Advertisement
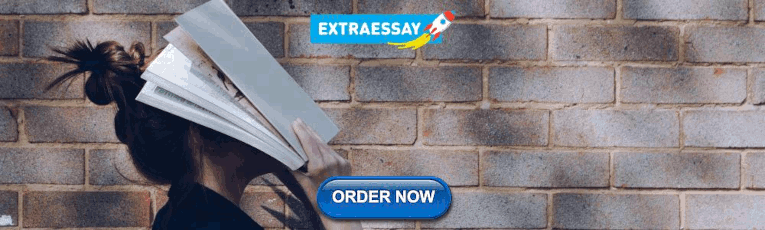
A catalog of curated breast cancer genes
- Epidemiology
- Open access
- Published: 10 November 2021
- Volume 191 , pages 431–441, ( 2022 )
Cite this article
You have full access to this open access article
- Muthiah Bose 1 , 2 ,
- Jan Benada 1 na1 ,
- Jayashree Vijay Thatte 1 na1 ,
- Savvas Kinalis 2 ,
- Bent Ejlertsen 3 ,
- Finn Cilius Nielsen 2 ,
- Claus Storgaard Sørensen 1 &
- Maria Rossing ORCID: orcid.org/0000-0003-4325-3027 2 , 4
3975 Accesses
2 Citations
5 Altmetric
Explore all metrics
Decades of research have identified multiple genetic variants associated with breast cancer etiology. However, there is no database that archives breast cancer genes and variants responsible for predisposition. We set out to build a dynamic repository of curated breast cancer genes.
A comprehensive literature search was performed in PubMed and Google Scholar, followed by data extraction and harmonization for downstream analysis.
Using a subset of 345 studies, we cataloged 652 breast cancer-associated loci across the genome. A majority of these were present in the non-coding region (i.e., intergenic (101) and intronic (345)), whereas only 158 were located within an exon. Using the odds ratio, we identified 429 loci to increase the disease risk and 198 to confer protection against breast cancer, whereas 25 were identified to both increase disease risk and confer protection against breast cancer. Chromosomal ideogram analysis indicated that chromosomes 17 and 19 have the highest density of breast cancer loci. We manually annotated and collated breast cancer genes in which a previous association between rare-monogenic variant and breast cancer has been documented. Finally, network and functional enrichment analysis revealed that steroid metabolism and DNA repair pathways were predominant among breast cancer genes and variants.
Conclusions
We have built an online interactive catalog of curated breast cancer genes ( https://cbcg.dk ). This will expedite clinical diagnostics and support the ongoing efforts in managing breast cancer etiology. Moreover, the database will serve as an essential repository when designing new breast cancer multigene panels.
Similar content being viewed by others
A knowledge-based framework for the discovery of cancer-predisposing variants using large-scale sequencing breast cancer data.
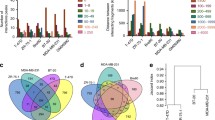
Capture Hi-C identifies putative target genes at 33 breast cancer risk loci
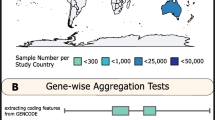
Aggregation tests identify new gene associations with breast cancer in populations with diverse ancestry
Avoid common mistakes on your manuscript.
Breast cancer is the most common cancer diagnosed in women and most importantly, it is the leading cause of cancer-related deaths among women worldwide [ 1 , 2 ]. Breast cancer is a multifactorial disease resulting from genetic, hormonal, and environmental factors. In concordance with cancer disease in general, inherited mutations play a causal role in up to ten percent of all breast cancers [ 3 , 4 ]. For decades, genetic screens have played a vital role in the identification of genes and variants responsible for breast cancer predisposition. Various sequencing methods such as Sanger sequencing, gene panel testing, whole-exome sequencing (WES), and ultimately whole-genome sequencing (WGS) have been employed to identify genetic variation responsible for breast cancer predisposition [ 5 , 6 ] .
Genetic variation can predispose to breast cancer through both rare-monogenic variant causing a large increase in disease risk and common-polygenic variant (alias SNPs) that possess small individual effects on disease, however, cumulatively cause a large increase in disease risk [ 7 ]. Rare germline variants in the high-risk genes BRCA1 and BRCA2 together with the moderate-risk genes such as PALB2 , ATM , CHEK2, and BRIP1 account for about 30% of breast cancer predisposition [ 8 , 9 ]. Similarly, cancer syndrome genes ( CDH1, PTEN and STK11 etc.) together with SNPs explain around 20% of breast cancer predisposition [ 9 ]. Most SNPs are identified through genome-wide association studies (GWAS) and recent studies have suggested that polygenic risk score (PRS) accounts for around 18% of the familial breast cancer risk [ 10 ]. The remaining heritability (around 50%) for breast cancer is most likely caused by yet unidentified moderate-risk genes or a specific cluster of common-polygenic variants [ 11 ]. Identification of these unknown factors responsible for breast cancer etiology is of utmost importance and could expedite personalized breast cancer medicine, including therapeutic and preventive strategies [ 12 ].
Breast cancer genes and SNPs responsible for disease etiology play a significant role during clinical management. The clinical utility of rare-monogenic variant containing genes and SNPs differs due to varied disease penetrance. Specifically, rare-monogenic variant containing breast cancer genes is used to design (or update) a focused panel of breast cancer genes for genetic screening. Similarly, a list of such genes could ensure that the clinical investigators has an updated breast cancer gene list, when screening patients for disease etiology using WES or WGS. For clinical purposes, the use of breast cancer genes and SNPs can be augmented significantly by rapidly integrating newly identified breast cancer genes and variants. However, with a constant flow of new studies, it is challenging to seamlessly translate these findings into the clinical setting. We believe the presence of a freely accessible database comprising breast cancer-associated genes (and variants) will aid a rapid translation into clinical diagnostics. This led us to initiate a meta-analysis of breast cancer susceptibility genes, by applying comprehensive and stringent criteria, with the aim of generating an online interactive catalog of curated breast cancer genes ( https://cbcg.dk ).
Materials and methods
Literature search and study selection.
A comprehensive literature search for eligible studies was performed in PubMed and Google Scholar (Fig. 1 ). The following terms were used either alone or in combination: “Breast cancer”, “risk”, “loci”, “single nucleotide polymorphism”, “SNP”, “polymorphism”, “susceptibility gene”, “genetic variants”, “association”, “polymorphisms”, “genetic mutation”, “germline”, and “variant”. The inclusion criteria for the studies were as follows: (1) studies must be reported in English; (2) studies must be published in peer-reviewed journals; (3) studies must be available as full-text articles; (4) studies must be either case–control, kin-cohort, or prospective in design; (5) case–control studies must report genotype frequencies (or OR with 95% confidence interval (CI) values); and (6) for non-case–control study in design, other relevant metrics such as standardized incidence ratios (SIR), relative risk and cumulative risk etc. were taken into account (Fig. 1 ). The exclusion criteria were as follows: (1) publications that were reviews, meta-analysis, case reports, and meeting abstracts; (2) studies that did not provide genotype distributions among cohorts; and 3) studies performed on tumor tissue for breast cancer association (Fig. 1 ).
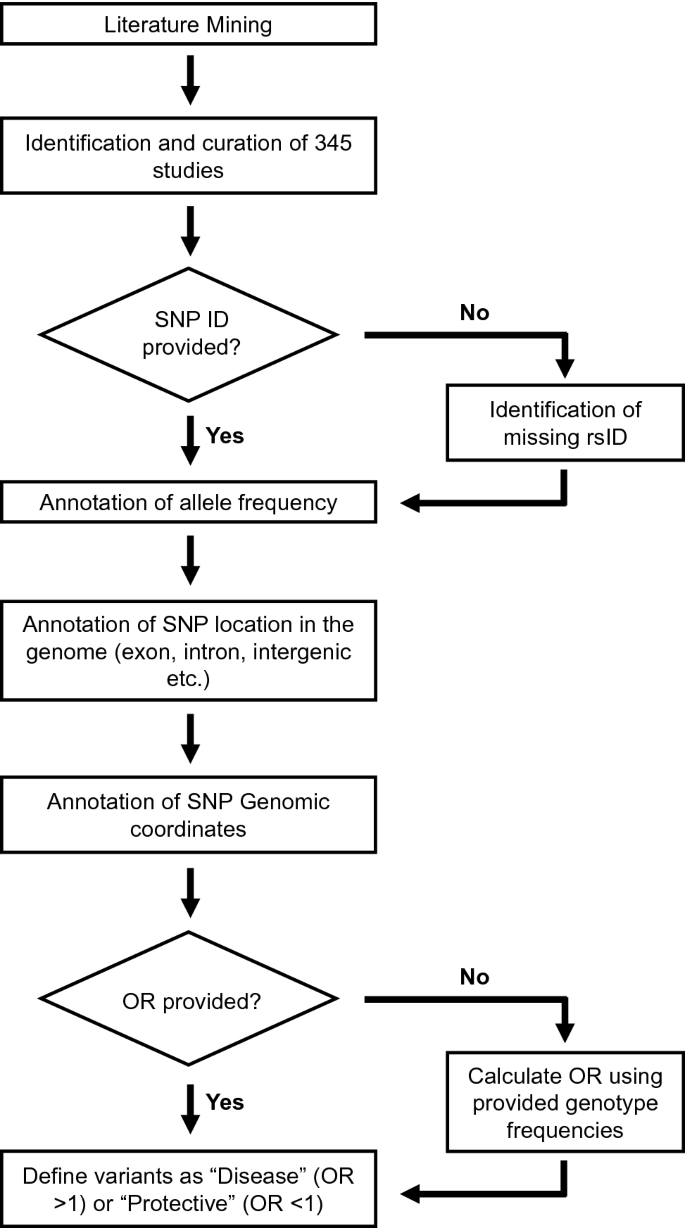
Flow chart outlining multiple steps involved in the database design such as literature search, data extraction, data annotation, and data harmonization
Data extraction
Three independent investigators extracted all data and any discrepancies were resolved by discussion. The following information were collected from the enrolled studies: (1) SNP identifiers (rsID) (if reported) or the sequence variation of the reported mutation, (2) OR (if reported) or the genotypic frequency of both cases and controls, and (3) in relevant studies: SIR, relative risk, and cumulative risk were also collected (Fig. 1 ). Of note, information on population background and breast cancer subtypes was initially extracted from the enrolled studies. However, due to ambiguous use of descent, ethnicity, and nationality, as well as lack of consistent subtype annotation, these records were not included in the database.
Data harmonization
Historically, different nomenclatures have been used to report the findings among the included studies (Fig. 1 ). Specifically, few studies have reported the breast cancer-associated variant using its rsID, whereas other studies reported only the consequent “sequence variation”. Similarly, few studies reported the OR of the identified breast cancer-associated variant (with 95% CI values), whereas other studies only reported the genotype frequencies between their study subjects. Thus, in order to standardize the data for this database, we performed data harmonization as shown in Fig. 1 . The breast cancer-associated mutation that was reported only by its “sequence variation” was manually converted into its corresponding “rsID” using GnomAD database. Similarly, in those studies, which only reported the genotype frequencies between their study subjects, we manually calculated the corresponding OR (Fig. 1 ). The odds ratio, its standard error, and 95% confidence interval are calculated according to Altman, 1991 [ 13 ]. Specifically, OR is calculated using the formulae: OR = (a/b)/(c/d); where a = number of patients in disease cohort carrying the variant; b = number of patients in disease cohort not carrying the variant; c = number of patients in control cohort carrying the variant; and d = number of patients in control cohort not carrying the variant.
Database design
This database was designed and created using the rsID and OR that were extracted as mentioned above (Fig. 1 ). Using the rsID, we manually annotated its allele frequency (AF), SNP location, and genomic loci. AF (GnomAD [ 14 ]) was used to differentiate the rare-monogenic variants (AF < 0.01) between the common-polygenic variants (AF > 0.01). SNP location illustrates whether a variant is located within a gene (intron, exon or UTR region etc.) or in an intergenic region. Genomic loci categorize both chromosomal regions with high clustering of breast cancer-associated mutations, as well as chromosomal segments that are devoid of breast cancer-associated mutation. The OR (also SIR, relative risk and cumulative risk) was used to differentiate between a potentially disease-causing genetic variant (OR > 1; hereafter referred as disease variant) and a genetic variant that may confer protection against breast cancer (OR < 1; hereafter referred as a protective variant).
Chromosomal ideogram visualization
Chromosomal ideogram was constructed with PhenoGram software tool [ 15 ] (visualization.ritchielab.org) using proximity algorithm for phenotype spacing, with each circle representing one gene or variant. For the clarity of visualization, genomic coordinates were rounded to the nearest multiple of 1 Mb, and thus, genes or variants within this proximity were binned to a single line of adjacent circles. Final graphical adjustments were performed in Adobe Photoshop 2019 and Adobe Illustrator 2019.
Network analysis
The protein–protein interaction network was constructed using STRING version 11.0 database [ 16 ] ( https://string-db.org/ ). "Experiments" dataset was used as an active interaction source with a minimum required interaction score of 0.4 (medium confidence). Subsequently, the network visualization was graphically adjusted in Cytoscape 3.8.2 [ 17 ] ( https://cytoscape.org/ ) in order to highlight proteins encoded by DNA repair genes.
Functional enrichment analysis
g:Profiler [ 18 ], g:GOSt tool, was used to perform functional enrichment analysis in the breast cancer-associated genes resulting in a list (Supplementary Table 1) of 2068 significantly enriched terms (Benjamini–Hochberg FDR < 0.05). The gene list was treated as an unordered query and only annotated genes were considered for statistical tests under the statistical domain scope function. For term sizes, between 4 and 500 genes were considered. Electronic GO annotations were removed, while GO molecular function (MF), GO cellular component (CC), GO biological process (BP), KEGG, Reactome, and WikiPathways data sources were analyzed. The Ensembl ID with the most GO annotations was chosen for all 5 ambiguous genes ( AHRR , BABAM1 , FOXP1 , LRTOMT, and SULT1A1 ).
Literature search, data extraction, and annotation
The presence of genetic risk factors and positive family history of breast cancer is the single most important risk factor for breast cancer development [ 19 ]. Currently, there is no available breast cancer gene repository assisting clinical translation; thus, we set out to build a manually curated database of breast cancer-associated genes and variants, using the flow chart outlined in Fig. 1 .
The literature search yielded a multitude of publications and after manual evaluation the database was constructed based on a subset of 345 studies. Among these 345 studies, we manually extracted “rsID” and “OR” (also SIR, relative risk and cumulative risk; in relevant studies) for every reported breast cancer-associated genetic variant. Further, using the “rsID”, we manually mapped the (1) AF (GnomAD), (2) SNP location (to identify whether the mutation is located within a gene or in an intergenic region), and (3) genomic loci of every reported breast cancer-associated variant. Meanwhile, using the OR, we manually annotated every breast cancer-associated variants as either (1) Disease (OR > 1; variant that increases disease risk), (2) Protective (OR < 1; variant that confers protection against breast cancer), or (3) Both (variants that were shown to have both OR > 1 and OR < 1 in different studies). Following data extraction and annotation, we constructed the Curated Breast Cancer Gene ( https://cbcg.dk ) database, a freely available database for the future collation of new breast cancer-associated variants and genes.
Demography of breast cancer-associated variants
We indexed 925 records in total; the term records instead of SNP/gene is used because similar SNPs/genes were reported multiple times to be associated with breast cancer by different studies. Same SNPs (or genes) that were reported by multiple studies were indexed separately as a unique record. Similarly, different SNPs (or genes) that were reported by a specific study were indexed separately as a unique record.
As depicted in Fig. 2 a, we cataloged in total 652 breast cancer-associated loci across the genome. Among these, 551 breast cancer loci (85%) were located within a gene (intron, exon or UTR region, etc.). Interestingly, a large number of 101 breast cancer loci (15%) were present in the intergenic region. Of the 551 breast cancer loci located within a gene, a majority of them (345, 63%) were present in the intron. Breast cancer-associated variants were also reported in the UTR and splice site regions etc. accounting for 9% (48) of the breast cancer loci located within a gene. However, only 29% (158) of the reported breast cancer loci were located within an exonic region. Taken together, most of the reported breast cancer-associated variants (446) were present in the non-coding region such as intergenic (101) and intronic (345). SNPs located in the intergenic and intronic regions are suggested to play a role in the regulation of gene expression [ 20 , 21 ].
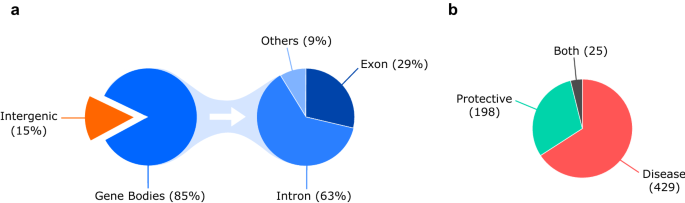
a Pie chart outlining the distribution of 652 breast cancer-associated loci across the genome. b Pie chart outlining the distribution of variants that either predisposes to breast cancer (Disease; OR > 1) or confers protection against breast cancer (Protective; OR < 1)
Further, we cataloged the breast cancer-associated variants based on their OR to identify variants that either predispose to breast cancer (Disease; OR > 1) or confer protection against breast cancer (Protective; OR < 1). In our analyses, 429 breast cancer-associated variants were identified to predispose to breast cancer, whereas 198 breast cancer-associated variants were identified to confer protection against breast cancer (Fig. 2 b). We also identified 25 breast cancer-associated variants that were reported to both predispose to breast cancer and confer protection against breast cancer in different studies (Fig. 2 b). These conflicting results are mostly observed in studies performed in different populations, suggesting population-based effects.
Chromosomal ideogram analysis
In order to identify chromosomal regions that are enriched or devoid of breast cancer-associated variants, we performed ideogram analysis in the 652 breast cancer-associated loci (Fig. 3 ) [ 15 ]. The highest number of breast cancer-associated loci (60) was found on chromosome 2, whereas the lowest number of breast cancer-associated loci (6) was found on chromosome 21 (excluding sex chromosomes). Since chromosomes are of differing length, we next analyzed the number of breast cancer-associated loci relative to its length for every chromosome (Fig. 4 a). Despite its larger size, the density of breast cancer-associated loci was lower in chromosome 4 (Fig. 4 a). Similarly, chromosomes 17 and 19 had the highest density of breast cancer-associated loci when compared to its chromosomal size (Fig. 4 a).
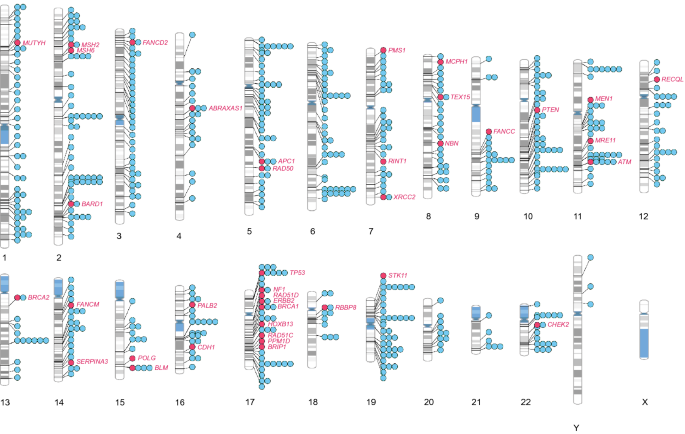
Chromosomal ideogram illustrating the distribution of 652 breast cancer-associated loci across the chromosomes. Chromosomal ideogram was constructed using PhenoGram software tool [ 15 ] with each dot representing one gene or variant
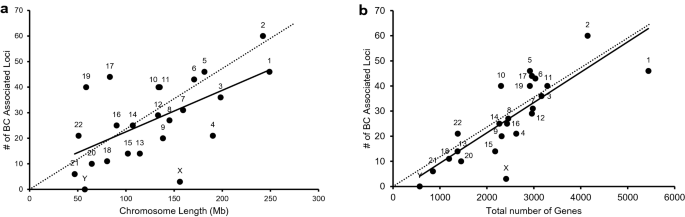
a Scatter plot illustrating the number of breast cancer-associated loci relative to its length for every chromosome. The chromosomal length for each chromosome was retrieved from Ensembl under Chromosome Statistics. b Scatter plot illustrating the number of breast cancer-associated loci relative to the total number of genes present in each chromosome. The total number of genes for each chromosome was calculated using Ensembl (Chromosome Statistics) by adding the number of coding genes, non-coding genes, and pseudogenes. The thick continuous line depicts the trendline for the number of breast cancer-associated loci present in each chromosome compared to its length ( a ) or the total number of genes present in that chromosome ( b ). a and b The thin dotted line is an imaginary trendline to illustrate a perfect positive correlation
Since, chromosome 17 and 19 have been shown to possess the highest gene density of all human chromosomes [ 22 , 23 ], we next analyzed the number of breast cancer-associated loci relative to the number of genes present in each chromosome (Fig. 4 b). The presence of increased breast cancer-associated loci in chromosome 17 and 19 correlates with the presence of larger number of genes in these chromosomes (Fig. 4 b).
Manual curation of rare-monogenic variants
Breast cancer gene panels are commonly used by diagnostic laboratories to identify disease etiology among patients. The gene panels include genes with a well-documented association between a rare-monogenic variant and breast cancer (e.g., BRCA1 , BRCA2 and PALB2 ). Inclusion of bonafide breast cancer-associated genes in future diagnostic gene panels would increase the odds of uncovering disease etiology among patients. Hence, we manually annotated and collated the breast cancer genes in which a previous association between rare-monogenic variant and breast cancer were established.
In total, we identified 459 genes with breast cancer-associated variants (Fig. 5 ). In order to annotate and collate the rare-monogenic variant containing breast cancer genes, we set out the following criteria: (1) genes should contain at least one rare variant (49 genes); (2) these rare variants should be rare across all population (45 genes); (3) these rare variants should be present in protein coding genes (43 genes); and (4) these rare variants should be present in the coding regions of a gene and not in intron (39 genes) (Fig. 5 ). The 39 genes that we identified to contain disease-causing monogenic variants are ABRAXAS1, APC, ATM, BARD1, BLM, BRCA1, BRCA2, BRIP1, CDH1, CHEK2, ERBB2, FANCC, FANCD2, FANCM, HOXB13, MCPH1, MEN1, MRE11, MSH2, MSH6, MUTYH, NBN, NF1, PALB2, PMS2, POLG, PPM1D, PTEN, RAD50, RAD51C, RAD51D, RBBP8, RECQL, RINT1, SERPINA3, STK11, TEX15, TP53, and XRCC2 (Fig. 5 ).
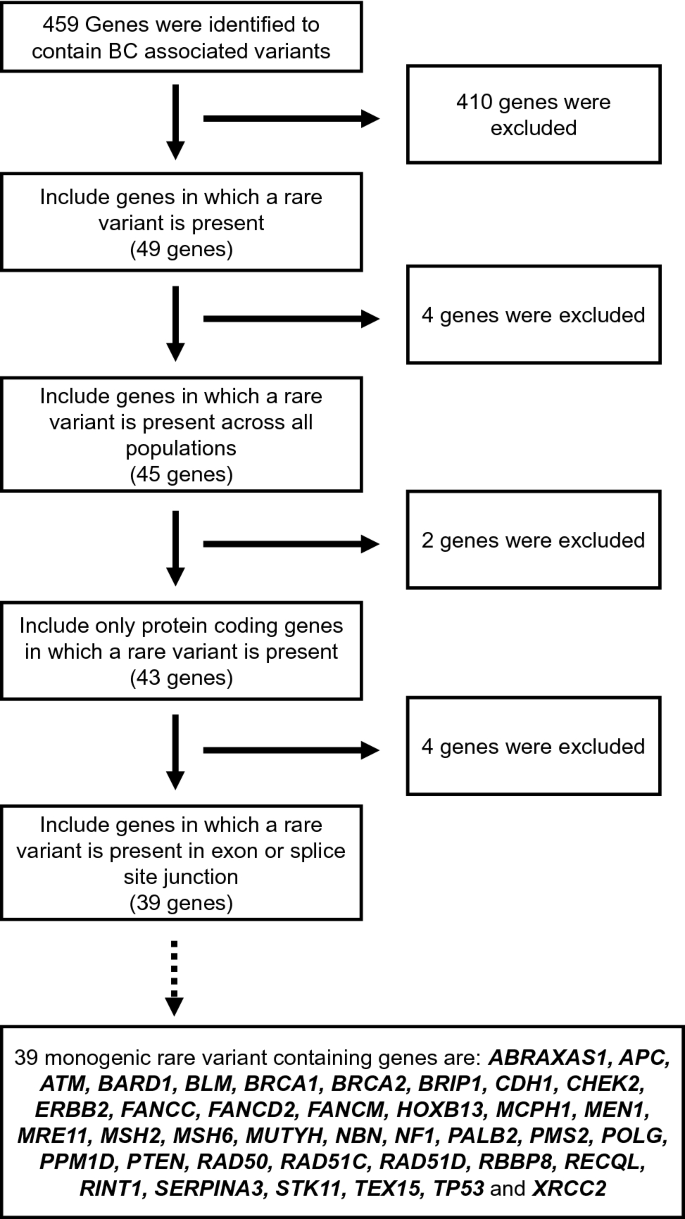
Flow chart outlining the different criteria used to annotate and collate the rare-monogenic variant containing breast cancer genes. Out of the 459 breast cancer genes, our manual curation effort has identified 39 genes to contain disease-causing monogenic variants
A majority of these are well-known cancer syndrome genes or genes that maintain genomic stability, such breast cancer genes are marked with red dots and annotated, respectively, in Fig. 3 . The majority of the 39 monogenic rare variant containing genes are either well-known tumor suppressors or suspected to have a tumor suppressor role, whereas only PPM1D and ERBB2 are classified as bonafide oncogenes by the Cancer Gene Census [ 24 ]. Of note, Chromosome 17 contains many rare-monogenic variants containing breast cancer genes (Fig. 3 ). Using the new platform ( https://cbcg.dk ), this monogenic breast cancer gene list will be continually updated for clinical and diagnostic purposes.
Gene-set enrichment analyses
To identify enrichment of specific molecular pathways and biological processes in the cataloged 459 breast cancer-associated genes, we performed both network analysis and functional enrichment analysis. The protein network analysis performed using cytoscape/String revealed a major cluster enriched among the DNA repair pathways, attributable to the rare-monogenic variant containing breast cancer genes (red dots) that were mainly present within this cluster (Fig. 6 ). On the contrary, a vast majority of the common-polygenic variant containing breast cancer genes (blue dots) were devoid of any protein–protein interaction and thus lacking pathway clustering (Fig. 6 ).
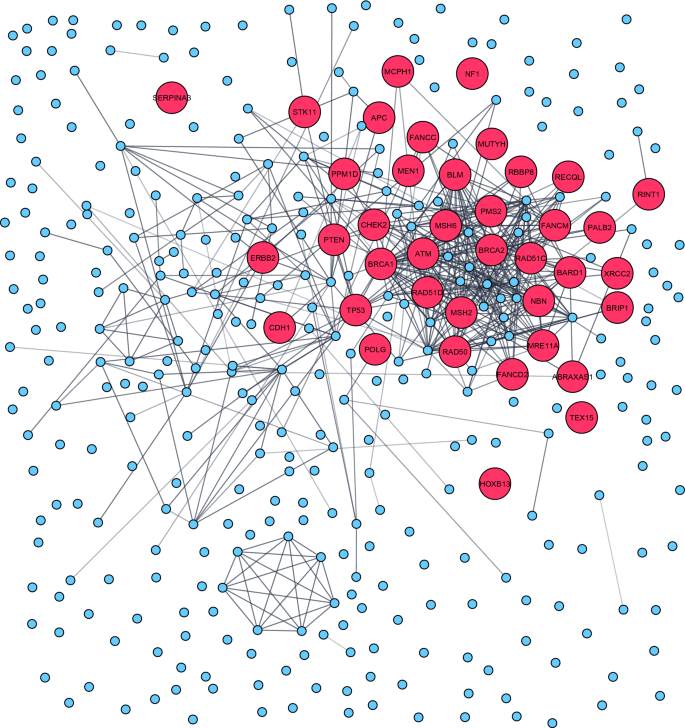
Protein network analysis performed in the 459 breast cancer genes revealed a major cluster enriched among the DNA repair pathways. Rare-monogenic variant containing breast cancer genes (red dots) was mainly present within this cluster. The protein–protein interaction network was constructed using STRING database [ 16 ] and graphically adjusted in Cytoscape [ 17 ]
To further characterize, we next performed functional enrichment analysis using the g:Profiler [ 18 ] g:GOSt tool. In agreement with network analysis, the functional enrichment analysis (using KEGG) also indicated DNA repair pathways such as homologous recombination and Fanconi anemia to be significantly enriched among the cataloged breast cancer-associated genes (Supplementary Table 1). These DNA repair pathways together with platinum drug resistance pathway comprise the majority of annotated rare-monogenic variant containing breast cancer genes (Supplementary Table 1). However, pathways such as steroid hormone biosynthesis, chemical carcinogenesis, and metabolism of xenobiotics by cytochrome P450 were found to be significantly enriched among the common-polygenic variant containing breast cancer genes (Supplementary Table 1). Thus, the results from both network and functional enrichment analysis indicate that the rare-monogenic and common-polygenic variant containing breast cancer genes were implicated mainly in DNA repair and steroid metabolism, respectively.
The potential use of breast cancer genes and variants during clinical management is prodigious, thus, identification of new factors responsible for breast cancer etiology is of paramount importance. An expedited translation of these newly identified breast cancer genes and variants could greatly augment personalized breast cancer treatment. However, with a constant influx of new studies, it is challenging to rapidly integrate these new findings into clinical use. The presence of a database comprising breast cancer-associated factors would enable rapid translation into clinical diagnostics. However, to the best of our knowledge, currently there is no database that archives known breast cancer genes and variants. Thus, there is a pressing need for an interactive and accessible database of curated breast cancer susceptibility genes.
We built a database of curated breast cancer genes ( https://cbcg.dk ) that can be readily used by both breast cancer researchers and clinicians. The main novelty of the study and linked database are that every breast cancer genes/variant, its rsID, SNP location, genomic location, AF and whether it is a potentially disease-causing genetic variant have been carefully and stringently curated. Another novelty of this study is the compilation of 39 genes that were identified to contain disease-causing monogenic variants. We believe that this database will not only readily provide information for both breast cancer researchers and clinicians but also help in saving their time. It is our view to provide continual updates of the data repository by curating new breast cancer genes/variants, most importantly, monogenic breast cancer gene list will be continually updated for clinical and diagnostic purposes.
Identification of breast cancer-associated rare-monogenic variants are typically performed using targeted gene sequencing that utilizes a focused panel of selected genes. The genes included in these breast cancer multigene panels are different among vendors (for a list of commonly used breast cancer multigene panel, please read Easton, DF et al. [ 25 ]). The only similarity between these multigene panels is that they mainly focus on DNA repair genes, other than that, there exists no clear consensus on the design of these multigene breast cancer panels [ 26 ]. One important aspect while designing a future (or custom) breast cancer multigene panel is to consider maximizing the likelihood of uncovering breast cancer-associated rare variants among the patients. We believe that including genes in which a previous association between rare-monogenic variant and breast cancer has been documented would maximize the odds of uncovering breast cancer-associated alterations.
A list of these breast cancer genes could be also used by the clinicians to narrow their search of breast cancer-associated alteration in the WES or WGS of patient data. Currently, to the best of our knowledge there exists no curated breast cancer gene list that could facilitate the screening of rare-monogenic variants. Therefore, we manually annotated and collated 39 breast cancer genes in which a previous association between rare-monogenic variant and breast cancer has been documented. Interestingly, 28 out of these 39 breast cancer genes were included in the screening panel (comprising 34 genes) of a recent study that aimed to identify overall breast cancer risk in more than 113,000 women [ 27 ]. This further exemplifies the appropriateness of our database in clinical high-throughput sequencing approaches such as multigene panel testing or in-silico panel testing from WES or WGS platforms. As the most cost-effective sequence method is soon to be the WGS, it enables the option of increasing the in-silico gene panel in clinical screening of breast cancer patient. However, as recently shown from a consortium of international breast cancer genetic screening laboratories, the gene panels (in-silico or capture based) are far from compatible, nor is it possible to update with the constant flow of new knowledge [ 26 ].
Dissecting of breast cancer genes includes not only the rare-monogenic variants but also the growing number of common-polygenic variants. While the individual common-polygenic variants have small effects on disease risk, cumulatively, they can cause an increased disease risk, similar to that of rare-monogenic variant [ 7 ]. Utilizing the GWAS identified common-polygenic variants, PRS are estimated and the prospect of utilizing PRS as a clinical tool is gaining traction [ 28 ]. Already, some clinics have chosen to offer a polygenic risk calculation through commercial test laboratories [ 29 ]. Translating breast cancer-associated SNPs into clinical practice is troublesome and there is currently a considerable debate over the clinical utility of PRS to assess breast cancer risk [ 11 ]. Although evidence for support of implementation of PRS into clinical practice is sparse, there is no doubt that PRS will play an enormous role in the future population screening programs, providing healthy persons a personalized risk assessment and managing tools [ 30 ]. For researchers and stakeholders, it is possible to assess the breast cancer-associated SNPs through the GWAS Catalog ( https://www.ebi.ac.uk/gwas/ ). We believe that the https://cbcg.dk database could also aid in the implementation of PRS into clinical practice.
There are few shortcomings in this current database mainly concerning the inability of us to provide unambiguous information about the population and breast cancer subtype for every curated breast cancer gene/variant. Moreover, during the construction of this database, we have also observed a great disparity between study populations among the enrolled studies, with most involving European/Caucasian patients. The genetic discovery efforts to date heavily underrepresent non-European populations globally and this has serious impact during PRS estimation in non-European patients. It has been shown several times that PRS predicts individual risk far more accurately in Europeans when compared to non-Europeans due to the overwhelming abundance of GWAS studies conducted in participants of European descent [ 31 ].
It is by now well established that the majority of known rare causal germline breast cancer genes are involved in genome maintenances pathways (Fig. 6 ). However, when searching for new causal breast cancer genes it is relevant to unravel if entirely new or interacting pathways are potential areas to seek for causal monogenic variants. We believe that our database could serve as an inspiration to find these new pathways where new breast cancer causal genes could function. Keeping this in mind, we have built an interactive and accessible database of curated breast cancer genes ( https://cbcg.dk ), to support the ongoing efforts in managing breast cancer etiology.
Data availability
The datasets generated during and/or analyzed during the current study are available in the [ https://cbcg.dk ] repository.
Code availability
Not applicable.
Vineis P, Wild CP (2014) Global cancer patterns: causes and prevention. Lancet (London, England) 383:549–557. https://doi.org/10.1016/s0140-6736(13)62224-2
Article Google Scholar
Sung H, Ferlay J, Siegel RL, Laversanne M, Soerjomataram I, Jemal A, Bray F (2021) Global Cancer Statistics 2020: GLOBOCAN estimates of incidence and mortality worldwide for 36 cancers in 185 Countries. CA Cancer J Clin 71:209–249. https://doi.org/10.3322/caac.21660
Article PubMed Google Scholar
Ellisen LW, Haber DA (1998) Hereditary breast cancer. Annu Rev Med 49:425–436. https://doi.org/10.1146/annurev.med.49.1.425
Article CAS PubMed Google Scholar
Mucci LA, Hjelmborg JB, Harris JR, Czene K, Havelick DJ, Scheike T, Graff RE, Holst K, Möller S, Unger RH, McIntosh C, Nuttall E, Brandt I, Penney KL, Hartman M, Kraft P, Parmigiani G, Christensen K, Koskenvuo M, Holm NV, Heikkilä K, Pukkala E, Skytthe A, Adami HO, Kaprio J (2016) Familial risk and heritability of cancer among twins in Nordic Countries. JAMA 315:68–76. https://doi.org/10.1001/jama.2015.17703
Article CAS PubMed PubMed Central Google Scholar
Feliubadaló L, Tonda R, Gausachs M, Trotta JR, Castellanos E, López-Doriga A, Teulé À, Tornero E, Del Valle J, Gel B, Gut M, Pineda M, González S, Menéndez M, Navarro M, Capellá G, Gut I, Serra E, Brunet J, Beltran S, Lázaro C (2017) Benchmarking of whole exome sequencing and Ad hoc designed panels for genetic testing of hereditary cancer. Sci Rep 7:37984. https://doi.org/10.1038/srep37984
Rossing M, Sørensen CS, Ejlertsen B, Nielsen FC (2019) Whole genome sequencing of breast cancer. APMIS 127:303–315. https://doi.org/10.1111/apm.12920
Article PubMed PubMed Central Google Scholar
Fahed AC, Wang M, Homburger JR, Patel AP, Bick AG, Neben CL, Lai C, Brockman D, Philippakis A, Ellinor PT, Cassa CA, Lebo M, Ng K, Lander ES, Zhou AY, Kathiresan S, Khera AV (2020) Polygenic background modifies penetrance of monogenic variants for tier 1 genomic conditions. Nat Commun 11:3635. https://doi.org/10.1038/s41467-020-17374-3
Shiovitz S, Korde LA (2015) Genetics of breast cancer: a topic in evolution. Ann Oncol 26:1291–1299. https://doi.org/10.1093/annonc/mdv022
Melchor L, Benítez J (2013) The complex genetic landscape of familial breast cancer. Hum Genet 132:845–863. https://doi.org/10.1007/s00439-013-1299-y
Michailidou K, Lindström S, Dennis J, Beesley J, Hui S, Kar S, Lemaçon A, Soucy P, Glubb D, Rostamianfar A, Bolla MK, Wang Q, Tyrer J, Dicks E, Lee A, Wang Z, Allen J, Keeman R, Eilber U, French JD, Qing Chen X, Fachal L, McCue K, McCart Reed AE, Ghoussaini M, Carroll JS, Jiang X, Finucane H, Adams M, Adank MA, Ahsan H, Aittomäki K, Anton-Culver H, Antonenkova NN, Arndt V, Aronson KJ, Arun B, Auer PL, Bacot F, Barrdahl M, Baynes C, Beckmann MW, Behrens S, Benitez J, Bermisheva M, Bernstein L, Blomqvist C, Bogdanova NV, Bojesen SE, Bonanni B, Børresen-Dale AL, Brand JS, Brauch H, Brennan P, Brenner H, Brinton L, Broberg P, Brock IW, Broeks A, Brooks-Wilson A, Brucker SY, Brüning T, Burwinkel B, Butterbach K, Cai Q, Cai H, Caldés T, Canzian F, Carracedo A, Carter BD, Castelao JE, Chan TL, David Cheng TY, Seng Chia K, Choi JY, Christiansen H, Clarke CL, Collée M, Conroy DM, Cordina-Duverger E, Cornelissen S, Cox DG, Cox A, Cross SS, Cunningham JM, Czene K, Daly MB, Devilee P, Doheny KF, Dörk T, Dos-Santos-Silva I, Dumont M, Durcan L, Dwek M, Eccles DM, Ekici AB, Eliassen AH, Ellberg C, Elvira M, Engel C et al (2017) Association analysis identifies 65 new breast cancer risk loci. Nature 551:92–94. https://doi.org/10.1038/nature24284
Yanes T, Young MA, Meiser B, James PA (2020) Clinical applications of polygenic breast cancer risk: a critical review and perspectives of an emerging field. Breast Cancer Res 22:21. https://doi.org/10.1186/s13058-020-01260-3
Pujana MA (2014) Integrating germline and somatic data towards a personalized cancer medicine. Trends Mol Med 20:413–415. https://doi.org/10.1016/j.molmed.2014.05.004
Altman DG (1991) Practical statistics for medical research. Chapman and Hall, London
Karczewski KJ, Francioli LC, Tiao G, Cummings BB, Alföldi J, Wang Q, Collins RL, Laricchia KM, Ganna A, Birnbaum DP, Gauthier LD, Brand H, Solomonson M, Watts NA, Rhodes D, Singer-Berk M, England EM, Seaby EG, Kosmicki JA, Walters RK, Tashman K, Farjoun Y, Banks E, Poterba T, Wang A, Seed C, Whiffin N, Chong JX, Samocha KE, Pierce-Hoffman E, Zappala Z, O’Donnell-Luria AH, Minikel EV, Weisburd B, Lek M, Ware JS, Vittal C, Armean IM, Bergelson L, Cibulskis K, Connolly KM, Covarrubias M, Donnelly S, Ferriera S, Gabriel S, Gentry J, Gupta N, Jeandet T, Kaplan D, Llanwarne C, Munshi R, Novod S, Petrillo N, Roazen D, Ruano-Rubio V, Saltzman A, Schleicher M, Soto J, Tibbetts K, Tolonen C, Wade G, Talkowski ME, Aguilar Salinas CA, Ahmad T, Albert CM, Ardissino D, Atzmon G, Barnard J, Beaugerie L, Benjamin EJ, Boehnke M, Bonnycastle LL, Bottinger EP, Bowden DW, Bown MJ, Chambers JC, Chan JC, Chasman D, Cho J, Chung MK, Cohen B, Correa A, Dabelea D, Daly MJ, Darbar D, Duggirala R, Dupuis J, Ellinor PT, Elosua R, Erdmann J, Esko T, Färkkilä M, Florez J, Franke A, Getz G, Glaser B, Glatt SJ, Goldstein D, Gonzalez C, Groop L et al (2020) The mutational constraint spectrum quantified from variation in 141,456 humans. Nature 581:434–443. https://doi.org/10.1038/s41586-020-2308-7
Wolfe D, Dudek S, Ritchie MD, Pendergrass SA (2013) Visualizing genomic information across chromosomes with PhenoGram. BioData Min 6:18. https://doi.org/10.1186/1756-0381-6-18
Szklarczyk D, Gable AL, Lyon D, Junge A, Wyder S, Huerta-Cepas J, Simonovic M, Doncheva NT, Morris JH, Bork P, Jensen LJ, von Christian M (2018) STRING v11: protein–protein association networks with increased coverage, supporting functional discovery in genome-wide experimental datasets. Nucleic Acids Res 47:D607–D613. https://doi.org/10.1093/nar/gky1131
Article CAS PubMed Central Google Scholar
Shannon P, Markiel A, Ozier O, Baliga NS, Wang JT, Ramage D, Amin N, Schwikowski B, Ideker T (2003) Cytoscape: a software environment for integrated models of biomolecular interaction networks. Genome Res 13:2498–2504. https://doi.org/10.1101/gr.1239303
Raudvere U, Kolberg L, Kuzmin I, Arak T, Adler P, Peterson H, Vilo J (2019) g:Profiler: a web server for functional enrichment analysis and conversions of gene lists (2019 update). Nucleic Acids Res 47:W191–W198. https://doi.org/10.1093/nar/gkz369
Singletary SE (2003) Rating the risk factors for breast cancer. Ann Surg 237:474–482. https://doi.org/10.1097/01.SLA.0000059969.64262.87
Fagny M, Platig J, Kuijjer ML, Lin X, Quackenbush J (2020) Nongenic cancer-risk SNPs affect oncogenes, tumour-suppressor genes, and immune function. Br J Cancer 122:569–577. https://doi.org/10.1038/s41416-019-0614-3
Bartonicek N, Clark MB, Quek XC, Torpy JR, Pritchard AL, Maag JLV, Gloss BS, Crawford J, Taft RJ, Hayward NK, Montgomery GW, Mattick JS, Mercer TR, Dinger ME (2017) Intergenic disease-associated regions are abundant in novel transcripts. Genome Biol 18:241–241. https://doi.org/10.1186/s13059-017-1363-3
Grimwood J, Gordon LA, Olsen A, Terry A, Schmutz J, Lamerdin J, Hellsten U, Goodstein D, Couronne O, Tran-Gyamfi M, Aerts A, Altherr M, Ashworth L, Bajorek E, Black S, Branscomb E, Caenepeel S, Carrano A, Caoile C, Chan YM, Christensen M, Cleland CA, Copeland A, Dalin E, Dehal P, Denys M, Detter JC, Escobar J, Flowers D, Fotopulos D, Garcia C, Georgescu AM, Glavina T, Gomez M, Gonzales E, Groza M, Hammon N, Hawkins T, Haydu L, Ho I, Huang W, Israni S, Jett J, Kadner K, Kimball H, Kobayashi A, Larionov V, Leem SH, Lopez F, Lou Y, Lowry S, Malfatti S, Martinez D, McCready P, Medina C, Morgan J, Nelson K, Nolan M, Ovcharenko I, Pitluck S, Pollard M, Popkie AP, Predki P, Quan G, Ramirez L, Rash S, Retterer J, Rodriguez A, Rogers S, Salamov A, Salazar A, She X, Smith D, Slezak T, Solovyev V, Thayer N, Tice H, Tsai M, Ustaszewska A, Vo N, Wagner M, Wheeler J, Wu K, Xie G, Yang J, Dubchak I, Furey TS, DeJong P, Dickson M, Gordon D, Eichler EE, Pennacchio LA, Richardson P, Stubbs L, Rokhsar DS, Myers RM, Rubin EM, Lucas SM (2004) The DNA sequence and biology of human chromosome 19. Nature 428:529–535. https://doi.org/10.1038/nature02399
Zody MC, Garber M, Adams DJ, Sharpe T, Harrow J, Lupski JR, Nicholson C, Searle SM, Wilming L, Young SK, Abouelleil A, Allen NR, Bi W, Bloom T, Borowsky ML, Bugalter BE, Butler J, Chang JL, Chen C-K, Cook A, Corum B, Cuomo CA, de Jong PJ, DeCaprio D, Dewar K, FitzGerald M, Gilbert J, Gibson R, Gnerre S, Goldstein S, Grafham DV, Grocock R, Hafez N, Hagopian DS, Hart E, Norman CH, Humphray S, Jaffe DB, Jones M, Kamal M, Khodiyar VK, LaButti K, Laird G, Lehoczky J, Liu X, Lokyitsang T, Loveland J, Lui A, Macdonald P, Major JE, Matthews L, Mauceli E, McCarroll SA, Mihalev AH, Mudge J, Nguyen C, Nicol R, O’Leary SB, Osoegawa K, Schwartz DC, Shaw-Smith C, Stankiewicz P, Steward C, Swarbreck D, Venkataraman V, Whittaker CA, Yang X, Zimmer AR, Bradley A, Hubbard T, Birren BW, Rogers J, Lander ES, Nusbaum C (2006) DNA sequence of human chromosome 17 and analysis of rearrangement in the human lineage. Nature 440:1045–1049. https://doi.org/10.1038/nature04689
Sondka Z, Bamford S, Cole CG, Ward SA, Dunham I, Forbes SA (2018) The COSMIC cancer gene census: describing genetic dysfunction across all human cancers. Nat Rev Cancer 18:696–705. https://doi.org/10.1038/s41568-018-0060-1
Easton DF, Pharoah PDP, Antoniou AC, Tischkowitz M, Tavtigian SV, Nathanson KL, Devilee P, Meindl A, Couch FJ, Southey M, Goldgar DE, Evans DGR, Chenevix-Trench G, Rahman N, Robson M, Domchek SM, Foulkes WD (2015) Gene-panel sequencing and the prediction of breast-cancer risk. N Engl J Med 372:2243–2257. https://doi.org/10.1056/NEJMsr1501341
Nielsen SM, Eccles DM, Romero IL, Al-Mulla F, Balmaña J, Biancolella M, Bslok R, Caligo MA, Calvello M, Capone GL, Cavalli P, Chan TLC, Claes KBM, Cortesi L, Couch FJ, de la Hoya M, De Toffol S, Diez O, Domchek SM, Eeles R, Efremidis A, Fostira F, Goldgar D, Hadjisavvas A, Hansen TVO, Hirasawa A, Houdayer C, Kleiblova P, Krieger S, Lázaro C, Loizidou M, Manoukian S, Mensenkamp AR, Moghadasi S, Monteiro AN, Mori L, Morrow A, Naldi N, Nielsen HR, Olopade OI, Pachter NS, Palmero EI, Pedersen IS, Piane M, Puzzo M, Robson M, Rossing M, Sini MC, Solano A, Soukupova J, Tedaldi G, Teixeira M, Thomassen M, Tibiletti MG, Toland A, Törngren T, Vaccari E, Varesco L, Vega A, Wallis Y, Wappenschmidt B, Weitzel J, Spurdle AB, De Nicolo A, Gómez-García EB (2018) Genetic testing and clinical management practices for variants in non-BRCA1/2 Breast (and Breast/Ovarian) cancer susceptibility genes: an International Survey by the evidence-based network for the interpretation of germline mutant alleles (ENIGMA) Clinical Working Group. JCO Precis Oncol. https://doi.org/10.1200/po.18.00091
Breast Cancer Association Consortium (2021) Breast cancer risk genes—association analysis in more than 113,000 women. N Engl J Med 384:428–439. https://doi.org/10.1056/NEJMoa1913948
De La Vega FM, Bustamante CD (2018) Polygenic risk scores: a biased prediction? Genome Med 10:100. https://doi.org/10.1186/s13073-018-0610-x
Hughes E, Tshiaba P, Gallagher S, Wagner S, Judkins T, Roa B, Rosenthal E, Domchek S, Garber J, Lancaster J, Weitzel J, Kurian AW, Lanchbury JS, Gutin A, Robson M (2020) Development and validation of a clinical polygenic risk score to predict breast cancer risk. JCO Precis Oncol. https://doi.org/10.1200/po.19.00360
Janssens A, Joyner MJ (2019) Polygenic risk scores that predict common diseases using millions of single nucleotide polymorphisms: Is more, better? Clin Chem 65:609–611. https://doi.org/10.1373/clinchem.2018.296103
Martin AR, Kanai M, Kamatani Y, Okada Y, Neale BM, Daly MJ (2019) Clinical use of current polygenic risk scores may exacerbate health disparities. Nat Genet 51:584–591. https://doi.org/10.1038/s41588-019-0379-x
Download references
MB is funded by Lundbeck Foundation (R223-2016-956) and Danish Cancer Society (R269-A15884). JB is funded by Danish Cancer Society (R204-A12415). JVT is funded by Marie Curie Individual Fellowship (896102). FCN is funded by Lundbeck Foundation (R223-2016-956) and Research Council of the Capital Region of Denmark. CSS is funded by Danish Cancer Society, Danish Medical Research Council, and Lundbeck Foundation (R223-2016-956). MR is funded by Lundbeck Foundation (R223-2016-956) and Neye-Fonden.
Author information
Jan Benada and Jayashree Vijay Thatte have equally contributed to this work.
Authors and Affiliations
Biotech Research and Innovation Centre (BRIC), Faculty of Medical and Health Sciences, University of Copenhagen, Copenhagen, Denmark
Muthiah Bose, Jan Benada, Jayashree Vijay Thatte & Claus Storgaard Sørensen
Centre for Genomic Medicine, Rigshospitalet, Copenhagen University Hospital, Blegdamsvej 9, 2100, Copenhagen, Denmark
Muthiah Bose, Savvas Kinalis, Finn Cilius Nielsen & Maria Rossing
Department of Clinical Oncology, Rigshospitalet, Copenhagen University Hospital, Copenhagen, Denmark
Bent Ejlertsen
Department of Clinical Medicine, University of Copenhagen, Copenhagen, Denmark
Maria Rossing
You can also search for this author in PubMed Google Scholar
Contributions
MR, CSS, and FCN conceived, designed, and oversaw the study. MR and MB set out the criteria for study selection and data extraction. MB, JB, and JVT performed the literature search, data extraction, and analysis. SV created the database. MB and MR drafted the manuscript and all authors contributed to the critical review of the paper.
Corresponding author
Correspondence to Maria Rossing .
Ethics declarations
Conflict of interest.
The authors declare that they have no potential competing interests.
Ethical approval
Consent to participate, consent for publication, additional information, publisher's note.
Springer Nature remains neutral with regard to jurisdictional claims in published maps and institutional affiliations.
Supplementary Information
Below is the link to the electronic supplementary material.
10549_2021_6441_MOESM1_ESM.xlsx
Supplementary Table 1: Functional enrichment analysis performed in the 459 breast cancer genes using the g:Profiler [18] g:GOSt tool. Results from the analysis performed in data sources such as GO MF, GO CC, GO BP, KEGG, Reactome and WikiPathways are reported in separate tabs. (XLSX 348 kb)
Rights and permissions
Open Access This article is licensed under a Creative Commons Attribution 4.0 International License, which permits use, sharing, adaptation, distribution and reproduction in any medium or format, as long as you give appropriate credit to the original author(s) and the source, provide a link to the Creative Commons licence, and indicate if changes were made. The images or other third party material in this article are included in the article's Creative Commons licence, unless indicated otherwise in a credit line to the material. If material is not included in the article's Creative Commons licence and your intended use is not permitted by statutory regulation or exceeds the permitted use, you will need to obtain permission directly from the copyright holder. To view a copy of this licence, visit http://creativecommons.org/licenses/by/4.0/ .
Reprints and permissions
About this article
Bose, M., Benada, J., Thatte, J.V. et al. A catalog of curated breast cancer genes. Breast Cancer Res Treat 191 , 431–441 (2022). https://doi.org/10.1007/s10549-021-06441-y
Download citation
Received : 09 August 2021
Accepted : 21 October 2021
Published : 10 November 2021
Issue Date : January 2022
DOI : https://doi.org/10.1007/s10549-021-06441-y
Share this article
Anyone you share the following link with will be able to read this content:
Sorry, a shareable link is not currently available for this article.
Provided by the Springer Nature SharedIt content-sharing initiative
- Breast cancer
- Genetic predisposition
- Rare-monogenic variants
- Common-polygenic variants
- DNA repair pathways
- Find a journal
- Publish with us
- Track your research
- Open access
- Published: 27 May 2024
Genomic dissection and mutation-specific target discovery for breast cancer PIK3CA hotspot mutations
- Adam X. Miranda 1 ,
- Justin Kemp 1 ,
- Brad A. Davidson 1 ,
- Sara Erika Bellomo 3 ,
- Verda E. Miranda 2 ,
- Alexandra Manoni 1 ,
- Caterina Marchiò 3 , 4 ,
- Sarah Croessmann 1 ,
- Ben H. Park 1 , 5 &
- Emily Hodges 2 , 5 , 6
BMC Genomics volume 25 , Article number: 519 ( 2024 ) Cite this article
267 Accesses
Metrics details
Recent advancements in high-throughput genomics and targeted therapies have provided tremendous potential to identify and therapeutically target distinct mutations associated with cancers. However, to date the majority of targeted therapies are used to treat all functional mutations within the same gene, regardless of affected codon or phenotype.
In this study, we developed a functional genomic analysis workflow with a unique isogenic cell line panel bearing two distinct hotspot PIK3CA mutations, E545K and H1047R, to accurately identify targetable differences between mutations within the same gene. We performed RNA-seq and ATAC-seq and identified distinct transcriptomic and epigenomic differences associated with each PIK3CA hotspot mutation. We used this data to curate a select CRISPR knock out screen to identify mutation-specific gene pathway vulnerabilities. These data revealed AREG as a E545K-preferential target that was further validated through in vitro analysis and publicly available patient databases.
Conclusions
Using our multi-modal genomics framework, we discover distinct differences in genomic regulation between PIK3CA hotspot mutations, suggesting the PIK3CA mutations have different regulatory effects on the function and downstream signaling of the PI3K complex. Our results demonstrate the potential to rapidly uncover mutation specific molecular targets, specifically AREG and a proximal gene regulatory region, that may provide clinically relevant therapeutic targets. The methods outlined provide investigators with an integrative strategy to identify mutation-specific targets for the treatment of other oncogenic mutations in an isogenic system.
Peer Review reports
In the past few decades, significant strides in precision medicine and the advancement of targeted therapies have led to personalized treatment options and improved outcomes for patients with cancer, while limiting off-target toxicities. However, response to treatment still varies widely and the ability to better identify patients that would benefit from targeted therapies remains complex [ 1 ]. For the most part, current clinical practices regard mutations within the same gene as clinically equivalent despite distinct molecular differences, creating a significant obstacle in the implementation of targeted therapies [ 2 , 3 ]. PIK3CA , which encodes the p110α subunit of phosphoinositide 3-kinase (PI3K), is the most commonly mutated gene in breast cancer and is responsible for regulating a diverse range of cellular functions including cell proliferation and survival [ 4 , 5 , 6 ]. PIK3CA has two distinct and highly prevalent hotspot mutations, E545K and H1047R, which occur in the helical and kinase domains, respectively. Mutations in PIK3CA are more common in the luminal A subtype of breast cancer and occur at a lower frequency in the triple negative subtype. Yet, the hotspot mutations of PIK3CA consistently occur at roughly a 2:1 (H1047R:E545K) ratio regardless of breast cancer subtype (Fig. S1 and Table S1 )[ 7 ]. These two hotspot mutations have been shown to have distinct molecular changes and sensitivity to targeted therapeutics [ 5 , 6 , 8 , 9 , 10 ]. Despite these differences, current clinical application of PI3Kinase inhibitors in breast cancer do not distinguish between different mutations or between normal and mutated PI3K. This results in significant issues of toxicity, often times leading to dose reduction or discontinuation of the drug [ 2 , 11 , 12 , 13 , 14 ]. To date, there is a distinct unmet need in the treatment of cancer, to accurately identify and understand molecular differences between mutations to effectively target cancer cells, improve selectivity, and decrease off-target effects.
Recent advancements in genomics technology and the affordability of generating high throughput genomics data have allowed researchers to begin to better understand the nuanced differences between mutations within the same gene. Furthermore, bioinformatic efforts have begun integrating transcriptomic and epigenomic data to better understand distinct molecular differences among unique mutational profiles from cancer patients. However, due to the significant mutational variability among individual cancers, as well as tumor heterogeneity and clonality, attributing observed differences to a single mutation has proven difficult. To better understand the molecular differences of PIK3CA hotspot mutations, our group has developed an integrative discovery platform to better identify key differences induced by different PIK3CA hotspot mutations in an isogenic human breast epithelial cell line panel [ 15 ]. The utilization of an isogenic mutation panel allows comparisons of the individual PIK3CA mutations under the expression of the endogenous promoter in near isolation, allowing for the identification of potential mutation-specific and mutation-preferential therapeutic targets.
The discovery platform presented here integrates RNA-seq, an assay for transposase-accessible chromatin with sequencing (ATAC-seq), and a select CRISPR knockout (KO) screen to uniquely identify distinct molecular targets attributed to either the PIK3CA E545K or H1047R mutations within a well-controlled model. RNA-seq allows for the identification and quantification of genes and pathways with altered expression due to the presence of either mutation [ 16 ]. ATAC-seq measures chromatin accessibility and can identify putative gene regulatory elements to provide additional insight into how regulation of genes and binding activities of transcription factors differ between two mutations within the same gene [ 17 , 18 , 19 ]. In our framework, data from these two assays are used to tailor a CRISPR screen that can accurately confirm genes with high essentiality in either mutant cell line; in doing so, we identify potential mutation-specific targets for treatment [ 20 , 21 , 22 ]. Combined application of these assays provides improved understanding of differences in cell function induced by distinct hotspot mutants as well as providing potential means of mutation-preferential inhibition.
Herein we describe a systematic approach (Fig. 1 ) to identify potential mutation-preferential therapeutic targets. The utilization of an isogenic mammary epithelial cell model allows for the direct attribution of differences to specific mutations. This in return should improve selectivity of targeted therapies and decrease off-target effects. Our goal is to create a framework with “plug and play” accessibility for the evaluation of other hotspot mutants across cancer types using isogenic cell line models and to provide a foundation for future studies to identify a candidate list to maximize the potential for therapeutic benefit.
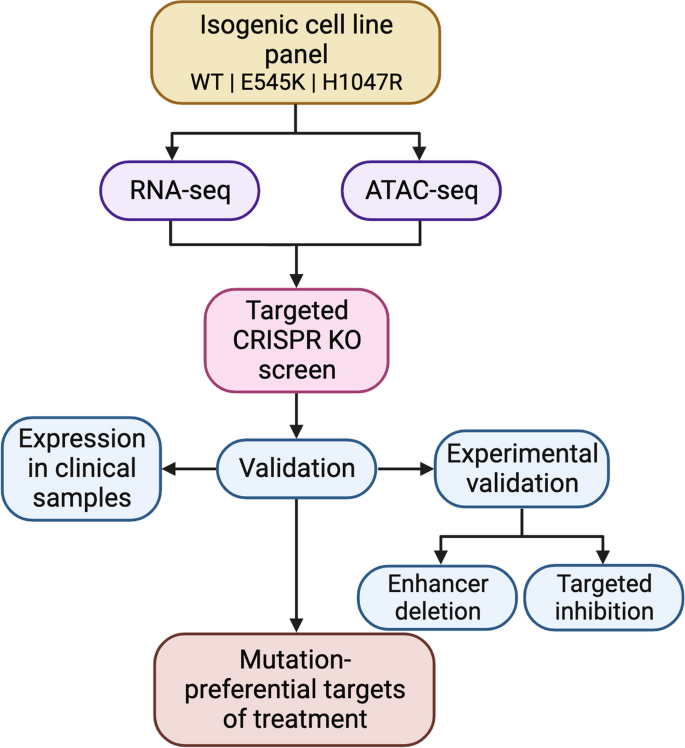
Discovery platform identifies mutation-preferential gene targets from isogenic cell line models. Flowchart breaking down the process of identifying selective gene targets from an isogenic cell line model
RNA sequencing uncovers distinct transcriptional profiles and differential regulation of key cancer pathways in E545K and H1047R PIK3CA mutant cells
To evaluate differences in the transcriptomes of cells harboring the PIK3CA hotspot mutations E545K and H1047R, we performed RNA-seq on a panel of isogenically modified nontumorigenic breast epithelial MCF-10A cell lines harboring the respective mutations. RNA-seq identified 1271 genes with differential expression between the two mutant cell lines (Fig. 2 A and B ) [ 23 ]. A complete summary of the differentially expressed genes (DEGs) can be found in Table S2 and are displayed in Fig. 2 B. Interestingly, hierarchical clustering revealed the gene expression patterns of the E545K cell line shared greater similarity with the WT parental cell line than the H1047R mutant cell line (Fig. 2 A). It is important to note that there is no differential expression of PIK3CA (Fig. S2 ). Thus, differential gene expression can be attributed to the effects of the mutations and not altered total expression of the mutant transcripts.
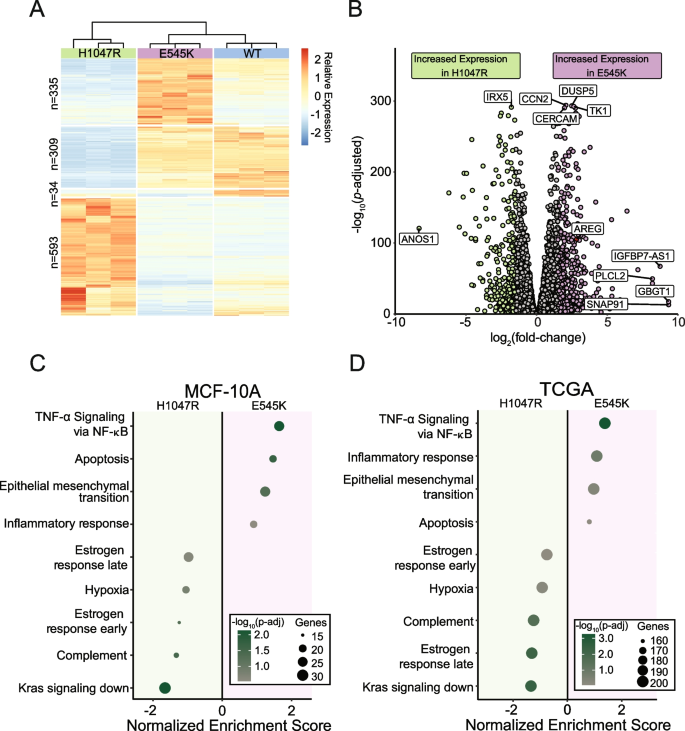
RNA-seq captures distinct gene expression differences induced by PIK3CA hotspot mutations in isogenic cell line models which are reflected in TCGA patient samples. ( A ) Heatmap of normalized counts for 1271 differentially expressed genes. Hierarchical clustering of these genes reveals that E545K cells bear more similarity to WT than H1047R. ( B ) Volcano plot of differential expression between mutant isogenic cell lines. Differentially expressed genes (DEGs) were defined by the criteria: fold change >|1.5|, P adj < 0.05. ( C , D ) Dot plot showing results from GSEA pathway enrichment analyses using the hallmark gene sets for ( C ) MCF-10A DEGs and ( D ) expression data from TCGA-BRCA samples. Pathways shown in panel D are those that are significantly enriched, shared and concordant with those identified as significant in the MCF-10A cell line
Gene set enrichment analysis using the MSigDB Hallmark pathway collection was performed to identify patterns of shared function across DEGs [ 24 ]. Multiple uniquely enriched pathways were associated with each mutant (Fig. 2 C). Genes within pathways related to cell cycle and proliferation, as well as epithelial-mesenchymal transition genes, exhibited greater increased expression in the E545K cells, while genes in estrogen response pathways and K-ras associated genes had greater increased expression in the H1047R cells. It is important to note that while the MCF-10A lineage is considered an ER- cell line, these cells do still express ESR1 mRNA (Fig. S2 ) and may therefore still exhibit changes in estrogen regulated genes. A more detailed look at the altered expression of genes within the estrogen response early and estrogen response late pathways can be found in Fig. S3 . These differences in gene expression patterns suggest distinct modes of tumorigenic activity between the different mutants, despite being treated as clinically equivalent. Using patient data from The Cancer Genome Atlas (TCGA) Breast Cancer (BRCA) data set, RNA-seq samples from tumors bearing each PIK3CA mutation confirmed observations made within our isogenic MCF-10A panel (Fig. 2 D). Of the 14 pathways found to be significantly enriched in our panel, all were confirmed to be significantly enriched in differentially expressed gene sets from corresponding TCGA mutant samples. These findings demonstrate single amino acid substitutions in the same gene can have wide-ranging and distinct disruption of gene expression, which translates directly to expression differences observed in clinical samples.
PIK3CA mutants demonstrate unique differences in chromatin accessibility and gene regulation
Considering the gene expression changes observed with RNA-seq, we performed ATAC-seq to identify genomic regions with altered regulatory landscapes, which may contribute to changes in gene expression. In addition to the identification of dynamic regions of chromatin accessibility (ChrAcc), differences in transcription factor (TF) binding activities were also estimated from Tn5 cut-site profiles. Comparing accessibility profiles between E545K and H1047R mutants identified 8672 differentially accessible regions. We performed unsupervised clustering to define 4 distinct groups of accessibility patterns (Fig. 3 A). Two distinct groups, designated as E545K-preferred and H1047R-preferred, represented putative regulatory loci with increased accessibility in either E545K or H1047R mutant cells, respectively. In addition to providing insight into gene regulatory mechanisms, ChrAcc provides insight into cis -regulatory elements including enhancers. Within both the E545K-preferred and H1047R-preferred region clusters, over 50% of the regions fall within intronic and distal intergenic sequences (Fig. 3 B). These results suggest that a significant amount of chromatin remodeling that is driven by the different PIK3CA mutations occurs at noncoding enhancer elements that can bind TFs and influence gene expression [ 25 ].
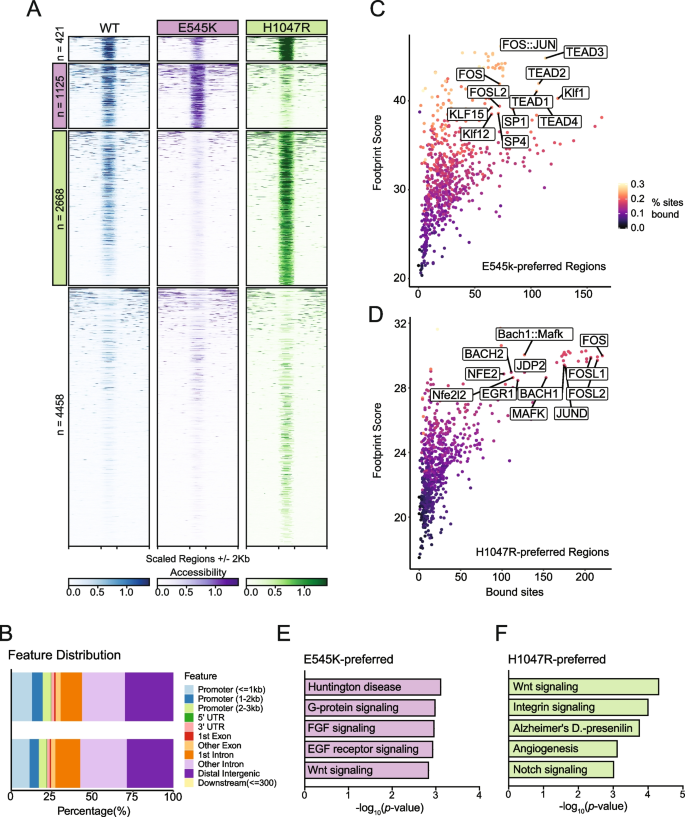
ATAC-Seq identifies mutation-specific gene regulatory mechanisms near genes of key pathways. ( A ) Heatmap of accessibility at 8672 peaks exhibiting differential accessibility between the mutant isogenic cell lines. Peaks are divided based on k-means clustering. The second cluster highlighted in pink has been designated as E545K-preferred. The third cluster highlighted in green has been designated as H1047R-preferred ( B ) Distribution of genomic feature annotations of regions within the E545K-preferred and H1047R-preferred clusters. ( C ) Scatter plots of TOBIAS transcription factor footprinting of accessibility in the E545K-preferred regions. ( D ) Scatter plots of TOBIAS transcription factor footprinting of accessibility in the H1047R-preferred regions. ( E ) Bar plot of pathway enrichment from the PANTHER pathway database analysis performed on genes uniquely annotated to the E545K-preferred cluster. ( F ) Bar plot of pathway enrichment from the PANTHER pathway database analysis performed on genes uniquely annotated to the H1047R-preferred cluster regions
Indeed, TF motif analysis revealed that each accessibility cluster is enriched for distinct families of TF binding sites identified from the JASPAR database [ 26 , 27 ] (Fig. S4 ). In the E545K-preferred cluster, strong enrichment for the hormone receptor transcription factors ARE and PGR were observed as well as the TEAD transcription factor family. The TEAD TF family has been shown to have a strong association with canonical PI3K/AKT signaling and can promote epithelial to mesenchymal transition [ 28 , 29 ]. In the H1047R-preferred cluster, there was increased enrichment of AP-1 family TFs. AP-1 family TFs have been shown to interact with chromatin remodelers and promote a proliferative gene expression program [ 30 , 31 ], and have also been associated with signaling through the MAPK cascade [ 32 ].
While TF motif analysis informs which sequences are enriched within ChrAcc regions, it does not predict TF occupancy. To better understand differential TF binding activities, we performed TF footprinting using TOBIAS, which uses Tn5 cut-site profiles to identify differences in proteins bound at TF binding motifs [ 18 ]. Our results show high levels of TEAD TF binding in E545K-preferred regions (Fig. 3 C) and high levels of AP-1 binding (FOS, FOSL1, FOSL2, JUND) in H1047R-preferred regions (Fig. 3 D). These results are consistent with the motif enrichment results and point to activity of the TEAD and AP-1 TF families as key regulators of differential gene expression between the PIK3CA hotspot mutants. The differential binding activity of TFs from these TF families are influenced by the PIK3CA mutation status of the cells and cofactors of these TFs likely alter the ChrAcc at these mutation-preferred regions. See discussion for more detailed description.
Nearest neighbor gene annotation using GREAT was used for gene ontology analysis to identify genes uniquely associated with either mutation-preferred accessibility cluster and analyzed for pathway enrichment using Enrichr [ 33 , 34 , 35 , 36 ]. Using the PANTHER database, we identified enrichment of distinct pathways promoted by either PIK3CA mutants [ 37 , 38 ]. Within the E545K-preferred cluster regions, unique enrichment of multiple growth factor receptor signaling pathways was observed and is likely due to changes in PI3Kα signaling induced by the E545K mutant cells (Fig. 3 E) [ 4 ]. Interestingly, enrichment from the H1047R-preferred cluster regions showed enrichment for both the Notch and Wnt signaling pathways. Both of these pathways are associated with the promotion of tumor growth in breast cancers, but neither are canonically associated with increased activity of PI3Kα ( Fig. 3 F) [ 39 , 40 ]. This suggests H1047R mutant cells may drive alternative proliferative cell signaling outside of canonical PI3K signaling. These gene ontology results are consistent with the observed TF enrichment and footprinting between clusters, and provide additional context to the differential gene expression observed from RNA-seq. The differences in ChrAcc demonstrate distinct differences in genomic regulation between PIK3CA mutations and suggest the PIK3CA mutations have different effects on the function and downstream signaling of the PI3K complex.
Select CRISPR-Cas9 knockout screen identifies genes with mutation-specific essentiality
A key advantage of our isogenic cell line model is the ability to compare both mutants to the unmodified parental cell line. Therefore, a CRISPR KO screen could accurately identify essential genes specific to PIK3CA mutations, but not PIK3CA WT cells, and may provide a list of promising therapeutic targets with limited off-target effects in normal cells. Performing a whole genome CRISPR screen can be both time and resource intensive. To circumvent these limitations, we used the data generated from both the previously performed RNA-seq and ATAC-seq assays to curate a select list of genes to investigate within our CRISPR KO screen. Analysis of RNA-seq data identified 616 unique DEGs, 160 for E545K mutants and 456 for H1047R mutants, with significantly upregulated expression in a mutant cell line relative to the parental (Fig. 4 A and B ). An additional 410 genes were identified to have increased expression in both mutant cell lines compared to WT; however, gene selection was limited to those with uniquely increased expression in either the E545K or H1047R cells (Fig. 4 B). Among the 9677 combined mutant-specific genes that annotate to regions of differential chromatin accessibility, 312 were identified as DEGs (Fig. 4 C).
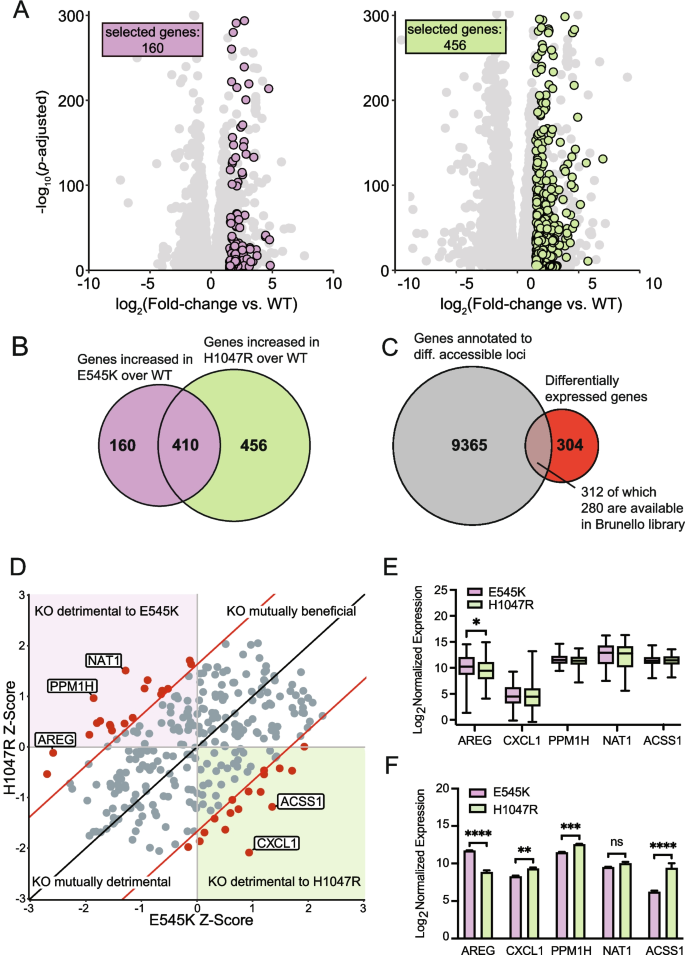
Genes with altered expression and nearby chromatin accessibility were selected for a CRISPR KO screen to identify gene targets with mutation-specific essentiality. ( A ) Scatter plots of gene expression in mutant cells relative to parental cells. Unique genes meeting the fold change and significance threshold (fold change >|1.5|, P adj < 0.05) shown in color. ( B ) Euler plot showing the overlap of genes with increased expression in cells with either mutation compared to the parental cell line. ( C ) Euler plot showing the overlap between genes annotated to regions of differential accessibility with those exhibiting increased expression in a mutant cell line compared to the parental cell line. ( D ) Scatter plot showing the results of the CRISPR KO screen. Significant hits with a Z-score difference >|1.65| are shown in red. ( E ) Box plots showing expression of the top 5 hits from the CRISPR screen in TCGA-BRCA samples. AREG shows significant difference in expression between samples bearing either of the hotspot mutations. Significance was calculated using an ANOVA with a post-hoc Fisher’s LSD test. Significance threshold is 0.05. ( F ) Bar plots showing the expression of the top 5 hits from the CRISPR screen in the MCF-10A RNA-seq samples. Significance was calculated using an ANOVA with a post-hoc Fisher’s LSD test; * = p-value 0.05–0.0332, ** = p-value .0332–0.0021, *** = p-value 0.0021–0.0002, **** = p-value < 0.0002
Among the 312 selected genes, 280 genes with targeting single guide RNAs (sgRNAs) were available from the Brunello full genome library for a select CRISPR KO screen [ 41 ] (Table S3 ). Using the MAGeCK software package, Z-score differences between each of the mutant cell lines were compared to the parental cell line for each gene [ 42 ] (Fig. 4 D). From this analysis, we identified 36 genes with a Z-score difference greater than a significance threshold of 1.65, which corresponds to a confidence interval of 95% (Table S4 ). When knocked out, these genes specifically disrupt the survival of either mutant cell line with minimal disruption to the parental line (Table S4 and Fig. 4 D). The top five genes (NAT1, PPM1H, AREG, ACSS1, CXCL1) with the greatest differences in Z-scores were evaluated in the PIK3CA mutant breast cancer samples from TCGA (Fig. 4 E). Of the top 5 genes, AREG was the only gene with significant differential expression between the PIK3CA mutations. Samples with E545K mutations demonstrated a significant increase in expression when compared to the H1047R, recapitulating the differential expression of AREG observed in our isogenic panel (Fig. 4 F). This association was independently confirmed using data from two other databases. The first of these databases was the Molecular Taxonomy of Breast Cancer International Consortium (METABRIC) database, which shows increased expression of AREG in E545K mutant samples with an increased difference within the luminal B subtype (Fig. S5 ) [ 43 ]. We also show that E545K mutant breast cancer cell lines show unique sensitivity to loss of AREG in data from project Achilles and DepMap (Fig. S6 ) [ 44 ]. This project contains a collection of CRISPR KO screen results from 1100 cancer cell lines, and this result is consistent with the findings from our CRISPR KO screen in the MCF-10A model, which shows a loss of AREG is much more deleterious to cells with the E545K mutation compared to other PIK3CA genotypes. Clinical confirmation of a unique molecular target identified from this select CRISPR screen emphasizes the translational potential of hits identified from isogenic mutant cell lines analyzed with our strategy.
Disruption of differentially accessible locus identified by ATAC-seq exhibits regulatory function over AREG expression
Selection criteria for inclusion of the ATAC-seq data in the CRISPR screen required identification of differentially accessible peaks between the two mutants. The accessible peak annotated to AREG (chr4:74,435,384–74,435,596 locus) was identified as significantly more accessible in the E545K mutant cells and exhibits many qualities of a gene regulatory region (Fig. 5 A). A previous study using CTCF ChIA-PET in MCF-10A cells published by ENCODE (ENCSR403ZYJ) showed that this locus interacts with the promoter of the AREG gene and could influence expression [ 45 , 46 ]. Furthermore, the Genotype-Tissue Expression project (GTEx) identifies 5 different AREG expression quantitative trait loci (eQTLs) single nucleotide polymorphisms (SNPs) within 1 kb of this region (Table S5 ) and these SNPs have been shown to influence the expression of AREG in multiple tissue types (Fig. 5 A). Specifically, the rs28570600 SNP (gold square, Fig. 5 A) has previously been shown to be significantly associated with breast cancer susceptibility [ 47 ].
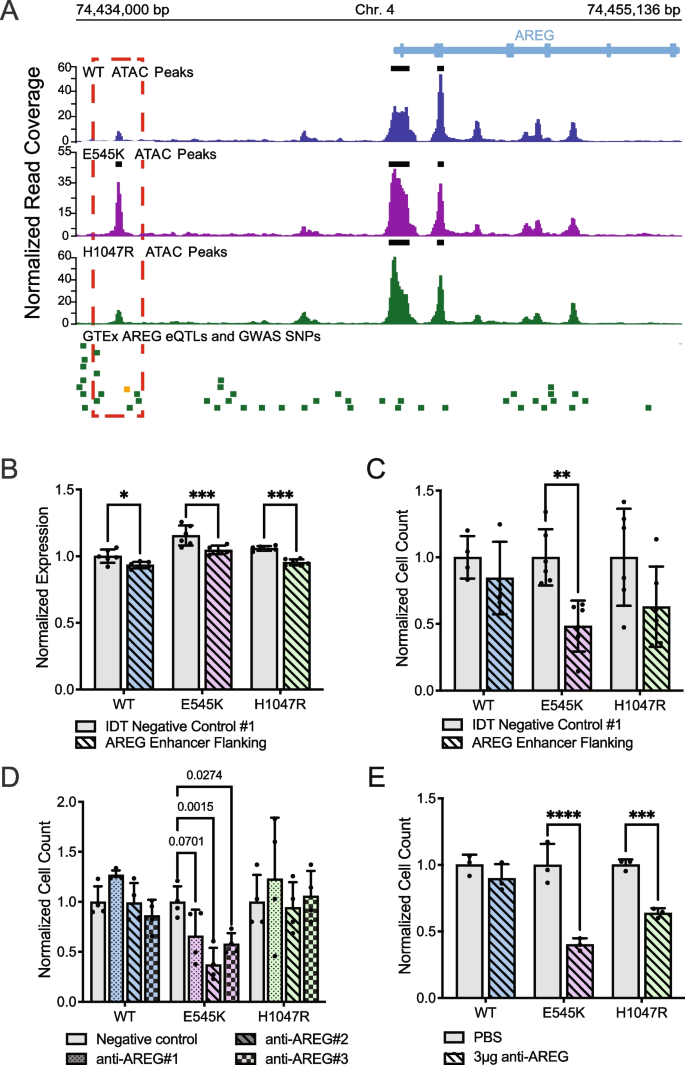
E545K mutant cells exhibit specific dependence on AREG , which is regulated by nearby accessibility peak/putative enhancer. ( A ) Genomic tracks showing the ATAC-seq data across the isogenic cell lines alongside key SNPs at the AREG gene locus. The red box highlights the differentially accessible region annotated to the AREG gene. The green bars designate GTEx AREG eQTLs. The gold bar designates a GWAS SNP associated with breast cancer. ( B ) Bar plot of AREG expression following CRISPR-mediated deletion of the putative AREG enhancer. ( C ) Bar plot of cell counts following CRISPR-mediated deletion of the putative AREG enhancer. Significance of B and C was calculated using an ANOVA with a post-hoc Šidák’s test. ( D ) Bar plot showing differences in survival/proliferation following inhibition of AREG expression using siRNA (Oligos on Table S6 , expression of AREG shown in Fig. S6 ). Significance was calculated using an ANOVA with a post-hoc Tukey’s test. ( E ) Bar plots showing differences in survival/proliferation following inhibition of AREG using a neutralizing antibody (R&D Systems, MAB262-SP). Significance was calculated using an ANOVA with a post-hoc Šidák’s test; * = p-value 0.05–0.0332, ** = p-value .0332–0.0021, *** = p-value 0.0021–0.0002, **** = p-value < 0.0002
To investigate the function of this peak, the region was deleted using CRISPR-Cas9 and a pair of sgRNAs targeting the discussed locus upstream of the AREG TSS (oligo sequences in Table S6 ). Loss of this enhancer region significantly reduced AREG expression in all cell lines in the isogenic model (Fig. 5 B). There was also an observed decline in the proliferation/survivability of the mutant cell lines, however only significant within the E545K cells. (Fig. 5 C). This experiment was also performed in a previously developed isogenic model for the E545K mutation in the MCF7 breast cancer cell line background [ 48 ]. Results from this experiment also show a specific decrease in proliferation/survivability of E545K mutant cells (Figure S7 ). These assays demonstrate the capability of our approach to identify regulatory regions that may themselves provide targets for mutation-specific treatment of PIK3CA mutant disease.
PIK3CA mutant cells exhibit specific dependency on AREG
To confirm the role of AREG expression on cell survival, short interfering RNA (siRNAs) were used to inhibit AREG in the isogenic panel (Fig. S8 ). With an siRNA knockdown, our goal was to assess the effect of a reduction of AREG expression without complete loss of expression in the system. Consistent with observations from the CRISPR KO screen, reduction of AREG expression significantly disrupted the survival and proliferation of E545K mutant cells, while exhibiting no significant changes in WT or H1047R mutant cells (Fig. 5 D). To validate this observation, cells were treated with a neutralizing antibody that disrupts the extracellular signaling of AREG. Both mutant cell lines exhibited sensitivity to AREG perturbation, while WT cells showed no significant change (Fig. 5 E). This effect is not exclusive to the E545K mutant cells but is consistent with the effect of AREG loss shown in Fig. 4 D. Knockout of the AREG gene had a small deleterious effect on H1047R cells compared to a larger effect observed in E545K cells. The extracellular nature of AREG makes it a particularly attractive target as inhibitors of AREG would not necessarily need to penetrate the cell membrane to be effective. This could simplify drug design and reduce potential off target toxicity [ 49 , 50 ]. Taken together, these results demonstrate the utility of our research strategy to identify potential molecular targets as an option for mutation-preferential therapeutic strategy. The in vitro and translational confirmation of our findings demonstrate the power of our model to accurately identify actionable gene targets and gene regulatory regions with high selectivity for mutant cells and minimal impact to WT cells.
Increased availability and advancements in multi-omics technology have begun to revolutionize translational research to better understand the interplay of molecular changes and provide new opportunities for targeted therapies. However, integration and implementation of multi-omics data for identifying new molecular targets for therapeutic development remains underutilized in the cancer setting. This study presents an analytical framework for employing an isogenic mutant panel to better understand and uniquely identify the molecular differences between mutations within the same gene. Traditionally, most cancer-associated mutations have been clinically evaluated and treated as a monolithic group with variable success. More contemporary targeted therapies such as inhibitors specific to mutant KRAS G12C, for example, highlight the success and feasibility of developing mutation specific inhibitors [ 51 ]. To improve upon this current paradigm, our workflow takes advantage of a model of isogenically incorporated mutations in a genetically stable background, integrating both RNA-seq and ATAC-seq data to design a uniquely tailored CRISPR KO screen enabling the detection of mutation-selective targets. The utilization of a mutant model that incorporates an isogenic background provides a system to identify a candidate list that demonstrates mutation-preferential gene regulatory dependencies. Previous studies have made use of this model to demonstrate how the mutant cells differ from the parental line, but our approach differs in focus in that the unique differences within the mutant cell lines are the priority [ 9 , 15 , 52 ]. Furthermore, the accessibility of CRISPR-Cas9 gene editing systems makes the development of isogenic models for cancer-associated mutations a relatively fast and straightforward process and can be scaled for a variety of mutations across tumor types. In addition to identifying mutation-preferential molecular targets, our comprehensive process paired with the isogenic panel can identify and characterize potential enhancer regions with mutation-specific activity that may offer alternative targets for treatment. These putative enhancers have affinity for distinct TF families that result in unique expression profiles and may be exploited as therapeutic vulnerabilities. The true utility of our process is in the identification of potential targets. Hits from our analyses still require additional validation to determine their effects beyond the CRISPR KO screen and ultimately their translation for potential clinical impact.
Evidence for the applicability of our workflow in breast cancer was used to analyze the two most common PIK3CA mutations in breast cancer to identify distinct molecular differences that impact downstream signaling, chromatin accessibility, and gene expression. RNA-seq and ATAC-seq analysis identified the disruption of epithelial-mesenchymal transition associated genes in E545K mutant cells and the MAPK cascade in H1047R mutant cells. These results suggest a model in which the hotspot mutations promote the activation of different biochemical pathways that in turn signal through different TF families. These TFs activate mutation-specific chromatin remodeling and expression of target genes (Fig. 6 ). Integration of these assays to create a uniquely tailored, focused CRISPR screen allowed us to identify AREG as an E545K-specific exploitable molecular difference in a highly efficient manner. This mutation-preferential dependence on AREG suggests a positive feedback loop in which increased AREG expression further promotes signaling through the E545K-mutant PI3K complex (Fig. 6 ). AREG has been previously and independently established as a signaling molecule required for the growth of PIK3CA-mutant breast cancer cells [ 53 ]. Independent identification of this molecular target utilizing our approach demonstrates its immediate biological application. Furthermore, we were able to confirm translational applicability through retrospective analyses of publicly available patient data. While our experiments were performed in a single isogenic cell line model, these clinical findings suggest the applicability of our results to actual breast cancer patients. Taken together, this study provides a framework for the independent evaluation of oncogenic hotspot mutations from a functional genomics perspective. This implies that in the era of patient-specific treatment and pharmacogenomics, our process may allow for the discovery of new targets and improved personalized medicine with the potential for increased specificity and decreased toxicity.
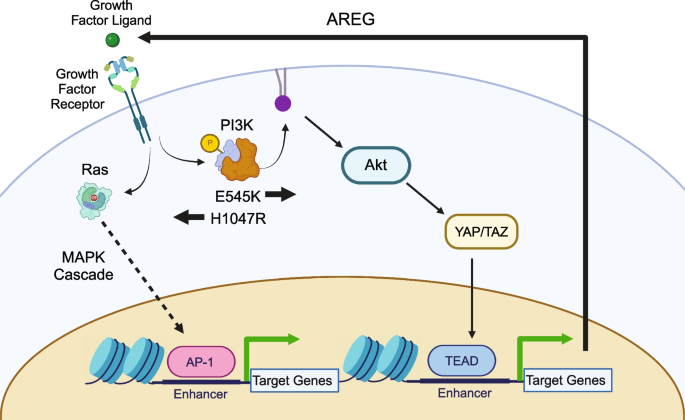
Model of Mutation-specific cell signaling. Pathway diagram depicting the effects of PIK3CA hotspot mutations on the signaling of breast cells in the MCF-10A model
This work highlights the utility of integrating multiomics data collected from an isogenic mutant model to better identify molecular targets for therapy. With the increased accessibility to genome editing technology and services, our strategy can provide investigators with a clear method for studying specific mutants in other cancer cell line models. Our workflow was able to identify AREG as an E545K-preferential molecular target, which was confirmed through in vitro assays and retrospective analyses of patient data, highlighting the potential clinical utility of our work.
Materials and methods
Cell culture.
MCF-10A parental cell lines were purchased from American Type Culture Collection (ATCC). MCF-10A cell line knock-ins were generated as previously described [ 15 ]. All cell lines were grown in 5% CO 2 at 37 °C with 1% Penicillin/Streptomycin in respective media conditions. Parental MCF-10A cell lines were cultured in DMEM/F12 (1:1) supplemented with 5% horse serum, 20 ng/ml epidermal growth factor (EGF), 10 µg/ml insulin (Roche), 0.5 µg/mL hydrocortisone (Sigma), and 100 ng/ml cholera toxin (Sigma). Knock-in cell lines were maintained in MCF-10A media in the absence of EGF. For all sequencing assays, cells were transferred to assay media 24 h prior to sample collection. Assay media contains phenol red-free DMEM/F12 (1:1) supplemented with 1% charcoal–dextran stripped FBS (Fisher), 0.2 ng/ml EGF, 10 µg/ml insulin, 0.5 µg/mL hydrocortisone, and 100 ng/ml cholera toxin.
RNA was isolated and prepared using the Qiagen RNeasy kit. Libraries were prepared by the Vanderbilt Technologies for Advanced Genomics (VANTAGE) Core using the Illumina Ribo-Zero Plus rRNA Depletion Kit. Each library was sequenced on an Illumina NovaSeq, PE150, at a requested depth of 50 million reads. All code and the specific parameters used in all data analyses can be found at: ( https://github.com/adamxmiranda/PIK3CA ). All sequencing library reads were trimmed of adapters and assessed for quality using the Trim Galore! (version 0.4.0) Wrapper of Cutadapt and FastQC[ 54 , 55 ]. Trimmed reads were mapped to the human genome assembly hg38 using the Spliced Transcripts Alignment to a Reference (STAR) aligner (version 2.5.4b) [ 56 ]. Mapped reads were sorted and filtered for a mapping quality score over 30 using the SAMtools package (version 1.5) [ 57 ]. Reads were counted to gene transcripts using featureCounts (version 2.0.0) to version 32 of the GENCODE transcripts [ 58 , 59 ]. A summary of the sequencing preprocessing can be found in Table S7 . The degree to which gene expression could be affected by the genetic modification process was also evaluated by comparing the correlation of gene expression in each of our cell lines to RNA-seq data of the TWT MCF-10A cell line from Dalton et al. 2019 [ 60 ]. This cell line underwent the same genetic modification process, but a WT clone was selected. Clustering of these samples showed the targeted WT (TWT) samples cluster between the WT and E545K cells. This suggests that although there are some differences in gene expression introduced by the modification process, that these do not completely explain the observed differences between the lines in our model (Fig. S9). This aligns with previous work from our lab that showed limited phenotypic changes in targeted WT controls using this method of genomic modification[ 61 ]. Batch correction for this analysis was performed using the limma package (version 3.50.3). Differential gene expression was identified between conditions using the DESeq2 package [ 23 ]. Pathway analysis was performed using the fgsea package on the Hallmark gene set from MsigDB [ 24 , 62 ].
Nuclei were isolated and ATAC-seq libraries were prepared using previously published methods [ 17 , 63 ]. Libraries were sequenced by the VANTAGE Core on an Illumina NovaSeq PE150, at a requested depth of 50 million reads. Reads from the ATAC-seq libraries were trimmed using the same process described in the RNA-seq section. All code and specific parameters used in all data analyses can be found at: ( https://github.com/adamxmiranda/PIK3CA ). Trimmed reads were mapped to the human genome assembly hg38 using the BBTools (version 38.69) package and Burrows-Wheeler Aligner (version 0.7.17) [ 20 , 64 ]. Quality filtering was performed on the mapped reads using SAMtools [ 57 ]. A summary of the sequencing preprocessing can be found in Table S7 . Peaks of accessibility were called using Genrich (version 0.6.1) and differential accessibility was determined using DESeq2 (version 1.34.0) [ 23 ]. Accessible regions were clustered using k-means clustering. Gene annotation and pathway enrichment was performed using GREAT (version 4.0.4) [ 33 , 34 ]. The gene annotation parameters used for GREAT were the default parameters of 5 kb upstream of the transcriptional start site (TSS), 1 kb downstream of the TSS, or up to 1000 kb in either direction for distal regions. Pathway enrichment was performed on uniquely annotated genes using the Enrichr web browser tool and the PANTHER database [ 35 , 36 , 37 , 38 ]. Motif enrichment and transcription factor (TF) footprinting were performed using HOMER (version 4.10) and TOBIAS (version 0.13.3), respectively, to identify TF potentially binding to identified accessible peak clusters [ 18 , 27 ].
CRISPR KO Screen
A modified CRISPR screen was performed with a select cohort of gene targets selected based on two criteria: 1. genes exhibited significantly increased RNA expression (log2 fold change greater than 1.5 and p-adjusted value less than 0.05) specifically in one of the PIK3CA -mutant cell lines compared to wild type, and 2. genes were annotated to regions that demonstrated significantly increased accessibility in either mutant cell line. Differential accessibility was assessed using DESeq2 and nearest neighbor annotation for these regions was performed using ChIPseeker (version 1.30.3) with the default annotation conditions of ± 3 kb from the TSS [ 23 , 65 ]. Based on this selection criteria, 312 genes were selected, for which 280 had guides available in the Brunello whole genome single guide RNA (sgRNA) library. The Brunello whole genome sgRNA library was modified for these 280 genes and prepared by the Vanderbilt Functional Genomics core in the lentiCRISPRv2 plasmid background (Full list of guides Table S3 ) [ 20 , 66 ].
MCF-10A cells were cultured in the maintenance media conditions at a density of 500,000 cells/well in a 6-well plate. 24 h after seeding, cells were infected with viral supernatant in maintenance media containing 5 μg/mL polybrene. 24 h post-infection cells were placed in selection media containing 1 μg/mL of puromycin and maintained for two weeks. Following two weeks of selection, libraries were prepared and sequenced following the protocol described in Sanjana et al. [ 66 ]. Libraries were sequenced by the VANTAGE core and analysis was performed using the maximum likelihood estimation (MLE) algorithm within the MAGeCK software package(version 0.5.9.5) [ 42 , 67 ].
Deletion of putative AREG Enhancer
Two sgRNAs were designed targeting the locus, chr4:74,435,384–74,435,596, which is annotated to the AREG gene as well as nearby AREG eQTLs identified from the GTEx Portal [ 68 , 69 , 70 ] (Table S5 ). The guide RNAs were purchased as sgRNAs from IDT with custom targeting sequences (full guide sequences in Table S6 ). Cells were plated in respective maintenance media conditions at a density of 50,000 cells/well in a 12-well plate. Transfection mixtures were prepared and added to each of the cell lines according to the guidelines described in the Lipofectamine CRISPRMAX Transfection Reagent kit (Invitrogen, CMAX00001) using the provided reagents and the Alt-R S.p. HiFi Cas9 Nuclease V3 (IDT, 1081060) in Opti-MEM Reduced Serum Media (ThermoFisher, 31985062). Separate mixtures were prepared containing either a 1:1 mixture of the designed AREG enhancer flanking guides or a control mixture of Alt-R® CRISPR-Cas9 Negative Control crRNA #1 (IDT, 1072544) duplexed to Alt-R® CRISPR-Cas9 tracrRNA (IDT, 1072532). DNA and RNA were collected from separate experimental replicates 24 h after transfection (at least 4 technical replicates were prepared for each cell line in each treatment condition). Cell counts and viability were measured 72 h following transfection using a Vi-CELL BLU cell viability analyzer (Beckman Coulter).
DNA was collected using the Wizard SV 96 Genomic DNA Purification System (Promega, A2370). PCR was performed using Phusion High-Fidelity PCR Master Mix (NEB, M0531) and the enhancer deletion validation primer set (Table S6 ). PCR mix and thermocycler conditions were set according to the Phusion Master Mix protocol provided by NEB. PCR products were measured and visualized using a D5000 ScreenTape System (Agilent, 5067) (Fig. S10).
RNA was isolated from cells using the RNeasy Plus Mini Kit (Qiagen, 74,134). RNA was converted to cDNA using the iScript cDNA Synthesis Kit (Bio-rad, 1708890). qPCR was performed using the AREG and ACTB qPCR primer sets (Table S6 ) for each sample with the SYBR Green PCR Master Mix (Applied Biosystems, 4309155). Expression of AREG was calculated relative to the expression of housekeeping gene ACTB.
Anti-AREG antibody assay
Cells were plated in their respective maintenance media conditions at a density of 50,000 cells/well in 12-well plates. 24 h following seeding, cells were treated with 1, 3, or 5 µg of AREG neutralizing antibody (R&D Systems, MAB262-SP) or an equivalent volume of PBS. This experiment was performed with three replicates for each cell line and each treatment condition. Cells were counted and viability was measured 72 h following treatment using a Vi-CELL BLU cell viability analyzer (Beckman Coulter).
siRNA assay
Cells were plated in their respective maintenance media at a density of 50,000 cells/well in a 12-well plate. 24 h following seeding, cells were treated with three different commercially validated AREG targeting siRNA (Ambion, see oligo sequences on Table S6 ), a negative control siRNA (Invitrogen, 4390843), or a null transfection condition using the Lipofectamine RNAiMAX Transfection Reagent (Invitrogen, 13778100) at a concentration of 10 pmol siRNA per well. 24 h post-transfection, RNA was prepared from cells using the Qiagen RNeasy kit. Four replicates were prepared from each cell line and each treatment condition. RNA was converted to cDNA using the iScript cDNA Synthesis Kit (Bio-rad, 1708890). qPCR was performed using the AREG and ACTB qPCR primer sets (Table S6 ) for each sample with the SYBR Green PCR Master Mix (Applied Biosystems, 4309155). Expression of AREG was calculated relative to the expression of housekeeping gene ACTB.
To assess the impact on survival and proliferation, cells were plated in their respective maintenance media at a density of 30,000 cells/well in a 24-well plate. Cells were treated with one of three different commercially validated AREG targeting siRNA (Ambion, see oligo sequences on Table S6 ) or a negative control siRNA (Invitrogen, 4390843) using the Lipofectamine RNAiMAX Transfection Reagent (Invitrogen, 13778100) at a concentration of 5 pmol siRNA per well. Cell counts and viability were measured 24 h following treatment using a Vi-CELL BLU cell viability analyzer (Beckman Coulter). This experiment was performed with four replicates in each cell line and each treatment condition.
Visualization and figure creation
Images and figures were generated using ggplot2 (version 3.4.1), plotgardener (1.4.1), deeptools (3.5.1), pheatmap (1.0.12), and graphpad Prism (Version 10) [ 71 , 72 , 73 , 74 ]. Schematic images and flow chart were created using Biorender.com.
Availability of data and materials
Raw sequencing datasets are available through the Gene Expression Omnibus (GEO) with the accession number: GSE247822. Detailed workflows and all code used in data analysis can be found at: ( https://github.com/adamxmiranda/PIK3CA ).
Abbreviations
Phosphatidylinositol-4,5-Bisphosphate 3-Kinase Catalytic Subunit Alpha
Phosphatidylinositol-4,5-Bisphosphate 3-Kinase
Assay for transposase-accessible chromatin with sequencing
Clustered Regularly Interspaced Palindromic Repeats
Amphiregulin
Differentially expressed genes
- Chromatin accessibility
Transcription factor
Expression quantitative trait locus
Waarts MR, Stonestrom AJ, Park YC, Levine RL. Targeting mutations in cancer. J Clin Invest. 2022;132(8):e154943.
Article CAS PubMed PubMed Central Google Scholar
Alaklabi S, Roy AM, Attwood K, George A, O’Connor T, Early A, Levine EG, Gandhi S. Real world outcomes with alpelisib in metastatic hormone receptor-positive breast cancer patients: A single institution experience. Front Oncol. 2022;12:1012391.
Lei JT, Gou X, Seker S, Ellis MJ. ESR1 alterations and metastasis in estrogen receptor positive breast cancer. J Cancer MetastasisTreat. 2019;5:38.
CAS Google Scholar
Yang J, Nie J, Ma X, Wei Y, Peng Y, Wei X. Targeting PI3K in cancer: mechanisms and advances in clinical trials. BioMed Central. 2019;18:1–28.
Google Scholar
Cerami E, Gao J, Dogrusoz U, Gross BE, Sumer SO, Aksoy BA, Jacobsen A, Byrne CJ, Heuer ML, Larsson E, et al. The cBio cancer genomics portal: an open platform for exploring multidimensional cancer genomics data. Cancer Discov. 2012;2(5):401–4.
Article PubMed Google Scholar
Gao J, Aksoy BA, Dogrusoz U, Dresdner G, Gross B, Sumer SO, Sun Y, Jacobsen A, Sinha R, Larsson E, et al. Integrative analysis of complex cancer genomics and clinical profiles using the cBioPortal. Sci Signal. 2013;6(269):p11.
Article Google Scholar
Martinez-Saez O, Chic N, Pascual T, Adamo B, Vidal M, Gonzalez-Farre B, Sanfeliu E, Schettini F, Conte B, Braso-Maristany F, et al. Frequency and spectrum of PIK3CA somatic mutations in breast cancer. Breast Cancer Res. 2020;22(1):45.
Garay JP, Smith R, Devlin K, Hollern DP, Liby T, Liu M, Boddapati S, Watson SS, Esch A, Zheng T, et al. Sensitivity to targeted therapy differs between HER2-amplified breast cancer cells harboring kinase and helical domain mutations in PIK3CA. Breast Cancer Res. 2021;23(1):81.
Hart JR, Zhang Y, Liao L, Ueno L, Du L, Jonkers M, Yates JR 3rd, Vogt PK. The butterfly effect in cancer: a single base mutation can remodel the cell. Proc Natl Acad Sci. 2015;112(4):1131–6.
Janku F, Wheler JJ, Naing A, Falchook GS, Hong DS, Stepanek VM, Fu S, Piha-Paul SA, Lee JJ, Luthra R, et al. PIK3CA mutation H1047R is associated with response to PI3K/AKT/mTOR signaling pathway inhibitors in early-phase clinical trials. Cancer Res. 2013;73(1):276–84.
Article CAS PubMed Google Scholar
Jacobson A. Alpelisib Plus Fulvestrant or Letrozole Demonstrates Sustained Benefits Across Subgroups of Patients with PIK3CA-Mutated HR+/HER2- Advanced Breast Cancer. Oncologist. 2022;27(Suppl 1):S13–4.
Article PubMed PubMed Central Google Scholar
Miller MS, Maheshwari S, McRobb FM, Kinzler KW, Amzel LM, Vogelstein B, Gabelli SB. Identification of allosteric binding sites for PI3Kalpha oncogenic mutant specific inhibitor design. Bioorg Med Chem. 2017;25(4):1481–6.
Andre F, Ciruelos E, Rubovszky G, Campone M, Loibl S, Rugo HS, Iwata H, Conte P, Mayer IA, Kaufman B, et al. Alpelisib for PIK3CA-Mutated, Hormone Receptor-Positive Advanced Breast Cancer. N Engl J Med. 2019;380(20):1929–40.
Andre F, Ciruelos EM, Juric D, Loibl S, Campone M, Mayer IA, Rubovszky G, Yamashita T, Kaufman B, Lu YS, et al. Alpelisib plus fulvestrant for PIK3CA-mutated, hormone receptor-positive, human epidermal growth factor receptor-2-negative advanced breast cancer: final overall survival results from SOLAR-1. Ann Oncol. 2021;32(2):208–17.
Gustin JP, Karakas B, Weiss MB, Abukhdeir AM, Lauring J, Garay JP, Cosgrove D, Tamaki A, Konishi H, Konishi Y, et al. Knockin of mutant PIK3CA activates multiple oncogenic pathways. Proc Natl Acad Sci U S A. 2009;106(8):2835–40.
Conesa A, Madrigal P, Tarazona S, Gomez-Cabrero D, Cervera A, McPherson A, Szczesniak MW, Gaffney DJ, Elo LL, Zhang X, Mortazavi A. A survey of best practices for RNA-seq data analysis. Genome Biol. 2016;17:13.
Buenrostro JD, Giresi PG, Zaba LC, Chang HY, Greenleaf WJ. Transposition of native chromatin for fast and sensitive epigenomic profiling of open chromatin, DNA-binding proteins and nucleosome position. Nat Methods. 2013;10(12):1213–8.
Bentsen M, Goymann P, Schultheis H, Klee K, Petrova A, Wiegandt R, Fust A, Preussner J, Kuenne C, Braun T, et al. ATAC-seq footprinting unravels kinetics of transcription factor binding during zygotic genome activation. Nat Commun. 2020;11(1):4267.
