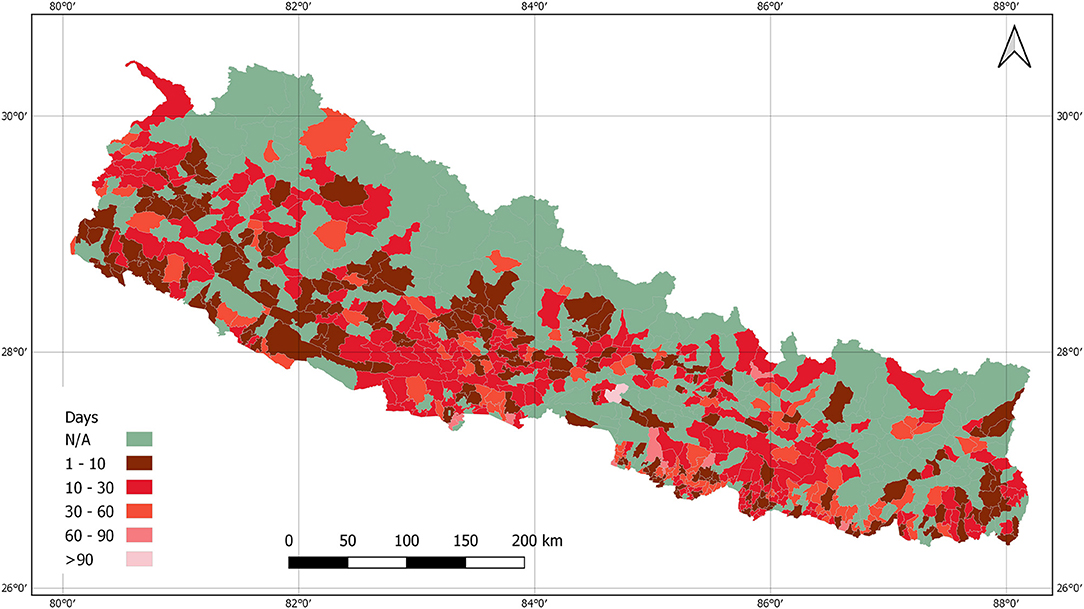
Figure 6 . Number of days since the last case reported on the day of lockdown lifted.
Government Efforts on Managing the Pandemic During the Lockdown
The Government of Nepal has initiated various preventive measures and strategies during the lockdown as shown in the list below ( 16 ).
• Guidelines issued for the management and handling of quarantine.
• Dissemination of information, education and communication materials on social distancing, handwashing, proper use of masks and hand sanitizers, mass awareness via television, radio, social media and pamphlets.
• Launch of mobile application ( Hamro Swasthya ), the web portal (covid19.mohp.gov.np).
• Two toll-free call centers to provide counseling on COVID-19 prevention and treatment.
• Daily briefings by the MOHP to update about the current situation.
• Travel restriction, testing, and tracing.
• Health sector emergency response plan for COVID-19 pandemic which includes strategies to deal quarantine management, case investigation, contact tracing, community-level screening and testing, strengthening laboratory capacity etc.
• Protocol on the safe management of dead bodies.
• Guidelines issued for the management of isolation of COVID-19 cases.
• National testing guidelines for COVID-19.
• Public health standards to be followed by people and institutions during the COVID-19 pandemic and lockdown.
• Allowed private laboratories to perform RT-PCR test.
• Availability of RT-PCR tests in all seven provinces of Nepal.
• Health standards for isolation of COVID-19 cases.
• Training of trainers on case investigation and contact tracing.
• MOHP endorsed the standards for home quarantine.
• The number of hospitals for the management of COVID-19 (as of 21 July 2020):
◦ Hospitals with COVID-19 clinics: 125
◦ Level 1 COVID Hospitals (for management of positive cases with mild symptoms): 16
◦ Level 2 COVID Hospitals (for management of positive cases with moderate or severe symptoms): 16
◦ Level 3 COVID Hospitals (for management of COVID positive cases who needs multi-speciality services): 4
• Total laboratories established capable of doings RT-PCR: 28
• Total intensive care unit beds allocated for COVID-19 cases: 942 of total 2,600 ICU beds available in the country.
• Total ventilators allocated for COVID-19 cases: 496 of total 900 ventilators available in the country.
Nepal had performed 7,791 tests for COVID-19, the highest number of tests during the lockdown. It has recorded its highest daily rise in coronavirus infections with a total of 740 new cases from the total of 4,483 tests performed on a single day. The number of people recovered from the coronavirus in Nepal increased during the lockdown. Nepal had reported a total of 17,994 positive cases and 40 deaths on the last date of nationwide lockdown. The spatial distribution clearly shows that the cases were rapidly spreading from the southern part of the country where most points of entry and exit from India are located.
Socioeconomic Impact
Nationwide lockdown restricted the socioeconomic activities all over the country, where very few essential services were run throughout the period. Multidimensional impacts of lockdown have been found in society, many people lost their jobs, and businesses along with other health care were impacted. It disrupted the supply chain, shut many informal and small enterprises, and pushed more vulnerable people into poverty ( 17 ). The tourism industry hit hard in Nepal where it fell below 10%, resulting in more than 13,000 job loss of trekkers and guides ( 18 ). There are 1.6 to 2 million jobs at risk due to the COVID-19 crisis where 80.8% of total jobs in the country are informal ( 19 ). A household survey on the impact of COVID-19 on food security and vulnerability conducted by the World Food Programme, Nepal and the Ministry of Agriculture and Livestock Development showed that of the 4,416 households from across the country, only 42% had 1 month worth of food stocks ( 20 ).
Healthcare Impact
The lockdown has affected the health of individuals and disrupted healthcare services, particularly emergency and regular health services. During the lockdown, at the individual level, one of the most notable impacts was on psychological health. Quarantine, social isolation, and travel restrictions had negatively impacted the mental health of people who have COVID-19 and their families. A few preliminary studies have shown psychological issues such as stress, anxiety, depression, insomnia among the general population ( 21 – 23 ) as well as frontline health workers ( 24 , 25 ). A study by Gupta and colleagues conducted among 150 health workers showed that 38 % of the healthcare workers on COVID-19 duty in Nepal suffered anxiety and/or depression ( 24 ). Another online survey conducted among 475 health workers showed that 41.9% of health workers had symptoms of anxiety, 37.5% had depression symptoms and 33.9% had symptoms of insomnia ( 25 ). Incidents of stigmatization and social discrimination of healthcare workers, people who have COVID-19 and their families were also reported in Nepal during the lockdown ( 26 , 27 ).
These effects of lockdown on psychological health are in line with evidence from other countries. Studies from these countries show that lockdown, quarantine, and isolations have increased social isolation, frustration, loneliness, boredom, inadequate supplies, financial insecurity, and stigma which are associated with increased risk of depression, stress, anxiety, confusion, fear, emotional disturbance, insomnia, grief and irritability ( 28 – 30 ). A study conducted in India shows that the prevalence of depression and anxiety has increased by eight to ten-fold among the adult population during lockdown ( 31 ). Women in general suffered more from lockdown reporting increased depression, anxiety, stress, and insomnia ( 32 , 33 ).
Nepal Police record shows that during the lockdown, the number of suicide cases has increased. Within 74 days of lockdown, a total of 1,227 people committed suicide, which is more than 15 suicidal death per month compared to the previous year ( 34 ). Although reasons for what had caused suicide and suicidal thoughts are still unknown in Nepal, they could be linked to the uncertainty about the pandemic, self-isolation, financial burden, loss of family members, stigma as evident in previous disasters and epidemic ( 35 – 37 ). In addition to suicide, domestic violence, sexual abuse, and rape were being perpetrated during the lockdown in Nepal ( 38 ).
The government's priority to combat COVID-19 and the lockdown adopted to contain its transmission put vulnerable populations such as pregnant women, children, the elderly and people with non-COVID diseases at risk by impacting their ability to access essential healthcare services. For example, pregnant women faced barriers to accessing regular antenatal care and delivery services ( 39 ) and patients with non-communicable diseases faced barriers to access long-term care and medicines ( 40 ) during the lockdown periods. Millions of children aged between 6 months and 5 years missed measles and rubella mass immunization, vitamin A, and deworming tablets because the Government of Nepal postponed these national-level campaigns ( 41 ). Limited ability to access such essential and routine health care services poses an urgent threat to the nation's health and could reverse some of the achievements in reducing maternal, newborn, and child deaths.
Future COVID-19 cases in Nepal will depend on the situation in India where the cases are increasing rapidly. Nepal shares an open border with India and there may be an increase in the number of Nepali workers returning from India who remain stranded in different parts of India due to the lockdowns in both countries. It is estimated that 600,000 migrant workers will return to Nepal within a few weeks of lifting the nationwide lockdown restrictions ( 42 ). This flow of migrant workers could increase the number of cases as the government has not been able to utilize the lockdown time efficiently to prepare and ready for responding to COVID-19. The challenge will be to test and trace these people for COVID-19. Before the lockdown, thousands of migrant Nepali workers returned to Nepal without proper screening from the Indian states of Maharashtra, Delhi and Gujarat, where the R0 value is more than one indicating an outbreak of COVID-19 in these states ( 4 ).
The testing capacity of the country has not increased due to a shortage of RT-PCR test kits, personal protective equipment, trained workers, and medical supplies. With limited testing capacity, it is challenging to monitor the transmission of the virus in Nepal because the suspected cases continue to transmit the virus while awaiting the COVID-19 test. Some COVID-19 cases remain asymptomatic, so it is difficult to predict the severity of the outbreak. There are only a few health facilities capable of treating and managing the cases with some degree of preparedness and readiness to provide the services ( 43 , 44 ). If the number of cases becomes higher than the capacity of these health facilities to cope with the increased demand it would be more challenging to contain the virus.
Another challenge is to control other communicable and non-communicable diseases amidst the COVID-19 pandemic. Non-communicable diseases such as cancer, hypertension, cardiovascular disease, diabetes, chronic respiratory diseases, mental illness are already a major public health problem in Nepal and accounted for about 71% of the total annual deaths in 2019 ( 45 ). These diseases will exacerbate and the impact could be much higher than the COVID-19 if they are left behind in the fight against COVID-19.
Strengths and Limitations
The study strength included the use of openly available official figures from the MOHP web portal to provide an overall scenario of the COVID-19 pandemic during the lockdown. The findings would be applicable to compare the post-lockdown situation of the COVID-19 pandemic in Nepal and to guide more effective measures to contain the spread of the coronavirus. One of the limitations with openly available data was individual-level patient details were not accessible to perform detail epidemiological analysis. The number of people who had COVID-19 represents only a reflection of those who were tested rather than the actual figure. Also, manual gathering and submitting data from hospitals to the central government means can result in a delay and the loss of some information in reporting the number of deaths or cases so may not be a true reflection of the daily case counts. Further, the MoHP data exclude deaths from COVID-19 outside hospitals, such as those in the home. In the daily situation reports shared by the MoHP, there was inconsistency in the details provided. Some daily situation reports of the COVID-19 had information on gender while others did not contain such key information.
Post-lockdown Scenario
This paper was mainly designed to present and discuss the lockdown scenario, however considering the publication date, data are updated and discussed for post-lockdown scenario. Figure 7 shows the RT-PCR tests including cases and Figure 8 shows the total cases, recoveries, and active cases as of 01 March 2021. The number of COVID-19 cases had increased until 21 October 2020 and after that the number of cases reported and number of tests conducted had declined ( Figure 7 ). The two dips in Figure 7 , that is, on 27 October 2020 and 17 November 2020, were the festival days where less tests were performed and consequently less cases were identified.
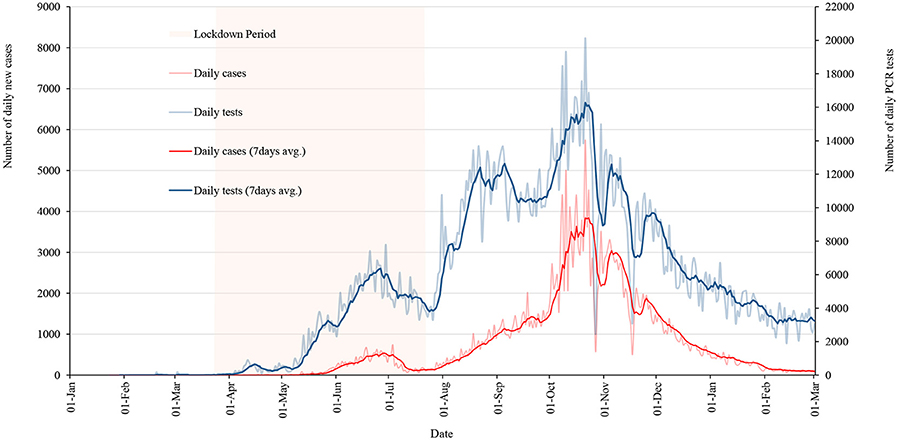
Figure 7 . Daily new cases and RT-PCR tests along with 7 days average of cases and tests as of 01 March 2021.
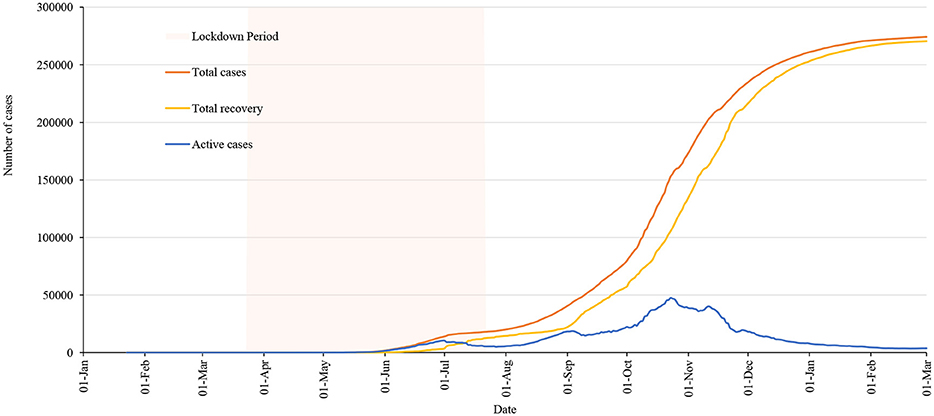
Figure 8 . Total cases, recovery, and active cases in Nepal as of 01 March 2021.
Figure 9 shows the distribution of COVID-19 cases among the age group and gender as of 01 March 2021. It shows the community transmission of the coronavirus in the post-lockdown period contributed to the increase of cases among higher age groups and in the female. As discussed previously, one of the challenges is having a higher mortality rate when the virus spread among the older generation, which has been the case in recent days. As of 01 March 2021, mortality rate was above 1%.
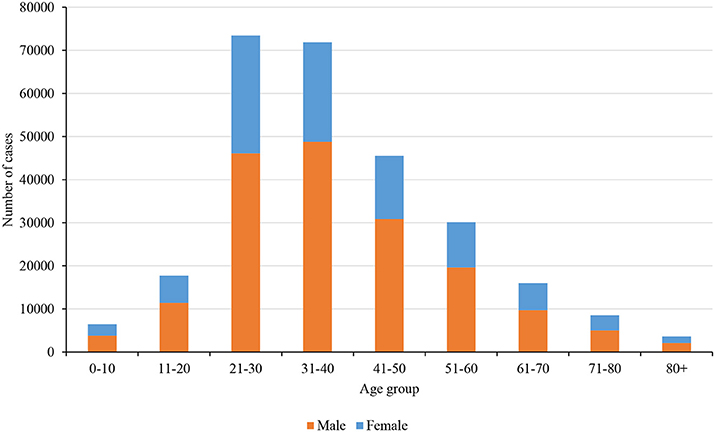
Figure 9 . COVID-19 cases distribution among age group and gender as of 01 March 2021.
Figure 10A shows the number of cases in the districts. Compared with the lockdown period, major cities including the capital city Kathmandu have a relatively higher number of cases, which resembles the overall risk scenario reported ( 15 ). Figure 10B shows the infected population percentage at the district level across the country. Mugu had a lowest (0.05%) infected population whereas Kathmandu had a maximum of 4.5% infected population.
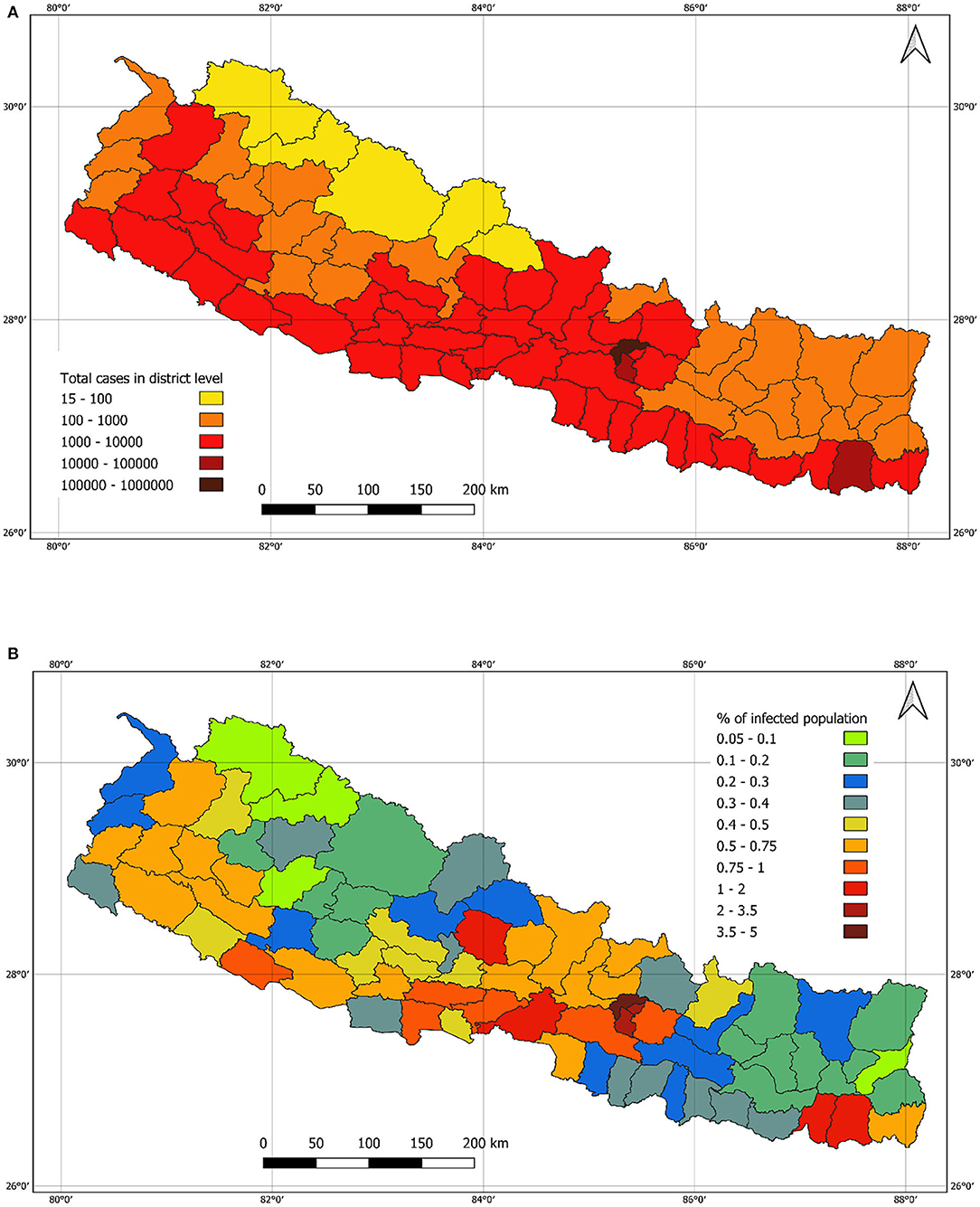
Figure 10. (A) : Number of cases in district level as of 01 March 2021. (B) : Population infection rate in district level as of 01 March 2021.
Conclusion and Recommendation
This study provides an overall scenario of the COVID-19 pandemic during the lockdown in Nepal. The capacity of the health system to quickly test to find out if anyone develops symptoms of COVID-19 and tracing and testing of close contacts of those who test positive for COVID-19 must be increased. The government needs to allocate resources, such as the necessary public health workforce, availability of personal protective equipment, expansion of intensive care unit beds, and purchase of extra ventilators. Other actions to stop spread include managed isolation in a designated setting for people who cannot afford to self-isolate or in a dedicated quarantine facility who can't self-quarantine.
