Instantly share code, notes, and snippets.
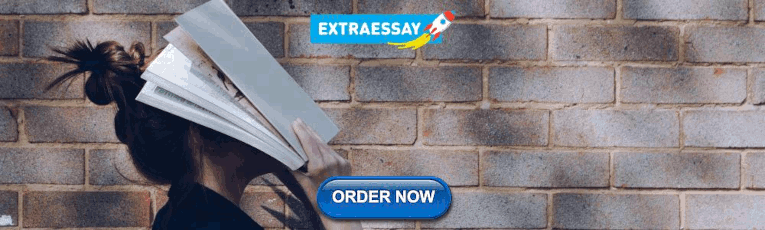
Zhefei123 / Assignment_3.py
- Download ZIP
- Star ( 0 ) 0 You must be signed in to star a gist
- Fork ( 0 ) 0 You must be signed in to fork a gist
- Embed Embed this gist in your website.
- Share Copy sharable link for this gist.
- Clone via HTTPS Clone using the web URL.
- Learn more about clone URLs
- Save Zhefei123/6342d32d8092bb23dbffaace1a6fe3a0 to your computer and use it in GitHub Desktop.
# coding: utf-8 | |
# --- | |
# | |
# _You are currently looking at **version 1.5** of this notebook. To download notebooks and datafiles, as well as get help on Jupyter notebooks in the Coursera platform, visit the [Jupyter Notebook FAQ](https://www.coursera.org/learn/python-data-analysis/resources/0dhYG) course resource._ | |
# | |
# --- | |
# # Assignment 3 - More Pandas | |
# This assignment requires more individual learning then the last one did - you are encouraged to check out the [pandas documentation](http://pandas.pydata.org/pandas-docs/stable/) to find functions or methods you might not have used yet, or ask questions on [Stack Overflow](http://stackoverflow.com/) and tag them as pandas and python related. And of course, the discussion forums are open for interaction with your peers and the course staff. | |
# ### Question 1 (20%) | |
# Load the energy data from the file `Energy Indicators.xls`, which is a list of indicators of [energy supply and renewable electricity production](Energy%20Indicators.xls) from the [United Nations](http://unstats.un.org/unsd/environment/excel_file_tables/2013/Energy%20Indicators.xls) for the year 2013, and should be put into a DataFrame with the variable name of **energy**. | |
# | |
# Keep in mind that this is an Excel file, and not a comma separated values file. Also, make sure to exclude the footer and header information from the datafile. The first two columns are unneccessary, so you should get rid of them, and you should change the column labels so that the columns are: | |
# | |
# `['Country', 'Energy Supply', 'Energy Supply per Capita', '% Renewable']` | |
# | |
# Convert `Energy Supply` to gigajoules (there are 1,000,000 gigajoules in a petajoule). For all countries which have missing data (e.g. data with "...") make sure this is reflected as `np.NaN` values. | |
# | |
# Rename the following list of countries (for use in later questions): | |
# | |
# ```"Republic of Korea": "South Korea", | |
# "United States of America": "United States", | |
# "United Kingdom of Great Britain and Northern Ireland": "United Kingdom", | |
# "China, Hong Kong Special Administrative Region": "Hong Kong"``` | |
# | |
# There are also several countries with numbers and/or parenthesis in their name. Be sure to remove these, | |
# | |
# e.g. | |
# | |
# `'Bolivia (Plurinational State of)'` should be `'Bolivia'`, | |
# | |
# `'Switzerland17'` should be `'Switzerland'`. | |
# | |
# <br> | |
# | |
# Next, load the GDP data from the file `world_bank.csv`, which is a csv containing countries' GDP from 1960 to 2015 from [World Bank](http://data.worldbank.org/indicator/NY.GDP.MKTP.CD). Call this DataFrame **GDP**. | |
# | |
# Make sure to skip the header, and rename the following list of countries: | |
# | |
# ```"Korea, Rep.": "South Korea", | |
# "Iran, Islamic Rep.": "Iran", | |
# "Hong Kong SAR, China": "Hong Kong"``` | |
# | |
# <br> | |
# | |
# Finally, load the [Sciamgo Journal and Country Rank data for Energy Engineering and Power Technology](http://www.scimagojr.com/countryrank.php?category=2102) from the file `scimagojr-3.xlsx`, which ranks countries based on their journal contributions in the aforementioned area. Call this DataFrame **ScimEn**. | |
# | |
# Join the three datasets: GDP, Energy, and ScimEn into a new dataset (using the intersection of country names). Use only the last 10 years (2006-2015) of GDP data and only the top 15 countries by Scimagojr 'Rank' (Rank 1 through 15). | |
# | |
# The index of this DataFrame should be the name of the country, and the columns should be ['Rank', 'Documents', 'Citable documents', 'Citations', 'Self-citations', | |
# 'Citations per document', 'H index', 'Energy Supply', | |
# 'Energy Supply per Capita', '% Renewable', '2006', '2007', '2008', | |
# '2009', '2010', '2011', '2012', '2013', '2014', '2015']. | |
# | |
# *This function should return a DataFrame with 20 columns and 15 entries.* | |
# In[44]: | |
import pandas as pd | |
import numpy as np | |
def answer_one(): | |
# DELETE ROWS COLUMNS | |
energy = pd.read_excel('Energy Indicators.xls') | |
energy = energy.iloc[16:243,2:] | |
energy.columns = ['Country', 'Energy Supply', 'Energy Supply per Capita', '% Renewable'] | |
# REPLACE | |
energy.replace('...',np.nan, inplace = True) | |
energy['Energy Supply']= energy['Energy Supply']*1000000 | |
energy['Country']= energy['Country'].str.replace(r'\(.*\)','') | |
energy['Country']=energy['Country'].str.replace('[0-9()]+$','') | |
energy.replace('Republic of Korea', 'South Korea', inplace = True) | |
energy.replace('Iran ', 'Iran', inplace = True) | |
energy.replace('United States of America','United States', inplace = True) | |
energy.replace("United Kingdom of Great Britain and Northern Ireland", "United Kingdom", inplace = True) | |
energy.replace("China, Hong Kong Special Administrative Region", "Hong Kong", inplace = True) | |
##GDP DATA### | |
GDP = pd.read_csv('world_bank.csv') | |
GDP.columns = (GDP.iloc[3,:].values[0:4].astype(str).tolist())+ (GDP.iloc[3,:].values[4:].astype(int).tolist()) | |
GDP = GDP.iloc[4:, :] | |
GDP = GDP[['Country Name', 2006,2007,2008,2009,2010,2011,2012,2013,2014,2015]] | |
GDP.columns=['Country', '2006', '2007', '2008', '2009', '2010', '2011', '2012', '2013', '2014', '2015'] | |
GDP['Country']= GDP['Country'].str.replace(r'\(.*\)','') | |
GDP['Country']=GDP['Country'].str.replace('[0-9()]+$','') | |
GDP.replace("Korea, Rep.", "South Korea",inplace = True) | |
GDP.replace("Iran, Islamic Rep.", "Iran",inplace = True) | |
GDP.replace("Hong Kong SAR, China", "Hong Kong",inplace = True) | |
#ScimEn## | |
ScimEn = pd.read_excel('scimagojr-3.xlsx') | |
#merge# | |
alldata = pd.merge(pd.merge(energy, GDP,how = 'outer', on = 'Country'), ScimEn,how = 'outer',on = 'Country') | |
data = alldata.sort_values('Rank').head(15) | |
data.set_index('Country',inplace= True) | |
data= data[['Rank', 'Documents', 'Citable documents', 'Citations', 'Self-citations', 'Citations per document', 'H index', 'Energy Supply', 'Energy Supply per Capita', '% Renewable', '2006', '2007', '2008', '2009', '2010', '2011', '2012', '2013', '2014', '2015']] | |
return data | |
answer_one() | |