Galas DJ, Schmitz A. DNAse footprinting: a simple method for the detection of protein-DNA binding specificity. Nucleic Acids Res. 1978;5(9):3157–70.
Doench JG, Fusi N, Sullender M, Hegde M, Vaimberg EW, Donovan KF, Smith I, Tothova Z, Wilen C, Orchard R, et al. Optimized sgRNA design to maximize activity and minimize off-target effects of CRISPR-Cas9. Nat Biotechnol. 2016;34(2):184–91.
Hartenian E, Doench JG. Genetic screens and functional genomics using CRISPR/Cas9 technology. FEBS J. 2015;282(8):1383–93.
Boutros M, Ahringer J. The art and design of genetic screens: RNA interference. Nat Rev Genet. 2008;9(7):554–66.
Love MI, Huber W, Anders S. Moderated estimation of fold change and dispersion for RNA-seq data with DESeq2. Genome Biol. 2014;15(12):550.
Liberzon A, Birger C, Thorvaldsdottir H, Ghandi M, Mesirov JP, Tamayo P. The Molecular Signatures Database (MSigDB) hallmark gene set collection. Cell Syst. 2015;1(6):417–25.
Panigrahi A, O’Malley BW. Mechanisms of enhancer action: the known and the unknown. Genome Biol. 2021;22(1):108.
Castro-Mondragon JA, Riudavets-Puig R, Rauluseviciute I, Lemma RB, Turchi L, Blanc-Mathieu R, Lucas J, Boddie P, Khan A, Manosalva Perez N, et al. JASPAR 2022: the 9th release of the open-access database of transcription factor binding profiles. Nucleic Acids Res. 2022;50(D1):D165–73.
Heinz S, Benner C, Spann N, Bertolino E, Lin YC, Laslo P, Cheng JX, Murre C, Singh H, Glass CK. Simple combinations of lineage-determining transcription factors prime cis-regulatory elements required for macrophage and B cell identities. Mol Cell. 2010;38(4):576–89.
Borreguero-Munoz N, Fletcher GC, Aguilar-Aragon M, Elbediwy A, Vincent-Mistiaen ZI, Thompson BJ. The Hippo pathway integrates PI3K-Akt signals with mechanical and polarity cues to control tissue growth. PLoS Biol. 2019;17(10): e3000509.
Chi M, Liu J, Mei C, Shi Y, Liu N, Jiang X, Liu C, Xue N, Hong H, Xie J, et al. TEAD4 functions as a prognostic biomarker and triggers EMT via PI3K/AKT pathway in bladder cancer. J Exp Clin Cancer Res. 2022;41(1):175.
Vierbuchen T, Ling E, Cowley CJ, Couch CH, Wang X, Harmin DA, Roberts CWM, Greenberg ME. AP-1 Transcription Factors and the BAF Complex Mediate Signal-Dependent Enhancer Selection. Mol Cell. 2017;68(6):1067–82 e1012.
Shaulian E, Karin M. AP-1 in cell proliferation and survival. Oncogene. 2001;20(19):2390–400.
Whitmarsh AJ, Davis RJ. Transcription factor AP-1 regulation by mitogen-activated protein kinase signal transduction pathways. J Mol Med (Berl). 1996;74(10):589–607.
McLean CY, Bristor D, Hiller M, Clarke SL, Schaar BT, Lowe CB, Wenger AM, Bejerano G. GREAT improves functional interpretation of cis-regulatory regions. Nat Biotechnol. 2010;28(5):495–501.
Tanigawa Y, Dyer ES, Bejerano G. WhichTF is functionally important in your open chromatin data? PLoS Comput Biol. 2022;18(8): e1010378.
Chen EY, Tan CM, Kou Y, Duan Q, Wang Z, Meirelles GV, Clark NR, Ma’ayan A. Enrichr: interactive and collaborative HTML5 gene list enrichment analysis tool. BMC Bioinformatics. 2013;14:128.
Kuleshov MV, Jones MR, Rouillard AD, Fernandez NF, Duan Q, Wang Z, Koplev S, Jenkins SL, Jagodnik KM, Lachmann A, et al. Enrichr: a comprehensive gene set enrichment analysis web server 2016 update. Nucleic Acids Res. 2016;44(W1):W90–97.
Mi H, Muruganujan A, Thomas PD. PANTHER in 2013: modeling the evolution of gene function, and other gene attributes, in the context of phylogenetic trees. Nucleic Acids Res. 2013;41 (Database issue):377–86.
Thomas PD, Ebert D, Muruganujan A, Mushayahama T, Albou LP, Mi H. PANTHER: Making genome-scale phylogenetics accessible to all. Protein Sci. 2022;31(1):8–22.
Zhou B, Lin W, Long Y, Yang Y, Zhang H, Wu K, Chu Q. Notch signaling pathway: architecture, disease, and therapeutics. Signal Transduct Target Ther. 2022;7(1):95.
Zhan T, Rindtorff N, Boutros M. Wnt signaling in cancer. Oncogene. 2017;36(11):1461–73.
Sanson KR, Hanna RE, Hegde M, Donovan KF, Strand C, Sullender ME, Vaimberg EW, Goodale A, Root DE, Piccioni F, Doench JG. Optimized libraries for CRISPR-Cas9 genetic screens with multiple modalities. Nat Commun. 2018;9(1):5416.
Li W, Xu H, Xiao T, Cong L, Love MI, Zhang F, Irizarry RA, Liu JS, Brown M, Liu XS. MAGeCK enables robust identification of essential genes from genome-scale CRISPR/Cas9 knockout screens. Genome Biol. 2014;15(12):554.
Curtis C, Shah SP, Chin SF, Turashvili G, Rueda OM, Dunning MJ, Speed D, Lynch AG, Samarajiwa S, Yuan Y, et al. The genomic and transcriptomic architecture of 2,000 breast tumours reveals novel subgroups. Nature. 2012;486(7403):346–52.
Tsherniak A, Vazquez F, Montgomery PG, Weir BA, Kryukov G, Cowley GS, Gill S, Harrington WF, Pantel S, Krill-Burger JM, et al. Defining a Cancer Dependency Map. Cell. 2017;170(3):564–76 e516.
Li X, Luo OJ, Wang P, Zheng M, Wang D, Piecuch E, Zhu JJ, Tian SZ, Tang Z, Li G, Ruan Y. Long-read ChIA-PET for base-pair-resolution mapping of haplotype-specific chromatin interactions. Nat Protoc. 2017;12(5):899–915.
Tang Z, Luo OJ, Li X, Zheng M, Zhu JJ, Szalaj P, Trzaskoma P, Magalska A, Wlodarczyk J, Ruszczycki B, et al. CTCF-Mediated Human 3D Genome Architecture Reveals Chromatin Topology for Transcription. Cell. 2015;163(7):1611–27.
Zhang H, Ahearn TU, Lecarpentier J, Barnes D, Beesley J, Qi G, Jiang X, O’Mara TA, Zhao N, Bolla MK, et al. Genome-wide association study identifies 32 novel breast cancer susceptibility loci from overall and subtype-specific analyses. Nat Genet. 2020;52(6):572–81.
Beaver JA, Gustin JP, Yi KH, Rajpurohit A, Thomas M, Gilbert SF, Rosen DM, Ho Park B, Lauring J. PIK3CA and AKT1 mutations have distinct effects on sensitivity to targeted pathway inhibitors in an isogenic luminal breast cancer model system. Clin Cancer Res. 2013;19(19):5413–22.
Zhou M, Zou X, Cheng K, Zhong S, Su Y, Wu T, Tao Y, Cong L, Yan B, Jiang Y. The role of cell-penetrating peptides in potential anti-cancer therapy. Clin Transl Med. 2022;12(5): e822.
Alves AC, Ribeiro D, Nunes C, Reis S. Biophysics in cancer: The relevance of drug-membrane interaction studies. Biochim Biophys Acta. 2016;1858(9):2231–44.
Liu J, Kang R, Tang D. The KRAS-G12C inhibitor: activity and resistance. Cancer Gene Ther. 2022;29(7):875–8.
Wu X, Renuse S, Sahasrabuddhe NA, Zahari MS, Chaerkady R, Kim MS, Nirujogi RS, Mohseni M, Kumar P, Raju R, et al. Activation of diverse signalling pathways by oncogenic PIK3CA mutations. Nat Commun. 2014;5:4961.
Young CD, Zimmerman LJ, Hoshino D, Formisano L, Hanker AB, Gatza ML, Morrison MM, Moore PD, Whitwell CA, Dave B, et al. Activating PIK3CA Mutations Induce an Epidermal Growth Factor Receptor (EGFR)/Extracellular Signal-regulated Kinase (ERK) Paracrine Signaling Axis in Basal-like Breast Cancer. Mol Cell Proteomics. 2015;14(7):1959–76.
Martin M. Cutadapt removes adapter sequences from high-throughput sequencing reads. EMBnet. 2011;17:10–2.
Andrews S. FastQC: a quality control tool for high throughput sequence data. 2010.
Dobin A, Davis CA, Schlesinger F, Drenkow J, Zaleski C, Jha S, Batut P, Chaisson M, Gingeras TR. STAR: ultrafast universal RNA-seq aligner. Bioinformatics. 2013;29(1):15–21.
Danecek P, Bonfield JK, Liddle J, Marshall J, Ohan V, Pollard MO, Whitwham A, Keane T, McCarthy SA, Davies RM, Li H. Twelve years of SAMtools and BCFtools. Gigascience. 2021;10(2):1–4.
Article CAS Google Scholar
Liao Y, Smyth GK, Shi W. featureCounts: an efficient general purpose program for assigning sequence reads to genomic features. Bioinformatics. 2014;30(7):923–30.
Frankish A, Diekhans M, Ferreira AM, Johnson R, Jungreis I, Loveland J, Mudge JM, Sisu C, Wright J, Armstrong J, et al. GENCODE reference annotation for the human and mouse genomes. Nucleic Acids Res. 2019;47(D1):D766–73.
Dalton WB, Helmenstine E, Walsh N, Gondek LP, Kelkar DS, Read A, Natrajan R, Christenson ES, Roman B, Das S, et al. Hotspot SF3B1 mutations induce metabolic reprogramming and vulnerability to serine deprivation. J Clin Invest. 2019;129(11):4708–23.
Zabransky DJ, Yankaskas CL, Cochran RL, Wong HY, Croessmann S, Chu D, Kavuri SM, Red Brewer M, Rosen DM, Dalton WB, et al. HER2 missense mutations have distinct effects on oncogenic signaling and migration. Proc Natl Acad Sci U S A. 2015;112(45):E6205–6214.
Korotkevich G, Sukhov V, Budin N, Shpak B, Artyomov MN, Sergushichev A: Fast gene set enrichment analysis. BioRxiv. 2021
Barnett KR, Decato BE, Scott TJ, Hansen TJ, Chen B, Attalla J, Smith AD, Hodges E. ATAC-Me Captures Prolonged DNA Methylation of Dynamic Chromatin Accessibility Loci during Cell Fate Transitions. Mol Cell. 2020;77(6):1350–64 e1356.
Bushnell B, Rood J, Singer E. BBMerge - Accurate paired shotgun read merging via overlap. PLoS ONE. 2017;12(10): e0185056.
Wang Q, Li M, Wu T, Zhan L, Li L, Chen M, Xie W, Xie Z, Hu E, Xu S, Yu G. Exploring Epigenomic Datasets by ChIPseeker. Curr Protoc. 2022;2(10): e585.
Sanjana NE, Shalem O, Zhang F. Improved vectors and genome-wide libraries for CRISPR screening. Nat Methods. 2014;11(8):783–4.
Li W, Koster J, Xu H, Chen CH, Xiao T, Liu JS, Brown M, Liu XS. Quality control, modeling, and visualization of CRISPR screens with MAGeCK-VISPR. Genome Biol. 2015;16:281.
Carithers LJ, Ardlie K, Barcus M, Branton PA, Britton A, Buia SA, Compton CC, DeLuca DS, Peter-Demchok J, Gelfand ET, et al. A Novel Approach to High-Quality Postmortem Tissue Procurement: The GTEx Project. Biopreserv Biobank. 2015;13(5):311–9.
Consortium G. The Genotype-Tissue Expression (GTEx) project. Nat Genet. 2013;45(6):580–5.
Consortium G. The GTEx Consortium atlas of genetic regulatory effects across human tissues. Science. 2020;369(6509):1318–30.
Wickham H. ggplot2: Elegant Graphics for Data Analysis. Verlag New York: Springer; 2016.
Book Google Scholar
Kramer NE, Davis ES, Wenger CD, Deoudes EM, Parker SM, Love MI, Phanstiel DH. Plotgardener: cultivating precise multi-panel figures in R. Bioinformatics. 2022;38(7):2042–5.
Ramirez F, Ryan DP, Gruning B, Bhardwaj V, Kilpert F, Richter AS, Heyne S, Dundar F, Manke T. deepTools2: a next generation web server for deep-sequencing data analysis. Nucleic Acids Res. 2016;44(W1):W160–165.
Kolde R. Pheatmap: pretty heatmaps. R package version. 2012;1(2):726.
Download references
Acknowledgements
The results shown here are in part based upon data generated by the TCGA Research Network: https://www.cancer.gov/tcga . Schematic images created with biorender.com.
The Genotype-Tissue Expression (GTEx) Project was supported by the Common Fund of the Office of the Director of the National Institutes of Health, and by NCI, NHGRI, NHLBI, NIDA, NIMH, and NINDS. The data used for the analyses described in this manuscript were obtained from: the GTEx Portal on 11/02/2022.
We are grateful for support of the project and the time invested in producing this manuscript by NIH awards [1R01GM147078-01 to E.H], Department of Defense Idea Award [W81XWH-20–1-0522 to E.H], American Cancer Society (ACS) Institutional Research Grant (#IRG-15–169-56), the Vanderbilt Stanley Cohen Innovation Fund and funds from the Vanderbilt Ingram Cancer Center. We would also like to acknowledge support from the Breast Cancer Research Foundation, the Susan G. Komen Foundation, and the NIH CA214494, CA194024 (B.H.P.). We would also like to thank and acknowledge the support of The Canney Foundation, the Sage Patient Advocates, the Marcie and Ellen Foundation, The Eddie and Sandy Garcia Foundation, the support of Amy and Barry Baker, the support of John and Donna Hall, and the Vanderbilt-Ingram Cancer Center support grant (NIH CA068485) and Breast Cancer SPORE (NIH CA098131).
Author information
Authors and affiliations.
Department of Medicine, Vanderbilt University Medical Center, Nashville, TN, USA
Adam X. Miranda, Justin Kemp, Brad A. Davidson, Alexandra Manoni, Sarah Croessmann & Ben H. Park
Department of Biochemistry, Vanderbilt University School of Medicine, Nashville, TN, USA
Verda E. Miranda & Emily Hodges
Candiolo Cancer Institute, FPO-IRCCS, Candiolo, TO, Italy
Sara Erika Bellomo & Caterina Marchiò
Department of Medical Sciences, University of Turin, Turin, Italy
Caterina Marchiò
Vanderbilt-Ingram Cancer Center, Nashville, TN, USA
Ben H. Park & Emily Hodges
Vanderbilt Genetics Institute, Vanderbilt University School of Medicine, Nashville, TN, USA
Emily Hodges
You can also search for this author in PubMed Google Scholar
Contributions
AXM, BHP and EH conceived of the project. AXM cultured the cells and performed the data analysis for the RNA-seq, ATAC-seq and CRISPR KO screen experiments. AXM performed the enhancer deletion and siRNA experiments. JK performed the anti-AREG antibody experiments. BD and AM assisted with cell culture. BD and VA contributed to experimental design. SEB and CM provided data and analysis of METABRIC RNA-seq samples. AXM, SC, BHP and EH wrote and edited the manuscript and figures. All authors reviewed and approved the final manuscript.
Corresponding authors
Correspondence to Ben H. Park or Emily Hodges .
Ethics declarations
Ethics approval and consent to participate.
Not applicable.
Consent for publication
Competing interests.
C.M. reports personal consultancy fees from Bayer, Roche, AstraZeneca, Novartis, outside the scope of the present work. B.H.P. is a paid consultant for Guardant Health, AstraZeneca, Caris and is a paid scientific advisory board member for Celcuity Inc. B.H.P. is an unpaid consultant for Tempus Inc. B.H.P. also receives research funding from Eli Lilly and Guardant Health. Under separate licensing agreements between Horizon Discovery, LTD and The Johns Hopkins University, B.H.P. and S.E.C. are entitled to a share of royalties received by the University on sales of products. The terms of this arrangement are being managed by the Johns Hopkins University in accordance with its conflict-of-interest policies. All other authors declare no competing interests.
Additional information
Publisher’s note.
Springer Nature remains neutral with regard to jurisdictional claims in published maps and institutional affiliations.
Supplementary Information
Supplementary material 1., supplementary material 2., supplementary material 3., supplementary material 4., supplementary material 5., supplementary material 6., supplementary material 7., supplementary material 8., rights and permissions.
Open Access This article is licensed under a Creative Commons Attribution 4.0 International License, which permits use, sharing, adaptation, distribution and reproduction in any medium or format, as long as you give appropriate credit to the original author(s) and the source, provide a link to the Creative Commons licence, and indicate if changes were made. The images or other third party material in this article are included in the article's Creative Commons licence, unless indicated otherwise in a credit line to the material. If material is not included in the article's Creative Commons licence and your intended use is not permitted by statutory regulation or exceeds the permitted use, you will need to obtain permission directly from the copyright holder. To view a copy of this licence, visit http://creativecommons.org/licenses/by/4.0/ . The Creative Commons Public Domain Dedication waiver ( http://creativecommons.org/publicdomain/zero/1.0/ ) applies to the data made available in this article, unless otherwise stated in a credit line to the data.
Reprints and permissions
About this article
Cite this article.
Miranda, A.X., Kemp, J., Davidson, B.A. et al. Genomic dissection and mutation-specific target discovery for breast cancer PIK3CA hotspot mutations. BMC Genomics 25 , 519 (2024). https://doi.org/10.1186/s12864-024-10368-1
Download citation
Received : 21 December 2023
Accepted : 02 May 2024
Published : 27 May 2024
DOI : https://doi.org/10.1186/s12864-024-10368-1
Share this article
Anyone you share the following link with will be able to read this content:
Sorry, a shareable link is not currently available for this article.
Provided by the Springer Nature SharedIt content-sharing initiative
- Integrative analysis
- Isogenic cell lines
- Breast cancer
- Epigenomics
- Gene expression
BMC Genomics
ISSN: 1471-2164
- Submission enquiries: [email protected]
- General enquiries: [email protected]
Thank you for visiting nature.com. You are using a browser version with limited support for CSS. To obtain the best experience, we recommend you use a more up to date browser (or turn off compatibility mode in Internet Explorer). In the meantime, to ensure continued support, we are displaying the site without styles and JavaScript.
- View all journals
- My Account Login
- Explore content
- About the journal
- Publish with us
- Sign up for alerts
- Open access
- Published: 23 September 2012
Comprehensive molecular portraits of human breast tumours
The cancer genome atlas network.
Nature volume 490 , pages 61–70 ( 2012 ) Cite this article
331k Accesses
8459 Citations
321 Altmetric
Metrics details
- Breast cancer
- Cancer genetics
- Molecular biology
This article has been updated
We analysed primary breast cancers by genomic DNA copy number arrays, DNA methylation, exome sequencing, messenger RNA arrays, microRNA sequencing and reverse-phase protein arrays. Our ability to integrate information across platforms provided key insights into previously defined gene expression subtypes and demonstrated the existence of four main breast cancer classes when combining data from five platforms, each of which shows significant molecular heterogeneity. Somatic mutations in only three genes ( TP53 , PIK3CA and GATA3 ) occurred at >10% incidence across all breast cancers; however, there were numerous subtype-associated and novel gene mutations including the enrichment of specific mutations in GATA3 , PIK3CA and MAP3K1 with the luminal A subtype. We identified two novel protein-expression-defined subgroups, possibly produced by stromal/microenvironmental elements, and integrated analyses identified specific signalling pathways dominant in each molecular subtype including a HER2/phosphorylated HER2/EGFR/phosphorylated EGFR signature within the HER2-enriched expression subtype. Comparison of basal-like breast tumours with high-grade serous ovarian tumours showed many molecular commonalities, indicating a related aetiology and similar therapeutic opportunities. The biological finding of the four main breast cancer subtypes caused by different subsets of genetic and epigenetic abnormalities raises the hypothesis that much of the clinically observable plasticity and heterogeneity occurs within, and not across, these major biological subtypes of breast cancer.
Similar content being viewed by others
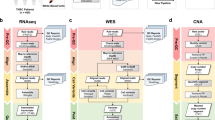
A comprehensive genomic and transcriptomic dataset of triple-negative breast cancers
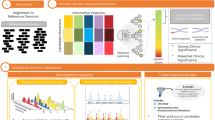
Automated next-generation profiling of genomic alterations in human cancers
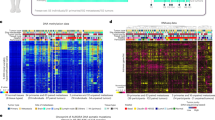
Multiomics in primary and metastatic breast tumors from the AURORA US network finds microenvironment and epigenetic drivers of metastasis
Breast cancer is one of the most common cancers with greater than 1,300,000 cases and 450,000 deaths each year worldwide. Clinically, this heterogeneous disease is categorized into three basic therapeutic groups. The oestrogen receptor (ER) positive group is the most numerous and diverse, with several genomic tests to assist in predicting outcomes for ER + patients receiving endocrine therapy 1 , 2 . The HER2 (also called ERBB2 ) amplified group 3 is a great clinical success because of effective therapeutic targeting of HER2 , which has led to intense efforts to characterize other DNA copy number aberrations 4 , 5 . Triple-negative breast cancers (TNBCs, lacking expression of ER, progesterone receptor (PR) and HER2 ), also known as basal-like breast cancers 6 , are a group with only chemotherapy options, and have an increased incidence in patients with germline BRCA1 mutations 7 , 8 or of African ancestry 9 .
Most molecular studies of breast cancer have focused on just one or two high information content platforms, most frequently mRNA expression profiling or DNA copy number analysis, and more recently massively parallel sequencing 10 , 11 , 12 . Supervised clustering of mRNA expression data has reproducibly established that breast cancers encompass several distinct disease entities, often referred to as the intrinsic subtypes of breast cancer 13 , 14 . The recent development of additional high information content assays focused on abnormalities in DNA methylation, microRNA (miRNA) expression and protein expression, provide further opportunities to characterize more completely the molecular architecture of breast cancer. In this study, a diverse set of breast tumours were assayed using six different technology platforms. Individual platform and integrated pathway analyses identified many subtype-specific mutations and copy number changes that identify therapeutically tractable genomic aberrations and other events driving tumour biology.
Samples and clinical data
Tumour and germline DNA samples were obtained from 825 patients. Different subsets of patients were assayed on each platform: 466 tumours from 463 patients had data available on five platforms including Agilent mRNA expression microarrays ( n = 547), Illumina Infinium DNA methylation chips ( n = 802), Affymetrix 6.0 single nucleotide polymorphism (SNP) arrays ( n = 773), miRNA sequencing ( n = 697), and whole-exome sequencing ( n = 507); in addition, 348 of the 466 samples also had reverse-phase protein array (RPPA) data ( n = 403). Owing to the short median overall follow up (17 months) and the small number of overall survival events (93 out of 818), survival analyses will be presented in a later publication. Demographic and clinical characteristics are presented in Supplementary Table 1 .
Significantly mutated genes in breast cancer
Overall, 510 tumours from 507 patients were subjected to whole-exome sequencing, identifying 30,626 somatic mutations comprised of 28,319 point mutations, 4 dinucleotide mutations, and 2,302 insertions/deletions (indels) (ranging from 1 to 53 nucleotides). The point mutations included 6,486 silent, 19,045 missense, 1,437 nonsense, 26 read-through, 506 splice-site mutations, and 819 mutations in RNA genes. Comparison to COSMIC and OMIM databases identified 619 mutations across 177 previously reported cancer genes. Of 19,045 missense mutations, 9,484 were predicted to have a high probability of being deleterious by Condel 15 . The MuSiC package 16 , which determines the significance of the observed mutation rate of each gene based on the background mutation rate, identified 35 significantly mutated genes (excluding LOC or Ensembl gene IDs) by at least two tests (convolution and likelihood ratio tests) with false discovery rate (FDR) <5% ( Supplementary Table 2 ).
In addition to identifying nearly all genes previously implicated in breast cancer ( PIK3CA , PTEN , AKT1 , TP53 , GATA3 , CDH1 , RB1 , MLL3 , MAP3K1 and CDKN1B ), a number of novel significantly mutated genes were identified including TBX3 , RUNX1 , CBFB , AFF2 , PIK3R1 , PTPN22 , PTPRD , NF1 , SF3B1 and CCND3 . TBX3 , which is mutated in ulnar-mammary syndrome and involved in mammary gland development 17 , harboured 13 mutations (8 frame-shift indels, 1 in-frame deletion, 1 nonsense, and 3 missense), suggesting a loss of function. Additionally, 2 mutations were found in TBX4 and 1 mutation in TBX5 , which are genes involved in Holt–Oram syndrome 18 . Two other transcription factors, CTCF and FOXA1 , were at or near significance harbouring 13 and 8 mutations, respectively. RUNX1 and CBFB , both rearranged in acute myeloid leukaemia and interfering with haematopoietic differentiation, harboured 19 and 9 mutations, respectively. PIK3R1 contained 14 mutations, most of which clustered in the PIK3CA interaction domain similar to previously identified mutations in glioma 19 and endometrial cancer 20 . We also observed a statistically significant exclusion pattern among PIK3R1 , PIK3CA , PTEN and AKT1 mutations ( P = 0.025). Mutation of splicing factor SF3B1 , previously described in myelodysplastic syndromes 21 and chronic lymphocytic leukaemia 22 , was significant with 15 non-silent mutations, of which 4 were a recurrent K700E substitution. Two protein tyrosine phosphatases ( PTPN22 and PTPRD ) were also significantly mutated; frequent deletion/mutation of PTPRD is observed in lung adenocarcinoma 23 .
Mutations and mRNA-expression subtype associations
We analysed the somatic mutation spectrum within the context of the four mRNA-expression subtypes, excluding the normal-like group owing to small numbers ( n = 8) ( Fig. 1 ). Several significantly mutated genes showed mRNA-subtype-specific ( Supplementary Figs 1–3 ) and clinical-subtype-specific patterns of mutation ( Supplementary Table 2 ). Significantly mutated genes were considerably more diverse and recurrent within luminal A and luminal B tumours than within basal-like and HER2-enriched (HER2E) subtypes; however, the overall mutation rate was lowest in luminal A subtype and highest in the basal-like and HER2E subtypes. The luminal A subtype harboured the most significantly mutated genes, with the most frequent being PIK3CA (45%), followed by MAP3K1 , GATA3 , TP53 , CDH1 and MAP2K4. Twelve per cent of luminal A tumours contained likely inactivating mutations in MAP3K1 and MAP2K4 , which represent two contiguous steps in the p38–JNK1 stress kinase pathway 24 . Luminal B cancers exhibited a diversity of significantly mutated genes, with TP53 and PIK3CA (29% each) being the most frequent. The luminal tumour subtypes markedly contrasted with basal-like cancers where TP53 mutations occurred in 80% of cases and the majority of the luminal significantly mutated gene repertoire, except PIK3CA (9%), were absent or near absent. The HER2E subtype, which has frequent HER2 amplification (80%), had a hybrid pattern with a high frequency of TP53 (72%) and PIK3CA (39%) mutations and a much lower frequency of other significantly mutated genes including PIK3R1 (4%).
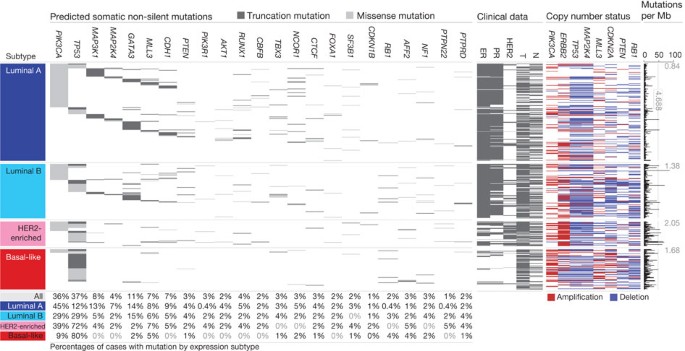
Tumour samples are grouped by mRNA subtype: luminal A ( n = 225), luminal B ( n = 126), HER2E ( n = 57) and basal-like ( n = 93). The left panel shows non-silent somatic mutation patterns and frequencies for significantly mutated genes. The middle panel shows clinical features: dark grey, positive or T2–4; white, negative or T1; light grey, N/A or equivocal. N, node status; T, tumour size. The right panel shows significantly mutated genes with frequent copy number amplifications (red) or deletions (blue). The far-right panel shows non-silent mutation rate per tumour (mutations per megabase, adjusted for coverage). The average mutation rate for each expression subtype is indicated. Hypermutated: mutation rates >3 s.d. above the mean (>4.688, indicated by grey line).
PowerPoint slide
Intrinsic mRNA subtypes differed not only by mutation frequencies but also by mutation type. Most notably, TP53 mutations in basal-like tumours were mostly nonsense and frame shift, whereas missense mutations predominated in luminal A and B tumours ( Supplementary Fig. 1 ). Fifty-eight somatic GATA3 mutations, some of which were previously described 25 , were detected including a hotspot 2-base-pair deletion within intron 4 only in the luminal A subtype (13 out of 13 mutants) ( Supplementary Fig. 2 ). In contrast, 7 out of 9 frame-shift mutations in exon 5 (DNA binding domain) occurred in luminal B cancers. PIK3CA mutation frequency and spectrum also varied by mRNA subtype ( Supplementary Fig. 3 ); the recurrent PIK3CA E545K mutation was present almost exclusively within luminal A (25 out of 27) tumours. CDH1 mutations were common (30 out of 36) within the lobular histological subtype and corresponded with lower CDH1 mRNA ( Supplementary Fig. 4 ) and protein expression. Finally, we identified 4 out of 8 somatic variants in HER2 within lobular cancers, three of which were within the tyrosine kinase domain.
We performed analyses on a selected set of genes 26 using the normal tissue DNA data and detected a number of germline predisposing variants. These analyses identified 47 out of 507 patients with deleterious germline variants, representing nine different genes ( ATM , BRCA1 , BRCA2 , BRIP1 , CHEK2 , NBN , PTEN , RAD51C and TP53; Supplementary Table 3 ), supporting the hypothesis that ∼ 10% of sporadic breast cancers may have a strong germline contribution. These data confirmed the association between the presence of germline BRCA1 mutations and basal-like breast cancers 7 , 8 .
Gene expression analyses (mRNA and miRNA)
Several approaches were used to look for structure in the mRNA expression data. We performed an unsupervised hierarchical clustering analysis of 525 tumours and 22 tumour-adjacent normal tissues using the top 3,662 variably expressed genes ( Supplementary Fig. 5 ); SigClust analysis identified 12 classes (5 classes with >9 samples per class). We performed a semi-supervised hierarchical cluster analysis using a previously published ‘intrinsic gene list’ 14 , which identified 13 classes (9 classes with >9 samples per class) ( Supplementary Fig. 6 ). We also classified each sample using the 50-gene PAM50 model 14 ( Supplementary Fig. 5 ). High concordance was observed between all three analyses; therefore, we used the PAM50-defined subtype predictor as a common classification metric. There were only eight normal-like and eight claudin-low tumours 27 , thus we did not perform focussed analyses on these two subtypes.
MicroRNA expression levels were assayed via Illumina sequencing, using 1,222 miRBase 28 v16 mature and star strands as the reference database of miRNA transcripts/genes. Seven subtypes were identified by consensus non-negative matrix factorization (NMF) clustering using an abundance matrix containing the 25% most variable miRNAs (306 transcripts/genes or MIMATs (miRNA IDs)). These subtypes correlated with mRNA subtypes, ER, PR and HER2 clinical status ( Supplementary Fig. 7 ). Of note, miRNA groups 4 and 5 showed high overlap with the basal-like mRNA subtype and contained many TP53 mutations. The remaining miRNA groups (1–3, 6 and 7) were composed of a mixture of luminal A, luminal B and HER2E with little correlation with the PAM50 defined subtypes. With the exception of TP53 —which showed a strong positive correlation—and PIK3CA and GATA3 —which showed negative associations with groups 4 and 5, respectively—there was little correlation with mutation status and miRNA subtype.
DNA methylation
Illumina Infinium DNA methylation arrays were used to assay 802 breast tumours. Data from HumanMethylation27 (HM27) and HumanMethylation450 (HM450) arrays were combined and filtered to yield a common set of 574 probes used in an unsupervised clustering analysis, which identified five distinct DNA methylation groups ( Supplementary Fig. 8 ). Group 3 showed a hypermethylated phenotype and was significantly enriched for luminal B mRNA subtype and under-represented for PIK3CA, MAP3K1 and MAP2K4 mutations. Group 5 showed the lowest levels of DNA methylation, overlapped with the basal-like mRNA subtype, and showed a high frequency of TP53 mutations. HER2-positive (HER2 + ) clinical status, or the HER2E mRNA subtype, had only a modest association with the methylation subtypes.
A supervised analysis of the DNA methylation and mRNA expression data was performed to compare DNA methylation group 3 ( N = 49) versus all tumours in groups 1, 2 and 4 (excluding group 5, which consisted predominantly of basal-like tumours). This analysis identified 4,283 genes differentially methylated (3,735 higher in group 3 tumours) and 1,899 genes differentially expressed (1,232 downregulated); 490 genes were both methylated and showed lower expression in group 3 tumours ( Supplementary Table 4 ). A DAVID (database for annotation, visualization and integrated discovery) functional annotation analysis identified ‘extracellular region part’ and ‘Wnt signalling pathway’ to be associated with this 490-gene set; the group 3 hypermethylated samples showed fewer PIK3CA and MAP3K1 mutations, and lower expression of Wnt-pathway genes.
DNA copy number
A total of 773 breast tumours were assayed using Affymetrix 6.0 SNP arrays. Segmentation analysis and GISTIC were used to identify focal amplifications/deletions and arm-level gains and losses ( Supplementary Table 5 ). These analyses confirmed all previously reported copy number variations and highlighted a number of significantly mutated genes including focal amplification of regions containing PIK3CA , EGFR , FOXA1 and HER2 , as well as focal deletions of regions containing MLL3 , PTEN , RB1 and MAP2K4 ( Supplementary Fig. 9 ); in all cases, multiple genes were included within each altered region. Importantly, many of these copy number changes correlated with mRNA subtype including characteristic loss of 5q and gain of 10p in basal-like cancers 5 , 29 and gain of 1q and/or 16q loss in luminal tumours 4 . NMF clustering of GISTIC segments identified five copy number clusters/groups that correlated with mRNA subtypes, ER, PR and HER2 clinical status, and TP53 mutation status ( Supplementary Fig. 10 ). In addition, this aCGH subtype classification was highly correlated with the aCGH subtypes recently defined by ref. 30 ( Supplementary Fig. 11 ).
Reverse phase protein arrays
Quantified expression of 171 cancer-related proteins and phospho-proteins by RPPA was performed on 403 breast tumours 31 . Unsupervised hierarchical clustering analyses identified seven subtypes; one class contained too few cases for further analysis ( Supplementary Fig. 12 ). These protein subtypes were highly concordant with the mRNA subtypes, particularly with basal-like and HER2E mRNA subtypes. Closer examination of the HER2-containing RPPA-defined subgroup showed coordinated overexpression of HER2 and EGFR with a strong concordance with phosphorylated HER2 (pY1248) and EGFR (pY992), probably from heterodimerization and cross-phosphorylation. Although there is a potential for modest cross reactivity of antibodies against these related total and phospho-proteins, the concordance of phosphorylation of HER2 and EGFR was confirmed using multiple independent antibodies.
In RPPA-defined luminal tumours, there was high protein expression of ER, PR, AR, BCL2, GATA3 and INPP4B, defining mostly luminal A cancers and a second more heterogeneous protein subgroup composed of both luminal A and luminal B cancers. Two potentially novel protein-defined subgroups were identified: reactive I consisted primarily of a subset of luminal A tumours, whereas reactive II consisted of a mixture of mRNA subtypes. These groups are termed ‘reactive’ because many of the characteristic proteins are probably produced by the microenvironment and/or cancer-activated fibroblasts including fibronectin, caveolin 1 and collagen VI. These two RPPA groups did not have a marked difference in the percentage tumour cell content when compared to each other, or the other protein subtypes, as assessed by SNP array analysis or pathological examination. In addition, supervised analyses of reactive I versus II groups using miRNA expression, DNA methylation, mutation, or DNA copy number data identified no significant differences between these groups, whereas similar supervised analyses using protein and mRNA expression identified many differences.
Multiplatform subtype discovery
To reveal higher-order structure in breast tumours based on multiple data types, significant clusters/subtypes from each of five platforms were analysed using a multiplatform data matrix subjected to unsupervised consensus clustering ( Fig. 2 ). This ‘cluster of clusters’ (C-of-C) approach illustrated that basal-like cancers had the most distinct multiplatform signature as all the different platforms for the basal-like groups clustered together. To a great extent, the four major C-of-C subdivisions correlated well with the previously published mRNA subtypes (driven, in part, by the fact that the four intrinsic subtypes were one of the inputs). Therefore, we also performed C-of-C analysis with no mRNA data present ( Supplementary Fig. 13 ) or with the 12 unsupervised mRNA subtypes ( Supplementary Fig. 14 ), and in each case 4–6 groups were identified. Recent work identified ten copy-number-based subgroups in a 997 breast cancer set 30 . We evaluated this classification in a C-of-C analysis instead of our five-class copy number subtypes, with either the PAM50 ( Supplementary Fig. 15 ) or 12 unsupervised mRNA subtypes ( Supplementary Fig. 16 ); each of these C-of-C classifications was highly correlated with PAM50 mRNA subtypes and with the other C-of-C analyses ( Fig. 2 ). The transcriptional profiling and RPPA platforms demonstrated a high correlation with the consensus structure, indicating that the information content from copy number aberrations, miRNAs and methylation is captured at the level of gene expression and protein function.
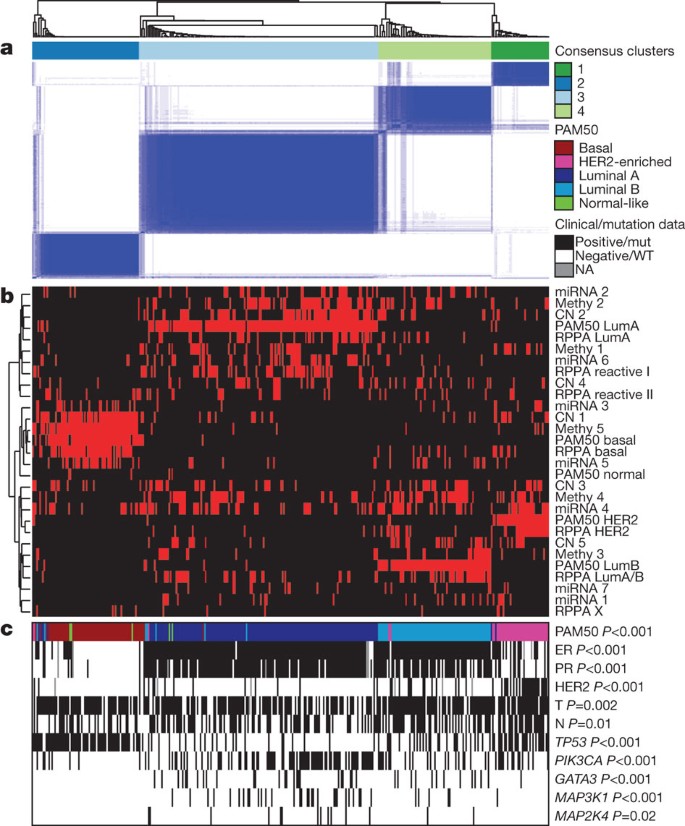
a , Consensus clustering analysis of the subtypes identifies four major groups (samples, n = 348). The blue and white heat map displays sample consensus. b , Heat-map display of the subtypes defined independently by miRNAs, DNA methylation, copy number (CN), PAM50 mRNA expression, and RPPA expression. The red bar indicates membership of a cluster type. c , Associations with molecular and clinical features. P values were calculated using a chi-squared test.
Luminal/ER + summary analysis
Luminal/ER + breast cancers are the most heterogeneous in terms of gene expression ( Supplementary Fig. 5 ), mutation spectrum ( Fig. 1 ), copy number changes ( Supplementary Fig. 9 ) and patient outcomes 1 , 14 . One of the most dominant features is high mRNA and protein expression of the luminal expression signature ( Supplementary Fig. 5 ), which contains ESR1 , GATA3 , FOXA1 , XBP1 and MYB ; the luminal/ER + cluster also contained the largest number of significantly mutated genes. Most notably, GATA3 and FOXA1 were mutated in a mutually exclusive fashion, whereas ESR1 and XBP1 were typically highly expressed but infrequently mutated. Mutations in RUNX1 and its dimerization partner CBFB may also have a role in aberrant ER signalling in luminal tumours, as RUNX1 functions as an ER ‘DNA tethering factor’ 32 . PARADIGM 33 analysis comparing luminal versus basal-like cancers further emphasized the presence of a hyperactivated FOXA1–ER complex as a critical network hub differentiating these two tumour subtypes ( Supplementary Fig. 17 ).
A confirmatory finding here was the high mutation frequency of PIK3CA in luminal/ER + breast cancers 34 , 35 . Through multiple technology platforms, we examined possible relationships between PIK3CA mutation, PTEN loss, INPP4B loss and multiple gene and protein expression signatures of pathway activity. RPPA data demonstrated that pAKT, pS6 and p4EBP1, typical markers of phosphatidylinositol-3-OH kinase (PI(3)K) pathway activation, were not elevated in PIK3CA -mutated luminal A cancers; instead, they were highly expressed in basal-like and HER2E mRNA subtypes (the latter having frequent PIK3CA mutations) and correlated strongly with INPP4B and PTEN loss, and to a degree with PIK3CA amplification. Similarly, protein 36 and three mRNA signatures 37 , 38 , 39 of PI(3)K pathway activation were enriched in basal-like over luminal A cancers ( Fig. 3a ). This apparent disconnect between the presence of PIK3CA mutations and biomarkers of pathway activation has been previously noted 36 .
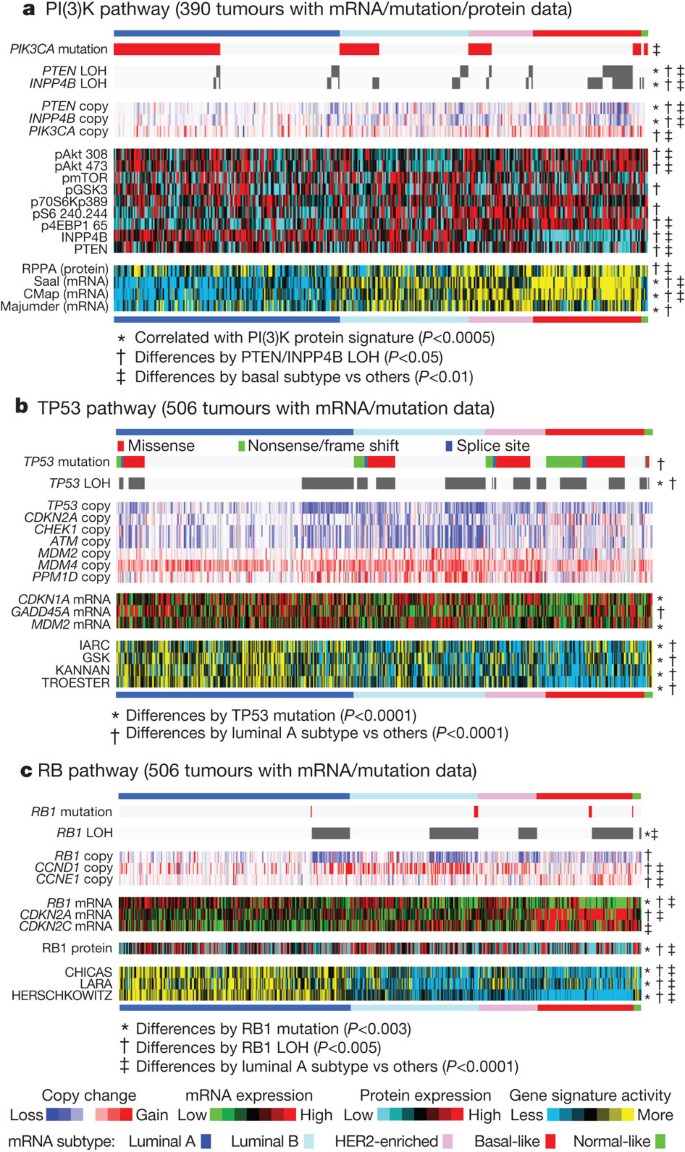
Breast cancer subtypes differ by genetic and genomic targeting events, with corresponding effects on pathway activity. a – c , For PI(3)K ( a ), TP53 ( b ) and RB1 ( c ) pathways, key genes were selected using prior biological knowledge. Multiple mRNA expression signatures for a given pathway were defined (details in Supplementary Methods ; PI(3)K:Saal, PTEN loss in human breast tumours; CMap, PI(3)K/mTOR inhibitor treatment in vitro ; Majumder, Akt overexpression in mouse model; TP53: IARC, expert-curated p53 targets; GSK, TP53 mutant versus wild-type cell lines; KANNAN, TP53 overexpression in vitro ; TROESTER, TP53 knockdown in vitro ; RB: CHICAS, RB1 mouse knockout versus wild type; LARA, RB1 knockdown in vitro ; HERSCHKOWITZ, RB1 loss of heterozygosity (LOH) in human breast tumours) and applied to the gene expression data, in order to score each tumour for relative signature activity (yellow, more active). The PI(3)K panel includes a protein-based (RPPA) proteomic signature. Tumours were ordered first by mRNA subtype, although specific ordering differs between the panels. P values were calculated by a Pearson’s correlation or a Chi-squared test.
Another striking luminal/ER + subtype finding was the frequent mutation of MAP3K1 and MAP2K4 , which represent two contiguous steps within the p38–JNK1 pathway 24 , 40 . These mutations are predicted to be inactivating, with MAP2K4 also a target of focal DNA loss in luminal tumours ( Supplementary Fig. 9 ). To explore the possible interplay between PIK3CA , MAP3K and MAP2K4 signalling, MEMo analysis 41 was performed to identify mutually exclusive alterations targeting frequently altered genes likely to belong to the same pathway ( Fig. 4 ). Across all breast cancers, MEMo identified a set of modules that highlight the differential activation events within the receptor tyrosine kinase (RTK)–PI(3)K pathway ( Fig. 4a ); mutations of PIK3CA were very common in luminal/ER + cancers whereas PTEN loss was more common in basal-like tumours. Almost all MAP3K1 and MAP2K4 mutations were in luminal tumours, yet MAP3K1 and MAP2K4 appeared almost mutually exclusive relative to one another.
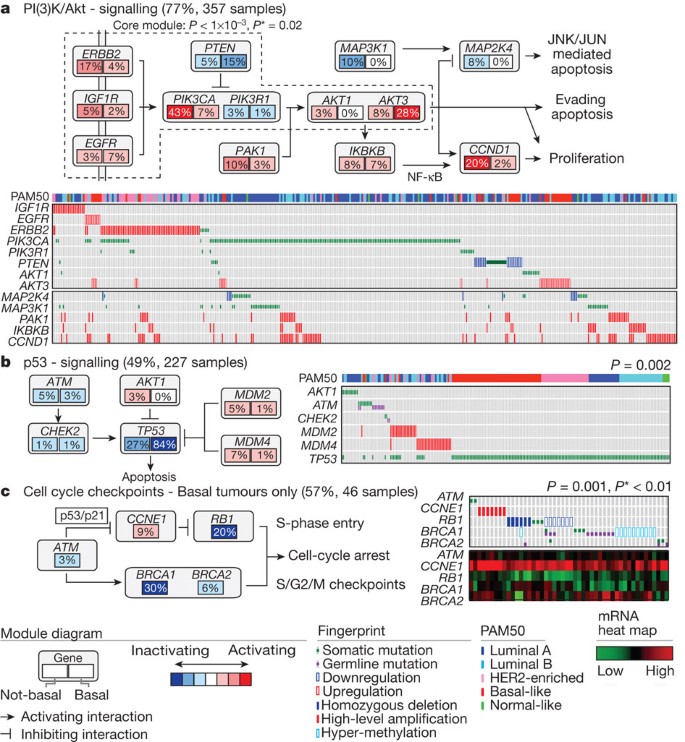
Mutual exclusivity modules are represented by their gene components and connected to reflect their activity in distinct pathways. For each gene, the frequency of alteration in basal-like (right box) and non-basal (left box) is reported. Next to each module is a fingerprint indicating what specific alteration is observed for each gene (row) in each sample (column). a , MEMo identified several overlapping modules that recapitulate the RTK–PI(3)K and p38–JNK1 signalling pathways and whose core was the top-scoring module. b , MEMo identified alterations to TP53 signalling as occurring within a statistically significant mutually exclusive trend. c , A basal-like only MEMo analysis identified one module that included ATM mutations, defects at BRCA1 and BRCA2 , and deregulation of the RB1 pathway. A gene expression heat map is below the fingerprint to show expression levels.
The TP53 pathway was differentially inactivated in luminal/ER + breast cancers, with a low TP53 mutation frequency in luminal A (12%) and a higher frequency in luminal B (29%) cancers ( Fig. 1 ). In addition to TP53 itself, a number of other pathway-inactivating events occurred including ATM loss and MDM2 amplification ( Figs 3b and 4b ), both of which occurred more frequently within luminal B cancers. Gene expression analysis demonstrated that individual markers of functional TP53 ( GADD45A and CDKN1A ), and TP53 activity 42 , 43 signatures, were highest in luminal A cancers ( Fig. 3b ). These data indicate that the TP53 pathway remains largely intact in luminal A cancers but is often inactivated in the more aggressive luminal B cancers 44 . Other PARADIGM-based pathway differences driving luminal B versus luminal A included hyperactivation of transcriptional activity associated with MYC and FOXM1 proliferation.
The critical retinoblastoma/RB1 pathway also showed mRNA-subtype-specific alterations ( Fig. 3c ). RB1 itself, by mRNA and protein expression, was detectable in most luminal cancers, with highest levels within luminal A. A common oncogenic event was cyclin D1 amplification and high expression, which preferentially occurred within luminal tumours, and more specifically within luminal B. In contrast, the presumed tumour suppressor CDKN2C (also called p18 ) was at its lowest levels in luminal A cancers, consistent with observations in mouse models 45 . Finally, RB1 activity signatures were also high in luminal cancers 46 , 47 , 48 . Luminal A tumours, which have the best prognosis, are the most likely to retain activity of the major tumour suppressors RB1 and TP53.
These genomic characterizations also provided clues for druggable targets. We compiled a drug target table in which we defined a target as a gene/protein for which there is an approved or investigational drug in human clinical trials targeting the molecule or canonical pathway ( Supplementary Table 6 ). In luminal/ER + cancers, the high frequency of PIK3CA mutations suggests that inhibitors of this activated kinase or its signalling pathway may be beneficial. Other potential significantly mutated gene drug candidates include AKT1 inhibitors (11 out of 12 AKT1 variants were luminal) and PARP inhibitors for BRCA1 / BRCA2 mutations. Although still unapproved as biomarkers, many potential copy-number-based drug targets were identified including amplifications of fibroblast growth factor receptors (FGFRs) and IGFR1 , as well as cyclin D1, CDK4 and CDK6 . A summary of the general findings in luminal tumours and the other subtypes is presented in Table 1 .
HER2-based classifications and summary analysis
DNA amplification of HER2 was readily evident in this study ( Supplementary Fig. 9 ) together with overexpression of multiple HER2-amplicon-associated genes that in part define the HER2E mRNA subtype ( Supplementary Fig. 5 ). However, not all clinically HER2 + tumours are of the HER2E mRNA subtype, and not all tumours in the HER2E mRNA subtype are clinically HER2 + . Integrated analysis of the RPPA and mRNA data clearly identified a HER2 + group ( Supplementary Fig. 12 ). When the HER2 + protein and HER2E mRNA subtypes overlapped, a strong signal of EGFR, pEGFR, HER2 and pHER2 was observed. However, only ∼ 50% of clinically HER2 + tumours fall into this HER2E-mRNA-subtype/HER2-protein group, the rest of the clinically HER2 + tumours were observed predominantly in the luminal mRNA subtypes.
These data indicate that there exist at least two types of clinically defined HER2 + tumours. To identify differences between these groups, a supervised gene expression analysis comparing 36 HER2E-mRNA-subtype/HER2 + versus 31 luminal-mRNA-subtype/HER2 + tumours was performed and identified 302 differentially expressed genes ( q -value = 0%) ( Supplementary Fig. 18 and Supplementary Table 7 ). These genes largely track with ER status but also indicated that HER2E-mRNA-subtype/HER2 + tumours showed significantly higher expression of a number of RTKs including FGFR4 , EGFR , HER2 itself, as well as genes within the HER2 amplicon (including GRB7 ). Conversely, the luminal-mRNA-subtype/HER2 + tumours showed higher expression of the luminal cluster of genes including GATA3 , BCL2 and ESR1 . Further support for two types of clinically defined HER2 + disease was evident in the somatic mutation data supervised by either mRNA subtype or ER status; TP53 mutations were significantly enriched in HER2E or ER-negative tumours whereas GATA3 mutations were only observed in luminal subtypes or ER + tumours.
Analysis of the RPPA data according to mRNA subtype identified 36 differentially expressed proteins ( q -value <5%) ( Supplementary Fig. 18G and Supplementary Table 8 ). The EGFR/pEGFR/HER2/pHER2 signal was again observed and present within the HER2E-mRNA-subtype/HER2 + tumours, as was high pSRC and pS6; conversely, many protein markers of luminal cancers again distinguished the luminal-mRNA-subtype/HER2 + tumours. Given the importance of clinical HER2 status, a more focused analysis was performed based on the RPPA-defined protein expression of HER2 ( Supplementary Fig. 19 )—the results strongly recapitulated findings from the RPPA and mRNA subtypes including a high correlation between HER2 clinical status, HER2 protein by RPPA, pHER2, EGFR and pEGFR. These multiple signatures, namely HER2E mRNA subtype, HER2 amplicon genes by mRNA expression, and RPPA EGFR/pEGFR/HER2/pHER2 signature, ultimately identify at least two groups/subtypes within clinically HER2 + tumours ( Table 1 ). These signatures represent breast cancer biomarker(s) that could potentially predict response to anti-HER2 targeted therapies.
Many therapeutic advances have been made for clinically HER2 + disease. This study has identified additional somatic mutations that represent potential therapeutic targets within this group, including a high frequency of PIK3CA mutations (39%), a lower frequency of PTEN and PIK3R1 mutations ( Supplementary Table 6 ), and genomic losses of PTEN and INPP4B . Other possible druggable mutations included variants within HER family members including two somatic mutations in HER2 , two within EGFR , and five within HER3 . Pertuzumab, in combination with trastuzumab, targets the HER2–HER3 heterodimer 49 ; however, these data suggest that targeting EGFR with HER2 could also be beneficial. Finally, the HER2E mRNA subtype typically showed high aneuploidy, the highest somatic mutation rate ( Table 1 ), and DNA amplification of other potential therapeutic targets including FGFRs, EGFR , CDK4 and cyclin D1.
Basal-like summary analysis
The basal-like subtype was discovered more than a decade ago by first-generation cDNA microarrays 13 . These tumours are often referred to as triple-negative breast cancers (TNBCs) because most basal-like tumours are typically negative for ER, PR and HER2. However, ∼ 75% of TNBCs are basal-like with the other 25% comprised of all other mRNA subtypes 6 . In this data set, there was a high degree of overlap between these two distinctions with 76 TNBCs, 81 basal-like, and 65 that were both TNBCs and basal-like. Given the known heterogeneity of TNBCs, and that the basal-like subtype proved to be distinct on every platform, we chose to use the basal-like distinction for comparative analyses.
Basal-like tumours showed a high frequency of TP53 mutations (80%) 9 , which when combined with inferred TP53 pathway activity suggests that loss of TP53 function occurs within most, if not all, basal-like cancers ( Fig. 3b ). In addition to loss of TP53 , a MEMo analysis reconfirmed that loss of RB1 and BRCA1 are basal-like features ( Fig. 4c ) 47 , 50 . PIK3CA was the next most commonly mutated gene ( ∼ 9%); however, inferred PI(3)K pathway activity, whether from gene 37 , 38 , 39 , protein 36 , or high PI(3)K/AKT pathway activities, was highest in basal-like cancers ( Fig. 3a ). Alternative means of activating the PI(3)K pathway in basal-like cancers probably includes loss of PTEN and INPP4B and/or amplification of PIK3CA . A recent paper 12 performed exome sequencing of 102 TNBCs. Five of the top six most frequent TNBC mutations in ref. 12 were also observed at a similar frequency in our TNBC subset ( Myo3A not present here); of those five, three passed our test as a significantly mutated gene in TNBCs ( Supplementary Table 2 ).
Expression features of basal-like tumours include a characteristic signature containing keratins 5, 6 and 17 and high expression of genes associated with cell proliferation ( Supplementary Fig. 5 ). A PARADIGM 33 analysis of basal-like versus luminal tumours emphasized the importance of hyperactivated FOXM1 as a transcriptional driver of this enhanced proliferation signature ( Supplementary Fig. 17 ). PARADIGM also identified hyperactivated MYC and HIF1-α/ARNT network hubs as key regulatory features of basal-like cancers. Even though chromosome 8q24 is amplified across all subtypes ( Supplementary Fig. 9 ), high MYC activation seems to be a basal-like characteristic 51 .
Given the striking contrasts between basal-like and luminal/HER2E subtypes, we performed a MEMo analysis on basal-like tumours alone. The top-scoring module included ATM mutations, BRCA1 and BRCA2 inactivation, RB1 loss and cyclin E1 amplification ( Fig. 4c ). Notably, these same modules were identified previously for serous ovarian cancers 41 . Furthermore, the basal-like (and TNBC) mutation spectrum was reminiscent of the spectrum seen in serous ovarian cancers 52 with only one gene (that is, TP53 ) at >10% mutation frequency. To explore possible similarities between serous ovarian and the breast basal-like cancers, we performed a number of analyses comparing ovarian versus breast luminal, ovarian versus breast basal-like, and breast basal-like versus breast luminal cancers ( Fig. 5 ). Comparing copy number landscapes, we observed several common features between ovarian and basal-like tumours including widespread genomic instability and common gains of 1q, 3q, 8q and 12p, and loss of 4q, 5q and 8p ( Supplementary Fig. 20A ). Using a more global copy number comparison, we examined the overall fraction of the genome altered and the overall copy number correlation of ovarian cancers versus each breast cancer mRNA subtype ( Supplementary Fig. 20A, B ); in both cases, basal-like tumours were the most similar to the serous ovarian carcinomas.
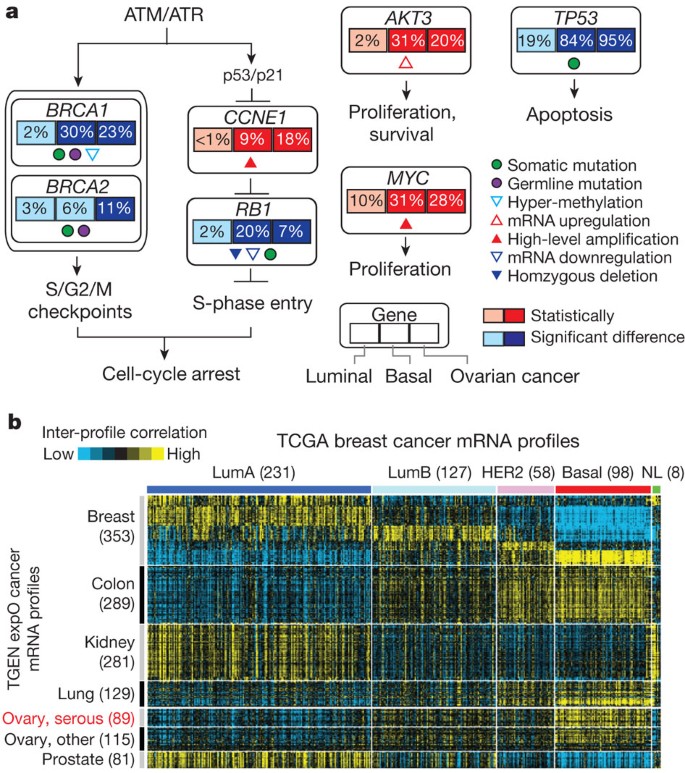
a , Significantly enriched genomic alterations identified by comparing basal-like or serous ovarian tumours to luminal cancers. b , Inter-sample correlations (yellow, positive) between gene transcription profiles of breast tumours (columns; TCGA data, arranged by subtype) and profiles of cancers from various tissues of origin (rows; external ‘TGEN expO’ data set, GSE2109) including ovarian cancers.
We systematically looked for other common features between serous ovarian and basal-like tumours when each was compared to luminal. We identified: (1) BRCA1 inactivation; (2) RB1 loss and cyclin E1 amplification; (3) high expression of AKT3 ; (4) MYC amplification and high expression; and (5) a high frequency of TP53 mutations ( Fig. 5a ). An additional supervised analysis of a large, external multitumour type transcriptomic data set (Gene Expression Omnibus accession GSE2109) was performed where each TCGA (The Cancer Genome Atlas) breast tumour expression profile was compared via a correlation analysis to that of each tumour in the multitumour set. Basal-like breast cancers clearly showed high mRNA expression correlations with serous ovarian cancers, as well as with lung squamous carcinomas ( Fig. 5b ). A PARADIGM analysis that calculates whether a gene or pathway feature is both differentially activated in basal-like versus luminal cancers and has higher overall activity across the TCGA ovarian samples was performed; this identified comparably high pathway activity of the HIF1-α/ARNT, MYC and FOXM1 regulatory hubs in both ovarian and basal-like cancers ( Supplementary Fig. 20C ). The common findings of TP53 , RB1 and BRCA1 loss, with MYC amplification, strongly suggest that these are shared driving events for basal-like and serous ovarian carcinogenesis. This suggests that common therapeutic approaches should be considered, which is supported by the activity of platinum analogues and taxanes in breast basal-like and serous ovarian cancers.
Given that most basal-like cancers are TNBCs, finding new drug targets for this group is critical. Unfortunately, the somatic mutation repertoire for basal-like breast cancers has not provided a common target aside from BRCA1 and BRCA2. Here we note that ∼ 20% of basal-like tumours had a germline ( n = 12) and/or somatic ( n = 8) BRCA1 or BRCA2 variant, which suggests that one in five basal-like patients might benefit from PARP inhibitors and/or platinum compounds 53 , 54 . The copy number landscape of basal-like cancers showed multiple amplifications and deletions, some of which may provide therapeutic targets ( Supplementary Table 6 ). Potential targets include losses of PTEN and INPP4B , both of which have been shown to sensitize cell lines to PI(3)K pathway inhibitors 55 , 56 . Interestingly, many of the components of the PI(3)K and RAS–RAF–MEK pathway were amplified (but not typically mutated) in basal-like cancers including PIK3CA (49%), KRAS (32%), BRAF (30%) and EGFR (23%). Other RTKs that are plausible drug targets and amplified in some basal-like cancers include FGFR1 , FGFR2 , IGFR1 , KIT , MET and PDGFRA . Finally, the PARADIGM identification of high HIF1-α/ARNT pathway activity suggests that these malignancies might be susceptible to angiogenesis inhibitors and/or bioreductive drugs that become activated under hypoxic conditions.
Concluding remarks
The integrated molecular analyses of breast carcinomas that we report here significantly extends our knowledge base to produce a comprehensive catalogue of likely genomic drivers of the most common breast cancer subtypes ( Table 1 ). Our novel observation that diverse genetic and epigenetic alterations converge phenotypically into four main breast cancer classes is not only consistent with convergent evolution of gene circuits, as seen across multiple organisms, but also with models of breast cancer clonal expansion and in vivo cell selection proposed to explain the phenotypic heterogeneity observed within defined breast cancer subtypes.
Methods Summary
Specimens were obtained from patients with appropriate consent from institutional review boards. Using a co-isolation protocol, DNA and RNA were purified. In total, 800 patients were assayed on at least one platform. Different numbers of patients were used for each platform using the largest number of patients available at the time of data freeze; 466 samples (463 patients) were in common across 5 out of 6 platforms (excluding RPPA) and 348 patients were in common on 6 out of 6 platforms. Technology platforms used include: (1) gene expression DNA microarrays 52 ; (2) DNA methylation arrays; (3) miRNA sequencing; (4) Affymetrix SNP arrays; (5) exome sequencing; and (6) reverse phase protein arrays. Each platform, except for the exome sequencing, was used in a de novo subtype discovery analysis ( Supplementary Methods ) and then included in a single analysis to define an overall subtype architecture. Additional integrated across-platform computational analyses were preformed including PARADIGM 33 and MEMo 41 .
Change history
03 october 2012.
The spelling of an author name (J.B.) was corrected.
Paik, S. et al. A multigene assay to predict recurrence of tamoxifen-treated, node-negative breast cancer. N. Engl. J. Med. 351 , 2817–2826 (2004)
Article CAS Google Scholar
van 't Veer, L. J. et al. Gene expression profiling predicts clinical outcome of breast cancer. Nature 415 , 530–536 (2002)
Slamon, D. J. et al. Human breast cancer: correlation of relapse and survival with amplification of the HER-2/neu oncogene. Science 235 , 177–182 (1987)
Article ADS CAS Google Scholar
Chin, K. et al. Genomic and transcriptional aberrations linked to breast cancer pathophysiologies. Cancer Cell 10 , 529–541 (2006)
Bergamaschi, A. et al. Distinct patterns of DNA copy number alteration are associated with different clinicopathological features and gene-expression subtypes of breast cancer. Genes Chromosom. Cancer 45 , 1033–1040 (2006)
Perou, C. M. Molecular stratification of triple-negative breast cancers. Oncologist 16 (suppl. 1). 61–70 (2011)
Article Google Scholar
Sorlie, T. et al. Repeated observation of breast tumor subtypes in independent gene expression data sets. Proc. Natl Acad. Sci. USA 100 , 8418–8423 (2003)
Foulkes, W. D. et al. Germline BRCA1 mutations and a basal epithelial phenotype in breast cancer. J. Natl Cancer Inst. 95 , 1482–1485 (2003)
Carey, L. A. et al. Race, breast cancer subtypes, and survival in the Carolina Breast Cancer Study. J. Am. Med. Assoc. 295 , 2492–2502 (2006)
Ding, L. et al. Genome remodelling in a basal-like breast cancer metastasis and xenograft. Nature 464 , 999–1005 (2010)
Shah, S. P. et al. Mutational evolution in a lobular breast tumour profiled at single nucleotide resolution. Nature 461 , 809–813 (2009)
Shah, S. P. et al. The clonal and mutational evolution spectrum of primary triple-negative breast cancers. Nature 486 , 395–399 (2012)
Perou, C. M. et al. Molecular portraits of human breast tumours. Nature 406 , 747–752 (2000)
Parker, J. S. et al. Supervised risk predictor of breast cancer based on intrinsic subtypes. J. Clin. Oncol. 27 , 1160–1167 (2009)
González-Pérez, A. & Lopez-Bigas, N. Improving the assessment of the outcome of nonsynonymous SNVs with a consensus deleteriousness score, Condel. Am. J. Hum. Genet. 88 , 440–449 (2011)
Dees, N. D. et al. MuSiC: Identifying mutational significance in cancer genomes. Genome Res. 22 , 1589–1598 (2012)
Bamshad, M. et al. Mutations in human TBX3 alter limb, apocrine and genital development in ulnar-mammary syndrome. Nature Genet. 16 , 311–315 (1997)
Li, Q. Y. et al. Holt-Oram syndrome is caused by mutations in TBX5, a member of the Brachyury (T) gene family. Nature Genet. 15 , 21–29 (1997)
The Cancer Genome Atlas Research Network. Comprehensive genomic characterization defines human glioblastoma genes and core pathways. Nature 455 , 1061–1068 (2008)
Cheung, L. W. et al. High frequency of PIK3R1 and PIK3R2 mutations in endometrial cancer elucidates a novel mechanism for regulation of PTEN protein stability. Cancer Discov. 1 , 170–185 (2011)
Malcovati, L. et al. Clinical significance of SF3B1 mutations in myelodysplastic syndromes and myelodysplastic/myeloproliferative neoplasms. Blood 118 , 6239–6246 (2011)
Wang, L. et al. SF3B1 and other novel cancer genes in chronic lymphocytic leukemia. N. Engl. J. Med. 365 , 2497–2506 (2011)
Ding, L. et al. Somatic mutations affect key pathways in lung adenocarcinoma. Nature 455 , 1069–1075 (2008)
Johnson, G. L. & Lapadat, R. Mitogen-activated protein kinase pathways mediated by ERK, JNK, and p38 protein kinases. Science 298 , 1911–1912 (2002)
Usary, J. et al. Mutation of GATA3 in human breast tumors. Oncogene 23 , 7669–7678 (2004)
Walsh, T. et al. Detection of inherited mutations for breast and ovarian cancer using genomic capture and massively parallel sequencing. Proc. Natl Acad. Sci. USA 107 , 12629–12633 (2010)
Prat, A. et al. Phenotypic and molecular characterization of the claudin-low intrinsic subtype of breast cancer. Breast Cancer Res. 12 , R68 (2010)
Kozomara, A. & Griffiths-Jones, S. miRBase: integrating microRNA annotation and deep-sequencing data. Nucleic Acids Res. 39 , D152–D157 (2011)
Weigman, V. J. et al. Basal-like breast cancer DNA copy number losses identify genes involved in genomic instability, response to therapy, and patient survival. Breast Cancer Res. Treat. 133 , 865–880 (2011)
Curtis, C. et al. The genomic and transcriptomic architecture of 2,000 breast tumours reveals novel subgroups. Nature 486 , 346–352 (2012)
Hennessy, B. T. et al. A technical assessment of the utility of reverse phase protein arrays for the study of the functional proteome in non-microdissected human breast cancers. Clin. Proteomics 6 , 129–151 (2010)
Daub, H. et al. Kinase-selective enrichment enables quantitative phosphoproteomics of the kinome across the cell cycle. Mol. Cell 31 , 438–448 (2008)
Vaske, C. J. et al. Inference of patient-specific pathway activities from multi-dimensional cancer genomics data using PARADIGM. Bioinformatics 26 , 237–245 (2010)
Campbell, I. G. et al. Mutation of the PIK3CA gene in ovarian and breast cancer. Cancer Res. 64 , 7678–7681 (2004)
Bachman, K. E. et al. The PIK3CA gene is mutated with high frequency in human breast cancers. Cancer Biol. Ther. 3 , 772–775 (2004)
Stemke-Hale, K. et al. An integrative genomic and proteomic analysis of PIK3CA, PTEN, and AKT mutations in breast cancer. Cancer Res. 68 , 6084–6091 (2008)
Creighton, C. J. et al. Proteomic and transcriptomic profiling reveals a link between the PI3K pathway and lower estrogen-receptor (ER) levels and activity in ER + breast cancer. Breast Cancer Res. 12 , R40 (2010)
Majumder, P. K. et al. mTOR inhibition reverses Akt-dependent prostate intraepithelial neoplasia through regulation of apoptotic and HIF-1-dependent pathways. Nature Med. 10 , 594–601 (2004)
Saal, L. H. et al. Recurrent gross mutations of the PTEN tumor suppressor gene in breast cancers with deficient DSB repair. Nature Genet. 40 , 102–107 (2008)
Wagner, E. F. & Nebreda, A. R. Signal integration by JNK and p38 MAPK pathways in cancer development. Nature Rev. Cancer 9 , 537–549 (2009)
Ciriello, G., Cerami, E., Sander, C. & Schultz, N. Mutual exclusivity analysis identifies oncogenic network modules. Genome Res. 22 , 398–406 (2012)
Kannan, K. et al. DNA microarrays identification of primary and secondary target genes regulated by p53. Oncogene 20 , 2225–2234 (2001)
Troester, M. A. et al. Gene expression patterns associated with p53 status in breast cancer. BMC Cancer 6 , 276 (2006)
Deisenroth, C., Thorner, A. R., Enomoto, T., Perou, C. M. & Zhang, Y. Mitochondrial Hep27 is a c-Myb target gene that inhibits Mdm2 and stabilizes p53. Mol. Cell. Biol. 30 , 3981–3993 (2010)
Pei, X. H. et al. CDK inhibitor p18 INK4c is a downstream target of GATA3 and restrains mammary luminal progenitor cell proliferation and tumorigenesis. Cancer Cell 15 , 389–401 (2009)
Chicas, A. et al. Dissecting the unique role of the retinoblastoma tumor suppressor during cellular senescence. Cancer Cell 17 , 376–387 (2010)
Herschkowitz, J. I., He, X., Fan, C. & Perou, C. M. The functional loss of the retinoblastoma tumour suppressor is a common event in basal-like and luminal B breast carcinomas. Breast Cancer Res. 10 , R75 (2008)
Lara, M. F. et al. Gene profiling approaches help to define the specific functions of retinoblastoma family in epidermis. Mol. Carcinog. 47 , 209–221 (2008)
Baselga, J. et al. Pertuzumab plus trastuzumab plus docetaxel for metastatic breast cancer. N. Engl. J. Med. 366 , 109–119 (2012)
Jiang, Z. et al. Rb deletion in mouse mammary progenitors induces luminal-B or basal-like/EMT tumor subtypes depending on p53 status. J. Clin. Invest. 120 , 3296–3309 (2010)
Chandriani, S. et al. A core MYC gene expression signature is prominent in basal-like breast cancer but only partially overlaps the core serum response. PLoS ONE 4 , e6693 (2009)
Article ADS Google Scholar
The Cancer Genome Atlas Research Network. Integrated genomic analyses of ovarian carcinoma. Nature 474 , 609–615 (2011)
Audeh, M. W. et al. Oral poly(ADP-ribose) polymerase inhibitor olaparib in patients with BRCA1 or BRCA2 mutations and recurrent ovarian cancer: a proof-of-concept trial. Lancet 376 , 245–251 (2010)
Fong, P. C. et al. Inhibition of poly(ADP-ribose) polymerase in tumors from BRCA mutation carriers. N. Engl. J. Med. 361 , 123–134 (2009)
Fedele, C. G. et al. Inositol polyphosphate 4-phosphatase II regulates PI3K/Akt signaling and is lost in human basal-like breast cancers. Proc. Natl Acad. Sci. USA 107 , 22231–22236 (2010)
Gewinner, C. et al. Evidence that inositol polyphosphate 4-phosphatase type II is a tumor suppressor that inhibits PI3K signaling. Cancer Cell 16 , 115–125 (2009)
Download references
Acknowledgements
We thank M. Sheth and S. Lucas for administrative coordination of TCGA activities, and C. Gunter for critical reading of the manuscript. This work was supported by the following grants from the USA National Institutes of Health: U24CA143883, U24CA143858, U24CA143840, U24CA143799, U24CA143835, U24CA143845, U24CA143882, U24CA143867, U24CA143866, U24CA143848, U24CA144025, U54HG003079, P50CA116201 and P50CA58223. Additional support was provided by the Susan G. Komen for the Cure, the US Department of Defense through the Henry M. Jackson Foundation for the Advancement of Military Medicine, and the Breast Cancer Research Foundation. The views expressed in this paper are those of the authors and do not reflect the official policy of the Department of Defense, or US Government.
Author information
Authors and affiliations.
The Genome Institute, Washington University, St Louis, Missouri 63108, USA.,
Daniel C. Koboldt, Robert S. Fulton, Michael D. McLellan, Heather Schmidt, Joelle Kalicki-Veizer, Joshua F. McMichael, Lucinda L. Fulton, David J. Dooling, Li Ding, Elaine R. Mardis & Richard K. Wilson
Department of Genetics, Washington University, St Louis, Missouri 63110, USA.,
Li Ding, Elaine R. Mardis & Richard K. Wilson
Siteman Cancer Center, Washington University, St Louis, Missouri 63110, USA.,
Elaine R. Mardis, Richard K. Wilson, Matthew J. Ellis & Ron Bose
Canada’s Michael Smith Genome Sciences Centre, BC Cancer Agency, Vancouver, British Columbia V5Z, Canada.,
Adrian Ally, Miruna Balasundaram, Yaron S. N. Butterfield, Rebecca Carlsen, Candace Carter, Andy Chu, Eric Chuah, Hye-Jung E. Chun, Robin J. N. Coope, Noreen Dhalla, Ranabir Guin, Carrie Hirst, Martin Hirst, Robert A. Holt, Darlene Lee, Haiyan I. Li, Michael Mayo, Richard A. Moore, Andrew J. Mungall, Erin Pleasance, A. Gordon Robertson, Jacqueline E. Schein, Arash Shafiei, Payal Sipahimalani, Jared R. Slobodan, Dominik Stoll, Angela Tam, Nina Thiessen, Richard J. Varhol, Natasja Wye, Thomas Zeng, Yongjun Zhao, Inanc Birol, Steven J. M. Jones & Marco A. Marra
The Broad Institute of MIT and Harvard, Cambridge, 02142, Massachusetts, USA
Andrew D. Cherniack, Gordon Saksena, Robert C. Onofrio, Nam H. Pho, Scott L. Carter, Steven E. Schumacher, Barbara Tabak, Bryan Hernandez, Jeff Gentry, Huy Nguyen, Andrew Crenshaw, Kristin Ardlie, Rameen Beroukhim, Wendy Winckler, Gad Getz, Stacey B. Gabriel & Matthew Meyerson
Department of Cancer Biology, Dana-Farber Cancer Institute, Boston, Massachusetts 02115, USA.,
Steven E. Schumacher & Barbara Tabak
Department of Medicine, Harvard Medical School, Boston, 02215, Massachusetts, USA
Rameen Beroukhim
Departments of Cancer Biology and Medical Oncology, and the Center for Cancer Genome Discovery, Dana-Farber Cancer Institute, Boston, Massachusetts 02115, USA.,
Department of Medical Oncology and the Center for Cancer Genome Discovery, Dana-Farber Cancer Institute, Boston, Massachusetts 02115, USA.,
Matthew Meyerson & Lynda Chin
Department of Pathology, Harvard Medical School, Boston, 02215, Massachusetts, USA
Matthew Meyerson & Andrew H. Beck
Belfer Institute for Applied Cancer Science, Dana-Farber Cancer Institute, Boston, Massachusetts 02115, USA.,
The Center for Biomedical Informatics, Harvard Medical School, Boston, 02115, Massachusetts, USA
Peter J. Park, Nils Gehlenborg & Peter J. Park
Department of Genetics, Harvard Medical School and Division of Genetics, Brigham and Women’s Hospital, Boston, 02115, Massachusetts, USA
Raju Kucherlapati
Department of Genetics, University of North Carolina at Chapel Hill, Chapel Hill, North Carolina 27599, USA.,
Katherine A. Hoadley, Xiaping He, Hann-Hsiang Chao, Aleix Prat, Grace O. Silva, Michael D. Iglesia, Wei Zhao, Jonathan S. Berg, Michael Adams & Charles M. Perou
Lineberger Comprehensive Cancer Center, University of North Carolina at Chapel Hill, Chapel Hill, 27599, North Carolina, USA
Katherine A. Hoadley, Cheng Fan, Yidi J. Turman, Yan Shi, Ling Li, Michael D. Topal, Xiaping He, Hann-Hsiang Chao, Aleix Prat, Grace O. Silva, Michael D. Iglesia, Wei Zhao, Jerry Usary, Jonathan S. Berg, Junyuan Wu, Anisha Gulabani, Tom Bodenheimer, Alan P. Hoyle, Janae V. Simons, Matthew G. Soloway, Lisle E. Mose, Stuart R. Jefferys, Saianand Balu, Joel S. Parker, D. Neil Hayes, Charles M. Perou, W. Kimryn Rathmell, Leigh Thorne, Mei Huang, Lori Boice & Ashley Hill
Eshelman School of Pharmacy, University of North Carolina at Chapel Hill, Chapel Hill, North Carolina 27599, USA.,
J. Todd Auman
Institute for Pharmacogenetics and Individualized Therapy, University of North Carolina at Chapel Hill, Chapel Hill, 27599, North Carolina, USA
Department of Pathology and Laboratory Medicine, University of North Carolina at Chapel Hill, Chapel Hill, Chapel Hill, 27599, North Carolina, USA
Michael D. Topal, Jessica Booker & Charles M. Perou
Department of Internal Medicine, Division of Medical Oncology, University of North Carolina at Chapel Hill, Chapel Hill, 27599, North Carolina, USA
D. Neil Hayes
USC Epigenome Center, University of Southern California, Los Angeles, California 90033, USA.,
Simeen Malik, Swapna Mahurkar, Hui Shen, Daniel J. Weisenberger, Timothy Triche Jr, Phillip H. Lai, Moiz S. Bootwalla, Dennis T. Maglinte, Benjamin P. Berman, David J. Van Den Berg & Peter W. Laird
Cancer Biology Division, The Sidney Kimmel Comprehensive Cancer Center at Johns Hopkins University, Baltimore, 21231, Maryland, USA
Stephen B. Baylin
Dan L Duncan Cancer Center, Baylor College of Medicine, Houston, 77030, Texas, USA
Chad J. Creighton & Lawrence A. Donehower
Human Genome Sequencing Center, Baylor College of Medicine, Houston, 77030, Texas, USA
Department of Molecular and Cellular Biology, Baylor College of Medicine, Houston, 77030, Texas, USA
Lawrence A. Donehower
Department of Molecular Virology and Microbiology, Baylor College of Medicine, Houston, Texas 77030, USA.,
The Eli and Edythe L. Broad Institute of Massachusetts Institute Of Technology and Harvard University, Cambridge, 02142, Massachusetts, USA
Gad Getz, Michael Noble, Doug Voet, Gordon Saksena, Nils Gehlenborg, Daniel DiCara, Hailei Zhang, Spring Yingchun Liu, Michael S. Lawrence, Lihua Zou, Andrey Sivachenko, Pei Lin, Petar Stojanov, Rui Jing, Juok Cho, Raktim Sinha, Richard W. Park, Marc-Danie Nazaire, Jim Robinson, Helga Thorvaldsdottir, Jill Mesirov & Lynda Chin
Department of Genomic Medicine, Institute for Applied Cancer Science, University of Texas MD Anderson Cancer Center, Houston, 77054, Texas, USA
Juinhua Zhang & Lynda Chin
Department of Genomic Medicine, University of Texas MD Anderson Cancer Center, Houston, 77054, Texas, USA
Chang-Jiun Wu
Division of Genetics, Brigham and Women’s Hospital, Boston, 02115, Massachusetts, USA
Peter J. Park
Informatics Program, Children’s Hospital, Boston, 02115, Massachusetts, USA
Institute for Systems Biology, Seattle, 98109, Washington, USA
Sheila Reynolds, Richard B. Kreisberg, Brady Bernard, Ryan Bressler, Jake Lin, Vesteinn Thorsson & Ilya Shmulevich
Tampere University of Technology, Tampere, Finland
Timo Erkkila
Cancer Genomics Core Laboratory, MD Anderson Cancer Center, Houston, 77030, Texas, USA
Computational Biology Center, Memorial Sloan-Kettering Cancer Center, New York, 10065, New York, USA
Giovanni Ciriello, Nils Weinhold, Nikolaus Schultz, Jianjiong Gao, Ethan Cerami, Benjamin Gross, Anders Jacobsen, Rileen Sinha, B. Arman Aksoy, Yevgeniy Antipin, Boris Reva, Barry S. Taylor & Chris Sander
Department of Epidemiology and Biostatistics, Memorial Sloan-Kettering Cancer Center, New York, 10065, New York, USA
Ronglai Shen
Human Oncology and Pathogenesis Program, Memorial Sloan-Kettering Cancer Center, New York, 10065, New York, USA
Marc Ladanyi
Oregon Health and Science University, 3181 Southwest Sam Jackson Park Road, Portland, Oregon 97239, USA.,
Pavana Anur & Paul T. Spellman
Department of Systems Biology, The University of Texas MD Anderson Cancer Center, Houston, 77030, Texas, USA
Yiling Lu, Gordon B. Mills, Ana Maria Gonzalez-Angulo & Ana Maria Gonzalez-Angulo
Kleberg Center for Molecular Markers, The University of Texas MD Anderson Cancer Center, Houston, 77030, Texas, USA
Yiling Lu & Gordon B. Mills
Department of Bioinformatics and Computational Biology, The University of Texas MD Anderson Cancer Center, Houston, 77030, Texas, USA
Wenbin Liu, Roel R. G. Verhaak, Rehan Akbani, Nianxiang Zhang, Bradley M. Broom, Tod D. Casasent, Chris Wakefield, Anna K. Unruh, Keith Baggerly, Kevin Coombes & John N. Weinstein
Department of Biomolecular Engineering and Center for Biomolecular Science and Engineering, University of California Santa Cruz, Santa Cruz, 95064, California, USA
David Haussler, Joshua M. Stuart, Stephen C. Benz, Jingchun Zhu, Christopher C. Szeto, Evan O. Paull, Daniel Carlin, Christopher Wong, Artem Sokolov, Sam Ng, Theodore C. Goldstein, Kyle Ellrott, Mia Grifford, Christopher Wilks, Singer Ma & Brian Craft
Howard Hughes Medical Institute, University of California Santa Cruz, Santa Cruz, 95064, California, USA
- David Haussler
Buck Institute for Research on Aging, Novato, 94945, California, USA
Christopher C. Benz, Gary K. Scott, Christina Yau, Janita Thusberg & Sean Mooney
Center for Bioinformatics and Information Technology, National Cancer Institute, Rockville, 20852, Maryland, USA
Chunhua Yan, Ying Hu, Daoud Meerzaman & Kenneth Buetow
Department of Pathology, The Ohio State University College of Medicine, Columbus, 43205, Ohio, USA
Julie M. Gastier-Foster, Nilsa C. Ramirez & Robert E. Pyatt
Department Pediatrics, The Ohio State University College of Medicine, Columbus, 43205, Ohio, USA
Julie M. Gastier-Foster & Peter White
The Research Institute at Nationwide Children’s Hospital, Columbus, 43205, Ohio, USA
Julie M. Gastier-Foster, Jay Bowen, Nilsa C. Ramirez, Aaron D. Black, Robert E. Pyatt, Peter White, Erik J. Zmuda, Jessica Frick, Tara M. Lichtenberg, Robin Brookens, Myra M. George, Mark A. Gerken, Hollie A. Harper, Kristen M. Leraas, Lisa J. Wise, Teresa R. Tabler, Cynthia McAllister, Thomas Barr & Melissa Hart-Kothari
ABS Inc., Indianapolis, 46204, Indiana, USA
- Katie Tarvin
ABS Inc., Wilmington, 19801, Delaware, USA
Charles Saller
Indiana University School of Medicine, Indianapolis, 46202, Indiana, USA
George Sandusky & Colleen Mitchell
Helen F. Graham Cancer Center, Christiana Care, Newark, 19713, Delaware, USA
Mary V. Iacocca, Jennifer Brown, Brenda Rabeno, Christine Czerwinski & Nicholas Petrelli
Moscow City Clinical Oncology Dispensary 1 and the Central IHC Laboratory of the Moscow Health Department, Moscow 105005, Russia.,
- Oleg Dolzhansky
Russian Cancer Research Center, Moscow 115478, Russia.,
Mikhail Abramov
Cureline, Inc., South San Francisco, 94080, California, USA
Olga Voronina & Olga Potapova
Department of Surgery, Duke University Medical Center, Durham, 27710, North Carolina, USA
Jeffrey R. Marks & Jeffrey R. Marks
The Greater Poland Cancer Centre, Poznań 61-866, Poland.,
Wiktoria M. Suchorska, Dawid Murawa, Witold Kycler, Matthew Ibbs, Konstanty Korski, Arkadiusz Spychała, Paweł Murawa, Jacek J. Brzeziński, Hanna Perz, Radosław Łaźniak, Marek Teresiak, Honorata Tatka, Ewa Leporowska, Marta Bogusz-Czerniewicz, Julian Malicki, Andrzej Mackiewicz, Maciej Wiznerowicz & Maciej Wiznerowicz
Poznan University of Medical Sciences, Poznań 61-701, Poland.,
Marta Bogusz-Czerniewicz, Julian Malicki, Andrzej Mackiewicz, Maciej Wiznerowicz & Maciej Wiznerowicz
ILSBio, LLC, Chestertown, 21620, Maryland, USA
Xuan Van Le & Bernard Kohl
Ministry of Health, Hanoi, Vietnam
Nguyen Viet Tien
ILSBio LLC, Karachi, Pakistan
Richard Thorp & Khurram Zaki Khan
Hue Central Hospital, Hue City, Vietnam.,
Nguyen Van Bang & Bui Duc Phu
Stanford University Medical Center, Stanford, 94305, California, USA
Howard Sussman
Center for Minority Health Research, University of Texas, MD Anderson Cancer Center, Houston, 07703, Texas, USA
Richard Hajek
National Cancer Institute, Hanoi, Vietnam
Nguyen Phi Hung
Ho Chi Minh City Cancer Center, Vietnam
Tran Viet The Phuong
Can Tho Cancer Center, Can Tho, Vietnam.,
Huynh Quyet Thang
International Genomics Consortium, Phoenix, 85004, Arizona, USA
Robert Penny, David Mallery, Erin Curley, Candace Shelton & Peggy Yena
Mayo Clinic, Rochester, 55905, Minnesota, USA
James N. Ingle, Fergus J. Couch, Wilma L. Lingle, Fergus J. Couch & James N. Ingle
Department of Surgery, Breast Service, Memorial Sloan-Kettering Cancer Center, New York, 10065, New York, USA
Tari A. King & Tari A. King
Department of Breast Medical Oncology, The University of Texas, MD Anderson Cancer Center, Houston, 77030, Texas, USA
Ana Maria Gonzalez-Angulo, Gordon B. Mills, Mary D. Dyer, Shuying Liu, Xiaolong Meng, Modesto Patangan & Ana Maria Gonzalez-Angulo
University of California at San Francisco,
Frederic Waldman & Frederic Waldman
San Francisco, 94143, California, USA
Cancer Diagnostics; Nichols Institute, Quest Diagnostics; San Juan Capistrano,
Nichols Institute, Quest Diagnostics, 92675, California, USA
Helen Diller Family Comprehensive Cancer Center, University of California San Francisco, San Francisco, 94115, California, USA
Hubert Stöppler
Department of Pathology, UNC Tissue Procurement Facility, UNC Lineberger Cancer Center, Chapel Hill, 27599, North Carolina, USA
Leigh Thorne, Mei Huang & Lori Boice
Department of Pathology, Roswell Park Cancer Institute, Buffalo, 14263, New York, USA
Carl Morrison, Carmelo Gaudioso & Wiam Bshara
Department of Pathology, University of Miami Miller School of Medicine, Sylvester Comprehensive Cancer Center, Miami, 33136, Florida, USA
Kelly Daily, Sophie C. Egea, Mark D. Pegram & Carmen Gomez-Fernandez
University of Pittsburgh, Pittsburgh, 15213, Pennsylvania, USA
Magee-Womens Hospital of University of Pittsburgh Medical Center, Pittsburgh, 15213, Pennsylvania, USA
Rohit Bhargava & Adam Brufsky
Walter Reed National Military Medical Center, Bethesda, 20899-5600, Maryland, USA
Craig D. Shriver, Jeffrey A. Hooke & Jamie Leigh Campbell
Windber Research Institute, Windber, 15963, Pennsylvania, USA
Richard J. Mural, Hai Hu, Stella Somiari, Caroline Larson, Brenda Deyarmin, Leonid Kvecher, Hai Hu, Richard J. Mural, Chunqing Luo & Yaqin Chen
MDR Global, LLC, Windber, 15963, Pennsylvania, USA
Albert J. Kovatich
Breast Cancer Program, Washington University, St Louis, Missouri 63110, USA.,
Matthew J. Ellis & Ron Bose
Department of Internal Medicine, Division of Oncology, Washington University, St Louis, Missouri 63110, USA.,
Institute for Genomics and Systems Biology, University of Chicago, Chicago, 60637, Illinois, USA
Thomas Stricker & Kevin White
Center for Clinical Cancer Genetics, The University of Chicago, Chicago, 60637, Illinois, USA
Olufunmilayo Olopade
Department of Pathology and Immunology, Washington University School of Medicine, St Louis, Missouri 63110, USA.,
Li-Wei Chang
SRA International, 4300 Fair Lakes Court, Fairfax, 22033, Virginia, USA
Todd Pihl, Mark Jensen, Robert Sfeir, Ari Kahn, Anna Chu, Prachi Kothiyal, Zhining Wang, Eric Snyder, Joan Pontius, Brenda Ayala, Mark Backus, Jessica Walton, Julien Baboud, Dominique Berton, Matthew Nicholls, Deepak Srinivasan, Rohini Raman, Stanley Girshik, Peter Kigonya, Shelley Alonso, Rashmi Sanbhadti, Sean Barletta & David Pot
The Cancer Genome Atlas Program Office, Center for Cancer Genomics, National Cancer Institute, Bethesda, 20852, Maryland, USA
Margi Sheth, John A. Demchok, Kenna R. Mills Shaw, Liming Yang, Roy W. Tarnuzzer, Jiashan Zhang, Laura A. L. Dillon & Peter Fielding
TCGA Consultant, Scimentis, LLC, Statham, Georgia 30666, USA.,
MLF Consulting, Arlington, 02474, Massachusetts, USA
Martin L. Ferguson
National Human Genome Research Institute, National Institutes of Health, Bethesda, 20892, Maryland, USA
Bradley A. Ozenberger, Mark S. Guyer, Heidi J. Sofia & Jacqueline D. Palchik
Genome sequencing centres: Washington University in St Louis
- Daniel C. Koboldt
- , Robert S. Fulton
- , Michael D. McLellan
- , Heather Schmidt
- , Joelle Kalicki-Veizer
- , Joshua F. McMichael
- , Lucinda L. Fulton
- , David J. Dooling
- , Elaine R. Mardis
- & Richard K. Wilson
Genome characterization centres: BC Cancer Agency
- Adrian Ally
- , Miruna Balasundaram
- , Yaron S. N. Butterfield
- , Rebecca Carlsen
- , Candace Carter
- , Eric Chuah
- , Hye-Jung E. Chun
- , Robin J. N. Coope
- , Noreen Dhalla
- , Ranabir Guin
- , Carrie Hirst
- , Martin Hirst
- , Robert A. Holt
- , Darlene Lee
- , Haiyan I. Li
- , Michael Mayo
- , Richard A. Moore
- , Andrew J. Mungall
- , Erin Pleasance
- , A. Gordon Robertson
- , Jacqueline E. Schein
- , Arash Shafiei
- , Payal Sipahimalani
- , Jared R. Slobodan
- , Dominik Stoll
- , Angela Tam
- , Nina Thiessen
- , Richard J. Varhol
- , Natasja Wye
- , Thomas Zeng
- , Yongjun Zhao
- , Inanc Birol
- , Steven J. M. Jones
- & Marco A. Marra
Broad Institute
- Andrew D. Cherniack
- , Gordon Saksena
- , Robert C. Onofrio
- , Nam H. Pho
- , Scott L. Carter
- , Steven E. Schumacher
- , Barbara Tabak
- , Bryan Hernandez
- , Jeff Gentry
- , Huy Nguyen
- , Andrew Crenshaw
- , Kristin Ardlie
- , Rameen Beroukhim
- , Wendy Winckler
- , Stacey B. Gabriel
- & Matthew Meyerson
Brigham & Women’s Hospital & Harvard Medical School
- , Peter J. Park
- & Raju Kucherlapati
University of North Carolina, Chapel Hill
- Katherine A. Hoadley
- , J. Todd Auman
- , Cheng Fan
- , Yidi J. Turman
- , Michael D. Topal
- , Xiaping He
- , Hann-Hsiang Chao
- , Aleix Prat
- , Grace O. Silva
- , Michael D. Iglesia
- , Jerry Usary
- , Jonathan S. Berg
- , Michael Adams
- , Jessica Booker
- , Junyuan Wu
- , Anisha Gulabani
- , Tom Bodenheimer
- , Alan P. Hoyle
- , Janae V. Simons
- , Matthew G. Soloway
- , Lisle E. Mose
- , Stuart R. Jefferys
- , Saianand Balu
- , Joel S. Parker
- , D. Neil Hayes
- & Charles M. Perou
University of Southern California/Johns Hopkins
- Simeen Malik
- , Swapna Mahurkar
- , Daniel J. Weisenberger
- , Timothy Triche Jr
- , Phillip H. Lai
- , Moiz S. Bootwalla
- , Dennis T. Maglinte
- , Benjamin P. Berman
- , David J. Van Den Berg
- , Stephen B. Baylin
- & Peter W. Laird
Genome data analysis: Baylor College of Medicine
- Chad J. Creighton
- & Lawrence A. Donehower
- , Michael Noble
- , Doug Voet
- , Nils Gehlenborg
- , Daniel DiCara
- , Juinhua Zhang
- , Hailei Zhang
- , Chang-Jiun Wu
- , Spring Yingchun Liu
- , Michael S. Lawrence
- , Lihua Zou
- , Andrey Sivachenko
- , Petar Stojanov
- , Raktim Sinha
- , Richard W. Park
- , Marc-Danie Nazaire
- , Jim Robinson
- , Helga Thorvaldsdottir
- , Jill Mesirov
- & Lynda Chin
Institute for Systems Biology
- Sheila Reynolds
- , Richard B. Kreisberg
- , Brady Bernard
- , Ryan Bressler
- , Timo Erkkila
- , Vesteinn Thorsson
- , Wei Zhang
- & Ilya Shmulevich
Memorial Sloan-Kettering Cancer Center
- Giovanni Ciriello
- , Nils Weinhold
- , Nikolaus Schultz
- , Jianjiong Gao
- , Ethan Cerami
- , Benjamin Gross
- , Anders Jacobsen
- , Rileen Sinha
- , B. Arman Aksoy
- , Yevgeniy Antipin
- , Boris Reva
- , Ronglai Shen
- , Barry S. Taylor
- , Marc Ladanyi
- & Chris Sander
Oregon Health & Science University
- Pavana Anur
- & Paul T. Spellman
The University of Texas MD Anderson Cancer Center
- , Wenbin Liu
- , Roel R. G. Verhaak
- , Gordon B. Mills
- , Rehan Akbani
- , Nianxiang Zhang
- , Bradley M. Broom
- , Tod D. Casasent
- , Chris Wakefield
- , Anna K. Unruh
- , Keith Baggerly
- , Kevin Coombes
- & John N. Weinstein
University of California, Santa Cruz/Buck Institute
- , Christopher C. Benz
- , Joshua M. Stuart
- , Stephen C. Benz
- , Jingchun Zhu
- , Christopher C. Szeto
- , Gary K. Scott
- , Christina Yau
- , Evan O. Paull
- , Daniel Carlin
- , Christopher Wong
- , Artem Sokolov
- , Janita Thusberg
- , Sean Mooney
- , Theodore C. Goldstein
- , Kyle Ellrott
- , Mia Grifford
- , Christopher Wilks
- , Singer Ma
- & Brian Craft
- Chunhua Yan
- & Daoud Meerzaman
Biospecimen core resource: Nationwide Children’s Hospital Biospecimen Core Resource
- Julie M. Gastier-Foster
- , Jay Bowen
- , Nilsa C. Ramirez
- , Aaron D. Black
- , Robert E. Pyatt
- , Peter White
- , Erik J. Zmuda
- , Jessica Frick
- , Tara M. Lichtenberg
- , Robin Brookens
- , Myra M. George
- , Mark A. Gerken
- , Hollie A. Harper
- , Kristen M. Leraas
- , Lisa J. Wise
- , Teresa R. Tabler
- , Cynthia McAllister
- , Thomas Barr
- & Melissa Hart-Kothari
Tissue source sites: ABS-IUPUI
- , Charles Saller
- , George Sandusky
- & Colleen Mitchell
- Mary V. Iacocca
- , Jennifer Brown
- , Brenda Rabeno
- , Christine Czerwinski
- & Nicholas Petrelli
- , Mikhail Abramov
- , Olga Voronina
- & Olga Potapova
Duke University Medical Center
- Jeffrey R. Marks
The Greater Poland Cancer Centre
- Wiktoria M. Suchorska
- , Dawid Murawa
- , Witold Kycler
- , Matthew Ibbs
- , Konstanty Korski
- , Arkadiusz Spychała
- , Paweł Murawa
- , Jacek J. Brzeziński
- , Hanna Perz
- , Radosław Łaźniak
- , Marek Teresiak
- , Honorata Tatka
- , Ewa Leporowska
- , Marta Bogusz-Czerniewicz
- , Julian Malicki
- , Andrzej Mackiewicz
- & Maciej Wiznerowicz
- Xuan Van Le
- , Bernard Kohl
- , Nguyen Viet Tien
- , Richard Thorp
- , Nguyen Van Bang
- , Howard Sussman
- , Bui Duc Phu
- , Richard Hajek
- , Nguyen Phi Hung
- , Tran Viet The Phuong
- , Huynh Quyet Thang
- & Khurram Zaki Khan
International Genomics Consortium
- Robert Penny
- , David Mallery
- , Erin Curley
- , Candace Shelton
- & Peggy Yena
Mayo Clinic
- James N. Ingle
- , Fergus J. Couch
- & Wilma L. Lingle
- Tari A. King
MD Anderson Cancer Center
- Ana Maria Gonzalez-Angulo
- , Mary D. Dyer
- , Shuying Liu
- , Xiaolong Meng
- & Modesto Patangan
University of California San Francisco
- Frederic Waldman
- & Hubert Stöppler
University of North Carolina
- W. Kimryn Rathmell
- , Leigh Thorne
- , Mei Huang
- , Lori Boice
- & Ashley Hill
Roswell Park Cancer Institute
- Carl Morrison
- , Carmelo Gaudioso
- & Wiam Bshara
University of Miami
- Kelly Daily
- , Sophie C. Egea
- , Mark D. Pegram
- & Carmen Gomez-Fernandez
University of Pittsburgh
- , Rohit Bhargava
- & Adam Brufsky
Walter Reed National Military Medical Center
- Craig D. Shriver
- , Jeffrey A. Hooke
- , Jamie Leigh Campbell
- , Richard J. Mural
- , Stella Somiari
- , Caroline Larson
- , Brenda Deyarmin
- , Leonid Kvecher
- & Albert J. Kovatich
Disease working group
- Matthew J. Ellis
- , Tari A. King
- , Thomas Stricker
- , Kevin White
- , Olufunmilayo Olopade
- , James N. Ingle
- , Chunqing Luo
- , Yaqin Chen
- , Jeffrey R. Marks
- , Frederic Waldman
- , Maciej Wiznerowicz
- , Li-Wei Chang
- , Andrew H. Beck
- & Ana Maria Gonzalez-Angulo
Data coordination centre
- , Mark Jensen
- , Robert Sfeir
- , Prachi Kothiyal
- , Zhining Wang
- , Eric Snyder
- , Joan Pontius
- , Brenda Ayala
- , Mark Backus
- , Jessica Walton
- , Julien Baboud
- , Dominique Berton
- , Matthew Nicholls
- , Deepak Srinivasan
- , Rohini Raman
- , Stanley Girshik
- , Peter Kigonya
- , Shelley Alonso
- , Rashmi Sanbhadti
- , Sean Barletta
- & David Pot
Project team: National Cancer Institute
- Margi Sheth
- , John A. Demchok
- , Kenna R. Mills Shaw
- , Liming Yang
- , Greg Eley
- , Martin L. Ferguson
- , Roy W. Tarnuzzer
- , Jiashan Zhang
- , Laura A. L. Dillon
- , Kenneth Buetow
- & Peter Fielding
National Human Genome Research Institute
- Bradley A. Ozenberger
- , Mark S. Guyer
- , Heidi J. Sofia
- & Jacqueline D. Palchik
Contributions
TCGA research network contributed collectively to this study. Biospecimens were provided by tissue source sites and processed by a Biospecimens Core Resource. Data generation and analyses were performed by genome sequencing centres, cancer genome characterization centres, and genome data analysis centres. RPPA analysis was performed at the MD Anderson Cancer Center in association with the genome data analysis centre. All data were released through the Data Coordinating Center. Project activities were coordinated by NCI and NHGRI project teams. We also acknowledge the following TCGA investigators of the Breast Analysis Working Group who contributed substantially to the analysis and writing of this manuscript: Project leaders, C.M.P., M.J.E.; manuscript coordinator, C.M.P., K.A.H.; data coordinator, K.A.H.; analysis coordinator, C.M.P., K.A.H.; DNA sequence analysis, D.C.K., L.D.; mRNA microarray analysis; K.A.H., C.F.; miRNA sequence analysis, A.G.R., A.C.; DNA methylation analysis, S. Malik, S. Mahurkar, P.W.L.; copy number analysis; A.D.C., M.M.; protein analysis, W.L., R.G.W.V., G.B.M.; pathway/integrated analysis, C.J.C., C.Y., J.M.S., C.C.B., G.C., C.S., S.R., I.S.; biospecimen core resource, T.L., J.B., J.M.G.; pathology and clinical expertise, T.A.K., H.H., R.J.M., J.N.I., T.S., F.W.
Corresponding author
Correspondence to Charles M. Perou .
Ethics declarations
Competing interests.
Charles M. Perou and Matthew J. Ellis are inventors on patent filing for PAM50 and have equity interest in Bioclassifier LLC. Joel S. Parker is an inventor on patent filing for PAM50.
Additional information
All of the primary sequence files are deposited in CGHub ( https://cghub.ucsc.edu/ ); all other data including mutation annotation file are deposited at the Data Coordinating Center ( http://cancergenome.nih.gov/ ). Sample lists, data matrices and supporting data can be found at http://tcga-data.nci.nih.gov/docs/publications/brca_2012/ . The data can be explored via the ISB Regulome Explorer ( http://explorer.cancerregulome.org/ ) and the cBio Cancer Genomics Portal ( http://cbioportal.org ). Data descriptions can be found at https://wiki.nci.nih.gov/display/TCGA/TCGA+Data+Primer and in Supplementary Methods.
Supplementary information
Supplementary information.
This file contains Supplementary Figures 1-20, Supplementary Methods 1-15 (with additional figures and tables) and Supplementary References. (PDF 14439 kb)
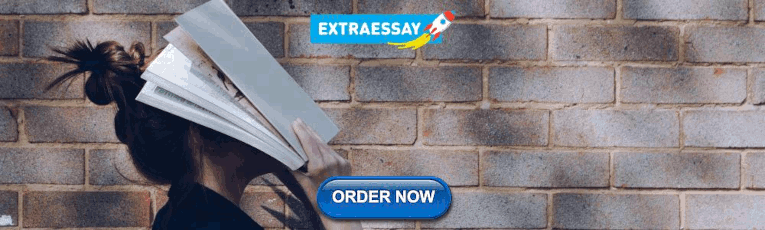
Supplementary Tables
This zipped file contains Supplementary Tables 1-8. This file was replaced on 15 November 2012 to correct an error in Supplementary Table 5 . (ZIP 1009 kb)
PowerPoint slides
Powerpoint slide for fig. 1, powerpoint slide for fig. 2, powerpoint slide for fig. 3, powerpoint slide for fig. 4, powerpoint slide for fig. 5, rights and permissions.
This article is distributed under the terms of the Creative Commons Attribution-Non-Commercial-Share Alike licence ( http://creativecommons.org/licenses/by-nc-sa/3.0/ ).
Reprints and permissions
About this article
Cite this article.
The Cancer Genome Atlas Network. Comprehensive molecular portraits of human breast tumours. Nature 490 , 61–70 (2012). https://doi.org/10.1038/nature11412
Download citation
Received : 22 March 2012
Accepted : 11 July 2012
Published : 23 September 2012
Issue Date : 04 October 2012
DOI : https://doi.org/10.1038/nature11412
Share this article
Anyone you share the following link with will be able to read this content:
Sorry, a shareable link is not currently available for this article.
Provided by the Springer Nature SharedIt content-sharing initiative
This article is cited by
Feature-specific quantile normalization and feature-specific mean–variance normalization deliver robust bi-directional classification and feature selection performance between microarray and rnaseq data.
- Daniel Skubleny
- Sunita Ghosh
- Gina R. Rayat
BMC Bioinformatics (2024)
Clustering of HR + /HER2− breast cancer in an Asian cohort is driven by immune phenotypes
- Jia-Wern Pan
- Mohana Ragu
- Soo-Hwang Teo
Breast Cancer Research (2024)
The impact of lipidome on breast cancer: a Mendelian randomization study
- Chunjun Liu
Lipids in Health and Disease (2024)
Basal–epithelial subpopulations underlie and predict chemotherapy resistance in triple-negative breast cancer
- Mohammed Inayatullah
- Arun Mahesh
- Vijay K Tiwari
EMBO Molecular Medicine (2024)
Mutational landscape of HSP family on human breast cancer
- Juan Manuel Fernandez-Muñoz
- Martin Eduardo Guerrero-Gimenez
- Felipe Carlos Martin Zoppino
Scientific Reports (2024)
By submitting a comment you agree to abide by our Terms and Community Guidelines . If you find something abusive or that does not comply with our terms or guidelines please flag it as inappropriate.
Quick links
- Explore articles by subject
- Guide to authors
- Editorial policies
Sign up for the Nature Briefing: Cancer newsletter — what matters in cancer research, free to your inbox weekly.

