
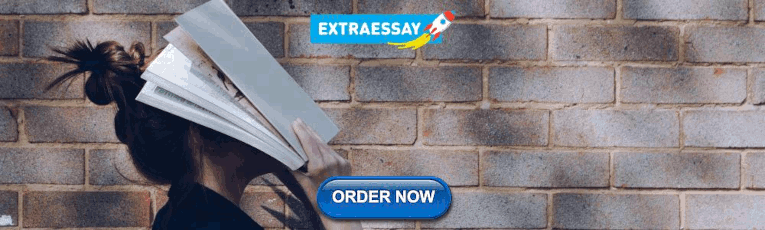
Application for our joint international PhD program: ATLAS - Talent Academy for TransLAtional Science is now open
ATLAS, the joint PhD program between TRON and BioNTech provides a unique chance for young researchers to pursue their scientific career in the field of translational science. The call is now open for application until October 31st, 2023 .
We are happy to offer 9 new PhD projects in this call. While the focus on “translation” is combined in all of them, the projects are based in various scientific fields: immunotherapy, computational biology, aging & regenerative biomedicine and cardiovascular & infectious diseases.
Students will not only get comprehensive training in cutting-edge technologies but will also be trained in transferable skills that are important for a successfull career in academia or industry.
Please check out the ATLAS website for information about the projects, general information about the program, application details or benefits of being an ATLAS PhD student ➡ https://atlas-phdprogram.com/how-to-apply
Modtag jobnotifikationer der matcher dine præferencer.
38 Ph.d. stillinger i Tyskland
Find Ph.d. stilling i Tyskland her. Tilmeld dig vores jobnotifikationer for at få tilsendt job den dag de bliver slået op.
- Ph.d. positions in Köln (6)
- Ph.d. positions in München (5)
- Ph.d. positions in Jena (4)
- Ph.d. positions in Ilmenau (4)
- Ph.d. positions in Karlsruhe (2)
- Ph.d. positions in Berlin (2)
- Ph.d. positions in Erlangen (2)
- Ph.d. positions in Frankfurt (Oder) (2)
- Ph.d. positions in Freiburg im Breisgau (1)
- Ph.d. positions in Stuttgart (1)
Andre lande
- Ph.d. positions in Belgien (236)
- Ph.d. positions in Holland (102)
- Ph.d. positions in Sverige (85)
- Ph.d. positions in Norge (47)
- Ph.d. positions in Schweiz (38)
- Ph.d. positions in Luxembourg (26)
- Ph.d. positions in Frankrig (21)
- Ph.d. positions in Finland (20)
- Ph.d. positions in Østrig (11)
- Ph.d. positions in Danmark (6)
Søgeresultat (38)
PhD Positions in Cancer Research
Are you looking for excellent research opportunities for your PhD studies at the forefront of cancer research? The German Cancer Research Center (DKFZ) in Heidelberg invites international students holding a Master’s degree in (molecular) biology, ...
PhD Students
The CISPA Helmholtz Center for Information Security is looking for PhD Students in areas related to:Cybersecurity, Privacy and CryptographyMachine Learning and Data ScienceEfficient Algorithms and Foundations of Theoretical Computer ScienceSoftwar...
Three 2-year Postdoctoral Fellowships at the Zukunftskolleg
(Fulltime, E 13 TV-L)Reference No: 2024/114. The preferred start date is April 1st, 2025. Conditionally on the submission of an external grant, the position can be extended for an additional year. In principle, the position can be divided into two...
PhD position: Developing experiments to study ultrafast molecular photochemistry
A fully-funded PhD position in experimental molecular physics/physical chemistry is available in the group of Lukas Bruder at the University of Freiburg, Germany. The candidate will tackle important challenges in molecular photochemistry. Examples...
Research Assistant (f/m/d)
Upcoming PhD Positions at the International Max Planck Research School for Molecules of Life in Autumn 2024
The next application round will start in September 2024. Stay tuned!The International Max Planck Research School for Molecules of Life (IMPRS-ML) currently has an open call for fully funded PhD student positions in the areas of biochemistry, struc...
Upcoming PhD positions at the IMPRS for Molecular Organ Biology in Autumn 2024
Thanks to all the academics who submitted their application!The application deadline has passed and the selection process started. More information for the new call-out will come up during this Autumn 2024. Stay tuned!PhD Studies in the Heart of E...
Project Manager/PHD Position in Production Management
We specialize in facilitating the digital evolution of businesses towards Industry 4.0 and fostering data-driven circular economies. Our expertise lies in crafting IT ecosystems that streamline and enhance sustainability across all facets of opera...
Six PhD positions in quantum physics CRC 183 (theory) (f/m/x) Wiss2407-01 | Institute for Theoretical Physics
Six PhD positions in quantum physics CRC 183 (theory) (f/m/x) CRC 183 | Entangled States of Matter We are one of the largest and oldest universities in Europe and one of the most important employer...
Upcoming PhD positions at the CGA
Call for applications for up to 12 PhD positions at the Cologne Graduate School of Ageing Research opens soon!The Cologne Graduate School of Ageing Research (CGA) in Germany is a joint venture of the University of Cologne Excellence Cluster on Str...
Upcoming fully funded PhD Positions in Neurosciences and Translational Psychiatry research, including a residency/PhD track option for medical doctors
There are no tuition fees for this PhD program.We welcome applications through our online application portal starting on August 15, 2024 for a start in fall 2025. The application deadline will be on October 31, 2024. The International Max Planck R...
Upcoming Doctoral positions available at the Graduate School Life Science Munich as of September 2024
Stay tuned for the next application round which will start in September 2024!The Graduate School LSM offers an international doctoral program for motivated and highly qualified next-generation researchers at one of Europe’s top universities. LMU i...
PhD Student in Computational Biology (f/m/x) Wiss2406-21 | Cellular Networks and Systems Biology | CECAD | Faculty of Mathematics and Natural Sciences
PhD Student in Computational Biology (f/m/x) Cellular Networks and Systems Biology | CECAD We are one of the largest and oldest universities in Europe and one of the most important employers in our...
Doctoral Researcher (m/f/div) in Microbiome Systems Biology
Job AdvertisementLeibniz-HKI-29/2024The Leibniz Institute for Natural Product Research and Infection Biology (Leibniz-HKI) investigates the pathobiology of human-pathogenic fungi and identifies targets for the development of novel natural product-...
2 PhD positions (experiment + simulation) laser based additive manufacturing in space
Institute for Multiscale Simulation (MSS)Friedrich-Alexander-University Erlangen-Nürnberg (FAU), Erlangen, GermanyEnvironmentAt MSS, we investigate the multiscale physics of particulate systems. The international research team offers an interdisci...
PhD position (f/m/x) Wiss2407-05 | MESH - Multidisciplinary Environmental Studies in the Humanities
Faculty of Arts and Humanities PhD position (f/m/x) MESH - Multidisciplinary Environmental Studies in the Humanities We are one of the largest and oldest universities in Europe and one of the most ...
Doctoral Researcher (m/f/div) in Automated Processing of Bioimages
Job AdvertisementLeibniz-HKI-16/2024The Leibniz Institute for Natural Product Research and Infection Biology (Leibniz-HKI) investigates the pathobiology of human-pathogenic fungi and identifies targets for the development of novel natural product-...
Doctoral Researcher (m/f/div) in Computational Biology
Job AdvertisementLeibniz-HKI-15/2024The Leibniz Institute for Natural Product Research and Infection Biology (Leibniz-HKI) investigates the pathobiology of human-pathogenic fungi and identifies targets for the development of novel natural product-...
PhD position (f/m/x) in Biological Physics Wiss2406-27 | Institute for Biological Physics | Maier Group
Faculty of Mathematics and Natural Sciences PhD position (f/m/x) in Biological Physics Institute for Biological Physics | Group Maier We are one of the largest and oldest universities in Europe and...
A PhD position on fragmentation across scales: simulation of fragmentation processes
is available at theInstitute for Multiscale Simulationat the Friedrich-Alexander-University Erlangen-Nürnberg www.mss.cbi.fau.deEnvironment Opportunity to be a part of the interdisciplinary research training group FRASCAL as a member of Institute ...
PhD position (m/f/d) for the investigation of structural, optical, and thermal properties of SiGeSn alloys and their heterostructures
Job-ID: 0052/24 | Department: Materials Research | Salary: as per tariff (TV-L) | Working Time: 40h/week with part-time option | Limitation: initially 2 years with option of extension | Starting Date: as soon as possibleIHP is an institute of the ...
PhD position in plankton ecology (m/f/d); Aquatic Ecology
BackgroundPlanktonic organisms are at the base of aquatic food webs and, while being crucial organisms for ecosystem functioning, they are particularly sensitive to environmental change. The impact global change has on planktonic organisms, may in...
PhD Student Position (f/m/x) Wiss2407-09 | Chair of Organizational and Economic Psychology | Dep. of Psychology
Faculty of Human Sciences PhD Student Position (f/m/x) Chair of Organizational and Economic Psychology | Dep. of Psychology We are one of the largest and oldest universities in Europe and one of th...
More information for the new call-out will come up during Autumn 2024. Stay tuned!The International Max Planck Research School for Molecules of Life (IMPRS-ML) will have an open call for fully-funded PhD student positions in the areas of biochemis...
PhD position (m/f/d) for the electrical properties of graphene for the realization of advanced opto-electronic devices
Job-ID: 0041/24 | Department: Material Research | Salary: as per tariff (TV-L) | Working Time: 40h/week (part-time work option) | Limitation: initially 2 years with option of extension for three more years | Starting Date: as soon as possibleIHP i...
Computer Scientist (M.Sc./Dipl.-Inf.) as Research Associate (f/m/d)
In the Department of Economic Sciences and Media in the Virtual Worlds and Digital Games Group at the Ilmenau University of Technology (TU Ilmenau, Germany) is a vacancy for aComputer Scientist (M.Sc./Dipl.-Inf.) as Research Associate (f/m/d)as so...
PhD Position / Research Assistent (f/m/d) Development and characterization of a reaction device for NMR measurements under harsh process conditions
Karlsruhe Institute of Technology (KIT) – The Research University in the Helmholtz Association creates and imparts knowledge for the society and the environment. It is our goal to make significant contributions to mastering the global challenges o...
PhD student for the research of microbiological risks in food from aquaculture (f/m/d)
The German Federal Institute for Risk Assessment (BfR) is the national institute which prepares expert reports and opinions on questions of food, feed and chemical safety and consumer health protection in Germany on the basis of internationally re...
Research associate / PhD candidate (f/m/d)
Job sorteret efter område.
- Elektroteknik 225
- Molekylærbiologi 195
- Programmeringssprog 194
- Machine Learning 192
- Kunstig intelligens 178
- Cytologi 164
- Maskinteknik 157
- Materialelære 152
- Elektronik 147
- Biokemi 131
Job sorteret efter type
- Post doc 406
- Adjunkt / Lektor 170
- Professor 128
- Forsker 121
- Ingeniør 108
- Videnskabelig assistant 106
- Underviser 98
- Ledelse og management 61
- Tenure Track 48
Job sorteret efter land
- Belgien 399
- Sverige 293
- Holland 166
- Schweiz 117
- Marokko 115
- Tyskland 100
- Luxembourg 68
Job sorteret efter arbejdsgiver
- KU Leuven 144
- KTH Royal Institute of Techno... 126
- Mohammed VI Polytechnic Unive... 119
- Ghent University 83
- Karolinska Institutet 81
- Eindhoven University of Techn... 79
- University of Luxembourg 67
- ETH Zürich 55
- University of Antwerp 53
- # mitochondria
- # Stem Cells
Explore the frontiers of Biotechnology, Pharma industry and Science with BiotechReality.com. We publish news, reports, and articles. We are exclusively publishing fellowship and studentship updates to light up the way for graduates to find a suitable research position in academia or industry.
ATLAS – Joint PhD Program from BioNTech and TRON | 9 Fully funded PhD Positions
ATLAS is a joint PhD program by BioNTech and TRON (Translational Oncology Mainz), which are the leading immunotherapy institutions. Their joint international PhD program is seeking talented graduates as junior researchers from all nations.
- Click to share on Facebook (Opens in new window)
- Click to share on X (Opens in new window)
- Click to share on LinkedIn (Opens in new window)
- Click to email a link to a friend (Opens in new window)
- Click to print (Opens in new window)
Table of Contents
Their PhD program focuses on translational medicine with experienced faculties, they provide a supportive environment for the scholars to conduct cutting-edge research projects.
ATLAS is offering 9 PhD positions in biology , life science , biochemistry , chemistry , medicine , and computer science or related areas.
Benefits of joining ATLAS
1 . Collaborative interdisciplinary research between academia and pharmaceutical
2 . Opportunity to publish your research work in top journals.
3 . Doctoral degree from their parenting university
Who can apply?
- You should hold an M.Sc. degree or equivalent.
- You should have a strong passion for science.
- You are able to work well in an international, multi-disciplinary team and also capable of working independently.
- Professional proficiency in both written and spoken English]
Read carefully the instructions before applying.
Also Check: The University of Edinburgh, Scotland – PhD Openings – September 2023
Projects offered
Project Number | Title of the project | Unit/Location |
---|---|---|
P01 | Targeting metastasizing tumor cells for the prevention of metastasis (MTC-target) | Therapeutic cardiology (Mainz, Germany) |
P02 | RNA-based modulation of iPSC-derived cardiomyocytes for heart repair (RiPS-Cardio) | Therapeutic cardiology (Mainz, Germany) |
P03 | Functional characterization of a novel uveal melanoma target | Expression and cell biology (Mainz, Germany) |
P04 | Metabolism Directed Cancer Immunotherapy (MERCI) | Immunotherapies and Preclinical Research: Cancer Vaccines (Mainz, Germany) |
P05 | Development of the circular RNA platform for protein expression | RNA Optimization & Internal Contract Synthesis (Mainz, Germany) |
P06 | Generation and engineering of a mRNA-encoded broadly neutralizing antibody cocktail against HIV | BiMAB & IDV (Mainz, Germany) |
P07 | Whole-Genome Sequencing and individualised cancer treatment | Bioinformatics (Mainz, Germany) |
P08 | Development of an mRNA firetruck to promote multi-targeted individualized mRNA therapy for chronic pain recovery | Inflammation and Tissue Damage (Mainz, Germany) |
P09 | Optimizing mRNA sequence design by combining data mining from nature with novel algorithmic approaches (OptiRNA) | RNA Biochemistry and CMC Development (Mainz, Germany) |
Deadline: October 31 st 2023
Interviews: Pre-selected candidates will be invited to Mainz, Germany for two-day interviews
Published: 18-September-2023
Updated: 19-September-2023, 23:11
Editor's Desk
- Editor's Desk https://www.biotechreality.com/author/editorsdesk New research suggests an improved method for the detection of thyroid cancer
- Editor's Desk https://www.biotechreality.com/author/editorsdesk Discovery of new T cells and genes related to immune disorders
- Editor's Desk https://www.biotechreality.com/author/editorsdesk In a young kid, gene therapy halts the advancement of a rare hereditary disease
- Editor's Desk https://www.biotechreality.com/author/editorsdesk Phosphate findings in NASA's OSIRIS-REx samples
Related Articles
Editor's Desk
Amplified fragment length polymorphism - aflp | explained, university of copenhagen, denmark – fully funded phd positions – september 2023, princeton university, new jersey – postdoctoral positions | september 2023, phd studentship in lipid platform (biochemistry) – vtt finland | january 2024, university of helsinki – phd positions | october 2023, phd in chemical and biological engineering – colorado state university, usa, phd studentship in biomedical and clinical sciences – the university of queensland, australia – january 2024.
- Postdoctoral Fellowship in AI-based Integrative Modelling of Macromolecular Complexes – EMBL Hamburg
- Postdoctoral Position in RNA Biology of B Cells – Human Technopole, Milan
- PhD Position in Biotechnology – Ghent University | January 2025 Start
- Research Associate in Innate Immunity – University of Cambridge | September 2024 Start
- Postdoctoral Research in Biomedical Research | Ludwig Maximilian University of Munich (LMU)
Subscribe to Newsletter
BiotechReality.com
SciRealityPress
Disclaimer: The content on this website is not meant to provide medical or any other professional advice. Understand and use the information, double check if necessary.
About the employer
Atlas is an international, structured, graduate program with a strong focus on translational medicine. atlas covers immunotherapy, computational biology and infectious diseases..

ATLAS our Talent A cademy for T rans LA tional S cience, guides and supports PhD students to conduct excellent research projects and offers comprehensive training in cutting-edge technologies. In addition, we ensure that you learn the transferable skills needed for a successful career in academia or industry. Scientific training within the program has a strong focus on "the art of translation", i.e. turning basic scientific discoveries into applications.
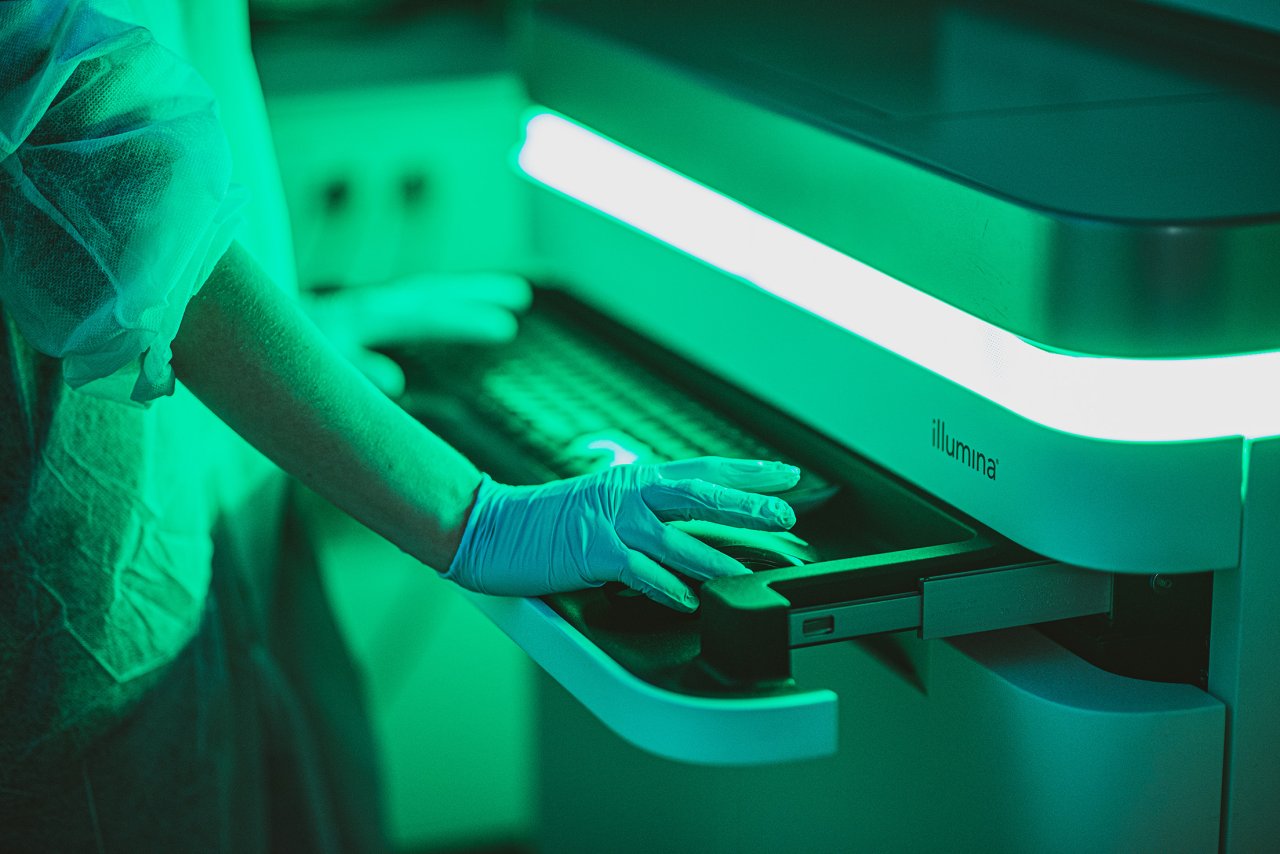
The main research topics at ATLAS evolve around:
- Immunotherapy of cancer, infectious diseases & autoimmunity
- RNA biochemistry
- Protein engineering
- Bioinformatics & systems biology
- Biomarker research
- Antimicrobial therapies
Employer location
Discover similar employers
This might interest you
Discover related jobs
Jobs by field
- Electrical Engineering 214
- Programming Languages 178
- Machine Learning 175
- Molecular Biology 165
- Artificial Intelligence 165
- Mechanical Engineering 149
- Materials Engineering 146
- Electronics 144
- Cell Biology 136
- Engineering Physics 116
Jobs by type
- Postdoc 365
- Assistant / Associate Professor 159
- Professor 121
- Researcher 109
- Research assistant 100
- Engineer 99
- Lecturer / Senior Lecturer 74
- Management / Leadership 57
- Tenure Track 47
Jobs by country
- Belgium 399
- Netherlands 166
- Switzerland 117
- Morocco 115
- Germany 100
- Luxembourg 68
Jobs by employer
- KU Leuven 144
- Mohammed VI Polytechnic Unive... 119
- Ghent University 83
- Eindhoven University of Techn... 79
- University of Luxembourg 67
- KTH Royal Institute of Techno... 59
- ETH Zürich 55
- University of Antwerp 53
- Karolinska Institutet 44
- TRON-Translationale Onkologie an der Universitätsmedizin der Johannes Gutenberg-Universität Mainz gemeinnützige GmbH
- Posted on: 15 September 2023
ATLAS - Joint PhD Program from BioNTech and TRON with a focus on translational medicine
Job information, offer description.
9 Fully funded PhD positions in biology, biochemistry, life science, chemistry, medicine, computer science or related fields BioNTech and TRON (Translational Oncology Mainz) are leading institutions in the field of immunotherapy. Our joint international PhD Program (ATLAS) is looking for talented, junior researchers of all nationalities . Our international PhD program ATLAS (Talent A cademy for T rans LA tional S cience), guides and supports PhD students to conduct excellent research projects and offers comprehensive training in cutting-edge technologies and professional skills. The scientific training within the program has a strong focus on "the art of translation", i.e. turning basic scientific discoveries into applications.
We offer the opportunity to work on innovative research projects focused on translational science in the field of medicine . As a PhD student*, you will join a community of world-class scientists working on diverse topics such as:
- Immunotherapy of cancer, infectious diseases & autoimmunity
- Cardiovascular, aging & regenerative biomedicine
- RNA biochemistry
- Protein engineering
- Bioinformatics & systems biology
- Biomarker research
- Antimicrobial therapies
The positions will be located in Mainz, Germany.
For details on the program and the specific projects, please visit www.atlas-phdprogram.com .
A short Testimonial video can be watched watched here.
We welcome applications from
- Aspiring students seeking to advance knowledge and explore novel therapies.
- We require: A master’s degree in Biology, Biochemistry, Chemistry, Life Science, Medicine, Immunology, Computer Science or Engineering related fields.
- Professional proficiency in both written and spoken English
Please carefully read " How to apply " and " Projects offered " before applying.
- Fully funded PhD positions with contracts with either BioNTech or TRON
- A highly collaborative and interdisciplinary research environment at the interface between academia and pharmaceutical applications
- A doctoral degree awarded by a partnering university (e.g. Mainz University)
- Dedicated supervision by leaders in their fields with a strong publication record
- Cutting-edge technologies and in-house services to support your research
- Extensive curriculum for scientific, technical and professional skills
- An active and engaging scientific and PhD community
- Possibility to directly be involved in the planning and organization of PhD events
- Excellent working conditions
- Family-friendliness
- Open and inclusive culture
- An international environment with English as our working language
How to apply
Then apply now and simply send us your application documents via our application portal .
- All mandatory documents (CV, letter of motivation, official transcript, and certificate of your master’s degree, if available) must be combined into one pdf file and uploaded on the field "*Resume/ CV" via the application portal.
- All other additional documents (e.g. reference letters, proof of proficiency in English, grading system of your home university, etc.) should be combined into one pdf file and uploaded on the field “Additional documents” via the application form.
Please watch our how to apply video here.
The deadline for applications is October 31st, 2023 . Interviews will take place in Mainz in February 2024. If you have any further questions, please visit www.atlas-phdprogram.com or email us at [email protected] . We look forward to your application!
Where to apply
Requirements, additional information, work location(s), share this page.

ATLAS: Advanced Translational Leadership in Academic Science
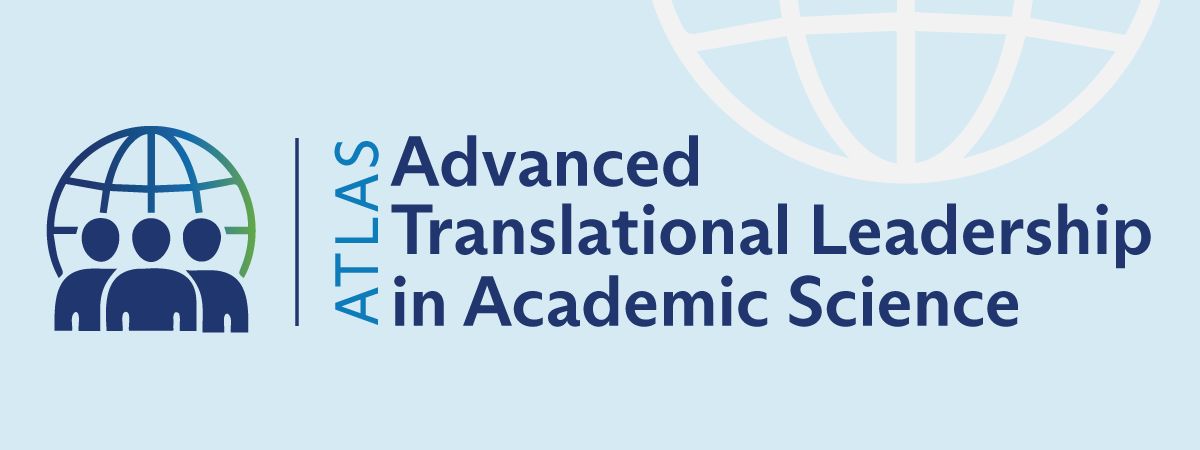
The ATLAS program is a year-long training program for both mid-career and senior investigators doing clinical or translational research.
The goals of ATLAS are to
- Increase collaboration among the partnering institutions
- Equip investigators with skills to lead team science.
This program also aims to:
- Provide an environment for new inter-institutional collaborations to be established
- Establish a group of researchers that can act as a resource to one another
- Provide knowledge of team science and reiterate the importance of doing multidisciplinary science in clinical and translational research.
The ATLAS program is modeled after the Leadership Innovation in Team Science (LITeS) program that originated at the University of Colorado and has since been running at the University of Minnesota.
Investigators will be nominated by their CTSC institutional research lead. We ask that research leads email [email protected] with the names and emails of their nominations during our application period ( deadlines for our 2025 cohort applications will be announced later in the year ). Individuals chosen for the program will then receive an email with a REDCap application. We will also allow self nominations. To do so, please contact the ATLAS Program: [email protected] .
2024 ATLAS nominations and applications are closed. View our 2024 ATLAS Cohort .
Participating institutions include Case Western Reserve University, Cleveland Clinic, VA Northeast Ohio Healthcare Systems, MetroHealth, Northeast Ohio Medical University, University Hospitals, and University of Toledo.
Components of the Program
- Investigators will be split into small groups consisting of 4-5 investigators (only 1 investigator from each partnering institution).
- Groups will take on a challenge that has been identified by leadership and work to establish a plan for solutions to this issue.
- Participation in a 360 evaluation and creating a plan based on the results of the evaluation
- Partaking in various assessments to understand how the investigators personality, behaviors, and conflict style ultimately influence their leadership
- Teach skills related to emotional intelligence, negotiation, teamwork, effective communication etc.
Meet our Team
Grace mccomsey.
Grace McComsey, MD is the Vice Dean for Clinical and Translational Research, Director of the Clinical and Translational Science Institute (CTSI), Principal Investigator of the Clinical and Translational Science Collaborative (CTSC) of Northern Ohio, and Professor of Pediatrics and Medicine at Case Western Reserve University. Dr. McComsey’s work focuses on HIV and Long-COVID complications and treatment.
McKenzie Ritter
McKenzie Ritter, PhD is the Director of the ATLAS program and the Assistant Director of Research Education and Training within the Clinical and Translational Science Collaborative at CWRU. She received her PhD in Physiology in the Spring of 2023 and has recently joined the CTSC (Summer 2023).
Judith Albino
Judith Albino, PhD is President Emerita and the former Director of the University of Colorado LITeS program. Dr. Albino is a health and community psychologist whose research focuses on health disparities and prevention interventions for oral health. She has brought her expertise from the LITeS program to facilitate sessions throughout the program.
Mary Dolansky
Mary Dolansky, PhD, RN is a Sarah C. Hirsh Professor in the CWRU School of Nursing, an Associate Professor in the Department of Population and Quantitative Health Sciences at CWRU, the Director of the QSEN Institute in the CWRU School of Nursing, Senior Faculty Scholar for the VA Quality Scholars Program, and Lead of the CTSC Workforce Development module. Dr. Dolansky’s work focuses on implementation science, interprofessional education, and quality and safety.
David Ingbar
David Ingbar, MD is the Director of the LITeS program at the University of Minnesota, the Assistant Dean for Faculty Development and Mentoring for the Office of Faculty Affairs, the Director of the Research Education, Training, and Career Development Core in the Clinical and Translational Science Institute (CTSI), and a Professor of Medicine in the Division of Pulmonary, Allergy, Critical Care and Sleep. Dr. Ingbar’s research focuses on lung repair following injury, and how that compares to lung development.
Tyler Reimschisel
Tyler Reimschisel, MD is the founding Associate Provost of Interprofessional and Interdisciplinary Education and Research, a Professor in the Department of Genetics and Genome Sciences at CWRU, a Physician for the Center for Human Genetics at University Hospitals, and a Physician for the Neurological Institute at Cleveland Clinic. Dr. Reimschisel is a team coach and focuses on interdisciplinary/interprofessional education.
Program Dates and Details
The program will consist of four 2-day sessions that will occur every 2 months. The first session will begin in March 2024.
2024 Program Dates
March 7 and 8, 2024
May 2 and 3, 2024
July 11 and 12, 2024
September 5 and 6, 2024
Program Completion
Attendance to all 8 sessions is mandatory. Upon successful completion of the program, investigators are eligible for a pilot award to be spent on research if a new research collaboration is established as a result of this program. Additionally, vouchers will also be available to investigators that successfully complete the entire program to be used towards an CTSC related service.
- Interesting for you
- My settings
ATLAS - Translational Medicine
ATLAS - Translational Medicine at Johannes Gutenberg University Mainz is an international graduate program with a strong focus on translational medicine. ATLAS covers immunotherapy, computational biology, infectious diseases, cardiovascular, aging and regenerative biomedicine.
Johannes Gutenberg University Mainz Multiple locations Mainz , Germany Top 2% worldwide Studyportals University Meta Ranking 4.1 Read 163 reviews
About the prorgam
ATLAS, our Talent Academy for TransLAtional Science, guides and supports PhD students to conduct excellent research projects and offers comprehensive training in cutting-edge technologies.
In addition, we ensure that you learn the transferable skills needed for a successful career in academia or industry. Scientific training within the program has a strong focus on "the art of translation", i.e. turning basic scientific discoveries into applications. The ATLAS - Translational Medicine PhD program is offered at Johannes Gutenberg University Mainz.
Programme Structure
- Immunotherapy of cancer, infectious diseases & autoimmunity
- Cardiovascular, aging & regenerative biomedicine
- RNA biochemistry
- Protein engineering
- Bioinformatics & systems biology
- Biomarker research
- Antimicrobial therapies
Key information
- 48 months
Start dates & application deadlines
- The starting date would be 1-4 months from the onsite interview days.
- This will also depend on the project and the project supervisor requirements.
Disciplines
Academic requirements.
We are not aware of any specific GRE, GMAT or GPA grading score requirements for this programme.
English requirements
We are not aware of any English requirements for this programme.
Student insurance
Make sure to cover your health, travel, and stay while studying abroad. Even global coverages can miss important items, so make sure your student insurance ticks all the following:
- Additional medical costs (i.e. dental)
- Repatriation, if something happens to you or your family
- Home contents and baggage
We partnered with Aon to provide you with the best affordable student insurance, for a carefree experience away from home.
Starting from €0.53/day, free cancellation any time.
Remember, countries and universities may have specific insurance requirements. To learn more about how student insurance work at Johannes Gutenberg University Mainz and/or in Germany, please visit Student Insurance Portal .
Other requirements
General requirements.
Who can apply?
- You hold (or expect to hold prior to the start of your PhD) an M.Sc. (or an equivalent degree) in Biology, Biochemistry, Chemistry, Life Science, Medicine, Immunology, Computer Science or related fields.
- You are a motivated researcher with a passion for science.
- You are able to work well in an international, multi-disciplinary team and also capable of working independently.
- You possess professional proficiency in both written and spoken English
- CV, letter of motivation, official transcript, and certificate of your master’s degree, if available
- reference letters, proof of proficiency in English, grading system of your home university etc.
Tuition Fee
International.
- fully funded; enrollment with the JGU incurs semester fees of approx. €330
Living costs for Mainz
The living costs include the total expenses per month, covering accommodation, public transportation, utilities (electricity, internet), books and groceries.
In order for us to give you accurate scholarship information, we ask that you please confirm a few details and create an account with us.
Scholarships Information
Below you will find PhD's scholarship opportunities for ATLAS - Translational Medicine.
Available Scholarships
You are eligible to apply for these scholarships but a selection process will still be applied by the provider.
Read more about eligibility
- missing or incomplete ?"> Missing or incomplete content
- wrong or outdated ?"> Wrong or outdated content
Other interesting programmes for you
Our partners.
Go to your profile page to get personalised recommendations!
Skip to Content
- News + Events
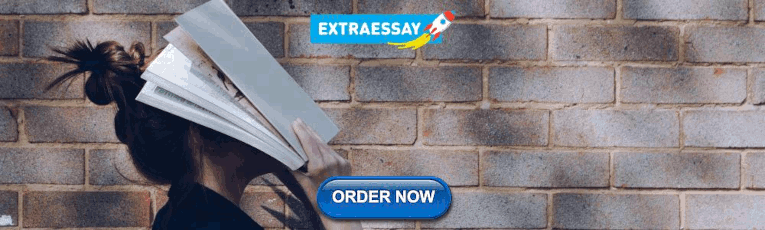
Other ways to search:
- Events Calendar

ATLAS PhD Program
The PhD program at CU Boulder’s ATLAS Institute emphasizes out-of-the-box invention and radical inquiry. Suited to students whose interests transcend traditional disciplinary boundaries, ATLAS is a thriving academic community of researchers and students who blend engineering, design, art and humanitarianism.
The University of Colorado Boulder has one of the top 12 public engineering graduate programs in the U.S., according to U.S. News and World Report ’s Best Graduate Schools rankings for 2024-25.
The ATLAS PhD program is sctructured like any other research-based doctoral program in which you:
- Form an advisory committee
- Complete course
- Pass a qualifying examination
- Propose a dissertation
- Conduct research
- Write and defend your dissertation
PhD Student Bios
Dissertations.
Submit your materials to receive feedback from an ATLAS faculty member before your apply to the PhD program.
Application Feedback Request
How to apply, request information, atlas research lab directors.
ATLAS lab directors advise PhD students, create collaborative environments and guide research across a broad range of multi-disciplinary fields.
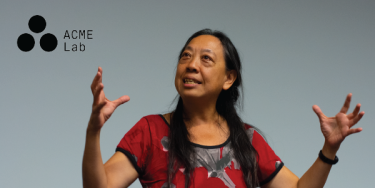
ACME Lab Ellen Yi-Luen Do, Director
The ACME Creativity Machine Environment Lab explores and develops computational tools for design, creativity, cognition, tangible and embedded interaction, and computing for health and wellness.
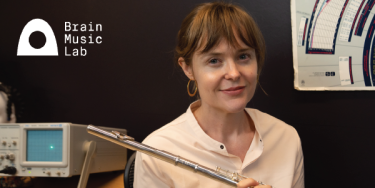
Brain Music Lab Grace Leslie, Director
The Brain Music Lab pursues research to promote health and well-being by combining new music technologies with biometric data from EEG brainwave data and other physiological measurement techniques.

Emergent Nanomaterials Lab Carson Bruns, Director
By synthesizing and combining nanoscale building blocks, the Emergent Nanomaterials Lab designs technologies that enhance the quality of human lives in health, sensory augmentation and self-expression.

Living Matter Lab Mirela Alistar, Director
Working at the intersection of biology, engineering and art, the Living Matter Lab interfaces with biological matter, the micro-world and the environment in unique ways.

TYPO Lab Joel Swanson, Director
TYPO Lab is an experimental studio for creative work and research related to the technologies of language. It empowers faculty and students to explore and appreciate the media and methods of words.
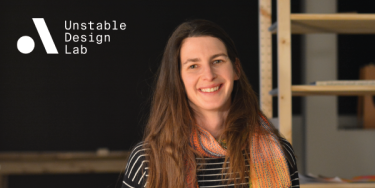
Unstable Design Lab Laura Devendorf, Director
An advocate for the arts within engineering fields, the Unstable Design Lab focuses on advancing textile design and fabrication, including the integration of circuitry and other technologies.

Utility Research Lab Michael Rivera, Director
The Utility Research Lab invents and investigates digital fabrication technology, tools and techniques that empower us to create things that positively impact people, society and the environment.