Another approach the government could take to manage local COVID-19 outbreaks is to impose local restrictions. This could be in the form of local lockdown based on risk assessment rather than the nationwide lockdown. As the restrictions of COVID-19 lockdown are eased, there would be more flow of people which leads to more exposure so preventive measures should be established in shopping centers, cities, shops and workplaces. Citizen and institutional/governance awareness is always a key factor in disaster risk reduction and managing pandemic, that often lacking in the developing world ( 46 ). The government should continue disseminating information on following social distancing, hand hygiene, and face covering. Data from the post lockdown period also shows the ways to tackle future pandemics considering the socioeconomic condition of the community.
Data Availability Statement
The original contributions presented in the study are included in the article/supplementary material, further inquiries can be directed to the corresponding author/s.
Author Contributions
KS, AB, and RP conceptualized the study and prepared the first draft of the paper. KS and RP produced most of the figures, analyzed, and interpreted the data. All authors have approved the final version.
Conflict of Interest
The authors declare that the research was conducted in the absence of any commercial or financial relationships that could be construed as a potential conflict of interest.
Acknowledgments
We acknowledge the Ministry of Health and Population, Nepal for making COVID-19 data publicly available.
1. WHO. Situation Reports . (2021). Available online at: https://www.who.int/docs/default-source/coronaviruse/wou_2021_1march2021.pdf?sfvrsn=18b1db33_1&download=true (accessed March 01, 2021).
Google Scholar
2. WHO. Responding to Community Spread of COVID-19: Interim Guidance . (2020). Available online at: https://apps.who.int/iris/handle/10665/331421 (accessed July 25, 2020).
3. Khajanchi S, Sarkar K. Forecasting the daily and cumulative number of cases for the COVID-19 pandemic in India. Chaos. (2020) 30:071101. doi: 10.1063/5.0016240
PubMed Abstract | CrossRef Full Text | Google Scholar
4. Sarkar K, Khajanchi S, Nieto JJ. Modeling and forecasting the COVID-19 pandemic in India. Chaos Solitons Fractals. (2020) 139:110049. doi: 10.1016/j.chaos.2020.110049
5. Khajanchi S, Sarkar K, Mondal J. Dynamics of the COVID-19 pandemic in India. arXiv [Preprint]. (2021). arXiv:2005.06286.
6. Giordano G, Blanchini F, Bruno R, Colaneri P, Di Filippo A, Di Matteo A, et al. Modelling the COVID-19 epidemic and implementation of population-wide interventions in Italy. Nat Med. (2020) 26:855–60. doi: 10.1038/s41591-020-0883-7
7. Tang B, Wang X, Li Q, Bragazzi NL, Tang S, Xiao Y, et al. Estimation of the transmission risk of the 2019-nCoV and its implication for public health interventions. J Clin Med. (2020) 9:462. doi: 10.3390/jcm9020462
8. Anderson RM, Heesterbeek H, Klinkenberg D, Hollingsworth TD. How will country-based mitigation measures influence the course of the COVID-19 epidemic? Lancet. (2020) 395:931–4. doi: 10.1016/s0140-6736(20)30567-5
CrossRef Full Text | Google Scholar
9. Lin Q, Zhao S, Gao D, Lou Y, Yang S, Musa SS, et al. A conceptual model for the coronavirus disease 2019 (COVID-19) outbreak in Wuhan, China with individual reaction and governmental action. Int J Infect Dis. (2020) 93:211–6. doi: 10.1016/j.ijid.2020.02.058
10. Piryani RM, Piryani S, Shah JN. Nepal's Response to Contain COVID-19 Infection. J Nepal Health Res Counc. (2020) 18:128–34. doi: 10.33314/jnhrc.v18i1.2608
11. Zhao S, Lin Q, Ran J, Musa SS, Yang G, Wang W, et al. Preliminary estimation of the basic reproduction number of novel coronavirus (2019-nCoV) in China, from 2019 to 2020: A data-driven analysis in the early phase of the outbreak. Int J Infect Dis. (2020) 92:214–7. doi: 10.1016/j.ijid.2020.01.050
12. Ministry of Health and Population. Health Sector Response to COVID-19 Situation Report #43. (2020). Available online at: https://drive.google.com/file/d/1z6XBao8nCS9OTmhlY-nU6sg15WUV4L-R/view?usp=sharing (accessed July 25, 2020).
13. Ministry of Health and Population. Health Sector Response to Novel Coronavirus (2019-nCoV) Situation Report #1 . (2020). Available online at: https://drive.google.com/file/d/1mp0zAbQvNgy9kr1hgjqPZlQhtqMJFoil/view?usp=sharing (accessed July 25, 2020).
14. Pun SB, Mandal S, Bhandari L, Jha S, Rajbhandari S, Mishra AK, et al. Understanding COVID-19 in Nepal. J Nepal Health Res Counc. (2020) 18:126–7. doi: 10.33314/jnhrc.v18i1.2629
15. Parajuli RR, Mishra B, Banstola A, Ghimire BR, Poudel S, Sharma K, et al. Multidisciplinary approach to COVID-19 risk communication: a framework and tool for individual and regional risk assessment. Sci Rep. (2020) 10:21650. doi: 10.1038/s41598-020-78779-0
16. Ministry of Health and Population. Coronavirus disease (COVID-19) outbreak updates & resource materials . (2020). Available online at: https://heoc.mohp.gov.np/update-on-novel-corona-virus-covid-19/ (accessed 25 July, 2020).
17. UNDP. Rapid Assessment of Socio Economic Impact of COVID-19 in Nepal . (2020). Available online at: https://www.np.undp.org/content/dam/nepal/docs/Reports_2020/Nepal%20Rapid%20Assessment%20COVID19%20Final.pdf (accessed July 25, 2020).
18. WTO. COVID-19 and its effect on Nepal . (2020). Available online at: https://www.wto.org/english/tratop_e/covid19_e/sawdf_nepal_e.pdf (accessed July 26, 2020).
19. International Labour Organization. COVID-19 labour market impact in Nepal . (2020). Available online at: https://www.ilo.org/wcmsp5/groups/public/—asia/—ro-bangkok/—ilo-kathmandu/documents/briefingnote/wcms_745439.pdf (accessed July 26, 2020).
20. WFP. The Impact of COVID-19 on Households in Nepal: mVAM Household Livelihoods, Food Security and Vulnerability Survey. (2020). Available online at: https://docs.wfp.org/api/documents/WFP-0000116728/download/?_ga=2.17578425.1015156072.1598117657-459637742.1598117657 (accessed July 26, 2020).
21. Gupta AK, Sahoo S, Mehra A, Grover S. Psychological impact of 'Lockdown' due to COVID-19 pandemic in Nepal: an online survey. Asian J Psychiatr. (2020) 54:102243. doi: 10.1016/j.ajp.2020.102243
22. Samadarshi SCA, Sharma S, Bhatta J. An online survey of factors associated with self-perceived stress during the initial stage of the COVID-19 outbreak in Nepal. Ethiop J Health Dev. (2020) 34:84–9.
23. Sigdel A, Bista A, Bhattarai N, Pun BC, Giri G, Marqusee H, et al. Depression, Anxiety and Depression-anxiety comorbidity amid COVID-19 Pandemic: an online survey conducted during lockdown in Nepal. medRxiv [Preprint]. (2020). doi: 10.1101/2020.04.30.20086926
24. Gupta AK, Mehra A, Niraula A, Kafle K, Deo SP, Singh B, et al. Prevalence of anxiety and depression among the healthcare workers in Nepal during the COVID-19 pandemic. Asian J Psychiatr. (2020) 54:102260. doi: 10.1016/j.ajp.2020.102260
25. Khanal P, Devkota N, Dahal M, Paudel K, Joshi D. Mental health impacts among health workers during COVID-19 in a low resource setting: a cross-sectional survey from Nepal. Global Health. (2020) 16:89. doi: 10.1186/s12992-020-00621-z
26. Singh R, Subedi M. COVID-19 and stigma: Social discrimination towards frontline healthcare providers and COVID-19 recovered patients in Nepal. Asian J Psychiatr. (2020) 53:102222. doi: 10.1016/j.ajp.2020.102222
27. Aacharya RP, Shah A. Ethical dimensions of stigma and discrimination in Nepal during COVID-19 pandemic. Ethics Med Public Health. (2020) 14:100536. doi: 10.1016/j.jemep.2020.100536
28. Brooks SK, Webster RK, Smith LE, Woodland L, Wessely S, Greenberg N, et al. The psychological impact of uarantine and how to reduce it: rapid review of the evidence. Lancet. (2020) 395:912–20. doi: 10.1016/s0140-6736(20)30460-8
29. Dagnino P, Anguita V, Escobar K, Cifuentes S. Psychological effects of social isolation due to quarantine in chile: an exploratory study. Front Psychiatry. (2020) 11:591142. doi: 10.3389/fpsyt.2020.591142
30. Wang Y, Shi L, Que J, Lu Q, Liu L, Lu Z, et al. The impact of quarantine on mental health status among general population in China during the COVID-19 pandemic. Mol Psychiatry. (2021). doi: 10.1038/s41380-021-01019-y. [Epub ahead of print].
31. Pandey D, Bansal S, Goyal S, Garg A, Sethi N, Pothiyill DI, et al. Psychological impact of mass quarantine on population during pandemics-The COVID-19 Lock-Down (COLD) study. PLoS ONE. (2020) 15:e0240501. doi: 10.1371/journal.pone.0240501
32. Mattioli AV, Sciomer S, Maffei S, Gallina S. Lifestyle and stress management in women during COVID-19 pandemic: impact on cardiovascular risk burden. Am J Lifestyle Med. (2020). doi: 10.1177/1559827620981014. [Epub ahead of print].
33. Qiu J, Shen B, Zhao M, Wang Z, Xie B, Xu Y. A nationwide survey of psychological distress among Chinese people in the COVID-19 epidemic: implications and policy recommendations. Gen Psychiatr. (2020) 33:e100213. doi: 10.1136/gpsych-2020-100213
34. Poudel A. Over 1,200 people killed themselves during 74 days of lockdown. (2020). Available online at: https://kathmandupost.com/health/2020/06/14/over-1-200-people-killed-themselves-during-74-days-of-lockdown (accessed 26 July, 2020).
35. Chan SM, Chiu FK, Lam CW, Leung PY, Conwell Y. Elderly suicide and the 2003 SARS epidemic in Hong Kong. Int J Geriatr Psychiatry. (2006) 21:113–8. doi: 10.1002/gps.1432
36. Safarpour H, Sohrabizadeh S, Malekyan L, Safi-Keykaleh M, Pirani D, Daliri S, et al. Suicide death rate after disasters: a meta-analysis study. Arch Suicide Res. (2020) 1–14. doi: 10.1080/13811118.2020.1793045
37. Cousins S. Nepal's silent epidemic of suicide. Lancet. (2016) 387:16–7. doi: 10.1016/S0140-6736(15)01352-5
38. Sharma N. In Nepal lockdown, a domestic violence spike . (2020). Available online at: https://www.nepalitimes.com/latest/in-nepal-lockdown-a-domestic-violence-spike/ (accessed 25 July, 2020).
39. UNFPA. In Nepal, COVID-19 turning pregnancy excitement into fear . (2020). Available online at: https://www.unfpa.org/news/nepal-covid-19-turning-pregnancy-excitement-fear (accessed July 26, 2020).
40. Singh BP. Patients under regular medication face shortage of medicines due to prolonged lockdown . (2020). Available online at: https://kathmandupost.com/national/2020/05/06/patients-under-regular-medication-face-shortage-of-medicines-due-to-prolonged-lockdown (accessed July 26, 2020).
41. UNICEF. Disruption of child vaccination in South Asia poses an urgent threat to children's health – UNICEF . (2020). Available online at: https://www.unicef.org/rosa/press-releases/disruption-child-vaccination-south-asia-poses-urgent-threat-childrens-health-unicef (accessed July 26, 2020).
42. ACAPS. Nepal: COVID-19 & the return of migrants . (2020). Available online at: https://reliefweb.int/sites/reliefweb.int/files/resources/20200612_acaps_start_briefing_note_nepal_migrant_returnees_covid-19.pdf (accessed 26 July, 2020).
43. NHRC. Assessment of Health-Related Country Preparedness and Readiness of Nepal for Responding to COVID-19 Pandemic . Kathmandu: Nepal Health Research Council (2020).
44. Bhattarai S, Dhungana J, Ensor T, Shrestha UB. Assessment of service availability and Infection prevention measures in hospitals of Nepal during the transition phase of COVID-19 case surge. medRxiv. (2020). doi: 10.1101/2020.05.13.20097675
45. Global Burden of Disease Collaborative Network. Global Burden of Disease Study 2019 (GBD 2019) Results . (2020). Available online at: http://ghdx.healthdata.org/gbd-results-tool (accessed December 21, 2020).
46. Parajuli RR. Citizen Disaster Science Education for effective disaster risk reduction in developing countries. Geoenvironmental Disasters . (2020) 7:12. doi: 10.1186/s40677-020-00150-2
Keywords: COVID-19, coronavirus, public health, Nepal, spatial distribution analysis, lockdown, impacts, challenges
Citation: Sharma K, Banstola A and Parajuli RR (2021) Assessment of COVID-19 Pandemic in Nepal: A Lockdown Scenario Analysis. Front. Public Health 9:599280. doi: 10.3389/fpubh.2021.599280
Received: 26 August 2020; Accepted: 09 March 2021; Published: 08 April 2021.
Reviewed by:
Copyright © 2021 Sharma, Banstola and Parajuli. This is an open-access article distributed under the terms of the Creative Commons Attribution License (CC BY) . The use, distribution or reproduction in other forums is permitted, provided the original author(s) and the copyright owner(s) are credited and that the original publication in this journal is cited, in accordance with accepted academic practice. No use, distribution or reproduction is permitted which does not comply with these terms.
*Correspondence: Amrit Banstola, amritbanstola@outlook.com
Disclaimer: All claims expressed in this article are solely those of the authors and do not necessarily represent those of their affiliated organizations, or those of the publisher, the editors and the reviewers. Any product that may be evaluated in this article or claim that may be made by its manufacturer is not guaranteed or endorsed by the publisher.
- CBSE Class 10th
- CBSE Class 12th
- UP Board 10th
- UP Board 12th
- Bihar Board 10th
- Bihar Board 12th
Top Schools
- Top Schools in India
- Top Schools in Delhi
- Top Schools in Mumbai
- Top Schools in Chennai
- Top Schools in Hyderabad
- Top Schools in Kolkata
- Top Schools in Pune
- Top Schools in Bangalore
Products & Resources
- JEE Main Knockout April
- Free Sample Papers
- Free Ebooks
- NCERT Notes
- NCERT Syllabus
- NCERT Books
- RD Sharma Solutions
- Navodaya Vidyalaya Admission 2024-25
- NCERT Solutions
- NCERT Solutions for Class 12
- NCERT Solutions for Class 11
- NCERT solutions for Class 10
- NCERT solutions for Class 9
- NCERT solutions for Class 8
- NCERT Solutions for Class 7
- JEE Main 2024
- MHT CET 2024
- JEE Advanced 2024
