National University Volume 86C-2 Catalog - July 2024 | | 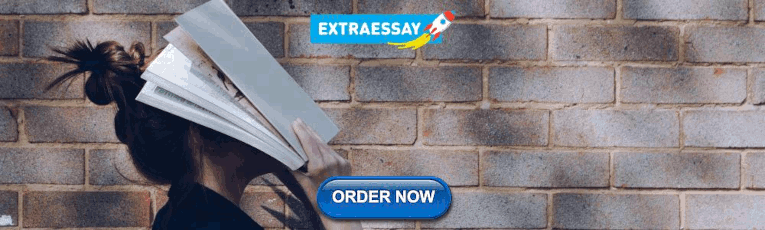 Doctor of Philosophy in Data ScienceDescription of program. The Doctor of Philosophy in Data Science (PhD-DS) focuses on data analytics, statistical modeling, and machine learning. Data science involves mining data and maintaining, processing, modeling data, and communicating the results in a professional or scientific setting. The PhD-DS program will prepare you for research spanning big data analytics, artificial intelligence, and data-driven decision-making by exploring each stage of the data science life cycle in depth from an applied and a theoretical perspective. Click here for potential career opportunities within the PhD-DS. Learning Outcomes- Develop knowledge in data science based on a synthesis of current theories.
- Explain theories, applications, and perspectives related to data science.
- Evaluate theories of ethics and risk management in information systems.
- Formulate strategies for data and knowledge management in global organizations.
- Contribute to the body of theory and practice in data science.
Basis for AdmissionsAdmission to the Doctor of Philosophy in Data Science program requires a conferred master’s or doctoral degree from a regionally or nationally accredited academic institution. Degree RequirementsThe University may accept a maximum of 12 semester credit hours in transfer toward the doctoral degree for graduate coursework completed at an accredited college or university with a grade of “B” or better. The PhD-DS degree program also has the following requirements: - GPA of 3.0 (letter grade of “B”) or higher
- University approval of Dissertation Manuscript and Oral Defense completed
- Submission of approved final dissertation manuscript to the University Registrar, including the original unbound manuscript and an electronic copy
- Official transcripts on file for all transfer credit hours accepted by the University
- All financial obligations must be met before the student will be issued their complimentary diploma
Dissertation Completion PathwayThe University’s mission is dedicated to assisting students in achieving their academic aspirations and helping them become valuable contributors to their community and profession. To support our mission, the University now offers a dissertation completion pathway for students who have successfully completed their doctoral coursework and achieved doctoral candidacy at a previous institution but were unable to complete their dissertation. The University’s Dissertation Completion Pathway (DCP) offers a unique opportunity for students to complete their doctorate in one of the doctoral programs offered at the University (excluding the PhD-MFT and DNP). Students successfully meeting the entrance and application requirements will complete a minimum of 23 credit hours to earn their doctorate. Click for more information on the Dissertation Completion Pathway. Time to CompletionThe University allows 7 years to complete all doctoral programs of 60 credits or less. The median time to completion for this program is 49 months. Time to completion varies depending upon the pace in which a student completes courses and the number of transfer credits accepted. As most students are working adults, balancing educational, professional, and personal commitments, our academic and finance advisors will work with you to develop a program schedule that works best for your needs. Students following the preferred schedule designed by the Dean for this program, and applying no transfer credits, can expect to finish in as little as 40 months. Dissertation ProcessFaculty assists each Doctoral student to reach this high goal through a systematic process leading to a high-quality completed dissertation. A PhD dissertation is a scholarly documentation of research that makes an original contribution to the field of study. This process requires care in choosing a topic, documenting its importance, planning the methodology, and conducting the research. These activities lead smoothly into the writing and oral presentation of the dissertation. A doctoral candidate must be continuously enrolled throughout the series of dissertation courses. Dissertation courses are automatically scheduled and accepted without a break in scheduling to ensure that students remain in continuous enrollment throughout the dissertation course sequence. If additional time is required to complete any of the dissertation courses, students must re-enroll and pay the tuition for that course. Continuous enrollment will only be permitted when students demonstrate progress toward completing dissertation requirements. The Dissertation Committee determines progress. Course SequenceThe PhD program may be completed in a minimum of 60 credits. Additional credit hours may be allowed as needed to complete the dissertation research. If granted, additional courses will be added to the student degree program in alignment with the SAP and Academic Maximum Time to Completion policies. Students who do not complete their program in accordance with these policies may be dismissed. - TIM-8500 - Principles of Data Science