Required classes (to be taken during the first two years of study)
- ATLAS Research Methods
- ATLAS Research Professional Development
- ATLAS Colloquium at least once each during the first two years of study.
Expertise development
Beyond required classes, the curriculum is crafted for each student based on background and experience. You consult your advisory committee to identify skills needed to perform your research and select courses to develop that expertise.
PhD Application Fee Waiver
The College of Engineering and Applied Science at CU Boulder is once again offering a program that allows qualifying PhD applicants to waive their application fees until Nov. 15, 2024.
The program is intended to support our college's strategic vision to become a premier destination for U.S. graduate students.
Minimum requirements :
- Applicant must be a U.S. citizen or U.S. permanent resident
- Applicant must be applying for a PhD program in the College of Engineering and Applied Science
- Applicant must be applying to enroll in fall 2025
- Applicant must have at least a 3.4 GPA from their accredited undergraduate institution
- Applicant must meet all department requirements for their PhD programs
- Application must be submitted by 11:59 p.m. MST on Nov. 15, 2024
The offer will be applied automatically to qualifying students in the application portal; no code is needed. Questions may be directed to Meredith Canode, senior director of graduate programs , at [email protected].
Further details can be found on the College of Engineering and Applied Science Fee Waiver page .
Additional Opportunities
Combined PhD in Cognitive Science : ATLAS PhD students can earn a combined degree with the Institute of Cognitive Science .
Opportunity for underrepresented students : The Colorado Advantage Graduate Preview Weekend gives underrepresented students a chance to visit campus, meet faculty, and preview doctoral programs in science, math, and engineering departments at CU-Boulder. Application Deadline: The deadline has passed for 2024 admissions.
Opportunity for U.S. citizens, U.S. nationals and permanent residents : The National Science Foundation Graduate Research Fellowship Program provides three years of support over a five-year fellowship period for the graduate education of individuals who have demonstrated their potential for significant research achievements in STEM or STEM education. Deadlines in October.
Application Review Process
We consider three main criteria when reviewing applications:
- Do you have a clearly defined area of interest? We understand that over the course of PhD study, interests may shift. We won't hold you to your initial expressions of area of interest and we don't expect that you know the topic of your dissertation when you apply to the program. Still, having a clear idea of the topics to be explored in PhD study is a good indicator of future success.
- Do you have the background and skills to work in that area? We look at whether you are prepared to begin working on the topics you identify as your area of interest. For example, if you have a background in nuclear engineering and you plan to work on computer music, we'd like to know more about what skills you'll bring to bear on your new direction.
- Do we have the capacity to supervise work in that area? We look at whether we have faculty members who can supervise research in your area of interest. No matter how interesting the area of research and how capable you are to work in that area, we can only take students for whom we have the faculty to advise and supervise. We require a commitment from at least one faculty member to advise and fund any applicant we admit to the ATLAS PhD program.

An official website of the United States government
The .gov means it’s official. Federal government websites often end in .gov or .mil. Before sharing sensitive information, make sure you’re on a federal government site.
The site is secure. The https:// ensures that you are connecting to the official website and that any information you provide is encrypted and transmitted securely.
- Publications
- Account settings
- My Bibliography
- Collections
- Citation manager
Save citation to file
Email citation, add to collections.
- Create a new collection
- Add to an existing collection
Add to My Bibliography
Your saved search, create a file for external citation management software, your rss feed.
- Search in PubMed
- Search in NLM Catalog
- Add to Search
An atlas of transcribed enhancers across helper T cell diversity for decoding human diseases
Collaborators.
- Matteo Guerrini , Hiroaki Hatano , Kazuyoshi Ishigaki , Yuki Ishikawa , Shuichiro Komatsu , Michihiro Kono , Yasuhiro Murakawa , Masahiro Nakano , Akiko Oguchi , Raku Son , Akari Suzuki , Kazuhiro Takeuchi , Nao Tanaka , Chikashi Terao , Kohei Tomizuka , Kazuhiko Yamamoto , Soichiro Yoshino
Affiliations
- 1 RIKEN-IFOM Joint Laboratory for Cancer Genomics, RIKEN Center for Integrative Medical Sciences, Yokohama, Japan.
- 2 Institute for the Advanced Study of Human Biology, Kyoto University, Kyoto, Japan.
- 3 Department of Nephrology, Graduate School of Medicine, Kyoto University, Kyoto, Japan.
- 4 Laboratory for Autoimmune Diseases, RIKEN Center for Integrative Medical Sciences, Yokohama, Japan.
- 5 IFOM ETS - the AIRC Institute of Molecular Oncology, Milan, Italy.
- 6 Department of Immunology, Graduate School of Medicine, Kyoto University, Kyoto, Japan.
- 7 Genome Immunobiology RIKEN Hakubi Research Team, RIKEN Center for Integrative Medical Sciences, Yokohama, Japan.
- 8 Division of Molecular Pathology, Department of Cancer Biology, Institute of Medical Science, The University of Tokyo, Tokyo, Japan.
- 9 Laboratory of Complex Trait Genomics, Department of Computational Biology and Medical Sciences, Graduate School of Frontier Sciences, The University of Tokyo, Tokyo, Japan.
- 10 Laboratory for Statistical and Translational Genetics, RIKEN Center for Integrative Medical Sciences, Yokohama, Japan.
- 11 Department of Medical Systems Genomics, Graduate School of Medicine, Kyoto University, Kyoto, Japan.
- 12 Department of Gastroenterological Surgery, Yokohama City University Graduate School of Medicine, Yokohama City University, Yokohama, Japan.
- 13 K.K. DNAFORM, Yokohama, Japan.
- 14 Department of Hematology and Oncology, Graduate School of Medicine, Kyoto University, Kyoto, Japan.
- 15 Division of Precision Medicine, Kyushu University Graduate School of Medical Sciences, Kyushu University, Fukuoka, Japan.
- 16 Inter-Organ Communication Research Team, Institute for Life and Medical Sciences, Kyoto University, Kyoto, Japan.
- 17 Department of Nephro-Urologic Surgery and Andrology, Mie University Graduate School of Medicine, Mie University, Tsu, Japan.
- 18 Department of Biosciences and Nutrition, Karolinska Institutet, Stockholm, Sweden.
- 19 Stem Cells and Metabolism Research Program, University of Helsinki, Helsinki, Finland.
- 20 Folkhalsan Research Center, Helsinki, Finland.
- 21 Department of Radiation Genetics, Graduate School of Medicine, Kyoto University, Kyoto, Japan.
- 22 Shenzhen University School of Medicine, Shenzhen, Guangdong, China.
- 23 Department of Integrative Bioanalytics, Institute of Development, Aging and Cancer, Tohoku University, Sendai, Japan.
- 24 Research Center for Genome & Medical Sciences, Tokyo Metropolitan Institute of Medical Science, Tokyo, Japan.
- 25 Preventive Medicine and Applied Genomics Unit, RIKEN Center for Integrative Medical Science, Yokohama, Japan.
- 26 RIKEN Preventive Medicine and Diagnosis Innovation Program, Wako, Japan.
- 27 Laboratory for Human Immunogenetics, RIKEN Center for Integrative Medical Sciences, Yokohama, Japan.
- 28 Department of Medical Biotechnology and Translational Medicine, Università degli Studi, Milan, Italy.
- 29 Laboratory for Transcriptome Technology, RIKEN Center for Integrative Medical Sciences, Yokohama, Japan.
- 30 Human Technopole, Milan, Italy.
- 31 Clinical Research Center, Shizuoka General Hospital, Shizuoka, Japan.
- 32 Department of Applied Genetics, School of Pharmaceutical Sciences, University of Shizuoka, Shizuoka, Japan.
- PMID: 38963856
- DOI: 10.1126/science.add8394
Transcribed enhancer maps can reveal nuclear interactions underpinning each cell type and connect specific cell types to diseases. Using a 5' single-cell RNA sequencing approach, we defined transcription start sites of enhancer RNAs and other classes of coding and noncoding RNAs in human CD4 + T cells, revealing cellular heterogeneity and differentiation trajectories. Integration of these datasets with single-cell chromatin profiles showed that active enhancers with bidirectional RNA transcription are highly cell type-specific and that disease heritability is strongly enriched in these enhancers. The resulting cell type-resolved multimodal atlas of bidirectionally transcribed enhancers, which we linked with promoters using fine-scale chromatin contact maps, enabled us to systematically interpret genetic variants associated with a range of immune-mediated diseases.
PubMed Disclaimer
- Search in MeSH
Related information
Linkout - more resources, full text sources, research materials.
- NCI CPTC Antibody Characterization Program
- Citation Manager
NCBI Literature Resources
MeSH PMC Bookshelf Disclaimer
The PubMed wordmark and PubMed logo are registered trademarks of the U.S. Department of Health and Human Services (HHS). Unauthorized use of these marks is strictly prohibited.
UChicago data scientists update powerful cancer data sharing platform
July 10, 2024
The University of Chicago Center for Translational Data Science (CTDS) has completed an update to the National Cancer Institute’s Genomic Data Commons , a repository and computational platform for cancer researchers who seek to understand cancer, its clinical progression and response to therapy. This new version is known as GDC 2.0.
Designed by the University’s CTDS, the NCI’s GDC launched in 2016. The CTDS operates and updates the data commons via funding from a subcontract with Leidos Biomedical Research, Inc. (Leidos Biomed), operator of the Frederick National Laboratory for Cancer Research on behalf of the NCI. Funding is provided under Leidos Biomed’s Prime Contract 75N91019D00024.
Among the enhancements is the GDC Analysis Tool Software Development Kit (SDK). “The SDK makes it easy for the GDC to integrate the best tools developed by the cancer genomics research community,” said Robert Grossman, PhD , Frederick H. Rawson Distinguished Service Professor in Medicine and Computer Science, who leads the GDC program at the University’s CTDS. “By using the SDK, developers have a clear path to easily integrate their tools with the GDC, so they are available to the over 90,000 researchers who use it each month.”
Additional updates completed by the CTDS include:
- A cohort-centric workflow allowing users to build and save custom cohorts to use across GDC tools
- Gene expression clustering to visualize gene expression levels for a custom cohort and gene set
- ProteinPaint: to visualize somatic mutations as they appear on a gene or polypeptide
- Sequencing reads viewer: Display the reads within a GDC-harmonized alignment
- Cohort-level MAF tool: Aggregate somatic mutations into one file for a custom cohort
- Tool-based framework - Seamlessly move a cohort across analysis tools in GDC’s new analysis center
The GDC contains data generated by the NCI from some of the largest and most comprehensive cancer genomic datasets, including the Cancer Genome Atlas and Therapeutically Applicable Research to Generate Effective Therapies. The platform accepts data from cancer programs and individual researchers. Information is processed using standardized bioinformatics pipelines to align the data and to generate higher-level data such as variant calls and expression quantification. As more researchers add clinical and genomic data to the GDC, it will become an even more powerful tool for discoveries about the molecular basis of cancer that may lead to better care for patients.
For more information, visit the GDC Data Commons Portal 2.0 .
Explore the Biological Sciences Division
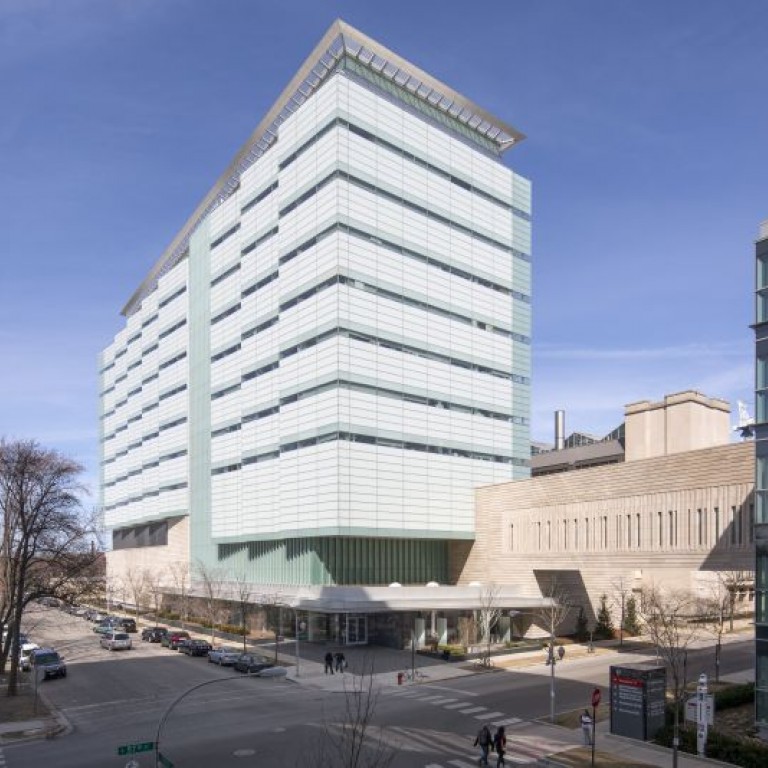

Official websites use .gov
A .gov website belongs to an official government organization in the United States.
Secure .gov websites use HTTPS
A lock ( ) or https:// means you've safely connected to the .gov website. Share sensitive information only on official, secure websites.
Preventive Service Usage and New Chronic Disease Diagnoses: Using PCORnet Data to Identify Emerging Trends, United States, 2018–2022
ORIGINAL RESEARCH — Volume 21 — July 3, 2024
Sandra L. Jackson, PhD, MPH 1 ; Akaki Lekiachvili, MD, MBA 2 ; Jason P. Block, MD, MPH 3 ; Thomas B. Richards, MD 4 ; Kshema Nagavedu, MPH 5 ; Christine C. Draper 5 ; Alain K. Koyama, ScD 6 ; Lindsay S. Womack, PhD, MPH 7 ; Thomas W. Carton, PhD, MS 8 ; Kenneth H. Mayer, MD 9 ; Sonja A. Rasmussen, MD, MS 10 ; William E. Trick, MD 11 ; Elizabeth A. Chrischilles, PhD 12 ; Mark G. Weiner, MD 13 ; Pradeep S. B. Podila, PhD, MHA, MS 14 ; Tegan K. Boehmer, PhD, MPH 15 ,16 ; Jennifer L. Wiltz, MD, MPH 2 ,16 ; on behalf of PCORnet Network Partners ( View author affiliations )
Suggested citation for this article: Jackson SL, Lekiachvili A, Block JP, Richards TB, Nagavedu K, Draper CC, et al. Preventive Service Usage and New Chronic Disease Diagnoses: Using PCORnet Data to Identify Emerging Trends, United States, 2018–2022. Prev Chronic Dis 2024;21:230415. DOI: http://dx.doi.org/10.5888/pcd21.230415 .
PEER REVIEWED
Introduction
Acknowledgments, author information.
What is already known on this topic?
Preventive services and screenings facilitate prevention, early detection, and treatment of chronic diseases, but the COVID-19 pandemic disrupted care.
What is added by this report?
This study of over 30 million US adults during 2018 through 2022 leveraged electronic health record (EHR) data from PCORnet, a national network, to examine use of preventive services and diagnoses of new chronic disease, including trends over time and stratifications by demographic characteristics. Preventive services and chronic disease diagnoses declined during 2020 and subsequently rebounded to nearly prepandemic levels but lagged behind prepandemic levels for some services and diagnoses.
What are the implications for public health practice?
EHR data can augment traditional surveillance and highlight key emerging patterns in health care service usage and in diagnosis of chronic disease. Such data may help optimize prevention and outcomes.
Data modernization efforts to strengthen surveillance capacity could help assess trends in use of preventive services and diagnoses of new chronic disease during the COVID-19 pandemic, which broadly disrupted health care access.
This cross-sectional study examined electronic health record data from US adults aged 21 to 79 years in a large national research network (PCORnet), to describe use of 8 preventive health services (N = 30,783,825 patients) and new diagnoses of 9 chronic diseases (N = 31,588,222 patients) during 2018 through 2022. Joinpoint regression assessed significant trends, and health debt was calculated comparing 2020 through 2022 volume to prepandemic (2018 and 2019) levels.
From 2018 to 2022, use of some preventive services increased (hemoglobin A 1c and lung computed tomography, both P < .05), others remained consistent (lipid testing, wellness visits, mammograms, Papanicolaou tests or human papillomavirus tests, stool-based screening), and colonoscopies or sigmoidoscopies declined ( P < .01). Annual new chronic disease diagnoses were mostly stable (6% hypertension; 4% to 5% cholesterol; 4% diabetes; 1% colonic adenoma; 0.1% colorectal cancer; among women, 0.5% breast cancer), although some declined (lung cancer, cervical intraepithelial neoplasia or carcinoma in situ, cervical cancer, all P < .05). The pandemic resulted in health debt, because use of most preventive services and new diagnoses of chronic disease were less than expected during 2020; these partially rebounded in subsequent years. Colorectal screening and colonic adenoma detection by age group aligned with screening recommendation age changes during this period.
Among over 30 million patients receiving care during 2018 through 2022, use of preventive services and new diagnoses of chronic disease declined in 2020 and then rebounded, with some remaining health debt. These data highlight opportunities to augment traditional surveillance with EHR-based data.
Chronic diseases are primary causes of illness, disability, and death in the United States, and prevention or early detection can facilitate treatment and improve outcomes (1). The US Preventive Services Task Force (USPSTF) recommends screening for chronic diseases including hypertension, diabetes, breast cancer, cervical cancer, colorectal cancer, and lung cancer (2). However, the COVID-19 pandemic disrupted health care access and impaired the ability of US adults to obtain preventive care such as screening for chronic disease, resulting in health debt (ie, missed services, screenings, treatment, or diagnoses) that could have long-lasting negative health consequences (1,3–5). Given health care system burden, as well as concerns about exposure to COVID-19 for at-risk populations during office visits, some experts recommended deferring screening during the most acute phase of the pandemic (6,7). The extent of the disruption, its duration, and its potential impact on subsequent chronic disease diagnoses have been difficult to assess by using existing public health data.
As a component of data modernization efforts, electronic health record (EHR) data have the potential to augment traditional chronic disease surveillance and provide timely and actionable data for public health policies and programs (8). In contrast to some existing surveys such as the National Health Interview Survey and the Behavioral Risk Factor Surveillance System (BRFSS), EHR data offer objective measurements of chronic disease status and screening practices (rather than self-report), large population sizes that allow for examination of rare events, and more timely insights. PCORnet, the National Patient-Centered Clinical Research Network, is an EHR-based network designed to generate high-quality, actionable evidence to support clinical care and high-priority research (9,10). As of 2020, PCORnet collected data from over 300 hospitals, 3,500 primary care practices, 1,000 community clinics, and 80 million patients, with broad geographic coverage across the US (9). Because of the large, diverse population receiving care in its participating health systems, PCORnet has the potential to complement traditional surveillance efforts.
The primary objectives of this study were to examine recent trends in use of preventive services and in new diagnoses of chronic disease and quantify health debt during the COVID-19 pandemic by using a large multisite cohort of US adults. A secondary objective was to explore and describe the usefulness of PCORnet data for chronic disease surveillance.
Participants and procedures
PCORnet is a distributed research network wherein participating health systems perform quarterly data updates and quality checks, and map raw clinical data to a standardized Common Data Model, enabling cross-site interoperability. With this standardization, modular statistical programs can query data across sites in a distributed model and combine results to produce aggregate reports. This cross-sectional study used a modular program (https://github.com/PCORnet-DRN-OC/Query-Details/) to generate site-level aggregate data in 2 separate queries; all results were returned to a coordinating center and combined. The first query examined preventive services (distributed in March 2023; N = 30,783,825 patients) and the second query examined new chronic disease diagnoses (distributed in June 2023; N = 31,588,222 patients). Both queries were performed by 36 PCORnet sites (each representing 1 or more health systems); a data refresh occurred between the 2 queries, resulting in additional patients (9,10). Adults aged 21 to 79 years who had at least 1 encounter at a PCORnet site from January 1, 2018, through December 31, 2022, were included (Supplemental Table 1, available at https://stacks.cdc.gov/view/cdc/156743). Diagnoses and services were identified by procedure codes (Current Procedural Terminology; Healthcare Common Procedure Coding Systems [CPT/HCPCS], 9th and 10th Revisions), diagnostic codes (International Classification of Diseases, 9th and 10th Revisions, Clinical Modification [ICD-9-CM and ICD-10-CM]), laboratory codes (Logical Observation Identifiers Names and Codes [LOINC]), or prescribing codes (National Drug Code [NDC]; vocabulary for normalizing prescription and over-the-counter drugs [RxNorm]). All codes are available at the GitHub link above.
Preventive services
We examined 8 preventive services: 1) wellness visits; 2) glycated hemoglobin (HbA 1c ) laboratory tests; 3) lipid panel laboratory tests; 4) mammograms (among women); 5) Papanicolaou (Pap) tests or human papillomavirus (HPV) tests (among women); 6) low dose computed tomography (CT) scans for lung cancer; 7) colonoscopies or sigmoidoscopies; and 8) stool-based colorectal cancer screening tests, including fecal immunochemical tests or stool DNA-fecal immunochemical tests (eg, Cologuard). Results for mammograms and Pap tests/HPV tests are presented as percentages of all unique female patients; the other percentages are of all unique patients.
New chronic disease diagnoses
We examined new diagnoses of 9 chronic conditions — among patients with no prior record in PCORnet of each condition at any point since January 1, 2009. For new conditions in each year, exclusions were made for any period before that specific year. The 9 conditions were defined by using ICD codes unless specified: 1) type 2 diabetes mellitus (defined as HbA 1c value ≥6.5%, or an RxNorm or NDC code for a diabetes medication [other than metformin or a SGLT2 receptor antagonist, which are often used for conditions other than diabetes], or an ICD code for diabetes); 2) hypertension; 3) hypercholesterolemia (defined as starting a cholesterol medication by using RxNorm or NDC code); 4) breast cancer (among women); 5) cervical cancer (among women); 6) cervical intraepithelial neoplasia or carcinoma in situ (CIN/CIS) (among women); 7) lung cancer; 8) colorectal cancer; and 9) colonic adenoma. Some of these new diagnoses were merely new diagnoses in the PCORnet system, as some patients may have been previously diagnosed in a nonparticipating health system.
Health debt
Health debt was estimated by averaging the prepandemic (2018 and 2019) annual percentages of unique patients receiving each preventive service or new chronic disease diagnosis. Next, these prepandemic percentages were multiplied by the annual total unique patients per year (2020, 2021, and 2022) to calculate expected annual services or chronic disease diagnoses during the pandemic period if prepandemic levels of preventive services and diagnoses had continued. The observed annual numbers were then divided by the expected annual numbers, for each year individually (2020, 2021, and 2022), as well as collectively (the sum of observed services or diagnoses for 2020 through 2022 divided by the sum of expected services or diagnoses for 2020 through 2022). The resulting percentages indicate health debt if less than 100% (ie, observed services or diagnoses were less than expected).
Sociodemographic and clinical characteristics
Variables included age, sex (male, female, other/missing), and race and ethnicity (non-Hispanic American Indian or Alaska Native, non-Hispanic Asian, non-Hispanic Black, non-Hispanic multiple race, non-Hispanic Native Hawaiian or Other Pacific Islander, non-Hispanic White, other, missing, and Hispanic). Some US Census measures were linked at the ZIP Code Tabulation Area level, including urbanicity (isolated, small rural, large rural, urban, missing) based on Rural-Urban Commuting Area codes (https://depts.washington.edu/uwruca/) and socioeconomic status measured by Area Deprivation Index (ADI) (Quartile 1, ADI 0–38; Quartile 2, ADI 39–43; Quartile 3, ADI 44–49; Quartile 4, ADI 50–100; missing) (11,12). Clinical characteristics included body mass index (BMI, with obesity defined as BMI ≥30 calculated as weight in kilograms divided by the square of height in meters) and comorbidity status (by using up to 5-year diagnostic history for heart disease, diabetes, cancer, hypertension, mental health disorders, and smoking, for those with available data).
Statistical analysis
Each PCORnet site executed the SAS-based modular statistical program query (SAS Institute Inc) on patient-level data that remained behind each health system’s firewall. The coordinating center combined all data across sites, and descriptive analyses were performed on the aggregate data in 2023 using Excel (Microsoft) and R (The R Foundation). Joinpoint regression was conducted to assess significant trends from 2018 to 2022 by using the National Cancer Institute’s Joinpoint Trend Analysis Software (version 5.0.2). Results were calculated overall and were stratified by select demographic characteristics. For example, results related to colorectal cancer screening and diagnoses were examined by age group to examine potential changes in relation to the 2021 USPSTF recommendation that lowered the colorectal cancer screening threshold from age 50 to age 45 (2). This activity was reviewed by the Harvard Pilgrim Health Care Institutional Review Board and by the Centers for Disease Control and Prevention (CDC), was deemed not human subjects research, and was conducted consistent with applicable federal law and CDC policy. The data for this study were shared by using existing data use agreements between sites involved. For data requests from outside collaborators, PCORnet has a standard process called the Front Door (https://pcornet.org/front-door/).
During 2018 through 2022, approximately 12 to 16 million adult patients per year received care in participating PCORnet sites ( Table 1 , preventive services query results; Supplemental Table 2, available at https://stacks.cdc.gov/view/cdc/156743, shows results for new chronic disease diagnoses). Of these, 59% were women and most (>75%) lived in urban areas. Over half were non-Hispanic White, approximately 16% were non-Hispanic Black, 13% were Hispanic, and 3% were non-Hispanic Asian (6%–9% missing, 2%–3% other; there was some variation by year). Among those with available BMI (~70%), approximately 40% had obesity.
Preventive service use
During 2018 through 2022 ( Figure 1 ), there was a significant increasing trend in usage of some preventive services, including HbA 1c testing (18% to 22%, P = .03) and lung CT scans (0.2% to 0.4%, P < .01). Usage of other preventive services did not change significantly: lipid testing, approximately 26%; wellness visits, 20%; mammograms among women, 18%; Pap tests or HPV tests among women, approximately 10%; and stool-based screening tests, 2%. However, there was a significant increasing trend in stool-based screening among those aged 45 to 49 years, with prevalence increasing from 0.6% in 2018 to 4.6% in 2022 ( P = .02; shown in Supplemental Figure 1, available at https://stacks.cdc.gov/view/cdc/156743). Among all patients, there was a significant decreasing trend in colonoscopies or sigmoidoscopies, from approximately 3% to 2% ( P < .01) .
Most preventive services experienced a decrease in usage during 2020 compared with 2019 ( Figure 1 ). Examining the monthly distribution of usage of each preventive service during 2020, few preventive services occurred during April and May compared with the rest of the year (Supplemental Figure 2, available at https://stacks.cdc.gov/view/cdc/156743). There was estimated health debt for nearly all preventive services in 2020, although lung CT scans exceeded prepandemic expected levels (113%; Table 2 ). By 2022, all but 2 preventive services (Pap tests or HPV tests, 81%; colonoscopies or sigmoidoscopies, 81%; Table 2 ) had rebounded to, or exceeded, prepandemic levels (≥99%).
There was variation by race and ethnicity in preventive service usage (Supplemental Figure 3, available at https://stacks.cdc.gov/view/cdc/156743). For example, in 2022, non-Hispanic Asian adults had the highest percentage receiving lipid panel testing (34%) and wellness visits (26%); Hispanic adults had the highest percentage receiving HbA 1c tests (32%), Pap tests or HPV tests (15% of women), and stool-based screening tests (6%); and non-Hispanic White adults had the highest percentage receiving mammograms (20% of women) and lung CT scans (0.6%).
Preventive service trends showed some variation by race and ethnicity from 2018 to 2022. For example, there was a significant decreasing trend in colonoscopy or sigmoidoscopy among non-Hispanic White adults ( P < .01; Supplemental Figure 4, available at https://stacks.cdc.gov/view/cdc/156743). All race and ethnicity groups had a significant increase in lung CT scans ( P < .05 for all).
From 2018 to 2022, annual chronic disease diagnoses remained mostly stable, with a dip during 2020 ( Figure 2 ). Over this period, approximately 6% of patients annually received a new hypertension diagnosis, 4% to 5% began a cholesterol medication, and 4% received a new diabetes diagnosis. Approximately 1% had a new colonic adenoma; 0.1% had new colorectal cancer; and among women, 0.5% had a new diagnosis of breast cancer. There was a significant decreasing trend in CIN/CIS among women (0.30% in 2018 to 0.26% in 2022, P = .01), cervical cancer among women (0.05% to 0.04%, P = .02), and lung cancer among all patients (0.18% to 0.16%, P = .04). For colonic adenoma, there was an increasing trend in new diagnoses among those aged 45 to 49 years (0.7% to 1.9%, P < .05; Supplemental Figure 1, available at https://stacks.cdc.gov/view/cdc/156743). Among patients aged 45 to 49 years, approximately 60 patients per 100,000 were diagnosed with colonic adenoma during 2018 through 2020; this increased to 88 per 100,000 in 2021 and 179 per 100,000 in 2022 .
The monthly distribution of new chronic disease diagnoses during 2020 showed few new diagnoses during April and May compared with the rest of the year (Supplemental Figure 5, available at https://stacks.cdc.gov/view/cdc/156743). There was estimated health debt for all chronic diseases in 2020, with observed levels ranging from 81% (colonic adenoma) to 92% (breast cancer) of expected prepandemic levels ( Table 2 ). In 2022, new diagnoses of 3 conditions (hypertension, diabetes, and colonic adenoma) and new prescriptions for cholesterol medications had rebounded to, or exceeded, prepandemic levels (≥99%). However, any excess during 2022 was not sufficient to make up for the lost volume of diagnoses in 2020 and 2021. Over the combined 3-year pandemic period, 2020 through 2022, health debt remained for all 9 conditions. For the combined period 2020 through 2022, 3-year health debt ranged from 84% (cervical cancer) to 98% (new cholesterol medications).
Chronic disease diagnoses varied by race and ethnicity (Supplemental Figure 6, available at https://stacks.cdc.gov/view/cdc/156743). For example, in 2022, non-Hispanic Black adults had the highest percentage of new hypertension (8%) and diabetes (5%) diagnoses. Hispanic women had the highest percentage of new CIN/CIS (0.4%). During 2018 through 2022, diagnoses of new CIN/CIS decreased significantly among non-Hispanic White women ( P < .01), and diagnoses of new cervical cancer decreased significantly among both non-Hispanic White ( P < .01) and Hispanic ( P = .02) women (Supplemental Figure 7, available at https://stacks.cdc.gov/view/cdc/156743).
In this EHR-based assessment of over 30 million US adults receiving care during 2018 through 2022, usage of preventive services and new diagnoses of chronic disease decreased during 2020, especially in the initial months of the COVID-19 pandemic. Preventive services and chronic disease diagnoses rebounded during 2021 and 2022, returning to nearly prepandemic levels, but health debt remained for several preventive services and chronic disease diagnoses. Use of EHR data allowed for stratification by sociodemographic factors, revealing racial and ethnic screening disparities as well as differential trends by age for those affected by colorectal cancer screening guideline changes (2). Overall, these findings highlight the usefulness of PCORnet data for chronic disease surveillance.
The observed decrease in preventive service usage during 2020 is consistent with prior work, although most existing studies were limited to specific settings, populations, or conditions. Researchers have reported decreases during 2020 for routine medical visits (4), HbA 1c testing (13), lipid testing (13,14), mammograms (7), colonoscopies (7), Pap tests (7), and lung CT scans (15,16). Telehealth and home-based services with clinical support, such as self-measured blood pressure monitoring (17,18), blood glucose monitoring (19,20), home-based HPV screening kits (21,22), and at-home colorectal screening (7) can reduce barriers to care and may have partially mitigated disruption from the pandemic, at least for some tests and diagnoses (14). The pandemic’s effects on screening choices may have contributed to results of this study, such as the observed decrease in colonoscopies or sigmoidoscopies and simultaneous increase in stool-based testing. Expanded implementation of alternative, more accessible modalities of care could support future chronic disease prevention, detection, and management.
Similar to the observed decrease in preventive service usage, there was a decrease in new chronic disease diagnoses during 2020. This finding was expected and is consistent with prior studies. For example, pandemic-related decreases occurred in cancer diagnoses (23–26), including colorectal, female breast, cervical, and lung cancer, as well as hypertension diagnostic procedures (27) and new statin prescriptions (13). Although diagnoses rebounded in 2021 and 2022, any delay in diagnosis can delay treatment, potentially leading to worse outcomes for affected patients. In a national, hospital-based registry capturing about 70% of all US cancer diagnoses annually, the proportion of cancers detected at early stages decreased during 2020 (24); also, a modeling study from England suggested that pandemic-related diagnostic delays for colorectal, breast, and lung cancers could have resulted in approximately 15%, 8%, and 5% increases, respectively, in deaths over 5 years (28). In addition, patients with chronic conditions such as diabetes, hypertension, and cancer are at increased risk of severe outcomes from COVID-19 (29,30), underscoring the importance of continued diagnosis and management of chronic conditions even amidst acute pandemic phases.
Racial and ethnic disparities are well documented for screening and burden of chronic disease. For example, non-Hispanic Black men have higher incidence of lung cancer than non-Hispanic White men; however non-Hispanic Black men are less likely to be screened, receive later-stage diagnoses, have lower rates of treatment, and have more deaths from lung cancer (31). In our study, non-Hispanic Black adults had lower receipt of lung CT scans compared with non-Hispanic White adults. In a prior study, non-Hispanic Black women had higher breast cancer mortality than non-Hispanic White women (32); however, another study found that non-Hispanic Black women were more likely to meet breast cancer screening guidelines than non-Hispanic White women (33). Our results were inconsistent with this prior study in that we observed lower mammography screening in non-Hispanic Black women compared with non-Hispanic White women. This difference may have been due to differences in population, measurement, or methodology. To some extent, our results stratified by race and ethnicity, with no further adjustment for age differences between these populations, may mask the degree of inequality. For example, a much younger age distribution among communities of color may partly explain the higher observed prevalence of Pap tests or HPV testing among Hispanic and non-Hispanic Asian women compared with non-Hispanic White women.
Age stratification revealed a sharp increase in both colonoscopy and stool-based screening among patients aged 45 to 49 in 2021 and 2022, corresponding to the 2021 USPSTF recommendation lowering the colorectal cancer screening threshold from age 50 to age 45 (2). Among this age group, we also observed increased diagnoses of new colonic adenoma. However, the observed volume of colorectal screening test usage remained low, consistent with prior estimates that nearly 30% of eligible US adults were not up to date with screening in 2021 (34). The ability to track implementation and potential impact of new screening recommendations or guidelines in clinical practice with timely, real-world data is a unique strength of EHR-based surveillance using PCORnet.
Limitations
This study had several limitations. First, PCORnet is not a nationally representative sample of the US population: PCORnet has overrepresentation in academic health care settings and represents a care-seeking population (ie, patients who have some degree of access to and engagement in a health system). Second, included patients could have also obtained preventive services and diagnoses from other facilities that did not contribute data to PCORnet. Third, denominators for each preventive service were all adults, or all women, rather than the specific population recommended for each service based on age, risk factors, and recommended screening time intervals. Thus, this study was not designed to estimate whether patients were individually up to date with screening recommendations. Fourth, some patients may have received preventive care services as disease monitoring rather than screening; thus, our estimates likely overestimate the percentage of patients who received these tests for screening purposes alone. Furthermore, some lung CT scans during the COVID-19 pandemic may reflect pulmonary evaluations for COVID-19 rather than lung cancer screenings. Fifth, if a patient’s first encounter with a PCORnet health system occurred during our study period and led to the first documentation of a chronic disease, then some prevalent cases could have been included as new chronic disease diagnoses. Thus, our estimates for new diagnoses may be higher than actual incidence. Sixth, it is impossible to disentangle whether any changes in recorded new diagnoses were a consequence of altered testing and treatment during the pandemic rather than other secular trends that may have occurred even without the pandemic, such as increasing disease incidence or declining health care usage. Seventh, some patients could have been represented in the data more than once if they received care from multiple PCORnet sites. Finally, as EHR data are real-world data, they are subject to limitations such as missingness and variation across sites; further analyses could replicate these findings using varied data sources and phenotype definitions.
Despite these limitations, trends show a clear national level health debt with a drop in usage of preventive services and new chronic disease diagnoses during 2020 through 2022, despite some services rebounding to prepandemic levels. If trends in preventive services or diagnoses were not flat during the prepandemic period (2018–2019), then our method of estimating health debt could lead to overestimates (if trends were declining) or underestimates (if trends were increasing). Also, if fewer patients received care of any kind during the pandemic period (2020–2022), our method may underestimate health debt, since expected numbers of services and diagnoses were based on the number of patients who had encounters in institutions participating in PCORnet. Furthermore, while our study examined trends in yearly cross-sectional data, a longitudinal study examining patient-level data could better assess whether results are clinically meaningful, and what opportunities might exist for public health intervention. Future work could investigate health debt in more detail, including geographic variation, underlying causes of trends (such as health care capacity or delivery issues, or misinformation among patients), factors associated with returning to care, populations most affected by health debt, and potential effects of delayed diagnoses, later-stage disease detection, or delayed treatment initiation. Further exploration of incidence and services, such as whether eligible patients are up to date with their screenings, will be important for informing public health activities. In support of future efforts, this study’s codes and methodology have been shared publicly for replication, refinement, and use.
Conclusions
This multisite, nationwide assessment of over 30 million patients revealed a decrease in both preventive services and new chronic disease diagnoses during the first year of the COVID-19 pandemic, followed by an increase in 2021 and 2022 to nearly prepandemic levels. In addition to examining health debt in preventive services and chronic disease diagnoses during the COVID-19 pandemic, PCORnet data also allow for examination of disparities by race and ethnicity as well as implementation of new screening recommendations. Overall, this study highlights ways to augment traditional chronic disease surveillance with data from PCORnet or other EHR-based surveillance systems — for timely assessment of emerging trends in chronic disease nationwide.