- BITSAT 2024
- View All Engineering Exams
- Colleges Accepting B.Tech Applications
- Top Engineering Colleges in India
- Engineering Colleges in India
- Engineering Colleges in Tamil Nadu
- Engineering Colleges Accepting JEE Main
- Top IITs in India
- Top NITs in India
- Top IIITs in India
- JEE Main College Predictor
- JEE Main Rank Predictor
- MHT CET College Predictor
- AP EAMCET College Predictor
- GATE College Predictor
- KCET College Predictor
- JEE Advanced College Predictor
- View All College Predictors
- JEE Advanced Cutoff
- JEE Main Cutoff
- MHT CET Result 2024
- JEE Advanced Result
- Download E-Books and Sample Papers
- Compare Colleges
- B.Tech College Applications
- AP EAMCET Result 2024
- MAH MBA CET Exam
- View All Management Exams
Colleges & Courses
- MBA College Admissions
- MBA Colleges in India
- Top IIMs Colleges in India
- Top Online MBA Colleges in India
- MBA Colleges Accepting XAT Score
- BBA Colleges in India
- XAT College Predictor 2024
- SNAP College Predictor
- NMAT College Predictor
- MAT College Predictor 2024
- CMAT College Predictor 2024
- CAT Percentile Predictor 2024
- CAT 2024 College Predictor
- Top MBA Entrance Exams 2024
- AP ICET Counselling 2024
- GD Topics for MBA
- CAT Exam Date 2024
- Download Helpful Ebooks
- List of Popular Branches
- QnA - Get answers to your doubts
- IIM Fees Structure
- AIIMS Nursing
- Top Medical Colleges in India
- Top Medical Colleges in India accepting NEET Score
- Medical Colleges accepting NEET
- List of Medical Colleges in India
- List of AIIMS Colleges In India
- Medical Colleges in Maharashtra
- Medical Colleges in India Accepting NEET PG
- NEET College Predictor
- NEET PG College Predictor
- NEET MDS College Predictor
- NEET Rank Predictor
- DNB PDCET College Predictor
- NEET Result 2024
- NEET Asnwer Key 2024
- NEET Cut off
- NEET Online Preparation
- Download Helpful E-books
- Colleges Accepting Admissions
- Top Law Colleges in India
- Law College Accepting CLAT Score
- List of Law Colleges in India
- Top Law Colleges in Delhi
- Top NLUs Colleges in India
- Top Law Colleges in Chandigarh
- Top Law Collages in Lucknow
Predictors & E-Books
- CLAT College Predictor
- MHCET Law ( 5 Year L.L.B) College Predictor
- AILET College Predictor
- Sample Papers
- Compare Law Collages
- Careers360 Youtube Channel
- CLAT Syllabus 2025
- CLAT Previous Year Question Paper
- NID DAT Exam
- Pearl Academy Exam
Predictors & Articles
- NIFT College Predictor
- UCEED College Predictor
- NID DAT College Predictor
- NID DAT Syllabus 2025
- NID DAT 2025
- Design Colleges in India
- Top NIFT Colleges in India
- Fashion Design Colleges in India
- Top Interior Design Colleges in India
- Top Graphic Designing Colleges in India
- Fashion Design Colleges in Delhi
- Fashion Design Colleges in Mumbai
- Top Interior Design Colleges in Bangalore
- NIFT Result 2024
- NIFT Fees Structure
- NIFT Syllabus 2025
- Free Design E-books
- List of Branches
- Careers360 Youtube channel
- IPU CET BJMC
- JMI Mass Communication Entrance Exam
- IIMC Entrance Exam
- Media & Journalism colleges in Delhi
- Media & Journalism colleges in Bangalore
- Media & Journalism colleges in Mumbai
- List of Media & Journalism Colleges in India
- CA Intermediate
- CA Foundation
- CS Executive
- CS Professional
- Difference between CA and CS
- Difference between CA and CMA
- CA Full form
- CMA Full form
- CS Full form
- CA Salary In India
Top Courses & Careers
- Bachelor of Commerce (B.Com)
- Master of Commerce (M.Com)
- Company Secretary
- Cost Accountant
- Charted Accountant
- Credit Manager
- Financial Advisor
- Top Commerce Colleges in India
- Top Government Commerce Colleges in India
- Top Private Commerce Colleges in India
- Top M.Com Colleges in Mumbai
- Top B.Com Colleges in India
- IT Colleges in Tamil Nadu
- IT Colleges in Uttar Pradesh
- MCA Colleges in India
- BCA Colleges in India
Quick Links
- Information Technology Courses
- Programming Courses
- Web Development Courses
- Data Analytics Courses
- Big Data Analytics Courses
- RUHS Pharmacy Admission Test
- Top Pharmacy Colleges in India
- Pharmacy Colleges in Pune
- Pharmacy Colleges in Mumbai
- Colleges Accepting GPAT Score
- Pharmacy Colleges in Lucknow
- List of Pharmacy Colleges in Nagpur
- GPAT Result
- GPAT 2024 Admit Card
- GPAT Question Papers
- NCHMCT JEE 2024
- Mah BHMCT CET
- Top Hotel Management Colleges in Delhi
- Top Hotel Management Colleges in Hyderabad
- Top Hotel Management Colleges in Mumbai
- Top Hotel Management Colleges in Tamil Nadu
- Top Hotel Management Colleges in Maharashtra
- B.Sc Hotel Management
- Hotel Management
- Diploma in Hotel Management and Catering Technology
Diploma Colleges
- Top Diploma Colleges in Maharashtra
- UPSC IAS 2024
- SSC CGL 2024
- IBPS RRB 2024
- Previous Year Sample Papers
- Free Competition E-books
- Sarkari Result
- QnA- Get your doubts answered
- UPSC Previous Year Sample Papers
- CTET Previous Year Sample Papers
- SBI Clerk Previous Year Sample Papers
- NDA Previous Year Sample Papers
Upcoming Events
- NDA Application Form 2024
- UPSC IAS Application Form 2024
- CDS Application Form 2024
- CTET Admit card 2024
- HP TET Result 2023
- SSC GD Constable Admit Card 2024
- UPTET Notification 2024
- SBI Clerk Result 2024
Other Exams
- SSC CHSL 2024
- UP PCS 2024
- UGC NET 2024
- RRB NTPC 2024
- IBPS PO 2024
- IBPS Clerk 2024
- IBPS SO 2024
- Top University in USA
- Top University in Canada
- Top University in Ireland
- Top Universities in UK
- Top Universities in Australia
- Best MBA Colleges in Abroad
- Business Management Studies Colleges
Top Countries
- Study in USA
- Study in UK
- Study in Canada
- Study in Australia
- Study in Ireland
- Study in Germany
- Study in China
- Study in Europe
Student Visas
- Student Visa Canada
- Student Visa UK
- Student Visa USA
- Student Visa Australia
- Student Visa Germany
- Student Visa New Zealand
- Student Visa Ireland
- CUET PG 2024
- IGNOU B.Ed Admission 2024
- DU Admission 2024
- UP B.Ed JEE 2024
- LPU NEST 2024
- IIT JAM 2024
- IGNOU Online Admission 2024
- Universities in India
- Top Universities in India 2024
- Top Colleges in India
- Top Universities in Uttar Pradesh 2024
- Top Universities in Bihar
- Top Universities in Madhya Pradesh 2024
- Top Universities in Tamil Nadu 2024
- Central Universities in India
- CUET DU Cut off 2024
- IGNOU Date Sheet 2024
- CUET DU CSAS Portal 2024
- CUET Response Sheet 2024
- CUET Result 2024
- CUET Participating Universities 2024
- CUET Previous Year Question Paper
- CUET Syllabus 2024 for Science Students
- E-Books and Sample Papers
- CUET College Predictor 2024
- CUET Exam Date 2024
- CUET Cut Off 2024
- NIRF Ranking 2024
- IGNOU Exam Form 2024
- CUET PG Counselling 2024
- CUET Answer Key 2024
Engineering Preparation
- Knockout JEE Main 2024
- Test Series JEE Main 2024
- JEE Main 2024 Rank Booster
Medical Preparation
- Knockout NEET 2024
- Test Series NEET 2024
- Rank Booster NEET 2024
Online Courses
- JEE Main One Month Course
- NEET One Month Course
- IBSAT Free Mock Tests
- IIT JEE Foundation Course
- Knockout BITSAT 2024
- Career Guidance Tool
Top Streams
- IT & Software Certification Courses
- Engineering and Architecture Certification Courses
- Programming And Development Certification Courses
- Business and Management Certification Courses
- Marketing Certification Courses
- Health and Fitness Certification Courses
- Design Certification Courses
Specializations
- Digital Marketing Certification Courses
- Cyber Security Certification Courses
- Artificial Intelligence Certification Courses
- Business Analytics Certification Courses
- Data Science Certification Courses
- Cloud Computing Certification Courses
- Machine Learning Certification Courses
- View All Certification Courses
- UG Degree Courses
- PG Degree Courses
- Short Term Courses
- Free Courses
- Online Degrees and Diplomas
- Compare Courses
Top Providers
- Coursera Courses
- Udemy Courses
- Edx Courses
- Swayam Courses
- upGrad Courses
- Simplilearn Courses
- Great Learning Courses
Covid 19 Essay in English
Essay on Covid -19: In a very short amount of time, coronavirus has spread globally. It has had an enormous impact on people's lives, economy, and societies all around the world, affecting every country. Governments have had to take severe measures to try and contain the pandemic. The virus has altered our way of life in many ways, including its effects on our health and our economy. Here are a few sample essays on ‘CoronaVirus’.
100 Words Essay on Covid 19
200 words essay on covid 19, 500 words essay on covid 19.
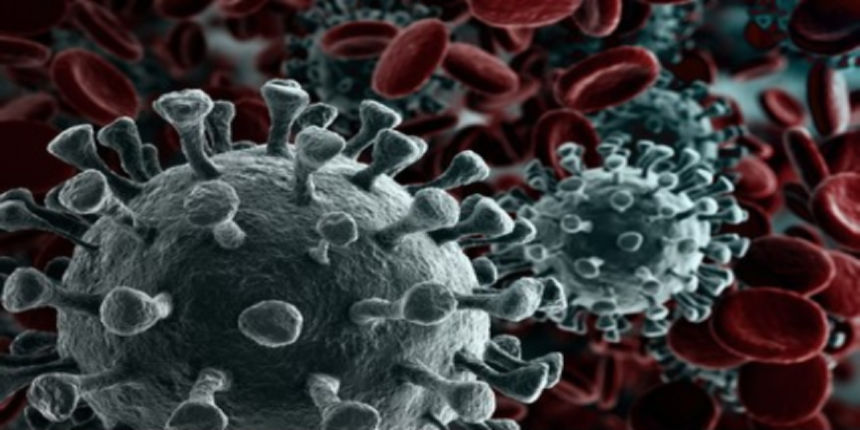
COVID-19 or Corona Virus is a novel coronavirus that was first identified in 2019. It is similar to other coronaviruses, such as SARS-CoV and MERS-CoV, but it is more contagious and has caused more severe respiratory illness in people who have been infected. The novel coronavirus became a global pandemic in a very short period of time. It has affected lives, economies and societies across the world, leaving no country untouched. The virus has caused governments to take drastic measures to try and contain it. From health implications to economic and social ramifications, COVID-19 impacted every part of our lives. It has been more than 2 years since the pandemic hit and the world is still recovering from its effects.
Since the outbreak of COVID-19, the world has been impacted in a number of ways. For one, the global economy has taken a hit as businesses have been forced to close their doors. This has led to widespread job losses and an increase in poverty levels around the world. Additionally, countries have had to impose strict travel restrictions in an attempt to contain the virus, which has resulted in a decrease in tourism and international trade. Furthermore, the pandemic has put immense pressure on healthcare systems globally, as hospitals have been overwhelmed with patients suffering from the virus. Lastly, the outbreak has led to a general feeling of anxiety and uncertainty, as people are fearful of contracting the disease.
My Experience of COVID-19
I still remember how abruptly colleges and schools shut down in March 2020. I was a college student at that time and I was under the impression that everything would go back to normal in a few weeks. I could not have been more wrong. The situation only got worse every week and the government had to impose a lockdown. There were so many restrictions in place. For example, we had to wear face masks whenever we left the house, and we could only go out for essential errands. Restaurants and shops were only allowed to operate at take-out capacity, and many businesses were shut down.
In the current scenario, coronavirus is dominating all aspects of our lives. The coronavirus pandemic has wreaked havoc upon people’s lives, altering the way we live and work in a very short amount of time. It has revolutionised how we think about health care, education, and even social interaction. This virus has had long-term implications on our society, including its impact on mental health, economic stability, and global politics. But we as individuals can help to mitigate these effects by taking personal responsibility to protect themselves and those around them from infection.
Effects of CoronaVirus on Education
The outbreak of coronavirus has had a significant impact on education systems around the world. In China, where the virus originated, all schools and universities were closed for several weeks in an effort to contain the spread of the disease. Many other countries have followed suit, either closing schools altogether or suspending classes for a period of time.
This has resulted in a major disruption to the education of millions of students. Some have been able to continue their studies online, but many have not had access to the internet or have not been able to afford the costs associated with it. This has led to a widening of the digital divide between those who can afford to continue their education online and those who cannot.
The closure of schools has also had a negative impact on the mental health of many students. With no face-to-face contact with friends and teachers, some students have felt isolated and anxious. This has been compounded by the worry and uncertainty surrounding the virus itself.
The situation with coronavirus has improved and schools have been reopened but students are still catching up with the gap of 2 years that the pandemic created. In the meantime, governments and educational institutions are working together to find ways to support students and ensure that they are able to continue their education despite these difficult circumstances.
Effects of CoronaVirus on Economy
The outbreak of the coronavirus has had a significant impact on the global economy. The virus, which originated in China, has spread to over two hundred countries, resulting in widespread panic and a decrease in global trade. As a result of the outbreak, many businesses have been forced to close their doors, leading to a rise in unemployment. In addition, the stock market has taken a severe hit.
Effects of CoronaVirus on Health
The effects that coronavirus has on one's health are still being studied and researched as the virus continues to spread throughout the world. However, some of the potential effects on health that have been observed thus far include respiratory problems, fever, and coughing. In severe cases, pneumonia, kidney failure, and death can occur. It is important for people who think they may have been exposed to the virus to seek medical attention immediately so that they can be treated properly and avoid any serious complications. There is no specific cure or treatment for coronavirus at this time, but there are ways to help ease symptoms and prevent the virus from spreading.
Applications for Admissions are open.