- See us on facebook
- See us on twitter
- See us on youtube
- See us on linkedin
- See us on instagram
Gene variants foretell the biology of future breast cancers in Stanford Medicine study
In a finding that vastly expands the understanding of tumor evolution, researchers discover genetic biomarkers that can predict the breast cancer subtype a patient is likely to develop.
May 30, 2024 - By Krista Conger

Stanford Medicine researchers found that inherited gene sequences can predict what type of breast cancer a patient is likely to develop, along with how aggressive that cancer may be. Emily Moskal
A Stanford Medicine study of thousands of breast cancers has found that the gene sequences we inherit at conception are powerful predictors of the breast cancer type we might develop decades later and how deadly it might be.
The study challenges the dogma that most cancers arise as the result of random mutations that accumulate during our lifetimes. Instead, it points to the active involvement of gene sequences we inherit from our parents — what’s known as your germline genome — in determining whether cells bearing potential cancer-causing mutations are recognized and eliminated by the immune system or skitter under the radar to become nascent cancers.
“Apart from a few highly penetrant genes that confer significant cancer risk, the role of hereditary factors remains poorly understood, and most malignancies are assumed to result from random errors during cell division or bad luck,” said Christina Curtis , PhD, the RZ Cao Professor of Medicine and a professor of genetics and of biomedical data science. “This would imply that tumor initiation is random, but that is not what we observe. Rather, we find that the path to tumor development is constrained by hereditary factors and immunity. This new result unearths a new class of biomarkers to forecast tumor progression and an entirely new way of understanding breast cancer origins.”
Curtis is the senior author of the study, which will be published May 31 in Science . Postdoctoral scholar Kathleen Houlahan , PhD, is the lead author of the research.
“Back in 2015, we had posited that some tumors are ‘born to be bad’ — meaning that their malignant and even metastatic potential is determined early in the disease course,” Curtis said. “We and others have since corroborated this finding across multiple tumors, but these findings cast a whole new light on just how early this happens.”
A new take on cancer’s origin
The study, which gives a nuanced and powerful new understanding of the interplay between newly arisen cancer cells and the immune system, is likely to help researchers and clinicians better predict and combat breast tumors.
Currently, only a few high-profile cancer-associated mutations in genes are regularly used to predict cancers, but these account for a small minority of cases. Those include BRCA1 and BRCA2, which occur in about one of every 500 women and confer an increased risk of breast or ovarian cancer, and rarer mutations in a gene called TP53 that causes a disease called Li Fraumeni syndrome, which predisposes to childhood and adult-onset tumors.