The authors report no conflicts of interest and have no funders to report for this work. No copyrighted material was adapted or reused in this article.
The findings and conclusions in this report are those of the authors and do not necessarily represent the official position of the Centers for Disease Control and Prevention.
We thank Sharon Saydah and Emilia Koumans from the CDC Coronavirus and Other Respiratory Viruses Division, the National Patient-Centered Clinical Research Network, and the Patient-Centered Outcomes Research Institute.
PCORnet Network Partners: Faraz S. Ahmad, MD, MS, Division of Cardiology, Department of Medicine, Northwestern University Feinberg School of Medicine; Saul Blecker, MD, MHS, NYU Grossman School of Medicine; Olveen Carrasquillo, MD, MPH, University of Miami; Bernard P. Chang, MD, PhD, Ochsner Health; Dimitri A. Christakis, MD, MPH, Seattle Children’s Research Institute; Katherine Chung-Bridges, MD, MPH, Health Choice Network; Lauren P. Cleveland, MS, MPH, Harvard Pilgrim Health Care Institute; Lindsay G. Cowell, MD, PhD, O’Donnell School of Public Health, University of Texas Southwestern Medical Center; Janis L. Curtis, MSPH, MA, Duke University School of Medicine; Christopher B. Forrest, MD, PhD, Children’s Hospital of Philadelphia; Daniel Fort, PhD, MPH, Ochsner Health; Carol Reynolds Geary, PhD, MBA, RN, University of Nebraska Medical Center; Philip A. Giordano, MD, Orlando Health, Inc; David Hanauer, MD, MS, University of Michigan; Rachel Hess, MD, MS, University of Utah Health; Benjamin D. Horne, PhD, MStat, MPH, Intermountain Medical Center Heart Institute; Wenke Hwang, PhD, Penn State University College of Medicine; Harold P. Lehmann, MD, PhD, Johns Hopkins School of Medicine; Abu Saleh Mohammad Mosa, PhD, MS, University of Missouri; Samyuktha Nandhakumar, MS, North Carolina Translational and Clinical Sciences Institute, UNC School of Medicine; Jihad S. Obeid, MD, Medical University of South Carolina; Brian Ostasiewski, BS, Wake Forest School of Medicine; Nathan M. Pajor, MD, MS, University of Cincinnati College of Medicine and Cincinnati Children’s Hospital Medical Center; Lav P. Patel, MS, University of Kansas Medical Center; Suchitra Rao, MBBS, MSCS, University of Colorado; Pedro Rivera, MS, OCHIN; Patricia S. Robinson, PhD, APRN, AdventHealth; Marc Rosenman, MD, Ann & Robert H. Lurie Children’s Hospital of Chicago; Russell L. Rothman, MD, MPP, Vanderbilt University Medical Center; Jonathan C. Silverstein, MD, MS, University of Pittsburgh; Alexander Stoddard, MS, Medical College of Wisconsin.
Corresponding Author: Sandra Jackson, PhD, MPH, National Center for Chronic Disease Prevention and Health Promotion, Centers for Disease Control and Prevention, 4770 Buford Highway NE, Mailstop S107-1, Atlanta, GA 30341 ( [email protected] ).
Author Affiliations: 1 Division for Heart Disease and Stroke Prevention, National Center for Chronic Disease Prevention and Health Promotion, Centers for Disease Control and Prevention, Atlanta, Georgia. 2 National Center for Chronic Disease Prevention and Health Promotion, Centers for Disease Control and Prevention, Atlanta, Georgia. 3 Division of Chronic Disease Research Across the Lifecourse, Department of Population Medicine, Harvard Pilgrim Health Care Institute, Harvard Medical School, Boston, Massachusetts. 4 Division of Cancer Prevention and Control, National Center for Chronic Disease Prevention and Health Promotion, Centers for Disease Control and Prevention, Atlanta, Georgia. 5 Division of Therapeutics Research and Infectious Disease Epidemiology, Department of Population Medicine, Harvard Pilgrim Health Care Institute, Harvard Medical School, Boston, Massachusetts. 6 Division of Diabetes Translation, National Center for Chronic Disease Prevention and Health Promotion, Centers for Disease Control and Prevention, Atlanta, Georgia. 7 Division of Reproductive Health, National Center for Chronic Disease Prevention and Health Promotion, Centers for Disease Control and Prevention, Atlanta, Georgia. 8 Louisiana Public Health Institute, New Orleans. 9 The Fenway Institute, Fenway Health and the Division of Infectious Diseases, Department of Medicine, Beth Israel Deaconess Medical Center/Harvard Medical School, Boston, Massachusetts. 10 Johns Hopkins School of Medicine, Baltimore, Maryland. 11 Center for Health Equity and Innovation, Cook County Health, Chicago, Illinois. 12 Department of Epidemiology, College of Public Health, University of Iowa, Iowa City. 13 Department of Population Health Sciences, Weill Cornell Medicine, New York, New York. 14 Office of Informatics and Information Resource Management, National Center for Chronic Disease Prevention and Health Promotion, Centers for Disease Control and Prevention, Atlanta, Georgia. 15 Office of Public Health Data, Surveillance, and Technology, Centers for Disease Control and Prevention, Atlanta, Georgia. 16 US Public Health Service, Atlanta, Georgia.
- Virani SS, Alonso A, Aparicio HJ, Benjamin EJ, Bittencourt MS, Callaway CW, et al; American Heart Association Council on Epidemiology and Prevention Statistics Committee and Stroke Statistics Subcommittee. Heart disease and stroke statistics — 2021 update: a report from the American Heart Association. Circulation . 2021;143(8):e254–e743. PubMed doi:10.1161/CIR.0000000000000950
- US Preventive Services Task Force. A & B recommendations. 2023. Accessed October 23, 2023. https://www.uspreventiveservicestaskforce.org/uspstf/recommendation-topics/uspstf-a-and-b-recommendations
- Balasuriya L, Briss PA, Twentyman E, Wiltz JL, Richardson LC, Bigman ET, et al. Impacts of the COVID-19 pandemic on nationwide chronic disease prevention and health promotion activities. Am J Prev Med . 2023;64(3):452–458. PubMed doi:10.1016/j.amepre.2022.09.012
- Czeisler ME, Marynak K, Clarke KEN, Salah Z, Shakya I, Thierry JM, et al. Delay or avoidance of medical care because of COVID-19–related concerns — United States, June 2020. MMWR Morb Mortal Wkly Rep . 2020;69(36):1250–1257. PubMed doi:10.15585/mmwr.mm6936a4
- Biancalana E, Parolini F, Mengozzi A, Solini A. Short-term impact of COVID-19 lockdown on metabolic control of patients with well-controlled type 2 diabetes: a single-centre observational study. Acta Diabetol . 2021;58(4):431–436. PubMed doi:10.1007/s00592-020-01637-y
- Mazzone PJ, Gould MK, Arenberg DA, Chen AC, Choi HK, Detterbeck FC, et al. Management of lung nodules and lung cancer screening during the COVID-19 pandemic: CHEST Expert Panel Report. J Am Coll Radiol . 2020;17(7):845–854. PubMed doi:10.1016/j.jacr.2020.04.024
- Gorin SNS, Jimbo M, Heizelman R, Harmes KM, Harper DM. The future of cancer screening after COVID-19 may be at home. Cancer . 2021;127(4):498–503. PubMed doi:10.1002/cncr.33274
- Birkhead GS, Klompas M, Shah NR. Uses of electronic health records for public health surveillance to advance public health. Annu Rev Public Health . 2015;36(1):345–359. PubMed doi:10.1146/annurev-publhealth-031914-122747
- Forrest CB, McTigue KM, Hernandez AF, Cohen LW, Cruz H, Haynes K, et al. PCORnet® 2020: current state, accomplishments, and future directions. J Clin Epidemiol . 2021;129:60–67. PubMed doi:10.1016/j.jclinepi.2020.09.036
- Califf RM. The Patient-Centered Outcomes Research Network: a national infrastructure for comparative effectiveness research. N C Med J . 2014;75(3):204–210. PubMed doi:10.18043/ncm.75.3.204
- Kind AJH, Buckingham WR. Making neighborhood-disadvantage metrics accessible — the Neighborhood Atlas. N Engl J Med . 2018;378(26):2456–2458. PubMed doi:10.1056/NEJMp1802313
- University of Wisconsin School of Medicine and Public Health. 2015 Area Deprivation Index. Accessed May 23, 2019. https://www.neighborhoodatlas.medicine.wisc.edu/
- Wright A, Salazar A, Mirica M, Volk LA, Schiff GD. The invisible epidemic: neglected chronic disease management during COVID-19. J Gen Intern Med . 2020;35(9):2816–2817. PubMed doi:10.1007/s11606-020-06025-4
- Alexander GC, Tajanlangit M, Heyward J, Mansour O, Qato DM, Stafford RS. Use and content of primary care office-based vs telemedicine care visits during the COVID-19 pandemic in the US. JAMA Netw Open . 2020;3(10):e2021476. PubMed doi:10.1001/jamanetworkopen.2020.21476
- Li J, Stults CD, Liang SY, Martinez M. Factors associated with adherence to provider referrals for lung cancer screening with low dose computed tomography before and during COVID-19 pandemic. BMC Cancer . 2023;23(1):809. PubMed doi:10.1186/s12885-023-11256-9
- Van Haren RM, Delman AM, Turner KM, Waits B, Hemingway M, Shah SA, et al. Impact of the COVID-19 pandemic on lung cancer screening program and subsequent lung cancer. J Am Coll Surg . 2021;232(4):600–605. PubMed doi:10.1016/j.jamcollsurg.2020.12.002
- Wall HK, Wright JS, Jackson SL, Daussat L, Ramkissoon N, Schieb LJ, et al. How do we jump-start self-measured blood pressure monitoring in the United States? Addressing barriers beyond the published literature. Am J Hypertens . 2022;35(3):244–255. PubMed doi:10.1093/ajh/hpab170
- Abbas A, Hannan J, Stolp H, Coronado F, Sperling LS. Commitment to hypertension control during the COVID-19 pandemic: Million Hearts Initiative exemplars. Prev Chronic Dis . 2022;19:E47. PubMed doi:10.5888/pcd19.210439
- Fisher L, Polonsky W, Asuni A, Jolly Y, Hessler D. The early impact of the COVID-19 pandemic on adults with type 1 or type 2 diabetes: a national cohort study. J Diabetes Complications . 2020;34(12):107748. PubMed doi:10.1016/j.jdiacomp.2020.107748
- Chircop J, Sheffield D, Kotera Y. Systematic review of self-monitoring of blood glucose in patients with type 2 diabetes. Nurs Res . 2021;70(6):487–497. PubMed doi:10.1097/NNR.0000000000000542
- Parker S, Deshmukh AA, Chen B, Lairson DR, Daheri M, Vernon SW, et al. Perceived barriers to cervical cancer screening and motivators for at-home human papillomavirus self-sampling during the COVID-19 pandemic: results from a telephone survey. eLife . 2023;12:e84664. PubMed doi:10.7554/eLife.84664
- Chao YS, McCormack S. CADTH Rapid Response Reports. HPV Self-Sampling for Primary Cervical Cancer Screening: A Review of Diagnostic Test Accuracy and Clinical Evidence — An Update . Canadian Agency for Drugs and Technologies in Health; 2019.
- Moubarak S, Merheb D, Basbous L, Chamseddine N, Bou Zerdan M, Assi HI. COVID-19 and lung cancer: update on the latest screening, diagnosis, management and challenges. J Int Med Res . 2022;50(9):3000605221125047. PubMed doi:10.1177/03000605221125047
- Han X, Yang NN, Nogueira L, Jiang C, Wagle NS, Zhao J, et al. Changes in cancer diagnoses and stage distribution during the first year of the COVID-19 pandemic in the USA: a cross-sectional nationwide assessment. Lancet Oncol . 2023;24(8):855–867. PubMed doi:10.1016/S1470-2045(23)00293-0
- Patt D, Gordan L, Diaz M, Okon T, Grady L, Harmison M, et al. Impact of COVID-19 on cancer care: how the pandemic is delaying cancer diagnosis and treatment for American seniors. JCO Clin Cancer Inform . 2020;4(4):1059–1071. PubMed doi:10.1200/CCI.20.00134
- Negoita S, Chen HS, Sanchez PV, Sherman RL, Henley SJ, Siegel RL, et al. Annual report to the nation on the status of cancer, part 2: Early assessment of the COVID-19 pandemic’s impact on cancer diagnosis. Cancer . 2024;130(1):117–127. PubMed doi:10.1002/cncr.35026
- Weber T, Amar J, de Backer T, Burkard T, van der Giet M, Gosse P, et al; Covid-19 Task Force of the European Society of Hypertension. Covid-19 associated reduction in hypertension-related diagnostic and therapeutic procedures in Excellence Centers of the European Society of Hypertension. Blood Press . 2022;31(1):71–79. PubMed doi:10.1080/08037051.2022.2060182
- Maringe C, Spicer J, Morris M, Purushotham A, Nolte E, Sullivan R, et al. The impact of the COVID-19 pandemic on cancer deaths due to delays in diagnosis in England, UK: a national, population-based, modelling study. Lancet Oncol . 2020;21(8):1023–1034. PubMed doi:10.1016/S1470-2045(20)30388-0
- Giannakoulis VG, Papoutsi E, Siempos II. Effect of cancer on clinical outcomes of patients with COVID-19: a meta-analysis of patient data. JCO Glob Oncol . 2020;6(6):799–808. PubMed doi:10.1200/GO.20.00225
- Centers for Disease Control and Prevention. Underlying medical conditions associated with high risk for severe COVID-19: information for healthcare professionals. Updated February 9, 2023. Accessed August 3, 2023. https://www.cdc.gov/coronavirus/2019-ncov/hcp/clinical-care/underlyingconditions.html
- Sosa E, D’Souza G, Akhtar A, Sur M, Love K, Duffels J, et al. Racial and socioeconomic disparities in lung cancer screening in the United States: a systematic review. CA Cancer J Clin . 2021;71(4):299–314. PubMed doi:10.3322/caac.21671
- Ellington TD, Henley SJ, Wilson RJ, Miller JW, Wu M, Richardson LC. Trends in breast cancer mortality by race/ethnicity, age, and US census region, United States — 1999–2020. Cancer . 2023;129(1):32–38. PubMed doi:10.1002/cncr.34503
- Benavidez GA, Zgodic A, Zahnd WE, Eberth JM. Disparities in meeting USPSTF breast, cervical, and colorectal cancer screening guidelines among women in the United States. Prev Chronic Dis . 2021;18:E37. PubMed doi:10.5888/pcd18.200315
- Sabatino SA, Thompson TD, White MC, Villarroel MA, Shapiro JA, Croswell JM, et al. . Up-to-date breast, cervical, and colorectal cancer screening test use in the United States, 2021. Prev Chronic Dis . 2023;20:E94. PubMed doi:10.5888/pcd20.230071
Characteristic | 2018 | 2019 | 2020 | 2021 | 2022 |
---|---|---|---|---|---|
12,236,819 | 13,026,666 | 13,353,937 | 15,590,610 | 13,826,891 | |
21–34 | 24 | 24 | 25 | 24 | 24 |
35–39 | 9 | 9 | 9 | 9 | 9 |
40–44 | 8 | 8 | 8 | 8 | 8 |
45–49 | 9 | 8 | 8 | 8 | 8 |
50–64 | 30 | 29 | 29 | 28 | 28 |
65–79 | 21 | 21 | 21 | 22 | 23 |
Female | 59 | 59 | 59 | 59 | 59 |
Male | 41 | 41 | 41 | 41 | 41 |
Non-Hispanic Asian | 3 | 3 | 3 | 3 | 3 |
Non-Hispanic Black | 16 | 16 | 15 | 15 | 16 |
Non-Hispanic White | 59 | 59 | 58 | 56 | 57 |
Hispanic | 13 | 13 | 13 | 13 | 13 |
Underweight (<18.5) | 2 | 2 | 2 | 2 | 2 |
Normal weight (18.5–<25) | 27 | 26 | 26 | 25 | 25 |
Overweight (25–<30) | 32 | 31 | 31 | 31 | 31 |
Obese (≥30) | 40 | 41 | 42 | 42 | 43 |
Heart disease | 11 | 11 | 11 | 10 | 12 |
Diabetes | 10 | 11 | 11 | 10 | 12 |
Cancer | 6 | 6 | 6 | 5 | 6 |
Hypertension | 24 | 24 | 24 | 23 | 25 |
Mental health disorders | 11 | 11 | 12 | 11 | 13 |
Smoking | 13 | 13 | 13 | 12 | 13 |
Isolated | 2 | 2 | 2 | 2 | 2 |
Small rural | 2 | 2 | 2 | 2 | 2 |
Large rural | 6 | 6 | 6 | 6 | 6 |
Urban | 84 | 83 | 82 | 80 | 76 |
Quartile 1: 0–38 | 27 | 26 | 26 | 26 | 24 |
Quartile 2: 39–43 | 23 | 23 | 23 | 22 | 21 |
Quartile 3: 44–49 | 22 | 22 | 22 | 21 | 21 |
Quartile 4: 50–100 | 23 | 22 | 21 | 20 | 20 |
a National Patient-Centered Clinical Research Network (PCORnet) sites: Duke University, Medical University of South Carolina, Vanderbilt University Medical Center, Wake Forest Baptist Health, Allina Health, Medical College of Wisconsin, University of Iowa Healthcare, University of Kansas, University of Missouri HC, University of Nebraska, University of Texas SW Medical Center, University of Utah, University Medical Center New Orleans, Ochsner Health System, Children’s Hospital Colorado, Children’s Hospital of Philadelphia, Cincinnati Children’s Hospital, Nationwide Children’s Hospital, Nemours Children’s Hospital, Seattle Children’s Hospital, Lurie Children’s Hospital, Columbia, Cook County, Northwestern University, Fenway Health, Health Choice Network, OCHIN, Inc, Johns Hopkins University, Penn State College of Medicine and Penn State Health Milton S. Hershey Medical Center, Temple University, University of Michigan, University of Pittsburgh Medical Center, AdventHealth, Orlando Health System, University of Florida Health, University of Miami. These sites represent academic and community health systems. Patients who receive care in these institutions reside across all 50 states; Washington, DC; Puerto Rico; US Virgin Islands; and Guam. b Age category based on patient age at the time of first encounter during the 1-year period. c Other/missing not shown. d Not shown: non-Hispanic American Indian or Alaska Native (<1%), non-Hispanic multiple race (<1%), non-Hispanic Native Hawaiian or Other Pacific Islander (<1%), other (2%), and missing (6%–9%; there were some variations by year). e Body mass index (BMI) data were missing from 25% to 35% of patients. BMI category percentages have been calculated among those with available BMI data. BMI calculation: weight in kilograms divided by the square of height in meters.
Service or diagnosis | 2020 | 2021 | 2022 | 2020–2022 |
---|---|---|---|---|
Wellness visit | 90 | 98 | 100 | |
Hemoglobin A | 100 | 110 | 120 | |
Lipid testing | 95 | 102 | 105 | |
Colonoscopy | 89 | 86 | 81 | |
Stool-based test | 94 | 103 | 130 | |
Lung computed tomography | 113 | 144 | 190 | |
Mammogram | 93 | 101 | 99 | |
Papanicolaou tests or human papillomavirus tests | 84 | 92 | 81 | |
Hypertension | 90 | 98 | 99 | |
Diabetes | 87 | 92 | 100 | |
Cholesterol | 90 | 96 | 106 | |
Colorectal cancer | 90 | 91 | 97 | |
Colonic adenoma | 81 | 93 | 105 | |
Lung cancer | 89 | 85 | 88 | |
Breast cancer | 92 | 95 | 97 | |
Cervical cancer | 87 | 81 | 83 | |
Cervical intraepithelial neoplasia or carcinoma in situ | 90 | 87 | 87 |
a The average from 2018 to 2019 was used as the baseline for estimation of health debt. The observed annual numbers were then divided by the expected annual numbers, for each year individually (2020, 2021, and 2022), as well as collectively (2020–2022). Percentages indicate health debt if less than 100%. Health debt for mammograms, Papanicolaou tests and/or human papillomavirus tests, breast cancer, cervical cancer, and cervical intraepithelial neoplasia or carcinoma in situ are presented for all women; all other health debt estimates are for all patients (men and women). Colonoscopy indicates colonoscopy or sigmoidoscopy. Stool-based test indicates stool-based colorectal test. Cholesterol indicates initiation of a new cholesterol medication.
The opinions expressed by authors contributing to this journal do not necessarily reflect the opinions of the U.S. Department of Health and Human Services, the Public Health Service, the Centers for Disease Control and Prevention, or the authors’ affiliated institutions.
Exit Notification / Disclaimer Policy
- The Centers for Disease Control and Prevention (CDC) cannot attest to the accuracy of a non-federal website.
- Linking to a non-federal website does not constitute an endorsement by CDC or any of its employees of the sponsors or the information and products presented on the website.
- You will be subject to the destination website's privacy policy when you follow the link.
- CDC is not responsible for Section 508 compliance (accessibility) on other federal or private website.
ATLAS - Joint PhD Program from BioNTech and TRON with a focus on translational medicine
Nature careers , germany.
ATLAS - Joint PhD Program from BioNTech and TRON with a focus on translational medicine
9 PhD positions in biology, biochemistry, life science, chemistry, medicine, computer science or related fields
BioNTech and TRON (Translational Oncology Mainz) are leading institutions in the field of immunotherapy. Our joint international PhD Program (ATLAS) is looking for talented, junior researchers of all nationalities . Our international PhD program ATLAS (Talent A cademy for T rans LA tional S cience), guides and supports PhD students to conduct excellent research projects and offers comprehensive training in cutting-edge technologies and professional skills. The scientific training within the program has a strong focus on "the art of translation", i.e. turning basic scientific discoveries into applications.
We offer the opportunity to work on innovative research projects focused on translational science in the field of medicine . As a PhD student*, you will join a community of world-class scientists working on diverse topics such as:
- Immunotherapy of cancer, infectious diseases & autoimmunity
- Cardiovascular, aging & regenerative biomedicine
- RNA biochemistry
- Protein engineering
- Bioinformatics & systems biology
- Biomarker research
- Antimicrobial therapies
For details on the program and the specific projects, please visit www.atlas-phdprogram.com .
Who can apply?
Aspiring students seeking to advance knowledge and explore novel therapies. The requirements are:
- A master’s degree in Biology, Biochemistry, Chemistry, Life Science, Medicine, Immunology, Computer Science or Engineering related fields.
- Motivation and passion for science
- Ability to work independently and in an international, multi-disciplinary team
- Professional proficiency in both written and spoken English
Please carefully read "How to apply " and "Projects offered " before applying.
- A highly collaborative and interdisciplinary research environment at the interface between academia and pharmaceutical applications
- A doctoral degree awarded by a partnering university (e.g. Mainz University)
- Dedicated supervision by leaders in their fields with a strong publication record
- Cutting-edge technologies and in-house services to support your research
- Extensive curriculum for scientific, technical and professional skills
- An active and engaging scientific and PhD community
- Possibility to directly be involved in the planning and organization of PhD events
Excellent working conditions:
- Fully funded PhD positions (contracts will be from either BioNTech or TRON)
- Family-friendliness
- Open and inclusive culture
- An international environment with English as our working language
Have we piqued your interest?
Then apply now and simply send us your application documents using our online form.
- All mandatory documents (CV, letter of motivation, official transcript, and certificate of your master’s degree, if available) must be combined into one pdf file and uploaded on the field "*Resume/ CV" via the application portal.
- All other additional documents (e.g. reference letters, proof of proficiency in English, grading system of your home university, etc.) should be combined into one pdf file and uploaded on the field “Additional documents” via the application form.
- For the field “expected annual remuneration package, please fill in “not applicable” since all PhD students will receive a fixed salary and benefits.
- Please make sure you will agree that both BioNTech and TRON can access your data. If you disagree, your application will not be further evaluated.
The deadline for applications is October 31st, 2023. Interviews will take place in Mainz in February 2024. If you have any further questions, please visit www.atlas-phdprogram.com or email us at [email protected] . We look forward to your application!
Please note that your application and data will be used by both BioNTech and TRON – for further information on the data protection policy please click here . *BioNTech and TRON do not differentiate on the basis of gender, race or ethnicity, religion, color, sexual orientation or identity, disability, age and other protected statuses as given by applicable law. We are committed to creating a diverse environment and are proud to be an equal opportunity employer. Most important – it’s a match!
Similar Positions
Description of the offer : We are pleased to announce the opening of PostDoc and PhD positions in computational solid-state physics in the Institute of Physics at the Johannes Gutenberg-Universitä...
Thank you for visiting nature.com. You are using a browser version with limited support for CSS. To obtain the best experience, we recommend you use a more up to date browser (or turn off compatibility mode in Internet Explorer). In the meantime, to ensure continued support, we are displaying the site without styles and JavaScript.
- View all journals
- Explore content
- About the journal
- Publish with us
- Sign up for alerts
- Open access
- Published: 10 July 2024
Single-cell atlas of the human brain vasculature across development, adulthood and disease
- Thomas Wälchli ORCID: orcid.org/0000-0002-8961-6551 1 , 2 , 3 , 4 na1 na2 ,
- Moheb Ghobrial 1 , 2 , 3 , 4 , 5 na1 ,
- Marc Schwab 1 , 2 , 3 , 4 , 6 ,
- Shigeki Takada ORCID: orcid.org/0000-0003-2977-888X 2 , 7 , 8 ,
- Hang Zhong 1 , 2 , 3 , 4 , 5 ,
- Samuel Suntharalingham 1 , 2 , 9 ,
- Sandra Vetiska 2 , 8 ,
- Daymé Rodrigues Gonzalez 10 ,
- Ruilin Wu 11 , 12 ,
- Hubert Rehrauer ORCID: orcid.org/0000-0001-7612-9394 10 ,
- Anuroopa Dinesh ORCID: orcid.org/0000-0002-0062-8004 13 ,
- Kai Yu 11 , 12 ,
- Edward L. Y. Chen 13 , 14 ,
- Jeroen Bisschop ORCID: orcid.org/0000-0001-8652-9535 1 , 2 , 3 , 4 , 9 ,
- Fiona Farnhammer ORCID: orcid.org/0000-0003-4149-8215 1 , 2 , 3 , 4 , 9 ,
- Ann Mansur 2 , 8 , 11 ,
- Joanna Kalucka 15 ,
- Itay Tirosh 16 ,
- Luca Regli ORCID: orcid.org/0000-0003-4639-4474 4 ,
- Karl Schaller 17 ,
- Karl Frei 3 , 4 ,
- Troy Ketela 18 ,
- Mark Bernstein 2 , 19 ,
- Paul Kongkham 2 , 19 , 20 ,
- Peter Carmeliet ORCID: orcid.org/0000-0001-7961-1821 21 , 22 , 23 ,
- Taufik Valiante ORCID: orcid.org/0000-0002-3443-3790 2 , 19 , 24 , 25 , 26 ,
- Peter B. Dirks ORCID: orcid.org/0000-0001-5718-6465 2 , 14 , 27 ,
- Mario L. Suva ORCID: orcid.org/0000-0001-9898-5351 28 , 29 ,
- Gelareh Zadeh ORCID: orcid.org/0000-0002-6637-4502 2 , 19 , 30 ,
- Viviane Tabar ORCID: orcid.org/0000-0002-1284-1670 31 ,
- Ralph Schlapbach 10 ,
- Hartland W. Jackson ORCID: orcid.org/0000-0001-6423-2415 13 , 14 , 32 ,
- Katrien De Bock ORCID: orcid.org/0000-0001-8232-4663 5 ,
- Jason E. Fish ORCID: orcid.org/0000-0003-0640-7277 11 , 12 , 33 ,
- Philippe P. Monnier 9 , 34 , 35 ,
- Gary D. Bader ORCID: orcid.org/0000-0003-0185-8861 13 , 14 , 18 , 36 na2 &
- Ivan Radovanovic 2 , 8 , 11 , 19 na2
Nature ( 2024 ) Cite this article
Metrics details
- Computational biology and bioinformatics
- Developmental biology
- Neuro–vascular interactions
A broad range of brain pathologies critically relies on the vasculature, and cerebrovascular disease is a leading cause of death worldwide. However, the cellular and molecular architecture of the human brain vasculature remains incompletely understood 1 . Here we performed single-cell RNA sequencing analysis of 606,380 freshly isolated endothelial cells, perivascular cells and other tissue-derived cells from 117 samples, from 68 human fetuses and adult patients to construct a molecular atlas of the developing fetal, adult control and diseased human brain vasculature. We identify extensive molecular heterogeneity of the vasculature of healthy fetal and adult human brains and across five vascular-dependent central nervous system (CNS) pathologies, including brain tumours and brain vascular malformations. We identify alteration of arteriovenous differentiation and reactivated fetal as well as conserved dysregulated genes and pathways in the diseased vasculature. Pathological endothelial cells display a loss of CNS-specific properties and reveal an upregulation of MHC class II molecules, indicating atypical features of CNS endothelial cells. Cell–cell interaction analyses predict substantial endothelial-to-perivascular cell ligand–receptor cross-talk, including immune-related and angiogenic pathways, thereby revealing a central role for the endothelium within brain neurovascular unit signalling networks. Our single-cell brain atlas provides insights into the molecular architecture and heterogeneity of the developing, adult/control and diseased human brain vasculature and serves as a powerful reference for future studies.
The brain vasculature is important for both the proper functioning of the normal brain as well as for a variety of vascular-dependent CNS pathologies such as brain tumours, brain vascular malformations, stroke and neurodegenerative diseases 1 , 2 , 3 , 4 , 5 , 6 , 7 , 8 , 9 . A better understanding of the underlying cellular and molecular mechanisms and architecture of the vasculature during brain development, in the healthy adult brain, as well as in vascular-dependent brain diseases, has broad implications for both the biological understanding as well as the therapeutic targeting of the pathological brain vasculature 10 , 11 , 12 , 13 , 14 , 15 . Vascular growth and network formation, involving endothelial cells (ECs) and other cells of the neurovascular unit (NVU), are highly dynamic during brain development, almost quiescent in the healthy adult brain and reactivated in a variety of angiogenesis-dependent brain pathologies, including brain tumours and brain vascular malformations 3 , 7 , 16 , 17 , 18 , 19 , 20 , 21 , thereby activating ECs and perivascular cells (PVCs) of the NVU and other tissue-derived cells (hereafter collectively referred to as PVCs). However, it is unclear which molecular signalling cascades are reactivated and how they regulate brain tumour and brain vascular malformation vascularization and growth.
The CNS vasculature has unique features such as the blood–brain barrier (BBB) and the NVU 22 , 23 , 24 . During development, various CNS-specific and general signalling pathways drive CNS angiogenesis 3 , 7 , 23 , 25 , 26 , 27 . The brain vasculature also displays an arteriovenous (AV) endothelial hierarchy similar to peripheral vascular beds 28 , 29 , 30 . Developmentally regulated signalling axes in ECs are thought to contribute to the establishment of CNS-specific properties as well as AV specification of the endothelium in the healthy adult brain and to their alteration in disease 13 , 14 . Over the past years, single-cell transcriptome atlases of human peripheral organs 31 , 32 , 33 , the human brain vasculature 34 , 35 , 36 , 37 , and the mouse brain and peripheral vasculature 28 , 38 were established. Nevertheless, a landscape of the human brain vasculature at the single-cell level across fetal development, adulthood and various vascular-dependent diseases with a focus on the brain vascular endothelium is lacking. Here we created a comprehensive molecular atlas of the human brain vasculature using single-cell RNA sequencing (scRNA-seq) analysis in the developing, adult/control and diseased human brain (Fig. 1a , Extended Data Fig. 1 and Supplementary Methods ). We identified extensive heterogeneity among ECs as well as common hallmarks across a spectrum of multiple brain pathologies, including commonly regulated angiogenic signalling pathways that significantly overlap with the fetal signalling axes, altered AV specification and CNS specificity, upregulation of MHC class II signalling and strong EC–EC/EC–PVC communication networks.
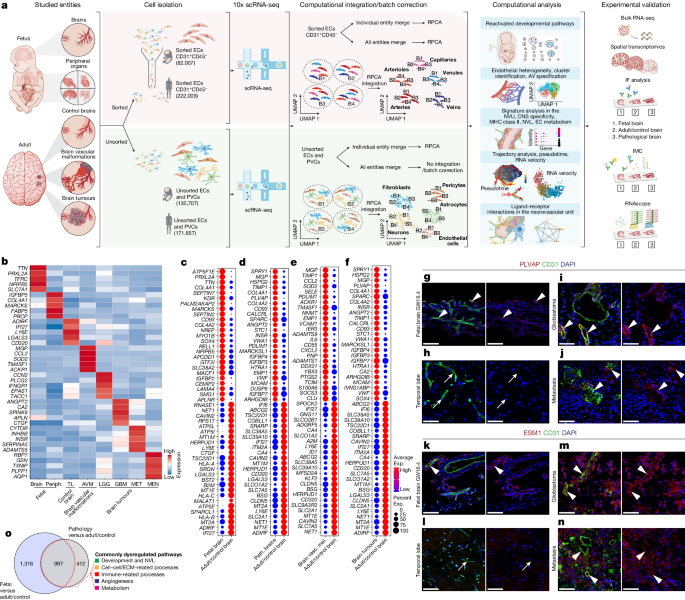
a , Schematic of the experimental workflow including scRNA-seq, computational analysis summary and validation experiments. b , Expression heat map of the top ranking marker genes in the indicated tissues. For the colour scale, red shows high expression, white shows intermediate expression and blue shows low expression. c – f , Dotplot heatmap of the fetal versus adult/control brain endothelium ( c ); pathological (path.) versus adult/control brain endothelium ( d ); brain vascular malformations (brain vasc. mal.) versus adult/control brain endothelium ( e ); and brain tumours versus adult/control brain endothelium ( f ) signatures based on differential gene expression analyses. g – n , IF imaging of tissue sections from the indicated entities, stained for PLVAP (red; g – j ), ESM1 (red; k – n ) and CD31 (green). Nuclei are stained with DAPI (blue). The arrowheads indicate expression of PLVAP or ESM1 in blood-vessel ECs in the different tissues, and the arrows indicate the absence of expression in blood-vessel ECs in the different tissues. For g – n , scale bars, 50 μm. o , The overlap between the 2,313 significant pathways enriched in fetal brain ECs as compared to adult/control brain ECs and the 1,409 significant gene sets enriched in pathological brain ECs as compared to adult/control brain ECs. ECM, extracellular matrix; NVL, neurovascular link; periph., periphery; RPCA, reciprocal principal component analysis; UMAP, uniform manifold approximation and projection.
Constructing a sc-atlas of the human brain vasculature
We constructed a human brain vasculature single-cell atlas (sc-atlas) using samples from fetal as well as adult control (undiseased atlas) and diseased brains, including adult brain vascular malformations and brain tumours (diseased atlas). We acquired freshly isolated cells (both fluorescence-activated cell sorting (FACS)-sorted ECs and unsorted ECs and PVCs; Supplementary Tables 1 – 4 ) from 8 individual fetuses 39 , 40 , 41 and from 61 adult brain samples (from 61 individual patients), covering adult temporal lobe (TL) controls and adult vascular-dependent pathologies, including brain vascular malformations, namely, brain AV malformation (AVM) 36 , 42 , and brain tumours, notably, lower-grade glioma (LGG) 22 , 43 , glioblastoma (GBM) 22 , 44 , 45 , lung cancer brain metastasis (MET) 22 , 46 and meningioma (MEN) 47 (Fig. 1a , Extended Data Fig. 1a,b and Supplementary Tables 1 – 4 ). Brain tissue samples were dissociated into single-cell suspensions, which were either FACS-sorted for ECs (CD31 + CD45 − ) or processed as unsorted samples to examine all cells of the NVU (Fig. 1a ). Single-cell transcriptomes were collected using the 10x Genomics Chromium system 48 and analysed. CD31 + CD45 − ECs showed consistent expression of classical endothelial markers, such as CD31 , VWF and CLDN5 , while not expressing PVC markers (Supplementary Fig. 1a–o ), thereby confirming the purity of EC isolations. In summary, 606,380 single cells, including 304,016 sorted ECs and 302,364 unsorted ECs and PVCs, passed the quality-control criteria (Fig. 1a and Supplementary Tables 3 – 5 ). The number of sorted ECs analysed here substantially exceeds the number of ECs analysed using scRNA-seq 36 , 37 or single-nucleus RNA-seq 34 , 35 in previous studies, and we directly compared single-cell transcriptomics of sorted ECs from the vasculature of the fetal and adult brain and of various brain pathologies. Notably, we report higher numbers of sorted ECs and of unsorted ECs and PVCs in the different brain entities compared with previous studies 34 , 35 , 36 , 37 , 49 , enabling us to assess EC heterogeneity across development, adulthood and disease at a high resolution.
To address the role of the endothelium within the brain NVU across different entities, fetal, adult/control and pathological unsorted EC and PVC transcriptomes from 31 patients were analysed (Fig. 1a and Supplementary Fig. 2a–f ). We identified 18 major brain cell types, including all known vascular, perivascular and other tissue-derived cell types in the human brain. The detected cell type distributions within the NVU differed between the fetal, adult control and pathological brain samples (Supplementary Figs. 2e–g and 3a–n and Supplementary Tables 10 – 16 ). Key signatures and differentially expressed genes (DEGs) were validated using bulk RNA-seq, RNAscope, spatial transcriptomics, immunofluorescence (IF) and imaging mass cytometry (IMC) (Fig. 1a ).