- TIM-8501 - Exploratory Data Analysis
- TIM-8521 - Statistical Modeling
- TIM-8555 - Predictive Analysis
- TIM-7020 - Databases & Business Intelligence
- TIM-8530 - Big Data Integration
- TIM-8131 - Data Mining
- TIM-8515 - Multivariate Analysis
- TIM-8536 - Current Topics in Data Science
- TIM-8150 - Artificial Intelligence
- TIM-8510 - Data Visualization & Communication
- TIM-7211 - Introduction to Research Design and Methodology for Technology Leaders
- TIM-7250 - Research Design in Data Science
- TIM-7255 - Advanced Research Design in Data Science
- TIM-8590 - Data, Information, Knowledge Policy and Strategy
- CMP-9701DS - PhD Pre-Candidacy Prospectus
- DIS-9901A - Components of the Dissertation
- DIS-9902A - The Dissertation Proposal
- DIS-9903A - Institutional Review Board (IRB) and Data Collection
- DIS-9904A - The Dissertation Manuscript and Defense
Chapman University Digital CommonsHome > Dissertations and Theses > Computational and Data Sciences (PhD) Dissertations Computational and Data Sciences (PhD) DissertationsBelow is a selection of dissertations from the Doctor of Philosophy in Computational and Data Sciences program in Schmid College that have been included in Chapman University Digital Commons. Additional dissertations from years prior to 2019 are available through the Leatherby Libraries' print collection or in Proquest's Dissertations and Theses database. Dissertations from 2024 2024A Novel Correction for the Multivariate Ljung-Box Test , Minhao Huang Medical Image Analysis Based on Graph Machine Learning and Variational Methods , Sina Mohammadi Machine Learning and Geostatistical Approaches for Discovery of Weather and Climate Events Related to El Niño Phenomena , Sachi Perera Global to Glocal: A Confluence of Data Science and Earth Observations in the Advancement of the SDGs , Rejoice Thomas Dissertations from 2023 2023Computational Analysis of Antibody Binding Mechanisms to the Omicron RBD of SARS-CoV-2 Spike Protein: Identification of Epitopes and Hotspots for Developing Effective Therapeutic Strategies , Mohammed Alshahrani Integration of Computer Algebra Systems and Machine Learning in the Authoring of the SANYMS Intelligent Tutoring System , Sam Ford Voluntary Action and Conscious Intention , Jake Gavenas Random Variable Spaces: Mathematical Properties and an Extension to Programming Computable Functions , Mohammed Kurd-Misto Computational Modeling of Superconductivity from the Set of Time-Dependent Ginzburg-Landau Equations for Advancements in Theory and Applications , Iris Mowgood Application of Machine Learning Algorithms for Elucidation of Biological Networks from Time Series Gene Expression Data , Krupa Nagori Stochastic Processes and Multi-Resolution Analysis: A Trigonometric Moment Problem Approach and an Analysis of the Expenditure Trends for Diabetic Patients , Isaac Nwi-Mozu Applications of Causal Inference Methods for the Estimation of Effects of Bone Marrow Transplant and Prescription Drugs on Survival of Aplastic Anemia Patients , Yesha M. Patel Causal Inference and Machine Learning Methods in Parkinson's Disease Data Analysis , Albert Pierce Causal Inference Methods for Estimation of Survival and General Health Status Measures of Alzheimer’s Disease Patients , Ehsan Yaghmaei Dissertations from 2022 2022Computational Approaches to Facilitate Automated Interchange between Music and Art , Rao Hamza Ali Causal Inference in Psychology and Neuroscience: From Association to Causation , Dehua Liang Advances in NLP Algorithms on Unstructured Medical Notes Data and Approaches to Handling Class Imbalance Issues , Hanna Lu Novel Techniques for Quantifying Secondhand Smoke Diffusion into Children's Bedroom , Sunil Ramchandani Probing the Boundaries of Human Agency , Sook Mun Wong Dissertations from 2021 2021Predicting Eye Movement and Fixation Patterns on Scenic Images Using Machine Learning for Children with Autism Spectrum Disorder , Raymond Anden Forecasting the Prices of Cryptocurrencies using a Novel Parameter Optimization of VARIMA Models , Alexander Barrett Applications of Machine Learning to Facilitate Software Engineering and Scientific Computing , Natalie Best Exploring Behaviors of Software Developers and Their Code Through Computational and Statistical Methods , Elia Eiroa Lledo Assessing the Re-Identification Risk in ECG Datasets and an Application of Privacy Preserving Techniques in ECG Analysis , Arin Ghazarian Multi-Modal Data Fusion, Image Segmentation, and Object Identification using Unsupervised Machine Learning: Conception, Validation, Applications, and a Basis for Multi-Modal Object Detection and Tracking , Nicholas LaHaye Machine-Learning-Based