Christina Curtis
The findings suggest there are tens or hundreds of additional gene variants — identifiable in healthy people — that through interactions with the immune system pull the strings that determine why some people remain cancer-free throughout their lives.
“Our findings not only explain which subtype of breast cancer an individual is likely to develop,” Houlahan said, “but they also hint at how aggressive and prone to metastasizing that subtype will be. Beyond that, we speculate that these inherited variants may influence a person’s risk of developing breast cancer. However, future studies will be needed to examine this.”
The genes we inherit from our parents are known as our germline genome. They’re mirrors of our parents’ genetic makeup, and they can vary among people in small ways that give some of us blue eyes, brown hair or type O blood. Some inherited genes include mutations that confer increased cancer risk from the get-go, such as BRCA1, BRCA2 and TP53.
In contrast, most cancer-associated genes are part of what’s known as our somatic genome. As we live our lives, our cells divide and die in the tens of millions. Each time the DNA in a cell is copied, mistakes happen and mutations can accumulate. DNA in tumors is often compared with the germline genomes in blood or normal tissues in an individual to pinpoint which changes likely led to the cell’s cancerous transformation.
Classifying breast cancers
In 2012, Curtis began a deep dive — assisted by machine learning — into the types of somatic mutations that occur in thousands of breast cancers. She was eventually able to categorize the disease into 11 subtypes with varying prognoses and risk of recurrence, finding that four of the 11 groups were significantly more likely to recur even 10 or 20 years after diagnosis — critical information for clinicians making treatment decisions and discussing long-term prognoses with their patients.
Prior studies had shown that people with inherited BRCA1 mutations tend to develop a subtype of breast cancer known as triple negative breast cancer. This correlation implies some behind-the-scenes shenanigans by the germline genome that affects what subtype of breast cancer someone might develop.
“We wanted to understand how inherited DNA might sculpt how a tumor evolves,” Houlahan said. To do so, they took a close look at the immune system.
It’s a quirk of biology that even healthy cells routinely decorate their outer membranes with small chunks of the proteins they have bobbing in their cytoplasm — an outward display that reflects their inner style.
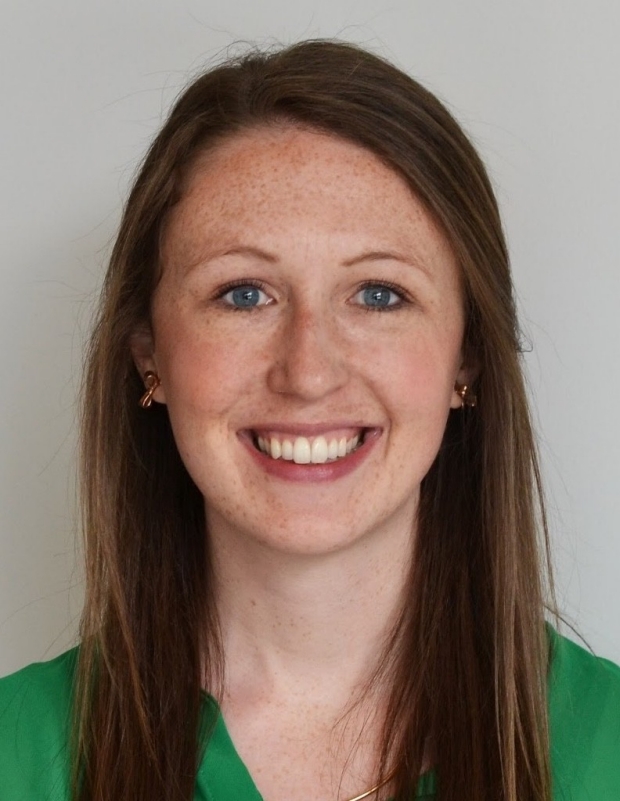
Kathleen Houlahan
The foundations for this display are what’s known as HLA proteins, and they are highly variable among individuals. Like fashion police, immune cells called T cells prowl the body looking for any suspicious or overly flashy bling (called epitopes) that might signal something is amiss inside the cell. A cell infected with a virus will display bits of viral proteins; a sick or cancerous cell will adorn itself with abnormal proteins. These faux pas trigger the T cells to destroy the offenders.
Houlahan and Curtis decided to focus on oncogenes, normal genes that, when mutated, can free a cell from regulatory pathways meant to keep it on the straight and narrow. Often, these mutations take the form of multiple copies of the normal gene, arranged nose to tail along the DNA — the result of a kind of genomic stutter called amplification. Amplifications in specific oncogenes drive different cancer pathways and were used to differentiate one breast cancer subtype from another in Curtis’ original studies.
The importance of bling
The researchers wondered whether highly recognizable epitopes would be more likely to attract T cells’ attention than other, more modest displays (think golf-ball-sized, dangly turquoise earrings versus a simple silver stud). If so, a cell that had inherited a flashy version of an oncogene might be less able to pull off its amplification without alerting the immune system than a cell with a more modest version of the same gene. (One pair of overly gaudy turquoise earrings can be excused; five pairs might cause a patrolling fashionista T cell to switch from tutting to terminating.)
The researchers studied nearly 6,000 breast tumors spanning various stages of disease to learn whether the subtype of each tumor correlated with the patients’ germline oncogene sequences. They found that people who had inherited an oncogene with a high germline epitope burden (read: lots of bling) — and an HLA type that can display that epitope prominently — were significantly less likely to develop breast cancer subtypes in which that oncogene is amplified.
There was a surprise, though. The researchers found that cancers with a large germline epitope burden that manage to escape the roving immune cells early in their development tended to be more aggressive and have a poorer prognosis than their more subdued peers.
“At the early, pre-invasive stage, a high germline epitope burden is protective against cancer,” Houlahan said. “But once it’s been forced to wrestle with the immune system and come up with mechanisms to overcome it, tumors with high germline epitope burden are more aggressive and prone to metastasis. The pattern flips during tumor progression.”
“Basically, there is a tug of war between tumor and immune cells,” Curtis said. “In the preinvasive setting, the nascent tumor may initially be more susceptible to immune surveillance and destruction. Indeed, many tumors are likely eliminated in this manner and go unnoticed. However, the immune system does not always win. Some tumor cells may not be eliminated and those that persist develop ways to evade immune recognition and destruction. Our findings shed light on this opaque process and may inform the optimal timing of therapeutic intervention, as well as how to make an immunologically cold tumor become hot, rendering it more sensitive to therapy.”
The researchers envision a future when the germline genome is used to further stratify the 11 breast cancer subtypes identified by Curtis to guide treatment decisions and improve prognoses and monitoring for recurrence. The study’s findings may also give additional clues in the hunt for personalized cancer immunotherapies and may enable clinicians to one day predict a healthy person’s risk of developing an invasive breast cancer from a simple blood sample.
“We started with a bold hypothesis,” Curtis said. “The field had not thought about tumor origins and evolution in this way. We’re examining other cancers through this new lens of hereditary and acquired factors and tumor-immune co-evolution.”
The study was funded by the National Institutes of Health (grants DP1-CA238296 and U54CA261719), the Canadian Institutes of Health Research and the Chan Zuckerberg Biohub.
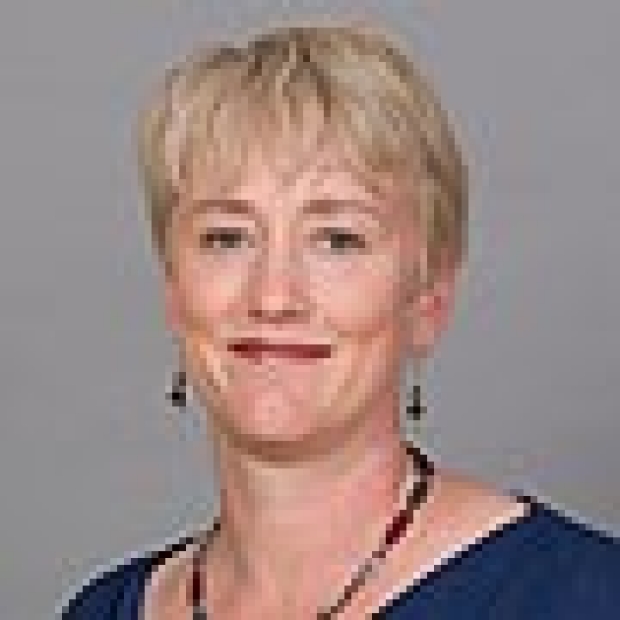
About Stanford Medicine
Stanford Medicine is an integrated academic health system comprising the Stanford School of Medicine and adult and pediatric health care delivery systems. Together, they harness the full potential of biomedicine through collaborative research, education and clinical care for patients. For more information, please visit med.stanford.edu .
Hope amid crisis
Psychiatry’s new frontiers
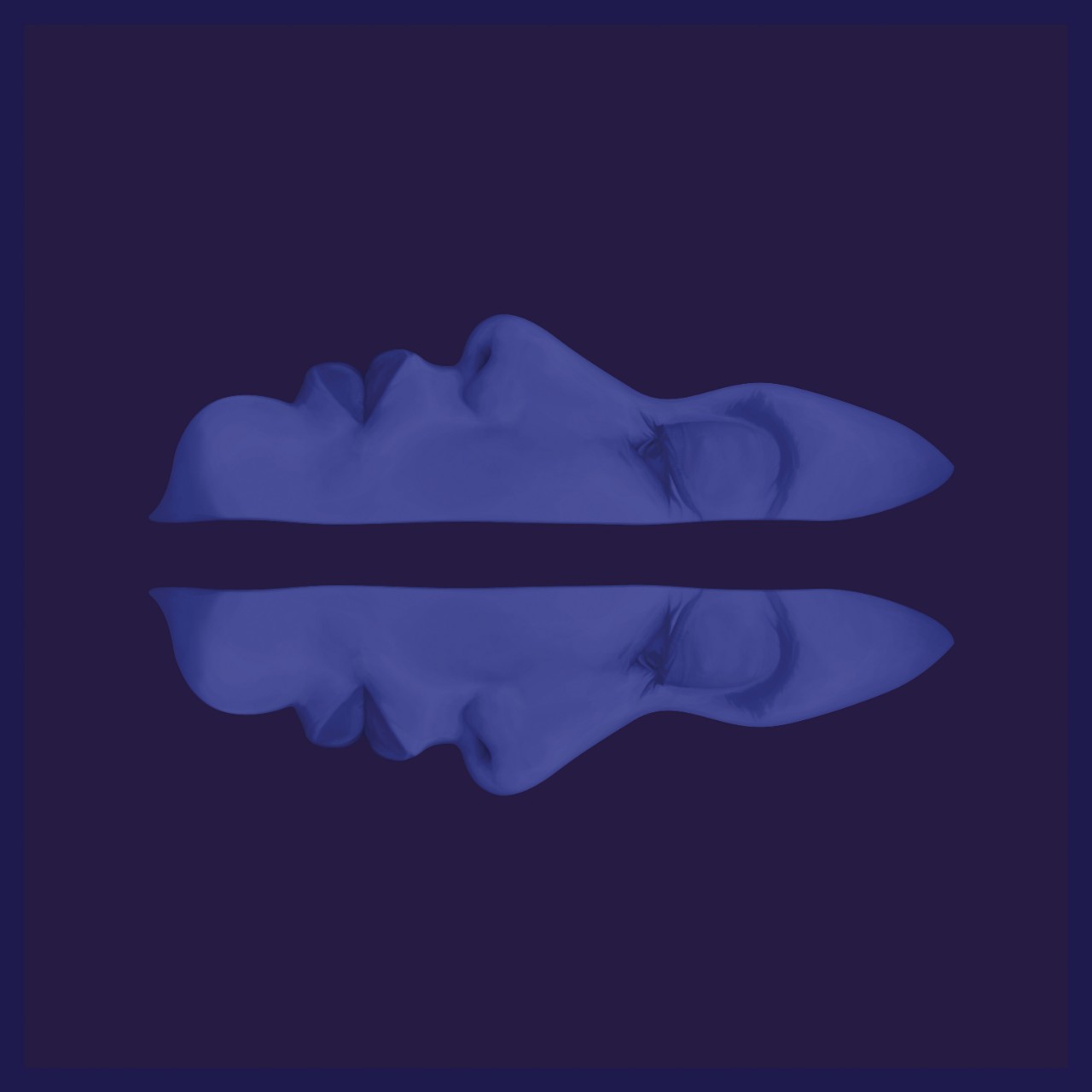