We next compared ECs in the sorted samples across entities and found that ECs from different entities exhibited prominent transcriptomic heterogeneity (Extended Data Fig. 1c,f ) as well as distinct gene expression signatures (Fig. 1b–f , Extended Data Fig. 1d,e,g,h and Supplementary Fig. 5 ). We defined major EC signatures, including a human fetal CNS (and peripheral) signature characterizing CNS and periphery-specific markers of the fetal vasculature (Extended Data Fig. 1g and Supplementary Table 6 ), a human fetal/developmental CNS/brain signature revealing properties of the developing and mature human brain vasculature, and a pathological signature of the diseased brain vasculature including a brain vascular malformation and a brain tumour signature (Fig. 1d–f , Supplementary Fig. 5a–c and Supplementary Table 25 ). The fetal and pathological brain EC signatures revealed differential expression of the well-known angiogenic markers PLVAP and ESM1 36 , 38 , 50 , 51 , 52 , 53 , 54 , 55 , 56 , which we confirmed in the fetal and diseased brain entities using IF analysis 36 , 38 , 52 , 53 , 54 , 55 , 56 (Fig. 1g–n and Extended Data Fig. 2 ).
Although all of the entities revealed distinct EC markers, some EC markers were conserved across two or more entities (such as ADIRF , EGR1 , PLPP1 and ANGPT2 ) (Fig. 1b , Extended Data Fig. 1e,h and Supplementary Tables 8 and 9 ).
Reactivation of fetal programmes in pathological brain ECs
We further assessed the differences in ECs across developmental stages and in pathological conditions (Supplementary Tables 7 and 25 ). DEGs between the fetal and adult/control stage and between the adult/control and pathological brain showed developmental and pathology-specific gene and pathway enrichments (Supplementary Fig. 5d–f ), providing insights into functional specialization of the human brain vasculature across development, homeostasis and disease (Supplementary Fig. 5 ). Using various approaches, including statistical regression, we found no evidence that age and sex 57 , 58 , 59 are confounders of our findings (Supplementary Tables 13 and 14 ). The top differentially regulated pathways in both fetal versus adult/control as well as in pathological versus adult/control brain EC signatures belonged to five main groups, including development and neurovascular link 3 , cell–cell/extracellular-matrix-related processes, immune-related processes, angiogenesis and metabolism (Supplementary Fig. 5d–f ). Notably, of the 1,409 differentially regulated pathways in pathology versus adult/control brain ECs, more than half (997) also showed differential regulation in fetal versus adult/control brain ECs (Fig. 1o and Supplementary Fig. 5f ), highlighting the importance of developmental pathways in vascular-dependent brain pathologies. Bulk RNA-seq analysis confirmed the scRNA-seq findings, including the dysregulated pathways across pathologies (Supplementary Fig. 6 and Supplementary Table 26 ). Together, these data indicate that signalling axes driving vascular growth during fetal brain development are silenced in the adult control brain and reactivated in the vasculature of brain tumours and brain vascular malformations and that common dysregulated pathways are observed in the pathological brain vascular endothelium across diseases.
Inter-tissue heterogeneity and AV zonation of brain ECs
To further address EC heterogeneity across different brain entities at the single-cell level, we pooled, integrated and batch-corrected (using RPCA) 60 , 61 , 62 , 63 , clustered and visualized all fetal (21,512), adult/control (76,125) and pathological (145,884) sorted brain EC transcriptomes from 43 patients (Fig. 2a and Supplementary Figs. 7a–e and 9 ). Brain vascular ECs are organized along the human brain AV axis, referred to as AV zonation 28 , 64 , 65 , 66 , 67 , 68 , 69 . Endothelial clusters were biologically annotated using DEGs across entities, and we identified 44 EC subclusters (Fig. 2e , Supplementary Fig. 7 and Supplementary Table 18 ) that were arranged according to AV zonation, which we grouped into 14 major EC clusters for further downstream analysis (Fig. 2a and Supplementary Fig. 7a,h ).
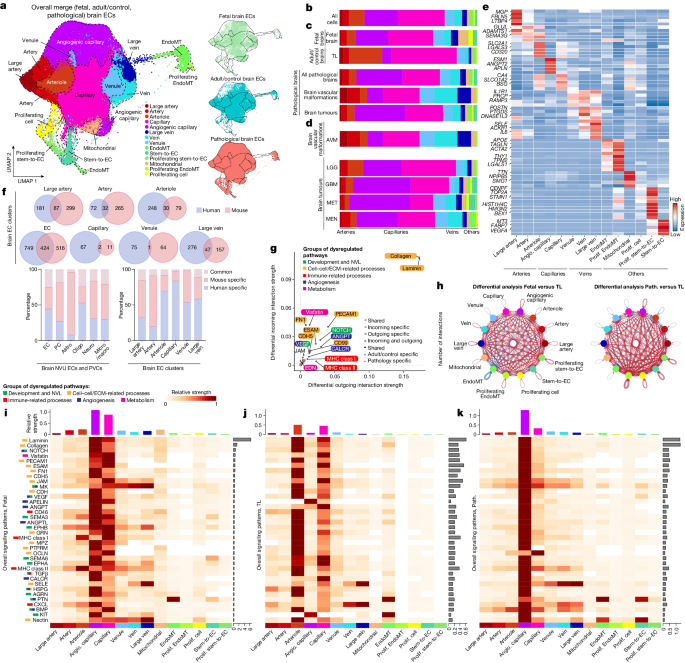
a , UMAP plot of the 243,521 integrated/batch corrected fetal, adult/control and pathological brain ECs across 5 (fetal), 9 (adult/control) and 29 (pathological) individuals (Supplementary Table 3 ), colour coded by EC AV specification, and UMAP plots split by tissue of origin: fetal brain (5 individuals), adult/control brain (9 individuals) and brain pathologies (29 individuals). b – d , The relative abundance of EC subtypes (AV specification cluster) from the indicated tissue of origin. b – d are coloured according to the colour code in a (Supplementary Table 10 ). The number of individuals analysed was as follows: n = 43 (all entities), n = 5 (fetal brain), n = 9 (adult/control brain (TL)), n = 29 (all pathological brains), n = 5 (brain vascular malformations), n = 24 (brain tumours), n = 5 (AVM), n = 6 (LGG), n = 8 (GBM), n = 5 (MET) and n = 5 (MEN). e , The top ranking marker gene expression levels in different EC subtypes. For the colour scale, red shows high expression, white shows intermediate expression and blue shows low expression. Angio., angiogenic; prolif., proliferating. f , The overlap between human and mouse AV specification markers (of large artery, artery, arteriole, capillary, venule and large vein) and endothelial (EC) markers (top). Bottom, the percentage of common, human-specific and mouse-specific cell/AV specification markers. Astro, astrocytes; micro/macro, microglia/macrophages; neuro, neurons; PC, pericytes. g , Scatter plot showing the differential incoming and outgoing interaction strength of pathways in angiogenic capillaries, identifying signalling changes in those cells in pathological as compared to the control conditions. h , The number of statistically significant ligand–receptor interactions between EC subtypes in fetal versus adult/control brains (left) and pathological (path.) versus adult/control brains (right). The circle plots show a differential analysis of the intercellular signalling interactions; red indicates upregulation and blue indicates downregulation. i , j , k , The overall signalling patterns of different EC subtypes in fetal ( i ), adult/control ( j ) and pathological ( k ) brains. Grey bars indicate signalling strength.
We characterized AV zonation markers 28 , 70 with arterial (subclustered into large artery, artery, arteriole) and venous (subclustered into large vein, vein, venule) clusters located at the opposite ends of the uniform manifold approximation and projection (UMAP), separated by major capillary clusters (subdivided into capillary and angiogenic capillary) in the fetal, adult and pathological brains (Fig. 2a,e , Supplementary Figs. 7 and 10 and Supplementary Tables 18 and 19 ), providing unprecedented transcriptional resolution by AV zonation 34 , 35 , 36 .
While we confirmed differential expression of known marker genes of AV specification 28 , 38 , we also identified AV-zonation markers that have not to our knowledge been identified previously in the human brain: LTBP4 (large arteries); ADAMTS1 (arteries); VSIR , AIF1L , CD320 and others (arterioles); SLC38A5 , BSG , SLC16A1 and SLCO1A2 (capillaries); JAM2 , PRCP , PRSS23 and RAMP3 (venules); PTGDS , POSTN and DNASE1L3 (veins); CCL2 (large veins); and PLVAP , ESM1 and CA2 (angiogenic capillaries) (Fig. 2e , Supplementary Fig. 7i and Supplementary Tables 18 and 19 ). PLVAP and ESM1 were among the top markers of the angiogenic capillary cluster and we confirmed PLVAP and ESM1 expression in diseased brain entities and in the fetal brain, indicating its role in developmental and pathological vascular growth 50 , 51 . Indeed, PLVAP and ESM1 exhibited RNA and protein expression in human fetal brain and human brain vascular malformation/tumour ECs on the basis of RNAscope and IF analysis (Extended Data Fig. 2 ).
We also assigned EC clusters outside AV zonation, notably (proliferating) stem-to-endothelial cell transdifferentiating (stem-to-EC) clusters and (proliferating) endothelial-to-mesenchymal transition (EndoMT) clusters (Fig. 2e and Supplementary Fig. 7a,i ), for which we identified specific molecular markers. We found proliferating ECs (such as TOP2A and MKI67 ) in the fetal (3.54%), adult (0.4%) and pathological brains (1.37%) (Fig. 2e , Supplementary Fig. 7i and Supplementary Table 19 ). We identified EndoMT clusters expressing both mesenchymal (such as APOE , ACTA2 and TAGLN ) and endothelial markers 71 (Fig. 2e , Supplementary Fig. 7i and Extended Data Fig. 2 q i –b ii ,g ii –j ii ′). Notably, we observed two subsets of EndoMT ECs (proliferating EndoMT and EndoMT) that were increased in certain pathologies (MEN > LGG > GBM). Proliferating EndoMT ECs expressed both EndoMT and proliferation markers (for example, ACTA2 and MKI67 ) (Fig. 2e , Supplementary Fig. 7i and Supplementary Tables 8 and 9 ). Using RNAscope, IF and IMC, we found ACTA2 + CD31 + CLDN5 + co-expressing but PDGFRβ − (suggesting no pericyte identity) ECs across pathologies (Extended Data Fig. 2 q i –b ii ,g ii –j ii ′,k ii –q ii 5 ), indicating the presence of EndoMT ECs in the diseased vasculature. In GBMs and METs, we observed stem-to-EC clusters that expressed classical EC markers (such as CD31 , CLDN5 , CDH5 and VWF ) to a lower level compared with other EC clusters, as well as some markers of (tumour) stem cells (Fig. 2e , Extended Data Fig. 3 and Supplementary Fig. 7i ), suggesting ECs undergoing stem-to-EC transdifferentiation. In GBMs, we identified a stem-to-EC cluster expressing the GBM stemness markers SOX2 , PTPRZ1 , POUR3F2 and OLIG1 72 , 73 , and EC markers 74 , 75 (Supplementary Fig. 7i and Extended Data Fig. 3 a–i,a i –n i ′). In METs, we noted a previously undescribed stem-to-EC population that co-expressed EC markers and stem cell markers of lung cancers (for example, SOX2 , EPCAM , CD44 and SFTPB ) 76 (Extended Data Fig. 3 n–v,g i –z i ′ and Supplementary Fig. 7i ). In GBMs and METs, we identified groups of stem-to-ECs that co-expressed stemness (for example, SOX2 , PTPRZ1 , EPCAM1 and SFTPB ) and proliferation markers (for example, MKI67 , BEX1 , HMGB2 and UBE2C ) (Fig. 2e and Extended Data Fig. 3 ). To validate the stem-to-EC clusters in GBM and MET, we used double immunostaining for EC and stemness markers. In GBM, we found SOX2 + CD31 + and PTPRZ1 + CD31 + co-expressing ECs, whereas, in MET, we observed EPCAM + CD31 + and SFTBP + CD31 + co-expressing ECs (Extended Data Fig. 3 ). The confirmation of tumour stemness marker enrichment in a subset of tumour ECs suggests the presence of stem-to-ECs in GBM and MET vasculature.
We next addressed the distributions of EC clusters between the fetal, adult/control and pathological brains. Capillaries accounted for around 60.5% of ECs, arterial ECs accounted for 18.2% and venous ECs accounted for 16.2%, in agreement with previous studies 3 , 17 . We further uncovered previously unrecognized EC heterogeneity across a wide range of human brain tissues (Fig. 2b–d , Supplementary Fig. 10 and Supplementary Tables 10 – 16 ). Angiogenic capillary proportions were significantly higher in the fetal brain and in brain tumours (GBM > MET > MEN > LGG), illustrating their angiogenic capacity 3 , 13 , 22 , 77 , whereas brain vascular malformations (AVM) revealed significantly elevated proportions of venous clusters, indicating their venous character 78 , 79 (Fig. 2c,d , Supplementary Fig. 10 and Supplementary Table 12 ). We next evaluated whether AV-zonation markers were conserved across species 34 , 35 , 36 , 49 , 80 (Supplementary Fig. 4 ). Although the overall structure of AV zonation was conserved between human and mouse, the number of conserved AV-zonation genes in the different AV compartments was low. Accordingly, we found the highest proportion of human-specific AV-zonation markers in small > large-calibre and venous > arterial vessel ECs (Fig. 2f , Supplementary Fig. 4 z xxix ,z xxx and Supplementary Table 17 ), and we validated these human-specific markers referring to the Human Protein Atlas (HPA) 35 , 81 , 82 , 83 (Supplementary Fig. 8 ).
In the brain NVU, mapping of our dataset to the freshly isolated mouse dataset 70 revealed high transcriptomic similarity between species for ECs and PVCs 84 (Supplementary Fig. 4 z xvii–xvix ). We further observed that neurons and astrocytes showed the greatest transcriptional divergence (Fig. 2f and Supplementary Table 17 ), while ECs and oligodendrocytes displayed the highest percentage of human-specific markers, in agreement with previous studies 34 , 35 , 36 . These species-specific differences along AV-zonation suggest fundamental disparities in brain vascular properties, indicating the necessity to directly study sorted/enriched ECs and PVCs of the human brain vasculature at the single-cell level.
As EC clusters reside in close proximity to each other along the AV tree, we next inferred cell–cell communication pathways 85 , 86 . Differential analysis revealed increased cellular cross-talk among EC clusters in pathological and fetal ECs, highlighting a key role for angiogenic capillaries. Angiogenic capillaries displayed upregulation of several signalling pathways, including the five above-mentioned groups in both the diseased and fetal (versus adult/control) brain (Fig. 2g–k and Supplementary Fig. 11 ), highlighting this cluster as a major signalling mediator within brain EC networks.
Alteration of AV specification in pathological brain ECs
Failure of proper AV specification in brain vascular malformations and formation of tortuous arteries and veins in brain tumours has been reported 77 , 87 , but AV specification in brain pathologies and fetal (brain) development remains poorly understood. We ordered ECs along a one-dimensional transcriptional gradient using Monocle 88 and TSCAN 89 to examine the AV axis in the different entities. Whereas arterial and venous markers peaked at opposite ends, capillary markers showed peaks in the mid-section throughout all entities (Fig. 3b,f,j , Extended Data Fig. 4b,f,j,n,r,v and Supplementary Fig. 13III ), indicating that in silico pseudospace and pseudotime recapitulate in vivo anatomical topography of EC clusters in the human brain vasculature 28 , 38 . We observed AV zonation throughout the fetal, control and pathological brains, but observed a partial alteration of EC ordering along the AV axis in disease (Fig. 3b,c,f,g,j,k and Extended Data Fig. 4 ).
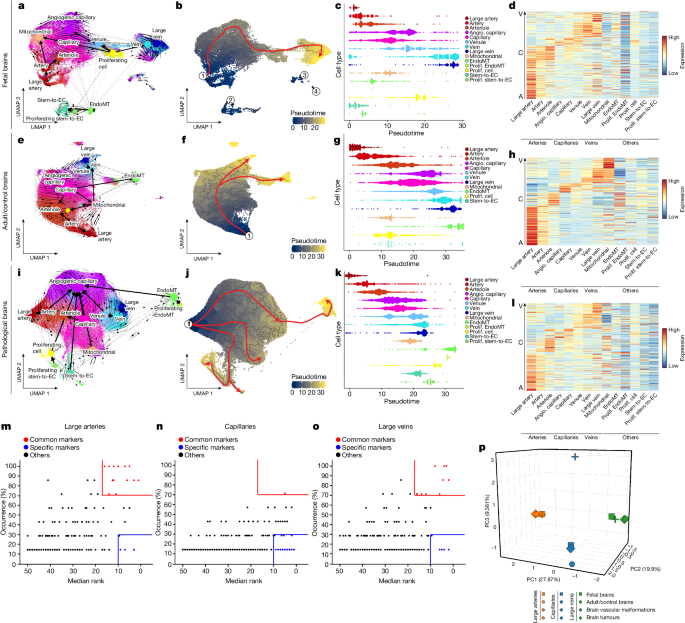
a , e , i , UMAP plots of human brain ECs isolated from fetal brains (21,512 ECs from 5 individuals; a ), adult/control brains (76,125 ECs from 9 individuals; e ) and pathological brains (145,884 ECs from 29 individuals; i ), coloured by AV specification. RNA velocity streamlines and partition-based graph abstraction (PAGA) vectors extended by velocity-inferred directionality are superimposed onto the UMAPs. b , f , j , UMAP plots of human brain ECs isolated from fetal brains ( b ), adult/control brains ( f ) and pathological brains ( j ), coloured by pseudotime. The red line, which was drawn manually, indicates the major trajectory flow. c , g , k , Pseudotime order of ECs, colour coded according to AV specification from fetal brains ( c ), control adult/control brains ( g ) and pathological brains ( k ). d , h , l , Heat map of adult/control brain EC AV specification signature gene expression in human brain ECs isolated from fetal brains ( d ), adult/control brains ( h ) and pathological brains ( l ). A, arterial; C, capillary; V, venous. m , n , o , Common and tissue-specific markers in ECs from large arteries ( m ), capillaries ( n ) and large veins ( o ) in different tissue types (fetal brain, adult/control brain, brain vascular malformations and brain tumours). The red boxes highlight conserved markers between ECs from different tissues; the blue boxes highlight tissue-specific markers. Dots are coloured as defined in the legend. p , Three-dimensional principal component analysis visualization of pairwise Jaccard similarity coefficients between the indicated ECs from the different tissues.
We defined an AV signature comprising genes revealing significant expression gradients of ECs along the AV axis (Fig. 3d,h,l and Extended Data Fig. 4d,h,l,p,t,x ). The seamless zonation continuum was recapitulated in all entities but again showed alteration in pathologies. While AV markers revealed a clear distinction between AV compartments in the fetal and adult/control brain, showing specific markers of large arteries (for example, VEGFC , FBLN5 ), arterioles (such as LGALS3 , AIF1L ), capillaries (for example, SLC35A5 , MFSD2A ), angiogenic capillaries (such as ESM1 , ANGPT2 ), venules (for example, JAM2 and PRCP ) and large veins (such as SELE and SELP ), some zonation markers showed a less-specific presence in pathologies (Figs. 2 and 3d,h,l and Extended Data Fig. 4d,h,l,p,t,x ).
Whereas almost all fetal and pathological ECs were quite similar to temporal-lobe EC clusters 60 , small-calibre vessel ECs were more different compared with their healthy temporal lobe counterparts (Extended Data Fig. 4 z v –z viii ), probably pertaining to a higher vulnerability of small-calibre vessel ECs to alterations in the local tissue microenvironment 38 .
To further address lineage relationships in AV specification, we referred to RNA velocity 90 , 91 and diffusion map 92 , revealing vectors from multiple EC clusters towards the angiogenic capillary cluster in angiogenic entities (tumours > fetal brain > vascular malformations), whereas, in vascular malformations, we observed vectors from various EC clusters towards venous clusters (Fig. 3a,e,i , Extended Data Fig. 5 and Supplementary Fig. 13 ). These results indicate that RNA velocity can suggest timeline relationships among human brain vascular ECs 23 , 29 .
We next addressed whether EC markers of AV clusters were conserved between vascular beds or expressed in a more tissue-specific manner 38 . While we identified multiple conserved markers for large arteries and large veins, capillaries were more tissue/entity specific, indicating a more pronounced transcriptional heterogeneity of the capillary bed across the different brain tissues 38 . Accordingly, capillaries showed more tissue-specific markers than large-calibre vessels (Fig. 3m–p ), indicating a higher susceptibility of capillary ECs to the local tissue microenvironment.
Alteration of CNS specificity in pathological brain ECs
We next examined CNS-specific properties distinguishing brain ECs from ECs outside the CNS 3 , 5 . Bulk RNA-seq analysis in mice revealed a BBB-enriched transcriptome 93 , but how the human brain EC CNS properties differ at the single-cell level and whether they are heterogeneous across developmental stages and in disease remains largely unclear. The development of the human fetal BBB (occurring between gestational week 8 and 18) 39 , 40 , 94 is controversial 41 , 95 . We therefore studied human fetal BBB development at a high resolution. Referring to a human/mouse BBB signature (Supplementary Table 20 and Extended Data Fig. 6a–j ), we observed an increased BBB signature expression with increased gestational age (Extended Data Fig. 6b–j ), in agreement with a previous study 96 . Along the AV compartments, the BBB signature revealed a higher expression in small- versus large-calibre vessels across developmental stages (Extended Data Fig. 6d ), in agreement with previous findings 97 and probably pertaining to the susceptibility of capillaries to the local microenvironment 38 .
Next, to address molecular differences of CNS and peripheral ECs at the single-cell level, we defined a human adult and fetal CNS signature (Fig. 4a–f , Supplementary Fig. 14l–q and Supplementary Table 20 ). These include known BBB ( MFSD2A 98 and CLDN5 99 ) and capillary markers ( CA4 28 , 100 and SPOCK2 38 ), as well as novel genes enriched in the CNS vasculature such as SPOCK3 , BSG and CD320 (Supplementary Fig. 14b,e and Supplementary Table 20 ). The adult and fetal CNS signatures showed elevated expression with increasing gestational age and in small- versus large-calibre vessels across developmental stages, reminiscent of the BBB signature expression pattern (Fig. 4g and Extended Data Fig. 6d ).
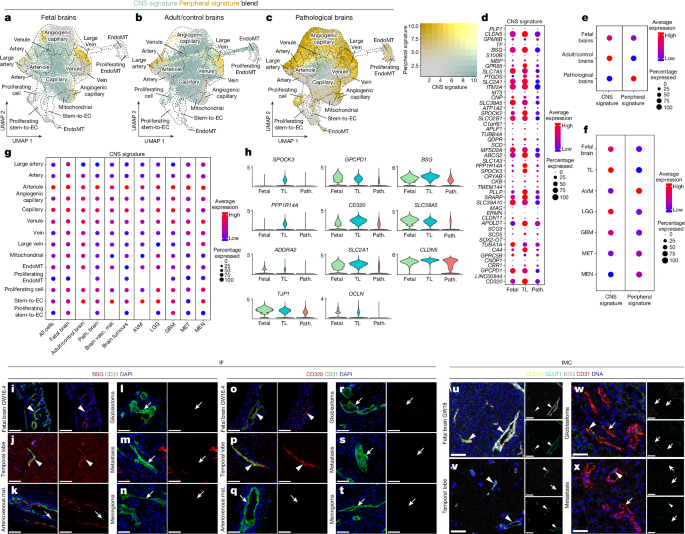
a – c , UMAP plots of the ECs from fetal brains (21,512 ECs from 5 individuals; a ), adult/control brains (76,125 ECs from 9 individuals; b ) and pathological brains (145,884 ECs from 29 individuals; c ). Plots are colour coded for CNS signature (green) and peripheral signature (yellow). d , CNS signature genes expression in fetal brain, adult/control brains (temporal lobes) and pathological brain ECs. e , f , CNS and peripheral signature expression in fetal brain, adult/control brain and pathological brain ECs ( e ) and in each individual entity ( f ). g , The CNS signature at the level of AV specification for the indicated entities. For the colour scale, red shows high expression and blue shows low expression. The dot size represents the percentage expression within the indicated entity. h , The expression of representative CNS-specific and BBB marker genes for fetal brain versus adult/control brain versus pathological brain ECs. i – t , IF images for the protein expression of BSG ( i – n ) and CD320 ( o – t ) in fetal brain ( i and o ), adult/control brain (TL; j and p ), brain AVMs ( k and q ), GBM/high-grade glioma ( l and r ), metastasis ( m and s ) and meningioma ( n and t ). For i – t , scale bars, 80 μm (fetal brain) and 50 μm (adult control and pathological brains). u – x , IMC imaging of fetal brain ( u ), adult/control brain (TL; v ), GBM/high-grade glioma ( w ) and metastasis ( x ) tissue samples visualizing five metal-conjugated antibodies (Supplementary Table 24 ). Representative pseudocolour images of CLDN5 , GLUT1 , BSG and CD31 combined, and individual GLUT1 and BSG channels are shown; white, overlap; yellow, CLDN5 ; cyan, GLUT1 ; grey, BSG ; red, CD31 ; blue, DNA intercalator. For u – x , scale bars, 50 μm. The arrowheads indicate blood-vessel ECs expressing the indicated markers in the different tissues. The arrows indicate blood-vessel ECs not expressing the indicated markers in the different tissue.
CNS properties were observed in the fetal and adult brains, whereas alteration of the CNS signature and concomitant acquisition of the peripheral signature was seen in pathologies (Fig. 4a–f and Supplementary Figs. 14 and 15 ). Comparing the CNS signature between pathological and adult/control brain ECs, we found downregulation of SLC2A1 , which is dysregulated in neurodegenerative conditions 69 ; the lipid transporter MFSD2A , which is expressed in brain ECs and restricts caveolae-mediated transcytosis at the BBB 98 , 101 , 102 ; and the BBB marker CLDN5 (Fig. 4d–f and Supplementary Table 20 ), therefore suggesting BBB alteration in pathologies 22 . The CNS signature was highest in the temporal lobe, followed by intra-axial primary brain tumours and fetal brain (LGG > fetal brain > GBM), brain vascular malformations, intra-axial secondary brain tumour MET and extra-axial brain tumour MEN, whereas the peripheral signature followed an inversed pattern (Fig. 4e,f and Supplementary Fig. 14p,q ).
We next addressed CNS-specific properties along the AV axis. In the fetal and adult brain, the CNS signature was mainly expressed by small-calibre vessels, while the peripheral signature was predominantly present in large arteries and large veins. We observed a similar pattern in pathological brains with, however, a notable decrease in cells expressing the CNS signature (most pronounced for angiogenic capillaries > capillaries), paralleled by an increase in cells expressing the peripheral signature predominantly for angiogenic capillaries and large-calibre vessels (Fig. 4g and Supplementary Fig. 14h ).
We next examined how the CNS, BBB and peripheral signatures changed along the AV axis in each pathological entity. The CNS and BBB signatures were downregulated in every pathology with a similar pattern to that described above and reaching the highest baseline values of CNS specificity at the capillary and arteriole levels, with the capillaries being the cluster mostly affected by pathologies 38 (Fig. 4g and Supplementary Fig. 14h ), probably pertaining to the influence of the local microenvironment for small-calibre vessels. The peripheral signature was upregulated in disease, peaking for AVM > MEN > MET > GBM and lower expression for LGG, predominantly affecting large-calibre vessels and angiogenic capillaries (Fig. 4h and Supplementary Figs. 14 and 15 ). These data indicate that CNS ECs acquire CNS-specific properties during fetal-to-adult transition and take on a peripheral EC signature in disease conditions.
The CNS and BBB signatures are tightly linked to a functional BBB in vivo 103 and BBB dysfunction affects the CNS properties of ECs 93 . We therefore investigated the human and mouse BBB dysfunction modules, with the latter being upregulated in CNS ECs after various disease triggers in the mouse brain, shifting CNS ECs into peripheral EC-like states 93 . We found that the human and mouse BBB dysfunction modules were upregulated in human brain tumours and brain vascular malformations as well as in the fetal brain (Supplementary Fig. 16a–n ), probably due to pathways related to BBB dysfunction 93 . Both the human and mouse BBB dysfunction modules were highest in AVM > GBM > MET > MEN (Supplementary Fig. 16a–n ). The BBB dysfunction modules expression along the AV axis revealed enrichment in large-calibre vessels and angiogenic capillaries, mimicking the peripheral signature expression, again indicating that pathological CNS ECs take on a peripheral endothelial gene expression 93 (Supplementary Fig. 16h–n ). Comparison to BBB dysfunction modules in human Alzheimer’s disease 35 , Huntington’s disease 34 and AVMs 36 revealed some overlap with the human and mouse BBB dysfunction modules 93 (Supplementary Fig. 16o–s and Supplementary Table 21 ), indicating common and distinct features among brain diseases.
We confirmed decreased expression of the CNS-signature genes SPOCK3 , BSG , CD320 , PPP1R14A and SLC38A5 in all brain tumours and brain vascular malformations (Fig. 4h–x and Extended Data Figs. 6k–t′ 4 , 9 and 10 ) using IF and IMC, thereby highlighting the alteration of CNS properties in the diseased human cerebrovasculature.
Upregulation of MHC class II in pathological brain ECs
We identified EC populations expressing the MHC class II genes CD74 , HLA-DRB5 , HLA-DMA , HLA-DPA1 and HLA-DRA in pathological CNS tissues. This antigen-presenting signature, indicating possible immune functions of human brain ECs, prompted us to investigate the heterogeneity of MHC class II transcripts between tissues at the single-cell level.
scRNA-seq identified endothelial MHC class II expression in peripheral human and mouse tissues 31 , 100 , but assessment of MHC class II expression in human brain vascular beds at the single-cell level is lacking. To assess MHC class II gene expression across brain development and disease, we defined a human MHC class II signature including MHC class II receptors (Fig. 5d and Supplementary Table 22 ). The MHC class II signature was upregulated in pathologies, and low in the fetal brain (Fig. 5a–f and Extended Data Fig. 9 ), in agreement with a previous study 31 . We found that the MHC class II signature was highest in AVM > MEN > MET, followed by LGG > GBM, the temporal lobe and the fetal brain, grossly following the peripheral signature expression gradient (Fig. 5f ). We examined MHC class II signature expression patterns according to AV zonation. Whereas, in the fetal brain, only very few ECs (large arteries and arterioles) expressed the MHC class II signature, mainly large-calibre vessel (large arteries and large veins) ECs expressed a signature of genes involved in MHC class II-mediated antigen presentation in the adult brain (Fig. 5a–c,g ).
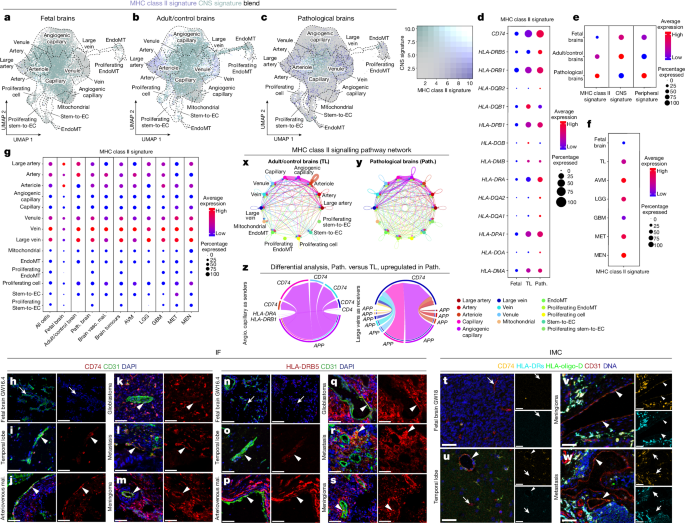
a – c , UMAP plots of ECs from fetal (21,512 ECs from 5 individuals; a ), adult/control (76,125 ECs from 9 individuals; b ) and pathological brains (145,884 ECs from 29 individuals; c ). Plots are colour coded for MHC class II (violet) and CNS (green) signatures. d , MHC class II signature gene expression in fetal, adult/control (temporal lobes) and pathological brain ECs. e , f , MHC class II, CNS and peripheral signature expression in fetal, adult/control and pathological brain ECs ( e ), and MHC class II signature expression in each individual entity ( f ). g , The MHC class II signature at the level of AV specification for the indicated entities. For the colour scale, red shows high expression and blue shows low expression. The dot size represents the percentage expression within the indicated entity. h – s , IF images for the protein expression of CD74 and HLA-DRB5 in the fetal brain ( h and n ) and the adult/control brain (TL; i and o ), in brain AVMs ( j and p ), in GBM/high-grade glioma ( k and q ), in metastasis ( l and r ) and in meningioma ( m and s ). For h – s , scale bars, 80 μm (fetal brain) and 50 μm (adult control and pathological brains). t – w , IMC imaging of fetal brain ( t ), adult/control brain ( u ), meningioma ( v ) and metastasis ( w ) tissue samples visualizing metal-conjugated CD74 , pan-HLA-DR, oligo-HLA-D and CD31 primary antibodies. An overlay of pseudocolour images as well as individual channels for CD74 and pan-HLA-DR are shown; white, overlap; orange, CD74 ; cyan, pan-HLA-DR; green, oligo-HLA-D; red, CD31 ; blue, DNA intercalator. For t – w , scale bars, 50 μm. x , y , The strength of MHC class II signalling interactions between the different EC subtypes of the adult/control brain ( x ) and pathological brain ( y ) ECs at the AV specification level. z , Differential analysis of MHC class II ligand–receptor pairs. Chord/circos plots showing upregulated MHC class II signalling in angiogenic capillaries as the source and all other cell clusters as targets (left), and large veins as receivers (right). The edge thickness represents its weight. The edge colour indicates the sender cell type. The arrowheads indicate ECs expressing the indicated markers. The arrows indicate ECs not expressing the indicated markers.
The MHC class II signature was upregulated in all pathologies according to the pattern described above 38 (Fig. 5a–c,g–z ). We observed a partial overlap of the MHC class II and peripheral signatures and of the BBB dysfunction module with a common predominance in large-calibre vessels, but a more widespread/stronger expression of the peripheral signature and BBB dysfunction module in angiogenic capillaries consistent with previous findings 69 . These data suggest that pathological CNS ECs upregulate MHC class II receptors in brain tumours and brain vascular malformations.
We confirmed enrichment of MHC class II genes including CD74 and others in the pathological human cerebrovasculature using IF, IMC and RNAscope (Fig. 5h–w and Extended Data Figs 10 , 11 , 12r and 13 ).
Spatial transcriptomics confirmed spatial co-localization of MHC class II ligands and receptors on ECs in the temporal lobe and in GBM (Supplementary Fig. 18 ). MHC class II signalling seems to be mediated mainly by APP , COPA and MIF ligands and the CD74 receptor in AV clusters (Supplementary Fig. 17 ), and APP–CD74 , COPA–CD74 and MIF–CD74 have been described as ligand–receptor pairs 104 , 105 .
A key role for ECs in the human brain NVU
Single-cell transcriptomics of unsorted ECs and PVCs offers the opportunity to address cellular cross-talk and the role of ECs within the NVU. To address cell–cell interactions across entities, we constructed ligand–receptor interaction maps 85 , 86 . In the majority of entities, ECs were at the centre of the network displaying numerous interactions with other ECs and PVCs (Extended Data Fig. 12a–i and Supplementary Fig. 19 ), indicating a crucial role of ECs in NVU function and EC–PVC cross-talk. In fetal and adult/control brains, ECs showed most interactions with fibroblasts, pericytes and astrocytes (Extended Data Fig. 12a–f ). In brain pathologies, ECs displayed increased interaction numbers as well as increased interactions with immune cells (Extended Data Fig. 12g–i and Supplementary Figs. 19 and 20 ). Intercellular signalling pathways were substantially increased in fetal and pathological ECs (and PVCs) (Extended Data Fig. 12j,k and Supplementary Fig. 20a–g ). Cell–cell communication analysis predicted upregulation of signalling pathways in the developing versus control brain as well as diseased versus control brain NVUs, including similar pathways as observed among EC clusters in EC–EC networks (Fig. 2i–k , Extended Data Fig. 12l–n and Supplementary Fig. 20k–n ). Intercellular cross-talk analysis predicted a key role for the ECs within the fetal, adult/control and diseased brain NVU signalling networks (Extended Data Fig. 12l–n and Supplementary Fig. 20e–g ).
During fetal brain development and in brain pathologies, we observed upregulation of ligands and receptors on ECs and PVCs as well as of the corresponding pathways, which partially overlapped with the ones in EC–EC cross-talk (Extended Data Fig. 12l–n ), suggesting that these ligand–receptor interactions contribute to brain EC–PVC signalling.
On the basis of our observation of MHC class II signalling in EC–EC communication, we next addressed MHC class II signalling in EC–PVC intercellular cross-talk, which predicted elevated MHC class II signalling (predominantly in microglia and macrophages, ECs and tumour cells/oligodendrocytes) in brain pathologies (Extended Data Fig. 12o,p,q and Supplementary Fig. 20h–j ). Spatial transcriptomics confirmed spatial co-localization of MHC class II ligands and receptors on ECs and PVCs (Supplementary Fig. 18 ) in the temporal lobe and in GBM, while IMC illustrated physical proximity between MHC class II-expressing ECs and microglia/macrophages across all entities (Extended Data Figs. 12r and 13 ). Notably, the APP–CD74 , COPA–CD74 and MIF–CD74 ligand–receptor pairs that were predicted to mediate MHC class II signalling in EC–EC interactions were also predicted ligand–receptor pairs in the developing, adult and diseased NVU (Supplementary Fig. 20h–j ), with ECs notably strongly expressing CD74 (Supplementary Fig. 19e,j,o,t,y ). These data suggest involvement of MHC class II in EC–immune cell interactions and indicate that the APP–CD74 , COPA–CD74 and MIF–CD74 ligand–receptor pairs contribute to NVU signalling.