Aakash iACST Scholarship Test 2024
Get up to 90% scholarship on NEET, JEE & Foundation courses

JEE Main Important Physics formulas
As per latest 2024 syllabus. Physics formulas, equations, & laws of class 11 & 12th chapters

JEE Main Important Chemistry formulas
As per latest 2024 syllabus. Chemistry formulas, equations, & laws of class 11 & 12th chapters

TOEFL ® Registrations 2024
Accepted by more than 11,000 universities in over 150 countries worldwide

PTE Exam 2024 Registrations
Register now for PTE & Save 5% on English Proficiency Tests with ApplyShop Gift Cards

JEE Main high scoring chapters and topics
As per latest 2024 syllabus. Study 40% syllabus and score upto 100% marks in JEE
Download Careers360 App's
Regular exam updates, QnA, Predictors, College Applications & E-books now on your Mobile

Certifications
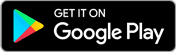
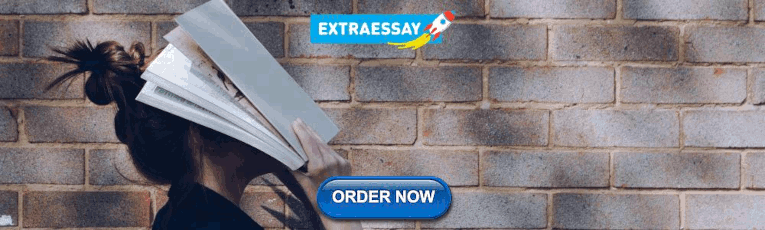
We Appeared in


45,000+ students realised their study abroad dream with us. Take the first step today
Meet top uk universities from the comfort of your home, here’s your new year gift, one app for all your, study abroad needs, start your journey, track your progress, grow with the community and so much more.

Verification Code
An OTP has been sent to your registered mobile no. Please verify
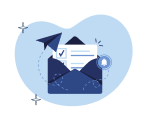
Thanks for your comment !
Our team will review it before it's shown to our readers.
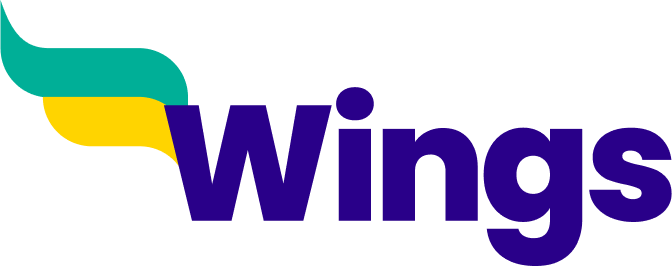
- School Education /
Essay On Covid-19: 100, 200 and 300 Words
- Updated on
- Apr 30, 2024

COVID-19, also known as the Coronavirus, is a global pandemic that has affected people all around the world. It first emerged in a lab in Wuhan, China, in late 2019 and quickly spread to countries around the world. This virus was reportedly caused by SARS-CoV-2. Since then, it has spread rapidly to many countries, causing widespread illness and impacting our lives in numerous ways. This blog talks about the details of this virus and also drafts an essay on COVID-19 in 100, 200 and 300 words for students and professionals.

Table of Contents
- 1 Essay On COVID-19 in English 100 Words
- 2 Essay On COVID-19 in 200 Words
- 3 Essay On COVID-19 in 300 Words
- 4 Short Essay on Covid-19
Essay On COVID-19 in English 100 Words
COVID-19, also known as the coronavirus, is a global pandemic. It started in late 2019 and has affected people all around the world. The virus spreads very quickly through someone’s sneeze and respiratory issues.
COVID-19 has had a significant impact on our lives, with lockdowns, travel restrictions, and changes in daily routines. To prevent the spread of COVID-19, we should wear masks, practice social distancing, and wash our hands frequently.
People should follow social distancing and other safety guidelines and also learn the tricks to be safe stay healthy and work the whole challenging time.
Also Read: National Safe Motherhood Day 2023
Essay On COVID-19 in 200 Words
COVID-19 also known as coronavirus, became a global health crisis in early 2020 and impacted mankind around the world. This virus is said to have originated in Wuhan, China in late 2019. It belongs to the coronavirus family and causes flu-like symptoms. It impacted the healthcare systems, economies and the daily lives of people all over the world.
The most crucial aspect of COVID-19 is its highly spreadable nature. It is a communicable disease that spreads through various means such as coughs from infected persons, sneezes and communication. Due to its easy transmission leading to its outbreaks, there were many measures taken by the government from all over the world such as Lockdowns, Social Distancing, and wearing masks.
There are many changes throughout the economic systems, and also in daily routines. Other measures such as schools opting for Online schooling, Remote work options available and restrictions on travel throughout the country and internationally. Subsequently, to cure and top its outbreak, the government started its vaccine campaigns, and other preventive measures.
In conclusion, COVID-19 tested the patience and resilience of the mankind. This pandemic has taught people the importance of patience, effort and humbleness.
Also Read : Essay on My Best Friend
Essay On COVID-19 in 300 Words
COVID-19, also known as the coronavirus, is a serious and contagious disease that has affected people worldwide. It was first discovered in late 2019 in Cina and then got spread in the whole world. It had a major impact on people’s life, their school, work and daily lives.
COVID-19 is primarily transmitted from person to person through respiratory droplets produced and through sneezes, and coughs of an infected person. It can spread to thousands of people because of its highly contagious nature. To cure the widespread of this virus, there are thousands of steps taken by the people and the government.
Wearing masks is one of the essential precautions to prevent the virus from spreading. Social distancing is another vital practice, which involves maintaining a safe distance from others to minimize close contact.
Very frequent handwashing is also very important to stop the spread of this virus. Proper hand hygiene can help remove any potential virus particles from our hands, reducing the risk of infection.
In conclusion, the Coronavirus has changed people’s perspective on living. It has also changed people’s way of interacting and how to live. To deal with this virus, it is very important to follow the important guidelines such as masks, social distancing and techniques to wash your hands. Getting vaccinated is also very important to go back to normal life and cure this virus completely.
Also Read: Essay on Abortion in English in 650 Words
Short Essay on Covid-19
Please find below a sample of a short essay on Covid-19 for school students:
Also Read: Essay on Women’s Day in 200 and 500 words
to write an essay on COVID-19, understand your word limit and make sure to cover all the stages and symptoms of this disease. You need to highlight all the challenges and impacts of COVID-19. Do not forget to conclude your essay with positive precautionary measures.
Writing an essay on COVID-19 in 200 words requires you to cover all the challenges, impacts and precautions of this disease. You don’t need to describe all of these factors in brief, but make sure to add as many options as your word limit allows.
The full form for COVID-19 is Corona Virus Disease of 2019.
Related Reads
Hence, we hope that this blog has assisted you in comprehending with an essay on COVID-19. For more information on such interesting topics, visit our essay writing page and follow Leverage Edu.
Simran Popli
An avid writer and a creative person. With an experience of 1.5 years content writing, Simran has worked with different areas. From medical to working in a marketing agency with different clients to Ed-tech company, the journey has been diverse. Creative, vivacious and patient are the words that describe her personality.
Leave a Reply Cancel reply
Save my name, email, and website in this browser for the next time I comment.
Contact no. *

Connect With Us
45,000+ students realised their study abroad dream with us. take the first step today..

Resend OTP in

Need help with?
Study abroad.
UK, Canada, US & More
IELTS, GRE, GMAT & More
Scholarship, Loans & Forex
Country Preference
New Zealand
Which English test are you planning to take?
Which academic test are you planning to take.
Not Sure yet
When are you planning to take the exam?
Already booked my exam slot
Within 2 Months
Want to learn about the test
Which Degree do you wish to pursue?
When do you want to start studying abroad.
January 2024
September 2024
What is your budget to study abroad?
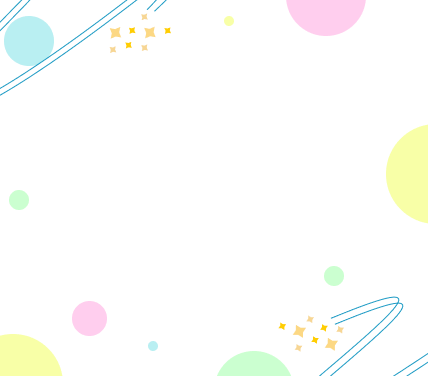
How would you describe this article ?
Please rate this article
We would like to hear more.
Have something on your mind?

Make your study abroad dream a reality in January 2022 with

India's Biggest Virtual University Fair

Essex Direct Admission Day
Why attend .

Don't Miss Out
- - Google Chrome
Intended for healthcare professionals
- My email alerts
- BMA member login
- Username * Password * Forgot your log in details? Need to activate BMA Member Log In Log in via OpenAthens Log in via your institution