Gene variants foretell the biology of future breast cancers
A Stanford Medicine study of thousands of breast cancers has found that the gene sequences we inherit at conception are powerful predictors of the breast cancer type we might develop decades later and how deadly it might be.
The study challenges the dogma that most cancers arise as the result of random mutations that accumulate during our lifetimes. Instead, it points to the active involvement of gene sequences we inherit from our parents -- what's known as your germline genome -- in determining whether cells bearing potential cancer-causing mutations are recognized and eliminated by the immune system or skitter under the radar to become nascent cancers.
"Apart from a few highly penetrant genes that confer significant cancer risk, the role of heredity factors remains poorly understood, and most malignancies are assumed to result from random errors during cell division or bad luck," said Christina Curtis, PhD, the RZ Cao Professor of Medicine and a professor of genetics and of biomedical data science. "This would imply that tumor initiation is random, but that is not what we observe. Rather, we find that the path to tumor development is constrained by hereditary factors and immunity. This new result unearths a new class of biomarkers to forecast tumor progression and an entirely new way of understanding breast cancer origins."
Curtis is the senior author of the study, which will be published May 31 in Science . Postdoctoral scholar Kathleen Houlahan, PhD, is the lead author of the research.
"Back in 2015, we had posited that some tumors are 'born to be bad' -- meaning that their malignant and even metastatic potential is determined early in the disease course," Curtis said. "We and others have since corroborated this finding across multiple tumors, but these findings cast a whole new light on just how early this happens."
A new take on cancer's origin
The study, which gives a nuanced and powerful new understanding of the interplay between newly arisen cancer cells and the immune system, is likely to help researchers and clinicians better predict and combat breast tumors.
Currently, only a few high-profile cancer-associated mutations in genes are regularly used to predict cancers. Those include BRCA1 and BRCA2, which occur in about one of every 500 women and confer an increased risk of breast or ovarian cancer, and rarer mutations in a gene called TP53 that causes a disease called Li Fraumeni syndrome, which predisposes to childhood and adult-onset tumors.
The findings indicate there are tens or hundreds of additional gene variants -- identifiable in healthy people -- pulling the strings that determine why some people remain cancer-free throughout their lives.
"Our findings not only explain which subtype of breast cancer an individual is likely to develop," Houlahan said, "but they also hint at how aggressive and prone to metastasizing that subtype will be. Beyond that, we anticipate that these inherited variants may influence a person's risk of developing breast cancer."
The genes we inherit from our parents are known as our germline genome. They're mirrors of our parents' genetic makeup, and they can vary among people in small ways that give some of us blue eyes, brown hair or type O blood. Some inherited genes include mutations that confer increased cancer risk from the get-go, such as BRCA1, BRCA2 and TP53. But identifying other germline mutations strongly associated with future cancers has proven difficult.
In contrast, most cancer-associated genes are part of what's known as our somatic genome. As we live our lives, our cells divide and die in the tens of millions. Each time the DNA in a cell is copied, mistakes happen and mutations can accumulate. DNA in tumors is often compared with the germline genomes in blood or normal tissues in an individual to pinpoint which changes likely led to the cell's cancerous transformation.
Classifying breast cancers
In 2012, Curtis began a deep dive -- assisted by machine learning -- into the types of somatic mutations that occur in thousands of breast cancers. She was eventually able to categorize the disease into 11 subtypes with varying prognoses and risk of recurrence, finding that four of the 11 groups were significantly more likely to recur even 10 or 20 years after diagnosis -- critical information for clinicians making treatment decisions and discussing long-term prognoses with their patients.
Prior studies had shown that people with inherited BRCA1 or BRCA2 mutations tend to develop a subtype of breast cancer known as triple negative breast cancer. This correlation implies some behind-the-scenes shenanigans by the germline genome that affects what subtype of breast cancer someone might develop.
"We wanted to understand how inherited DNA might sculpt how a tumor evolves," Houlahan said. To do so, they took a close look at the immune system.
It's a quirk of biology that even healthy cells routinely decorate their outer membranes with small chunks of the proteins they have bobbing in their cytoplasm -- an outward display that reflects their inner style.
The foundations for this display are what's known as HLA proteins, and they are highly variable among individuals. Like fashion police, immune cells called T cells prowl the body looking for any suspicious or overly flashy bling (called epitopes) that might signal something is amiss inside the cell. A cell infected with a virus will display bits of viral proteins; a sick or cancerous cell will adorn itself with abnormal proteins. These faux pas trigger the T cells to destroy the offenders.
Houlahan and Curtis decided to focus on oncogenes, normal genes that, when mutated, can free a cell from regulatory pathways meant to keep it on the straight and narrow. Often, these mutations take the form of multiple copies of the normal gene, arranged nose to tail along the DNA -- the result of a kind of genomic stutter called amplification. Amplifications in specific oncogenes drive different cancer pathways and were used to differentiate one breast cancer subtype from another in Curtis' original studies.
The importance of bling
The researchers wondered whether highly recognizable epitopes would be more likely to attract T cells' attention than other, more modest displays (think golf-ball-sized, dangly turquoise earrings versus a simple silver stud). If so, a cell that had inherited a flashy version of an oncogene might be less able to pull off its amplification without alerting the immune system than a cell with a more modest version of the same gene. (One pair of overly gaudy turquoise earrings can be excused; five pairs might cause a patrolling fashionista T cell to switch from tutting to terminating.)
The researchers studied nearly 6,000 breast tumors spanning various stages of disease to learn whether the subtype of each tumor correlated with the patients' germline oncogene sequences. They found that people who had inherited an oncogene with a high germline epitope burden (read: lots of bling) -- and an HLA type that can display that epitope prominently -- were significantly less likely to develop breast cancer subtypes in which that oncogene is amplified.
There was a surprise, though. The researchers found that cancers with a large germline epitope burden that manage to escape the roving immune cells early in their development tended to be more aggressive and have a poorer prognosis than their more subdued peers.
"At the early, pre-invasive stage, a high germline epitope burden is protective against cancer," Houlahan said. "But once it's been forced to wrestle with the immune system and come up with mechanisms to overcome it, tumors with high germline epitope burden are more aggressive and prone to metastasis. The pattern flips during tumor progression."
"Basically, there is a tug of war between tumor and immune cells," Curtis said. "In the preinvasive setting, the nascent tumor may initially be more susceptible to immune surveillance and destruction. Indeed, many tumors are likely eliminated in this manner and go unnoticed. However, the immune system does not always win. Some tumor cells may not be eliminated and those that persist develop ways to evade immune recognition and destruction. Our findings shed light on this opaque process and may inform the optimal timing of therapeutic intervention, as well as how to make an immunologically cold tumor become hot, rendering it more sensitive to therapy."
The researchers envision a future when the germline genome is used to further stratify the 11 breast cancer subtypes identified by Curtis to guide treatment decisions and improve prognoses and monitoring for recurrence. The study's findings may also give additional clues in the hunt for personalized cancer immunotherapies and may enable clinicians to one day predict a healthy person's risk of cancer from a simple blood sample.
"We started with a bold hypothesis," Curtis said. "The field had not thought about tumor origins and evolution in this way. We're examining other cancers through this new lens of heredity and acquired factors and tumor-immune co-evolution."
The study was funded by the National Institutes of Health (grants DP1-CA238296 and U54CA261719), the Canadian Institutes of Health Research and the Chan Zuckerberg Biohub.
- Breast Cancer
- Brain Tumor
- Lung Cancer
- Colon Cancer
- Diseases and Conditions
- Ovarian Cancer
- Breast cancer
- Monoclonal antibody therapy
- Mammography
- Breast implant
- Colorectal cancer
- Breast reconstruction
Story Source:
Materials provided by Stanford Medicine . Original written by Krista Conger. Note: Content may be edited for style and length.
Journal Reference :
- Kathleen E. Houlahan, Aziz Khan, Noah F. Greenwald, Cristina Sotomayor Vivas, Robert B. West, Michael Angelo, Christina Curtis. Germline-mediated immunoediting sculpts breast cancer subtypes and metastatic proclivity . Science , 2024; 384 (6699) DOI: 10.1126/science.adh8697
Cite This Page :
Explore More
- Giant Viruses Found On Greenland Ice Sheet
- Using AI to Decode Dog Vocalizations
- Humans and Woolly Rhinoceros' Extinction
- More Summer Droughts for Northern Hemisphere
- All Electrical Needs from Floating Solar Panels?
- Over 60% of US People Likely to Have CVD by 2050
- Gigantic Jurassic Pterosaur Fossil Unearthed
- Bringing Back an Ancient Bird
- How Quantum Field Theories Decay and Fission
- River Nile's Evolution During Ancient Egypt
Trending Topics
Strange & offbeat.
- About Breast Cancer
- Find Support
- Get Involved
- Free Resources
- Mammogram Pledge
- Wall of Support
- In The News
- Recursos en Espa ñ ol
About Breast Cancer > What is Breast Cancer? > Breast Cancer Genetics > BRCA: The Breast Cancer Gene
- What Is Cancer?
- Causes of Breast Cancer
- Breast Cancer Facts & Stats
- Breast Tumors
- Breast Anatomy
- Male Breast Cancer
- Growth of Cancer
- Risk Factors
- Genetic Testing for Breast Cancer
- Other Breast Cancer Genes
BRCA: The Breast Cancer Gene
- What To Do If You Tested Positive
- Breast Cancer Signs and Symptoms
- Breast Lump
- Breast Pain
- Breast Cyst
- Breast Self-Exam
- Clinical Breast Exam
- How to Schedule a Mammogram
- Healthy Habits
- Breast Cancer Screening
- Diagnostic Mammogram
- Breast Biopsy
- Waiting For Results
- Breast Cancer Stages
- Stage 2 (II) And Stage 2A (IIA)
- Stage 3 (III) A, B, And C
- Stage 4 (IV) Breast Cancer
- Ductal Carcinoma In Situ (DCIS)
- Invasive Ductal Carcinoma (IDC)
- Lobular Carcinoma In Situ (LCIS)
- Invasive Lobular Cancer (ILC)
- Triple Negative Breast Cancer (TNBC)
- Inflammatory Breast Cancer (IBC)
- Metastatic Breast Cancer (MBC)
- Breast Cancer During Pregnancy
- Other Types
- Choosing Your Doctor
- Lymph Node Removal & Lymphedema
- Breast Reconstruction
- Chemotherapy
- Radiation Therapy
- Hormone Therapy
- Targeted Therapy
- Side Effects of Breast Cancer Treatment and How to Manage Them
- Metastatic Breast Cancer Trial Search
- Standard Treatment vs. Clinical Trials
- Physical Activity, Wellness & Nutrition
- Bone Health Guide for Breast Cancer Survivors
- Follow-Up Care
- Myth: Finding a lump in your breast means you have breast cancer
- Myth: Men do not get breast cancer; it affects women only
- Myth: A mammogram can cause breast cancer or spread it
- Myth: If you have a family history of breast cancer, you are likely to develop breast cancer, too
- Myth: Breast cancer is contagious
- Myth: If the gene mutation BRCA1 or BRCA2 is detected in your DNA, you will definitely develop breast cancer
- Myth: Antiperspirants and deodorants cause breast cancer
- Myth: A breast injury can cause breast cancer
- Myth: Breast cancer is more common in women with bigger breasts
- Myth: Breast cancer only affects middle-aged or older women
- Myth: Breast pain is a definite sign of breast cancer
- Myth: Consuming sugar causes breast cancer
- Myth: Carrying a phone in your bra can cause breast cancer
- Myth: All breast cancers are the same
- Myth: Bras with underwire can cause breast cancer
- Can physical activity reduce the risk of breast cancer?
- Can a healthy diet help to prevent breast cancer?
- Does smoking cause breast cancer?
- Can drinking alcohol increase the risk of breast cancer?
- Is there a link between oral contraceptives and breast cancer?
- Is there a link between hormone replacement therapy (HRT) and breast cancer?
- How often should I do a breast self exam (BSE)?
- Does a family history of breast cancer put someone at a higher risk?
- Are mammograms painful?
- How does menstrual and reproductive history affect breast cancer risks?
- How often should I go to my doctor for a check-up?
- What kind of impact does stress have on breast cancer?
- What celebrities have or have had breast cancer?
- Where can I find a breast cancer support group?
- Can breastfeeding reduce the risk of breast cancer?
- Is dairy (milk) linked to a higher risk of breast cancer?
- Is hair dye linked to a higher risk of breast cancer?
- NEW! Just Diagnosed with Breast Cancer… Now What?
- Smart Bites Cookbook: 7 Wholesome Recipes in 35 Minutes (or Less!)
- Weekly Healthy Living Tips: Volume 2
- Most Asked Questions: Breast Cancer Signs & Symptoms
- Cancer Caregiver Guide
- Breast Cancer Surgery eBook
- 10 Prompts to Mindfulness
- How to Talk About Breast Health
- Family Medical History Checklist
- Healthy Recipes for Cancer Patients eBook
- Chemo Messages
- Most Asked Questions About Breast Cancer Recurrence
- Breast Problems That Arent Breast Cancer eBook
- Nutrition Care for Breast Cancer Patients eBook
- Finding Hope that Heals eBook
- Dense Breasts Q&A Guide
- Breast Cancer Recurrence eBook
- What to Say to a Cancer Patient eBook
- Weekly Healthy Living Tips
- Bra Fit Guide
- Know the Symptoms Guide
- Breast Health Guide
- Mammogram 101 eBook
- 3 Steps to Early Detection Guide
- Abnormal Mammogram eBook
- Healthy Living & Personal Risk Guide
- What Every Woman Needs to Know eBook
- Breast Cancer Resources
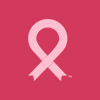
Last updated on Jan 17, 2024
What Is A Gene?
Each person’s DNA contains the code used to build the human body and keep it functioning. Genes are the small sections of DNA that code for individual traits. For example, someone with naturally red hair has a gene that causes his or her hair to be red.
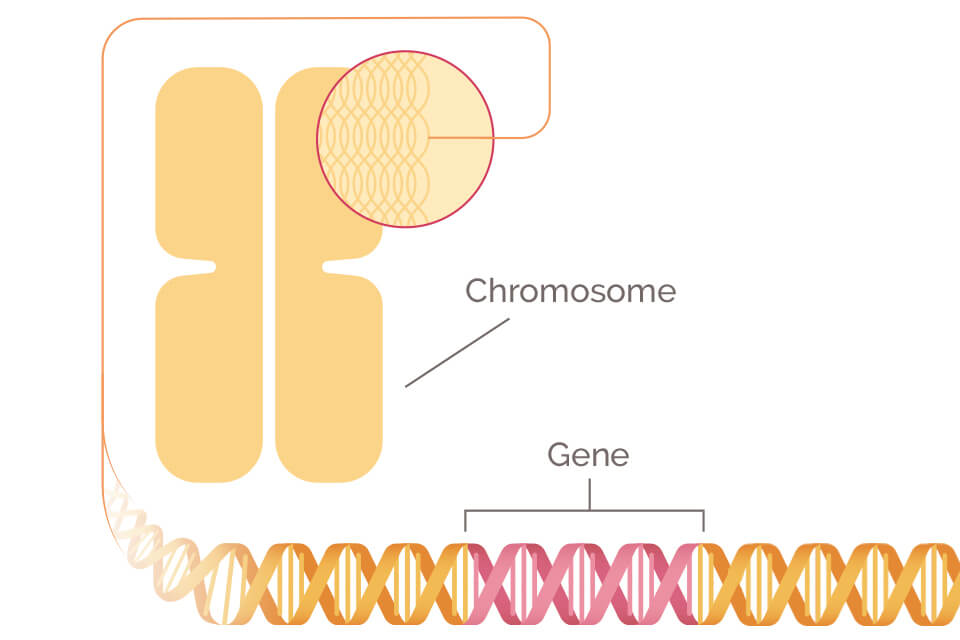
All inherited traits are passed down through genes. Each person has two copies of every gene: one gene from each parent. Since each parent passes down exactly half of their genes to each child, any of the parent’s genetic traits has a 50% chance of being passed on to their offspring.
What Is BRCA?
The name “BRCA” is an abbreviation for “BReast CAncer gene.” BRCA1 and BRCA2 are two different genes that have been found to impact a person’s chances of developing breast cancer.
Every human has both the BRCA1 and BRCA2 genes. Despite what their names might suggest, BRCA genes do not cause breast cancer. In fact, these genes normally play a big role in preventing breast cancer. They help repair DNA breaks that can lead to cancer and the uncontrolled growth of tumors . Because of this, the BRCA genes are known as tumor suppressor genes.
However, in some people these tumor suppression genes do not work properly. When a gene becomes altered or broken, it doesn’t function correctly. This is called a gene mutation.
BRCA Mutations
A small percentage of people (about one in 400, or 0.25% of the population) carry mutated BRCA1 or BRCA2 genes. A BRCA mutation occurs when the DNA that makes up the gene becomes damaged in some way.
When a BRCA gene is mutated, it may no longer be effective at repairing broken DNA and helping to prevent breast cancer. Because of this, people with a BRCA gene mutation are more likely to develop breast cancer, and more likely to develop cancer at a younger age. The carrier of the mutated gene can also pass a gene mutation down to his or her offspring.
BRCA Mutation Risks
It is estimated that one in eight women, or approximately 12%, will be diagnosed with breast cancer in her lifetime.
However, women with certain genetic mutations have a higher lifetime risk of the disease. It’s estimated that 55 – 65% of women with the BRCA1 mutation will develop breast cancer before age 70.
Approximately 45% of women with a BRCA2 mutation will develop breast cancer by age 70.
Women with a BRCA1 or BRCA2 mutation who overcome their breast cancer with treatment appear to have a higher-than-average chance of developing a second cancer. This is called a recurrence. Cancers related to a BRCA1 mutation are also more likely to be triple negative breast cancer , which can be more aggressive and difficult to treat.
You may find these statistics alarming. However, it’s important to note that less than 10% of women diagnosed with breast cancer have a BRCA mutation. Also, with early detection , the vast majority of breast cancer cases can be successfully treated—and that’s true even for people who have a BRCA1 or BRCA2 mutation.
Reducing Risks Associated with BRCA Gene Mutations
If you discover you have a BRCA1 or BRCA2 gene mutation, there are preventative measures you can take to help reduce your risk of developing breast cancer in the future. One of these measures may be taking a form of hormone therapy , such as tamoxifen. Another preventative measure may include taking a surgical prevention approach, such as a bilateral prophylactic mastectomy. This involves removing the breast tissue as a preventative measure, before cancer develops, and is usually done along with breast reconstruction. Some women may also opt to have their ovaries and fallopian tubes removed since BRCA gene mutations increase the risk of developing ovarian cancer as well. However, you should discuss all options available to you, and the benefits and risks of each, with your healthcare provider.
Related reading:
- What To Do If You’ve Tested Positive
We use cookies on our website to personalize your experience and improve our efforts. By continuing, you agree to the terms of our Privacy & Cookies Policies.
- Health Conditions
- Health Products
Researchers identify new genes linked to breast cancer