Approach to Decoding Physiological and Neural Signals , Elnaz Lashgari Learning-Based Modeling of Weather and Climate Events Related To El Niño Phenomenon via Differentiable Programming and Empirical Decompositions , Justin Le Quantum State Estimation and Tracking for Superconducting Processors Using Machine Learning , Shiva Lotfallahzadeh Barzili Novel Applications of Statistical and Machine Learning Methods to Analyze Trial-Level Data from Cognitive Measures , Chelsea Parlett Optimal Analytical Methods for High Accuracy Cardiac Disease Classification and Treatment Based on ECG Data , Jianwei Zheng Dissertations from 2020 2020Development of Integrated Machine Learning and Data Science Approaches for the Prediction of Cancer Mutation and Autonomous Drug Discovery of Anti-Cancer Therapeutic Agents , Steven Agajanian Allocation of Public Resources: Bringing Order to Chaos , Lance Clifner A Novel Correction for the Adjusted Box-Pierce Test — New Risk Factors for Emergency Department Return Visits within 72 hours for Children with Respiratory Conditions — General Pediatric Model for Understanding and Predicting Prolonged Length of Stay , Sidy Danioko A Computational and Experimental Examination of the FCC Incentive Auction , Logan Gantner Exploring the Employment Landscape for Individuals with Autism Spectrum Disorders using Supervised and Unsupervised Machine Learning , Kayleigh Hyde Integrated Machine Learning and Bioinformatics Approaches for Prediction of Cancer-Driving Gene Mutations , Oluyemi Odeyemi On Quantum Effects of Vector Potentials and Generalizations of Functional Analysis , Ismael L. Paiva Long Term Ground Based Precipitation Data Analysis: Spatial and Temporal Variability , Luciano Rodriguez Gaining Computational Insight into Psychological Data: Applications of Machine Learning with Eating Disorders and Autism Spectrum Disorder , Natalia Rosenfield Connecting the Dots for People with Autism: A Data-driven Approach to Designing and Evaluating a Global Filter , Viseth Sean Novel Statistical and Machine Learning Methods for the Forecasting and Analysis of Major League Baseball Player Performance , Christopher Watkins Dissertations from 2019 2019Contributions to Variable Selection in Complexly Sampled Case-control Models, Epidemiology of 72-hour Emergency Department Readmission, and Out-of-site Migration Rate Estimation Using Pseudo-tagged Longitudinal Data , Kyle Anderson Bias Reduction in Machine Learning Classifiers for Spatiotemporal Analysis of Coral Reefs using Remote Sensing Images , Justin J. Gapper Estimating Auction Equilibria using Individual Evolutionary Learning , Kevin James Employing Earth Observations and Artificial Intelligence to Address Key Global Environmental Challenges in Service of the SDGs , Wenzhao Li Image Restoration using Automatic Damaged Regions Detection and Machine Learning-Based Inpainting Technique , Chloe Martin-King Theses from 2017 2017Optimized Forecasting of Dominant U.S. Stock Market Equities Using Univariate and Multivariate Time Series Analysis Methods , Michael Schwartz Advanced Search - Notify me via email or RSS
Author Corner- Submit Research
- Rights and Terms of Use
- Leatherby Libraries
- Chapman University
ISSN 2572-1496 Home | About | FAQ | My Account | Accessibility Statement Privacy Copyright Network Optimisation using Big Data Analytics--> Hadi, Mohammed Safa (2020) Network Optimisation using Big Data Analytics. PhD thesis, University of Leeds. Interdisciplinary research is fuelling a paradigm shift to endow technology-based services with a personalised dimension. The main contributors for such innovatory change are the surge in data production rate, the proliferation of data generators in the form of IoT and other network-connected devices, the incorporation of innovative data technologies like Artificial Intelligence, Machine Learning and Big Data Analytics, and the advancements in computing powers that are getting closer to dethroning Moo la and delie moe poceing pe ni ime. Moeoe, hee i an ever-increasing demand for smart and fast-responsive applications such as predictive analytics, business analysis and digital marketing. In this thesis, patientcentric cellular network optimisation is investigated as a promising paradigm that can contribute to the personalisation of present and future cellular networks with the aim of saving people lie hee ee econd con. This calls for transforming current cellular networks from merely being blind tubes that convey data, into a conscious, cognitive, and self-optimizing entity that adapts intelligently according to he e need. The work carried out in this thesis started by comprehensively exploring the role of using big data analytics in network design. Subsequently, we considered incorporating the concepts of priority, e-healthcare, Big Data Analytics, and eoce allocaion in a ingle em. The em goal i o e big daa haeed from out-patient electronic health records and body-connected medical Internet of Things sensors to be processed and analysed in a big data analytics engine to predict the likelihood of a stroke. This prediction is then used to ensure that the out-patients are assigned optimal physical resource blocks that provide good signal to interference and noise ratio (SINR) dictated by the severity of their medical state. Hence, granting channels of high spectral efficiency to the out-patients, empowering them to transmit their critical data to the designated medical facility with minimal delay. The use of several Machine Learning algorithms residing within the big data analytics engine is investigated, namely, a naïve Bayesian classifier, a decision tree