# ### Question 2 (6.6%) | |
# The previous question joined three datasets then reduced this to just the top 15 entries. When you joined the datasets, but before you reduced this to the top 15 items, how many entries did you lose? | |
# | |
# *This function should return a single number.* | |
# In[20]: | |
get_ipython().run_cell_magic('HTML', '', '<svg width="800" height="300">\n <circle cx="150" cy="180" r="80" fill-opacity="0.2" stroke="black" stroke-width="2" fill="blue" />\n <circle cx="200" cy="100" r="80" fill-opacity="0.2" stroke="black" stroke-width="2" fill="red" />\n <circle cx="100" cy="100" r="80" fill-opacity="0.2" stroke="black" stroke-width="2" fill="green" />\n <line x1="150" y1="125" x2="300" y2="150" stroke="black" stroke-width="2" fill="black" stroke-dasharray="5,3"/>\n <text x="300" y="165" font-family="Verdana" font-size="35">Everything but this!</text>\n</svg>') | |
# In[45]: | |
def answer_two(): | |
alldata = pd.merge(pd.merge(energy, GDP,how = 'outer', on = 'Country'), ScimEn,how = 'outer',on = 'Country') | |
intersect = pd.merge(pd.merge(energy, GDP,how = 'inner', on = 'Country'), ScimEn,how = 'inner',on = 'Country') | |
a = len(alldata)-len(intersect) | |
return a | |
answer_two() | |
# ## Answer the following questions in the context of only the top 15 countries by Scimagojr Rank (aka the DataFrame returned by `answer_one()`) | |
# ### Question 3 (6.6%) | |
# What is the average GDP over the last 10 years for each country? (exclude missing values from this calculation.) | |
# | |
# *This function should return a Series named `avgGDP` with 15 countries and their average GDP sorted in descending order.* | |
# In[24]: | |
def answer_three(): | |
Top15 = answer_one() | |
columns = ['2006', '2007', '2008', '2009', '2010', '2011', '2012', '2013', '2014', '2015'] | |
Top15['aveGDP'] =Top15.apply(lambda x: np.average(x[columns]), axis =1) | |
Top15.sort_values('aveGDP', ascending= False)['aveGDP'] | |
return pd.Series(Top15['aveGDP']) | |
answer_three() | |
# ### Question 4 (6.6%) | |
# By how much had the GDP changed over the 10 year span for the country with the 6th largest average GDP? | |
# | |
# *This function should return a single number.* | |
# In[25]: | |
def answer_four(): | |
Top15 = answer_one() | |
columns = ['2006', '2007', '2008', '2009', '2010', '2011', '2012', '2013', '2014', '2015'] | |
Top15['aveGDP'] = Top15.apply(lambda x: np.average(x[columns]),axis=1) | |
Top15['end-start'] = Top15.apply(lambda x: (x['2015']-x['2006']),axis=1) | |
return Top15.sort_values('aveGDP', ascending= False).iloc[5,-1] | |
answer_four() | |
# ### Question 5 (6.6%) | |
# What is the mean `Energy Supply per Capita`? | |
# | |
# *This function should return a single number.* | |
# In[70]: | |
def answer_five(): | |
Top15 = answer_one() | |
a = Top15['Energy Supply per Capita'].mean() | |
return a | |
answer_five() | |
# ### Question 6 (6.6%) | |
# What country has the maximum % Renewable and what is the percentage? | |
# | |
# *This function should return a tuple with the name of the country and the percentage.* | |
# In[36]: | |
def answer_six(): | |
Top15 = answer_one() | |
maxR = Top15.sort_values('% Renewable',ascending= False).reset_index().iloc[0,:] | |
return (maxR['Country'], maxR['% Renewable']) | |
answer_six() | |
# ### Question 7 (6.6%) | |
# Create a new column that is the ratio of Self-Citations to Total Citations. | |
# What is the maximum value for this new column, and what country has the highest ratio? | |
# | |
# *This function should return a tuple with the name of the country and the ratio.* | |
# In[35]: | |
def answer_seven(): | |
Top15 = answer_one() | |
Top15['ratioCitation'] = Top15['Self-citations']/Top15['Citations'] | |
maxRatio = Top15.sort_values('ratioCitation',ascending= False).reset_index().iloc[0,:] | |
return (maxRatio['Country'], maxRatio['ratioCitation']) | |
answer_seven() | |
# ### Question 8 (6.6%) | |
# | |
# Create a column that estimates the population using Energy Supply and Energy Supply per capita. | |
# What is the third most populous country according to this estimate? | |
# | |
# *This function should return a single string value.* | |
# In[37]: | |
def answer_eight(): | |
Top15 = answer_one() | |
Top15['pop'] = Top15['Energy Supply']/Top15['Energy Supply per Capita'] | |
thirdP = Top15.sort_values('pop',ascending= False).reset_index().iloc[2,:]['Country'] | |
return thirdP | |
answer_eight() | |
# ### Question 9 (6.6%) | |
# Create a column that estimates the number of citable documents per person. | |
# What is the correlation between the number of citable documents per capita and the energy supply per capita? Use the `.corr()` method, (Pearson's correlation). | |
# | |
# *This function should return a single number.* | |
# | |
# *(Optional: Use the built-in function `plot9()` to visualize the relationship between Energy Supply per Capita vs. Citable docs per Capita)* | |
# In[75]: | |
def answer_nine(): | |
Top15 = answer_one() | |
Top15['Citable docs per Capita'] = Top15['Citable documents']/(Top15['Energy Supply']/Top15['Energy Supply per Capita']) | |
correlation = Top15[['Energy Supply per Capita','Citable docs per Capita']].corr(method='pearson').iloc[0,1] | |
return correlation | |
answer_nine() | |
# def plot9(): | |
# import matplotlib as plt | |
# %matplotlib inline | |
# | |
# Top15 = answer_one() | |
# Top15['PopEst'] = Top15['Energy Supply'] / Top15['Energy Supply per Capita'] | |
# Top15['Citable docs per Capita'] = Top15['Citable documents'] / Top15['PopEst'] | |
# Top15.plot(x='Citable docs per Capita', y='Energy Supply per Capita', kind='scatter', xlim=[0, 0.0006]) | |
# plot9() | |
# In[ ]: | |
#plot9() # Be sure to comment out plot9() before submitting the assignment! | |
# ### Question 10 (6.6%) | |
# Create a new column with a 1 if the country's % Renewable value is at or above the median for all countries in the top 15, and a 0 if the country's % Renewable value is below the median. | |
# | |
# *This function should return a series named `HighRenew` whose index is the country name sorted in ascending order of rank.* | |
# In[38]: | |
def answer_ten(): | |
Top15 = answer_one() | |
MedianRenew = Top15['% Renewable'].median() | |
Top15['HighRenew']= (Top15['% Renewable'] >= MedianRenew)*1 | |
return Top15.loc[:,'HighRenew'] | |
answer_ten() | |