Search form
- Advanced search
- Search responses
- Search blogs
- News & Views
- Twin crises in Nepal:...
Twin crises in Nepal: covid-19 and climate change
- Related content
- Peer review
- Basu Dev Pandey , doctor and professor 1 2 ,
- Kouichi Morita , professor 3 ,
- Anthony Costello , professor of global health and sustainable development 4
- 1 Everest International Clinic and Research Center, Kathmandu, Nepal,
- 2 Department of Molecular Epidemiology, Institute of Tropical Medicine, Nagasaki University, Japan
- 3 Department of Virology, Institute of Tropical Medicine, Nagasaki University, Japan
- 4 University College London, UK
Nepal has been on the frontline of both the covid-19 pandemic and climate change, and in both crises the response by the international community and Nepal’s government has been marked by a failure to prepare or to invest proactively in strong prevention measures.
The first case of covid-19 in Nepal was reported in January 2020 and the country’s modest first wave peaked in late October 2020 with a case fatality rate of less than 1%. 1 2 As cases fell steadily in January 2021, the government relaxed—a response that would turn out to be premature.
Throughout spring 2021, hundreds of thousands of people assembled on the streets in party political activities to prepare for the May election, adding to the number of people already joining gatherings for seasonal weddings and religious festivals. Meanwhile, in March 2021 the new delta variant, considered more infectious and virulent by the World Health Organization (WHO), was contributing to surging case numbers in India. 3 In April, at a time when cases in India were steadily rising, an estimated 50 000 Nepali pilgrims went to northern India for Kumbh Mela, a Hindu festival participated in by millions of people. 4 While there, many of the pilgrims caught covid-19.
Thousands of migrant workers also crossed over into Nepal from India, bringing the Delta strain with them, where it spread rapidly through the populous Kathmandu valley. 5 In Kathmandu, the hallways and courtyards of hospitals became crowded with patients competing to get a bed linked to an oxygen supply. Many patients were turned away due to lack of oxygen, ICU beds, and ventilators. Nepal’s president called a state of health emergency and 75 out of 77 districts had imposed a lockdown by 23 May. Nepal’s Ministry of Health and Population (MoHP) reported the country’s highest number of daily deaths (246) so far on 19 May 2021. 6
Restrictions were lifted as cases fell in July 2021 but the number of deaths surged again in August with a test positivity rate of 24% and more than 2500 cases recorded per day. 7 Many people in Nepal’s scattered rural population lack access to tests and deaths go unrecorded—a situation that is little different from India, where estimates suggest there were as many as 3.4-4.9 million excess deaths from the pandemic’s start to June 2021, numbers that are around 10-fold higher than official reports. 8 Hospitals and ICUs were full again during this period, including many severe cases in children. This wave hit the country so hard because communities were unprepared, the government had a false sense of security, residents relaxed social distancing, and authorities allowed religious festivals and political gatherings to go ahead as normal. The country still faces shortages of oxygen, ventilators, and other intensive care equipment.
Vaccines could have helped to relieve this pressure on Nepal’s hospitals, but the country has been beset by difficulties in obtaining them. At the end of March 2021, at a crucial point in the pandemic, India banned exports of AstraZeneca jabs until 2022. 9 This included one million doses already purchased by Nepal. By 11 December 2021, just 30% of the population had received two doses of the vaccine. 10 Nepal was not in a strong position then as the new omicron variant emerged and began to spread globally. First reported in Nepal by its MoHP in late December 2021, the omicron variant peaked with more than 10 000 daily cases on 18 January 2022. 11 12 More than 600 healthcare workers at the five biggest public hospitals in Kathmandu were infected and their absence added to the strain on the health system. 12 The number of daily deaths was much lower in the third wave (32 at its peak compared with 246 in the second wave) and cases dropped quickly to 2.9 per 100 000 population at the national level by the first week of March. 13 14 15 As before, the India-Nepal border was without strict screening, social distancing restrictions were relaxed, and compliance with public health protocols was often poor, all contributing to this third wave. But the introduction of covid-19 vaccination undoubtedly helped to reduce hospitalisations and mortality.
Against the backdrop of the covid-19 pandemic, another crisis unfolded. The annual monsoon season beginning in June 2021 brought flooding and landslides like never before across many of Nepal’s districts. Major rivers and streams swelled dangerously, and people lost their lives. After 200 mm of rain fell in six days up to 14 June, floods from the Melamchi river alone swept away 13 suspension bridges, seven motor bridges, and numerous stretches of road, destroying 337 houses, 259 enterprises, and thousands of acres of rice paddies. 16 The risk of flooding grows as glaciers in the Himalayas melt as a result of both rising temperatures and the proliferation of black carbon deposits from industry, vehicles, and cooking. 17
The government was overwhelmed, lacking as they do comprehensive plans for monsoon flooding. They mobilised the army and the Red Cross to assist communities, and arranged safe areas, drinking water, and food supplies. But they have no early warning systems, no anticipatory plans in place, and poor communication with many officials in local municipalities. A flood warning via Twitter was only sent out on 16 June—two days after dangerous flooding was first reported. 18 The country’s disaster budgets are tiny and procurement law does not allow relief materials and equipment to be bought in advance—only after disaster strikes subject to government approval. 19 Policies that build resilience against climate related disasters should be a top priority for the government because, while the pandemic may recede, the impacts of climate change will only worsen.
The latest reports from the Intergovernmental Panel on Climate Change have been red alerts for the world, describing climate change as widespread, rapid, and intensifying. 20 The mountain ranges of South Asia contain almost 55 000 glaciers that store more freshwater than anywhere but the North and South Poles. A World Bank report estimates that global black carbon emissions could be cut in half with policies that are currently economically and technically feasible, allied with the cuts in carbon emissions set out in the Paris Agreement. 21 Without urgent action, glacier melt will threaten hundreds of millions of people in Nepal, Afghanistan, India, Pakistan, China, and beyond.
As a low income country, Nepal deserves much greater international support through the Covax scheme to provide vaccines, diagnostics, and treatments, and for climate resilience through the “loss and damage” funds agreed at the COP26 summit in Glasgow. At the same time, Nepal’s government could have done far more to mobilise preventive and responsive measures for these twin crises; making sure the country is better prepared for the next one must be a priority.
Competing interests: None declared.
Provenance and peer review: Not commissioned; not peer reviewed.
- ↵ Bastola A, Sah R, Rodriguez-Morales A J, Kumar Lal B, et al. The first 2019 novel coronavirus case in Nepal. Lancet Infectious Diseases. 20:3:279 - 280. doi: 10.1016/S1473-3099(20)30067-0 OpenUrl CrossRef PubMed
- ↵ Ministry of Health and Population, Government of Nepal. Nepal: Covid 19 Response Situation Report No.XXXVI. Dec 14 2020. https://reliefweb.int/report/nepal/nepal-covid-19-response-situation-report-noxxxvi-14-december-2020
- ↵ Sharma G. Covid infections surge in Nepal fuelled by mutant strains in India. Reuters. 26 Apr 2021. https://www.reuters.com/world/china/covid-19-infections-surge-nepal-fueled-by-mutant-strains-india-2021-04-26/
- ↵ Pandey g. India Covid: Kumbh Mela pilgrims turn into super-spreaders. BBC News. 10 May 2021. https://www.bbc.com/news/world-asia-india-57005563
- Weissenbach B
- ↵ Nepal reports 242 new COVID cases, 1 death on Sunday. Nepal News Nationwide. 12 Dec 2021. https://www.nepalnews.com/s/nation/nepal-reports-242-new-covid-cases-1-death-on-sunday
- ↵ Update S. #68: Coronavirus Disease 2019 (COVID-19). WHO Country Office for Nepal. Reporting Date: 27 July – 2 August 2021. https://cdn.who.int/media/docs/default-source/nepal-documents/novel-coronavirus/who-nepal-sitrep/-68_weekly-who-nepal-situation-updates.pdf?sfvrsn=bb521cc0_5
- ↵ Anand A, Sandefur J, Subramanian A. Three New Estimates of India’s All-Cause Excess Mortality during the COVID-19 Pandemic. Centre for Global Development Working Paper. 20 Jul 2021. https://www.cgdev.org/publication/three-new-estimates-indias-all-cause-excess-mortality-during-covid-19-pandemic
- ↵ Gettleman J, Schmall E, Mashal M. India Cuts Back on Vaccine Exports as Infections Surge at Home. The New York Times. 25 Mar 2021. https://www.nytimes.com/2021/03/25/world/asia/india-covid-vaccine-astrazeneca.html
- ↵ Poudel A. Nepal receives 1 965 600 doses of Moderna vaccine from COVAX. The Kathmandu Post. 12 Dec 2021. https://kathmandupost.com/health/2021/12/12/nepal-receives-1-965-600-doses-of-moderna-vaccine-from-covax
- ↵ Poudel A. Nepal reports new Omicron case. The Kathmandu Post. 22 Dec 2021. https://kathmandupost.com/health/2021/12/22/nepal-reports-new-omicron-case-third-to-date
- ↵ Nepal faces new omicron-fueled coronavirus surge. Deutsche Welle. 19 Jan 2022. https://www.dw.com/en/nepal-faces-new-omicron-fueled-coronavirus-surge/a-60481393
- ↵ Omicron: Nepal makes quarantine mandatory for travellers arriving from 67 countries. The Times of India. 18 Dec 2021. https://timesofindia.indiatimes.com/articleshow/88352043.cms?utm_source=contentofinterest&utm_medium=text&utm_campaign=cppst
- ↵ Nepal Covid-19 tally: 3,540 new cases, 6,359 recoveries, 32 deaths in 24 hours. Onlinekhabar. 30 Jan 2022. https://english.onlinekhabar.com/nepal-covid-19-tally-3540-new-cases-6359-recoveries-32-deaths-in-24-hours.html
- ↵ World Health Organization Regional Office for South East Asia. COVID-19 Weekly Situation Report: Week #09 (03 March – 09 March 2022). 11 Mar 2022. https://cdn.who.int/media/docs/default-source/searo/whe/coronavirus19/sear-weekly-reports/searo-weekly-situation-report-9-2022.pdf?sfvrsn=f712043a_7
- ↵ Maharjan SB, Steiner JF, Bhakta Shrestha A, et al. The Melamchi flood disaster: Cascading hazard and the need for multihazard risk management. Disaster Task Force, ICIMOD. 4 Aug 2021. https://www.icimod.org/article/the-melamchi-flood-disaster/
- ↵ Mandal CK. Black carbon speeding up melting of glaciers, posing water scarcity threat to millions, report finds. The Kathmandu Post. 5 Jun 2021. https://kathmandupost.com/climate-environment/2021/06/05/black-carbon-speeding-up-melting-of-glaciers-posing-water-scarcity-threat-to-millions-report-finds
- ↵ Nepal Flood Alert. Twitter. 16 Jun 2021. https://twitter.com/DHM_FloodEWS/status/1405005910997540864
- ↵ Public Procurement Monitoring Office, Government Of Nepal. The Public Procurement Act, 2063 (2007). para 66 https://ppmo.gov.np/image/data/files/acts_and_regulations/public_procurement_act_2063.pdf
- ↵ Intergovernmental Panel on Climate Change (IPCC). Climate change widespread, rapid, and intensifying. IPCC Report. 9 Aug 2021 https://www.ipcc.ch/2021/08/09/ar6-wg1-20210809-pr/
- Paragraph Writing
- Paragraph Writing On Covid 19
Paragraph Writing on Covid 19 - Check Samples for Various Word Limits
The Covid-19 pandemic has been a deadly pandemic that has affected the whole world. It was a viral infection that affected almost everyone in some way or the other. However, the effects have been felt differently depending on various factors. As it is a virus, it will change with time, and different variants might keep coming. The virus has affected the lifestyle of human beings. The pandemic has affected the education system and the economy of the world as well. Many people have lost their lives, jobs, near and dear, etc.
Table of Contents
Paragraph writing on covid-19 in 100 words, paragraph writing on covid-19 in 150 words, paragraph writing on covid-19 in 200 words, paragraph writing on covid-19 in 250 words, frequently asked questions on covid-19.
Check the samples provided below before you write a paragraph on Covid-19.
Coronavirus is an infectious disease and is commonly called Covid-19. It affects the human respiratory system causing difficulty in breathing. It is a contagious disease and has been spreading across the world like wildfire. The virus was first identified in 2019 in Wuhan, China. In March, WHO declared Covid-19 as a pandemic that has been affecting the world. The virus was spreading from an infected person through coughing, sneezing, etc. Therefore, the affected people were isolated from everyone. The affected people were even isolated from their own family members and their dear ones. Other symptoms noticed in Covid – 19 patients include weariness, sore throat, muscle soreness, and loss of taste and smell.
Coronavirus, often known as Covid-19, is an infectious disease. It affects the human respiratory system, making breathing difficult. It’s a contagious disease that has been spreading like wildfire over the world. The virus was initially discovered in Wuhan, China, in 2019. Covid-19 was declared a global pandemic by the World Health Organization in March. The virus was transferred by coughing, sneezing, and other means from an infected person. As a result, the people who were affected were isolated from the rest of society. The folks who were afflicted were even separated from their own family members and loved ones. Weariness, sore throat, muscle stiffness, and loss of taste and smell are among the other complaints reported by Covid-19 individuals. Almost every individual has been affected by the virus. A lot of people have lost their lives due to the severity of the infections. The dropping of oxygen levels and the unavailability of oxygen cylinders were the primary concerns during the pandemic.
The Covid-19 pandemic was caused due to a man-made virus called coronavirus. It is an infectious disease that has affected millions of people’s lives. The pandemic has affected the entire world differently. It was initially diagnosed in 2019 in Wuhan, China but later, in March 2020, WHO declared that it was a pandemic that was affecting the whole world like wildfire. Covid-19 is a contagious disease. Since it is a viral disease, the virus spreads rapidly in various forms. The main symptoms of this disease were loss of smell and taste, loss of energy, pale skin, sneezing, coughing, reduction of oxygen level, etc. Therefore, all the affected people were asked to isolate themselves from the unaffected ones. The affected people were isolated from their family members in a separate room. The government has taken significant steps to ensure the safety of the people. The frontline workers were like superheroes who worked selflessly for the safety of the people. A lot of doctors had to stay away from their families and their babies for the safety of their patients and their close ones. The government has taken significant steps, and various protocols were imposed for the safety of the people. The government imposed a lockdown and shut down throughout the country.
The coronavirus was responsible for the Covid-19 pandemic. It is an infectious disease that has affected millions of people’s lives. The pandemic has impacted people all across the world in diverse ways. It was first discovered in Wuhan, China, in 2019. However, the World Health Organization (WHO) proclaimed it a pandemic in March 2020, claiming that it has spread throughout the globe like wildfire. The pandemic has claimed the lives of millions of people. The virus had negative consequences for those who were infected, including the development of a variety of chronic disorders. The main symptoms of this disease were loss of smell and taste, fatigue, pale skin, sneezing, coughing, oxygen deficiency, etc. Because Covid-19 was an infectious disease, all those who were infected were instructed to segregate themselves from those who were not. The folks who were affected were separated from their families and locked in a room. The government has prioritised people’s safety. The frontline personnel were like superheroes, working tirelessly to ensure the public’s safety. For the sake of their patients’ and close relatives’ safety, many doctors had to stay away from their families and babies. The government had also taken significant steps and implemented different protocols for the protection of people.
What is meant by the Covid-19 pandemic?
The Covid-19 pandemic was a deadly pandemic that affected the lives of millions of people. A lot of people lost their lives, and some people lost their jobs and lost their entire families due to the pandemic. Many covid warriors, like doctors, nurses, frontline workers, etc., lost their lives due to the pandemic.
From where did the Covid-19 pandemic start?
The Covid-19 pandemic was initially found in Wuhan, China and later in the whole world.
What are the symptoms of Covid-19?
The symptoms of Covid-19 have been identified as sore throat, loss of smell and taste, cough, sneezing, reduction of oxygen level, etc.
ENGLISH Related Links | |
Leave a Comment Cancel reply
Your Mobile number and Email id will not be published. Required fields are marked *
Request OTP on Voice Call
Post My Comment

Register with BYJU'S & Download Free PDFs
Register with byju's & watch live videos.

An official website of the United States government
The .gov means it’s official. Federal government websites often end in .gov or .mil. Before sharing sensitive information, make sure you’re on a federal government site.
The site is secure. The https:// ensures that you are connecting to the official website and that any information you provide is encrypted and transmitted securely.
- Publications
- Account settings
Preview improvements coming to the PMC website in October 2024. Learn More or Try it out now .
- Advanced Search
- Journal List
- Comput Intell Neurosci
- v.2021; 2021

Deep Learning-Based Methods for Sentiment Analysis on Nepali COVID-19-Related Tweets
1 Department of Electrical and Computer Systems Engineering, Monash University, VIC, Clayton, 3800, Australia
2 Central Department of Computer Science and Information Technology, Tribhuvan University, Kathmandu 44600, Nepal
3 Kist College, Kathmandu, Nepal
4 Aryan School of Engineering, Kathmandu, Nepal
T. B. Shahi
5 School of Engineering and Technology, Central Queensland University, Rockhampton, QLD 4701, Australia
Associated Data
Data and source code used to support the findings of the study are available from the corresponding author upon request.
COVID-19 has claimed several human lives to this date. People are dying not only because of physical infection of the virus but also because of mental illness, which is linked to people's sentiments and psychologies. People's written texts/posts scattered on the web could help understand their psychology and the state they are in during this pandemic. In this paper, we analyze people's sentiment based on the classification of tweets collected from the social media platform, Twitter, in Nepal. For this, we, first, propose to use three different feature extraction methods—fastText-based (ft), domain-specific (ds), and domain-agnostic (da)—for the representation of tweets. Among these three methods, two methods (“ds” and “da”) are the novel methods used in this study. Second, we propose three different convolution neural networks (CNNs) to implement the proposed features. Last, we ensemble such three CNNs models using ensemble CNN, which works in an end-to-end manner, to achieve the end results. For the evaluation of the proposed feature extraction methods and CNN models, we prepare a Nepali Twitter sentiment dataset, called NepCOV19Tweets, with 3 classes (positive, neutral, and negative). The experimental results on such dataset show that our proposed feature extraction methods possess the discriminating characteristics for the sentiment classification. Moreover, the proposed CNN models impart robust and stable performance on the proposed features. Also, our dataset can be used as a benchmark to study the COVID-19-related sentiment analysis in the Nepali language.
1. Introduction
Sentiment analysis is the process of identifying the polarity of documents into different classes, such as positive, negative, and neutral. It has a wider range of application areas such as opinion mining, recommendation systems, and health informatics. Based on the opinion of people, businesses make strategic plans to improve their services, thereby increasing profits. Similarly, based on the analysis of tweets, people can assess human psychology and their behaviors, which are linked to their health status. For the sentiment analysis, researchers have been using different kinds of textual documents such as Facebook posts and tweets. In this study, we study recent COVID-19 tweets related to sentiment classification works around the world. However, there are no such existing works in Nepali language to this date.
Existing works [ 2 – 14 ] related to COVID-19 tweets' analysis in other languages apart from the Nepali language not only underscore the efficacy of sentiment analysis but also support the role of traditional machine learning and deep learning algorithms on sentiment analysis tasks. In most of these works, they prefer using deep learning-based methods for sentiment analysis. Similarly, regarding the analysis of such tweets, researchers are also focusing on different aspects such as topic modeling and sentiment classification. Here, they prefer using the syntactic-based feature representation methods such as Term-Frequency and Inverse Document-Frequency (TF-IDF) method mostly.
There are three main limitations in the aforementioned works. First, most of the existing works [ 2 , 4 , 5 , 12 ] on COVID-19-related tweets are performed in high-resource languages such as English and Arabic. The approach used by high-resource language might be inapplicable to low-resource languages such as Nepali, which is based on Devanagari script and has 36 consonants (33 are distinct consonants and 3 are combined consonants), 13 vowels, and 10 numerals ( Figure 1 ) [ 1 , 15 , 16 ]. Second, their investigation mainly targets either clustering the tweets into various themes/topics or classifying their polarity into three classes (negative, positive, or neutral) using the well-established feature extraction methods such as BERT, Word2Vec, and Glove. However, these feature extraction methods might be insufficient to capture the semantic information of textual documents or tweets written in the Nepali language. Third, existing methods [ 17 , 18 ] capture the unimodal information only (e.g., bag-of-words using TF-IDF approach), which is insufficient to discriminate the complex tweets requiring the complementary semantic information (e.g., contextual) during classification. Also, their methods might not be appropriate to tweet dataset having a fewer number of tokens.