iii
classifier, and a logistic regression classifier. Further, the incorporation of the aforementioned classifiers in an ensemble system running as a soft voting classifier is examined and the performance of all classifiers is compared. The combinatorial optimisation problem of maximising he em oeall SINR hile pioiising the OPs in terms of radio resource assignment is solved using Mixed Integer Linear Programming and a heuristic. The use of two resource allocation approaches, namely, a Weighted Sum Rate Maximisation approach and a Proportional Fairness approach is considered and compared in terms of fairness and the attained SINRs. The proposed system was extended from a single-tier (homogenous) LTE-A network, to multi-tier Heterogeneous Networks employing spectrum partitioning strategy, and finally to a multi-tier Heterogeneous Network with no interferencemitigation strategies emploed. Th, enabling a fhe d of he em performance over different networks and interference strategies. Supervisors: | Elmirghani, Jaafar and Elgorashi, Taisir | Related URLs: | | Keywords: | wireless network optimization; MILP; OFDMA uplink optimization; big data analytics; cellular network design; naïve Bayesian classifier; patient-centric network optimization; resource allocation; resource management; Machine learning; Logistic Regression classifier; Decision Trees classifier; Soft voting classifier. classification. | Awarding institution: | University of Leeds | Academic Units: | | Identification Number/EthosID: | uk.bl.ethos.808670 | Depositing User: | Mr. Mohammed Safa Hadi Hadi | Date Deposited: | 26 Jun 2020 15:27 | Last Modified: | 11 Aug 2022 09:53 | --> Final eThesis - complete (pdf) -->Filename: Hadi_Mohammed_Safa_Hadi_Electronic_and_Electrical_Engineering_PhD_2020.pdf Description: PhD Thesis  Embargo Date: ![doctoral thesis in big data [img]](https://etheses.whiterose.ac.uk/27179/1.hassmallThumbnailVersion/Hadi_Mohammed_Safa_Hadi_Electronic_and_Electrical_Engineering_PhD_2020.pdf) You do not need to contact us to get a copy of this thesis. Please use the 'Download' link(s) above to get a copy. You can contact us about this thesis . If you need to make a general enquiry, please see the Contact us page.  Academia.edu no longer supports Internet Explorer. To browse Academia.edu and the wider internet faster and more securely, please take a few seconds to upgrade your browser . Enter the email address you signed up with and we'll email you a reset link. 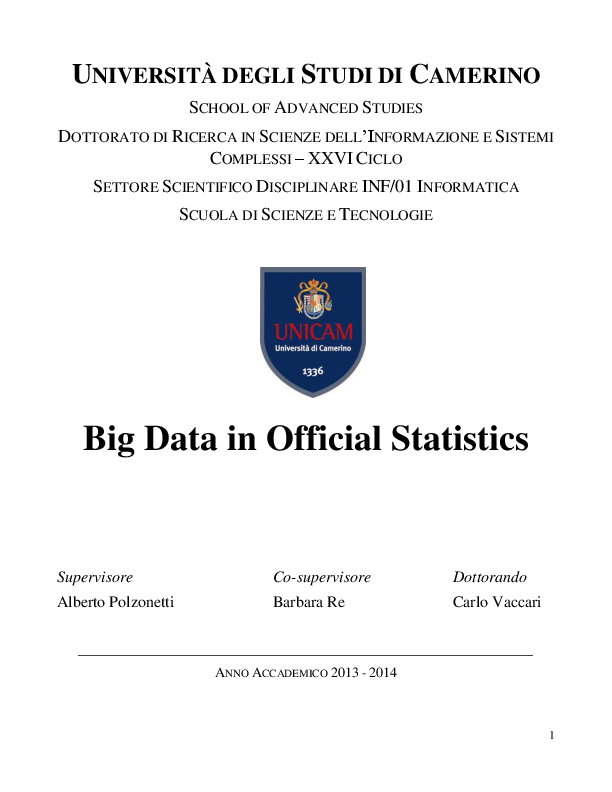 PhD Thesis on "Big Data in Official Statistics" The explosion in the amount of data, called “data deluge”, is forcing to redefine many scientific and technological fields, with the affirmation in any environment of Big Data as a potential source of data. Official statistics institutions a few years ago started to open up to external data sources such as administrative data. The advent of Big Data is introducing important innovations: the availability of additional external data sources, dimensions previously unknown and questionable consistency, poses new challenges to the institutions of official statistics, imposing a general rethinking that involves tools, software, methodologies and organizations. The relative newness of the field of study on Big Data requires first of all an introduction phase, for addressing the problems of definition and for defining the areas of technology involved and the possible fields of application. The challenges that the use of Big Data poses to institutions that deal with official statistics are then presented in detail, after a brief discussion of the relationship between the new "data science" and statistics. Although at an early stage, there is already a number, limited but growing practical experience in the use of Big Data as a data source for use in statistics by public (and private) institutions. The review of these experiences can serve as a stimulus to address in a more conscious and organized way the challenges that the use of this data source requires all producers of official statistics. The worldwide spread of data sources (web, e-commerce, sensors) has also prompted the statistical community to take joint action to