# ### Question 11 (6.6%) | |
# Use the following dictionary to group the Countries by Continent, then create a dateframe that displays the sample size (the number of countries in each continent bin), and the sum, mean, and std deviation for the estimated population of each country. | |
# | |
# ```python | |
# ContinentDict = {'China':'Asia', | |
# 'United States':'North America', | |
# 'Japan':'Asia', | |
# 'United Kingdom':'Europe', | |
# 'Russian Federation':'Europe', | |
# 'Canada':'North America', | |
# 'Germany':'Europe', | |
# 'India':'Asia', | |
# 'France':'Europe', | |
# 'South Korea':'Asia', | |
# 'Italy':'Europe', | |
# 'Spain':'Europe', | |
# 'Iran':'Asia', | |
# 'Australia':'Australia', | |
# 'Brazil':'South America'} | |
# ``` | |
# | |
# *This function should return a DataFrame with index named Continent `['Asia', 'Australia', 'Europe', 'North America', 'South America']` and columns `['size', 'sum', 'mean', 'std']`* | |
# In[39]: | |
ContinentDict = {'China':'Asia', | |
'United States':'North America', | |
'Japan':'Asia', | |
'United Kingdom':'Europe', | |
'Russian Federation':'Europe', | |
'Canada':'North America', | |
'Germany':'Europe', | |
'India':'Asia', | |
'France':'Europe', | |
'South Korea':'Asia', | |
'Italy':'Europe', | |
'Spain':'Europe', | |
'Iran':'Asia', | |
'Australia':'Australia', | |
'Brazil':'South America'} | |
def answer_eleven(): | |
Top15 = answer_one().reset_index() | |
Top15['Continent'] = Top15['Country'].map(ContinentDict) | |
Top15['pop'] = Top15['Energy Supply']/Top15['Energy Supply per Capita'] | |
df = Top15.set_index('Continent').groupby(level=0)['pop'].agg({'size': len, 'sum':np.sum,'mean':np.mean,'std':np.std}) | |
return df | |
answer_eleven() | |
# ### Question 12 (6.6%) | |
# Cut % Renewable into 5 bins. Group Top15 by the Continent, as well as these new % Renewable bins. How many countries are in each of these groups? | |
# | |
# *This function should return a __Series__ with a MultiIndex of `Continent`, then the bins for `% Renewable`. Do not include groups with no countries.* | |
# In[43]: | |
def answer_twelve(): | |
Top15 = answer_one().reset_index() | |
Top15['Continent'] = Top15['Country'].map(ContinentDict) | |
Top15['bins for % Renewable']= pd.cut(Top15['% Renewable'],5) | |
return Top15.groupby(['Continent','bins for % Renewable']).size() | |
answer_twelve() | |
# ### Question 13 (6.6%) | |
# Convert the Population Estimate series to a string with thousands separator (using commas). Do not round the results. | |
# | |
# e.g. 317615384.61538464 -> 317,615,384.61538464 | |
# | |
# *This function should return a Series `PopEst` whose index is the country name and whose values are the population estimate string.* | |
# In[42]: | |
def answer_thirteen(): | |
Top15 = answer_one() | |
Top15['PopEst'] = (Top15['Energy Supply']/Top15['Energy Supply per Capita']).apply(lambda x: '{:,}'.format(x)) | |
return Top15['PopEst'] | |
answer_thirteen() |
Introduction to Data Science with Python
Arvind Krishna, Lizhen Shi, Emre Besler, and Arend Kuyper
September 20, 2022
This book is developed for the course STAT303-1 (Data Science with Python-1). The first two chapters of the book are a review of python, and will be covered very quickly. Students are expected to know the contents of these chapters beforehand, or be willing to learn it quickly. Students may use the STAT201 book (https://nustat.github.io/Intro_to_programming_for_data_sci/) to review the python basics required for the STAT303 sequence. The core part of the course begins from the third chapter - Reading data .
Please feel free to let the instructors know in case of any typos/mistakes/general feedback in this book.
- Data Structures
- Linked List
- Binary Tree
- Binary Search Tree
- Segment Tree
- Disjoint Set Union
- Fenwick Tree
- Red-Black Tree
- Advanced Data Structures
- Hashing in Data Structure
Introduction to Hashing
What is hashing.
- Index Mapping (or Trivial Hashing) with negatives allowed
- Separate Chaining Collision Handling Technique in Hashing
- Open Addressing Collision Handling technique in Hashing
- Double Hashing
- Load Factor and Rehashing
Easy problems on Hashing
- Find whether an array is subset of another array
- Union and Intersection of two Linked List using Hashing
- Pair with given Sum (Two Sum)
- Maximum distance between two occurrences of same element in array
- Most frequent element in an array
- Find the only repetitive element between 1 to N-1
- How to check if two given sets are disjoint?
- Non-overlapping sum of two sets
- Check if two arrays are equal or not
- Find missing elements of a range
- Minimum number of subsets with distinct elements
- Remove minimum number of elements such that no common element exist in both array
- Count pairs with given sum
- Count quadruples from four sorted arrays whose sum is equal to a given value x
- Sort elements by frequency | Set 4 (Efficient approach using hash)
- Find all pairs (a, b) in an array such that a % b = k
- Group words with same set of characters
- k-th distinct (or non-repeating) element among unique elements in an array.
Intermediate problems on Hashing
- Find Itinerary from a given list of tickets
- Find number of Employees Under every Manager
- Longest subarray with sum divisible by K
- Find the length of largest subarray with 0 sum
- Longest Increasing consecutive subsequence
- Count distinct elements in every window of size k
- Design a data structure that supports insert, delete, search and getRandom in constant time
- Find subarray with given sum | Set 2 (Handles Negative Numbers)
- Implementing our Own Hash Table with Separate Chaining in Java
- Implementing own Hash Table with Open Addressing Linear Probing
- Maximum possible difference of two subsets of an array
- Sorting using trivial hash function
- Smallest subarray with k distinct numbers
Hard problems on Hashing
- Clone a Binary Tree with Random Pointers
- Largest subarray with equal number of 0s and 1s
- All unique triplets that sum up to a given value
- Range Queries for Frequencies of array elements
- Elements to be added so that all elements of a range are present in array
- Cuckoo Hashing - Worst case O(1) Lookup!
- Count subarrays having total distinct elements same as original array
- Maximum array from two given arrays keeping order same
- Find Sum of all unique sub-array sum for a given array.
- Length of longest strict bitonic subsequence
- Find All Duplicate Subtrees
- Find if there is a rectangle in binary matrix with corners as 1
- Top 20 Hashing Technique based Interview Questions
Hashing refers to the process of generating a fixed-size output from an input of variable size using the mathematical formulas known as hash functions. This technique determines an index or location for the storage of an item in a data structure.
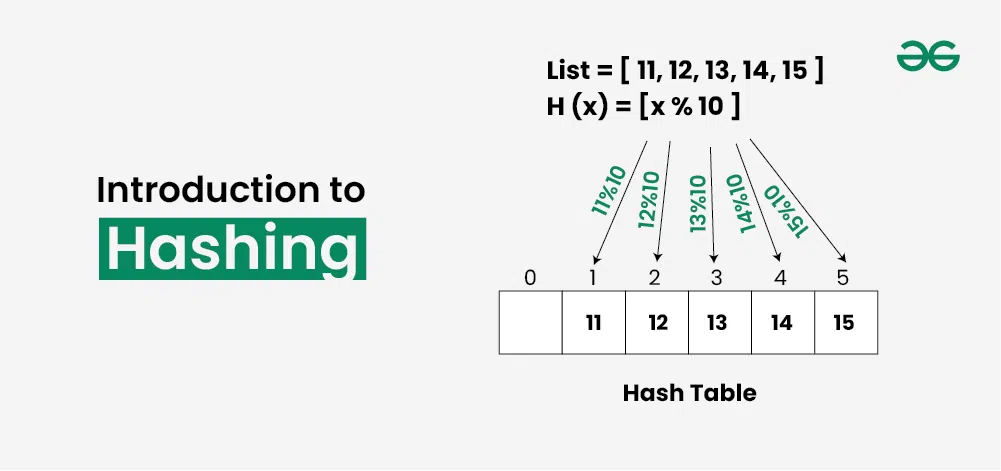
Table of Content
Need for Hash data structure
Components of hashing, how does hashing work, what is a hash function.