Here we generated a large-scale single-cell molecular atlas of the developing fetal, adult/control and diseased human brain vasculature at a very high resolution, using scRNA-seq, composed of 606,308 freshly isolated endothelial, perivascular and other tissue-derived cells covering a substantial diversity of human brain tissue.
We have provided molecular definitions of human brain cell types and their differences by brain developmental stage and pathology, thereby unravelling organizational principles of ECs and PVCs composing the human brain vasculature. Our experimental methodology relies on transcriptional profiles of human cerebrovascular cells generated from fresh human neurosurgical resections and fresh fetal abortions, reducing the likelihood of transcriptional alterations associated with post-mortem tissue acquisition ( Supplementary Discussion ).
Our human vascular brain atlas provides a basis for understanding the organizing principles and single-cell heterogeneity of universal, specialized and activated endothelial and PVCs with broad implications for physiology and medicine, and serves as a powerful publicly available reference for the field.
Reporting summary
Further information on research design is available in the Nature Portfolio Reporting Summary linked to this article.
Data availability
All data are now accessible under Gene Expression Omnibus accession number GSE256493 . An interactive website is available at https://waelchli-lab-human-brain-vasculature-atlas.ethz.ch , https://brain-vasc.cells.ucsc.edu and https://cellxgene.cziscience.com/collections/c95ca269-68a7-47c5-82db-da227f31b598 on which the data can visualized and downloaded.
Code availability
Most of our analyses are standard workflows and we have now deposited the source code at GitHub ( https://github.com/Waelchli-lab/Single-cell-atlas-of-the-human-brain-vasculature-accross-development-adulthood-and-disease ) to improve reproducibility our results. The generated Seurat objects of FACS-sorted (CD31 + CD45 − ) ECs for the individual entities can be downloaded from https://doi.org/10.5281/zenodo.10058183 , and for the overall merges from https://doi.org/10.5281/zenodo.10057779 . The generated Seurat objects of unsorted ECs and PVCs for individual entities can be downloaded from https://doi.org/10.5281/zenodo.10058371 , and for the overall merges from https://doi.org/10.5281/zenodo.10058563 . The generated Monocle 3 CDS pseudotime objects of FACS-sorted (CD31 + CD45 − ) ECs can be downloaded from https://doi.org/10.5281/zenodo.10058766 . The generated diffusion map objects of FACS-sorted (CD31 + CD45 − ) ECs can be downloaded from https://doi.org/10.5281/zenodo.10060876 . The generated RNA velocity objects of FACS-sorted (CD31 + CD45 − ) ECs of individual pathological entities can be downloaded from https://doi.org/10.5281/zenodo.10065659 ; the fetal and adult/control brains from https://doi.org/10.5281/zenodo.10066390 ; the overall merge of brain tumours from https://doi.org/10.5281/zenodo.10066538 ; and the overall merge of pathological entities from https://doi.org/10.5281/zenodo.10066703 .
Wälchli, T. et al. Shaping the brain vasculature in development and disease in the single-cell era. Nat. Rev. Neurosci. 24 , 271–298 (2023).
Article PubMed PubMed Central Google Scholar
Cho, C., Smallwood, P. M. & Nathans, J. Reck and Gpr124 are essential receptor cofactors for Wnt7a/Wnt7b-Specific signaling in mammalian CNS angiogenesis and blood-brain barrier regulation. Neuron 95 , 1221–1225 (2017).
Article CAS PubMed Google Scholar
Wälchli, T. et al. Wiring the vascular network with neural cues: a CNS perspective. Neuron 87 , 271–296 (2015).
Article PubMed Google Scholar
Sweeney, M. D., Kisler, K., Montagne, A., Toga, A. W. & Zlokovic, B. V. The role of brain vasculature in neurodegenerative disorders. Nat. Neurosci. 21 , 1318–1331 (2018).
Article CAS PubMed PubMed Central Google Scholar
Zlokovic, B. V. The blood-brain barrier in health and chronic neurodegenerative disorders. Neuron 57 , 178–201 (2008).
Kuhnert, F. et al. Essential regulation of CNS angiogenesis by the orphan G protein-coupled receptor GPR124. Science 330 , 985–989 (2010).
Chang, J. et al. Gpr124 is essential for blood-brain barrier integrity in central nervous system disease. Nat. Med. 23 , 450–460 (2017).
Anderson, K. D. et al. Angiogenic sprouting into neural tissue requires Gpr124, an orphan G protein-coupled receptor. Proc. Natl Acad. Sci. USA 108 , 2807–2812 (2011).
Zhou, Y. & Nathans, J. Gpr124 controls CNS angiogenesis and blood-brain barrier integrity by promoting ligand-specific canonical wnt signaling. Dev. Cell 31 , 248–256 (2014).
Carmeliet, P. Angiogenesis in health and disease. Nat. Med. 9 , 653–660 (2003).
Carmeliet, P. & Jain, R. K. Molecular mechanisms and clinical applications of angiogenesis. Nature 473 , 298–307 (2011).
Potente, M., Gerhardt, H. & Carmeliet, P. Basic and therapeutic aspects of angiogenesis. Cell 146 , 873–887 (2011).
Carmeliet, P. Angiogenesis in life, disease and medicine. Nature 438 , 932–936 (2005).
Quaegebeur, A., Lange, C. & Carmeliet, P. The neurovascular link in health and disease: molecular mechanisms and therapeutic implications. Neuron 71 , 406–424 (2011).
Ghobrial, M. The human brain vasculature shows a distinct expression pattern of SARS-CoV-2 entry factors. Preprint at bioRxiv https://doi.org/10.1101/2020.10.10.334664 (2020).
Wälchli, T. et al. Quantitative assessment of angiogenesis, perfused blood vessels and endothelial tip cells in the postnatal mouse brain. Nat. Protoc. 10 , 53–74 (2015).
Wälchli, T. et al. Hierarchical imaging and computational analysis of three-dimensional vascular network architecture in the entire postnatal and adult mouse brain. Nat. Protoc. 16 , 4564–4610 (2021).
Nikolaev, S. I. et al. Somatic activating KRAS mutations in arteriovenous malformations of the brain. N. Engl. J. Med. 378 , 250–261 (2018).
Wälchli, T. et al. Nogo-A is a negative regulator of CNS angiogenesis. Proc. Natl Acad. Sci. USA 110 , E1943–E1952 (2013).
Wälchli, T. et al. Nogo-A regulates vascular network architecture in the postnatal brain. J. Cereb. Blood Flow Metab. 37 , 614–631 (2017).
Schwab, M. et al. Nucleolin promotes angiogenesis and endothelial metabolism along the oncofetal axis in the human brain vasculature. JCI Insight 8 , e143071 (2023).
Arvanitis, C. D., Ferraro, G. B. & Jain, R. K. The blood-brain barrier and blood-tumour barrier in brain tumours and metastases. Nat. Rev. Cancer 20 , 26–41 (2020).
Engelhardt, B. Development of the blood-brain barrier. Cell Tissue Res. 314 , 119–129 (2003).
Engelhardt, B. Blood-brain barrier differentiation. Science 334 , 1652–1653 (2011).
Mancuso, M. R., Kuhnert, F. & Kuo, C. J. Developmental angiogenesis of the central nervous system. Lymphat. Res. Biol. 6 , 173–180 (2008).
Daneman, R. et al. Wnt/β-catenin signaling is required for CNS, but not non-CNS, angiogenesis. Proc. Natl Acad. Sci. USA 106 , 641–646 (2009).
Liebner, S. et al. Wnt/β-catenin signaling controls development of the blood-brain barrier. J. Cell Biol. 183 , 409–417 (2008).
Vanlandewijck, M. et al. A molecular atlas of cell types and zonation in the brain vasculature. Nature 554 , 475–480 (2018).
Majno, G. & Palade, G. E. Studies on inflammation. 1. The effect of histamine and serotonin on vascular permeability: an electron microscopic study. J. Biophys. Biochem. Cytol. 11 , 571–605 (1961).
Simionescu, M., Simionescu, N. & Palade, G. E. Segmental differentiations of cell junctions in the vascular endothelium. The microvasculature. J. Cell Biol. 67 , 863–885 (1975).
Han, X. et al. Construction of a human cell landscape at single-cell level. Nature 581 , 303–309 (2020).
Litviňuková, M. et al. Cells of the adult human heart. Nature 588 , 466–472 (2020).
Travaglini, K. J. et al. A molecular cell atlas of the human lung from single-cell RNA sequencing. Nature 587 , 619–625 (2020).
Garcia, F. J. et al. Single-cell dissection of the human brain vasculature. Nature 603 , 893–899 (2022).
Yang, A. C. et al. A human brain vascular atlas reveals diverse mediators of Alzheimer’s risk. Nature 603 , 885–892 (2022).
Winkler, E. A. et al. A single-cell atlas of the normal and malformed human brain vasculature. Science 375 , eabi7377 (2022).
Crouch, E. E. et al. Ensembles of endothelial and mural cells promote angiogenesis in prenatal human brain. Cell 185 , 3753–3769 (2022).
Kalucka, J. et al. Single-cell transcriptome atlas of murine endothelial cells. Cell 180 , 764–779 (2020).
Marin-Padilla, M. The human brain intracerebral microvascular system: development and structure. Front. Neuroanat. 6 , 38 (2012).
Saunders, N. R., Liddelow, S. A. & Dziegielewska, K. M. Barrier mechanisms in the developing brain. Front. Pharmacol. 3 , 46 (2012).
Saunders, N. R., Dziegielewska, K. M., Mollgard, K. & Habgood, M. D. Physiology and molecular biology of barrier mechanisms in the fetal and neonatal brain. J. Physiol. 596 , 5723–5756 (2018).
Jabbour, P. M., Tjoumakaris, S. I. & Rosenwasser, R. H. Endovascular management of intracranial aneurysms. Neurosurg. Clin. N. Am. 20 , 383–398 (2009).
Xu, R., Pisapia, D. & Greenfield, J. P. Malignant transformation in glioma steered by an angiogenic switch: defining a role for bone marrow-derived cells. Cureus 8 , e471 (2016).
PubMed PubMed Central Google Scholar
Jain, R. K. et al. Angiogenesis in brain tumours. Nat. Rev. Neurosci. 8 , 610–622 (2007).
Das, S. & Marsden, P. A. Angiogenesis in glioblastoma. N. Engl. J. Med. 369 , 1561–1563 (2013).
Lorger, M., Krueger, J. S., O’Neal, M., Staflin, K. & Felding-Habermann, B. Activation of tumor cell integrin alphavbeta3 controls angiogenesis and metastatic growth in the brain. Proc. Natl Acad. Sci. USA 106 , 10666–10671 (2009).
Barresi, V. Angiogenesis in meningiomas. Brain Tumor Pathol. 28 , 99–106 (2011).
Picelli, S. et al. Full-length RNA-seq from single cells using Smart-seq2. Nat. Protoc. 9 , 171–181 (2014).
Xie, Y. et al. Key molecular alterations in endothelial cells in human glioblastoma uncovered through single-cell RNA sequencing. JCI Insight 6 , e150861 (2021).
Parab, S., Quick, R. E. & Matsuoka, R. L. Endothelial cell-type-specific molecular requirements for angiogenesis drive fenestrated vessel development in the brain. eLife 10 , e64295 (2021).
Wisniewska-Kruk, J. et al. Plasmalemma vesicle-associated protein has a key role in blood-retinal barrier loss. Am. J. Pathol. 186 , 1044–1054 (2016).
Bosma, E. K., van Noorden, C. J. F., Schlingemann, R. O. & Klaassen, I. The role of plasmalemma vesicle-associated protein in pathological breakdown of blood-brain and blood-retinal barriers: potential novel therapeutic target for cerebral edema and diabetic macular edema. Fluids Barriers CNS 15 , 24 (2018).
Carson-Walter, E. B. et al. Plasmalemmal vesicle associated protein-1 is a novel marker implicated in brain tumor angiogenesis. Clin. Cancer Res. 11 , 7643–7650 (2005).
Zhang, H. et al. Targeting endothelial cell-specific molecule 1 protein in cancer: a promising therapeutic approach. Front. Oncol. 11 , 687120 (2021).
McCracken, I. R. et al. Transcriptional dynamics of pluripotent stem cell-derived endothelial cell differentiation revealed by single-cell RNA sequencing. Eur. Heart J. 41 , 1024–1036 (2020).
Dieterich, L. C. et al. Transcriptional profiling of human glioblastoma vessels indicates a key role of VEGF-A and TGFβ2 in vascular abnormalization. J. Pathol. 228 , 378–390 (2012).
Huang, Z. et al. Effects of sex and aging on the immune cell landscape as assessed by single-cell transcriptomic analysis. Proc. Natl Acad. Sci. USA 118 , e2023216118 (2021).
Huang, X. et al. Single-cell transcriptional profiling reveals sex and age diversity of gene expression in mouse endothelial cells. Front. Genet. 12 , 590377 (2021).
Hajdarovic, K. H. et al. Single-cell analysis of the aging female mouse hypothalamus. Nat. Aging 2 , 662–678 (2022).
Hao, Y. et al. Integrated analysis of multimodal single-cell data. Cell 184 , 3573–3587 (2021).
Stuart, T. et al. Comprehensive integration of single-cell data. Cell 177 , 1888–1902 (2019).
Butler, A., Hoffman, P., Smibert, P., Papalexi, E. & Satija, R. Integrating single-cell transcriptomic data across different conditions, technologies, and species. Nat. Biotechnol. 36 , 411–420 (2018).
Satija, R., Farrell, J. A., Gennert, D., Schier, A. F. & Regev, A. Spatial reconstruction of single-cell gene expression data. Nat. Biotechnol. 33 , 495–502 (2015).
Yang, A. C. et al. A human brain vascular atlas reveals diverse mediators of Alzheimer's risk. Nature 603 , 885–892 (2022).
Yang, A. C. et al. Physiological blood–brain transport is impaired with age by a shift in transcytosis. Nature 583 , 425–430 (2020).
Chen, M. B. et al. Brain endothelial cells are exquisite sensors of age-related circulatory cues. Cell Rep. 30 , 4418–4432 (2020).
Halpern, K. B. et al. Single-cell spatial reconstruction reveals global division of labour in the mammalian liver. Nature 542 , 352–356 (2017).
He, L. et al. Analysis of the brain mural cell transcriptome. Sci. Rep. 6 , 35108 (2016).
Schaum, N. et al. Single-cell transcriptomics of 20 mouse organs creates a Tabula Muris . Nature 562 , 367–372 (2018).
Article PubMed Central Google Scholar
Platel, V., Faure, S., Corre, I. & Clere, N. Endothelial-to-mesenchymal transition (EndoMT): roles in tumorigenesis, metastatic extravasation and therapy resistance. J. Oncol. 2019 , 8361945–8361945 (2019).
Suvà, M. L. & Tirosh, I. The glioma stem cell model in the era of single-cell genomics. Cancer Cell 37 , 630–636 (2020).
Suvà, M. L. et al. Reconstructing and reprogramming the tumor-propagating potential of glioblastoma stem-like cells. Cell 157 , 580–594 (2014).
Wang, R. et al. Glioblastoma stem-like cells give rise to tumour endothelium. Nature 468 , 829–833 (2010).
Ricci-Vitiani, L. et al. Tumour vascularization via endothelial differentiation of glioblastoma stem-like cells. Nature 468 , 824–828 (2010).
Prabavathy, D., Swarnalatha, Y. & Ramadoss, N. Lung cancer stem cells-origin, characteristics and therapy. Stem Cell Invest. 5 , 6 (2018).
Article CAS Google Scholar
Lawton, M. T. et al. Brain arteriovenous malformations. Nat. Rev. Dis. Primers 1 , 15008 (2015).
Malinverno, M. et al. Endothelial cell clonal expansion in the development of cerebral cavernous malformations. Nat. Commun. 10 , 2761 (2019).
Orsenigo, F. et al. Mapping endothelial-cell diversity in cerebral cavernous malformations at single-cell resolution. eLife 9 , e61413 (2020).
Zhu, I. et al. Modular design of synthetic receptors for programmed gene regulation in cell therapies. Cell 185 , 1431–1443 (2022).
Uhlen, M. et al. Tissue-based map of the human proteome. Science 347 , 1260419 (2015).
Tang, M. et al. Evaluating single-cell cluster stability using the Jaccard similarity index. Bioinformatics 37 , 2212–2214 (2021).
Sjostedt, E. et al. An atlas of the protein-coding genes in the human, pig, and mouse brain. Science 367 , eaay5947 (2020).
Hodge, R. D. et al. Conserved cell types with divergent features in human versus mouse cortex. Nature 573 , 61–68 (2019).
Jin, S. et al. Inference and analysis of cell-cell communication using CellChat. Nat. Commun. 12 , 1088 (2021).
Efremova, M., Vento-Tormo, M., Teichmann, S. A. & Vento-Tormo, R. CellPhoneDB: inferring cell–cell communication from combined expression of multi-subunit ligand–receptor complexes. Nat. Protoc. 15 , 1484–1506 (2020).
Farnsworth, R. H., Lackmann, M., Achen, M. G. & Stacker, S. A. Vascular remodeling in cancer. Oncogene 33 , 3496–3505 (2014).
Trapnell, C. et al. The dynamics and regulators of cell fate decisions are revealed by pseudotemporal ordering of single cells. Nat. Biotechnol. 32 , 381–386 (2014).
Ji, Z. & Ji, H. TSCAN: tools for single-cell analysis. R package v.1.34.0 (2022).
La Manno, G. et al. RNA velocity of single cells. Nature 560 , 494–498 (2018).
Bergen, V., Lange, M., Peidli, S., Wolf, F. A. & Theis, F. J. Generalizing RNA velocity to transient cell states through dynamical modeling. Nat. Biotechnol. 38 , 1408–1414 (2020).
Angerer, P. et al. destiny: diffusion maps for large-scale single-cell data in R. Bioinformatics 32 , 1241–1243 (2016).
Munji, R. N. et al. Profiling the mouse brain endothelial transcriptome in health and disease models reveals a core blood–brain barrier dysfunction module. Nat. Neurosci. 22 , 1892–1902 (2019).
Saili, K. S. et al. Blood-brain barrier development: systems modeling and predictive toxicology. Birth Defects Res. 109 , 1680–1710 (2017).
Saunders, N. R. et al. The rights and wrongs of blood-brain barrier permeability studies: a walk through 100 years of history. Front. Neurosci. 8 , 404 (2014).
Virgintino, D. et al. Immunolocalization of tight junction proteins in the adult and developing human brain. Histochem. Cell Biol. 122 , 51–59 (2004).
Daneman, R. & Prat, A. The blood-brain barrier. Cold Spring Harb. Perspect. Biol. 7 , a020412 (2015).
Ben-Zvi, A. et al. Mfsd2a is critical for the formation and function of the blood–brain barrier. Nature 509 , 507–511 (2014).
Ma, S. C. et al. Claudin-5 regulates blood-brain barrier permeability by modifying brain microvascular endothelial cell proliferation, migration, and adhesion to prevent lung cancer metastasis. CNS Neurosci. Ther. 23 , 947–960 (2017).
Goveia, J. et al. An integrated gene expression landscape profiling approach to identify lung tumor endothelial cell heterogeneity and angiogenic candidates. Cancer Cell 37 , 21–36 (2020).
Andreone, B. J. et al. Blood-brain barrier permeability is regulated by lipid transport-dependent suppression of caveolae-mediated transcytosis. Neuron 94 , 581–594 (2017).
O’Brown, N. M., Megason, S. G. & Gu, C. Suppression of transcytosis regulates zebrafish blood-brain barrier function. eLife 8 , e47326 (2019).
Zhao, Z., Nelson, A. R., Betsholtz, C. & Zlokovic, B. V. Establishment and dysfunction of the blood-brain barrier. Cell 163 , 1064–1078 (2015).
Kim, N. et al. Single-cell RNA sequencing demonstrates the molecular and cellular reprogramming of metastatic lung adenocarcinoma. Nat. Commun. 11 , 2285 (2020).
Jiang, Y. Q. et al. Investigating mechanisms of response or resistance to immune checkpoint inhibitors by analyzing cell-cell communications in tumors before and after programmed cell death-1 (PD-1) targeted therapy: an integrative analysis using single-cell RNA and bulk-RNA sequencing data. Oncoimmunology 10 , 1908010 (2021).
Download references
Acknowledgements
We thank N. Krayenbühl, M. Germans, O. Bozinov, P. Bijlenga, P.-Y. Dietrich and V. Dutoit for help with the human adult tissue asservation; the donors and the staff at the RCWIH Biobank, the Lunenfeld-Tanenbaum Research Institute, the Mount Sinai Hospital/UHN Department of Obstetrics and Gynaecology, and Maximilian Niit for help with the human fetal specimen asservation, preparation and IHC staining ( https://biobank.lunenfeld.ca ); E. Speck and the members of the Flow Cytometry Facility, Krembil Discovery Tower, University Health Network for help with the FACS sorting; G. Basi, J. Cirlan, C. Dumrese and M. Kisielow for help with the scRNA-seq experiments; A. M. Sababi and M. M. Saad for help with the computational analysis; J. L. Gorman for help with the IMC experiments; the members of the University Health Network, Laboratory Medicine-Pathology Research Program and Melanie Peralta for help with adult specimen preparation and IHC staining; N. C. Ji for help with the illustrations; A. Thomson for help with English proofreading; A. Keller, F. Kern, T. Nowakowski, E. Winkler, M. Kellis, N. Sun, A. Regev, G. Eraslan, F. J. Theis, J. Shin, M. Prinz, R. Sankowski, R. Adams, S. Teichmann, L. Yang, A. Maria Cujba and N. Huang for their scientific inputs and advice regarding computational integration methods, the covariates age and sex, and overall discussion of our manuscript and figures. We acknowledge the following financial support for the research, and/or publication of this Article: T.W. was supported by the OPO Foundation, the Swiss Cancer Research foundation (KFS-3880-02-2016-R, KFS-4758-02-2019-R), the Stiftung zur Krebsbekämpfung, the Kurt und Senta Herrmann Foundation, Forschungskredit of the University of Zurich, the Zurich Cancer League, the Theodor und Ida Herzog Egli Foundation, the Novartis Foundation for Medical-Biological Research and the HOPE Foundation; I.R. by the Canadian Institutes of Health Research (funding reference number 155922) and the Irwin & Mariel Michael and Family through the University Health Network Foundation; I.R. and T.W. by the Gill Family Charitable Trust through the University Health Network Foundation; P.P.M. by the Canadian Institutes of Health Research; G.D.B. by NRNB (US National Institute of Health, National Center for Research Resources grant number P41GM103504). IMC work was supported by an NSERC Discovery grant (RGPIN-2021-03404) and an Early Career Investigator Award from Ontario Institute for Cancer Research (IA-1-020) and the Canada Research Chairs program to H.W.J. This publication is part of the Human Cell Atlas, www.humancellatlas.org/publications .
Author information
These authors contributed equally: Thomas Wälchli, Moheb Ghobrial
These authors jointly supervised this work: Thomas Wälchli, Gary D. Bader, Ivan Radovanovic
Authors and Affiliations
Group Brain Vasculature and Perivascular Niche, Division of Experimental and Translational Neuroscience, Krembil Brain Institute, Krembil Research Institute, Toronto Western Hospital, University Health Network, University of Toronto, Toronto, Ontario, Canada
Thomas Wälchli, Moheb Ghobrial, Marc Schwab, Hang Zhong, Samuel Suntharalingham, Jeroen Bisschop & Fiona Farnhammer
Division of Neurosurgery, Department of Surgery, University of Toronto, Toronto, Ontario, Canada
Thomas Wälchli, Moheb Ghobrial, Marc Schwab, Shigeki Takada, Hang Zhong, Samuel Suntharalingham, Sandra Vetiska, Jeroen Bisschop, Fiona Farnhammer, Ann Mansur, Mark Bernstein, Paul Kongkham, Taufik Valiante, Peter B. Dirks, Gelareh Zadeh & Ivan Radovanovic
Group of CNS Angiogenesis and Neurovascular Link, Neuroscience Center Zurich, University of Zurich and University Hospital Zurich, Zurich, Switzerland
Thomas Wälchli, Moheb Ghobrial, Marc Schwab, Hang Zhong, Jeroen Bisschop, Fiona Farnhammer & Karl Frei
Division of Neurosurgery, University Hospital Zurich, Zurich, Switzerland
Thomas Wälchli, Moheb Ghobrial, Marc Schwab, Hang Zhong, Jeroen Bisschop, Fiona Farnhammer, Luca Regli & Karl Frei
Laboratory of Exercise and Health, Institute of Exercise and Health, Department of Health Sciences and Technology; Swiss Federal Institute of Technology (ETH Zurich), Zurich, Switzerland
Moheb Ghobrial, Hang Zhong & Katrien De Bock
Institute for Regenerative Medicine, University of Zurich, Zurich, Switzerland
Marc Schwab
Department of Neurosurgery, Kyoto University Graduate School of Medicine, Kyoto, Japan
Shigeki Takada
Division of Experimental and Translational Neuroscience, Krembil Brain Institute, Krembil Research Institute, Toronto Western Hospital, University Health Network, University of Toronto, Toronto, Ontario, Canada
Shigeki Takada, Sandra Vetiska, Ann Mansur & Ivan Radovanovic
Department of Physiology, Faculty of Medicine, University of Toronto, Toronto, Ontario, Canada
Samuel Suntharalingham, Jeroen Bisschop, Fiona Farnhammer & Philippe P. Monnier
Functional Genomics Center Zurich, ETH Zurich/University of Zurich, Zurich, Switzerland
Daymé Rodrigues Gonzalez, Hubert Rehrauer & Ralph Schlapbach
Department of Laboratory Medicine and Pathobiology, University of Toronto, Toronto, Ontario, Canada
Ruilin Wu, Kai Yu, Ann Mansur, Jason E. Fish & Ivan Radovanovic
Toronto General Hospital Research Institute, University Health Network, Toronto, Ontario, Canada
Ruilin Wu, Kai Yu & Jason E. Fish
The Lunenfeld-Tanenbaum Research Institute, Mount Sinai Health System, Toronto, Ontario, Canada
Anuroopa Dinesh, Edward L. Y. Chen, Hartland W. Jackson & Gary D. Bader
Department of Molecular Genetics, University of Toronto, Toronto, Ontario, Canada
Edward L. Y. Chen, Peter B. Dirks, Hartland W. Jackson & Gary D. Bader
Department of Biomedicine, Aarhus University, Aarhus, Denmark
Joanna Kalucka
Department of Molecular Cell Biology, Weizmann Institute of Science, Rehovot, Israel
Itay Tirosh
Department of Neurosurgery, University of Geneva Medical Center & Faculty of Medicine, University of Geneva, Geneva, Switzerland
Karl Schaller
The Donnelly Centre, University of Toronto, Toronto, Ontario, Canada
Troy Ketela & Gary D. Bader
Division of Neurosurgery, Sprott Department of Surgery, University of Toronto, Toronto, Ontario, Canada
Mark Bernstein, Paul Kongkham, Taufik Valiante, Gelareh Zadeh & Ivan Radovanovic
MacFeeters-Hamilton Centre for Neuro-Oncology Research, University Health Network, Toronto, Ontario, Canada
Paul Kongkham
Laboratory of Angiogenesis and Vascular Metabolism, Center for Cancer Biology, VIB & Department of Oncology, KU Leuven, Leuven, Belgium
Peter Carmeliet
State Key Laboratory of Ophthalmology, Zhongshan Ophthalmic Center, Sun Yat-sen University, Guangzhou, P. R. China
Laboratory of Angiogenesis and Vascular Heterogeneity, Department of Biomedicine, Aarhus University, Aarhus, Denmark
Krembil Brain Institute, Division of Clinical and Computational Neuroscience, Krembil Research Institute, Toronto Western Hospital, University Health Network, University of Toronto, Toronto, Ontario, Canada
Taufik Valiante
Institute of Biomaterials and Biomedical Engineering and Electrical and Computer Engineering, University of Toronto, Toronto, Ontario, Canada
Institute of Medical Science Faculty of Medicine, University of Toronto, Toronto, Ontario, Canada
Division of Neurosurgery, Arthur and Sonia Labatt Brain Tumor Research Center, Departments of Surgery and Molecular Genetics, Hospital for Sick Children, Toronto, Ontario, Canada
Peter B. Dirks
Department of Pathology and Center for Cancer Research, Massachusetts General Hospital and Harvard Medical School, Boston, MA, USA
Mario L. Suva
Broad Institute of Harvard and MIT, Cambridge, MA, USA
Princess Margaret Cancer Centre, University Health Network, Toronto, Ontario, Canada
Gelareh Zadeh
Department of Neurosurgery, Memorial Sloan Kettering Cancer Center, New York, NY, USA
Viviane Tabar
Ontario Institute of Cancer Research, Toronto, Ontario, Canada
Hartland W. Jackson
Peter Munk Cardiac Centre, University Health Network, Toronto, Ontario, Canada
Jason E. Fish
Krembil Research Institute, Vision Division, Krembil Discovery Tower, Toronto, Ontario, Canada
Philippe P. Monnier
Department of Ophthalmology and Vision Sciences, Faculty of Medicine, University of Toronto, Toronto, Ontario, Canada
Department of Computer Science, University of Toronto, Toronto, Ontario, Canada
Gary D. Bader
You can also search for this author in PubMed Google Scholar
Contributions
T.W. had the idea for the study. T.W. and I.R. conceived the study. T.W. designed the experiments, wrote the manuscript, analysed the data, designed the figures and made the figures with M.G. with the help of M.S.; I.R., T.W., L.R., K.S., M.B., P.K., G.Z. and T.V. acquired the tissue. M.G., M.S., S.S. and S.V. performed the cell isolation experiments. T.W. and K.F. developed the initial isolation experiments. M.G. (majority of the analysis), M.S., H.Z., D.R.G. and H.R. performed the single-cell data processing and analysed the data with T.W.; T.W. and G.D.B. supervised the data analysis and interpreted data. R.S. and T.K. helped with single-cell data processing. M.G., M.S. and G.D.B. developed the computational methods with the help of T.W.; S.T., S.S., R.W. and K.Y. performed the IF stainings and RNA scope experiments, and S.T., R.W., K.Y., M.G., M.S., J.E.F. and T.W. analysed and interpreted the data. M.S., R.W., J.E.F. and T.W. selected the final IF images. A.D. E.L.Y.C. and H.W.J. performed the IMC staining, and A.D., M.S., H.W.J. and T.W. analysed and interpreted the data. M.S., H.W.J. and T.W. selected the final IMC images. T.W., I.R., G.D.B. and P.P.M. acquired funding. T.W., P.P.M., I.R. and G.D.B. edited the final version of the manuscript. M.G., M.S. and J.B. helped edit the manuscript. P.P.M., K.D.B., J.E.F., M.L.S., P.B.D., P.C., V.T., G.Z., T.V., I.R. and G.D.B. provided critical inputs to the manuscript. T.W. supervised all of the research. All of the authors read and approved the final manuscript.
Corresponding author
Correspondence to Thomas Wälchli .
Ethics declarations
Competing interests.
The authors declare no competing interests.
Peer review
Peer review information.
Nature thanks Konstantin Khodosevich and the other, anonymous, reviewer(s) for their contribution to the peer review of this work. Peer reviewer reports are available.
Additional information
Publisher’s note Springer Nature remains neutral with regard to jurisdictional claims in published maps and institutional affiliations.
Extended data figures and tables
Extended data fig. 1 construction of a molecular single-cell atlas of the human brain vasculature across development, adulthood and disease and of the fetal peripheral vasculature - studied entities and inter-tissue heterogeneity..
Figure shows studied entities and inter-tissue heterogeneity of sorted vascular endothelial cells. a , b , Scheme of the different tissue types present in the study with respective sample/patient numbers of fetal ( a ) and adult ( b ) origins. c , Piechart showing relative abundance and percentage of ECs from each tissue collected. d , Composite UMAP plots of sorted and in silico-quality checked (ECs, coloured by tissue of origin. e , Violin plots of the expression of the top marker genes of each tissue type, percentage of cells expressing the marker gene is indicated on the right. f , Endothelial cells transcriptome correlation heatmap and hierarchical clustering of all tissues analysed. g , Dotplot heatmap of the fetal brain vs fetal periphery endothelial signature. h , Expression heatmap of top ranking marker genes in the indicated tissues. Colour scale: red, high expression; white, intermediate expression; blue, low expression.
Extended Data Fig. 2 Validation of angiogenic and EndoMT markers in vascular ECs of the fetal, adult and pathological brain vasculature using RNAscope, IF and IMC.
a - g , Volcano plots showing the differential expression analysis comparing endothelial cells from adult/control brains (left) and the indicated entity (right) (Wald test, Benjamini Hochberg correction; P -value < 0.05 and log2FC ≥ 0.25 coloured significant in red, PLVAP dot is coloured blue). Number of individuals analysed is as follows: Fetal brain=5, Adult/control brain (TL) = 9, AV malformations=5, AVM = 5, LGG = 6, GBM = 8, MET = 5, MEN = 5. h , Expression heatmap of the top 25 differentially expressed genes in adult/control ECs (TL) vs. pathological brain ECs (PATH). i-d i ‘ , Immunofluorescence (IF) and RNAscope imaging of tissue sections from the indicated entities, stained for PLVAP (red), (RNAscope: i-t ; IF: u - d i ‘ ) and CD31 (green). Nuclei are stained with DAPI (blue). Arrowheads indicate ECs expressing PLVAP and dotted lines (RNAscope) delineate vascular structures in the different tissues. Scale bars: 50 μm in overviews (RNAscope); 50 μm in (IF) and 12.5 μm in zooms (RNAscope). a i -d i ‘ , IF of fetal brain tissue sections from the indicated gestational ages, stained for PLVAP (red) and CD31 (green). Nuclei are stained with DAPI (blue). Arrowheads indicate ECs expressing PLVAP. Arrows indicate absence of expression in ECs. Scale bars: 20 μm. e i -b ii , RNAscope imaging of tissue sections from the indicated entities, stained for ESM1 (red; e i -p i ), ACTA2 (red; q i -b ii ) and CD31 (green). Nuclei are stained with DAPI (blue). Boxed area is magnified on the right; arrowheads indicate ECs expressing ESM1 or ACTA2 and dotted lines delineate vascular structures in the different tissues. Scale bars: 50 μm in overviews and 12.5 μm in zooms. c ii -j ii ‘ , IF of tissue sections from the indicated entities, stained for ESM1 (red; c ii -f ii ‘ ), ACTA2 (red; g ii - j ii ‘ ) and CD31 (green). Nuclei are stained with DAPI (blue). Boxed area is magnified on the right; arrowheads indicate ECs expressing ESM1 or ACTA2. Arrows indicate absence of expression in ECs. Scale bars: 80 μm in overviews and 20 μm in zooms. k ii - q‘ 4 , Mass cytometry (IMC) imaging of the indicated fetal, adult/control and pathological brain tissue samples visualizing metal-conjugated ACTA2, PDGFRB, CD31 and CLDN5 primary antibodies out of all 39 stained panel (Supplementary Table 24 ). Overlay of pseudocolor images as well as individual channels of the different markers (white, overlap; cyan, ACTA2; green, PDGFRB; red, CD31; yellow, CLDN5; blue, DNA intercalator), Scale bars: 250 μm in overviews, 50 μm in zooms. Arrowheads identify blood vessels ECs expressing the indicated markers in the different tissues. Arrows identify blood vessel ECs not expressing the indicated markers in the different tissues. r ii -t ii , Violin plots showing the expression of PLVAP ( r ii ), ESM1 ( s ii ) and ACTA2 ( t ii ) in the indicated fetal brain (CNS), adult/control and pathological brain tissues.
Extended Data Fig. 3 Validation of stem-to-endothelial transdifferentiation in vascular ECs of glioblastoma and of lung cancer brain metastasis using IF.
a , n , UMAP plot of glioblastoma (49,999 ECs from 8 individuals) (GBM) and lung cancer brain metastasis (23,962 ECs from 5 individuals) (MET) endothelial cells, coloured by AV specification. b - i , o - v , Violin plots showing the expression of the indicated endothelial- and stem cell specific markers in the different EC subtypes (AV clusters). j - m , a i -z i ’ , Immunofluorescence staining of SOX2 ( j , k , a i -h i ‘ ), PTPRZ1 ( l , m , i i -n i ‘ ), EPCAM ( w , x , o i -t i ‘ ), SFTPB ( y , z , u i -z i ‘ ) (red) and CD31 (green) in the indicated tissue samples. Arrowheads identify blood vessels ECs expressing the indicated markers in the different tissues. Arrows identify blood vessel ECs not expressing the indicated markers in the different tissues. Boxed area is magnified on the right. Scale bars: 80 µm in overviews except for c i -c i ‘ , k i -k i ‘ , q i -q i ‘ , w i -w i ‘ (200 µm), 20 µm in zooms except for d i -d i ‘ , l i -l i ‘ , r i -r i ‘ , x i -x i ‘ (50 µm).
Extended Data Fig. 4 Alteration of AV-specification in pathological brain vascular ECs.