tackle the complex set of methodological, technical and legal problems. And so many national statistical institutes along with the most prestigious international organizations have initiated joint projects that will develop in the coming years to address the complex issues raised by Big Data for statistical methodology and computer technology. Related PapersIntersections Johanna Giczi More than five years ago Eurostat started a project with the aim to 'tame' sources of Big Data in a way that they can be incoporated into official statistical systems. In order to solve the problems a statistician might be faced with during the official statistical application of Big Data, first of all, we give an overview of traditional data collection, and then point to the differences one has to face when dealing with Big Data. We introduce common sources of data (traditional, administrative) and highlight the ways huge sets of data are different compared to them. Next, we discuss characteristics of Big Data versus traditional statistical methods based on the qualitative criteria of official statistics, and we also elaborate on the problems of analysing Big Data. Finally, we provide a list of use cases for Big Data in official statistical data collections. 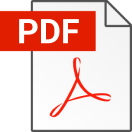 Carlo Vaccari Gregory Farmakis The aim of this paper is to assess the feasibility of employing novel methodologies for producing high quality Official Statistics based on Big Data. Big Data can be described as high volume, high velocity and high variety of information that require new methods of processing. Big Data is such a rich source of data that the Official Statistics community cannot ignore. Official statistics are statistics published by government agencies or other public bodies that aim to response to policy needs. They are based almost exclusively on survey data collections and administrative data. On the other hand, Big Data challenge the way we think about data assets, the sources we collect them from and the way we analyze them. This illustrates that paradigms are shifting, since a reverse approach of designing statistics is being applied. With Big Data, it is fundamental to explore the vast amounts of data available first and then to decide on the quantities to be measured. Thus, inference techniques used for Official Statistics will need to make a shift too. In this paper, we explore the potential of using Big Data sources as input for supplemeting or replacing Official Statistics is examined. The work is carried out under the framework of a European Commission / Eurostat project. Five sources are examined: (a) the Automatic Identification System (AIS) records for replacing Official Maritime Transport Statistics on vessel movement, (b) a classifieds site concerning house sales and rental prices in order to produce Housing Price Official Statistics, (c) the Facebook and Twitter content to produce well-being quality of life measures, complementary to Official Statistics, (d) Credit card transaction data for supplementing consumption expenditure statistics and (e) the ‘‘δι@ύγεια’’ site publishing government expenditure decisions for national accounting purposes. The potential of using the selected Big Data sources as input of Official Statistics is investigated in terms of the quality of the statistics they produce. The quality of the data is disscussed according to the dimensions defined by the European Statistical System, namely: a) relevance, b) accuracy, c) timeliness and puctuality, d) accessibility and clarity and e) coherence. The cost involved in the production prossess is also discussed. The evaluation of the effect of methodological restrictions on the overall quality of the statistics produced reveals several potential benefits as well as disadvantages. Among all, Big Data are characterized by the amount of information and the frequency at which they are produced. The high geographical coverage and the accuracy of the data, are some of the pros. On the other hand, a major issue concerning Big Data is the accessibility due to confidentiality or other policies. In addition, the volume of the data increases further the processing needs for validation. It is highlighted, that the quantities produced can be used supplementary to the respective Official Statistics’ indicators, while at most of the cases, it is not feasible to replace them. However, exploiting the vast ammounts of data available in a methodologically sound way may enhance the fast production of low cost and high quality official statistics. That is, Big Data is a field that requires new tailored methods to accelerate the analysis of large amount of data. Big Data is an extremely interesting data source for statistics. Since more and more data is generated in our modern world and is digitally stored, it could certainly be used to replace traditional sources or provide additional information