- Types of Hash functions
Properties of a Good hash function
Complexity of calculating hash value using the hash function, problem with hashing, what is collision, how to handle collisions.
- Separate Chaining
- Linear Probing
- Quadratic Probing
What is meant by Load Factor in Hashing?
What is rehashing, applications of hash data structure, real-time applications of hash data structure, advantages of hash data structure, disadvantages of hash data structure.
- Frequently Asked Questions(FAQs) on Hashing
Hashing in Data Structures refers to the process of transforming a given key to another value. It involves mapping data to a specific index in a hash table using a hash function that enables fast retrieval of information based on its key. The transformation of a key to the corresponding value is done using a Hash Function and the value obtained from the hash function is called Hash Code .
Every day, the data on the internet is increasing multifold and it is always a struggle to store this data efficiently. In day-to-day programming, this amount of data might not be that big, but still, it needs to be stored, accessed, and processed easily and efficiently. A very common data structure that is used for such a purpose is the Array data structure.
Now the question arises if Array was already there, what was the need for a new data structure! The answer to this is in the word ” efficiency “. Though storing in Array takes O(1) time, searching in it takes at least O(log n) time. This time appears to be small, but for a large data set, it can cause a lot of problems and this, in turn, makes the Array data structure inefficient.
So now we are looking for a data structure that can store the data and search in it in constant time, i.e. in O(1) time. This is how Hashing data structure came into play. With the introduction of the Hash data structure, it is now possible to easily store data in constant time and retrieve them in constant time as well.
There are majorly three components of hashing:
- Key: A Key can be anything string or integer which is fed as input in the hash function the technique that determines an index or location for storage of an item in a data structure.
- Hash Function: The hash function receives the input key and returns the index of an element in an array called a hash table. The index is known as the hash index .
- Hash Table: Hash table is a data structure that maps keys to values using a special function called a hash function. Hash stores the data in an associative manner in an array where each data value has its own unique index.
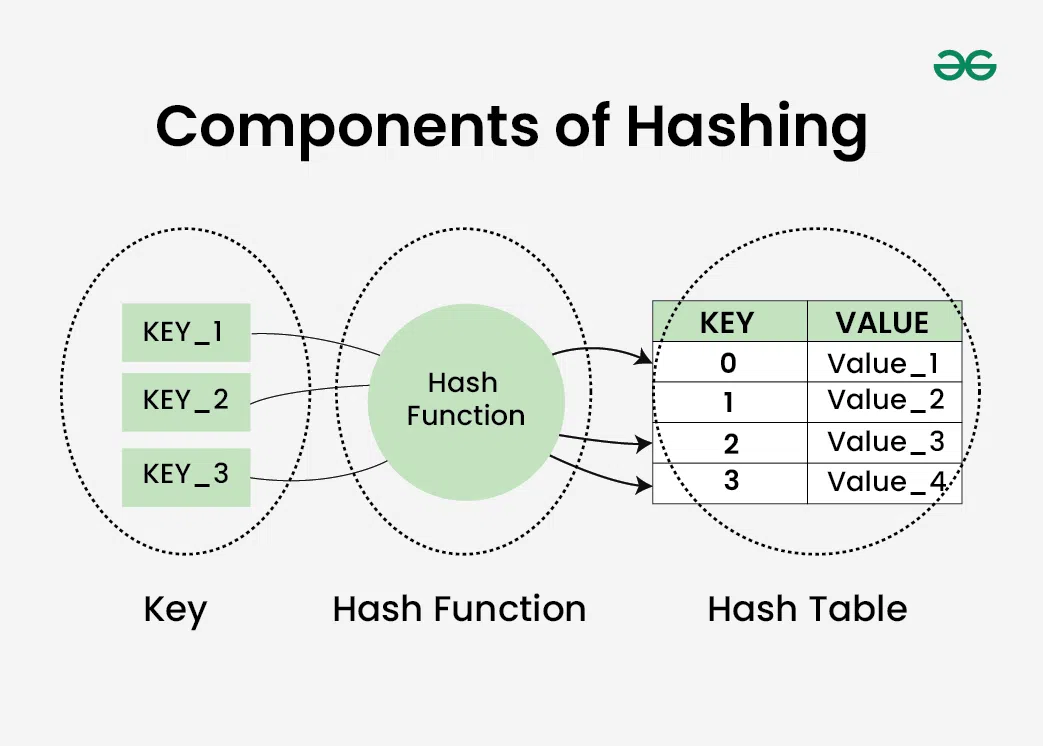
Suppose we have a set of strings {“ab”, “cd”, “efg”} and we would like to store it in a table.
Our main objective here is to search or update the values stored in the table quickly in O(1) time and we are not concerned about the ordering of strings in the table. So the given set of strings can act as a key and the string itself will act as the value of the string but how to store the value corresponding to the key?
- Step 1: We know that hash functions (which is some mathematical formula) are used to calculate the hash value which acts as the index of the data structure where the value will be stored.
- “b”=2, .. etc, to all alphabetical characters.
- Step 3: Therefore, the numerical value by summation of all characters of the string:
“ab” = 1 + 2 = 3, “cd” = 3 + 4 = 7 , “efg” = 5 + 6 + 7 = 18
- Step 4: Now, assume that we have a table of size 7 to store these strings. The hash function that is used here is the sum of the characters in key mod Table size . We can compute the location of the string in the array by taking the sum(string) mod 7 .
- “ab” in 3 mod 7 = 3,
- “cd” in 7 mod 7 = 0, and
- “efg” in 18 mod 7 = 4.
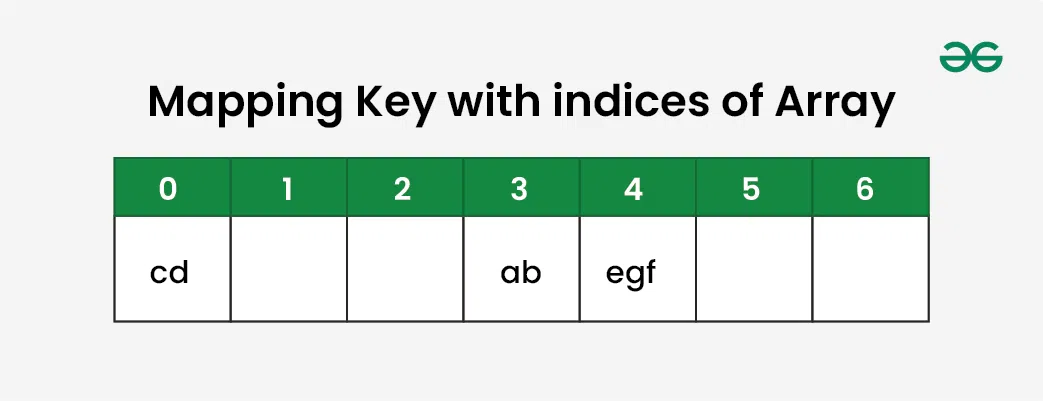
The above technique enables us to calculate the location of a given string by using a simple hash function and rapidly find the value that is stored in that location. Therefore the idea of hashing seems like a great way to store (key, value) pairs of the data in a table.
The hash function creates a mapping between key and value, this is done through the use of mathematical formulas known as hash functions. The result of the hash function is referred to as a hash value or hash. The hash value is a representation of the original string of characters but usually smaller than the original.