Nepali alphabets and numerals [ 1 ].
Given the limitations of existing works, we propose to use three kinds of feature extraction methods (fastText-based method, domain-specific method, and domain-agnostic method) for Nepali tweets' representation. First, with the help of the pretrained fastText-based method [ 19 ], we capture the semantic information. Here, it imparts a 300-D sized embedding vector for each word. Second, the domain-specific method helps to preserve the focused semantic information of the particular domain. For example, semantic features based on the COVID-19-related tweets could learn more informative features. For this, we employ the probabilistic feature extraction approach as suggested by Sitaula et al. [ 1 ] recently, which calculates the probability of each input word across all categories and finally and attains the feature vector depending on the number of categories present in the dataset. Last, with the help of the domain-agnostic method, we capture the semantic information using the cross-domain approach, which means that we transfer the knowledge to current COVID-19 domain from another domain such as news categories. For this, we follow a similar probabilistic approach as in the domain-specific method to extract this kind of feature. Furthermore, given the prominent classification abilities of deep learning methods in natural language processing, we propose four different CNN models to carry out the sentiment analysis of COVID-19 tweets written in the Nepali language. The first CNN model is used to train the contextual information achieved from the pretrained fastText [ 19 ] model. The second CNN model is used to train the domain-specific feature vectors. The third CNN model is used to train the domain-agnostic feature vectors. Since three different CNN models yield three different decision scores based on the corresponding information for each tweet, we, finally, aggregate them to include all three information using the ensemble CNN model.
In summary, our paper has the following contributions:
- We prepare a public Nepali COVID-19 tweets' dataset, called NepCOV19Tweets, for COVID-19-related sentiment analysis in the Nepali language. This dataset can be used as a benchmark in Nepali COVID-19 tweets related to sentiment analysis research.
- To the best of our knowledge, this is the first work of sentiment analysis on Nepali COVID-19 tweets with three classes.
- We propose to use three different feature extraction methods—fastText-based feature extraction (ft), domain-specific probability-based (ds), and domain-agnostic probability-based (da) feature extraction—for the representation of tweets written in the Nepali language. Among these three methods, two methods (“ds” and “da”) are novel feature extraction methods used in this study.
- We propose three different CNN models for the sentiment classification of tweets using three different feature extraction methods based on fs, ds, and da, respectively. In addition, for the end results, we design an ensemble CNN model that captures the three different information on the fly.
- We validate our proposed methods against traditional machine learning methods and state-of-the-art methods on Nepali COVID-19 tweets' dataset (NepCOV19Tweets). The experimental results on such dataset show that our method produces a stable and promising classification performance.
The rest of this paper is organized as follows. Section 2 studies the related works of COVID-19-related tweets' classification. Similarly, Section 3 explains the materials, proposed methods, and implementation. Furthermore, Section 4 discusses the results and compares them with the existing state-of-the-art methods, and Section 5 concludes the paper with limitations and future works.
2. Related Works
Under the COVID-19 tweets' analysis, several works have been carried out around the world [ 2 – 14 ] in a short span of time. Most of the research works are based on high-resource languages such as English.
Boon et al. [ 2 ] proposed a sentiment analysis and topic modeling study on COVID-19 tweets to increase the understanding of its trend and concern. They analyzed COVID-19-related 107,990 tweets extracted from 13 December to 9 March 2020. Their results indicate that the people have a negative outlook towards COVID-19 and express them into three different themes such as COVID-pandemic, Control, and Reports. Another work related to the classification of tweets into either positive or negative was carried out by Nemes et al. [ 3 ] using the recurrent neural network (RNN). They established their tweets' dataset based on COVID-19 with four fine-grained classes: weak positive, strong positive, weak negative, and strong negative. Their method outperforms TextBlob [ 22 ]. Furthermore, Manguri et al. [ 4 ] collected a seven-day tweets related to COVID-19 and performed sentiment analysis using TextBlob [ 22 ]. Their results show that 60% of tweets are about “smooth and relaxed,” whereas 13% of tweets are related to “feeling hopeful. ” On the other hand, only 7% are related to “relieved mood. ” Similarly, Naseem et al. [ 5 ] compared the traditional machine learning methods such as support vector machine (SVM), Naive Bayes (NB), decision tree (DT), random forest (RF), and deep learning methods such as convolution neural network (CNN), and bidirectional long short-term memory (BiLSTM), in combination with various embedding vectors such as fastText [ 19 ], Glove [ 23 ], and Word2Vec [ 24 ] on their COVID-19 tweets' datasets into three classes (negative, positive, and neutral). Their results depict that the deep learning (DL)-based methods outperform the traditional ML methods. They also conducted the fine tuning of the transformer-based learning methods, such as BERT [ 25 ], DistilBERT [ 26 ], XLNET [ 27 ], and ALBERT [ 28 ], where the highest accuracy of 92.90% was achieved by ALBERT.
Furthermore, Rustam et al. [ 7 ] compared the performance of five machine learning algorithms: random forest, extra tree classifier, XGBoost classifier, decision tree, and long short-term memory (LSTM) for COVID-19 tweet sentiment classification into three classes: positive, negative, and neutral. For this, they utilized two widely used feature extraction methods: Bag-of-Words (BOW) and Term-Frequency and Inverse Document Frequency (TF-IDF). Their results show that the extra tree classifier with an accuracy of 93.00% outperforms all remaining classifiers. In the meantime, Basiri et al. [ 8 ] proposed the ensemble deep learning method to classify tweets sentiment using five base learners: Naive Bayes support vector machine (NBSVM), CNN, BiGRU, FastText-based model, and DistilBERT-based model. Their results show that the stacked ensemble method outperforms all other methods with an accuracy of 85.8%. Similarly, Kaur et al. [ 9 ] created a dataset of COVID-19 tweets using five different hashtags such as “#COVID-19”, “#coronavirus,” “#deaths,” “#new case,” and “#recovered. ” They compared the performance of a hybrid heterogeneous support vector machine (SVM) with the recurrent neural network (RNN) for tweets' sentiment classification into three classes (positive, negative, and neutral). Their results show that SVM classifies more tweets into neutral class, whereas RNN categorizes more tweets into positive class. A long short-term memory (LSTM) model was employed by Imran et al. [ 11 ] to access the sentiment polarity of people from different cultures to the coronavirus using COVID-19-related tweets dataset, called Sentiment140. Their results show a higher correlation between the USA and Canada (0.96 for positive and 0.97 for negative sentiments) and between India and Pakistan (0.81 for positive and 0.86 for negative sentiments). However, a low correlation between Norway and Sweden (0.50 for negative and 0.40 for positive sentiments) exists. Furthermore, sentiment analysis on 13.9 million COVID-19-related tweets was conducted by Chandrasekaran et al. [ 12 ], which identified the trends and variations of COVID-19-related tweets, key topics, and associated sentiments before and after the pandemic. They inferred 26 topics on COVID-19-related tweets using latent Dirichlet analysis (LDA) and grouped them into 10 broader themes such as the impact of COVID-19 into spread and growth in cases, impact on economy and market, treatment and recovery, and impact on the health sector and governance response. Their result shows that average negative sentiments are seen on themes such as the growth of cases, whereas positive sentiments are seen on themes such as prevention, impact on the economy and market, government response, and treatment and recovery theme. Meanwhile, a public discourse and sentiment analysis of tweets related to COVID-19 in English language was analyzed using machine learning approaches by Xue et al. [ 29 ]. In their work, a total of eleven COVID-19-related topics were extracted using latent Dirichlet analysis (LDA) from all tweets collected between 23 January 2020 and 7 March 2020. Their result shows that the sentiment of fear is dominant in all topics.
Furthermore, Satu et al. [ 10 ] proposed a cluster-based classification and topic modeling (TClustVID), which first clusters the tweets into different clusters, each cluster containing positive, negative, and neutral classes. They performed classification using four classifiers such as decision tree, logistic regression, multilayer perception, and random forest. The evaluation results show that the second cluster produces the highest accuracy of 98.80% while comparing with baseline classifiers. Moreover, Aljameel et al. [ 14 ] conducted the sentiment analysis of COVID-19 based on tweets in the Arabic language. They investigated the uni-gram and bigram-based TF-IDF features with various classifiers such as SVM, KNN, and Naive Bayes. Their results show that the SVM classifier achieves the highest accuracy of 85% while using bigram TF-IDF-based features. Similarly, De et al. [ 30 ] performed the sentiment analysis of COVID-19 on tweets and the news article datasets in Brazil. A study on topic modeling and sentiment analysis of Brazilian text from news articles and tweets was presented in [ 30 ]. Their results show that both Twitter and news media provide similar kinds of information related to sentiment classification. Last but not the least, Ramya et al. [ 21 ] adapted logistic regression and Naive Bayes classifiers to analyze sentiments of COVID-19-related tweets into positive, negative, and neutral. Their result shows that logistic regression and Naive Bayes impart 91% and 74% accuracy, respectively. The sample summary of the performance of the few latest methods is presented in Table 1 .
Summary performance (%) of sentiment classification of some state-of-the-art methods on tweets.
Method | Dataset | Accuracy (%) | Methodology | Limitations |
---|---|---|---|---|
Basir et al. [ ] | StanfordSentiment140 [ ] | 85.4 | (i) Stacked ensemble method | (i) Ignore the effects of global COVID-19 news on sentiment analysis beside the specific country |
Rustam et al. [ ] | Covid-19Tweets [ ] | 93.0 | (ii) BoW with various ML methods | (ii) Limited performance on small datasets |
Aljameel et al. [ ] | Self-created dataset | 85.0 | (iii) N-gram with various ML methods | (iii) Feature selection and hyperparameter tuning operation is not performed |
Ramya et al. [ ] | Self-created dataset | 91.0 | (iv) Naive Bayes | (iv) Experimented with a limited data |
Naseem et al. [ ] | COVIDSenti [ ] | 92.2 | (v) ML methods such as Naive Bayes, support vector machine, and random forest | (v) Limited to English tweets |
Satu et al. [ ] | Covid-19Tweets [ ] | 98.8 | (vi) Cluster-based classification | (vi) Tweets search limited to a few keywords in English text |
Considering that there are no well-established Nepali COVID-19 tweets' classification works conducted in the literature, we report some recent Nepali text/news document classification works in this study. Initially, Shahi et al. [ 31 ] classified Nepali documents using a support vector machine (SVM), which provides 74.65% accuracy. However, their method still has insufficient datasets for the evaluation. Similarly, Basnet and Timalsina [ 32 ] classified Nepali documents using the long short-term memory (LSTM) model, which provides 84.63% classification accuracy. Compared to Shahi et al., their method imparts a higher accuracy. However, their method still suffers from the problem of overfitting because of the lower amount of datasets. More recently, Sitaula et al. [ 1 ] proposed a supervised codebook approach and classified Nepali documents using a traditional machine learning classifier, called support vector machine (SVM), which reports 77.46%, 67.53%, 80.54%, and 89.58% accuracy on four different Nepali news datasets. Although they used four datasets in their study for the evaluation compared to other existing works, their method still suffers from the computational complexities triggered by the supervised codebook used in their work.
3. Materials and Methods
3.1. dataset.
We collect tweets from 11 Feb 2020 to 10 Jan 2021 using the geo-location of Nepal. To search the tweets on Twitter, we use only one keyword, called #COVID-19 (in the Nepali language). Each tweet is preprocessed and annotated by four annotators (co-authors) to set the sentiment labels using majority voting approach. The detailed statistics of our dataset is presented in Table 2 .
Our NepCOV19Tweets dataset statistics.
Class | No. of raw tokens | No. of raw tweets | No. of clean tokens | No. of clean tweets |
---|---|---|---|---|
Positive | 291,593 | 14,982 | 198,504 | 14,957 |
Neutral | 85,175 | 4860 | 55,679 | 4744 |
Negative | 247,548 | 13,593 | 162,669 | 13,546 |
Total | 624,316 | 33,435 | 416,852 | 33,247 |
3.2. Evaluation Metrics
We present the performance metrics used in our study. For the performance evaluation, we utilize widely popular metrics such as Precision (equation ( 1 )), Recall (equation ( 2 )), F1-score (equation ( 3 )), and Accuracy (equation ( 4 )):
where TP, TN, FP, and FN represent true positive, true negative, false positive, and false negative, respectively. Similarly, P , R , F , and A represent Precision, Recall, F1-score, and Accuracy, respectively.
3.3. Machine Learning Algorithms
We discuss eight traditional machine learning (ML) algorithms used in our work. They are support vector machine (SVM) with both linear and RBF (radial basis function) kernels, XGBoost (eXtreme gradient boosting), ANN (artificial neural networks), RF (random forest), NB (Naive Bayes), LR (logistic regression), and K-NN (K-nearest neighbors).
3.3.1. Support Vector Machine (SVM)
Basically, the support vector machine (SVM) is a binary classifier [ 33 ], which learns to optimize a hyperplane defined in equation ( 5 ) using training data:
where x is a feature vector, w is a weight vector, and b is a bias. When the data are not linearly separable, the SVM uses kernel trick that implicitly maps the input features in another feature space (usually of higher dimension). The popular kernel functions used in various implementation of SVM are listed in equations ( 6 )–( 8 ) for linear, polynomial, and radial basis function (RBF) kernel, respectively [ 34 ]. Note that we use the linear and RBF kernel in this work, and classification results with these kernels are presented in Table 3 .
where K ( x i , x j ) = ϕ ( x i ). ϕ ( x j ). Similarly, d and γ > 0 denote degree of polynomial and free parameter, respectively.
Comparison of our method with existing machine learning algorithms in terms of classification performance (%).
Algorithms | Ft | da | ds | |||||||||
---|---|---|---|---|---|---|---|---|---|---|---|---|
SVM + Linear | 67.1 | 62.2 | 62.2 | 63.9 | 57.3 | 44.0 | 44.0 | 47.2 | 54.1 | 54.1 | 56.3 | |
SVM + RBF | 51.0 | 51.0 | 40.2 | 58.5 | 53.9 | 53.9 | 55.5 | 63.2 | 51.5 | 51.5 | 54.6 | |
XGBoost | 69.0 | 58.3 | 59.8 | 59.8 | 56.3 | 61.5 | 58.9 | |||||
ANN | 63.1 | 63.7 | 63.7 | 63.4 | 56.8 | 58.8 | 58.8 | 54.7 | 62.0 | 61.9 | 61.9 | 58.0 |
RF | 69.6 | 67.5 | 67.5 | 63.5 | 59.9 | 61.9 | 61.9 | 59.5 | ||||
NB | 59.4 | 56.1 | 56.1 | 57.5 | 47.0 | 48.7 | 48.7 | 44.9 | 48.5 | 50.0 | 50.0 | 45.8 |
LR | 65.1 | 67.4 | 67.4 | 64.7 | 54.2 | 56.6 | 56.6 | 52.0 | 63.2 | 61.8 | 61.8 | |
K-NN | 65.2 | 65.2 | 65.2 | 60.3 | 51.8 | 57.5 | 57.5 | 52.8 | 61.3 | 61.6 | 61.6 | 57.4 |
Note that P , R , F , and A denote overall Precision, Recall, F1-score, and Accuracy for three types of embeddings (ft: fastText, da: domain-agnostic, and ds: domain-specific), respectively. The hyperparameters of traditional machine learning algorithms are as follows: SVM + Linear ( c : 1, Gamma: 0.1), SVM + RBF ( c : 100, Gamma: 0.1), XGBoost (learning-rate: 0.1, max-depth: 7, n -estimators: 150), ANN (Hidden-layer-size: 20, learning-rate-init: 0.01, max-iter: 1000), RF (min-sample-leaf: 3, min-sample-split: 6, n -estimators: 200), LR ( C : 10, solver: lbfgs, max-iter: 1000), and K-NN (leaf-size: 35, n -neighbor: 120, p: 1). Boldface denotes the highest performance.
3.3.2. XGBoost
XGBoost [ 35 ] is an ensemble-based tree boosting algorithm designed for large-scale machine learning applications. A group of weak learners are combined into strong learners using two methods: bagging and boosting. In gradient boosting technique, a new base learner is constructed in such a way that it will be maximally correlated with the negative gradient of the loss function with the whole ensemble in each iteration [ 36 ]. The XGBoost uses three kinds of boosting—gradient boosting, regularized boosting, and stochastic boosting—to surge the performance, reduce the computation time, and save the memory resources [ 37 ].
3.3.3. Artificial Neural Network (ANN)
The artificial neural network is a combination of highly interconnected processing elements, known as neurons or nodes, aligned in three types of layers: one input layer, one or more hidden layers, and one output layer [ 31 ]. Each node represents a function that maps a weighted combination of input to activation of the neurons. An input vector is presented to the network, and its corresponding output is calculated. The weights are adjusted with optimization techniques such as “Adam,” “AdaGrad,” and “RMSProp” along with a backpropagation to compute the gradient of the loss function [ 38 ].
3.3.4. Random Forest (RF)
Random forest is a popular learning algorithm that is based on the ensemble of decision trees with bagging approaches [ 39 ]. It starts with a decision tree by drawing a random sample of training data as a subset and creates a forest of decision tree classifiers. The size of the subsample is always the same, but the samples are drawn with replacement. A decision tree that is organized in the hierarchy is constructed through the binary partition starting from a root to a leaf node in the tree. Once the tree is fully formed, the validation data points traverse through the tree following a specific path and reach a leaf node that gives the corresponding output value. Finally, the output from the forest of trees is averaged to get the final output for a data point [ 40 ].
3.3.5. Naive Bayes (NB)
A Naive Bayes classifier is based on the Bayes theorem of probability with strong independence assumptions between every pair of input features [ 41 ]. For input feature vector x = ( x 1 ,…, x n ), given the class y , the estimation of probability distribution P ( x i / y ) in equation ( 9 ) defines the various types of Naive Bayes classifiers. For instance, multinomial Naive Bayes estimate the distribution parameters by a smoothed version of maximum likelihood or relative frequency counting. In this work, we use multinomial Naive Bayes implemented in Scikit-learn [ 34 ]:
3.3.6. Logistic Regression (LR)
It is an extension of the linear regression model for the classification problems [ 42 ]. Instead of fitting straight line as in linear regression, the logistic regression uses the function defined in equation ( 10 ) to squeeze the output between 0 and 1 as the probabilities:
In this work, we use multinomial logistic regression with L2-regularization [ 43 ] as our COVID-19 tweets' sentiment classification is multiclass problem.
3.3.7. K-Nearest Neighbor (K-NN)
K-nearest neighbor (KNN) is a nonparametric learning algorithm based on the simple but intuitive idea, where similar objects are within the closest proximity. It calculates the distance between a predefined number of training samples and the new query point to predict a label for a new point based on the majority. It is a kind of lazy learner that simply remembers all of its training data, and thus, it is nongeneralized. The common distance functions used are Euclidean distance, Manhattan distance, and Minkowski distance as defined in equation ( 11 )–( 13 ), respectively:
where p represents the order of Minkowski distance and x i and y i represent two points on k -dimensional coordinate space.
3.4. Proposed Approach
In our method, we follow three different steps for the COVID-19-related tweets' sentiment classification, namely, “embedding vector extraction and representation,” “CNNs design and training,” and “decision fusion.”
3.5. Embedding Vector Extraction and Representation
Before extracting the embedding vector of each word in the tweets, we preprocess each raw token (or word) using the following method. First, we tokenize and remove alphanumeric characters from the each tweet. Second, we remove stop words using a rule-based approach. Last, we apply a Stemmer to attain the root word of each token. In summary, we follow a similar preprocessing approach as suggested in previous work [ 1 ].
For the extraction of the embedding vector of each clean token ( n i ), we adapt three different kinds of embedding vectors: fastText-based word embedding, domain-specific probability-based embedding, and domain-agnostic probability-based embedding. Here, feature selection is an important step in feature engineering to extract the discriminating features as suggested in [ 44 ]; however, we do not perform it in our work as our compact features already possess a lower-sized discriminating characteristic for the better classification.
First, we use fastText-based embedding vector (ft) [ 19 ], which has been pretrained with multilingual datasets [ 1 ]. This produces the contextual features based on the important clues related to each token. The size of the feature vector is 300-D. In this way, we represent each tweet as n × 300-sized matrix and perform average-pooling operation.
Second, we use the cross-domain dataset, Nepalinewsdataset [ 1 ], for the domain-agnostic probability-based (da) embedding vector extraction. This helps capture the cross-domain (non-COVID-19 related documents) information related to each token. For this, we first design the list of tokens, also called filterbank, for each category. Then, we calculate the probability of each token for each list as a feature value. For example, if there are 17 categories, the feature size of each token will be 17-D. In our work, Nepalinewsdataset has 17 categories, which, therefore, impart a 17-D sized feature vector for each token. This results in n × 17 matrix for each tweet, resulting in a 17-D vector after the average-pooling operation.
Third, we use the domain-specific probability-based approach (ds) to extract the embedding vector for each token as suggested by Sitaula et al. [ 1 ]. This helps capture the domain-specific (COVID-19 tweets' related) information corresponding to each token. Since our COVID-19 tweets' dataset has 3 classes, it provides a 3D feature vector for each token. In this way, we achieve each tweet as a matrix n × 3 tensor and then perform average-pooling operation.
3.6. CNNs' Design and Training
After the representation of each tweet, we design three different CNN models corresponding to each embedding type (“ft,” “ds,” and “da”). CNN models have been extensively used in different domains such as tweets' classification [ 45 ], scene image representation [ 46 ], remote-sensing image fusion [ 47 ], and biomedical image analysis [ 48 ]. It has produced a ground-breaking performance compared to the traditional approaches. With the help of several intermediate layers with convolution and pooling operations, it has been able to capture the discriminating information of several kinds of input data, including images and texts.
Here, we design simple, yet efficient, CNN models in our study. Three separate CNN models (equations ( 14 )–( 16 )) are designed for fastText-based embedding (ft), domain-specific probability-based embedding (ds), and domain-agnostic-based embedding (da), respectively:
where x , CNN ft , CNN ds , and CNN da denote the input tensor, CNN using fastText embedding, CNN using domain-specific probability embedding, and CNN using domain-agnostic probability embedding, respectively. The detailed architecture of each CNN used in our study is presented in Table 4 .
The architecture of three CNNs proposed in our work.
Layer | CNN | CNN | CNN | |||
---|---|---|---|---|---|---|
( , ) | Output shape | ( , ) | Output shape | ( , ) | Output shape | |
Input | — | (17, 1) | — | (3, 1) | — | (300, 1) |
Conv1D + Relu | (32, 3) | (15, 32) | (8, 2) | (2, 8) | (32, 3) | (298, 32) |
Conv1D + Relu | (16, 3) | (13, 16) | (8, 2) | (1, 8) | (16, 3) | (296, 16) |
Flatten + Dropout (0.2) | — | 208 | — | 8 | — | 4736 |
Dense + Dropout (0.2) | — | 128 | — | 6 | — | 128 |
Dense | — | 64 | — | 4 | — | 64 |
Softmax | — | 3 | — | 3 | — | 3 |
Note that ( n , s ) denotes the number of filters and filter size for the corresponding CNN model.
3.7. Decision Fusion
We perform a fusion of decisions obtained from three different pretrained CNNs using ensemble CNN model for the end results (equation ( 17 )). For the decision fusion using the ensemble CNN, we provide weights of 0.70, 0.20, and 0.10 empirically for CNN ft , CNN da , and CNN ds , respectively.
In our ensemble CNN model, we only unfreeze the top three layers of each fine-tuned CNN model and add the weighted decision fusion layer to attain the end results. The overall decision fusion pipeline of ensemble CNN is presented in Figure 2 :
where CNN c denote the fused CNNs' model using the weighted average method.