- Researchers analyzed genetic data to identify new genes linked to breast cancer.
- They found several new genes that may be linked to the condition.
- Further research is needed to know how variants on these genes affect breast cancer risk.
Breast cancer is the most common cancer globally, accounting for around 12.5% of new cancer cases worldwide. Genetic testing can be used to assess risk for the condition. Current tests consider risk variants on a small number of genes, including BRCA1 , BRCA2 , and PALB2 .
However, known variants explain less than half of the familial relative risk of breast cancer, which is the probability of developing a condition if a family member has had it before. How much rare coding variants in other genes account for breast cancer risk remains largely unknown.
Understanding more about different breast cancer genes could improve the accuracy of genetic testing for predicting breast cancer risk.
Recently, researchers analyzed genetic data from 244,041 women to identify new gene variants linked to breast cancer. They found evidence for several new breast cancer risk genes and potential evidence for others.
The study was published in Nature Genetics .
“The study helps to identify additional genes that could be inherited and increase risk or explain family history of breast cancer. This becomes the launching pad for the next generation of data to help explain for the nearly 50% of individuals with family history that is not currently explained with genetics that we have available.” — Dr. Louise Morrell, medical director of Lynn Cancer Institute, part of Baptist Health at Boca Raton Regional Hospital, who was not involved in the study, speaking to Medical News Today .
40 genes linked to breast cancer
For the study, the researchers analyzed genetic data from 26,368 women with breast cancer and 217,673 without. The women were primarily of European ancestry, although the researchers included some data from Malaysia and Singapore as well.
After analyzing the data, they identified 30 genes linked to breast cancer, of which six were particularly significant. These included five known susceptibility genes as well as one new gene: MAP3K1 .
When the researchers restricted their analysis to patients ages 50 years and younger, they identified 40 genes linked to breast cancer.
“Although most of the variants identified in these new genes are rare, the risks can be significant for women who carry them. For example, alterations in one of the new genes, MAP3K1 , appear to give rise to a particularly high risk of breast cancer,” said Dr. Jacques Simard, Ph.D. , professor of medicine at Québec-Université Laval Research Center, in a press release.
Breast cancer risk in ‘European’ populations
MNT asked Dr. Jessica Jones , assistant professor of oncology with McGovern Medical School at UTHealth Houston, who was not involved in the study, about its limitations.
She indicated that as the study predominantly included women from Europe, the findings may not apply to more diverse populations.
“For example, the article overlooks some important genes, such as STK11 and TP53 , because of how rare they are in Europe. They are not so rare here in the United States. These carry very high risks of cancer,” she noted.
She added that the study did not take into account how lifestyle factors such as obesity or alcohol consumption may impact breast cancer risk or the expression of genes.
MNT also spoke with Dr. Ora Karp Gordon , regional director of clinical genetics and genomics for Providence Southern California and Professor of Genetics at Saint John’s Cancer Institute in Santa Monica, California, who was also not involved in the study, about its limitations.
“The one significant new breast cancer susceptibility gene MAP3K1 is estimated to explain 0.14% of breast cancer risk, and all other genes combined [accounted for less than 1% of increased risk]. Thus, whether this approach can really lead to meaningful advancement from both a cost and data standpoint is a big unknown, [and perhaps unlikely],” said Dr. Gordon.
She added that the study only investigated coding regions of the genome and not other areas.
Genetic testing for breast cancer
“Despite more than ten years of utilizing next-generation sequencing techniques to investigate breast cancer susceptibility genes, more than 30% of the familial risk for breast cancer is still unidentified,” said Dr. Gordon.
She noted that this means current genetic testing cannot account for many cases of breast cancer.
“The majority of the missing ‘heritability’ of breast cancer risk may be in the non-coding genome and therefore only discoverable with whole genome sequencing, which remains cost-prohibitive at scale,” she added.
Dr. Jones noted that there is still a lot to learn about genes and that until they are ‘perfectly’ understood, genetic testing will not be offered to the entire population.
“A woman’s family history trumps this article. Regardless of whether we know a woman’s DNA make-up, if she has a strong family history [of breast cancer], she probably qualifies for enhanced breast cancer screening. If a woman reading this article wonders if her family has a genetic defect, science may need time to catch up with every gene—but she doesn’t have to wait to get more enhanced breast cancer screening.” — Dr. Jones
Will these genes be tested in screening?
“Confirming this approach [with other demographics and datasets could significantly improve breast cancer risk assessments] for the many women who have a personal or family history [of the condition] but have had negative genetic testing results,” said Dr. Gordon.
Meanwhile, Dr. Jones said, “The study sheds new light on breast cancer risk related to ATRIP , MAP3K1 , and SAMHD1 genes.”
“If a woman with breast cancer undergoes genetic testing and has [the risk variant], we can offer testing to her family to see if any children have also inherited [it]. If a child has inherited [a risk variant], we can change how we screen for breast cancer to include enhanced screening with breast MRI imaging,” she concluded.
- Breast Cancer
- Cancer / Oncology
Share this article
Latest news
- Alcohol-based mouthwash may disrupt oral microbiome, leading to gum disease and certain cancers
- GLP-1 receptor agonists: Are they friends or foes in colorectal cancer?
- Intermittent fasting plus protein pacing may boost weight loss, gut health
- Adding omega-3s to diet may help improve mood, reduce aggression
- HPV vaccine prevents cancer in males as well as females, researchers say
Related Coverage
Dr. Rachel Natrajan and Dr. Liz O'Riordan speak about breast cancer, discussing why cancer comes back, and what may influence recurrence risk.
October is National Breast Cancer Awareness Month, so this edition of Medical Myths focuses on common myths associated with breast cancer.
In 1972, I survived breast cancer only to receive a diagnosis of stage 4 ovarian cancer in the following decade. This is my story.
My name is Chris Fry, and I received a diagnosis of terminal cancer this year. This is how I came to terms with my diagnosis, and what helped me cope.
Get the facts on cost and Truqap, how generics compare with brand names, what financial assistance may be available, and more.
- Introduction to Genomics
- Educational Resources
- Policy Issues in Genomics
- The Human Genome Project
- Funding Opportunities
- Funded Programs & Projects
- Division and Program Directors
- Scientific Program Analysts
- Contact by Research Area
- News & Events
- Research Areas
- Research investigators
- Research Projects
- Clinical Research
- Data Tools & Resources
- Genomics & Medicine
- Family Health History
- For Patients & Families
- For Health Professionals
- Jobs at NHGRI
- Training at NHGRI
- Funding for Research Training
- Professional Development Programs
- NHGRI Culture
- Social Media
- Broadcast Media
- Image Gallery
- Press Resources
- Organization
- NHGRI Director
- Mission & Vision
- Policies & Guidance
- Institute Advisors
- Strategic Vision
- Leadership Initiatives
- Diversity, Equity, and Inclusion
- Partner with NHGRI
- Staff Search
Questions About the BRCA1 and BRCA2 Gene Study and Breast Cancer
What was the purpose of the study, what was unique about the current study, what is known about the brca1 and brca2 genes, why were these particular alterations chosen to be tested, what were the findings of the current study, how is inherited breast cancer different from other genetic diseases, are further studies planned with the jewish population in the washington d.c. area, are nih scientists planning any studies involving alterations in brca1 and brca2 genes that are unique to other ethnic groups, what are the implications of this study for non-jewish populations, do the results have implications for jews getting tested for these alterations, do these results have implications for the prevention or treatment of breast, ovarian or prostate cancer.
In 1995 scientists from the National Institutes of Health ( NIH ) discovered that a particular alteration in the breast cancer gene called BRCA1 was present in 1 percent of the general Jewish population. The researchers did a follow-up study in 1996 to estimate the cancer risk associated with this alteration as well as two other alterations subsequently reported to be present in the Ashkenazi Jewish population. The following questions and answers serve as background information for the follow-up study published in the May 15, 1997 issue of The New England Journal of Medicine .
The primary purpose of the study was to estimate the risk of cancer associated with having three specific alterations in the breast cancer genes, BRCA1 and BRCA2. The study was conducted in the Washington, D.C. Ashkenazi Jewish population (Jews from eastern or central Europe). Two of the alterations tested were in the BRCA1 gene (185delAG and 5382insC) and one in the BRCA2 gene (6174delT).
The researchers tested the DNA in blood provided by a finger-prick to see which of the more than 5,000 volunteers had an alteration. Then, using the family cancer histories reported by the volunteers, the scientists estimated the cancer risk by comparing the histories of cancer in the relatives of the volunteers with the alteration to the histories of cancer in the relatives of the volunteers without the alteration.
This was the first study to test directly the DNA from volunteers who are outside cancer-prone families and estimate the cancer risk associated with each alteration. For years, researchers have studied families with breast cancer throughout several generations to help identify the altered genes passed on from one generation to the next.
This was the first community-based study where people with varying degrees of family cancer history participated. In fact, three-quarters of the volunteers had no personal or close family history of breast or ovarian cancer and 30 percent were men. About 8 percent of the women were breast or ovarian cancer survivors.
Earlier, the scientists involved in the new study tested for one of the alterations (185delAG) in anonymous stored blood samples from the general Jewish population. Even though the frequencies they found were unexpectedly high (see references in question 4), it was impossible to estimate the cancer risk associated with the alterations because the cancer history of the blood donors was not known.
This study was designed both to test for the frequency of the alterations and to find out if volunteers from the general population with an alteration were at greater risk for cancer than those without an alteration.
Because family history is the strongest single predictor of a woman's chance of developing breast cancer, researchers turned to cancer-prone families - those with a high incidence of cancer in several generations - to find specific inherited gene alterations that are passed on from one generation to the next. After a long search, two genes were found that are altered in many families with hereditary breast cancer. The first, BRCA1 (for BReast CAncer gene), was discovered in 1994, and the second, BRCA2, in 1995. The search for other genes continues.
Within families with cancer in multiple generations, it had been estimated previously that a woman with an alteration in the BRCA1 gene has about an 85 percent chance of developing breast cancer and a 44 percent chance of developing ovarian cancer by age 70. Prior research in these high-risk families reported that women with BRCA2 alterations have a lower risk of developing both breast and ovarian cancer than women with BRCA1 alterations. Previous studies had reported an increased risk of colon and prostate cancer associated with alteration carriers in these same families.
Most alterations result in a shortened protein product which scientists assume prevents the protein from carrying out its normal function in the cell. The precise biological roles of BRCA1 and BRCA2 are not known.
Once the genes were isolated, it was possible to analyze the specific alterations inherited in each cancer-prone family. Today over 100 different alterations scattered throughout BRCA1 have been identified. In general, most families have a unique alteration. A similar pattern is emerging for BRCA2 alterations seen in cancer-prone families; a large number of distinct, family-specific alterations are scattered through the gene.
The initial impetus for the current study was the observation in late 1994 that three high-risk Ashkenazi families studied at the NIH carried an identical alteration in BRCA1 (185delAG). These families were not known to be related. This observation led to the study which found that 1 percent of the Jewish population has this alteration. This was the first alteration associated with a particular ethnic group. A few other alterations frequently occurring in other ethnic groups (Icelandic, Norweigan and Dutch) have been found since then and are now being studied.
Of the more than 100 alterations identified in each gene (BRCA1 and BRCA2) in families with hereditary breast cancer, a few are found in subgroups of the general population. In particular, three alterations were initially identified in Ashkenazi families with hereditary breast cancer and later were found in an unusually high percentage of the general Jewish population. The estimated frequencies of the three alterations in the general Ashkenazi population are listed below:
In comparison, the percentage of people in the general U.S. population that have any mutation in BRCA1 has been estimated to be between 0.1 - 0.6 percent.
* Nature Genetics 1995; 11: 198-200 and Nature Genetics 1996; 14: 185-187, 188-190.
- Supported previous studies testing the frequency of three BRCA1 and BRCA2 alterations in the general Jewish population: The frequencies reported in the current study are consistent with those previously reported for the general Jewish population. The DNA analysis in the new study showed that 120 of the 5,318 volunteers had one of the three alterations or about 1 person in 44 (2.3 percent). No individual carried more than one of the three alterations. By comparison, the frequency of all BRCA1 and BRCA2 alterations combined in the non-Jewish population is less than 1 percent.
- Estimated the average risk of breast and ovarian cancer associated with three BRCA1 and BRCA2 alterations in the general Ashkenazi population: The researchers found that women carrying one of the three alterations have on average a 56 percent chance of getting breast cancer by the age of 70 (compared with a 13 percent chance without the alterations) and a 16 percent chance of getting ovarian cancer by age 70 (compared with a 1.6 percent chance for non-carriers). In other words, the researchers estimate that by the age of 70, slightly more than half of all women with an alteration will develop breast cancer and about one out of every six carriers will develop ovarian cancer.
- Found breast and ovarian cancer risks well below previous estimates: Until now, small studies of families with cancer in several generations had estimated that women with an alteration had a 76 percent to 87 percent chance of developing breast cancer; for ovarian cancer, the estimated risk ranged from 11 percent to 84 percent.
- Confirmed the link between prostate cancer and the alterations: Previous studies had suggested a link between BRCA1 and prostate cancer. The current study confirmed this association and showed a significant excess of prostate cancer among men with the alterations.
- Estimated the prostate cancer risk in the general Jewish population: Men carrying one of the three alterations have on average a 16 percent chance of getting prostate cancer (compared with a 1.6 percent chance for non-carriers) by the age of 70. In other words, by age 70 the researchers estimate that about 1 out of every 6 men carrying an alteration will develop prostate cancer.
- Found the average risks for breast, ovarian, and prostate cancers: The study estimated the average risk of cancer for alteration carriers. The cancer risk for an individual man or woman who carries one of the alterations may be higher or lower than the average.
- Found no link with colon cancer: A previous report showed a link between BRCA1 alterations and colon cancer that was not confirmed in the current study. found that each alteration carries a similar breast cancer risk: Previous reports suggested that the risk of getting breast cancer was different for two of the alterations studied. Specifically, in studies involving Jewish early-onset breast cancer patients, data suggested that the risk associated with the 6174delT mutation (in BRCA2) was considerably lower than the risk associated with 185delAG. In the current study, the risk associated with the 6174delT was slightly lower, but the risks for the three alterations were not significantly different from each other. The study found that the three alterations account for only a small proportion of breast cancer cases in Jewish women: Of the women in this study who were breast or ovarian cancer survivors, only 9 percent had one of the alterations. In fact, only about 7 percent of breast cancer in Jewish women is due to the three alterations in BRCA1 and BRCA2.
On average, by the age of 70, women with one of the alterations tested for in this study have about a 50 percent chance of being diagnosed with breast cancer and 16 percent chance of developing ovarian cancer. Men with an alteration have about a 16 percent chance of developing prostate cancer by the age of 70. However, for any individual with an alteration, a precise estimate of risk is not possible.
Family history helps to place an individual's cancer risk in perspective, but is also an imperfect tool. For example, family history will be most useful in determining risk if a carrier has multiple relatives affected with breast or ovarian cancer. In this case, a woman's risk of breast cancer may be higher than the average of 56 percent.
If a carrier has little or no family history of breast and ovarian cancer, his or her risk will be much more difficult to assess. This is particularly true of women in small families with very few close female relatives.
Unless someone already has a strong family history of breast or ovarian cancer, it will be very difficult to know his or her precise risk until other risk factors for cancer are identified.
Yes. The NIH researchers are developing a follow-up study in the greater Washington, D.C. Jewish community with an option to be tested and receive individual test results for the three alterations in BRCA1 and BRCA2. All participants will receive counseling as to the risks and benefits of genetic testing. This study will also try to identify risk factors that might interact with BRCA1 and BRCA2 and modify someone's chance of getting breast, ovarian, and prostate cancer. Risk factors under consideration are hormonal factors and additional gene alterations.
No. Although certain alterations that may be unique to Norwegian, Icelandic and Dutch families have been identified, the frequency in the general population is not known, and no such studies have been planned to date.
This is the first community-based study to estimate the cancer risk associated with alterations in BRCA1 and BRCA2 in the general population. The researchers found that the risks for breast and ovarian cancer were lower on average in this population than in hereditary breast cancer families. Even though there is no data for other ethnic groups, the researchers speculate that future findings may be similar; that is, it is likely that most alterations in BRCA1 or BRCA2 that produce a shortened protein product will increase the cancer risk in the general population, but the average risk will probably not be as high as in cancer-prone families.
The decision of whether to be tested for a gene alteration is complex and personal. One of the factors to be considered is the cancer risk associated with having a positive or negative test result.
Based on this study, the average risk of breast, ovarian, and prostate cancer for people with BRCA1 and BRCA2 alterations is known more accurately. For example, the average risk of breast cancer is lower than previously thought, but is still significantly higher than for those who don't carry the alteration.
But gene alterations linked to cancer do not have the same effect on each person who carries them. For example, the findings from this study suggest that nearly half of the women with these alterations may never develop cancer. And since BRCA1 and BRCA2 alterations account for only a small portion of breast cancer, many women without an alteration will develop breast cancer.
Part of the complexity of someone's decision to be tested is that the medical consequences of an individual's test result - positive or negative - are not predictable. This is especially true of a carrier who does not have a personal or family history of cancer.
Besides the cancer risks, other considerations are important. There may be psychological and social effects of both positive and negative results for the individual tested and family members. Individuals should also consider how a positive or negative result might affect them and their relatives, especially if they have a strong history of cancer in the family.
In addition, privacy issues are important, since it is possible that having a positive or negative result may affect health insurance and employment.
Until recently, genetic testing for alterations that increase susceptibility to cancer was performed only in a research setting. With the past year, however, this kind of testing has become commercially available. Still, there is no consensus about the circumstances in which genetic testing might be useful, and this kind of testing is certainly not routine.
Scientists and physicians are still uncertain about how best to help alteration carriers. Even if the precise risk of cancer for an individual carrier were known, there are no proven effective risk reduction strategies. And physicians are not sure about the best ways to monitor those at high risk to assure early detection if they do develop cancer. More research is needed.
The hope is that these gene alterations as well as any others discovered in future studies will provide novel targets for the development of anticancer drugs. The interaction between the alterations and environmental factors may also present new strategies for cancer prevention.
For more information about genetic testing:
Several documents about genetics and genetic testing are available at About Cancer [cancer.gov].
The site includes:
- A directory of genetic counselors, physicians, geneticists, and nurses who have expertise in genetic testing and who will accept physicians' referrals for familial cancer risk counseling and/or genetic susceptibility testing. Because the issues surrounding genetic testing are highly personal and can have far-reaching consequences, a health professional trained in genetics is a good resource for exploring these issues.
- Position papers of several professional and advocacy organizations on the issue of genetic testing for susceptibility to cancer as well as fact sheet on genetics.
- "PDQ Screening and Prevention Information" is a document "Genetic Testing for Cancer Risk." PDQ is NCI's cancer information database.
Another resource is NCI's Cancer Information Service (CIS). By calling the CIS at 1-800-4-CANCER or 1-800-422-6237. The staff can send printed information and answer questions about cancer and cancer genetics. The CIS can also identify facilities, offering cancer risk assessment, counseling related to familial cancer and genetic susceptibility to cancer, and centers conducting research.
Online Help
Our 24/7 cancer helpline provides information and answers for people dealing with cancer. We can connect you with trained cancer information specialists who will answer questions about a cancer diagnosis and provide guidance and a compassionate ear.