for official statistics. Especially given declines in survey response rates, information gathered from Big Data is an interesting addition. However, extracting statistically-relevant information from Big Data sources is not an easy task. In this paper the current state of the art of research on the use of Big Data for official statistics at Statistics Netherlands is described. The paper is based on the real world experiences of the authors obtained during these studies. The topics discussed are related to Big Data methodology, privacy and security concerns and the skills required for successfully employing Big Data. Introduction Big Data is a term that one hears more and more often at conferences, meetings and seminars. Since its first introductio... Rob Kitchin The development of big data is set to be a significant disruptive innovation in the production of official statistics offering a range of opportunities, challenges and risks to the work of National Statistical Institutions (NSIs). This paper provides a synoptic overview of these issues in detail, mapping out the various pros and cons of big data for producing official statistics, examining the work to date by NSIs in formulating a strategic and operational response to big data, and plotting some suggestions with respect to ongoing change management needed to address the use of big data for official statistics. Journal of Official Statistics More and more data are being produced by an increasing number of electronic devices physically surrounding us and on the internet. The large amount of data and the high frequency at which they are produced have resulted in the introduction of the term ‘Big Data’. Because these data reflect many different aspects of our daily lives and because of their abundance and availability, Big Data sources are very interesting from an official statistics point of view. This article discusses the exploration of both opportunities and challenges for official statistics associated with the application of Big Data. Experiences gained with analyses of large amounts of Dutch traffic loop detection records and Dutch social media messages are described to illustrate the topics characteristic of the statistical analysis and use of Big Data. More and more data are being produced by an increasing number of electronic devices physically surrounding us and on the internet. The large amount of data and the high frequency at which they are produced have resulted in the introduction of the term ‘Big Data’. Because of the fact that these data reflect many different aspects of our daily lives and because of their abundance and availability, Big Data sources are very interesting from an official statistics point of view. However, first experiences obtained with analyses of large amounts of Dutch traffic loop detection records, call detail records of mobile phones and Dutch social media messages reveal that a number of challenges need to be addressed to enable the application of these data sources for official statistics. These and the lessons learned during these initial studies will be addressed and illustrated by examples. More specifically, the following topics are discussed: the three general types of Big Data discerned, the... Giulio Barcaroli 1. Big data are becoming more and more important as additional data sources for Official Statistics (OS). Istat set up three projects aimed to experiment three different roles of Big data sources, namely: (i) “new” sources enabling access to data not yet collected by Official Statistics (ii) “additional” sources to be used in conjunction with traditional data sources and (iii) “alternative” sources fully or partially replacing traditional ones. Serena Signorelli This paper tries to give a short overview on the use of Big Data for statistical purposes, on the problems that arise with this kind of data, especially about quality, shows some applications that havee been done using Big Data in combination with traditional survey data. Finally, a small-scale case study is presented by critically highlighting problems and solutions arising the transition form Big Data to information. It is widely recognised that important methodological and quality issues are associated with Big Data. Especially selectivity issues become prominent when trying to apply established statistical methods. Using sampling theory as the framework for the production of estimates based on Big Data may not be effective, especially if the data cannot be linked to a known population of units. The question arises to what extent an approach originally developed for survey based statistics can be applied to Big Data. The paper discusses possible quality approaches to the production of official statistics when dealing with Big Data, for instance the compilation of statistics not aimed at predefined populations. These approaches could result in rapid information with high relevance