UMAP plots of human brain ECs isolated from the brain arteriovenous malformations (20,305 ECs from 5 individuals) ( a , b ), all brain tumours (125,579 ECs from 24 individuals) ( e , f ), lower-grade glioma (17,373 ECs from 6 individuals) ( i , j ) glioblastoma (49,999 ECs from 8 individuals) ( m , n ), brain metastasis (23,962 ECs from 5 individuals) ( q , r ), and meningioma (34,245 ECs from 5 individuals) ( u , v ) coloured by AV specification ( a , e , i , m , q , u ) and by pseudotime ( b , f , j , n , r , v ), the red line drawn manually indicates the major trajectory flow. c , g , k , o , s , w , Pseudotime order of ECs colour-coded according to AV specification from all theindicated entities. d , h , l , p , t , x , Heatmap of adult/control brain ECs AV specification signature gene expression in human brain ECs isolated from the indicated entities. y-ziv , Sankey plot showing the predicted annotation of the ECs of the indicated entities as mapped to adult/control brain (TL) ECs. Unassigned cells are indicated in grey. zv , Sankey plot of pathological brain ECs, showing the predicted annotation (right nodes) – as mapped to adult/control brain (TL) ECs – of the AV clusters assigned based on top cluster marker analysis (left nodes). Unassigned cells are indicated in grey. zvii , Sankey plot of fetal brain ECs, showing the predicted annotation (right nodes) – as mapped to adult/control brain (TL) ECs – of the AV clusters assigned based on top cluster marker analysis (left nodes). Unassigned cells are indicated in grey. zvi , zviii , Barplots showing the composition of unassigned cells – based on mapping to adult/control brain (TL) ECs – in pathological brain ECs ( zv ) and fetal brain ECs ( zvii ).
Extended Data Fig. 5 Trajectory analysis of fetal, adult and pathological human brain vascular ECs via RNA velocity along the AV-zonation/specification axis.
a , f , k , p , u , z , a‘ , f‘ , k‘ , UMAP plots of human brain ECs isolated from the indicated entities (fetal brains (21,512 ECs from 5 individuals) ( a ), adult/control brains (76,125 ECs from 9 individuals) ( f ), pathological brains (145,884 ECs from 29 individuals) ( k ), brain arteriovenous malformations (20,305 ECs from 5 individuals) ( p ), all brain tumours (125,579 ECs from 24 individuals) ( u ), lower-grade glioma (17,373 ECs from 6 individuals) ( z ) glioblastoma (49,999 ECs from 8 individuals) ( a‘ ), brain metastasis (23,962 ECs from 5 individuals) ( f‘ ), and meningioma (34,245 ECs from 5 individuals) ( k‘ )) coloured by AV specification, onto the UMAPs are superimposed RNA velocity streamlines extended by velocity-inferred directionality. b , g , l , q , v , zi , b‘ , g‘ , l‘ , UMAP plots of the indicated entities, coloured by RNA velocity pseudotime. c , h , m , r , w , zii , c‘ , h‘ , m‘ , UMAP plots of the indicated entities, coloured by RNA velocity length. d,i,n,s,x,ziii,d‘,i‘,n‘ , UMAP plots of the indicated entities, coloured by RNA velocity confidence. e , j , o , t , y , ziv , e‘ , j‘ , o‘ , UMAP plots of the indicated entities, coloured by AV specification, onto the UMAPs are superimposed RNA velocity PAGA vectors extended by velocity-inferred directionality.
Extended Data Fig. 6 Establishment of CNS, peripheral and BBB signatures in vascular ECs from the developing fetal to the adult brain vasculature.
a , UMAP plots of the ECs from fetal brains in ascending fetal age ranging from gestational week 9 (444 ECs), gestational week 14.4 and 16.4 (3762 ECs), gestational week 15.5 (18,314 ECs) to gestational week 18 (3601 ECs); plots are colour-coded for fetal CNS signature (green) and fetal peripheral signature (yellow). b , Dotplot heatmap of fetal CNS, fetal peripheral, and BBB signatures’ expression in fetal brains of indicated fetal age and adult/control brains (temporal lobes). c , Dotplot heatmap of fetal CNS signature genes’ expression in fetal brains of indicated fetal age and adult/control brains (temporal lobes). d , Dotplot heatmaps of fetal CNS and BBB signatures expression at the level of AV specification for the indicated entities. Colour scale: red, high expression; blue, low expression, whereas the dot size represents the percentage expression within the indicated entity. e - j , Violin plots showing the expression of selected CNS specific and BBB marker genes in fetal brains of indicated fetal age and adult/control brains (TL). Markers shown were further validated by IF and/or IMC. k-t’ 4 Mass cytometry (IMC) imaging of fetal brains of fetal age GW9 ( k-l’ 4 ), GW14 ( m-n’ 4 ), GW18 ( o-p’ 4 ), GW21 ( q-r’ 4 ) and adult/control brains (temporal lobe) ( s-t’ 4 ) tissue samples using metal-conjugated antibodies. Representative pseudocolor images of combined and individual channels of the different markers (white, overlap; yellow, CLDN5; cyan, GLUT1; grey, BSG; red, CD31; blue, DNA intercalator), Scale bars: 250 μm in overviews, 50 μm in zooms. Shown are 5 antibodies stained for (Supplementary Table 24 ). Arrowheads identify blood vessels ECs expressing the indicated markers in the different tissues. Arrows identify blood vessel ECs not expressing the indicated markers in the different tissues.
Extended Data Fig. 7 Validation of CNS signature markers in vascular ECs of the developing fetal, adult and pathological brain vasculature using IF.
a-z ii ‘ , Immunofluorescence (IF) imaging of tissue sections from the indicated entities, stained for SPOCK3 (red; a-f‘ ), BSG (red; g-l‘ , c ii -f ii ‘ ), CD320 (red; m-r‘ , g ii -j ii ‘ ), GPCPD1 (red; s-x‘ ), PPP1R141 (red; y-d i ‘ ), SLC38A5 (red; e i -j i ‘ , k ii -n ii ‘ ), GLUT1 (red; k i ‘-p i ‘ , o ii -r ii ‘ ), OCLN (red; q i ‘-v i ‘ , s ii -v ii ‘ ), ZO-1 (red; w i -b ii ‘ , w ii -z ii ‘ ) and CD31 (green). Nuclei are stained with DAPI (blue). Arrowheads identify blood vessel ECs expressing the indicated markers in the different tissues. Arrows identify blood vessel ECs not expressing the indicated markers in the different tissues. Scale bars: 200 μm in overviews, 50 μm in zooms. a iii -f iii , Violin plots showing the expression of CNS-specific genes in the indicated adult control and pathological brain tissues.
Extended Data Fig. 8 Validation of CNS signature markers in vascular ECs of the developing fetal, adult and pathological brain vasculature using IMC.
a - f , Violin plots showing the expression of CNS-specific and BBB marker genes in the indicated adult control and pathological brain tissues. Markers were further validated by IF and/or IMC. g-r‘ 4 Mass cytometry (IMC) imaging of the indicated adult control and pathological brain tissue samples using metal-conjugated antibodies. Representative pseudocolour images of combined and individual channels of the different markers (white, overlap; yellow, CLDN5; cyan, GLUT1; grey, BSG; red, CD31; blue, DNA intercalator), Scale bars: 250 μm in overviews, 50 μm in zooms. Shown are 5 antibodies stained for (Supplementary Table 24 ). Arrowheads identify blood vessels ECs expressing the indicated markers in the different tissues. Arrows identify blood vessel ECs not expressing the indicated markers in the different tissues.
Extended Data Fig. 9 Establishment of MHC class II and CNS signatures in vascular ECs from the developing fetal to the adult brain vasculature.
a , UMAP plots of the ECs from fetal brains in ascending fetal age ranging from gestational week 9 (444 ECs), gestational week 14.4 and 16.4 (3762 ECs), gestational week 15.5 (18,314 ECs) to gestational week 18 (3601 ECs); plots are colour-coded for fetal CNS signature (green) and MHC class II signature (blue). b , Dotplot heatmap of MHC class II, fetal CNS and peripheral signatures expression in fetal brains of indicated fetal age and adult/control brains (temporal lobes). c , Dotplot heatmap of MHC class II signature genes’ expression in fetal brains of indicated fetal age and adult/control brains (temporal lobes). d , Dotplot heatmaps of MHC class II signature expression at the level of AV specification for the indicated entities. Colour scale: red, high expression; blue, low expression, whereas the dot size represents the percentage expression within the indicated entity. e - i , Violin plots showing the expression of MHC class II marker genes in fetal brains of indicated fetal age and adult/control brains. Markers shown were further validated by IF and/or IMC. j-s’ 4 Mass cytometry (IMC) imaging of fetal brains of fetal age GW9 ( j-k’ 4 ), GW14 ( l-m’ 4 ), GW18 ( n-o’ 4 ), GW21 ( p-q’ 4 ) and adult/control brain ( r-s’ 4 ) tissue samples using metal-conjugated primary and secondary antibodies. Representative pseudocolour images of combined and individual channels of the different markers (white, overlap; orange, CD74; cyan, pan-HLA-DR; green, oligo-HLA-D; red, CD31; blue, DNA intercalator), Scale bars: 250 μm in overviews, 50 μm in zooms. Shown are 5 antibodies stained for (Supplementary Table 24 ). Arrowheads identify blood vessels ECs expressing the indicated markers in the different tissues. Arrows identify blood vessel ECs not expressing the indicated markers in the different tissues.
Extended Data Fig. 10 Validation of MHC class II signature markers in vascular ECs of the developing fetal brain and periphery vasculature, and of the adult and pathological brain vasculature using RNAscope and IF.
Immunofluorescence (IF) and RNAscope imaging of tissue sections from the indicated entities, stained for HLA-DPA1 (red; a-j‘ , IF; o i -t ii , RNAscope), HLA-DRA (red; k-t‘ , IF), CD74 (red; u-d i ‘ , IF; u i -z ii , RNAscope), HLA-DRB5 (red; e i -n i ‘ , IF) and CD31 (green). Nuclei are stained with DAPI (blue). Arrowheads identify blood vessel ECs expressing the indicated markers, arrows identify blood vessel ECs not expressing the indicated markers in the different tissues and dotted lines (RNAscope) indicate vascular structures in the different tissues. Scale bars: 50 μm in (IF), 50 μm in overviews (RNAscope) and 12.5 μm in zooms (RNAscope).
Extended Data Fig. 11 Validation of MHC class II signature markers in vascular ECs of the adult and pathological brain vasculature using IMC.
a - e , Violin plots showing the expression of MHC class II marker genes in the indicated fetal brain (CNS), adult/control and pathological brain tissues. Markers shown were further validated by IF and/or IMC. f-q‘ 4 Mass cytometry (IMC) imaging of the indicated adult control and pathological brain tissue samples using metal-conjugated primary and secondary antibodies. Representative pseudocolor images of combined and individual channels of the different markers (white, overlap; orange, CD74; cyan, pan-HLA-DR; green, oligo-HLA-D; yellow, red, CD31), Scale bars: 250 μm in overviews, 50 μm in zooms. Shown are 5 antibodies stained for (Supplementary Table 24 ). Arrowheads identify blood vessels ECs in close contact with immune cell expressing the indicated markers in the different tissues. Arrows identify blood vessel ECs not in close contact with immune cell expressing the indicated markers.
Extended Data Fig. 12 A key role for vascular ECs in the human brain neurovascular unit across development, adulthood and disease, highlighting MHC class II mediated interactions.
a , d , g , UMAP plots of endothelial and perivascular cells derived from fetal brains (40,557 cells from 7 individuals) ( a ), adult/control brains (80,515 cells from 6 individuals) ( d ) and pathological brains (Arteriovenous malformations: 12,013 cells from 3 individuals; Lower-grade glioma: 20,712 cells from 4 individuals; Glioblastoma: 22,297 cells from 5 individuals; Metastasis: 9,204 cells from 3 individuals; Meningioma: 26,916 cells from 3 individuals) ( g ). b , e , h , Ligand and receptor analysis of fetal brain ( b ), adult control brain ( e ), and brain pathology ( h ) cells using CellphoneDB. Line thickness indicates the number of interactions between cell types. Tables summarize the number of interactions for each cell type. c , f , i , Heatmaps showing the number of ligand-receptor interactions between the different cells of fetal brains ( c ), adult/control brains ( f ), and pathological brains ( i ) computed using CellphoneDB. j , k , Circle plot showing differential analysis of strength of ligand receptor interactions in fetal over adult/control brains ( j ) and pathology over adult/control brains ( k ), red indicating upregulation, while blue indicating downregulation. l - n , Heatmap showing overall signalling patterns of different cell types in adult control ( o ) and pathological ( p ) brains. o , p , q , Circle plots showing the strength of MHC class II signalling interactions between the different cell types of fetal brain ( o ), adult/control brain ( p ), and pathological brain ( q ). r-r’ 6 Mass cytometry (IMC) imaging of metastasis tissue sample visualizing metal-conjugated CD74, pan-HLA-DR, oligo-HLA-D, CD68, CD11b, CD4, CD8 and CD31 primary antibodies and metal-conjugated secondary stains, a subset of the antibody set (Supplementary Table 24 ) stains present in all images. Overlay of pseudocolor images as well as individual channels for CD74, pan-HLA-DR, oligo-HLA-D, CD68, CD11b and CD4, (white, overlap; orange, CD74; cyan, pan-HLA-DR; green, oligo-HLA-D; yellow, CD68; purple, CD11b; blue, CD4; grey, CD8; red, CD31), Scale bars = 50 μm. Shown are 8 antibodies stained for. Arrowheads identify blood vessel ECs in close contact with immune cell expressing the indicated markers in the different tissues. Arrows identify blood vessel ECs not in close contact with immune cell expressing the indicated markers.
Extended Data Fig. 13 Validation of MHC class II mediated endothelial-immune cell interactions in the adult and pathological brain vasculature using IMC.
a , d , g , j , m , p , Circle plots showing the strength of MHC class II signalling interactions between the different cell types of adult/control brain ( a ) arteriovenous malformation ( d ), lower-grade glioma ( g ), glioblastoma ( j ), metastasis ( m ) and meningioma ( p ) cells. b-c’ 8 , e-f’ 8 , h-i’ 8 , k-l’ 8 , n-o’ 8 , q-r’ 8 , Mass cytometry (IMC) imaging of the indicated adult control and pathological brain tissue samples using metal-conjugated CD74, pan-HLA-DR, oligo-HLA-D, CD68, CD11b, CD4, CD8 and CD31 primary antibodies and metal-conjugated secondary stains. Overlay of pseudocolor images as well as individual channels of the different markers (white, overlap; orange, CD74; cyan, pan-HLA-DR; green, oligo-HLA-D; yellow, CD68; purple, CD11b; blue, CD4; grey, CD8; red, CD31), Scale bars: 250 μm in overviews, 50 μm in zooms. Shown are 5 antibodies stained for (Supplementary Table 24 ). Arrowheads identify blood vessels ECs in close contact with immune cell expressing the indicated markers in the different tissues. Arrows identify blood vessel ECs not in close contact with immune cells expressing the indicated markers.
Supplementary information
Supplementary information.
Supplementary Figs. 1–20.
Reporting Summary
Peer review file, supplementary methods.
A detailed description of the methods used in our article with a separate references section.
Supplementary Discussion
Supplementary Discussion.
Supplementary Tables 1–27
Supplementary Tables 1–27 and the legends for the Supplementary Tables.
Rights and permissions
Open Access This article is licensed under a Creative Commons Attribution 4.0 International License, which permits use, sharing, adaptation, distribution and reproduction in any medium or format, as long as you give appropriate credit to the original author(s) and the source, provide a link to the Creative Commons licence, and indicate if changes were made. The images or other third party material in this article are included in the article’s Creative Commons licence, unless indicated otherwise in a credit line to the material. If material is not included in the article’s Creative Commons licence and your intended use is not permitted by statutory regulation or exceeds the permitted use, you will need to obtain permission directly from the copyright holder. To view a copy of this licence, visit http://creativecommons.org/licenses/by/4.0/ .
Reprints and permissions
About this article
Cite this article.
Wälchli, T., Ghobrial, M., Schwab, M. et al. Single-cell atlas of the human brain vasculature across development, adulthood and disease. Nature (2024). https://doi.org/10.1038/s41586-024-07493-y
Download citation
Received : 17 October 2021
Accepted : 30 April 2024
Published : 10 July 2024
DOI : https://doi.org/10.1038/s41586-024-07493-y
Share this article
Anyone you share the following link with will be able to read this content:
Sorry, a shareable link is not currently available for this article.
Provided by the Springer Nature SharedIt content-sharing initiative
By submitting a comment you agree to abide by our Terms and Community Guidelines . If you find something abusive or that does not comply with our terms or guidelines please flag it as inappropriate.
Quick links
- Explore articles by subject
- Guide to authors
- Editorial policies
Sign up for the Nature Briefing: Cancer newsletter — what matters in cancer research, free to your inbox weekly.

This website requires Javascript for some parts to function properly. Your experience may vary.
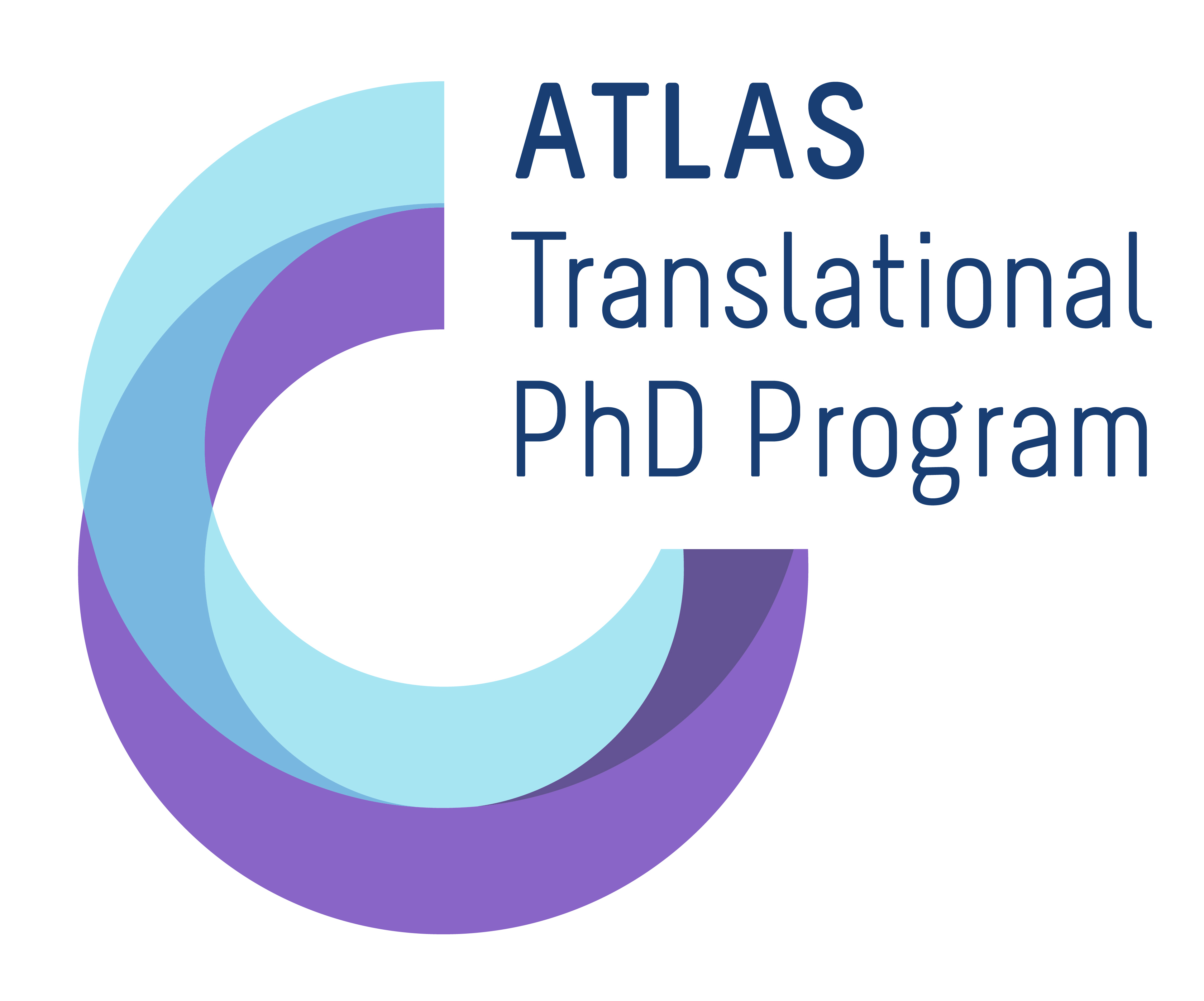
Selected publications
Lang F, Sorn P, Suchan M, Heinrich A, Albrecht C, Köhl N, Beicht A, Riesgo-Ferreiro P, Holsträter C, Schrörs B, Weber D, Löwer M, Sahin U, Ibn-Salem J
Prediction of tumor-specific splicing from somatic mutations as a source of neoantigen candidates
Bioinformatics Advances, 2024
Yıldız A, Hasani A, Hempel T, Köhl N, Beicht A, Becker R, Hubich-Rau S, Suchan M, Poleganov M, Sahin U, Beissert T
Trans-amplifying RNA expressing functional miRNA mediates target gene suppression and simultaneous transgene expression
Molecular Therapy: Nucleic Acids 2024
Beck J, Diken M, Suchan M, Streuber M, Diken E, Kolb L, Allnoch L, Vascotto F, Peters D, Beißert T, Akilli-Öztürk Ö, Türeci Ö, Kreiter- S, Vormehr M, Sahin U
Long-lasting mRNA-encoded interleukin-2 restores CD8+ T cell neoantigen immunity in MHC class I-deficient cancers
Cancer Cell, 2024
Lang F, Sorn P, Schrörs B, Weber D, Kramer S, Sahin U, Löwer L
Multiple instance learning to predict immune checkpoint blockade efficacy using neoantigen candidates
iScience, Volume 26, Issue 11, 2023
Muñoz FM, Sher LD, Sabharwal C, Gurtman A, Xu X, Kitchin N, et al. Evaluation of BNT162b2 Covid-19 Vaccine in Children Younger than 5 Years of Age. New England Journal of Medicine, 2023
Picard FSR, Lutz V, Brichkina A, Neuhaus F, Ruckenbrod T, Hupfer A, Raifer H, Klein M, Bopp T, Pfefferle PI, Savai R, Prinz I, Waisman A, Moos S, Chang HD, Heinrich S, Bartsch DK, Buchholz M, Singh S, Tu M, Klein L, Bauer C, Liefke R, Burchert A, Chung HR, Mayer P, Gress TM, Lauth M, Gaida M, Huber M. IL-17A-producing CD8+ T cells promote PDAC via induction of inflammatory cancer-associated fibroblasts. Gut. 2023 Feb 9:gutjnl-2022-327855.
Saerchen V, Reindl L, Wiedemann S, Shanmugalingam S, Bukur T, Becker J, Suchan M, Ullrich E, Vogler M. Characterization of BV6-Induced Sensitization to the NK Cell Killing of Pediatric Rhabdomyosarcoma Spheroids. Cells 2023, 12(6), 906
Lang F, Schrörs B, Löwer M, Türeci Ö, Sahin U. Identification of neoantigens for individualized therapeutic cancer vaccines. Nature Reviews Drug Discovery, 2022
Muik A., Lui B., Wallisch A., Bacher M., Mühl J., Reinholz J., Ozhelvaci O., Beckmann N., Güimil Garcia R., Poran A., Shpyro S., Finlayson A., Cai H., Yang Q., Swanson K., Türeci Ö., Şahin U. Neutralization of SARS-CoV-2 Omicron by BNT162b2 mRNA vaccine-elicited human sera . Science 2022 ; 375(6581):678-680
Weber D., Ibn-Salem J., Sorn P., Suchan M., Holtsträter C., Lahrmann U., Vogler I., Schmoldt K., Lang F., Schrörs B., Löwer M., Şahin U. Accurate detection of tumor-specific gene fusions reveals strongly immunogenic personal neo-antigens . Nat Biotechnol 2022 ;40(8):1276-1284
Krienke C., Kolb L., Diken E., Streuber M., Kirchhoff S., Bukur T., Akilli-Öztürk Ö., Kranz L., Berger H., Petschenka J., Diken M., Kreiter S., Yogev N., Waisman A., Karikó K., Türeci Ö., Şahin U. A. Noninflammatory mRNA vaccine for treatment of experimental autoimmune encephalomyelitis. Science 2021 ; 371(6525):145-153.
Muik A., Wallisch A., Sänger B., Swanson K., Mühl J., Chen W., Cai H., Maurus D., Sarkar R., Türeci Ö., Dormitzer P., Şahin U. Neutralization of SARS-CoV-2 lineage B.1.1.7 pseudovirus by BNT162b2 vaccine-elicited human sera. Science 2021 ; 371(6534):1152-1153
Şahin U., Muik A., Vogler I., Derhovanessian E., Kranz L., Vormehr M., Quandt J., Bidmon N., et al. BNT162b2 vaccine induces neutralizing antibodies and poly-specific T cells in humans . Nature 2021 ; 595(7868):572-577
Vogel A., Kanevsky I., Che Y., Swanson K., Muik A., Vormehr M., Kranz L., Walzer K., et al. BNT162b vaccines protect rhesus macaques from SARS-CoV-2 . Nature 2021 ; 592(7853):283-289
Mulligan M., Lyke K., Kitchin N., Absalon J., Gurtman A., Lockhart S., Neuzil K., Raabe V., et al. Phase I/II study of COVID-19 RNA vaccine BNT162b1 in adults . Nature 2020 ; 586(7830):589-593
Mulligan M., Lyke K., Kitchin N., Absalon J., Gurtman A., Lockhart S., Neuzil K., Raabe V., Bailey R., Swanson K., Li P., Koury K., Kalina W., Cooper D., Fontes-Garfias C., Shi P., Türeci Ö., Tompkins K., Walsh E., Frenck R., Falsey A., Dormitzer P., Gruber W., Şahin U., Jansen K. Phase I/II study of COVID-19 RNA vaccine BNT162b1 in adults . Nature 2020 ; 586(7830):589-593
Reinhard K., Rengstl B., Oehm P., Michel K., Billmeier A., Hayduk N., Klein O., Kuna K., Ouchan Y., Wöll S., Christ E., Weber D., Suchan M., Bukur T., Birtel M., Jahndel V., Mroz K., Hobohm K., Kranz L., Diken M., Kühlcke K., Türeci Ö., Şahin U. An RNA vaccine drives expansion and efficacy of claudin-CAR-T cells against solid tumors . Science 2020 ;367(6476):446-453
Şahin U., Muik A., Derhovanessian E., Vogler I., Kranz L., Vormehr M., Baum A., Pascal K., et al. COVID-19 vaccine BNT162b1 elicits human antibody and T H 1 T cell responses . Nature 2020 ; 586(7830):594-599
Şahin U., Oehm P., Derhovanessian E., Jabulowsky R., Vormehr M., Gold M., Maurus D., Schwarck-Kokarakis D.,et al. An RNA vaccine drives immunity in checkpoint-inhibitor-treated melanoma . Nature 2020 ; 585(7823):107-112
Şahin U., Oehm P., Derhovanessian E., Jabulowsky R., Vormehr M., Gold M., Maurus D., Schwarck-Kokarakis D., et al. An RNA vaccine drives immunity in checkpoint-inhibitor-treated melanoma . Nature 2020 ; 585(7823):107-112
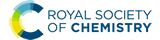
Mass spectrometry-intensive top-down proteomics: an update on technology advancements and biomedical applications

First published on 27th June 2024
Proteoforms are all forms of protein molecules from the same gene because of variations at the DNA, RNA, and protein levels, e.g. , alternative splicing and post-translational modifications (PTMs). Delineation of proteins in a proteoform-specific manner is crucial for understanding their biological functions. Mass spectrometry (MS)-intensive top-down proteomics (TDP) is promising for comprehensively characterizing intact proteoforms in complex biological systems. It has achieved substantial progress in technological development, including sample preparation, proteoform separations, MS instrumentation, and bioinformatics tools. In a single TDP study, thousands of proteoforms can be identified and quantified from a cell lysate. It has also been applied to various biomedical research to better our understanding of protein function in regulating cellular processes and to discover novel proteoform biomarkers of diseases for early diagnosis and therapeutic development. This review covers the most recent technological development and biomedical applications of MS-intensive TDP.
Tian Xu |
Qianjie Wang |
Qianyi Wang |
Liangliang Sun |
1. Introduction
Schematic of a typical top-down proteomics (TDP) workflow, including proteoform extraction from various biological samples, separation by liquid chromatography (LC) or capillary electrophoresis (CE), mass spectrometry (MS) and tandem MS (MS/MS) via various gas-phase fragmentation techniques, and database search using bioinformatics tools for proteoform identification and quantification to discover new proteoform biomarkers of diseases. The figure is created using the BioRender and is used here with permission. |
The complexity of proteoform identification arises from the need to identify and localize PTMs, determine amino acid sequences, and account for unknown or ambiguous genes. 9 Based on the five-level classification system for proteoform identifications, around 40–60% of level 1 proteoforms can be identified without ambiguity. 10–12 However, MS-intensive TDP is still considered challenging in multiple aspects. First, current large-scale TDP studies mainly focused on proteoforms smaller than 30 kDa. Complete characterization of large proteoforms is difficult due to coelution in separation and low sensitivity in MS detection. Second, MS-intensive TDP of membrane proteins is challenging due to their low solubility in MS-compatible buffers. Third, delineating heavily modified proteoforms ( e.g. , histones) requires much more effort to improve separations, fragmentations, and bioinformatics tools for accurate PTM determination and localization. Fourth, achieving the identification (ID) of proteoforms of thousands of protein-coding genes from human cells in a single study is challenging for MS-intensive TDP due to the extremely high sample complexity. It has been estimated that more than one million proteoforms are present in the human body. 13 Early large-scale MS-based TDP studies have generally achieved hundreds to thousands of proteoform IDs from several hundred genes in human cells. Lastly, more work is needed to improve the bioinformatics tools for proteoform ID and quantification to advance TDP in the abovementioned areas. 14–17
During the last several years, MS-intensive TDP has made substantial progress in addressing these challenges. This process has been driven by drastic technological improvements in sample preparation, separation, MS instrumentation, fragmentation strategies, and bioinformatics tools. Several reviews have summarized the related topics in earlier years. 8,18–20 In this review, we updated the most recent technological advancements in MS-intensive TDP, with a particular focus on large proteoforms, membrane proteins, heavily modified proteoforms, global proteoform profiling, high-throughput quantification, and bioinformatics tools. In addition, the review introduced the latest biomedical applications of MS-intensive TDP.
2. Technological development
2.1 characterization of large proteoforms.
Sample fractionation has been extensively applied in recent years to reduce sample complexity, which significantly benefits the downstream characterization of large proteoforms with top-down MS. Size exclusion chromatography (SEC) has been considered as a practical approach to fractionate proteoforms by size, enabling better characterization of large proteoforms. Coupling serial size exclusion chromatography (sSEC) fractionation and reversed-phase liquid chromatography (RPLC)-MS allowed a 15-fold increase in the number of detected proteoforms above 60 kDa. 25 Further application of sSEC-RPLC-MS and MS/MS with Proteoform Suite software 17 achieved the ID of large proteoforms up to 140 kDa from human heart samples. 26 The 140 kDa protein (endogenous human cardiac myosin binding protein C) was identified by top-down MS for the first time. Most recently, the ID of intact large proteoforms (myosin heavy chain, ∼223 kDa) from single cells (single muscle fibers, SMFs) was reported via advanced RPLC-MS-based TDP. 27 The Zhang group developed an SEC-RPLC-MS platform utilizing a novel monolithic RP capillary column for TDP of an E. coli lysate, identifying 347 proteoforms larger than 30 kDa in a single RPLC run. 28 While advanced SEC and RPLC techniques have been widely utilized to identify large proteoforms, the global characterization of large proteoforms from cell lysates remains challenging. One of the primary reasons is the limited separation capacity of SEC and RPLC for large proteoforms.
Besides LC, electrophoretic methods are promising for large proteoform separations. Capillary zone electrophoresis (CZE) separates analytes under an electric field according to their charge-to-size ratios. As CZE performs separation in an open tubular fused silica capillary containing no stationary phase, it can achieve higher separation efficiency and less sample loss for large proteoforms than many conventional LC techniques. 29–33 Additionally, CZE-MS/MS has been well recognized as a valuable technique for TDP as the nanoflow CE-MS interface ( i.e. , 50–300 nL min −1 ) provides high sensitivity for detection. 32–38 The Kelleher group utilized a GELFrEE (gel-eluted liquid fraction entrapment electrophoresis)-CZE-MS/MS platform to improve the detection of large proteoforms. 39 Thirty proteins in the mass range of 30–80 kDa from the Pseudomonas aeruginosa whole-cell lysate were identified. The Sun group coupled FAIMS (high field asymmetric waveform ion mobility spectrometry) to CZE-MS/MS to achieve online two-dimensional separations (IMS and CZE) for improving the ID of large proteoforms, Fig. 2 . 40 By altering the compensation voltages from −50 to 30 V, FAIMS enabled the efficient fractionation of proteoform ions in the gas phase, with a median proteoform mass range from less than 10 kDa to about 30 kDa. CZE-FAIMS-MS/MS boosted the number of proteoform IDs six-fold in the 20–45 kDa range. Apart from CZE, capillary isoelectric focusing (cIEF) has been well known for ultra-high resolution protein separation based on their isoelectric points (pIs). 41,42 cIEF-MS has been widely used for top-down MS characterization of proteoforms. 32,43 Several research groups have demonstrated that cIEF-MS is highly efficient for the characterization of charge variants of monoclonal antibodies (mAbs, ∼150 kDa). 43–47 Recently, the Sun group developed a native cIEF-MS technique for the delineation of large protein complexes and the accurate determination of pIs of various forms of large protein complexes. 48 More studies are still needed to improve the performance of CE-MS/MS for the global characterization of large proteoforms from cell lysates. The Takemori group developed passive eluting proteins from polyacrylamide gels as intact species for MS (“PEPPI-MS”) for MS-intensive TDP. 49 The PEPPI is a gel-based electrophoresis method for high-resolution separation of proteoforms based on their masses and recovery of proteoforms from polyacrylamide gels. A 68% median recovery rate for <100 kDa proteins and a 57% median recovery rate for proteins larger than 100 kDa were reported previously. Coupling PEPPI to RPLC-MS and MS/MS identified nearly 60 proteoforms higher than 30 kDa from an E. coli cell lysate. Although PEPPI efficiently separates large proteoforms, the PEPPI workflow includes protein cleanup steps before MS analysis. More careful evaluations are needed for comprehensive proteoform ID and accurate quantification using the PEPPI-based approach. 50
Schematic of capillary zone electrophoresis (CZE)-field asymmetric waveform ion mobility spectrometry (FAIMS)-mass spectrometry (MS) and MS/MS for top-down proteomics (TDP) of a complex sample. Using nine different compensation voltages, FAIMS achieved online gas phase fractionation, resulting in a nearly 3-fold increase in the number of proteoform IDs. This method particularly benefited the identification of relatively large proteoforms compared to CZE-MS/MS alone. Reproduced from ref. with permission from the American Chemical Society, copyright 2023. |
Another big challenge of large proteoform ID with the TDP approach is the limited backbone cleavage coverage from conventional gas-phase fragmentation techniques ( i.e. , collision-induced dissociation, CID, and higher energy collision dissociation, HCD), impeding the accurate localization of PTMs and sequence variations. Electron or photon-based fragmentation techniques ( i.e. , electron transfer dissociation, ETD, electron capture dissociation, ECD, and ultraviolet photodissociation, UVPD) have demonstrated their substantially better performance than HCD for large proteoform fragmentation. 57–59 However, even with advanced fragmentation methods, resolving the overlapping peaks and complex spectra of large proteoforms remains challenging. The spectral complexity introduced by multiple charge states can be reduced by ion–ion reactions, such as proton transfer charge reaction (PTCR), where a multiply charged cation reacts with a singly charged reagent anion to generate cations with reduced charge. 60 For intact proteoforms, using PTCR with isolated precursors (<1.5 m / z ) has doubled the identification of proteoforms over 30 kDa. 61 Broadband isolation of the entire charge state distribution of intact proteins enabled the detection of proteins ranging from 14 to 148 kDa. 62 At the tandem mass spectra scale, PTCR limited signal overlap produced by ETD and improved the sequence coverage of proteins spanning from 29 to 56 kDa. 63 The PTCR MS 3 via HCD further increased the sequence coverage of 56 kDa glutamate dehydrogenase to 44%. Post-activation ion mobility spectrometry also simplified the mass spectra and enabled unambiguous assignment of typically overlapping product ions. 64
2.2 MS-intensive TDP of membrane proteins
Advanced MP purification/extraction methods with multidimensional liquid-phase separations have been used for MP characterization by TDP. The Ge group developed a novel photo-cleavable surfactant (Azo) to enable TDP of integral membrane proteins (IMPs) by providing the solubility of IMPs and compatibility with MS using UV irradiation-based degradation. 69 More work remains to be done to make Azo more broadly used in TDP studies, e.g. , more systematic and critical evaluations of Azo for IMPs in terms of degradation, MS interference, and its compatibility with online LC-MS/MS or CE-MS/MS. They also used cloud point extraction to enrich the MPs and identified 188 and 124 endogenous IMPs from human embryonic kidney cells and cardiac tissues, respectively, via coupling SEC with RPLC-MS/MS. 70 The Zhang group developed a novel C8-functional amine-bridged hybrid monolith capillary column for RPLC-MS/MS analysis of MPs. 71 Integrating ultracentrifugation-based MP purification, GELFrEE fractionation, and RPLC-MS/MS identified 3100 membrane proteoforms up to 50 kDa from a mouse hippocampus sample, representing the largest TDP dataset of membrane proteoforms. Beyond RPLC-MS/MS, CZE-MS/MS has also been deployed for the TDP of MPs. The Sun group recently developed an efficient CZE-MS/MS method for TDP of IMPs from a mouse brain sample. 72 The method employs a sample buffer containing 30% (v/v) formic acid and 60% (v/v) methanol for solubilizing IMPs and utilizes a separation buffer of 30% (v/v) acetic acid and 30% (v/v) methanol for maintaining the solubility of IMPs during the CZE separation. Coupling SEC fractionation with CZE-MS/MS identified 276 IMP proteoforms with 1–4 transmembrane domains (TMDs), demonstrating the high potential of SEC-CZE-MS/MS for TDP of IMPs. However, further improvements are required for the global characterization of large IMPs using CZE-MS/MS.