For example: Consider an array as a Map where the key is the index and the value is the value at that index. So for an array A if we have index i which will be treated as the key then we can find the value by simply looking at the value at A[i].
Types of Hash functions:
There are many hash functions that use numeric or alphanumeric keys. This article focuses on discussing different hash functions :
- Division Method.
- Mid Square Method
- Folding Method.
- Multiplication Method
A hash function that maps every item into its own unique slot is known as a perfect hash function. We can construct a perfect hash function if we know the items and the collection will never change but the problem is that there is no systematic way to construct a perfect hash function given an arbitrary collection of items. Fortunately, we will still gain performance efficiency even if the hash function isn’t perfect. We can achieve a perfect hash function by increasing the size of the hash table so that every possible value can be accommodated. As a result, each item will have a unique slot. Although this approach is feasible for a small number of items, it is not practical when the number of possibilities is large.
So, We can construct our hash function to do the same but the things that we must be careful about while constructing our own hash function.
A good hash function should have the following properties:
- Efficiently computable.
- Should uniformly distribute the keys (Each table position is equally likely for each.
- Should minimize collisions.
- Should have a low load factor(number of items in the table divided by the size of the table).
- Time complexity: O(n)
- Space complexity: O(1)
If we consider the above example, the hash function we used is the sum of the letters, but if we examined the hash function closely then the problem can be easily visualized that for different strings same hash value is begin generated by the hash function.
For example: {“ab”, “ba”} both have the same hash value, and string {“cd”,”be”} also generate the same hash value, etc. This is known as collision and it creates problem in searching, insertion, deletion, and updating of value.
Collision in Hashing occurs when two different keys map to the same hash value. Hash collisions can be intentionally created for many hash algorithms. The probability of a hash collision depends on the size of the algorithm, the distribution of hash values and the efficiency of Hash function.
The hashing process generates a small number for a big key, so there is a possibility that two keys could produce the same value. The situation where the newly inserted key maps to an already occupied, and it must be handled using some collision handling technology.
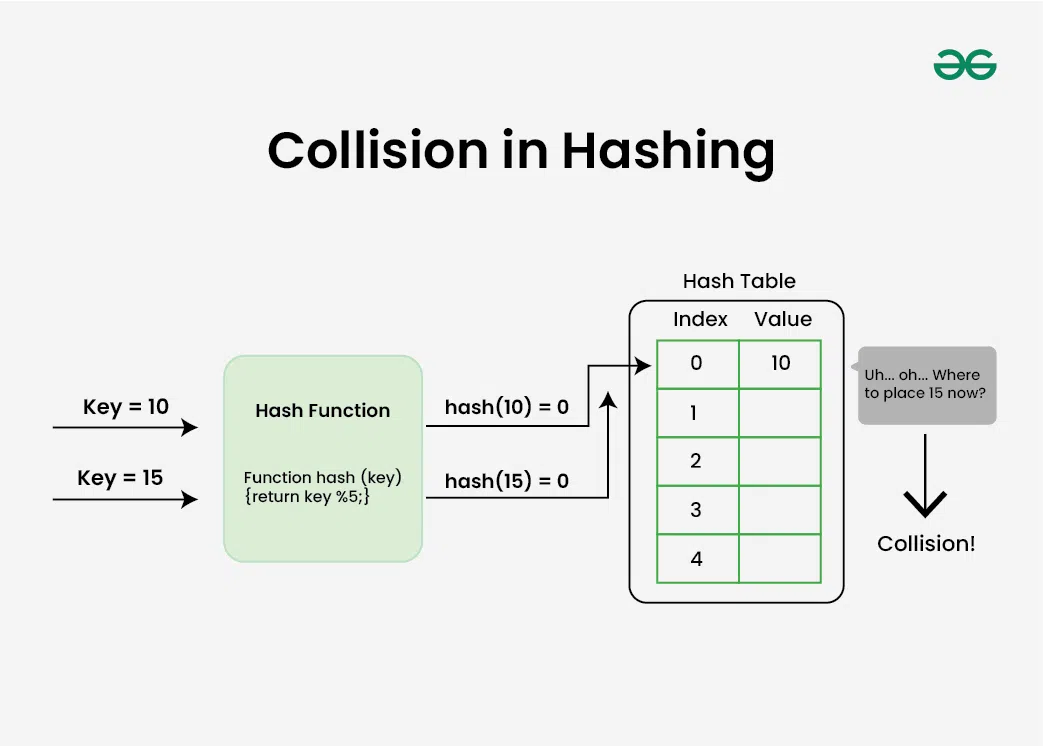
There are mainly two methods to handle collision:
- Open Addressing
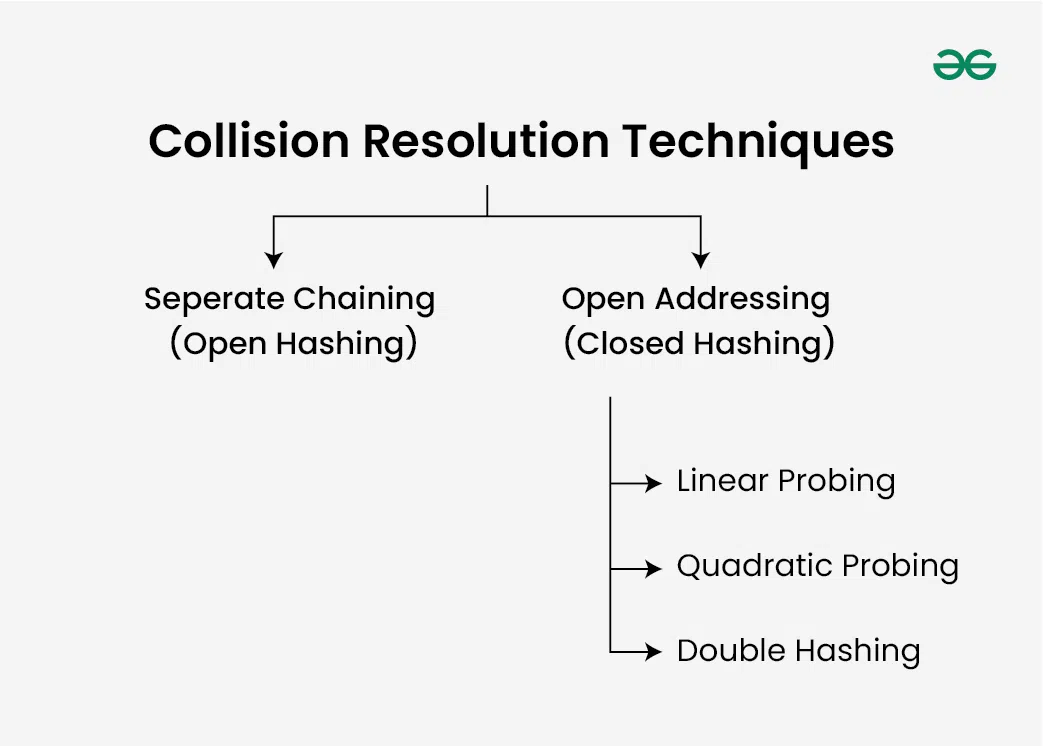
1) Separate Chaining
The idea is to make each cell of the hash table point to a linked list of records that have the same hash function value. Chaining is simple but requires additional memory outside the table.
Example: We have given a hash function and we have to insert some elements in the hash table using a separate chaining method for collision resolution technique.
Let’s see step by step approach to how to solve the above problem:
Hence In this way, the separate chaining method is used as the collision resolution technique.
2) Open Addressing
In open addressing, all elements are stored in the hash table itself. Each table entry contains either a record or NIL. When searching for an element, we examine the table slots one by one until the desired element is found or it is clear that the element is not in the table.