Decision fusion using weighted average method on ensemble CNN (CNN e ).
3.8. Implementation
For the implementation of our proposed method, we use two different tools, Sklearn [ 49 ] and Keras [ 50 ], implemented in Python [ 51 ]. Furthermore, we design 10 different train/test sets, each with 70 : 30 split ratio per category, and report the averaged performance measures over 10 runs for the analysis. To select the best hyperparameters in our study, we perform a grid search approach, which iterates over the range of different parameter values for the selection of optimal values. The detailed information of hyperparameters' selection approach for the corresponding ML algorithms is presented in Table 5 .
Grid search ranges used to tune the corresponding hyperparameters of each machine learning algorithm used in our study.
Algorithm | Range |
---|---|
SVM + Linear | : {1 to 1000}, Gamma: {0.001 to 0.1} |
SVM + RBF | : {1 to 1000}, Gamma: {0.001 to 0.1} |
XGBoost | Learning-rate: {0.01 to 0.1}, max-depth: {5 to 10}, -estimators: {120 to 200} |
ANN | Hidden-layer-size: {20 to 60}, learning-rate-init: {0.01 to 1}, max-iter: {10 to 1000} |
RF | Min-sample-leaf: {3 to 7}, min-sample-split: {2 to 6}, -estimators: {50 to 200} |
LR | : {1 to 1000}, solver: lbfgs, max-iter: {100 to 1000} |
K-NN | Leaf-size: {30 to 45}, -neighbor: {100 to 200}, : {1 to 3} |
Our CNN models | Learning-rate: {1 − 01, 1 − 02, 1 − 03, 1 − 04, 1 − 05}, batch-size: {8, 16, 32, 64}, epochs: {10, 20, 30, 40, 50, 100}, optimizer: {“RMSProp”, “Adam,” “SGD”} |
4. Result and Discussion
4.1. comparison of proposed feature extraction methods using traditional machine learning methods.
Here, we compare our proposed feature extraction methods based on traditional machine learning methods. The comparative results are presented in Table 3 . While looking at Table 3 , we notice that fastText-based embedding (ft) outperforms the other two embedding types (“ds” and “da”) in terms of Precision, Recall, F1-score, and Accuracy in most of the ML algorithms. For example, the XGBoost algorithm imparts 69.0%, 69.5%, 69.5%, and 66.7% for Precision, Recall, F1-score, and Accuracy, respectively. The performance superiority of “ft” among three methods is attributed to the compact contextual embedding vectors achieved from the pretrained fastText model, which has been trained with massive amount of Nepali documents. Similarly, the domain-specific (ds) embedding method is the second-best performing, which imparts a higher performance than the domain-agnostic (da) method on most of the ML algorithms. As an example, SVM + Linear imparts Precision of 63.5%, Recall of 54.1%, F1-score of 54.1%, and Accuracy of 56.3%. This interesting result reveals that the domain-specific (ds) information is very important to capture the domain-specific patterns of tweets. To this end, we are able to achieve prominent accuracy with a lower feature size (3-D). Finally, the domain-agnostic (da) method is the least performing, which has the lowest performance in most of the cases against two counterparts (“ft” and “ds”). We speculate that the reason of its lowest performance is responsible to the presence of less important contextual information.
While comparing different traditional machine learning methods on our proposed feature extraction techniques, we notice that the performance is differing from one ML algorithm to another algorithm. Under precision, SVM + RBF, RF, and SVM + Linear impart the highest performance on “ft” (70.2%), “da” (60.8%), and “ds” (63.5%), respectively. For Recall, XGBoost imparts the highest performance on “ft” (69.5%) and “ds” (62.3%), whereas RF yields the highest performance on “da” (60.7%). Under the F1-score, XGBoost imparts the highest performance on “ft” (69.5%) and “ds” (62.3%); however, RF imparts the highest performance on “da” (60.7%). Moreover, under accuracy, XGBoost imparts the highest performance on “ft” (66.7%) and RF imparts the highest performance on “da” (57.0%), whereas, on “ds” (65.7%) method, LR imparts the highest performance. Through this experiment, we stipulate that XGBoost is the high-performing algorithm in most cases as it can work on an optimal number of boosting trees, which could result in higher performance than its other counterparts.
Although the fastText-based method (ft) has the highest performance in comparison to other methods, it has a higher feature size (300-D) than other methods. Also, it is computationally infeasible to extract such features as we have to load pretrained models and compute them to achieve it. However, the second-best method (ds) has only a 3-D feature size and is easy to extract features. Achieving comparable performance with such a smaller-sized feature is an interesting research direction for future work.
4.2. Comparison of Our Methods with State-of-the-Art Methods
We compare our methods with the recent state-of-the-art methods [ 31 , 32 ] and [ 1 ] that have been used for the classification of Nepali documents, particularly in news domain. We choose them because there are no existing works available in the literature for Nepali COVID-19 tweets' classification, and the available existing works in other languages such as English and Arabic are not appropriate to Nepali COVID-19 tweets' classification because of the different linguistic structure and processing requirements. To this end, we implement each method on our NepCOV19Tweets dataset and compare them with our proposed methods. The detailed experimental results are presented in Table 6 .
Comparison of our methods based on overall classification accuracy (%) with state-of-the-art methods.
Methods | Feature size | Accuracy |
---|---|---|
Shahi et al. [ ] | 100-D | 62.1 |
Basnet and Timalsina [ ] | 300-D | 62.9 |
Sitaula et al. [ ] | 17-D | 59.8 |
Ours (ds) | 3-D | 61.5 |
Ours (da) | 17-D | 59.5 |
Ours (ft) | 300-D | 68.1 |
Ours (ensemble) | 320-D | 68.7 |
Note. Each reported accuracy in the table is the averaged value over 10 runs on our NepCOV19Tweets dataset.
While observing Table 6 , we notice the superiority of our methods based on two different aspects. First, our proposed methods impart the comparable feature size, 3-D (ds), 17-D (da), 300-D (ft), and 320-D (ensemble CNN). Second, our methods impart the significant boost in the classification accuracy (68.7%), which has 9.2% improvement over the least-performing method (59.5%) and 5.8% improvement over the second-best method (62.9%). Such significant improvement of classification accuracy with a comparable feature size imparted by our methods has underlined the efficacy of domain-specific, domain-agnostic, and pretrained word embedding for the Nepali COVID-19 tweets' representation and classification.
4.3. Comparative Study of Proposed CNN Models Used in Our Study
We discuss the component analysis of each CNN model in terms of classwise performance. The results are presented in Table 7 . Each CNN corresponds to three different kinds of features (“ft,” “ds,” and “da”) used in this study.
Classwise study of our proposed method using classification performance (%).
CNN | Positive | Neutral | Negative | Overall | |||||||||
---|---|---|---|---|---|---|---|---|---|---|---|---|---|
CNN | 69.4 | 71.8 | 51.8 | 76.8 | 72.8 | 63.5 | 68.1 | ||||||
CNN | 68.0 | 59.6 | 62.6 | 12.7 | 00.2 | 00.5 | 59.0 | 82.7 | 68.6 | 46.5 | 47.5 | 43.9 | 61.5 |
CNN | 64.1 | 57.6 | 60.5 | 43.9 | 04.5 | 08.2 | 57.4 | 78.6 | 66.3 | 55.1 | 46.9 | 45.0 | 59.5 |
CNN | 72.0 | 14.7 | 23.7 | 66.8 | 56.4 | 56.4 |
Note/ P , R , and F denote Precision, Recall, and F1-score for three classes (positive, negative, and neutral), respectively. Note that the hyperparameters used in our models are as follows: learning-rate: 1 e − 05, batch-size: 32, epochs: 50, and optimizer: RMSProp. Boldface denotes the highest performance.
While looking at the performance of the fastText-based CNN model, called CNN ft , we notice that it provides an average accuracy of 68.1%, where it has the highest F1-score on the negative class and the lowest F1-score on neutral class. Similarly, the domain-specific CNN model (CNN ds ) has the second-best performance (61.5% accuracy) among three components. It also imparts the highest F1-score on the negative class. We also notice a similar trend on CNN da , where it provides 59.5% accuracy with the highest performance on the Negative class (F1-score: 66.3%). Last but not the least, the ensemble of three CNN models (CNN ft , CNN ds , and CNN da ) imparts an accuracy of 68.7%, which not only improves the overall performance but also outperforms each CNN models in this study.
In summary, the ensemble of three CNN models helps preserve three different semantic information in parallel for decision-making. This experiment further underscores that these three different pieces of information (“ft,” “ds,” and “da”) could play a crucial role in the discrimination of tweets during the sentiment classification process.
4.4. Statistical Analysis
Here, we perform the statistical analysis of performance measures (Precision, Recall, F1-score, and Accuracy). The results are presented in Figure 3 . While looking at Figure 3 , we notice that our method imparts 95% confidence interval (CI) of [67.8, 68.6], [68.3 68.9], [65.4 66.3], and [68.2 68.9] for Precision, Recall, F1-score, and Accuracy, respectively. We notice that the neutral class is complex compared to the positive and negative classes, which not only get a lower Precision and Recall measure but also contribute to a lower overall F1-score. Thus, we observe the slight degradation in the F1-score measure while looking at the box plot because of the neutral class. Moreover, while performing the two-tailed t -test, we notice that our method provides a p value < 2.2 e − 16 for all performance measures (Precision, Recall, F1-score, and Accuracy). Because of such stable CI and significant two-tailed t -test results, we believe that our method is robust and prominent on sentiment classification for COVID-19-related tweets.