from a user’s perspective. However, the introduction of such approaches requires an assessment of the role that National Statistical Institutes aspire to play in the era of Big Data. Loading Preview Sorry, preview is currently unavailable. You can download the paper by clicking the button above. RELATED PAPERSAgostino Di Ciaccio , Giovanni Giorgi Teresa Oliveira Jan-philipp Kolb Fride J Eeg-Henriksen , P. Hackl Carlo Filippucci Saleha Habibullah Natalia Golini New Skills in the Changing World of Statistics Education Nureni Olawale Adeboye UNECE Expert Meeting on Dissemination and Communication of Statistics SSRN Electronic journal Leonid Gokhberg Jurnal pertahanan dan bela negara Dicky Ronny M Nainggolan Staff Discussion Notes Gabriel Quiros Statistical Journal of the IAOS Reza Hadizadeh Revd Popoola Peter Dimitrios Xanthidis , Paul Nikolaidis , Ourania Koutzampasopoulou Xanthidou Journal of Physics: Conference Series Alhaji Abdullahi Gwani Bo Sundgren Photis Stavropoulos Lutz Schubert Jim Ridgway RELATED TOPICS- We're Hiring!
- Help Center
- Find new research papers in:
- Health Sciences
- Earth Sciences
- Cognitive Science
- Mathematics
- Computer Science
- Academia ©2024
- ODSC EUROPE
- AI+ Training
- Speak at ODSC
 - Data Analytics
- Data Engineering
- Data Visualization
- Deep Learning
- Generative AI
- Machine Learning
- NLP and LLMs
- Business & Use Cases
- Career Advice
- Write for us
- ODSC Community Slack Channel
- Upcoming Webinars
 10 Compelling Machine Learning Ph.D. Dissertations for 2020Machine Learning Modeling Research posted by Daniel Gutierrez, ODSC August 19, 2020 Daniel Gutierrez, ODSC As a data scientist, an integral part of my work in the field revolves around keeping current with research coming out of academia. I frequently scour arXiv.org for late-breaking papers that show trends and reveal fertile areas of research. Other sources of valuable research developments are in the form of Ph.D. dissertations, the culmination of a doctoral candidate’s work to confer his/her degree. Ph.D. candidates are highly motivated to choose research topics that establish new and creative paths toward discovery in their field of study. Their dissertations are highly focused on a specific problem. If you can find a dissertation that aligns with your areas of interest, consuming the research is an excellent way to do a deep dive into the technology. After reviewing hundreds of recent theses from universities all over the country, I present 10 machine learning dissertations that I found compelling in terms of my own areas of interest. [Related article: Introduction to Bayesian Deep Learning ] I hope you’ll find several that match your own fields of inquiry. Each thesis may take a while to consume but will result in hours of satisfying summer reading. Enjoy! 1. Bayesian Modeling and Variable Selection for Complex DataAs we routinely encounter high-throughput data sets in complex biological and environmental research, developing novel models and methods for variable selection has received widespread attention. This dissertation addresses a few key challenges in Bayesian modeling and variable selection for high-dimensional data with complex spatial structures. 2. Topics in Statistical Learning with a Focus on Large Scale DataBig data vary in shape and call for different approaches. One type of big data is the tall data, i.e., a very large number of samples but not too many features. This dissertation describes a general communication-efficient algorithm for distributed statistical learning on this type of big data. The algorithm distributes the samples uniformly to multiple machines, and uses a common reference data to improve the performance of local estimates. The algorithm enables potentially much faster analysis, at a small cost to statistical performance. Another type of big data is the wide data, i.e., too many features but a limited number of samples. It is also called high-dimensional data, to which many classical statistical methods are not applicable. This dissertation discusses a method of dimensionality reduction for high-dimensional classification. The method partitions features into independent communities and splits the original classification problem into separate smaller ones. It enables parallel computing and produces more interpretable results. 3. Sets as Measures: Optimization and Machine LearningThe purpose of this machine learning dissertation is to address the following simple question: How do we design efficient algorithms to solve optimization or machine learning problems where the decision variable (or target label) is a set of unknown cardinality? Optimization and machine learning have proved remarkably successful in applications requiring the choice of single vectors. Some tasks, in particular many inverse problems, call for the design, or estimation, of sets of objects. When the size of these sets is a priori unknown, directly applying optimization or machine learning techniques designed for single vectors appears difficult. The work in this dissertation shows that a very old idea for transforming sets into elements of a vector space (namely, a space of measures), a common trick in theoretical analysis, generates effective practical algorithms. 