Advancements in MS instrumentation have allowed more accurate characterization of MPs and MP complexes. The Robinson group delineated rhodopsin receptor signaling cascade in a native membrane using an advanced Orbitrap mass spectrometer. 73 Various gas-phase fragmentation techniques ( i.e. , ETD, CID, HCD, UVPD, and infrared multiphoton dissociation, IRMPD) have been validated for IMP fragmentation. ETD favors the cleavage in the soluble region of IMPs, while CID and HCD have a dramatic bias towards TMDs, due to the lack of basic residues. 74 Compared with the 28% sequence coverage of the c-subunit of ATP synthase (8 kDa) by CAD, activated ion ECD (ai-ECD) achieved 72% coverage by irradiating the precursor ion using infrared prior to ECD. 75 Regarding the fragmentation of MP complexes from native MS, the sequence coverage is typically relatively low (10–20%). 76 UVPD fragments protein ions via the absorption of high-energy photons. The ions are directly dissociated without energy redistribution, preserving bound ligands and labile PTMs. 77 UVPD enhanced the sequence coverage of aquaporin Z (AqpZ, 99 kDa) and mechanosensitive channel of large conductance (MscL, 79 kDa) to 45% and 53%, respectively, compared with 30% and 34% obtained from HCD. 78 However, the enhancement of UVPD is also highly dependent on the membrane complex structure and sequence. Infrared multiphoton dissociation (IRMPD) accumulates many low-energy photons and gradually increases the vibrational energy to break the amide (C–N) bonds. It has also been used to liberate IMPs from micelles. 79 Using a modified Orbitrap Eclipse Tribrid MS with a continuous wave far IR CO 2 laser, the Robinson group achieved selective detergent removal and a successive cleavage of adjacent residues in TMDs with a 26% sequence coverage of AmtB. 80 Moreover, the Ruotolo group improved IMP sequence coverage to 40–60% by applying IR photoactivation before HCD. 81 While significant progress has been made in MS instrumentation for more comprehensive characterization of MPs, combining advanced MP extraction and separation techniques with novel MS/MS methodologies for global characterization of MPs in whole cell lysates has not been done and needs further effort.
2.3 MS-intensive TDP for global profiling of proteoforms in complex samples
With the improvement of the sample preparation method and LC-MS/MS or CE-MS/MS, achieving TDP measurement of proteoforms in mass-limited biological samples ( i.e. , nanoproteomics in single cell scale) is possible. Nanoproteomics refers to the proteomics analysis of small cell populations, spanning from individual cells to clusters of cells that contain less than 1 μg protein amount in total. 85,86 Coupling nanoPOTS-based sample preparation 87 and advanced capillary RPLC-MS/MS has enabled TDP of a small number of mammalian cells with the ID of hundreds of proteoforms from tens to hundreds of HeLa cells. 88 The Ge group developed a highly sensitive TDP workflow for SMFs, Fig. 3 . 27 They employed a one-pot sample processing approach to reduce protein loss and a capillary RPLC-MS/MS system with micro-flow-nanospray ionization (MnESI) to boost the sensitivity of MS measurement. They observed significant phosphorylation heterogeneity of proteoforms of myosin light chain 2 (MLC-2) in different SMFs. The Ivanov group reported TDP of single mammalian cells using CZE-MS/MS with the assistance of on-capillary cell lysis. 89 Dozens of proteoforms were identified by MS/MS from single HeLa cells. The Sun group integrated laser capture microdissection, one-step sample processing, and CZE-MS/MS for TDP of isolated zebrafish brain regions. 11 Over 400 proteoforms were identified from one LCM brain section with a 500 μm 2 area and 20 μm thickness. Recently, the Wu group developed a novel CZE-MS platform (Spray capillary) for handling pL–nL level biological samples for CZE-MS analysis. 90 Although the technique has not been thoroughly investigated for TDP of small numbers of mammalian cells, it showed exciting results for single-cell analysis of metabolites. These technical advancements open the door to MS-based TDP of mass-limited biological samples. However, substantial work is still needed to improve the whole TDP workflow for reliable and quantitative measurement of proteoforms in trace samples.
Schematic of the highly sensitive top-down proteomics (TDP) workflow for the characterization of proteoforms in single muscle fibers, including the mechanical dissociation of SMFs (A), velocity measurement of each muscle in a force transducer (B), protein extraction (C), capillary RPLC separation of SMF extracts (D), MnESI ionization of SMF proteins coupled with high-resolution MS analysis (E), and data analysis of the TDP data by MS/MS as well as the large proteoform detection (F). Reproduced from ref. with permission from the National Academy of Sciences (NAS), copyright 2023. |
2.4 MS-intensive TDP of highly modified proteoforms
Histones, acting as the fundamental framework within the nucleosome where genomic DNA is intricately packed, are essential for both the regulation of epigenetic gene expression and the maintenance of chromatin's structural stability 91–95 The protruded N-terminal tails of the four core histones (H2A, H2B, H3, and H4) from the nucleosome structure contain a wide variety of PTMs, such as methylation, acetylation, phosphorylation, and citrullination. 93,96 With the diverse PTMs and high heterogeneity of histones, fully deciphering how histone proteoforms contribute to epigenetic gene regulation remains difficult. 97,98 High-resolution separation and extensive gas-phase fragmentation of histone proteoforms are crucial. The Sun group developed SEC-CZE-MS/MS (HCD) and CZE-FAIMS-MS/MS (HCD) for high-capacity separation and highly sensitive TDP measurement of histone proteoforms, leading to the ID of nearly 400 and 600 histone proteoforms from a commercial calf thymus sample. 99,100 PTM localization for TDP analysis continues to pose a hindrance because the production of substantial fragment ions from highly efficient gas-phase fragmentation is vital for confidently mapping the sequence and confirming the PTM information. 101 To accurately localize the PTMs on histone proteoforms, the Brodbelt group integrated UVPD with gas-phase PTCR to achieve up to 91% sequence coverage of calf thymus histone H4 with N-terminal acetylation, K12 acetylation, and R3 dimethylation. 102 To separate highly isomeric histone proteoforms, the Jensen group applied trapped ion mobility spectrometry (TIMS)-time-of-flight (TOF) to resolve the isomers of monoacetylated H4 histone, providing a useful approach for the separation of highly similar histone proteoforms by their conformational differences. 103 Another challenge for TDP analysis of histone proteins is associated with accurately differentiating the near-isobaric modifications, such as distinguishing acetylation and trimethylation as well as formylation and dimethylation (Δ M = 0.0364 Da). The high mass resolution MS/MS and the low mass tolerance are extremely essential to assigning correct fragment ions of histone proteoforms. 104 We expect that researchers will integrate the advanced separation techniques, gas-phase fragmentation methods and high-resolution mass spectrometers together soon to characterize histone proteoforms better.
Protein glycosylation modulates a wide range of cell functions and is a highly diverse type of PTM. 105,106 N - and O -linked glycosylation are the two main types of glycosylation. Due to the enormous structural microheterogeneity, efficient separation and ultra-high-resolution MS measurement are necessary for the top-down MS characterization of glycoproteoforms. The spike (S) protein, essential for severe acute respiratory syndrome coronavirus 2 (SARS-CoV-2) virus entry, is highly glycosylated and contains 66 potential N -linked glycosylation sites within each trimer. 107–109 Interestingly, three possible O -linked glycosylation sites have been predicted, and two of them were identified at the receptor-binding domain (RBD) of the S protein subunit S1. 110,111 The abundant glycosylation on S protein enables the SARS-CoV-2 to escape innate and adaptive immune responses by protecting the epitopes from antibody neutralization. 109,112 However, due to the enormous microheterogeneity of N -glycans and O -glycans, each glycosylation site could result in numerous possibilities of variable glycans with different arrangements and branched structures. Therefore, interpreting highly glycosylated S protein in a proteoform-specific manner is essential to provide new insights into virus binding, fusion, cell entry, and the development of vaccines. The Ge group explored the structural heterogeneity of different conformers of S protein RBD by native top-down TIMS-MS/MS and applied ultrahigh-resolution Fourier transform ion cyclotron resonance (FTICR)-MS/MS to deeply analyze the glycosylation sites and the corresponding glycans by denatured top-down MS. 113 Overall, they disclosed eight S protein RBD O -glycosylated proteoforms, a core 2-fucosylated glycan structure, and the relative quantification information, proving that the combination of IMS and ultrahigh-resolution MS is capable of characterizing highly glycosylated S protein RBD. Another approach to investigate the glycosylation heterogeneity of S protein is using CDMS. The Jarrold group utilized top-down CDMS measurements to study the heterogeneity of glycosylation at the 66 N -glycan sites of S protein trimer, offering much more accurate insights into the glycosylation heterogeneity of S protein compared to bottom-up glycoproteomics data. 114 Those works studied purified glycoproteins using the top-down MS approach. However, much effort must be made to achieve global profiling of glycoproteoforms in cells or tissues.
2.5 MS-intensive TDP for high-throughput proteoform quantification
2.6 bioinformatics tools for ms-intensive tdp.
The current bioinformatics tools for MS-based TDP have unsatisfied performance for ID of large proteoforms ( i.e. , >30 kDa) because the software typically relies on isotopically resolved peaks in each charge state to determine the accurate monoisotopic mass of proteoforms or their fragment ions for subsequent database searches, which is called deconvolution. Typical high-resolution mass spectrometers cannot offer high enough mass resolution to achieve isotopically resolved peaks for large proteoforms. Alternatively, for unresolved isotopic peaks, the average mass can be determined based on the charge state distribution, which is utilized in UniDec and TDPortal. 129 Minor changes in deconvolution parameters may resolve distinct results, and the erroneous deconvolution of proteoforms leads to incorrect false discovery rate estimation at the proteoform-level. 130
Recently, Jeong et al. presented an ultrafast deconvolution tool for TDP, FLASHDeconv, capable of analyzing both isotopically resolved and unresolved peaks for broad charge and mass range in MS spectra, which is especially useful for large proteoform ID. 131 The same research group further developed an intelligent online data acquisition algorithm FLASHIda, enabling ultrafast proteoform deconvolution in real time during data acquisition. 132 The commonly used data-dependent acquisition (DDA) method selects the top N most intense peaks for fragmentation. However, this method tends to choose multiple charge state peaks from a high abundance proteoform, leading to the difficulty in the ID of low abundance proteoforms. FLASHIda enables less redundant selection of precursor ions via real-time FLASHDeconv, boosting the proteoform coverage in MS-based TDP studies. There is another real-time deconvolution and precursor selection software for MS-based TDP, MetaDrive, which aims to combine the multiple charge states of the same proteoform to improve the quality of the MS/MS spectra and the backbone cleavage coverage of proteoforms. 133 This approach could be potentially valuable for bettering the sequence coverage of large proteoforms.
Another reason of complex spectra is the internal fragment ions, which contain neither N- nor C-terminus of proteoforms and are not recognized by most software, especially when a combination of fragmentation is employed. 134,135 More recently, the Loo group highlighted the value of internal fragment ions for boosting proteoform sequence coverage from various fragmentation techniques and developed a bioinformatics tool ( i.e. , ClipsMS) to facilitate the use of internal fragment ions in top-down MS studies. 136–140 The information on internal fragment ions improves the sequence coverage of large proteoforms substantially. The Loo group combined ECD (e-MSion), HCD, and internal fragment ion assignments for top-down MS analysis of the whole NIST mAb, producing a 75% sequence coverage, the highest sequence coverage of an intact mAb by TDP so far. 139 Besides the ClipsMS software by the Loo group, the Smith group implemented internal fragment ion functionality in their MetaMorpheus software for MS-based TDP of cell lysates with internal fragment ion annotation. 141 The approach improved the number of proteoform IDs and the distinguishment of similar proteoforms significantly compared to the conventional terminal fragment ion approach. However, the internal fragment ion assignment requires high mass accuracy and special attention in controlling false positive hits. Additionally, to make the internal fragment ions more useful for general MS-intensive TDP studies, integration of automated annotation of internal fragment ions in the most widely used bioinformatics tools ( i.e. , ProSight 15 and TopPIC 14 ) will be critically important.
Although various bioinformatics tools have been developed to improve the quality of proteoform characterization, alternative methods are still needed to verify the identified proteoforms from the database search, especially those proteoforms carrying PTMs ( e.g. , phosphorylation and acetylation). The Sun group reported high linear correlations ( R 2 = 0.98) between predicted and experimental electrophoretic mobility of proteoforms identified by CZE-MS/MS-based TDP. 142 The Liu group also demonstrated the solid linear correlations between the predicted and experimental migration/retention time of proteoforms identified in CZE-MS/MS and RPLC-MS/MS studies. 143 More importantly, the proteoforms carrying phosphorylation and/or acetylation PTM can be confirmed by comparing their predicted and experimental electrophoretic mobility due to the charge reduction from those PTMs. 142 The results indicate that integrating the predicted and experimental electrophoretic mobility or retention time information into the database search bioinformatics tools could potentially improve the confidence and accuracy of proteoform IDs. The Liu group also developed a new bioinformatics tool, PTM-TBA (PTM characterization by top-down and bottom-up MS and annotations), based on the TopPIC software to better the localization of PTMs on identified proteoforms via combining the top-down and bottom-up MS data and PTM annotations in the UniProt database. 144
Besides proteoform ID, some new bioinformatics tools have been developed for label-free proteoform quantification. The Tholey group developed MSTopDiff to improve the annotation of artificial and biological modifications of the detected proteoforms. 145 The Petyuk group developed a companion R package (TopPICR) for TopPIC to enable label-free quantification. 146 The TopPICR tool performs many functions related to label-free quantification, including ID filter, retention time alignment across runs, mass recalibration, and proteoform feature intensity calculation with the match-between-runs approach.
One significant problem in bioinformatics for MS-based TDP is that proteoform IDs from the same dataset using different bioinformatics tools are substantially different in deconvolution results and proteoform IDs. 147 This phenomenon might be due to the differences in, for example, mass deconvolution, the database for search, and the scoring algorithm for matching experimental and theoretical data for the TDP bioinformatics tools. The Ge group built MASH Explorer as a universal and user-friendly software for MS-based TDP. 148 The software combines several widely used spectral deconvolution tools and database search algorithms into one platform, providing flexibility and consistency for proteoform IDs using TDP. It can also process TDP data from different vendor formats, which is particularly useful for the broad TDP community.
3. Biomedical applications of MS-intensive TDP
3.1 cancer biology.
Summary of the label-free proteoform quantification data of metastatic (SW620) and non-metastatic (SW480) colorectal cancer (CRC) cells from size exclusion chromatography (SEC)-capillary zone electrophoresis (CZE)-tandem mass spectrometry (MS/MS). (A) Heatmap and clusters of the quantified proteoforms from the two cell lines. (B) Volcano plot analysis indicating significantly differentially expressed proteoforms. Reproduced from ref. with permission from the American Association for the Advancement of Science (AAAS), copyright 2022. |
3.2 Neurodegenerative diseases
3.3 cardiac injury, 3.4 diabetes and cardiovascular diseases (cvd), 3.5 infectious disease, 4. conclusions and future directions.
Much more effort is needed to advance the technique of MS-intensive TDP to facilitate its broad adoption in biological research. Technical development is needed to boost proteome coverage, throughput, and quality of proteoform characterization. Integration of novel sample preparation methods, efficient liquid-phase separations, advanced MS ( e.g. , charge detection MS, individual ion MS, and native MS), and better bioinformatics tools could be extremely valuable for solving the technical challenges of TDP. We must point out that high-quality and global top-down MS measurement of intact proteoforms, especially large and heavily modified ones, needs mass spectrometers with high speed, high mass resolution, and extensive proteoform gas-phase fragmentation. Those instrumentation are extremely expensive and have high maintenance costs, which is one critical factor limiting the broad use of MS-based TDP.
There are several new frontiers in the MS-intensive TDP field. First, increasing TDP applications are rolling from the conventional denaturing TDP into the native mode (native proteomics) to characterize protein complexes in cells. 48,186–191 Second, single-cell TDP will be another hot research area to decipher cell-to-cell heterogeneity at the proteoform level. 27,88,89 Third, the human proteoform project 185 will be one focus of the TDP community. Fourth, deciphering proteoform function in biological processes and disease progression is essential for the TDP field. As mentioned in this review, many TDP studies have been done to better our understanding of protein function in various diseases. We expect more and more such studies to be carried out with better proteome coverage and proteoform characterization due to the continuous advancements of MS-based TDP techniques. Finally, combining MS-intensive TDP and BUP is crucial to advance TDP for high throughput and extensive characterization of proteoforms in complex samples. BUP data can provide rich information on types and sites of PTMs, which is extremely useful to interpret PTM combinations on proteoforms from TDP measurement. It is also essential to have a standardized procedure from sample preparation to database search, as the emerging field of TDP continues to develop. 68,147 Unbiased quality control that singles out high-quality mass spectra is a gateway for building up TDP spectral libraries and, ultimately, the human proteoform atlas. 192
Data availability
Conflicts of interest, acknowledgements.
- L. M. Smith and N. L. Kelleher, Consortium for Top Down Proteomics. Proteoform: a single term describing protein complexity, Nat. Methods , 2013, 10 (3), 186–187 CrossRef CAS .
- L. M. Smith and N. L. Kelleher, Proteoforms as the next proteomics currency, Science , 2018, 359 (6380), 1106–1107 CrossRef CAS PubMed .
- H. A. Costa, M. G. Leitner and M. L. Sos, et al. , Discovery and functional characterization of a neomorphic PTEN mutation, Proc. Natl. Acad. Sci. U. S. A. , 2015, 112 (45), 13976–13981 CrossRef CAS PubMed .
- X. Yang, J. Coulombe-Huntington and S. Kang, et al. , Widespread Expansion of Protein Interaction Capabilities by Alternative Splicing, Cell , 2016, 164 (4), 805–817 CrossRef CAS .
- E. N. McCool, T. Xu and W. Chen, et al. , Deep top-down proteomics revealed significant proteoform-level differences between metastatic and nonmetastatic colorectal cancer cells, Sci. Adv. , 2022, 8 (51), eabq6348 CrossRef CAS , * The first example of quantitative TDP of colorectal cancer (CRC) cells to understand CRC metastasis better..
- T. Tucholski, W. Cai and Z. R. Gregorich, et al. , Distinct hypertrophic cardiomyopathy genotypes result in convergent sarcomeric proteoform profiles revealed by top-down proteomics, Proc. Natl. Acad. Sci. U. S. A. , 2020, 117 (40), 24691–24700 CrossRef CAS .
- L. M. Adams, C. J. DeHart and B. S. Drown, et al. , Mapping the KRAS proteoform landscape in colorectal cancer identifies truncated KRAS4B that decreases MAPK signaling, J. Biol. Chem. , 2023, 299 (1), 102768 CrossRef CAS .
- L. V. Schaffer, R. J. Millikin and R. M. Miller, et al. , Identification and Quantification of Proteoforms by Mass Spectrometry, Proteomics , 2019, 19 (10), e1800361 CrossRef , ** A nice review about TDP technologies..
- L. M. Smith, P. M. Thomas and M. R. Shortreed, et al. , A five-level classification system for proteoform identifications, Nat. Methods , 2019, 16 , 939–940 CrossRef CAS PubMed .
- Q. Wang, L. Sun and P. K. Lundquist, Large-scale top-down proteomics of the Arabidopsis thaliana and chloroplast proteomes, Proteomics , 2023, e2100377 CrossRef .
- R. A. Lubeckyj and L. Sun, Laser capture microdissection-capillary zone electrophoresis- tandem mass spectrometry (LCM-CZE-MS/MS) for spatially resolved top-down proteomics: a pilot study of zebrafish brain, Mol. Omics , 2022, 18 , 112–122 RSC .
- B. S. Drown, K. Jooß and R. D. Melani, et al. , Mapping the Proteoform Landscape of Five Human Tissues, J. Proteome Res. , 2022, 21 (5), 1299–1310 CrossRef CAS PubMed .
- R. Aebersold, J. N. Agar and I. J. Amster, et al. , How many human proteoforms are there?, Nat. Chem. Biol. , 2018, 14 (3), 206–214 CrossRef CAS PubMed .
- Q. Kou, L. Xun and X. Liu, TopPIC: a software tool for top-down mass spectrometry-based proteoform identification and characterization, Bioinformatics , 2016, 32 (22), 3495–3497 CrossRef CAS .
- R. D. LeDuc, G. K. Taylor and Y. B. Kim, et al. , ProSight PTM: an integrated environment for protein identification and characterization by top-down mass spectrometry, Nucleic Acids Res. , 2004, 32 (Web Server issue), W340–W345 CrossRef CAS .
- W. Cai, H. Guner and Z. R. Gregorich, et al. , MASH Suite Pro: A Comprehensive Software Tool for Top-Down Proteomics, Mol. Cell. Proteomics , 2016, 15 (2), 703–714 CrossRef CAS PubMed .
- A. J. Cesnik, M. R. Shortreed and L. V. Schaffer, et al. , Proteoform Suite: Software for Constructing, Quantifying, and Visualizing Proteoform Families, J. Proteome Res. , 2018, 17 (1), 568–578 CrossRef CAS PubMed .
- K. A. Brown, J. A. Melby and D. S. Roberts, et al. , Top-down proteomics: challenges, innovations, and applications in basic and clinical research, Expert Rev. Proteomics , 2020, 17 (10), 719–733 CrossRef CAS PubMed .
- J. S. Brodbelt, Deciphering combinatorial post-translational modifications by top-down mass spectrometry, Curr. Opin. Chem. Biol. , 2022, 70 , 102180 CrossRef CAS PubMed .
- T. K. Toby, L. Fornelli and N. L. Kelleher, Progress in Top-Down Proteomics and the Analysis of Proteoforms, Annu. Rev. Anal. Chem. , 2016, 9 (1), 499–519 CrossRef CAS PubMed .
- T. N. Tiambeng, Z. Wu and J. A. Melby, et al. , Size Exclusion Chromatography Strategies and MASH Explorer for Large Proteoform Characterization, Methods Mol. Biol. , 2022, 2500 , 15–30 CrossRef CAS PubMed .
- L. Fornelli and T. K. Timothy, Characterization of large intact protein ions by mass spectrometry: What directions should we follow?, Biochim. Biophys. Acta, Proteins Proteomics , 1870,(4), 140758 CrossRef PubMed .
- P. D. Compton, L. Zamdborg and P. M. Thomas, et al. , On the scalability and requirements of whole protein mass spectrometry, Anal. Chem. , 2011, 83 (17), 6868–6874 CrossRef CAS PubMed .
- J. O. Kafader, R. D. Melani and L. F. Schachner, et al. , Native vs Denatured: An in-Depth Investigation of Charge State and Isotope Distributions, J. Am. Soc. Mass Spectrom. , 2020, 31 , 574–581 CrossRef CAS PubMed .
- W. Cai, T. Tucholski and B. Chen, et al. , Top-Down Proteomics of Large Proteins up to 223 kDa Enabled by Serial Size Exclusion Chromatography Strategy, Anal. Chem. , 2017, 89 (10), 5467–5475 CrossRef CAS PubMed .
- L. V. Schaffer, T. Tucholski and M. R. Shortreed, et al. , Intact-Mass Analysis Facilitating the Identification of Large Human Heart Proteoforms, Anal. Chem. , 2019, 91 (17), 10937–10942 CrossRef CAS .
- J. A. Melby, K. A. Brown and Z. R. Gregorich, et al. , High sensitivity top-down proteomics captures single muscle cell heterogeneity in large proteoforms, Proc. Natl. Acad. Sci. U. S. A. , 2023, 120 (19), e2222081120 CrossRef CAS , * An excellent example of TDP measurment of mass-limited biological samples via advanced technologies..
- C. Wang, Y. Liang and B. Zhao, et al. , Ethane-Bridged Hybrid Monolithic Column with Large Mesopores for Boosting Top-Down Proteomic Analysis, Anal. Chem. , 2022, 94 (16), 6172–6179 CrossRef CAS PubMed .
- Y. Li, S. Miao and J. Tan, et al. , Capillary electrophoresis: A three-year literature review, Anal. Chem. , 2024, 96 , 7799–7816 CrossRef CAS PubMed .
- K. Bagwe, N. Gould and K. R. Johnson, et al. , Single-cell omic molecular profiling using capillary electrophoresis-mass spectrometry, Trends Anal. Chem. , 2023, 165 , 117117 CrossRef CAS .
- A.-K. Schwenzer, L. Kruse and K. Jooß, et al. , Capillary electrophoresis-mass spectrometry for protein analyses under native conditions: Current progress and perspectives, Proteomics , 2024, 24 (2–4), e2300135 CrossRef .
- D. Chen, E. N. McCool and Z. Yang, et al. , Recent advances (2019-2021) of capillary electrophoresis-mass spectrometry for multilevel proteomics, Mass Spectrom. Rev. , 2023, 42 (2), 617–642 CrossRef CAS PubMed , ** A comprehensive review of CE-MS for BUP, TDP and native proteomics..
- F. P. Gomes and J. R. Yates 3rd, Recent trends of capillary electrophoresis-mass spectrometry in proteomics research, Mass Spectrom. Rev. , 2019, 38 (6), 445–460 CrossRef CAS PubMed .
- R. A. Lubeckyj, A. R. Basharat and X. Shen, et al. , Large-Scale Qualitative and Quantitative Top-Down Proteomics Using Capillary Zone Electrophoresis-Electrospray Ionization-Tandem Mass Spectrometry with Nanograms of Proteome Samples, J. Am. Soc. Mass Spectrom. , 2019, 30 (8), 1435–1445 CrossRef CAS PubMed .
- T. T. T. N. Nguyen, N. J. Petersen and K. D. Rand, A simple sheathless CE-MS interface with a sub-micrometer electrical contact fracture for sensitive analysis of peptide and protein samples, Anal. Chim. Acta , 2016, 936 , 157–167 CrossRef CAS PubMed .
- E. J. Maxwell, X. Zhong and H. Zhang, et al. , Decoupling CE and ESI for a more robust interface with MS, Electrophoresis , 2010, 31 , 1130–1137 CrossRef CAS .
- J. Schlecht, A. Stolz and A. Hofmann, et al. , nanoCEasy: An Easy, Flexible, and Robust Nanoflow Sheath Liquid Capillary Electrophoresis-Mass Spectrometry Interface Based on 3D Printed Parts, Anal. Chem. , 2021, 93 , 14593–14598 CrossRef CAS PubMed .
- X. Han, Y. Wang and A. Aslanian, et al. , In-line separation by capillary electrophoresis prior to analysis by top-down mass spectrometry enables sensitive characterization of protein complexes, J. Proteome Res. , 2014, 13 (12), 6078–6086 CrossRef CAS PubMed .
- Y. Li, P. D. Compton and J. C. Tran, et al. , Optimizing capillary electrophoresis for top-down proteomics of 30-80 kDa proteins, Proteomics , 2014, 14 (10), 1158–1164 CrossRef PubMed .
- T. Xu, Q. Wang and Q. Wang, et al. , Coupling High-Field Asymmetric Waveform Ion Mobility Spectrometry with Capillary Zone Electrophoresis-Tandem Mass Spectrometry for Top-Down Proteomics, Anal. Chem. , 2023, 95 (25), 9497–9504 CrossRef CAS PubMed .
- Q. Tang, A. K. Harrata and C. S. Lee, Capillary isoelectric focusing-electrospray mass spectrometry for protein analysis, Anal. Chem. , 1995, 67 , 3515–3519 CrossRef CAS .
- P. T. Ljiljana, K. J. Pamela and A. A. Gordon, et al. , High Throughput Proteome-Wide Precision Measurements of Protein Expression Using Mass Spectrometry, J. Am. Chem. Soc. , 1999, 121 , 7949–7950 CrossRef .
- T. Xu and L. Sun, A Mini Review on Capillary Isoelectric Focusing-Mass Spectrometry for Top-Down Proteomics, Front. Chem. , 2021, 9 , 651757 CrossRef CAS .
- T. Xu, L. Han and A. M. George Thompson, et al. , An improved capillary isoelectric focusing-mass spectrometry method for high-resolution characterization of monoclonal antibody charge variants, Anal. Methods , 2022, 14 (4), 383–393 RSC .
- J. Dai, J. Lamp and Q. Xia, et al. , Capillary Isoelectric Focusing-Mass Spectrometry Method for the Separation and Online Characterization of Intact Monoclonal Antibody Charge Variants, Anal. Chem. , 2018, 90 (3), 2246–2254 CrossRef CAS PubMed .
- L. Wang, T. Bo and Z. Zhang, et al. , High Resolution Capillary Isoelectric Focusing Mass Spectrometry Analysis of Peptides, Proteins, And Monoclonal Antibodies with a Flow-through Microvial Interface, Anal. Chem. , 2018, 90 (15), 9495–9503 CrossRef CAS PubMed .
- S. Mack, D. Arnold and G. Bogdan, et al. , A novel microchip-based imaged CIEF-MS system for comprehensive characterization and identification of biopharmaceutical charge variants, Electrophoresis , 2019, 40 (23–24), 3084–3091 CrossRef CAS .
- T. Xu, L. Han and L. Sun, Automated Capillary Isoelectric Focusing-Mass Spectrometry with Ultrahigh Resolution for Characterizing Microheterogeneity and Isoelectric Points of Intact Protein Complexes, Anal. Chem. , 2022, 94 (27), 9674–9682 CrossRef CAS PubMed .
- A. Takemori, D. S. Butcher and V. M. Harman, et al. , PEPPI-MS: Polyacrylamide-Gel-Based Prefractionation for Analysis of Intact Proteoforms and Protein Complexes by Mass Spectrometry, J. Proteome Res. , 2020, 19 (9), 3779–3791 CrossRef CAS PubMed .
- A. Takemori, P. T. Kaulich and L. Cassidy, et al. , Size-Based Proteome Fractionation through Polyacrylamide Gel Electrophoresis Combined with LC–FAIMS–MS for In-Depth Top-Down Proteomics, Anal. Chem. , 2022, 94 (37), 12815–12821 CrossRef CAS PubMed .
- D. Z. Keifer, T. Motwani and C. M. Teschke, et al. , Measurement of the accurate mass of a 50 MDa infectious virus, Rapid Commun. Mass Spectrom. , 2016, 30 (17), 1957–1962 CrossRef CAS PubMed .
- T. P. Wörner, J. Snijder and A. Bennett, et al. , Resolving heterogeneous macromolecular assemblies by Orbitrap-based single-particle charge detection mass spectrometry, Nat. Methods , 2020, 17 , 395–398 CrossRef PubMed .
- J. O. Kafader, R. D. Melani and K. R. Durbin, et al. , Multiplexed mass spectrometry of individual ions improves measurement of proteoforms and their complexes, Nat. Methods , 2020, 17 (4), 391–394 CrossRef CAS PubMed , * A strong demonstration of the principle and application of I2MS for highly sensitive TDP..
- P. Su, J. P. McGee and K. R. Durbin, et al. , Highly multiplexed, label-free proteoform imaging of tissues by individual ion mass spectrometry, Sci. Adv. , 2022, 8 (32), eabp9929 CrossRef CAS PubMed .
- T. P. Wörner, K. Aizikov and J. Snijder, et al. , Frequency chasing of individual megadalton ions in an Orbitrap analyser improves precision of analysis in single-molecule mass spectrometry, Nat. Chem. , 2022, 14 (5), 515–522 CrossRef PubMed .
- M. A. Zenaidee and J. A. Loo, Seeing flying molecular elephants more clearly, Nat. Chem. , 2022, 14 (5), 482–483 CrossRef CAS PubMed .
- N. M. Riley, M. S. Westphall and J. J. Coon, Sequencing Larger Intact Proteins (30-70 kDa) with Activated Ion Electron Transfer Dissociation, J. Am. Soc. Mass Spectrom. , 2018, 29 (1), 140–149 CrossRef CAS .
- J. F. Greisch, M. A. den Boer and S. H. Lai, et al. , Extending Native Top-Down Electron Capture Dissociation to MDa Immunoglobulin Complexes Provides Useful Sequence Tags Covering Their Critical Variable Complementarity-Determining Regions, Anal. Chem. , 2021, 93 (48), 16068–16075 CrossRef CAS PubMed .
- J. B. Shaw, W. Li and D. D. Holden, et al. , Complete protein characterization using top-down mass spectrometry and ultraviolet photodissociation, J. Am. Chem. Soc. , 2013, 135 (34), 12646–12651 CrossRef CAS PubMed .
- S. J. Pitteri and S. A. McLuckey, Recent developments in the ion/ion chemistry of high-mass multiply charged ions, Mass Spectrom. Rev. , 2005, 24 , 931–958 CrossRef CAS PubMed .
- R. Huguet, C. Mullen and K. Srzentić, et al. , Proton transfer charge reduction enables high-throughput top-down analysis of large proteoforms, Anal. Chem. , 2019, 91 , 15732–15739 CrossRef CAS PubMed .
- A. O. Bailey, R. Huguet and C. Mullen, et al. , Ion–ion charge reduction addresses multiple challenges common to denaturing intact mass analysis, Anal. Chem. , 2022, 94 , 3930–3938 CrossRef CAS PubMed .
- J. T. Kline, C. Mullen and K. R. Durbin, et al. , Sequential ion–ion reactions for enhanced gas-phase sequencing of large intact proteins in a tribrid orbitrap mass spectrometer, J. Am. Soc. Mass Spectrom. , 2021, 32 , 2334–2345 CrossRef CAS PubMed .
- N. B. Borotto and K. A. Graham, Fragmentation and mobility separation of peptide and protein ions in a trapped-ion mobility device, Anal. Chem. , 2021, 93 , 9959–9964 CrossRef CAS PubMed .
- C. V. Robinson, Mass spectrometry: From plasma proteins to mitochondrial membranes, Proc. Natl. Acad. Sci. U. S. A. , 2019, 116 (8), 2814–2820 CrossRef CAS PubMed .
- H. Chu, Q. Zhao and J. Liu, et al. , Ionic Liquid-Based Extraction System for In-Depth Analysis of Membrane Protein Complexes, Anal. Chem. , 2022, 94 (2), 758–767 CrossRef CAS PubMed .
- J. E. Keener, G. Zhang and M. T. Marty, Native Mass Spectrometry of Membrane Proteins, Anal. Chem. , 2021, 93 , 583–597 CrossRef CAS PubMed .
- D. P. Donnelly, C. M. Rawlins and C. J. DeHart, et al. , Best practices and benchmarks for intact protein analysis for top-down mass spectrometry, Nat. Methods , 2019, 16 (7), 587–594 CrossRef CAS PubMed .
- K. A. Brown, B. Chen and T. M. Guardado-Alvarez, et al. , A photocleavable surfactant for top-down proteomics, Nat. Methods , 2019, 16 (5), 417–420 CrossRef CAS PubMed .
- K. A. Brown, T. Tucholski and A. J. Alpert, et al. , Top-Down Proteomics of Endogenous Membrane Proteins Enabled by Cloud Point Enrichment and Multidimensional Liquid Chromatography-Mass Spectrometry, Anal. Chem. , 2020, 92 (24), 15726–15735 CrossRef CAS PubMed .
- Y. Sun, Y. Liang and C. Wang, et al. , Enhancing Top-Down Characterization of Membrane Proteoforms with C8-Functional Amine-Bridged Hybrid Monoliths, Anal. Chem. , 2023, 95 (17), 6846–6853 CrossRef CAS PubMed .
- Q. Wang, T. Xu and F. Fang, et al. , Capillary Zone Electrophoresis-Tandem Mass Spectrometry for Top-Down Proteomics of Mouse Brain Integral Membrane Proteins, Anal. Chem. , 2023, 95 (34), 12590–12594 CrossRef CAS PubMed .
- S. Chen, T. Getter and D. Salom, et al. , Capturing a rhodopsin receptor signalling cascade across a native membrane, Nature , 2022, 604 (7905), 384–390 CrossRef CAS PubMed .
- O. S. Skinner, A. D. Catherman and B. P. Early, et al. , Fragmentation of integral membrane proteins in the gas phase, Anal. Chem. , 2014, 86 (9), 4627–4634 CrossRef CAS PubMed .
- V. Zabrouskov and J. P. Whitelegge, Increased Coverage in the Transmembrane Domain with Activated-Ion Electron Capture Dissociation for Top-Down Fourier-Transform Mass Spectrometry of Integral Membrane Proteins, J. Proteome Res. , 2007, 6 , 2205–2210 CrossRef CAS PubMed .
- C. Lantz, B. Wei and B. Zhao, et al. , Native Top-Down Mass Spectrometry with Collisionally Activated Dissociation Yields Higher-Order Structure Information for Protein Complexes, J. Am. Chem. Soc. , 2022, 144 (48), 21826–21830 CrossRef CAS PubMed .