Chat live online
Select the Live Chat button at the bottom of the page

Schedule a Video Chat
Face to face support

Call us at 1-800-227-2345
Available any time of day or night
Our highly trained specialists are available 24/7 via phone and on weekdays can assist through video calls and online chat. We connect patients, caregivers, and family members with essential services and resources at every step of their cancer journey. Ask us how you can get involved and support the fight against cancer. Some of the topics we can assist with include:
- Referrals to patient-related programs or resources
- Donations, website, or event-related assistance
- Tobacco-related topics
- Volunteer opportunities
- Cancer Information
For medical questions, we encourage you to review our information with your doctor.
Breast Cancer
- What Is Breast Cancer?
- What Causes Breast Cancer?
- Ductal Carcinoma in Situ (DCIS)
- Invasive Breast Cancer (IDC/ILC)
- Triple-negative Breast Cancer
- Inflammatory Breast Cancer
- Angiosarcoma of the Breast
- Paget Disease of the Breast
- Phyllodes Tumors
- Key Statistics for Breast Cancer
- What’s New in Breast Cancer Research?
- Breast Cancer Risk Factors You Cannot Change
- Lifestyle-related Breast Cancer Risk Factors
- Factors with Unclear Effects on Breast Cancer Risk
- Disproven or Controversial Breast Cancer Risk Factors
- Can I Lower My Risk of Breast Cancer?
- Genetic Counseling and Testing for Breast Cancer Risk
- Deciding Whether to Use Medicine to Reduce Breast Cancer Risk
- Tamoxifen and Raloxifene for Lowering Breast Cancer Risk
- Aromatase Inhibitors for Lowering Breast Cancer Risk
- Preventive Surgery to Reduce Breast Cancer Risk
- American Cancer Society Recommendations for the Early Detection of Breast Cancer
- Mammogram Basics
- Tips for Getting a Mammogram
- What Does the Doctor Look for on a Mammogram?
- Getting Called Back After a Mammogram
- Understanding Your Mammogram Report
- Breast Density and Your Mammogram Report
- Limitations of Mammograms
- Mammograms After Breast Cancer Surgery
- Mammograms for Women with Breast Implants
- Breast Ultrasound
- Newer and Experimental Breast Imaging Tests
- Breast Cancer Signs and Symptoms
- Fine Needle Aspiration (FNA) of the Breast
- Core Needle Biopsy of the Breast
- Surgical Breast Biopsy
- Questions to Ask Before a Breast Biopsy
- Finding Breast Cancer During Pregnancy
- Breast Cancer Grades
- Breast Cancer Ploidy and Cell Proliferation
- Breast Cancer Hormone Receptor Status
- Breast Cancer HER2 Status
Breast Cancer Gene Expression Tests
- Other Breast Cancer Gene, Protein, and Blood Tests
- Imaging Tests to Find Out if Breast Cancer Has Spread
- Breast Cancer Stages
- Breast Cancer Survival Rates
- Questions to Ask Your Doctor About Breast Cancer
- Breast-conserving Surgery (Lumpectomy)
- Lymph Node Surgery for Breast Cancer
- Exercises After Breast Cancer Surgery
- Radiation for Breast Cancer
- Chemotherapy for Breast Cancer
- Hormone Therapy for Breast Cancer
- Targeted Drug Therapy for Breast Cancer
- Immunotherapy for Breast Cancer
- Treatment of Ductal Carcinoma in Situ (DCIS)
- Treatment of Breast Cancer Stages I-III
- Treatment of Stage IV (Metastatic) Breast Cancer
- Treatment of Recurrent Breast Cancer
- Treatment of Triple-negative Breast Cancer
- Treatment of Inflammatory Breast Cancer
- Treating Breast Cancer During Pregnancy
- Should I Get Breast Reconstruction Surgery?
- Breast Reconstruction Alternatives
- Breast Reconstruction After Breast-conserving Surgery
- Breast Reconstruction Using Implants
- Breast Reconstruction Using Your Own Tissues (Flap Procedures)
- Reconstructing the Nipple and Areola After Breast Surgery
- Questions to Ask Your Surgeon About Breast Reconstruction
- Preparing for Breast Reconstruction Surgery
- What to Expect After Breast Reconstruction Surgery
- Follow-up Care After Breast Cancer Treatment
- Can I Lower My Risk of Breast Cancer Progressing or Coming Back?
- Body Image and Sexuality After Breast Cancer
- Pregnancy After Breast Cancer
- Menopausal Hormone Therapy After Breast Cancer
- Second Cancers After Breast Cancer
- If You Have Breast Cancer
- Fibrosis and Simple Cysts
- Hyperplasia (Ductal or Lobular)
- Lobular Carcinoma in Situ (LCIS)
- Fibroadenomas
- Intraductal Papillomas
- Fat Necrosis and Oil Cysts
- Duct Ectasia
- Radial Scars and Other Non-cancerous Breast Conditions
- Breast Cancer Videos
- Breast Cancer Quiz
- Text Alternative for 7 Things to Know About Getting a Mammogram Infographic
- Frequently Asked Questions About the American Cancer Society’s Breast Cancer Screening Guideline
What do the test results mean?
Testing options.
Gene expression tests are a form of personalized medicine - a way to learn more about your cancer and tailor your treatment.
These tests are done on breast cancer cells after surgery or biopsy to look at the patterns of a number of different genes. This process or test is sometimes called gene expression profiling .
The patterns found can help predict if certain early-stage breast cancers are likely to come back after initial treatment.
Some gene expression testing/profiling can help predict which women will most likely benefit from chemotherapy after breast surgery (adjuvant chemotherapy.) Hormone therapy is a standard treatment for hormone receptor-positive breast cancers, but it’s not always clear when to use chemotherapy. These tests can help guide that decision. Still, these tests cannot tell any one woman for certain if her cancer will come back with or without chemotherapy.
These tests continue to be studied in large clinical trials to better understand how and when to best use them. In the meantime, ask your doctor if these tests might be useful for you.
The Oncotype DX, MammaPrint, and Prosigna are examples of tests that look at different sets of breast cancer genes to see if chemotherapy is needed to help reduce the risk of cancer coming back (recurrence). More tests are in development. The type of test that's used will depend on your situation. Keep in mind that these tests are used for early-stage cancers, and testing isn’t needed in all cases. For example, if breast cancer is advanced, it might be clear that chemotherapy is needed, even without gene expression testing.
Oncotype DX
The Oncotype DX test is used for stage I, II or IIIa hormone receptor-positive tumors that have not spread to more than 3 lymph nodes and are HER2 negative . It can also be used for DCIS (ductal carcinoma in situ or stage 0 breast cancer) .
This test looks at a set of 21 genes in cancer cells from tumor biopsy or surgery samples to get a “recurrence score,” which is a number between 0 and 100. The score reflects the risk of the breast cancer coming back (recurring) in the next 9 years if you are treated with hormone therapy alone and how likely you are to benefit from getting chemo after surgery.
For women who are older than 50 years and have no lymph nodes with cancer:
- A low score (0-25) means a low risk of recurrence . Most women with low-recurrence scores do not benefit from chemotherapy and have good outcomes when treated with hormone therapy.
- A high score (26-100) means a higher risk of recurrence . Women with high-recurrence scores are more likely to benefit from the addition of chemotherapy to hormone therapy to help lower the chance of the cancer coming back.
For women age 50 or younger and have no lymph nodes with cancer:
- A low score (0-15) means a low risk of recurrence . Most of these women with low-recurrence scores do not benefit from chemotherapy and have good outcomes when treated with hormone therapy.
- An intermediate score (16-25) means that some women in this group might have a small benefit from adding chemotherapy to hormone therapy to lower the risk of the cancer coming back. Talk to your doctor about options.
For women age 50 or younger that have cancer in the lymph nodes:
- A low score (0-25) means a lower risk of recurrence, but women in this group might have a benefit from adding chemotherapy to hormone therapy. Another option might be ovarian suppression along with tamoxifen or an aromatase inhibitor .
- A high score (26-100) means a higher risk of recurrence . Women in this group are more likely to benefit from the addition of chemotherapy to hormone therapy to help lower the chance of the cancer coming back.
The MammaPrint test can be used to help determine how likely breast cancers are to recur in a distant part of the body after treatment. It can be used for any type of invasive breast cancer that’s 5cm (about 2 inches) or smaller and has spread to no more than 3 lymph nodes. This test can be done regardless of a woman's age or the cancer's hormone or HER2 status.
The test looks at 70 different genes to determine if the cancer is at low risk or high risk of coming back (recurring) in the next 10 years. The test results come back as either “low risk” or “high risk.” This test is also being studied as a way to determine whether certain women might benefit from chemotherapy.
The Prosigna test can be used to predict the risk of recurrence in the next 10 years in women who have gone through menopause (postmenopausal) and whose invasive breast cancers are hormone receptor-positive and HER2-negative. It can be used to test early-stage cancers that have not spread to the lymph nodes, or early-stage cancers with no more than 3 positive lymph nodes.
The test looks at 50 genes and classifies the results as low, intermediate, or high risk.
Breast Cancer Index
The Breast Cancer Index test is done on your tumor sample from when you are first diagnosed. It can be used to predict the risk of recurrence in the 5 to 10 years after diagnosis in women whose invasive breast cancers are hormone receptor-positive and have not spread to nearby lymph nodes or have not spread to more than 3 lymph nodes. It can also help predict who might benefit from hormone therapy for longer than 5 years.
The test looks at 11 genes and classifies the results as low or high risk.
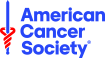
The American Cancer Society medical and editorial content team
Our team is made up of doctors and oncology certified nurses with deep knowledge of cancer care as well as editors and translators with extensive experience in medical writing.
Cardoso F, van't Veer LJ, Bogaerts J, Slaets L, Viale G, Delaloge S et al. 70-Gene Signature as an Aid to Treatment Decisions in Early-Stage Breast Cancer. N Engl J Med . 2016;375(8):717-29.
Foukakis T, and Bergh J, and Hurvitz SA. Deciding when to use adjuvant chemotherapy for hormone receptor-positive, HER2-negative breast cancer. In Vora SR, ed. UpToDate . Waltham, Mass.: UpToDate, 2021. https://www.uptodate.com. Last updated August 17, 2021. Accessed September 14, 2021.
Gnant M, Filipits M, Dubsky P, et al. Predicting risk for late metastasis: The PAM50 risk of recurrence (ROR) score after 5 years of endocrine therapy in postmenopausal women with HR+ early breast cancer: A study on 1,478 patients for the ABCSG-8 trial. Ann Oncol. 2013; 24(Suppl 3): iii29-iii37.
Harris LN, Ismaila N, McShane LM, et al. Use of Biomarkers to Guide Decisions on Adjuvant Systemic Therapy for Women With Early-Stage Invasive Breast Cancer: American Society of Clinical Oncology Clinical Practice Guideline. J Clin Oncol . 2016;34(10):1134–1150.
Knauer M, Mook S, Rutgers EJ, Bender RA, Hauptmann M, van de Vijver MJ et al. The predictive value of the 70-gene signature for adjuvant chemotherapy in early breast cancer. Breast Cancer Res Treat. 2010 Apr;120(3):655-61.
Krop I, Ismaila N, Andre F, et al. Use of Biomarkers to Guide Decisions on Adjuvant Systemic Therapy for Women With Early-Stage Invasive Breast Cancer: American Society of Clinical Oncology Clinical Practice Guideline Focused Update. J Clin Oncol . 2017;35(24):2838–2847.
National Comprehensive Cancer Network (NCCN). Practice Guidelines in Oncology: Breast Cancer. Version 8.2021. Accessed at https://www.nccn.org/professionals/physician_gls/pdf/breast.pdf on Sept. 14, 2021.
Paik, S. Development and Clinical Utility of a 21-Gene Recurrence Score Prognostic Assay in Patients with Early Breast Cancer Treated with Tamoxifen. The Oncologist . 2007;12(6): 631-635.
Sparano JA, Gray RJ, Makower DF, Pritchard KI, Albain DF, Hayes DF, et al. Adjuvant chemotherapy guided by a 21-gene expression assay in breast cancer. N Engl J Med . 2018;379(2):111-121.
Sparano JA, Gray RJ, Ravdin PM, Makower DF, Pritchard KI, Albain KS et al. Clinical and Genomic Risk to Guide the Use of Adjuvant Therapy for Breast Cancer. N Engl J Med . 2019;380(25):2395-2405.
Wallden B, Storhoff J, Nielsen T, et al. Development and verification of the PAM50-based Prosigna breast cancer gene signature assay. BMC Med Genomics . 2015;8:54.
Last Revised: November 8, 2021
American Cancer Society medical information is copyrighted material. For reprint requests, please see our Content Usage Policy .
American Cancer Society Emails
Sign up to stay up-to-date with news, valuable information, and ways to get involved with the American Cancer Society.
More in Breast Cancer
- About Breast Cancer
- Risk and Prevention
- Early Detection and Diagnosis
- Understanding a Breast Cancer Diagnosis
- Breast Reconstruction Surgery
- Living as a Breast Cancer Survivor
Help us end cancer as we know it, for everyone.

- Alzheimer's disease & dementia
- Arthritis & Rheumatism
- Attention deficit disorders
- Autism spectrum disorders
- Biomedical technology
- Diseases, Conditions, Syndromes
- Endocrinology & Metabolism
- Gastroenterology
- Gerontology & Geriatrics
- Health informatics
- Inflammatory disorders
- Medical economics
- Medical research
- Medications
- Neuroscience
- Obstetrics & gynaecology
- Oncology & Cancer
- Ophthalmology
- Overweight & Obesity
- Parkinson's & Movement disorders
- Psychology & Psychiatry
- Radiology & Imaging
- Sleep disorders
- Sports medicine & Kinesiology
- Vaccination
- Breast cancer
- Cardiovascular disease
- Chronic obstructive pulmonary disease
- Colon cancer
- Coronary artery disease
- Heart attack
- Heart disease
- High blood pressure
- Kidney disease
- Lung cancer
- Multiple sclerosis
- Myocardial infarction
- Ovarian cancer
- Post traumatic stress disorder
- Rheumatoid arthritis
- Schizophrenia
- Skin cancer
- Type 2 diabetes
- Full List »
share this!
June 4, 2024
This article has been reviewed according to Science X's editorial process and policies . Editors have highlighted the following attributes while ensuring the content's credibility:
fact-checked
peer-reviewed publication
trusted source
Combining biomedical data from breast cancer patients could lead to 'groundbreaking discoveries'
by University of Strathclyde, Glasgow
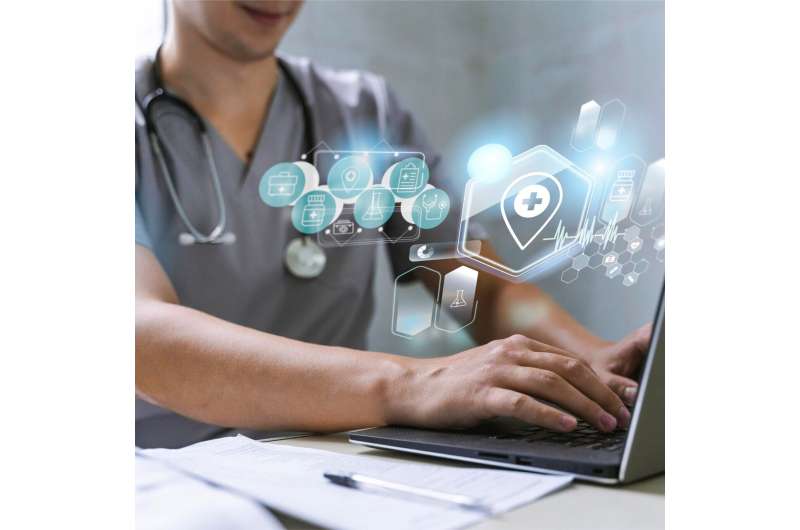
Studying combined data from the UK Biobank, a unique record of patient information from more than half a million Britons, could help make "groundbreaking discoveries" in the understanding and treatment of breast cancer, say researchers.
Breast cancer is one of the leading causes of death of women globally—accounting for 685,000 deaths in 2020—but some forms of the disease are more deadly than others.
Understanding the unique characteristics of different variants of the disease could help doctors to diagnose and classify patients and develop new, more personalized therapies.
The UK Biobank is a vast database of health information—including clinical, genetic, protein, and metabolic data from volunteer participants. But scientists from the University of Strathclyde say there is more scope to study these data sets together to identify the unique biological features of different variants of breast cancer in the future.
The researchers reviewed studies from the past five years that used UK Biobank data to research breast cancer.
Genetic data
Their results, published in the Computational and Structural Biotechnology Journal , found 125 studies, with 76 focusing on genetic data , and only two studies looking at protein and metabolic data. None used all types of data together to study breast cancer.
A closer look at the 76 genetic studies identified 2,870 genetic variants in 445 genes linked to breast cancer. Thirteen of these genes showed different changes in different types of breast cancer, and 59 were well-known breast cancer genes. These genes are involved in general cancer processes like DNA repair and gene expression.
Lead author Dr. Nicholas Rattray, said, "It is critical that new ways are developed to understand how breast cancer develops in patients with differing susceptibility. The UK Biobank is a huge resource that is allowing scientists use far larger cohort numbers to understand disease in far great detail."
Dr. Zahra Rattray, senior lecturer in the Strathclyde Institute of Pharmacy and Biomedical Sciences, said, "Our review showed that most research so far has focused on genetic differences in breast cancer.
"Few studies have effectively combined data types thus far. Using combined data methods in the future could help us better understand different types of breast cancer."
Harnessing multiomics
The researchers say the lack of research focusing on the analysis of proteomics and metabolomics datasets could be because genetic data was more readily available than proteomic and metabolomic data.
In addition, combining different types of data is complicated, making it hard to take this "multi-omic" approach in breast cancer studies.
Dr. Zahra Rattray added, "The UK Biobank has not, until now, been effectively used to identify previously unknown breast cancer associated genes."
Lisa van den Driest, a Ph.D. student at Strathclyde University and the study's first author said, "Our findings reveal the promising potential of harnessing multi-omics approaches or other combinations of data types using the large amount of information in the UK Biobank to unravel the intricate cancer biology underlying distinct subtypes of breast cancer.
"It is a complex disease and a major cause of illness and death among women worldwide. Despite progress in diagnosing and treating breast cancer, some types, like triple-negative breast cancer , still have poor outcomes. By 2040, it is expected that 3 million women will be diagnosed each year. Because of this, it is crucial to find new ways to detect and categorize breast cancer to reduce its impact globally."
Clinical lead on the project, Dr. Alison Lannigan, NHS Lanarkshire, said, "This is an exciting analysis of multi-omics in breast cancer using the UK Biobank data.
"Understanding the complexities and variations in disease biology paves the way to develop new and individualized patient treatments. As breast cancer clinicians we would encourage all breast centers and patients to participate in breast cancer studies and contribute to enhancing the UK Biobank and subsequent research."
Explore further
Feedback to editors

Commonly used alcohol-based mouthwash brand may disrupt the balance of your oral microbiome, scientists say
5 hours ago

Women's mental agility is better during menstruation, shows study
6 hours ago

Injury prediction rule could decrease radiographic imaging exposure in children, study shows

A promising vaccine approach to induce longer-lasting protective immunity against COVID-19
7 hours ago

How tumor stiffness alters immune cell behavior to escape destruction
9 hours ago

Veterans with service dogs found to have fewer PTSD symptoms, higher quality of life

Scientists reveal how a potassium ion channel reprograms energy production in cancer cells

Virus that causes COVID-19 can remain in sperm for 110 days after infection

An anti-inflammatory curbs spread of fungi causing serious blood infections

New molecular tool shows how telomeres relate to heart health
10 hours ago
Related Stories

Assessing breast cancer risk
Mar 18, 2024

International study identifies new breast cancer susceptibility genes
Aug 17, 2023

What is a mammogram, and why are they important?
Oct 24, 2022

Genetic breast cancer study alters guidelines in Sweden
Aug 24, 2023

New breast cancer targets
May 4, 2018

New breast cancer susceptibility gene candidate identified
Mar 30, 2023
Recommended for you

Phase III trial shows lorlatinib highly effective against ALK-positive non–small cell lung cancer
12 hours ago

New role of tumor suppressor STAT3β discovered in leukemia
14 hours ago

New test detects more cases of cervical cancer

Novel software combines gene activity and tissue location to decode disease mechanisms

AI tool helps monitor brain development in children and identify neurodevelopmental delays
15 hours ago
Let us know if there is a problem with our content
Use this form if you have come across a typo, inaccuracy or would like to send an edit request for the content on this page. For general inquiries, please use our contact form . For general feedback, use the public comments section below (please adhere to guidelines ).
Please select the most appropriate category to facilitate processing of your request
Thank you for taking time to provide your feedback to the editors.
Your feedback is important to us. However, we do not guarantee individual replies due to the high volume of messages.
E-mail the story
Your email address is used only to let the recipient know who sent the email. Neither your address nor the recipient's address will be used for any other purpose. The information you enter will appear in your e-mail message and is not retained by Medical Xpress in any form.
Newsletter sign up
Get weekly and/or daily updates delivered to your inbox. You can unsubscribe at any time and we'll never share your details to third parties.
More information Privacy policy
Donate and enjoy an ad-free experience
We keep our content available to everyone. Consider supporting Science X's mission by getting a premium account.
E-mail newsletter