Statistical analysis of performance measures over results of 10 folds used in our study.
5. Conclusion and Future Works
In this paper, we have proposed three CNN models to classify the Nepali COVID-19-related tweets into three sentiment classes (positive, negative, and neutral). These CNN models show stable and robust performance. Also, we have proposed to use three different kinds of feature extraction methods for the representation of tweets during classification. We have validated our proposed features' extraction methods using traditional machine learning algorithms, which show that our proposed features can discriminate the complex COVID-19 tweets in most cases.
Our method has three main limitations. First, our method ignores the sequential approach of tokens, which could be an important clue for tweets classification. To this end, the sequential-based model such as the LSTM (long short-term memory) model could contribute to the performance improvement. Second, our method exploits fastText (“ft”) and probability-based embeddings (“ds” and “da”) for the classification. The combination of other kinds of embeddings such as Word2vec and GloVe could enhance the performance further.
Data Availability
Conflicts of interest.
The authors declare that there are no conflicts of interest.
कोरोना वायरस रोग 2019 (COVID-19) - नेपाली (Nepali)
कोरोना वायरस रोग 2019 (COVID-19) (Coronavirus Disease 2019)
सरकारले COVID-19 को लागि प्रतिक्रिया स्तर घटाएको छ। विस्तृत जानकारी को लागि, कृपया सम्बन्धित प्रेस विज्ञप्ति हेर्नुहोस् । The Government has lowered response level for COVID-19. For details, please refer to the relevant press release . https://www.info.gov.hk/gia/general/202305/30/P2023053000552.htm
खोपहरूको जानकारी (Information on Vaccines)
निष्क्रिय कोभिड-19 खोपको लागि खोपको तथ्यपत्र (Factsheet for Vaccination of Inactivated COVID-19 Vaccine)
mRNA कोभिड-19 खोपको लागि खोपको तथ्यपत्र (Factsheet for Vaccination of mRNA COVID-19 Vaccine)
XBB COVID-19 खोपको दोस्रो चरण, खोप र बुकिङ (Second phase of XBB COVID-19 Vaccination, booking and vaccination)
कोविड-19 खोप र मौसमी इन्फ्लुएन्जा खोपको सह-प्रशासन (Co-administration of COVID-19 Vaccine and Seasonal Influenza Vaccine)
COVID-19 खोपको नयाँ व्यवस्था (COVID-19 vaccination new arrangement)
खोप पछिको लक्षणहरू (Symptoms after Vaccination)
COVID-19 खोपहरूको साइड इफेक्ट ह्यान्डल गर्ने (Handling Side Effects of COVID-19 Vaccines)
व्यक्तिगत डाटा संग्रहको उद्देश्यको बयान (Statement of Purpose of Collection of Personal Data)
व्यक्तिगत डाटा संग्रहको उद्देश्यको बयान (Statement of Purpose of Collection of Personal Data (B&W leaflet))
भाइरस परीक्षण (Virus Testing)
Video - COVID-19 Rapid Antigen Test | Demo Video (Video - COVID-19 Rapid Antigen Test | Demo Video)
स्वास्थ्य सल्लह (Health Advice)
चपस्टिक्स र चम्चा प्रयोग गर्नुहोस (Use serving chopsticks and spoon)
कीटाणुहरू न फैलाउनु होस् शौचालय प्रयोग गरिसकेपछि फ्लश गर्नु अघि शौचालयको आवरण तल राख्नुहोस् (Don't spread germs: After using toilet, put the toilet lid down before flushing)
ड्रेनेज पाइपहरू राम्रोसँग मर्मत गर्नुहोस् र नियमित रूपमा यू-जालमा पानी थप्नुहोस् (Maintain drainage pipes properly and add water to the U-traps regularly)
तपाईंको वरिपरिकामा कीटाणुहरू नफैलाउनुहोस् (Don't spread germs to those around you: Cover your cough)
खोक्ने तरिकाहरू कायम राख्नुहोस् (Maintain Cough Manners)
राम्रोसँग सर्जिकल मास्क लगाउनुहोस् (Wear a Surgical Mask Properly)
शल्यचिकितस्क मास्कको उचित काढ्ने र सुरक्षित डिस्पोजल गर्ने (Proper Removal and Safe Disposal of a Surgical Mask)
सर्जिकल मास्कको बारेमा थप जानकारी लिनुहोस (Know more about Surgical Mask)
ममास्कको प्रयोग ठीकसँगले गर्नुहोस् - आफूलाई सुरक्षित राख्नुहोस् अनि अरूको सुरक्षा गर्नुहोस् (Use mask properly – Protect ourselves and protect others)
हातको सरसफाई - संक्रमण रोक्न एउटा सजिलो र प्रभावकारी तरिका (Hand Hygiene - An easy and effective way to prevent infection)
- पनिमोनिया र श्वसन पथ संक्रमणको रोकथाममा स्वास्थ्य सल्लाह (सार संस्करण) (Health Advice on Prevention of Pneumonia and Respiratory Tract Infection (Abstract version))
विविध (Miscellaneous)
ड्रेनेज पाइपको मर्मत-सम्भारका बारेमा तपाईंलाई के-के कुराहरू थाहा हुन आवश्यक छ (What you need to know about drainage pipe maintenance)
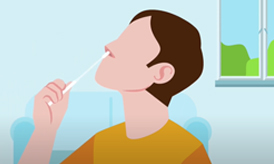
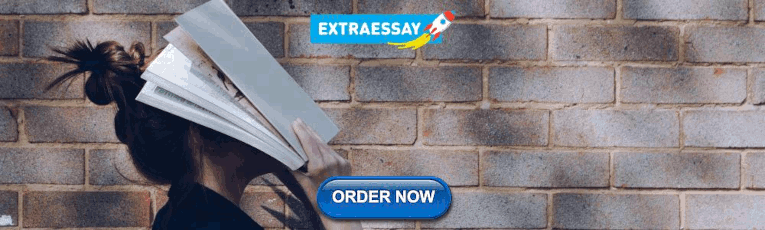
IMAGES
VIDEO
COMMENTS
We have essays on covid 19 in nepali and essay on coronavirus in nepali for class1, 2, 3, 4, 5, 6, 7, 8, 9, 10, 11 and 12 in 150, 200, 250, 300 words.Hello E...
#EssayinnepaliWe have essays on covid 19 in nepali and essay on coronavirus in nepali for all class in 150, 200, 250, 300 words.Hello Everyone,Welcome to our...
Essay on Coronavirus in Nepali. This essay will help students with coronavirus. It is very simple and easy essay in Nepali to understand. कोरोना भाइरसको बारे...
COVID-19 Presentation in Nepali; COVID-19 Presentation in Nepali . DOWNLOAD. Published on: 2020-03-08. Sections. Epidemiology & Outbreak Management Section; News & Update. Jan 1. 2024_07_08_Dengue Situation Update. 2024_07_08_Dengue Situation Update Read More.
Key messages in Nepali language- Audios capsules. WHO, Country Ofce for Nepal Communication Product heet nternational Podcast ay Audio ... Brief description This is a set of short explainer audio capsules that address different facets of the COVID-19 pandemic and the response. Language Nepali Format Each capsule is an answer to one question ...
The audio contents that seek to explain different facets of the COVID-19 pandemic and the response mechanisms. Language Nepali Format Each audio capsule is an answer to one question. Each interview is in a discussion format between the interviewee and interviewer. The answers are based on MoHP and WHO guidance on the topic but drafted in words ...
Communication Product heet Public Concerns Related to COVID-19, Key Messages in Nepali language- Audio Content 2 Value-add This audio content attempts to counter rumours, allay concerns and clarify misinformation using the science and evidence that is currently available on COVID-19, in a language that is accessible to a wide audience. Topics ...
Nepali (नेपाली) Audio. Rapid Antigen Testing (audio) 2021-11-01 00:00:00. Fact sheets. COVID-19 Glossary. 2021-07-16 00:00:00. COVID-19 Self-Isolation change. ... COVID-19 can live on surfaces for days, but simple cleaning can kill it. Covid-19 testing and antiviral information.
All the doctors and nurses were of Nepali origin and so were the patients. Mental health related issues following infection from SARS-CoV-2 and its positive test was the primary reason people contacted the service. Symptoms of Covid-19 such as fever, cough, difficulty breathing were the major symptoms for contacting the telehealth services.
Key Messages Audio Content in Nepali language. al Public Health Standards- Audio ContentMediumAudio contentBrief descriptionThis is a set of short explainer audio contents that address the essential publi. health standards to be followed by the general public during COVID-19 pandemic.The audio contents that seeks.
The COVID-19 pandemic in Nepal is part of the worldwide pandemic of coronavirus disease 2019 (COVID-19) caused by severe acute respiratory syndrome coronavirus 2 (SARS-CoV-2).The first case in Nepal was confirmed on 23 January 2020 when a 31-year-old student, who had returned to Kathmandu from Wuhan on 9 January, tested positive for the disease. It was also the first recorded case of COVID-19 ...
By April 6, 2021, the total confirmed cases worldwide are 132.28 million cases and 2.87 million deaths with more than 200 countries affected, and in Nepal alone, there are 278,470 cases confirmed and 3,036 deaths. [ 1] Nepal had its first COVID-19 case on January 13, 2020. The index case was a student studying in Wuhan and had returned to Nepal ...
Introduction. Coronavirus disease (COVID-19) outbreak originating from Wuhan, China in late 2019 has spread worldwide claiming more than 2.5 million lives all over the world as of 01 March 2021 ().On 11 March 2020, the World Health Organization (WHO) declared it as a pandemic ().Since the outbreak of the disease WHO through its guidelines has prioritized the actions for responding to the virus ...
100 Words Essay on Covid 19. COVID-19 or Corona Virus is a novel coronavirus that was first identified in 2019. It is similar to other coronaviruses, such as SARS-CoV and MERS-CoV, but it is more contagious and has caused more severe respiratory illness in people who have been infected. The novel coronavirus became a global pandemic in a very ...
Students can choose to write a full-length college essay on the coronavirus or summarize their experience in a shorter form. To help students explain how the pandemic affected them, The Common App ...
Essay On Covid-19: 100, 200 and 300 Words. COVID-19, also known as the Coronavirus, is a global pandemic that has affected people all around the world. It first emerged in a lab in Wuhan, China, in late 2019 and quickly spread to countries around the world. This virus was reportedly caused by SARS-CoV-2. Since then, it has spread rapidly to ...
1. Introduction. COVID-19, caused by the severe acute respiratory syndrome coronavirus 2 (SARS-CoV-2), was first reported in Wuhan, China, in December 2019 and is now a global pandemic [].Despite substantial efforts to manage and control the pandemic, morbidity, and mortality due to COVID-19 and its variants have led to significant alterations in daily life [].
Nepal has been on the frontline of both the covid-19 pandemic and climate change, and in both crises the response by the international community and Nepal's government has been marked by a failure to prepare or to invest proactively in strong prevention measures. The first case of covid-19 in Nepal was reported in January 2020 and the country's modest first wave peaked in late October 2020 ...
The audio also seeks to explain different facets of COVID-19 pandemic and the response. Language Nepali Format Each capsule is an answer to one question. The answers are based on WHO guidance on the topic but drafted in words that would be understandable to a wide audience. Duration The audio capsules range in duration from 40 seconds to 60 ...
Paragraph Writing on Covid-19 in 200 Words. The Covid-19 pandemic was caused due to a man-made virus called coronavirus. It is an infectious disease that has affected millions of people's lives. The pandemic has affected the entire world differently. It was initially diagnosed in 2019 in Wuhan, China but later, in March 2020, WHO declared ...
3.4. Proposed Approach. In our method, we follow three different steps for the COVID-19-related tweets' sentiment classification, namely, "embedding vector extraction and representation," "CNNs design and training," and "decision fusion.". 3.5. Embedding Vector Extraction and Representation.
The COVID-19 pandemic has had a profound impact on individuals, societies, and economies worldwide. Its multifaceted nature presents a wealth of topics suitable for academic exploration. This essay provides guidance on developing engaging and insightful essay topics related to COVID-19, offering a comprehensive range of perspectives to choose from.
कोरोना वायरस रोग 2019 (COVID-19) (Coronavirus Disease 2019) पनिमोनिया र श्वसन पथ संक्रमणको रोकथाममा स्वास्थ्य सल्लाह (सार संस्करण) (Health Advice on Prevention of Pneumonia and Respiratory Tract ...