2.a) Linear Probing
In linear probing, the hash table is searched sequentially that starts from the original location of the hash. If in case the location that we get is already occupied, then we check for the next location.
Calculate the hash key. i.e. key = data % size Check, if hashTable[key] is empty store the value directly by hashTable[key] = data If the hash index already has some value then check for next index using key = (key+1) % size Check, if the next index is available hashTable[key] then store the value. Otherwise try for next index. Do the above process till we find the space.
Example: Let us consider a simple hash function as “key mod 5” and a sequence of keys that are to be inserted are 50, 70, 76, 85, 93.
2.b) Quadratic Probing
Quadratic probing is an open addressing scheme in computer programming for resolving hash collisions in hash tables. Quadratic probing operates by taking the original hash index and adding successive values of an arbitrary quadratic polynomial until an open slot is found.
An example sequence using quadratic probing is:
H + 1 2 , H + 2 2 , H + 3 2 , H + 4 2 …………………. H + k 2
This method is also known as the mid-square method because in this method we look for i 2 ‘th probe (slot) in i’th iteration and the value of i = 0, 1, . . . n – 1. We always start from the original hash location. If only the location is occupied then we check the other slots.
Let hash(x) be the slot index computed using the hash function and n be the size of the hash table.
If the slot hash(x) % n is full, then we try (hash(x) + 1 2 ) % n. If (hash(x) + 1 2 ) % n is also full, then we try (hash(x) + 2 2 ) % n. If (hash(x) + 2 2 ) % n is also full, then we try (hash(x) + 3 2 ) % n. This process will be repeated for all the values of i until an empty slot is found
Example: Let us consider table Size = 7, hash function as Hash(x) = x % 7 and collision resolution strategy to be f(i) = i 2 . Insert = 22, 30, and 50
2.c) Double Hashing
Double hashing is a collision resolving technique in Open Addressed Hash tables. Double hashing make use of two hash function,
- The first hash function is h1(k) which takes the key and gives out a location on the hash table. But if the new location is not occupied or empty then we can easily place our key.
- But in case the location is occupied (collision) we will use secondary hash-function h2(k) in combination with the first hash-function h1(k) to find the new location on the hash table.
This combination of hash functions is of the form
- i is a non-negative integer that indicates a collision number,
- k = element/key which is being hashed
- n = hash table size.
Complexity of the Double hashing algorithm:
Example: Insert the keys 27, 43, 692, 72 into the Hash Table of size 7. where first hash-function is h1(k) = k mod 7 and second hash-function is h2(k) = 1 + (k mod 5)
The load factor of the hash table can be defined as the number of items the hash table contains divided by the size of the hash table. Load factor is the decisive parameter that is used when we want to rehash the previous hash function or want to add more elements to the existing hash table.
It helps us in determining the efficiency of the hash function i.e. it tells whether the hash function which we are using is distributing the keys uniformly or not in the hash table.
As the name suggests, rehashing means hashing again. Basically, when the load factor increases to more than its predefined value (the default value of the load factor is 0.75), the complexity increases. So to overcome this, the size of the array is increased (doubled) and all the values are hashed again and stored in the new double-sized array to maintain a low load factor and low complexity.
- Hash is used in databases for indexing.
- Hash is used in disk-based data structures.
- In some programming languages like Python, JavaScript hash is used to implement objects.
- Hash is used for cache mapping for fast access to the data.
- Hash can be used for password verification.
- Hash is used in cryptography as a message digest.
- Rabin-Karp algorithm for pattern matching in a string.
- Calculating the number of different substrings of a string.
- Hash provides better synchronization than other data structures.
- Hash tables are more efficient than search trees or other data structures
- Hash provides constant time for searching, insertion, and deletion operations on average.
- Hash is inefficient when there are many collisions.
- Hash collisions are practically not avoided for a large set of possible keys.
- Hash does not allow null values.
Frequently Asked Questions(FAQs) on Hashing:
1. what is a hash function.
Hashing refers to the process of transforming a given key to another value. It involves mapping data to a specific index in a hash table using a hash function that enables fast retrieval of information based on its key.
2. What is a Hash function?
Hash function is a function that takes an input and return a fixed-size string of bytes. The hash function receives the input key and returns the index of an element in an array called a hash table. The index is known as the hash index.
3. What are Hash collisions?
Hash collisions occur when two different inputs passed to the hash function produce the same hash value. The lesser the number of hash collisions, the better the hash function is.
4. What are hash tables?
Hash tables are data structures that use hash functions to map keys to values, allowing for efficient retrieval of data when needed. Hash table maps keys to values using a special function called a hash function. Hash stores the data in an associative manner in an array where each data value has its own unique index.
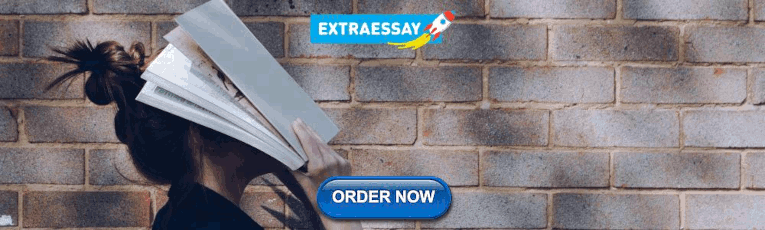
5. What are some applications of Hashing?
Hashing is used in databases for indexing, disk-based data structures and data compression algorithms. Hashing is also used to store passwords securely by applying a hash function to the password and storing the hashed result, rather than the plain text password.
From the above discussion, we conclude that the goal of hashing is to resolve the challenge of finding an item quickly in a collection. For example, if we have a list of millions of English words and we wish to find a particular term then we would use hashing to locate and find it more efficiently. It would be inefficient to check each item on the millions of lists until we find a match. Hashing reduces search time by restricting the search to a smaller set of words at the beginning.
Please Login to comment...
Similar reads.
- DSA Tutorials
Improve your Coding Skills with Practice
What kind of Experience do you want to share?
- Stack Overflow for Teams Where developers & technologists share private knowledge with coworkers
- Advertising & Talent Reach devs & technologists worldwide about your product, service or employer brand
- OverflowAI GenAI features for Teams
- OverflowAPI Train & fine-tune LLMs
- Labs The future of collective knowledge sharing
- About the company Visit the blog
Collectives™ on Stack Overflow
Find centralized, trusted content and collaborate around the technologies you use most.
Q&A for work
Connect and share knowledge within a single location that is structured and easy to search.
Get early access and see previews of new features.
Coursera Course - Introduction of Data Science in Python Assignment 1
I'm taking this course on Coursera, and I'm running some issues while doing the first assignment. The task is to basically use regular expression to get certain values from the given file. Then, the function should output a dictionary containing these values:
This is just a screenshot of the file. Due to some reasons, the link doesn't work if it's not open directly from Coursera. I apologize in advance for the bad formatting. One thing I must point out is that for some cases, as you can see in the first example, there's no username. Instead '-' is used.
This is what I currently have right now. However, the output is None. I guess there's something wrong in my pattern.