An official website of the United States government
The .gov means it’s official. Federal government websites often end in .gov or .mil. Before sharing sensitive information, make sure you’re on a federal government site.
The site is secure. The https:// ensures that you are connecting to the official website and that any information you provide is encrypted and transmitted securely.
- Publications
- Account settings
Preview improvements coming to the PMC website in October 2024. Learn More or Try it out now .
- Advanced Search
- Journal List
- Appl Clin Genet
The genetics of breast cancer: risk factors for disease
Andrew collins.
Genetic Epidemiology and Bioinformatics Research Group, Human Genetics Research Division, Southampton General Hospital, School of Medicine, University of Southampton, Southampton, UK
Ioannis Politopoulos
The genetic factors known to be involved in breast cancer risk comprise about 30 genes. These include the high-penetrance early-onset breast cancer genes, BRCA1 and BRCA2 , a number of rare cancer syndrome genes, and rare genes with more moderate penetrance. A larger group of common variants has more recently been identified through genome-wide association studies. Quite a number of these common variants are mapped to genomic regions without being firmly associated with specific genes. It is thought that most of these variants have gene regulatory functions, but their precise roles in disease susceptibility are not well understood. Common variants account for only a small percentage of the risk of disease because they have low penetrance. Collectively, the breast cancer genes identified to date contribute only ~30% of the familial risk. Therefore, there is much interest in accounting for the missing heritability, and possible sources include loss of information through ignoring phenotype heterogeneity (disease subtypes have genetic differences), gene–gene and gene–environment interaction, and rarer forms of variation. Identification of these rarer variations in coding regions is now feasible and cost effective through exome sequencing, which has already identified high-penetrance variants for some rare diseases. Targeting more ‘extreme’ breast cancer phenotypes, particularly cases with early-onset disease, a strong family history (not accounted for by BRCA mutations), and with specific tumor subtypes, provides a route to progress using next-generation sequencing methods.
Introduction
Family history of breast cancer is known to be one of the strongest risk factors for this disease. For example, meta-analysis of familial breast cancer studies gives lifetime risk ratios of 1.80 in families with one affected first-degree relative, 2.93 in families with two affected relatives, and 3.90 in families with three affected relatives. 1 Risk ratios are highest for cases at younger ages and, for a particular individual, are greater the younger their relative is diagnosed. The familial pattern of the disease provides clear evidence for the important role of genetic variation in determining risk. The identification of genetic factors involved in predisposition to breast cancer has been a topic of intensive study for more than 20 years. An important early breakthrough in the genetic dissection of the disease was linkage mapping, using breast cancer family data, of the BRCA1 2 and BRCA2 3 genes. Rare mutations in these genes confer high relative risks to carriers of 10- to 20-fold, corresponding to a 30%–60% risk by the age of 60 years, compared with 3% for the general population. 4 These mutations account for ~16%–20% of the familial risk of breast cancer in the general population. 4 , 5 In addition, there are a number of rare to very rare high-penetrance gene variants that underlie cancer syndromes and a few rare genes that have more moderate penetrance. Collectively, the rare genes found to date account for <25% of the familial risk. Recent studies have focused on the role of common genetic variation, through analysis of large samples of cases and controls tested for association at many thousands of single nucleotide polymorphism (SNP) markers. These studies have identified a number of common breast cancer genes and revealed new insights into the natural history of the disease. However, all these genes are low-penetrance variants that account for only a few percent of the familial risk. Because the bulk of the familial risk is unexplained by the genes identified thus far, research is focusing on identifying sources of the ‘missing’ heritability. This review considers what is known about the genetic basis of breast cancer and evaluates the clinical utility of the evidence, while emphasizing ongoing strategies to identify more of the genetic variation. New technologies, such as next-generation sequencing, and the development of novel bioinformatic approaches to analysis are at the forefront of this effort.
Mendelian high-penetrance genes
About 100 genes for genetic diseases showing Mendelian patterns of inheritance in families are known. 6 These are invariably rare genes and associated with high relative risks. Most of the genes have been identified through linkage analysis of carefully selected families, followed by positional cloning. Within this category are the breast cancer BRCA1 and BRCA2 genes, which contain over 1000 mutations. Genetic screening for the spectrum of important mutations in these genes in high-risk families is well established. The BRCA1 ‘breast cancer 1 early-onset’ gene 2 is involved in susceptibility to breast and ovarian cancer at a young age, and tumors can arise through somatic or germline mutations. Impaired or lost BRCA1 function underlies substantial genome instability including increases in the number of mutations, DNA breakage and chromatid exchanges, increased sensitivity to DNA damage, and defects in cell-cycle checkpoint functions. The role of BRCA1 in the DNA damage response is that of ‘caretaker’ or ‘master regulator’ in the genome. 7 – 9
Jensen et al 10 isolated the large protein encoded by the BRCA2 gene and showed it to be a key mediator of homologous recombination. It is a crucial element in the DNA repair process which, if impaired through mutation, can lead to chromosome instability and cancer. It is known to mediate recombinational DNA repair by promoting assembly of RAD51 onto single-stranded DNA. This has a key role in catalyzing the invasion and exchange of homologous DNA sequences. Mutations in the BRCA2 gene may disrupt this mechanism and impair repair of DNA breaks, using homologous sequences from an intact homolog or sister chromatid, leading to errors in the repair process and chromosome instability.
BRCA1 and BRCA2 are likely to be the only major high-penetrance genes underlying breast cancer. Germline mutations in the TP53 gene cause Li–Fraumeni syndrome, a phenotype which includes early-onset breast cancer, 11 but these mutations are far rarer. Both BRCA1 and BRCA2 genes were identified using linkage mapping in families, a method that has been successful in identifying many Mendelian disease genes. However, this strategy has contributed little to the study of more common or ‘complex’ forms of disease, mediated by genetic variants with reduced penetrance which may interact with environmental and other genetic factors. The complexity of this pattern of inheritance greatly reduces the power to detect genes through family-based studies.
Rare cancer syndromes and rare moderate-penetrance genes
There are a number of syndromes that include breast cancer as a component of the disease phenotype. Rare to uncommon mutations in the PTEN 12 and STK11 13 genes cause Cowden and Peutz–Jeghers syndromes, respectively, and both are associated with considerably increased breast cancer risk. 14 The E-cadherin gene ( CDH1 ) encodes a cellular adhesion protein and is a powerful tumor suppressor of breast cancer. 15 It is particularly implicated in invasive lobular breast carcinomas. RAD51C is another gene involved in the recombinational repair of double-stranded DNA breaks. Rare germline mutations have been shown to confer increased risks of breast and ovarian cancer. 16 Segregation in families follows Mendelian patterns, and the disease phenotype resembles that of BRCA1 and BRCA2 mutation carriers.
There are also a number of gene mutations associated with more moderate risks of breast cancer, which show marked departures from Mendelian patterns of inheritance. As a result, segregation of disease with the mutation may be unhelpful to confirm relationship with disease. Genes in this category include germline mutations in the ataxia-telangiectasia ( ATM ) gene, which are associated with increased risk (~2.2-fold) of breast cancer in carriers of heterozygous mutations, with apparently higher risks below the age of 50 years. 17 Other rare moderate-penetrance genes include heterozygous mutations in BRIP1 (encoding a BRCA1 -interacting protein) that confers elevated risks of breast cancer and Fanconi anemia subtype FA-J for bi-allelic mutations. The partner and localizer of BRCA2 ( PALB2 ) gene interacts with BRCA2 , and mono-allelic mutations are involved in familial breast cancer, conferring a 2.3-fold risk. Mutations in BRCA2 are also known to underlie Fanconi anemia (subtype FA-D1), and bi-allelic mutations of PALB2 underlie the very similar Fanconi anemia subtype FA-N. 18 Rare variants in the cell cycle checkpoint kinase 2 ( CHEK2 ) gene are known to underlie an approximately twofold increase in risk of breast cancer. Products of this gene are involved in DNA damage repair, and mutations are found in 1%–2% of unselected women with breast cancer. 19
Common low-penetrance breast cancer genes
Genome-wide association studies (GWAS) use panels of up to a million or more SNPs to identify common gene variants in large case and control samples. GWAS have identified more than 100 such low-penetrance loci involved in cancer, including at least 17 related to breast cancer ( Table 1 ). These variants have allele frequencies in the range 0.05–0.5, but they confer only small increases in disease risk. 4 Because of the greatly reduced penetrance and strongly non-Mendelian patterns of inheritance, there is often considerable uncertainty about the exact underlying genetic mutation. Not only are the most strongly associated SNPs unlikely to be the causal sites (these are ‘tags’ selected to represent variation at many polymorphic sites that are not tested directly) but there also may be uncertainty about the gene involved. It has also been suggested that multiple rare variants create ‘synthetic association’ signals in a GWAS if they occur more often in association with a common tag SNP. This implies that causal variants could be many megabases away from variants detected in GWAS, 20 although this scenario appears to be rare. 21 Perhaps, one of the unexpected findings from these studies is a greater-than-anticipated role for noncoding variants in common diseases. 22 From the analysis of population sequences, 23 <30% of common variants associated with disease are annotated as, or in linkage disequilibrium with, nonsynonymous (coding) variation. This supports the view that many of the common disease variants have gene regulatory roles.
Known breast cancer susceptibility genes and regions
Notes: ? refers to ‘possible’ gene or function in the breast cancer context. There is uncertainty about the exact genes and their functional roles in breast cancer.
Abbreviation: BC, breast cancer; GWAS, genome-wide association studies.
Among the set of well-established common susceptibility genes are variants in intron 2 of the FGFR2 gene, 24 which, among the common variants, are likely to make one of the larger contributions to relative risk, at least for postmenopausal disease. Easton et al 25 found that the rs2981582 SNP (allele frequency 0.38) contributes odds ratios of 1.23 and 1.63 for heterozygote and homozygote genotypes, respectively. The FGFR2 gene encodes a fibroblast growth factor (FGF) receptor. FGFs and their corresponding receptors are involved in regulation of the proliferation, survival, migration, and differentiation of cells. The considerable importance of FGF signaling in a range of tumor types is now becoming recognized. 26 SNPs within intron 2 are involved in FGFR2 upregulation, and aberrant signaling activation induces proliferation and survival of tumor cells. 27 The identification of this gene, which was unanticipated as a cancer gene, has prompted research into related genes and their potential roles in cancer. Other FGFs (eg, FGF-8) appear to be involved in breast cancer cell growth through stimulation of cell cycle and prevention of cell death. 28
Other low-penetrance variants that have been identified through GWAS include CASP8 (caspase 8), which encodes an apoptotic enzyme. 29 The variant rs1045485 is protective, contributing odds ratios of 0.89 and 0.74 for heterozygotes and rare homozygotes, respectively. Recently, variants in CASP8 have been shown to alter risks (in a protective direction) in individuals with a family history of breast cancer. 30
Breast tumors are classified according to whether they have receptor proteins that bind to estrogen and progesterone. Such cells are termed ER+ and PR+ and require estrogen and progesterone to grow. Conversely, ER− and PR− tumors lack the protein that allows the hormones to bind. Tumor classifications influence the choice of treatment regimes for the patient. A further classification arises through tumors that overexpress the human epidermal growth factor receptor 2 ( HER2 ) gene, which are termed HER2+ (conversely, HER2−). The triple-negative subtypes are ER−, PR−, and HER2− and are characterized by aggressive tumors and reduced range of effective treatment options. Several common gene variants are more strongly associated with specific cancer subtypes. These include the TOX3 gene, formerly called TNRC9 in which variant rs3803662 contributes a 1.64-fold homozygote risk, specifically in ER+ cancer. 31 This gene encodes a high-mobility group chromatin-associated protein and increased expression is implicated in bone metastasis. 32 Fine mapping has shown that hypothesized susceptibility variants lie in an intergenic region consistent with a gene regulatory function. 33 These authors note there remains uncertainty as to whether the causal variant is actually involved in the regulation of the nearby retinoblastoma-like gene 2 ( RBL2 ) gene, which is involved in cell cycle regulation, given gene expression evidence.
The mitogen-activate protein kinase ( MAP3K1 ) breast cancer gene 25 is a member of the Ras/Raf/MEK/ERK signaling pathway (as is FGFR2) and is involved in regulating transcription of a number of cancer genes. MAP3K1 has been found to be more strongly associated with ER+ and PR+ tumors than ER−/PR− subtypes. There is also a stronger association with HER2+ tumors. 34
The LSP1 gene was identified as a breast cancer susceptibility locus by Easton et al, 25 who identified an SNP within intron 10 as the most strongly associated. LSP1 encodes lymphocyte-specific protein 1, which is an F-actin binding cytoskeletal protein. The same study also identified a breast cancer variant in the 8q24 region containing no known genes. This region is also associated with prostate cancer. 35
Stacey et al 31 identified a SNP on 2q35, a region with no known genes, as associated with breast cancer in Icelandic patients with ER+ breast cancer. Milne et al 36 also found an association with ER− disease, although there was a stronger signal for ER+. Other breast cancer associations include signals on 3p24, potentially relating to the genes SLC4A7 or NEK10 , and on 17q22, perhaps related to COX11 . These SNPs contribute odds ratios of 1.11 and 0.97 for heterozygote and homozygote genotypes, respectively. 37 Additionally, a common variant close to MRPS30 on 5p12 was found to confer higher risk of ER+ disease. 38 Turnbull et al 39 described five new associations on chromosomes 9, 10 (three regions), and 11. Two further signals reported by Thomas et al 40 include a SNP in the pericentromeric part of chromosome 1, within a region containing NOTCH2 and FCGR1B , and a signal associated with another double-strand break repair gene ( RAD51L1 ) on 14q24.1. There is evidence that the chromosome 1 locus is more strongly associated with ER+ disease.
Considerable additional follow-up investigation will be required to establish the relationships between many of the SNPs and the actual causal variant(s) and to further elucidate the role in disease for many of these common genes.
The genetic basis of breast cancer subphenotypes
Analysis of breast cancer as a single phenotype is becoming less typical as genetic differences between disease subtypes are more clearly established. Increased power to detect genetic variants is expected using patients belonging to genetically more homogeneous subgroups, rather than analyzing more heterogeneous groupings. There is evidence that many breast cancer GWAS studies have been enriched with ER+ cases because ER positivity is found in a higher proportion of the later-onset (usually postmenopausal) cases used in most of these studies. For this reason, ER+ disease is better characterized genetically than ER− disease. For example, Stacey et al 38 identified two SNPs on chromosome 5p12 that confer risk preferentially for ER+ tumors. Garcia-Closas et al 41 showed that variants in FGFR2 are more strongly related to ER+ than ER− (and also more strongly associated with PR+, low tumor grade, and lymph node-positive tumors). The breast cancer association in the 8q24 region is significantly stronger for ER+, PR+, and low-grade tumors. Reeves et al 42 examined risk odds ratios for low-penetrance breast cancer genes in a sample of more than 10,000 cases and controls in relation to ER+ and ER− classification, for bilateral and unilateral disease, and for lobular versus ductal tumors. They noted higher odds ratios for ER+ disease for FGFR2 and TCNR9 , compared with ER− disease, greater association with bilateral, compared with unilateral, and for lobular disease compared with ductal disease in the 2q region. Using a polygenic risk score, based on seven breast cancer SNPs, the estimated cumulative incidence in the top fifth of the score distribution for ER+ disease is 7.4% compared with only 1.4% for ER− disease. Since the polygenic risk score is substantially more strongly predictive for ER+ disease, there is a strong case for more thorough evaluation of the genetic basis of the ER− subtype.
Triple-negative breast cancers are associated with poor prognosis due to aggressive tumor behavior and poor response to chemotherapy. 43 After screening 2301 triple-negative cases and 3949 controls, Antoniou et al 44 identified five SNPs on 19p13 that modify risk in BRCA1 mutation carriers and are specifically associated with triple-negative breast cancer. Additional phenotypic subtypes which are currently being interrogated genetically include differences in susceptibility variants between racial groups and in response to treatment and prognosis.
Genetic risk factors for breast cancer: clinical applications
Mutations in the BRCA1 and BRCA2 genes are rare but underlie severe and early-onset forms of the disease. Screening for mutations in women with a strong family history, usually linked to BRCA mutations, determines individual risks for this early-onset form of disease. However, most patients (~95%) do not show clear-cut family histories of early- or later-onset disease. The role of more common breast cancer variation in risk prediction is far less well established. Pharoah et al 45 determined a multiplicative model using the 12 most significant common variants to define individual relative risks in the range 0.4-fold to fourfold compared with the general population. Given that there is a 12% population lifetime risk, deleterious common mutations contribute a 24%–36% lifetime risk, which may be high enough to instigate earlier and more intensive screening for common genetic forms of the disease. Gulcher and Stefansson 46 point out that some women classified as at average risk would be reclassified as at higher risk based on their profile of common breast cancer variation. Similarly, some women might be reclassified as having lower-than-average risk based on their common gene profile. Risk estimates might be more reliably determined by multiplying risks from the genetic profiles with independent risks from conventional measures, such as family history, age at menarche, and pregnancy history. Successful application of common breast cancer gene profiles in clinical practice would have potential benefits by facilitating earlier diagnosis, reduced costs, less intensive therapeutic intervention, and disease management in the longer term.
As understanding of the genetic basis of breast cancer increases, further refinement in genetic risk models can be expected. The different genetic basis of tumor subtypes is a clear example of where refinement might take place as genetic profiles become predictive of tumor characteristics. At this stage, it is already well established that women with, or at higher risk for, ER+ cancer are a good candidates for treatment with tamoxifen or raloxifene that specifically targets ER+ disease.
Finding the missing heritability
The breast cancer genes identified thus far explain only about 30% of the heritability, which is the proportion of the phenotypic variance that can be attributed to genetic variation. There are several possible sources for the missing genes, and this is a subject of intense argument and ongoing research.
Undetected common variation
GWAS using SNPs target only high-frequency alleles, and risk alleles found through these methods all have frequencies well in excess of 0.05. 22 Even within this common allele ‘window’, the SNP panels provide incomplete genome coverage, due in part to technical limitations of the genotyping platforms, but mainly due to cost, which places reliance on tagging SNPs (using a SNP in linkage disequilibrium with many others to represent or tag a specific haplotype). Such an approach is cost effective but loses information. 47 Furthermore, these platforms are relatively enriched for nonsynonymous coding SNPs (cSNPs), so the coverage of synonymous cSNPs and noncoding SNPs is incomplete. Given that common disease variants include a higher proportion of regulatory SNPs, which lie outside coding regions, it is likely that important common variation has been missed by the GWAS undertaken thus far. Because effect sizes of common variants are low, very large samples of cases and controls are required for effective GWAS. Many as yet undetected common variants will have increasingly small effects on risk as variants with larger effect sizes will have already been detected through the completed GWAS. The largest study to date of common variation underlying a complex trait is the analysis of the genetic basis of height. Allen et al 48 tested data from 183,727 individuals and identified hundreds of common genetic variants in at least 180 loci that account for ~10% of the phenotypic variation in height. They estimated that as yet unidentified common variation (with similar effect sizes to those already found) will eventually account for ~20% of the heritable variation, but detecting these would require a sample size of 500,000 individuals. Importantly, they concluded that many genetic loci underlying variation in height show allelic heterogeneity suggesting that as yet unidentified causal variants will map to the loci already identified in GWAS. These missing variants are likely to span the allele frequency spectrum, including rare variants with higher penetrance, but the remaining low-penetrance variants can only be detected by ever-larger GWAS.
Structural variation
Structural variation, such as copy number variants (CNVs), which are not well tagged by SNPs in current arrays, may be a source of missing heritability in breast cancer. There is evidence that at least the common CNVs are in strong linkage disequilibrium with common SNPs genotyped in GWAS and hence may be adequately ‘tagged’ by existing panels. 49 Significant associations with rare CNVs (frequency range 0.2%–1%) have been identified for a number of neuropsychiatric traits, such as autism, epilepsy, and mental retardation, 50 although no CNVs have been convincingly associated with cancer phenotypes thus far. 49
Gene–gene and gene–environment interaction
Other possibilities include interaction effects between genes and between genes and environment. Exploring such scenarios presents analytical challenges and there is relatively limited evidence for an important role for interaction thus far. Ritchie et al 51 modeled data for 10 SNPs in the genes COMT , CYP1A1 , CYP1B1 , GSTM1 , and GSTT1 . They identified an interaction between all the genes that were significantly associated with increased risk for sporadic breast cancer. Briollais et al 52 also identified SNP–SNP interactions associated with breast cancer, including an interaction between XPD and IL10 genes as the most significant two-way interaction. Travis et al 53 examined the relationship between environmental variables, such as reproductive, behavioral, and anthropometric factors, with low-penetrance breast cancer genes. After allowing for multiple testing, they observed no evidence for increased breast cancer risk arising through gene–environment interaction in their sample of 7610 women. Because of the potentially huge number of statistical tests in such comparisons, obtaining a large enough sample to have power to demonstrate an effect can be difficult. Furthermore, confirmatory studies, along with functional analyses of the biological pathways involved, are essential to fully comprehend the importance of putative gene–gene and gene–environment interactions. 54 Moore et al 55 argued that the information gleaned from GWAS data collected thus far has been limited by failure to integrate existing knowledge about disease pathology: the ‘single SNP’ analysis approach ignores the genomic and environmental context. They recommend enhanced bioinformatic approaches to develop a holistic approach that recognizes the full complexity of gene–phenotype, gene–gene, and gene–environment interactions.
Undetected rare variation
Searching for rarer variants with larger effect sizes is likely to be a successful strategy for identifying more of the missing heritability. Rare variants have not been screened by GWAS, so this source of novel genetic variation is largely unexplored. Rare variants may contribute odds ratios in the range 2–5, compared with common variants that typically have odds ratios <1.5. 56 Targets for ongoing and future studies include low-frequency variants with minor allele frequencies in the 0.3%–5% range. Studies exploiting next-generation sequencing include Johansen et al, 57 who tested association with high triglyceride levels and resequenced loci previously identified as containing common variation. They found approximately twice as many rare coding genetic variants associated with high triglycerides located within the same genes. Determining the extent to which low-frequency and rare causal variants are colocated within breast cancer loci already identified in GWA studies depends on future sequencing efforts targeting these well-established genes. There is clearly a strong case to examine susceptibility variants over the full allele frequency range. 56 Next-generation sequencing for the analysis of breast cancer exomes (the ~30 Mb of sequence within protein-coding exons) of patients with early onset and a strong family history, which are negative for known highly penetrant rare mutations ( BRCA1 , BRCA2 , and TP53 ), are likely to be informative. Using exome sequencing, the full complement of, for example, SNPs and insertion–deletion polymorphisms can be characterized in every sample. Support for this strategy comes from the identification of rare highly penetrant mutations in the RAD51C gene. 16 For less completely penetrant variants, there are, however, many more difficulties in assessing the significance of the variation identified. The 1000 genomes project 23 provides reference sequence for studying relationships between phenotype and genotype. The pilot phase describes exon-targeted sequencing of 697 individuals and whole genome sequencing of 179 individuals. The study determined the location and frequency of 15 million SNPs, 1 million insertion/deletion polymorphisms, and 20,000 structural variants. Comparing the pattern of variation identified in disease samples with this catalog of ‘normal’ variation is a crucial step in the process of determining the disease significance of any variants found.
Scale-up to sequence exomes of much larger samples of cases to investigate variants with intermediate and lower penetrance has not been undertaken thus far. Large samples will be required, and genetic heterogeneity, combined with the huge volume of (mostly unimportant) variation uncovered, poses extreme challenges for data interpretation even given knowledge about ‘background’ variation provided by the 1000 genomes project. In these cases, assessment of potential functional roles for variants found requires integration of information on gene expression profiles and other sources of transcriptome data and implementation of bioinformatic approaches to predict functional effects. Exome sequencing has its limitations. Apart from the fact that only exons are screened and that much important variation is known to reside outside these regions, there is limited information on structural variation, such as CNVs. Whole genome sequencing will generate a complete catalog of the variation, but many issues concerning the management, analysis, and interpretation of the huge volumes of data generated are not yet resolved.
In recent years, ~30 genes and gene regions have been confirmed as containing variants underlying susceptibility to breast cancer. The majority of recent discoveries have been low-penetrance common variants identified through GWAS with SNPs. Disease risks associated with these SNPs are low, typically much <1.5-fold. In many cases, the causal variant is unknown, and the associated marker is only in linkage disequilibrium with the actual site. In the majority of the cases, the role of these variants in causing disease is also unknown, but ongoing study is revealing novel insights into breast cancer biology. Areas of intensive research include investigation of the genetic basis of disease subtypes, for which there appear to be marked genetic differences, the impact of genetic variation on prognosis and on response to treatment. Despite the huge amount of work undertaken thus far, ~70% of the disease heritability remains unexplained. The common, low-penetrance variants identified through GWAS have contributed only a small proportion of this missing heritability. Aside from rare variants in the BRCA1 and BRCA2 genes and a small number of other rare genes that show approximately Mendelian patterns of inheritance, the majority of breast cancer genes found contribute little toward the prediction of individual disease risk. A thorough understanding of the biological role of the variation detected is some way off, and much more detailed functional and bioinformatic analysis is required for further progress. In the meantime, analysis of breast cancer exomes to identify SNPs and insertion–deletion polymorphisms will provide important insights by providing the first opportunity to examine rarer forms of variation in coding regions. This strategy will be effective for variants with higher penetrance, but where penetrance is toward the lower end of the spectrum, interpretation of the roles of numerous rarer variants will present new challenges for bioinformatic and functional assays. Once these problems are resolved, exome and whole genome sequencing strategies are likely to offer the best opportunity to identify additional breast cancer genetic risk factors. The identification of these genes is the crucial first step in fully comprehending the biology of disease and moving toward individualized treatments.
The authors report no conflicts of interest in this work.
- U.S. Department of Health & Human Services

- Virtual Tour
- Staff Directory
- En Español
You are here
Nih research matters.
June 4, 2024
Gene variants and breast cancer risk in Black women
At a glance.
- In the largest study of its kind, researchers identified genetic variants that appear to boost breast cancer risk among females of African ancestry.
- The findings could help improve risk prediction in this population and lead to more targeted therapies and prevention strategies.

Breast cancer is the most often diagnosed cancer in many parts of the world, including the U.S. More than 310,000 new cases are expected nationwide this year.
Black women tend to develop breast cancer at a younger age than White women. Black women are also more likely than Whites to die from the disease, and they are twice as likely to develop an aggressive subtype called triple-negative breast cancer. But despite the increased risks faced by women of African descent, most large-scale genetic studies of breast cancer to date have focused on women of European ancestry.
To better understand their unique genetic risks, a research team led by Dr. Wei Zheng of Vanderbilt University analyzed genetic data from over 40,000 females of African descent. About 18,000 had been diagnosed with breast cancer. The data were gathered as part of the NIH-funded African Ancestry Breast Cancer Genetic consortium, which combined data from 26 studies. Most participants (85%) were African Americans. The rest were from Barbados or Africa.
The researchers conducted a genome-wide association study (GWAS) to look for genetic variants that are found more often in participants with breast cancer than in those without. This is believed to be the largest GWAS study to date of breast cancer in this population. Results were reported in Nature Genetics on May 13, 2024.
The analysis pinpointed 12 genetic regions, or loci, associated with breast cancer. Three of these loci were linked to the aggressive triple-negative cancer. About 8% of the women carried two genetic copies of risk variants in all three of these loci. Such women, the researchers found, were 4.2 times more likely to be diagnosed with triple-negative breast cancer than women who had only one or no copies of the variants.
Because this type of cancer lacks specific cell receptors often seen with breast cancer (like estrogen or HER2 receptors), there are fewer targeted options for treatment. These findings may help researchers identify new treatment targets.
The researchers also confirmed many breast cancer risk variants that were found earlier in other populations. And they identified an uncommon risk variant in the gene ARHGEF38 , which had been previously linked to aggressive prostate and lung cancers.
The scientists used their findings to create polygenic risk scores (PRS) for breast cancer risk in females of African descent. PRS use genomic data to gauge the chance that a person will develop a certain medical condition. PRS created previously, using results from other populations, tend to perform poorly at predicting breast cancer risk for Black women. The new PRS, based on genomic data from African descendants, outperformed previous PRS at predicting breast cancer risk in this population.
The findings and data could lead to improved detection of breast cancer in this at-risk population and provide clues for potential treatment targets. Studies with even larger, more diverse populations will be needed to further improve the prediction of breast cancer risk.
“We have worked with researchers from more than 15 institutions in the U.S. and Africa to establish this large genetic consortium,” Zheng says. “Data put together in this consortium have been and will continue to be used by researchers around the world.”
—by Vicki Contie
Related Links
- Human Pangenome Boosts Accuracy and Reflects Diversity
- Technique May Improve Detection of Breast Tumors
- Test Predicts Whether Chemotherapy Will Help Early-Stage Breast Cancer Patients
- Advances in Breast Cancer: Screening and Treatment Get Personal
- Breast Cancer
- Polygenic Risk Scores
References: Genome-wide association analyses of breast cancer in women of African ancestry identify new susceptibility loci and improve risk prediction. Jia G, Ping J, Guo X, Yang Y, Tao R, Li B, Ambs S, Barnard ME, Chen Y, Garcia-Closas M, Gu J, Hu JJ, Huo D, John EM, Li CI, Li JL, Nathanson KL, Nemesure B, Olopade OI, Pal T, Press MF, Sanderson M, Sandler DP, Shu XO, Troester MA, Yao S, Adejumo PO, Ahearn T, Brewster AM, Hennis AJM, Makumbi T, Ndom P, O'Brien KM, Olshan AF, Oluwasanu MM, Reid S, Butler EN, Huang M, Ntekim A, Qian H, Zhang H, Ambrosone CB, Cai Q, Long J, Palmer JR, Haiman CA, Zheng W. Nat Genet. 2024 May;56(5):819-826. doi: 10.1038/s41588-024-01736-4. Epub 2024 May 13. PMID: 38741014.
Funding: NIH’s National Cancer Institute (NCI).
Connect with Us
- More Social Media from NIH
For the best browsing experience please enable JavaScript. Instructions for Microsoft Edge and Internet Explorer , other browsers
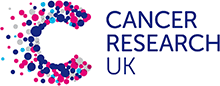
- About cancer
- Get involved
- Our research
- Funding for researchers
- Cancer types
- Cancer in general
- Causes of cancer
- Coping with cancer
- Health professionals
- Do your own fundraising
- By cancer type
- By cancer subject
- Our funding schemes
- Applying for funding
- Managing your research grant
- How we deliver our research
- Find a shop
- Shop online
- Our eBay shop
- Our organisation
- Current jobs
- Cancer news
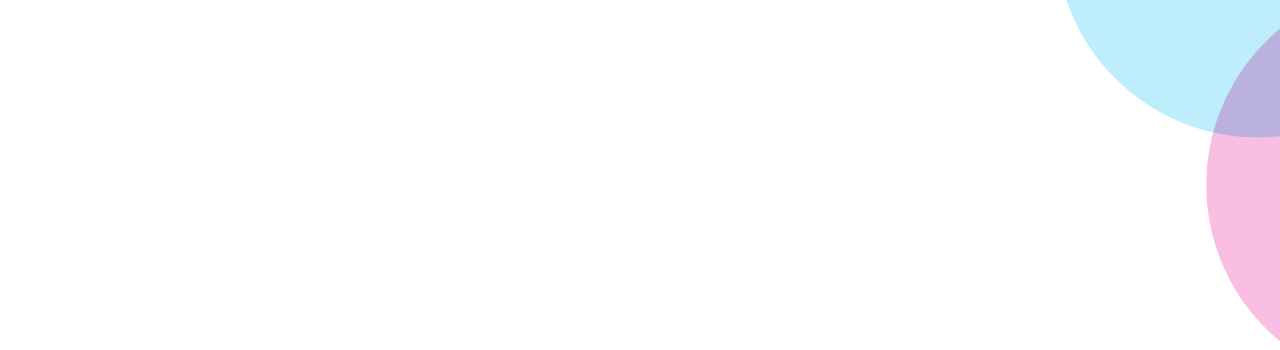
Family history of breast cancer and inherited genes
Some people have a higher risk of developing breast cancer than the general population because other members of their family have had particular cancers. This is called a family history of cancer.
Having a mother, sister or daughter (first degree relative) diagnosed with breast cancer approximately doubles the risk of breast cancer. This risk is higher when more close relatives have breast cancer, or if a relative developed breast cancer under the age of 50. But most women who have a close relative with breast cancer will never develop it.
UK guidelines help GPs to identify people who might have an increased risk of cancer due to their family history.
Referral to a specialist
Your GP will refer you to a specialist breast clinic or genetics clinic for assessment if you have any of the following:
- one first degree female relative diagnosed with breast cancer aged younger than 40 (a first degree relative is your parent, brother or sister, or your child)
- one first degree male relative diagnosed with breast cancer at any age
- one first degree relative with cancer in both breasts where the first cancer was diagnosed aged younger than 50
- two first degree relatives, or one first degree and one second degree relative, diagnosed with breast cancer at any age (second degree relatives are aunts, uncles, nephews, nieces, grandparents, and grandchildren)
- one first degree or second degree relative diagnosed with breast cancer at any age and one first degree or second degree relative diagnosed with ovarian cancer at any age (one of these should be a first degree relative)
- three first degree or second degree relatives diagnosed with breast cancer at any age
Your GP should also refer you if you have one first degree or second degree relative diagnosed with breast cancer when they were older than 40 years and one of the following:
- the cancer was in both breasts (bilateral)
- the cancer was in a man
- ovarian cancer
- Jewish ancestry
- sarcoma (cancer of the bone or soft tissue) in a relative younger than age 45 years
- a type of brain tumour called glioma or childhood adrenal cortical carcinomas
- complicated patterns of multiple cancers diagnosed at a young age
- two or more relatives with breast cancer on your father's side of the family
- Read NICE guidance about on increased risk due to family history
Breast cancer genes

Other genes that could increase your risk of developing breast cancer if they have a fault include:
- Find out more about breast cancer genes
Having one of these faulty genes means that you are more likely to get breast cancer than someone who doesn’t. But it is not a certainty.
Remember that most breast cancers happen by chance. Researchers estimate that only around 5 to 10 out of 100 breast cancers (5 to 10%) are caused by an inherited faulty gene.
- Read about testing for inherited cancer genes
Do I need extra screening?
Cancer screening is a test that looks for early signs of cancer in healthy people. Staff at the breast or genetics clinic can work out your risk of developing breast cancer. They can then tell you whether you might need extra screening.
- Find out more about breast screening
Related links
Risk factors for breast cancer.
Factors that increase the risk of breast cancer include getting older and inherited faulty genes. Read about these and other risk factors.
Breast screening
Find out about the UK breast screening programme, who has screening, and how you have it.
Inherited cancer genes and increased cancer risk
3-10% of cancers are caused by inherited genes in the UK. Genes can affect cancer risk, and some can be tested for.
What is breast cancer?
Breast cancer is cancer that starts in the breast tissue. Find out about who gets breast cancer and where it starts.
Symptoms of breast cancer
Symptoms of breast cancer include a lump or thickening in the breast. Find out more about this and other possible symptoms and when you should see your GP.
Breast cancer main page
Find out about breast cancer, including symptoms, diagnosis, treatment, survival, and how to cope with the effects on your life and relationships.
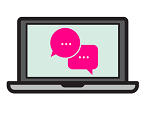
It’s a worrying time for many people and we want to be there for you whenever - and wherever - you need us. Cancer Chat is our fully moderated forum where you can talk to others affected by cancer, share experiences, and get support. Cancer Chat is free to join and available 24 hours a day.
Visit the Cancer Chat forum

About Cancer generously supported by Dangoor Education since 2010.
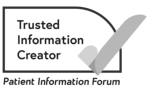
Find a clinical trial
Search our clinical trials database for all cancer trials and studies recruiting in the UK

Cancer Chat forum
Talk to other people affected by cancer

Nurse helpline 0808 800 4040
Questions about cancer? Call freephone 9 to 5 Monday to Friday or email us
Enhancing Breast and Ovarian Cancer Care: The Discovery of BRCA1 and BRCA2
- Research funded by the National Cancer Institute (NCI) helped to identify inherited BRCA1 and BRCA2 harmful gene mutations and to develop a screening test for these mutations in patients. These gene mutations are associated with an often aggressive form of breast cancer as well as ovarian cancer.
- Identification of the BRCA1 gene mutation by NCI-supported scientists as a hereditary predisposition to breast cancer was a landmark discovery.
- As a result of NCI's investment, people with a family history of breast and ovarian cancer can use their BRCA test results to help make informed decisions about screening and potential risk-reducing treatment.
- The finding that a small but significant number of cancers are caused by hereditary mutations also marked a turning point in determining the genetic basis of many forms of cancer. Continued support of a wide range of research activities, including a focus on BRCA1 and BRCA2 genes, is needed to advance the science behind cancer prevention and care.
Pathway to Discovery
Researchers long suspected that the risk of developing certain forms of cancer was inherited. Epidemiological evidence clearly showed that early-onset breast and ovarian cancers disproportionately affected women from certain families and populations, such as Ashkenazi Jews. This hinted at a hereditary cause for these types of cancer and suggested that a specific gene mutation could be the culprit.
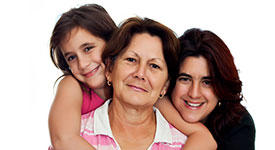
BRCA gene mutations are inherited
Early genetic research by NCI-supported investigators Mary-Claire King, Ph.D., Mark Skolnick, Ph.D., and their colleagues associated a DNA region with hereditary breast cancer. Researchers then identified the BRCA1 gene within this region, which established a clear association between inheriting the mutant forms of the gene (known as germline mutations ) with increased cases of cancer. Later, germline mutations identified in another gene, BRCA2 , were also associated with an increased risk of breast and ovarian cancers.
BRCA1 and BRCA2 mutations can lead to cancer
Both BRCA1 and BRCA2 belong to a class of genes known as tumor suppressor genes that function to prevent the growth of cancerous cells. Both play a role in cellular pathways that repair damaged DNA. Mutations in these pathways make cells more likely to accumulate DNA damage because they are less effective at repairing cells. This accumulated damage can lead to cancer.
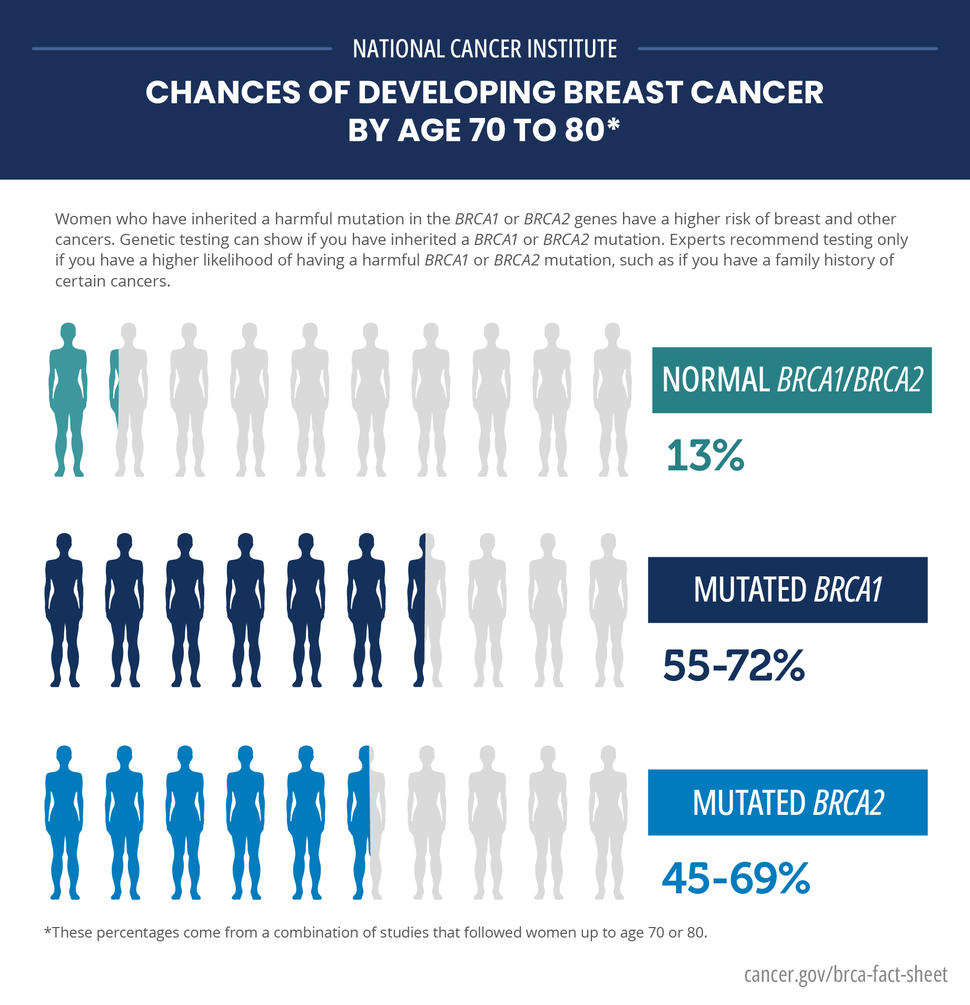
Enhancing Cancer Care
Having a hereditary susceptibility does not mean that a person will develop a specific cancer, but the risk of developing that cancer may be higher for that person. Consequently, the identification of BRCA1 and BRCA2 mutations has provided some important changes in breast cancer screening and prevention. Now testing for these genes in affected families can help to inform decisions about preventive treatment.
Women with direct family members who have BRCA1 and BRCA2 mutations now have the option of being tested to find out if they are at increased risk for developing breast and ovarian cancers. But the decision to be screened for inherited gene mutations is not a simple one and dealing with the test results can be difficult.
Additionally, interpreting the results of genetic tests , including BRCA1 and BRCA2 mutations, is not always straightforward. Genetic counselors and other health care professionals can help by explaining complex test results to make them more meaningful to patients and their families.
Several studies have shown that BRCA1 and BRCA2 testing and risk assessment can be integrated into primary care. For instance, a screening approach followed by preventive interventions—such as risk-reducing surgery, when appropriate—may reduce the number of new cases of breast and/or ovarian cancers as well as the number of deaths from these cancers.
Turning Discovery into Health
Since the initial discovery that BRCA1 and BRCA2 gene mutations are linked to hereditary breast and ovarian cancers, genetic testing has been used to determine the potential or likelihood that family members are at increased risk of developing cancer. If cancer is present, it has also been used to determine whether the cancer was caused by a BRCA1 or BRCA2 mutation.
Research also has shown that BRCA1 and BRCA2 mutations may affect a tumor’s response to treatment. Current NCI-supported studies are examining whether rapid testing for BRCA1 and BRCA2 mutations along with diagnosis can inform treatment.
Preclinical studies and phase I and II clinical trials have shown that platinum chemotherapy , like cisplatin , and poly(ADP)-ribose inhibitors (PARP inhibitors), are effective at treating BRCA1 and BRCA2 breast cancers. The DNA damage caused by platinum chemotherapeutics cannot be properly repaired in cells with defective BRCA1 or BRCA2 genes, resulting in the death of those cells.
PARP inhibitors are part of a new generation of chemotherapy drugs that function by synthetic lethality. Alone, this drug does not cause cell death but it will do so in combination with another defect such as the BRCA mutation. The findings from trials testing PARP inhibitors—alone or in combination with platinum chemotherapy drugs—are promising.
Research to Practice: NCI's Role
- The identification of germline mutations in the BRCA1 gene as a hereditary predisposition to breast cancer by NCI-supported scientists was a landmark discovery.
- The finding that a small but significant number of cancers are caused by hereditary mutations was a critical development in determining the genetic underpinnings of many forms of cancer.
- Since the initial discovery, NCI continues to support a wide range of research into the function of the BRCA1 and BRCA2 genes.
Key Takeaway
Families with a history of breast and ovarian cancer can now use genetic test results to make informed decisions about screening and potential risk-reducing treatment in the fight against cancer.
Selected Resources
Clark CC, Weitzel JN, O’Connor TR. Enhancement of synthetic lethality via combinations of ABT-888, a PARP inhibitor, and carboplatin in vitro and in vivo using BRCA1 and BRCA2 isogenic models. Mol Cancer Ther. 2012;11(9):1948-1958. [ PUBMED Abstract ]
Hall JM, Lee MK, Newman B, et al. Linkage of early-onset familial breast cancer to chromosome 17q21. Science . 1990;250(4988):1684-1689. [ PUBMED Abstract ]
Nelson HD,Huffman LH, Fu R, Harris EL, Walker M, Bougatsos C. Genetic risk assessment and BRCA mutation testing for breast and ovarian cancer susceptibility. Evidence Syntheses, No. 37 . Oregon Evidence-based Practice Center, Portland, OR. Rockville (MD): Agency for Healthcare Research and Quality. September 2005. [ NCBI Bookshelf ]
Pruthi S, Gostout BS, Lindor NM. Identification and management of women with BRCA mutations or hereditary predisposition for breast and ovarian cancer. Mayo Clin Proc. 2010;85(12):1111-1120. [ PUBMED Abstract ]
Petrucelli N, Daly MB, Feldman GL. BRCA1 and BRCA2 hereditary breast and ovarian cancer. GeneReviews™ [Internet]. Initial posting: September 4, 1998; Last update: September 26, 2013. [ NCBI Bookshelf ]
Rebbeck TR, Kauff ND, Domchek SM. Meta-analysis of risk reduction estimates associated with risk-reducing salpingo-oophorectomy in BRCA1 or BRCA2 mutation carriers. J Natl Cancer Inst . 2009; 101(2): 80-87. [ PUBMED Central ]
Sigal BM, Munoz DF, Kurian AW, Plevritis SK.A simulation model to predict the impact of prophylactic surgery and screening on the life expectancy of BRCA1 and BRCA2 mutation carriers . Cancer Epidemiol Biomarkers Prev. 2012;21(7):1066-1077. [ PUBMED Abstract ]
Wevers MR, Ausems MG, Verhoef S, et al. Behavioral and psychosocial effects of rapid genetic counseling and testing in newly diagnosed breast cancer patients: Design of a multicenter randomized clinical trial. BMC Cancer. 2011;11:6. [ PUBMED Abstract ]
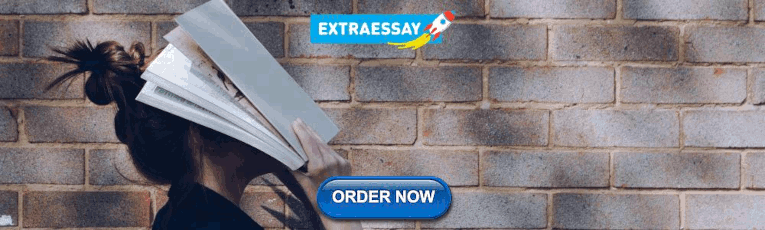
IMAGES
COMMENTS
Breast cancer (BC) genetics has become a fundamental aspect of BC management. It influences screening, follow-up, prophylactic and therapeutic recommendations in women harboring a germinal BC susceptibility gene. ... This Special Issue consists of one case report, two original research articles and five reviews, covering both diagnostic aspects ...
In 1994, the first gene associated with breast cancer - BRCA1 (for BReast CAncer1) was identified on chromosome 17. A year later, a second gene associated with breast cancer - BRCA2 - was discovered on chromosome 13. ... Further research showed that three specific mutations in these genes accounted for 90 percent of the BRCA1 and BRCA2 variants ...
Genetic testing for breast cancer susceptibility is widely used, but for many genes, evidence of an association with breast cancer is weak, underlying risk estimates are imprecise, and reliable sub...
A transcriptome-wide association study identifies associations of genetically predicted gene expression with breast cancer risk. This analysis finds 48 candidate genes implicated in breast cancer ...
Several genetic mutations were reported to be highly associated with an increased risk of breast cancer. Two major genes characterized by a high penetrance are BRCA1 (located on chromosome 17) and BRCA2 (located on chromosome 13). They are primarily linked to the increased risk of breast carcinogenesis . The mutations within the above-mentioned ...
The Confluence Project, from NCI's Division of Cancer Epidemiology and Genetics (DCEG), is developing a research resource that includes data from thousands of breast cancer patients and controls of different races and ethnicities. This resource will be used to identify genes that are associated with breast cancer risk, prognosis, subtypes ...
Metrics. ATM, BRCA1, BRCA2, CHEK2, PALB2 and TP53 are all established breast cancer susceptibility genes. Over the past 30 years, many other genes have been proposed as candidates. In these two ...
Purpose Decades of research have identified multiple genetic variants associated with breast cancer etiology. However, there is no database that archives breast cancer genes and variants responsible for predisposition. We set out to build a dynamic repository of curated breast cancer genes. Methods A comprehensive literature search was performed in PubMed and Google Scholar, followed by data ...
Breast cancer susceptibility gene 1 ( BRCA1) is a tumor suppressor gene, which is mainly involved in the repair of DNA damage, cell cycle regulation, maintenance of genome stability, and other important physiological processes. Mutations or defects in the BRCA1 gene significantly increase the risk of breast, ovarian, prostate, and other cancers ...
The top five genes (NAT1, PPM1H, AREG, ACSS1, CXCL1) with the greatest differences in Z-scores were evaluated in the PIK3CA mutant breast cancer samples from TCGA (Fig. 4E). Of the top 5 genes, AREG was the only gene with significant differential expression between the PIK3CA mutations.
Significantly mutated genes in breast cancer. Overall, 510 tumours from 507 patients were subjected to whole-exome sequencing, identifying 30,626 somatic mutations comprised of 28,319 point ...
The group concluded that HER2 might play a role in the development and growth of breast cancer. NCI-funded researcher Dennis J. Slamon, M.D., discovered the genetic link between HER2 and breast cancer. This led researchers to a groundbreaking hypothesis: If HER2 could be blocked, the growth of HER2-positive breast cancer might be slowed.
Stanford Medicine researchers found that inherited gene sequences can predict what type of breast cancer a patient is likely to develop, along with how aggressive that cancer may be. Emily Moskal A Stanford Medicine study of thousands of breast cancers has found that the gene sequences we inherit at conception are powerful predictors of the ...
Pathogenic variants in 12 established breast cancer-predisposition genes were detected in 5.03% of case patients and in 1.63% of controls. Pathogenic variants in BRCA1 and BRCA2 were associated ...
A woman's lifetime risk of developing breast and/or ovarian cancer is markedly increased if she inherits a harmful variant in BRCA1 or BRCA2, but the degree of increase varies depending on the mutation.. Breast cancer: About 13% of women in the general population will develop breast cancer sometime during their lives ().By contrast, 55% - 72% of women who inherit a harmful BRCA1 variant ...
Those include BRCA1 and BRCA2, which occur in about one of every 500 women and confer an increased risk of breast or ovarian cancer, and rarer mutations in a gene called TP53 that causes a disease ...
A large international group of researchers used a recently published gene aggregation method to uncover new genes implicated in the development of breast cancer. Two of the coauthors are with the Population Science team at the American Cancer Society (ACS): Lauren Teras, PhD, and Alpa Patel, PhD. They published their results in Genome Medicine.
BRCA Mutation Risks. It is estimated that one in eight women, or approximately 12%, will be diagnosed with breast cancer in her lifetime. However, women with certain genetic mutations have a higher lifetime risk of the disease. It's estimated that 55 - 65% of women with the BRCA1 mutation will develop breast cancer before age 70.
The term "breast cancer genes" means genes that, when altered (mutated), increase your risk of getting breast cancer. These gene mutations shut down some of your natural cancer-fighting genes ...
Some people inherit changes (mutations) in certain genes that increase their risk of breast cancer (and possibly other cancers). Genetic testing can look for mutations in some of these genes. While it can be helpful in some cases, not everyone needs to be tested, and each person should carefully consider the pros and cons of testing.
Further research is needed to know how variants on these genes affect breast cancer risk. Breast cancer is the most common cancer globally, accounting for around 12.5% of new cancer cases worldwide.
Within families with cancer in multiple generations, it had been estimated previously that a woman with an alteration in the BRCA1 gene has about an 85 percent chance of developing breast cancer and a 44 percent chance of developing ovarian cancer by age 70. Prior research in these high-risk families reported that women with BRCA2 alterations ...
On this page. [ show] Gene expression tests are a form of personalized medicine - a way to learn more about your cancer and tailor your treatment. These tests are done on breast cancer cells after surgery or biopsy to look at the patterns of a number of different genes. This process or test is sometimes called gene expression profiling.
A closer look at the 76 genetic studies identified 2,870 genetic variants in 445 genes linked to breast cancer. Thirteen of these genes showed different changes in different types of breast cancer ...
The genetic factors known to be involved in breast cancer risk comprise about 30 genes. These include the high-penetrance early-onset breast cancer genes, BRCA1 and BRCA2, a number of rare cancer syndrome genes, and rare genes with more moderate penetrance.A larger group of common variants has more recently been identified through genome-wide association studies.
But despite the increased risks faced by women of African descent, most large-scale genetic studies of breast cancer to date have focused on women of European ancestry. To better understand their unique genetic risks, a research team led by Dr. Wei Zheng of Vanderbilt University analyzed genetic data from over 40,000 females of African descent.
Breast Cancer Risk Genes — Association Analysis in More than 113,000 Women Breast Cancer Association Consortium New England Journal of Medicine, February 2021. Volume 384, Issue 5, Pages 428 to 439 ... Cancer Research UK is a registered charity in England and Wales (1089464), Scotland (SC041666), the Isle of Man (1103) and Jersey (247). A ...
BRCA gene mutations are inherited. Early genetic research by NCI-supported investigators Mary-Claire King, Ph.D., Mark Skolnick, Ph.D., and their colleagues associated a DNA region with hereditary breast cancer. Researchers then identified the BRCA1 gene within this region, which established a clear association between inheriting the mutant forms of the gene (known as germline mutations) with ...
Last week, researchers published a genome-wide association study on breast cancer in roughly 40,000 people of African descent in Nature Genetics, marking a leap forward in scientists' knowledge ...
A new type of liquid biopsy test can predict the recurrence of breast cancer in high-risk patients, months or even years before they relapse, research by a team from The Institute of Cancer Research, London, has shown. ... (WES) as it focuses on the exons - the protein-coding regions of genes - which are directly related to diseases ...