- S. D. Dunham, B. Wei and C. Lantz, et al. , Impact of Internal Fragments on Top-Down Analysis of Intact Proteins by 193 nm UVPD, J. Proteome Res. , 2023, 22 (1), 170–181 CrossRef CAS PubMed .
- S. N. Sipe, J. W. Patrick and A. Laganowsky, et al. , Enhanced Characterization of Membrane Protein Complexes by Ultraviolet Photodissociation Mass Spectrometry, Anal. Chem. , 2020, 92 (1), 899–907 CrossRef CAS PubMed .
- I. D. Campuzano, H. Li and D. Bagal, et al. , Native MS Analysis of Bacteriorhodopsin and an Empty Nanodisc by Orthogonal Acceleration Time-of-Flight, Orbitrap and Ion Cyclotron Resonance, Anal. Chem. , 2016, 88 (24), 12427–12436 CrossRef CAS PubMed .
- C. A. Lutomski, T. J. El-Baba and J. D. Hinkle, et al. , Infrared Multiphoton Dissociation Enables Top-Down Characterization of Membrane Protein Complexes and G Protein-Coupled Receptors, Angew. Chem., Int. Ed. Engl. , 2023, 62 (36), e202305694 CrossRef CAS PubMed .
- B. R. Juliano, J. W. Keating and B. T. Ruotolo, Infrared Photoactivation Enables Improved Native Top-Down Mass Spectrometry of Transmembrane Proteins, Anal. Chem. , 2023, 95 , 13361–13367 CrossRef CAS PubMed .
- T. Xu, X. Shen and Z. Yang, et al. , Automated Capillary Isoelectric Focusing-Tandem Mass Spectrometry for Qualitative and Quantitative Top-Down Proteomics, Anal. Chem. , 2020, 92 (24), 15890–15898 CrossRef CAS PubMed .
- Z. Wang, D. Yu and K. A. Cupp-Sutton, et al. , Development of an Online 2D Ultrahigh-Pressure Nano-LC System for High-pH and Low-pH Reversed Phase Separation in Top-Down Proteomics, Anal. Chem. , 2020, 92 (19), 12774–12777 CrossRef CAS PubMed .
- Y. Shen, N. Tolić and C. Masselon, et al. , Nanoscale proteomics, Anal. Bioanal. Chem. , 2004, 378 (4), 1037–1045 CrossRef CAS PubMed .
- L. Yi, P. D. Piehowski and T. Shi, et al. , Advances in microscale separations towards nanoproteomics applications, J. Chromatogr. A , 2017, 1523 , 40–48 CrossRef CAS PubMed .
- Y. Zhu, P. D. Piehowski and R. Zhao, et al. , Nanodroplet processing platform for deep and quantitative proteome profiling of 10-100 mammalian cells, Nat. Commun. , 2018, 9 (1), 882 CrossRef PubMed .
- M. Zhou, N. Uwugiaren and S. M. Williams, et al. , Sensitive Top-Down Proteomics Analysis of a Low Number of Mammalian Cells Using a Nanodroplet Sample Processing Platform, Anal. Chem. , 2020, 92 (10), 7087–7095 CrossRef CAS PubMed .
- K. R. Johnson, Y. Gao and M. Greguš, et al. , On-capillary Cell Lysis Enables Top-down Proteomic Analysis of Single Mammalian Cells by CE-MS/MS, Anal. Chem. , 2022, 94 (41), 14358–14367 CrossRef CAS PubMed .
- L. Huang, M. Fang and K. A. Cupp-Sutton, et al. , Spray-Capillary-Based Capillary Electrophoresis Mass Spectrometry for Metabolite Analysis in Single Cells, Anal. Chem. , 2021, 93 (10), 4479–4487 CrossRef CAS PubMed .
- A. I. Badeaux and Y. Shi, Emerging roles for chromatin as a signal integration and storage platform, Nat. Rev. Mol. Cell Biol. , 2013, 14 (4), 211–224 CrossRef CAS PubMed .
- H. Kimura, Histone modifications for human epigenome analysis, J. Hum. Genet. , 2013, 58 (7), 439–445 CrossRef CAS PubMed .
- T. Jenuwein and C. D. Allis, Translating the histone code, Science , 2001, 293 (5532), 1074–1080 CrossRef CAS PubMed .
- C. L. Woodcock, A. I. Skoultchi and Y. Fan, Role of linker histone in chromatin structure and function: H1 stoichiometry and nucleosome repeat length, Chromosome Res. , 2006, 14 (1), 17–25 CrossRef CAS PubMed .
- H. Kimura, Histone dynamics in living cells revealed by photobleaching, DNA Repair , 2005, 4 (8), 939–950 CrossRef CAS PubMed .
- C. D. Allis and T. Jenuwein, The molecular hallmarks of epigenetic control, Nat. Rev. Genet. , 2016, 17 (8), 487–500 CrossRef CAS PubMed .
- Y. Zhao and B. A. Garcia, Comprehensive Catalog of Currently Documented Histone Modifications, Cold Spring Harbor Perspect. Biol. , 2015, 7 (9), a025064 CrossRef PubMed .
- D. T. Brown, Histone variants: are they functionally heterogeneous?, Genome Biol. , 2001, 2 (7), REVIEWS0006 CrossRef CAS PubMed .
- D. Chen, Z. Yang and X. Shen, et al. , Capillary Zone Electrophoresis-Tandem Mass Spectrometry As an Alternative to Liquid Chromatography-Tandem Mass Spectrometry for Top-down Proteomics of Histones, Anal. Chem. , 2021, 93 (10), 4417–4424 CrossRef CAS PubMed .
- Q. Wang, F. Fang and Q. Wang, et al. , Capillary zone electrophoresis-high field asymmetric ion mobility spectrometry-tandem mass spectrometry for top-down characterization of histone proteoforms, Proteomics , 2024, 24 (3–4), e2200389 CrossRef PubMed .
- J. N. Walker, R. Lam and J. S. Brodbelt, Enhanced Characterization of Histones Using 193 nm Ultraviolet Photodissociation and Proton Transfer Charge Reduction, Anal. Chem. , 2023, 95 (14), 5985–5993 CrossRef CAS PubMed .
- F. Berthias, H. A. Thurman and G. Wijegunawardena, et al. , Top-Down Ion Mobility Separations of Isomeric Proteoforms, Anal. Chem. , 2023, 95 (2), 784–791 CAS .
- A. Moradian, A. Kalli and M. J. Sweredoski, et al. , The top-down, middle-down, and bottom-up mass spectrometry approaches for characterization of histone variants and their post-translational modifications, Proteomics , 2014, 14 (4–5), 489–497 CrossRef CAS PubMed .
- A. Varki, Biological roles of glycans, Glycobiology , 2017, 27 (1), 3–49 CrossRef CAS PubMed .
- C. Reily, T. J. Stewart, M. B. Renfrow and J. Novak, Glycosylation in health and disease, Nat. Rev. Nephrol. , 2019, 15 (6), 346–366 CrossRef PubMed .
- R. Yan, Y. Zhang and Y. Li, et al. , Structural basis for the recognition of SARS-CoV-2 by full-length human ACE2, Science , 2020, 367 (6485), 1444–1448 CrossRef CAS PubMed .
- M. Hoffmann, H. Kleine-Weber and S. Schroeder, et al. , SARS-CoV-2 Cell Entry Depends on ACE2 and TMPRSS2 and Is Blocked by a Clinically Proven Protease Inhibitor, Cell , 2020, 181 (2), 271–280.e8 CrossRef CAS PubMed .
- Y. Watanabe, J. D. Allen and D. Wrapp, et al. , Site-specific glycan analysis of the SARS-CoV-2 spike, Science , 2020, 369 (6501), 330–333 CrossRef CAS PubMed .
- K. G. Andersen, A. Rambaut and W. I. Lipkin, et al. , The proximal origin of SARS-CoV-2, Nat. Med. , 2020, 26 (4), 450–452 CrossRef CAS PubMed .
- A. Shajahan, N. T. Supekar and A. S. Gleinich, et al. , Deducing the N- and O-glycosylation profile of the spike protein of novel coronavirus SARS-CoV-2, Glycobiology , 2020, 30 (12), 981–988 CrossRef CAS PubMed .
- Y. Watanabe, T. A. Bowden and I. A. Wilson, et al. , Exploitation of glycosylation in enveloped virus pathobiology, Biochim. Biophys. Acta, Gen. Subj. , 2019, 1863 (10), 1480–1497 CrossRef CAS PubMed .
- D. S. Roberts, M. Mann and J. A. Melby, et al. , Structural O-Glycoform Heterogeneity of the SARS-CoV-2 Spike Protein Receptor-Binding Domain Revealed by Top-Down Mass Spectrometry, J. Am. Chem. Soc. , 2021, 143 (31), 12014–12024 CrossRef CAS PubMed .
- L. M. Miller, L. F. Barnes and S. A. Raab, et al. , Heterogeneity of Glycan Processing on Trimeric SARS-CoV-2 Spike Protein Revealed by Charge Detection Mass Spectrometry, J. Am. Chem. Soc. , 2021, 143 (10), 3959–3966 CrossRef CAS PubMed .
- S. Wu, J. N. Brown and N. Tolić, et al. , Quantitative analysis of human salivary gland-derived intact proteome using top-down mass spectrometry, Proteomics , 2014, 14 , 1211–1222 CrossRef CAS PubMed .
- I. Ntai, K. Kim and R. T. Fellers, et al. , Applying Label-Free Quantitation to Top Down Proteomics, Anal. Chem. , 2014, 86 , 4961–4968 CrossRef CAS PubMed .
- J. Sinclair and J. F. Timms, Quantitative profiling of serum samples using TMT protein labelling, fractionation and LC–MS/MS, Methods , 2011, 54 , 361–369 CrossRef CAS PubMed .
- H. Fang, K. Xiao and Y. Li, et al. , Intact Protein Quantitation Using Pseudoisobaric Dimethyl Labeling, Anal. Chem. , 2016, 88 , 7198–7205 CrossRef CAS PubMed .
- C.-W. Hung and A. Tholey, Tandem Mass Tag Protein Labeling for Top-Down Identification and Quantification, Anal. Chem. , 2012, 84 , 161–170 CrossRef CAS PubMed .
- S. Wiese, K. A. Reidegeld and H. E. Meyer, et al. , Protein labeling by iTRAQ: A new tool for quantitative mass spectrometry in proteome research, Proteomics , 2007, 7 , 340–350 CrossRef CAS PubMed .
- Z. Liu, R. Wang and J. Liu, et al. , Global Quantification of Intact Proteins via Chemical Isotope Labeling and Mass Spectrometry, J. Proteome Res. , 2019, 18 , 2185–2194 CrossRef CAS PubMed .
- D. Yu, Z. Wang and K. A. Cupp-Sutton, et al. , Quantitative Top-Down Proteomics in Complex Samples Using Protein-Level Tandem Mass Tag Labeling, J. Am. Soc. Mass Spectrom. , 2021, 32 , 1336–1344 CrossRef CAS PubMed .
- K. A. Cupp-Sutton and S. Wu, High-throughput quantitative top-down proteomics, Mol. Omics , 2020, 16 , 91–99 RSC .
- Y. Guo, D. Yu and K. A. Cupp-Sutton, et al. , Optimization of protein-level tandem mass tag (TMT) labeling conditions in complex samples with top-down proteomics, Anal. Chim. Acta , 2022, 1221 , 340037 CrossRef CAS PubMed .
- Y. Guo, T. Chowdhury and M. Seshadri, et al. , Optimization of Higher-Energy Collisional Dissociation Fragmentation Energy for Intact Protein-Level Tandem Mass Tag Labeling, J. Proteome Res. , 2023, 22 , 1406–1418 CrossRef CAS PubMed .
- R.-X. Sun, L. Luo and L. Wu, et al. , pTop 1.0: A High-Accuracy and High-Efficiency Search Engine for Intact Protein Identification, Anal. Chem. , 2016, 88 , 3082–3090 CrossRef CAS PubMed .
- J. Park, P. D. Piehowski and C. Wilkins, et al. , Informed-Proteomics: Open Source Software Package for Top-down Proteomics, Nat. Methods , 2017, 14 , 909–914 CrossRef CAS PubMed .
- S. K. Solntsev, M. R. Shortreed, B. L. Frey and L. M. Smith, Enhanced Global Post-translational Modification Discovery with MetaMorpheus, J. Proteome Res. , 2018, 17 (5), 1844–1851 CrossRef CAS PubMed .
- M. T. Marty, A. J. Baldwin and E. G. Marklund, et al. , Bayesian deconvolution of mass and ion mobility spectra: From binary interactions to polydisperse ensembles, Anal. Chem. , 2015, 87 , 4370–4376 CrossRef CAS PubMed .
- K. Jeong, P. T. Kaulich and W. Jung, et al. , Precursor deconvolution error estimation: The missing puzzle piece in false discovery rate in top-down proteomics, Proteomics , 2024, 3–4 , 2300068 CrossRef PubMed .
- K. Jeong, J. Kim and M. Gaikwad, et al. , FLASHDeconv: Ultrafast, High-Quality Feature Deconvolution for Top-Down Proteomics, Cell Syst. , 2020, 10 , 213–218.e6 CrossRef CAS PubMed .
- K. Jeong, M. Babović and V. Gorshkov, et al. , FLASHIda enables intelligent data acquisition for top–down proteomics to boost proteoform identification counts, Nat. Commun. , 2022, 13 , 4407 CrossRef CAS PubMed .
- L. Lu, M. Scalf and M. R. Shortreed, et al. , Mesh fragmentation improves dissociation efficiency in top-down proteomics, J. Am. Soc. Mass Spectrom. , 2021, 32 , 1319–1325 CrossRef CAS PubMed .
- S. R. Harvey, M. Porrini and A. Konijnenberg, et al. , Dissecting the dynamic conformations of the metamorphic protein lymphotactin, J. Phys. Chem. B , 2014, 118 (43), 12348–12359 CrossRef CAS PubMed .
- K. R. Durbin, O. S. Skinner and R. T. Fellers, et al. , Analyzing internal fragmentation of electrosprayed ubiquitin ions during beam-type collisional dissociation, J. Am. Soc. Mass Spectrom. , 2015, 26 (5), 782–787 CrossRef CAS PubMed .
- M. A. Zenaidee, C. Lantz and T. Perkins, et al. , Internal Fragments Generated by Electron Ionization Dissociation Enhance Protein Top-Down Mass Spectrometry, J. Am. Soc. Mass Spectrom. , 2020, 31 (9), 1896–1902 CrossRef CAS PubMed .
- M. A. Zenaidee, B. Wei and C. Lantz, et al. , Internal Fragments Generated from Different Top-Down Mass Spectrometry Fragmentation Methods Extend Protein Sequence Coverage, J. Am. Soc. Mass Spectrom. , 2021, 32 (7), 1752–1758 CrossRef CAS PubMed .
- B. Wei, M. A. Zenaidee and C. Lantz, et al. , Towards understanding the formation of internal fragments generated by collisionally activated dissociation for top-down mass spectrometry, Anal. Chim. Acta , 2022, 1194 , 339400 CrossRef CAS PubMed .
- B. Wei, C. Lantz and W. Liu, et al. , Added Value of Internal Fragments for Top-Down Mass Spectrometry of Intact Monoclonal Antibodies and Antibody-Drug Conjugates, Anal. Chem. , 2023, 95 (24), 9347–9356 CrossRef CAS PubMed .
- C. Lantz, M. A. Zenaidee and B. Wei, et al. , ClipsMS: An Algorithm for Analyzing Internal Fragments Resulting from Top-Down Mass Spectrometry, J. Proteome Res. , 2021, 20 (4), 1928–1935 CrossRef CAS PubMed .
- Z. Rolfs and L. M. Smith, Internal Fragment Ions Disambiguate and Increase Identifications in Top-Down Proteomics, J. Proteome Res. , 2021, 20 (12), 5412–5418 CrossRef CAS PubMed .
- D. Chen, R. A. Lubeckyj and Z. Yang, et al. , Predicting Electrophoretic Mobility of Proteoforms for Large-Scale Top-Down Proteomics, Anal. Chem. , 2020, 92 , 3503–3507 CrossRef CAS PubMed .
- W. Chen, E. N. McCool and L. Sun, et al. , Evaluation of Machine Learning Models for Proteoform Retention and Migration Time Prediction in Top-Down Mass Spectrometry, J. Proteome Res. , 2022, 21 , 1736 CrossRef CAS PubMed .
- W. Chen, Z. Ding and Y. Zang, et al. , Characterization of Proteoform Post-Translational Modifications by Top-Down and Bottom-Up Mass Spectrometry in Conjunction with Annotations, J. Proteome Res. , 2023, 22 , 3178–3189 CrossRef CAS PubMed .
- P. T. Kaulich, K. Winkels and T. B. Kaulich, et al. , MSTopDiff: A Tool for the Visualization of Mass Shifts in Deconvoluted Top-Down Proteomics Data for the Database-Independent Detection of Protein Modifications, J. Proteome Res. , 2022, 21 , 20–29 CrossRef CAS PubMed .
- E. A. Martin, J. M. Fulcher and M. Zhou, et al. , TopPICR: A Companion R Package for Top-Down Proteomics Data Analysis, J. Proteome Res. , 2023, 22 , 399–409 CrossRef CAS PubMed .
- D. L. Tabb, K. Jeong and K. Druart, et al. , Comparing Top-Down Proteoform Identification: Deconvolution, PrSM Overlap, and PTM Detection, J. Proteome Res. , 2023, 22 , 2199–2217 CrossRef CAS PubMed .
- Z. Wu, D. S. Roberts and J. A. Melby, et al. , MASH Explorer: A Universal Software Environment for Top-Down Proteomics, J. Proteome Res. , 2020, 19 , 3867–3876 CrossRef CAS PubMed .
- M. A. Eckert, F. Coscia and A. Chryplewicz, et al. , Proteomics reveals NNMT as a master metabolic regulator of cancer-associated fibroblasts, Nature , 2019, 569 (7758), 723–728 CrossRef CAS PubMed .
- A. A. Cohen, N. Geva-Zatorsky and E. Eden, et al. , Dynamic proteomics of individual cancer cells in response to a drug, Science , 2008, 322 (5907), 1511–1516 CrossRef CAS PubMed .
- P. R. Srinivas, M. Verma and Y. Zhao, et al. , Proteomics for cancer biomarker discovery, Clin. Chem. , 2002, 48 (8), 1160–1169 CAS .
- I. Ntai, R. D. LeDuc and R. T. Fellers, et al. , Integrated Bottom-Up and Top-Down Proteomics of Patient-Derived Breast Tumor Xenografts, Mol. Cell. Proteomics , 2016, 15 (1), 45–56 CrossRef CAS PubMed .
- I. Ntai, L. Fornelli and C. J. DeHart, et al. , Precise characterization of KRAS4b proteoforms in human colorectal cells and tumors reveals mutation/modification cross-talk, Proc. Natl. Acad. Sci. U. S. A. , 2018, 115 (16), 4140–4145 CrossRef CAS PubMed .
- R. A. D'Ippolito, D. Rabara, M. A. Blanco and E. Alberico, et al. , A Top-Down Proteomic Assay to Evaluate KRAS4B-Compound Engagement, Anal. Chem. , 2024, 96 (13), 5223–5231 CrossRef PubMed .
- M. V. Holt, T. Wang and N. L. Young, High-throughput quantitative top-down proteomics: histone H4, J. Am. Soc. Mass Spectrom. , 2019, 30 (12), 2548–2560 CrossRef CAS PubMed .
- J. P. McGee, P. Su, K. R. Durbin, M. A. Hollas, N. W. Bateman, G. L. Maxwell, T. P. Conrads, R. T. Fellers, R. D. Melani, J. M. Camarillo and J. O. Kafader, Automated imaging and identification of proteoforms directly from ovarian cancer tissue, Nat. Commun. , 2023, 14 (1), 6478 CrossRef CAS PubMed .
- J. M. Fulcher, A. Makaju and R. J. Moore, et al. , Enhancing Top-Down Proteomics of Brain Tissue with FAIMS, J. Proteome Res. , 2021, 20 (5), 2780–2795 CrossRef CAS PubMed .
- J. F. Kellie, R. E. Higgs and J. W. Ryder, et al. , Quantitative measurement of intact alpha-synuclein proteoforms from post-mortem control and Parkinson's disease brain tissue by intact protein mass spectrometry, Sci. Rep. , 2014, 4 , 5797 CrossRef CAS PubMed .
- N. D. Schmitt and J. N. Agar, Parsing disease-relevant protein modifications from epiphenomena: perspective on the structural basis of SOD1-mediated ALS, J. Mass Spectrom. , 2017, 52 (7), 480–491 CrossRef CAS PubMed .
- L. M. Forgrave, K. M. Moon, J. E. Hamden, Y. Li, P. Lu, L. J. Foster, I. R. Mackenzie and M. L. DeMarco, Truncated TDP-43 proteoforms diagnostic of frontotemporal dementia with TDP-43 pathology, Alzheimer’s Dementia , 2024, 20 (1), 103–111 CrossRef CAS PubMed .
- H. Wesseling, W. Mair and M. Kumar, et al. , Tau PTM Profiles Identify Patient Heterogeneity and Stages of Alzheimer's Disease, Cell , 2020, 183 (6), 1699–1713.e13 CrossRef CAS PubMed .
- M. Nshanian, C. Lantz, P. Wongkongkathep and T. Schrader, et al. , Native top-down mass spectrometry and ion mobility spectrometry of the interaction of tau protein with a molecular tweezer assembly modulator, J. Am. Soc. Mass Spectrom. , 2018, 30 (1), 16–23 CrossRef PubMed .
- H. V. Tran, M. Charles and R. C. Garrett, et al. , Ten-year trends in traumatic cardiac injury and outcomes: a trauma registry analysis, Ann. Thorac. Surg. , 2020, 110 (3), 844–848 CrossRef PubMed .
- J. Zhang, M. J. Guy and H. S. Norman, et al. , Top-down quantitative proteomics identified phosphorylation of cardiac troponin I as a candidate biomarker for chronic heart failure, J. Proteome Res. , 2011, 10 (9), 4054–4065 CrossRef CAS PubMed .
- T. N. Tiambeng, D. S. Roberts and K. A. Brown, et al. , Nanoproteomics enables proteoform-resolved analysis of low-abundance proteins in human serum, Nat. Commun. , 2020, 11 (1), 3903 CrossRef CAS PubMed .
- T. Tucholski, W. Cai and Z. R. Gregorich, et al. , Distinct hypertrophic cardiomyopathy genotypes result in convergent sarcomeric proteoform profiles revealed by top-down proteomics, Proc. Natl. Acad. Sci. U. S. A. , 2020, 117 (40), 24691–24700 CrossRef CAS PubMed .
- E. A. Chapman, T. J. Aballo and J. A. Melby, et al. , Defining the Sarcomeric Proteoform Landscape in Ischemic Cardiomyopathy by Top-Down Proteomics, J. Proteome Res. , 2023, 22 (3), 931–941 CrossRef CAS PubMed .
- A. Bodin, V. Labas, A. Bisson, A. P. Teixeira-Gomes, H. Blasco, D. Tomas, L. Combes-Soia, P. Marcelo, E. Miquelestorena-Standley, C. Baron and D. Angoulvant, Acute pathophysiological myocardial changes following intra-cardiac electrical shocks using a proteomic approach in a sheep model, Sci. Rep. , 2020, 10 (1), 20252 CrossRef CAS PubMed .
- A. Ramirez-Sagredo, A. Sunny, K. Cupp-Sutton, et al. , Characterizing Age-related Changes in Intact Mitochondrial Proteoforms in Murine Hearts using Quantitative Top-Down Proteomics, Res. Sq. [Preprint] , 2024, DOI: 10.21203/rs.3.rs-3868218/v1 .
- D. Nedelkov, Mass spectrometric studies of apolipoprotein proteoforms and their role in lipid metabolism and type 2 diabetes, Proteomes , 2017, 5 (4), 27 CrossRef CAS PubMed .
- P. Mao and D. Wang, Top-down proteomics of a drop of blood for diabetes monitoring, J. Proteome Res. , 2014, 13 (3), 1560–1569 CrossRef CAS PubMed .
- H. D. S. Seckler, L. Fornelli and R. K. Mutharasan, et al. , A targeted, differential top-down proteomic methodology for comparison of ApoA-I proteoforms in individuals with high and low HDL efflux capacity, J. Proteome Res. , 2018, 17 (6), 2156–2164 CrossRef CAS PubMed .
- C. Lloyd-Jones, H. dos Santos Seckler and N. DiStefano, et al. , Preparative Electrophoresis for HDL Particle Size Separation and Intact-Mass Apolipoprotein Proteoform Analysis, J. Proteome Res. , 2023, 22 (5), 1455–1465 CrossRef CAS PubMed .
- J. Borén, C. J. Packard and M. R. Taskinen, The roles of ApoC-III on the metabolism of triglyceride-rich lipoproteins in humans, Front. Endocrinol. , 2020, 11 , 474 CrossRef PubMed .
- M. T. Mazur, H. L. Cardasis, D. S. Spellman, A. Liaw, N. A. Yates and R. C. Hendrickson, Quantitative analysis of intact apolipoproteins in human HDL by top-down differential mass spectrometry, Proc. Natl. Acad. Sci. U. S. A. , 2010, 107 (17), 7728–7733 CrossRef CAS PubMed .
- H. N. Yassine, O. Trenchevska and A. Ramrakhiani, et al. , The association of human apolipoprotein C-III sialylation proteoforms with plasma triglycerides, PLoS One , 2015, 10 (12), e0144138 CrossRef PubMed .
- N. C. Kegulian, B. Ramms and S. Horton, et al. , ApoC-III glycoforms are differentially cleared by hepatic TRL (triglyceride-rich lipoprotein) receptors, Arterioscler., Thromb., Vasc. Biol. , 2019, 39 (10), 2145–2156 CrossRef CAS PubMed .
- Z. R. Gregorich and Y. Ge, Top-down proteomics in health and disease: Challenges and opportunities, Proteomics , 2014, 14 (10), 1195–1210 CrossRef CAS PubMed .
- Y. Simanjuntak, K. Schamoni-Kast and A. Grün, et al. , Top-Down and Bottom-Up Proteomics Methods to Study RNA Virus Biology, Viruses , 2021, 13 (4), 668 CrossRef CAS PubMed .
- D. S. Roberts, M. Mann and B. H. Li, et al. , Distinct core glycan and O-glycoform utilization of SARS-CoV-2 Omicron variant Spike protein RBD revealed by top-down mass spectrometry, Chem. Sci. , 2022, 13 (36), 10944–10949 RSC .
- C. Gstottner, T. Zhang and A. Resemann, et al. , Structural and functional characterization of SARS-CoV-2 RBD domains produced in mammalian cells, Anal. Chem. , 2021, 93 (17), 6839–6847 CrossRef PubMed .
- J. W. Wilson, A. Bilbao and J. Wang, et al. , Online hydrophilic interaction chromatography (HILIC) enhanced top-down mass spectrometry characterization of the SARS-CoV-2 spike receptor-binding domain, Anal. Chem. , 2022, 94 (15), 5909–5917 CrossRef CAS PubMed .
- R. D. Melani, B. J. Des Soye and J. O. Kafader, et al. , Next-generation serology by mass spectrometry: readout of the SARS-CoV-2 antibody repertoire, J. Proteome Res. , 2021, 21 (1), 274–288 CrossRef PubMed .
- F. Meissner, J. Geddes-McAlister and M. Mann, et al. , The emerging role of mass spectrometry-based proteomics in drug discovery, Nat. Rev. Drug Discovery , 2022, 21 (9), 637–654 CrossRef CAS PubMed .
- L. M. Smith, J. N. Agar and J. Chamot-Rooke, et al. , The Human Proteoform Project: Defining the human proteome, Sci. Adv. , 2021, 7 (46), eabk0734 CrossRef CAS PubMed .
- X. Shen, Q. Kou and R. Guo, et al. , Native Proteomics in Discovery Mode Using Size-Exclusion Chromatography-Capillary Zone Electrophoresis-Tandem Mass Spectrometry, Anal. Chem. , 2018, 90 (17), 10095–10099 CrossRef CAS PubMed .
- O. S. Skinner, N. A. Haverland and L. Fornelli, et al. , Top-down characterization of endogenous protein complexes with native proteomics, Nat. Chem. Biol. , 2018, 14 (1), 36–41 CrossRef CAS PubMed .
- A. M. Belov, R. Viner and M. R. Santos, et al. , Analysis of Proteins, Protein Complexes, and Organellar Proteomes Using Sheathless Capillary Zone Electrophoresis - Native Mass Spectrometry, J. Am. Soc. Mass Spectrom. , 2017, 28 (12), 2614–2634 CrossRef CAS PubMed .
- Y. Ge, E. Chapman and D. Roberts, et al. , Structure and dynamics of endogenous protein complexes in human heart tissue captured by native nanoproteomics, Res. Sq. , 2023, rs.3.rs-3108087 Search PubMed .
- J. Yates III, F. Gomes and K. Durbin, et al. , Native top-down proteomics reveals EGFR-ERα signaling crosstalk in breast cancer cells dissociates NUTF2 dimers to modulate ERα signaling and cell growth, Res. Sq. , 2023, rs.3.rs-3097806 Search PubMed .
- M. R. Mehaffey, Q. Xia and J. S. Brodbelt, Uniting Native Capillary Electrophoresis and Multistage Ultraviolet Photodissociation Mass Spectrometry for Online Separation and Characterization of Escherichia coli Ribosomal Proteins and Protein Complexes, Anal. Chem. , 2020, 92 (22), 15202–15211 CrossRef CAS PubMed .
- D. B. Lima, A. R. F. Silva and M. Dupré, et al. , Top-Down Garbage Collector: a tool for selecting high-quality top-down proteomics mass spectra, Bioinformatics , 2019, 35 , 3489–3490 CrossRef CAS PubMed .
† Those two authors contributed equally to this work. |
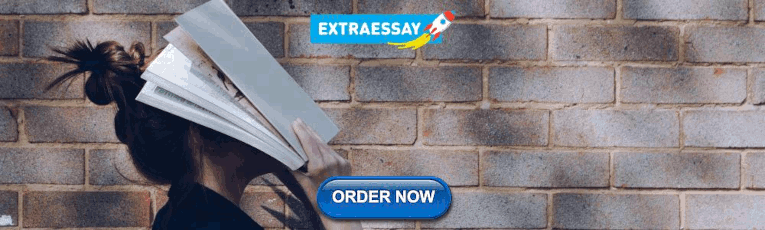
IMAGES
VIDEO
COMMENTS
ATLAS our Talent Academy for TransLAtional Science, guides and supports PhD students to conduct excellent research projects and offers comprehensive training in cutting-edge technologies.In addition, we ensure that you learn the transferable skills needed for a successful career in academia or industry. Scientific training within the program has a strong focus on "the art of translation", i.e ...
You possess professional proficiency in both written and spoken English. How to Apply. Please upload all your documents via the application portal: All mandatory documents (CV, letter of motivation, official transcript, and certificate of your master's degree, if available) must be combined into one pdf file and uploaded on the field "*Resume ...
Why you should join ATLAS. WORK WITH PIONEERS IN TRANSLATIONAL RESEARCH. A highly collaborative and interdisciplinary research environment at the interface between academia and the pharmaceutical industry. A doctoral degree from a partnering university. The opportunity to publish your work in top-tier journals.
Get to know the PhD program structure, organization, and career path from our alumni and current PhD candidates. Our ATLAS PhD program is open for applicatio...
The first ATLAS - Talent Academy for TransLAtional Science call is now open for application until January 15th, 2023. Starting in early 2023, the joint PhD program between TRON and BioNTech named ATLAS will provide a unique chance for young researchers to pursue their scientific career in the field of translational science. Within the program ...
Our Talent Academy for TransLAtional Science (ATLAS) is an international graduate program with a strong focus on translational medicine. The PhD program covers immunotheraphy, computational ...
ATLAS, the joint PhD program between TRON and BioNTech provides a unique chance for young researchers to pursue their scientific career in the field of translational science. The call is now open for application until October 31st, 2023. We are happy to offer 9 new PhD projects in this call.
Our PhD students are part of ATLAS - Talent Academy for TransLAtional Science , the joint PhD program with our longstanding cooperation partner BioNTech. The mission of ATLAS is to promote scientific excellence in a truly international research community. The program provides unique insight into our state-of the art science.
ATLAS - Joint PhD Program from BioNTech and TRON with a focus on translational medicine 12 PhD positions in biology, biochemistry, life science, chemistry, m... Career network for academics, researchers and scientists. Find and apply for jobs in research and higher education today!
Their PhD program focuses on translational medicine with experienced faculties, they provide a supportive environment for the scholars to conduct cutting-edge research projects. ATLAS is offering 9 PhD positions in biology, life science, biochemistry, chemistry, medicine, and computer science or related areas. Benefits of joining ATLAS
Our international PhD program ATLAS (Talent Academy for TransLAtional Science), guides and supports PhD students to conduct excellent research projects and offers comprehensive training in cutting-edge technologies and professional skills. The scientific training within the program has a strong focus on "the art of translation", i.e. turning ...
The annual PhD retreat will take place this year between 10th and 11th October in Frankfurt. The two-day event will be filled with inspiring scientific lectures, poster sessions, scientific presentations, and exchange between students and their supervisors. ATLAS Workshop during CURIOUS Conference. ( July 10, 2024 )
ATLAS is an international, structured, graduate program with a strong focus on translational medicine. ATLAS covers immunotherapy, computational biology and infectious diseases. ATLAS our Talent Academy for TransLAtional Science, guides and supports PhD students to conduct excellent research projects and offers comprehensive training in cutting ...
Offer Description. 9 Fully funded PhD positions in biology, biochemistry, life science, chemistry, medicine, computer science or related fields BioNTech and TRON (Translational Oncology Mainz) are leading institutions in the field of immunotherapy. Our joint international PhD Program (ATLAS) is looking for talented, junior researchers of all ...
McKenzie Ritter, PhD is the Director of the ATLAS program and the Assistant Director of Research Education and Training within the Clinical and Translational Science Collaborative at CWRU. She received her PhD in Physiology in the Spring of 2023 and has recently joined the CTSC (Summer 2023).
ATLAS - Translational Medicine at Johannes Gutenberg University Mainz is an international graduate program with a strong focus on translational medicine. ATLAS covers immunotherapy, computational biology, infectious diseases, cardiovascular, aging and regenerative biomedicine. Johannes Gutenberg University Mainz. Mainz , Germany. Top 2% worldwide.
ATLAS PhD Program. The PhD program at CU Boulder's ATLAS Institute emphasizes out-of-the-box invention and radical inquiry. Suited to students whose interests transcend traditional disciplinary boundaries, ATLAS is a thriving academic community of researchers and students who blend engineering, design, art and humanitarianism.
Most of our PhD students choose to be enrolled with the University of Mainz (JGU). This enrollment incurs semester fees of approx. €330. It comes with student benefits, such as free use of public transport in Mainz and a large region surrounding it. It further gives you subsidised access to the university´s cafeterias and sport center.
9 Laboratory of Complex Trait Genomics, Department of Computational Biology and Medical Sciences, Graduate School of Frontier Sciences, The University of Tokyo, Tokyo, Japan. 10 Laboratory for Statistical and Translational Genetics, RIKEN Center for Integrative Medical Sciences, Yokohama, Japan.
The University of Chicago Center for Translational Data Science (CTDS) has completed an update to the National Cancer Institute's Genomic Data Commons, a repository and computational platform for cancer researchers who seek to understand cancer, its clinical progression and response to therapy.This new version is known as GDC 2.0.
The authors constructed a CD4 + T cell-type resolved multimodal atlas to integrate and elucidate CD4 + T cell diversity and genetic risk of immune-mediated ... sequence motifs recognized by specific sets of transcription factor families were enriched to a different degree, ... which has hindered the translational application of GWAS studies.
Our 11th PhD Retreat (October, 2023) Not only a new record number of participants, but also the first major event since the first batch of ATLAS officially joined us: the 11th TRON & BioNTech PhD Retreat was a milestone since the establishment of the ATLAS joint PhD program. From October 11 to 13, over 150 PhD students, supervisors, and ...
Preventing Chronic Disease (PCD) is a peer-reviewed electronic journal established by the National Center for Chronic Disease Prevention and Health Promotion. PCD provides an open exchange of information and knowledge among researchers, practitioners, policy makers, and others who strive to improve the health of the public through chronic disease prevention.
Our international PhD program ATLAS (Talent Academy for TransLAtional Science), guides and supports PhD students to conduct excellent research projects and offers comprehensive training in cutting-edge technologies and professional skills. The scientific training within the program has a strong focus on "the art of translation", i.e. turning ...
Here we performed single-cell RNA sequencing analysis of 606,380 freshly isolated endothelial cells, perivascular cells and other tissue-derived cells from 117 samples, from 68 human fetuses and ...
Yıldız A, Hasani A, Hempel T, Köhl N, Beicht A, Becker R, Hubich-Rau S, Suchan M, Poleganov M, Sahin U, Beissert T. Trans-amplifying RNA expressing functional miRNA mediates target gene suppression and simultaneous transgene expression
She joined the PhD programs at Michigan State University Chemistry and Biochemistry in 2018 co-mentored by Dr Liangliang Sun in Chemistry and Dr Peter Lundquist in BMB. Her PhD research focused on combining top-down and bottom-up proteomics to study the plastoglobules (lipoprotein particles in chloroplast) proteome and remobilization under ...