2 Answers 2
You can use the following expression:
See the regex demo . See the Python demo :

- Thank you so much!!! It worked!!! However, may I just ask a question regarding your solution? It probably sounds stupid, but don't you need to include everything in the parenthesis? For example, ("?P<request>[^"]*"). Or are they the same? Also, may you please explain the meaning of "?:" in your regular expression – BryantHsiung Commented Oct 19, 2020 at 13:20
- @BryantHsiung You can't use ("?P<request>[^"]*") , it is an invalid regex construct. See more about non-capturing groups here . – Wiktor Stribiżew Commented Oct 19, 2020 at 13:42
- 1 Just did! Thanks again! – BryantHsiung Commented Oct 20, 2020 at 12:42
- 1 I am working on the same question but I don't know why my for loop doesn't give me an output! I check my regex pattern on regex101 and they are all seem to be working the way they should. – Anoushiravan R Commented Jan 9, 2022 at 20:22
- @AnoushiravanR Without seeing your code, I can't help. – Wiktor Stribiżew Commented Jan 9, 2022 at 20:29
Check using following code:
For more information regarding regex, read the following documentation, it would be very useful for beginners: https://docs.python.org/3/library/re.html#module-re
Your Answer
Reminder: Answers generated by artificial intelligence tools are not allowed on Stack Overflow. Learn more
Sign up or log in
Post as a guest.
Required, but never shown
By clicking “Post Your Answer”, you agree to our terms of service and acknowledge you have read our privacy policy .
Not the answer you're looking for? Browse other questions tagged python regex or ask your own question .
- The Overflow Blog
- Community Products Roadmap Update, July 2024
- Featured on Meta
- We spent a sprint addressing your requests — here’s how it went
- Upcoming initiatives on Stack Overflow and across the Stack Exchange network...
- Policy: Generative AI (e.g., ChatGPT) is banned
- The [lib] tag is being burninated
- What makes a homepage useful for logged-in users
Hot Network Questions
- Airtight beaks?
- What type of interaction in a π-complex?
- Don't make noise. OR Don't make a noise
- Can the US president kill at will?
- Pregnancy in a hibernated state
- Hourly pay rate calculation between Recruiting and Payroll Systems
- Why does independent research from people without formal academic qualifications generally turn out to be a complete waste of time?
- Spec sheet on Shimano FC-C201 seemingly does not match my bike
- Can you be charged with breaking and entering if the door was open, and the owner of the property is deceased?
- In-Place Reordering of Doubly Linked List Nodes to Ensure Memory Contiguity
- Sci-fi book series about a teenage detective, possibly named Diamond, with a floating AI assistant
- Cliffhanger ending?
- Why didn't Jimmy Neutron realize immediately when he read the note on the refrigerator that the note is phony, as the note says "son or daughter..."?
- Inconsistent result for NUMA node memory usage in SQL Server
- What is the purpose of the BJT in this circuit?
- What is a trillesti?
- What is the "closest approximation" to fields by an equational variety?
- Is there a generalization of factoring that can be extended to the Real numbers?
- 为什么字形不同的两个字「骨」的编码相同(从而是一个字)?
- How to manage talkover in meetings?
- Why do some JFETs oscillate and others don't?
- Strange Interaction with Professor
- tikzpicture - How to overlay a matrix of images with text
- Shimano grip shifter too hard for son
GSI - QMSS 201 (Fall 2024)
How to apply.
Applicants must submit a cover letter, curriculum vitae (CV) or resume, copies of previous teaching evaluations (if applicable), and a copy of your unofficial transcript(s) from current and/or previous institutions that showcase your current and previous coursework in social sciences and/or quantitative methods. In your cover letter, please explain why you would like to GSI for QMSS (including which course(s), if applicable) and the skills (e.g., analytical tools such as Excel, R, Tableau, Python, etc.) and experiences (e.g., jobs, internships, research, coursework, teaching, etc.) that contribute to your qualifications. If the files you need to upload in 1 document exceed the size limit of the application portal, please send them directly to the QMSS Program Manager at [email protected] .
About QMSS: The Quantitative Methods in the Social Science (QMSS) program in the College of Literature, Science, and the Arts at the University of Michigan aims to train undergraduate students in the theories and methods needed to be successful data literate social scientists. Todays job market is saturated with opportunities that either desire or require skills in data literacy, whether that means being able to find data, analyze data, or know how and when to use data; and this is true even for jobs outside of the data science or analyst fields specifically.
QMSS was designed to teach students how data can be used to generate solutions for social problems of today and tomorrow and give students opportunities to apply and practice their skills to hit the ground running in their internships and careers in the future. QMSS is unique relative to programs in statistics or data science in that we teach data-based skills from a social science perspective. We are strongly committed to meeting students where they R [are], and providing ample time and resources to help them succeed so they can leave our courses and minor program with both competence and confidence in applied data and statistical skills for the social world.
Course Description
These Graduate Student Instructor (GSI) positions in QMSS are 50% effort positions. There are 4 available positions for Fall 2024. Between Winter 2021 and Winter 2024, QMSS received an average of 22 applications for available GSI positions.
QMSS 201 - Introduction to Quantitative Methods in the Social Sciences: This course includes training in descriptive statistics, data collection, data management, and data cleaning. It provides an overview of research design and hands-on experience with using data to ask and answer research questions from various social science disciplines, and educates students about ethical issues around data, data analysis, and reporting. Students will be taught and asked to use Excel, Tableau, and R in this course.
For information about class size, please refer to the LSA GSI Class Size policy here: LSA GSI Class Size Policy .
Responsibilities*
Duties for these positions include, but are not limited to:
- Attend course lectures (3 hours/week)
- Teach 2 x 1-hour lab sections each week. Lab times are scheduled so that GSIs may be able to teach 2 back-to-back sections in the same room for ease of planning, though this is not guaranteed.
- Dedicate at least 3 hours per week of office hours and/or individual meeting times to address student questions.
- Attend a minimum of 7 QMSS Community Hours events throughout the semester for a total of 14 hours. Community Hours are designed to be a supplement to traditional office hours during which students from all QMSS courses can come to work independently or in groups (depending on the rules of given assignments) on problem sets, projects, and/or exam studying. During Community Hours, 1-2 GSIs from each QMSS 201 and QMSS 301 course will be expected to be present for possible student questions. You may be assisting students who are not enrolled in your lab sections. QMSS Community Hours will be scheduled once per week on a Monday, Tuesday, or Wednesday evening from 6pm - 9pm.
- Participate in weekly teaching team meetings.
- Assist with software/tools/datasets.
- Co-create problem sets and other assignments.
- Grade and provide constructive feedback on assignments and projects.
- Participate in QMSS program activities.
Required Qualifications*
To be appointed as a GSI or GSSA, a graduate student must be in good standing in their degree program and for Terms I and II, must be registered for not less than six (6) credit hours. With written approval of the student's faculty advisor, five (5) credit hours may be acceptable.
Proficiency with analytic tools (Excel, Tableau, Stata, R, Python, etc.) is required. You do not have to be proficient in all analytic tools associated with QMSS 201 to be considered or qualified.
Desired Qualifications*
LSA doctoral students within their funding package. A mastery of quantitative methods in the social sciences, pursuing a graduate degree in a quantitative methods- or social sciences-related field, and real-world job/internship, teaching, and/or research experience that will help in teaching undergraduate students how to perform quantitative analyses using common analytical tools in order to answer social science questions is desired. A strong commitment to serving as a resource for undergraduate students and ability to make quantitative methods in the social sciences accessible to students of all levels of previous experience (including none at all) is preferred.
Contact Information
For any questions, students can reach out to [email protected] .
Decision Making Process
The Director of Quantitative Methods in the Social Sciences awards all GSI positions based on stated qualifications, criteria, and academic discretion. Priority will be given to LSA doctoral students within their five year funding commitment, with a preference for students pursuing degrees in a social science discipline.
For any questions, students can reach out to [email protected] .
Selection Process
Geo contract information.
The University will not discriminate against any applicant for employment because of race, creed, color, religion, national origin, ancestry, genetic information, marital status, familial status, parental status or pregnancy status, sex, gender identity or expression (whether actual or perceived), sexual orientation, age, height, weight, disability, citizenship status, veteran status, HIV antibody status, political belief, membership in any social or political organization, participation in a grievance or complaint whether formal or informal, medical conditions including those related to pregnancy, childbirth and breastfeeding, arrest record, or any other factor where the item in question will not interfere with job performance and where the employee is otherwise qualified. The University of Michigan agrees to abide by the protections afforded employees with disabilities as outlined in the rules and regulations which implement Section 504 of the Rehabilitation Act of 1973 and the Americans with Disabilities Act.
Information for the Office for Institutional Equity may be found at https://oie.umich.edu/ and for the University Ombuds at https://ombuds.umich.edu/
Unsuccessful applications will be retained for consideration in the event that there are last minute openings for available positions. In the event that an employee does not receive their preferred assignment, they can request a written explanation or an in-person interview with the hiring agents(s) to be scheduled at a mutually agreed upon time.
This position, as posted, is subject to a collective bargaining agreement between the Regents of the University of Michigan and the Graduate Employees' Organization, American Federation of Teachers, AFL-CIO 3550.
Standard Practice Guide 601.38, Required Disclosure of Felony Charges and/or Felony Convictions applies to all Graduate Student Assistants (GSAs). SPG 601.38 may be accessed online at https://spg.umich.edu/policy/601.38 , and its relation to your employment can be found in MOU 10 of your employment contract.
U-M EEO/AA Statement
The University of Michigan is an equal opportunity/affirmative action employer.
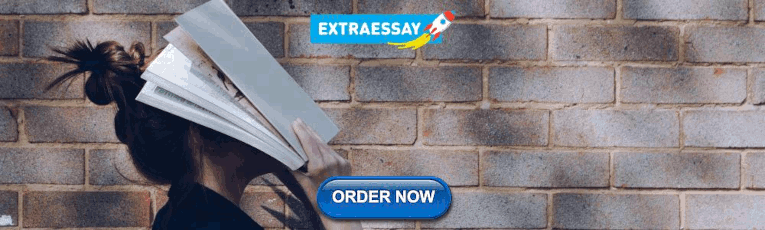
IMAGES
VIDEO
COMMENTS
This repository contains Ipython notebooks of assignments and tutorials used in the course introduction to data science in python, part of Applied Data Science using Python Specialization from Univ...
This repository includes course assignments of Introduction to Data Science in Python on coursera by university of michigan - tchagau/Introduction-to-Data-Science-in-Python
Introduction to data science in python Assignment_3 Coursera. This assignment requires more individual learning then the last one did - you are encouraged to check out the pandas documentation to find functions or methods you might not have used yet, or ask questions on Stack Overflow and tag them as pandas and python related. And of course ...
Download ZIP. Introduction to Data Science in Python Assignment-3. Raw. Assignment-3.py. This file contains bidirectional Unicode text that may be interpreted or compiled differently than what appears below. To review, open the file in an editor that reveals hidden Unicode characters. Learn more about bidirectional Unicode characters.
Stack Overflow Public questions & answers; Stack Overflow for Teams Where developers & technologists share private knowledge with coworkers; Talent Build your employer brand ; Advertising Reach developers & technologists worldwide; Labs The future of collective knowledge sharing; About the company
Learn how to use Pandas, a powerful Python library for data analysis, in this assignment from Introduction to Data Science with Python course. You will practice basic operations on DataFrames, such as filtering, grouping, and merging.
41:10 SCORE 100/100 uwu SKILLS YOU WILL GAIN* Understand techniques such as lambdas and manipulating csv files* Describe common Python functionality and feat...
You signed in with another tab or window. Reload to refresh your session. You signed out in another tab or window. Reload to refresh your session. You switched accounts on another tab or window.
problem to submit assignment 3 in course "introduction to data science in python" ... and tag them as pandas and python related. And of course, the discussion forums are open for interaction with your peers and the course staff. ^ SyntaxError: invalid syntax ... We can easily resolve the issue by just adding one cell before "Answer One" and ...
These may include the latest answers to Introduction to Data Science in Python's quizs and assignments. You can see the link in my blog or CSDN. Blog link: Coursera | Introduction to Data Science in Python(University of Michigan)| Quiz答案. Coursera | Introduction to Data Science in Python(University of Michigan)| Assignment1
NPTEL - PYTHON FOR DATA SCIENCE ASSIGNMENT - 3. Types of questions: MCQs - Multiple Choice Questions (a question has only one correct answer) MSQs - Multiple Select Questions (a question can have two, three or four correct options) In this case, equal weightage must be given to all options ... 3. c. 3. d. None of the above; Answer c) The ...
Last Updated : 01 Jul, 2024. This Data Science Tutorial with Python tutorial will help you learn the basics of Data Science along with the basics of Python according to the need in 2024 such as data preprocessing, data visualization, statistics, making machine learning models, and much more with the help of detailed and well-explained examples.
Also, make sure to exclude the footer and header information from the datafile. The first two columns are unneccessary, so you should get rid of them, and you should change the column labels so that the columns are: # Convert `Energy Supply` to gigajoules (there are 1,000,000 gigajoules in a petajoule). For all countries which have missing data ...
There are 4 modules in this course. This course will introduce the learner to the basics of the python programming environment, including fundamental python programming techniques such as lambdas, reading and manipulating csv files, and the numpy library. The course will introduce data manipulation and cleaning techniques using the popular ...
This course will teach you how to leverage the power of Python and artificial intelligence to create and test hypothesis. We'll start for the ground up, learning some basic Python for data science before diving into some of its richer applications to test our created hypothesis. We'll learn some of the most important libraries for exploratory ...
Preface. This book is developed for the course STAT303-1 (Data Science with Python-1). The first two chapters of the book are a review of python, and will be covered very quickly. Students are expected to know the contents of these chapters beforehand, or be willing to learn it quickly. Students may use the STAT201 book (https://nustat.github ...
This course will introduce the learner to the basics of the python programming environment, including fundamental python programming techniques such as lambdas, reading and manipulating csv files, and the numpy library. The course will introduce data manipulation and cleaning techniques using the popular python pandas data science library and ...
This course should be taken before any of the other Applied Data Science with Python courses: Applied Plotting, Charting & Data Representation in Python, Applied Machine Learning in Python, Applied Text Mining in Python, Applied Social Network Analysis in Python. I have some problem with Assignment 3 ( .
Components of Hashing . There are majorly three components of hashing: Key: A Key can be anything string or integer which is fed as input in the hash function the technique that determines an index or location for storage of an item in a data structure. Hash Function: The hash function receives the input key and returns the index of an element in an array called a hash table.
I'm taking this course on Coursera, and I'm running some issues while doing the first assignment. The task is to basically use regular expression to get certain values from the given file. ... Introduction of Data Science in Python Assignment 1. Ask Question Asked 3 years, 8 months ago. ... Please be sure to answer the question. Provide details ...
This project contains all the assignment's solution of university of Michigan. - sapanz/Applied-Data-Science-with-Python---Coursera
This course includes training in descriptive statistics, data collection, data management, and data cleaning. It provides an overview of research design and hands-on experience with using data to ask and answer research questions from various social science disciplines, and educates students about ethical issues around data, data analysis, and ...
In this assignment you must read in a file of metropolitan regions and associated sports teams from assets/wikipedia_data.html and answer some questions about each metropolitan region. Each of these regions may have one or more teams from the "Big 4": NFL (football, in assets/nfl.csv), MLB (baseball, in assets/mlb.csv), NBA (basketball, in ...