4. A Geometric Perspective on Some Topics in Statistical LearningModern science and engineering often generate data sets with a large sample size and a comparably large dimension which puts classic asymptotic theory into question in many ways. Therefore, the main focus of this dissertation is to develop a fundamental understanding of statistical procedures for estimation and hypothesis testing from a non-asymptotic point of view, where both the sample size and problem dimension grow hand in hand. A range of different problems are explored in this thesis, including work on the geometry of hypothesis testing, adaptivity to local structure in estimation, effective methods for shape-constrained problems, and early stopping with boosting algorithms. The treatment of these different problems shares the common theme of emphasizing the underlying geometric structure. 5. Essays on Random Forest EnsemblesA random forest is a popular machine learning ensemble method that has proven successful in solving a wide range of classification problems. While other successful classifiers, such as boosting algorithms or neural networks, admit natural interpretations as maximum likelihood, a suitable statistical interpretation is much more elusive for a random forest. The first part of this dissertation demonstrates that a random forest is a fruitful framework in which to study AdaBoost and deep neural networks. The work explores the concept and utility of interpolation, the ability of a classifier to perfectly fit its training data. The second part of this dissertation places a random forest on more sound statistical footing by framing it as kernel regression with the proximity kernel. The work then analyzes the parameters that control the bandwidth of this kernel and discuss useful generalizations. 6. Marginally Interpretable Generalized Linear Mixed ModelsA popular approach for relating correlated measurements of a non-Gaussian response variable to a set of predictors is to introduce latent random variables and fit a generalized linear mixed model. The conventional strategy for specifying such a model leads to parameter estimates that must be interpreted conditional on the latent variables. In many cases, interest lies not in these conditional parameters, but rather in marginal parameters that summarize the average effect of the predictors across the entire population. Due to the structure of the generalized linear mixed model, the average effect across all individuals in a population is generally not the same as the effect for an average individual. Further complicating matters, obtaining marginal summaries from a generalized linear mixed model often requires evaluation of an analytically intractable integral or use of an approximation. Another popular approach in this setting is to fit a marginal model using generalized estimating equations. This strategy is effective for estimating marginal parameters, but leaves one without a formal model for the data with which to assess quality of fit or make predictions for future observations. Thus, there exists a need for a better approach. This dissertation defines a class of marginally interpretable generalized linear mixed models that leads to parameter estimates with a marginal interpretation while maintaining the desirable statistical properties of a conditionally specified model. The distinguishing feature of these models is an additive adjustment that accounts for the curvature of the link function and thereby preserves a specific form for the marginal mean after integrating out the latent random variables. 7. On the Detection of Hate Speech, Hate Speakers and Polarized Groups in Online Social MediaThe objective of this dissertation is to explore the use of machine learning algorithms in understanding and detecting hate speech, hate speakers and polarized groups in online social media. Beginning with a unique typology for detecting abusive language, the work outlines the distinctions and similarities of different abusive language subtasks (offensive language, hate speech, cyberbullying and trolling) and how we might benefit from the progress made in each area. Specifically, the work suggests that each subtask can be categorized based on whether or not the abusive language being studied 1) is directed at a specific individual, or targets a generalized “Other” and 2) the extent to which the language is explicit versus implicit. The work then uses knowledge gained from this typology to tackle the “problem of offensive language” in hate speech detection. 8. Lasso Guarantees for Dependent DataSerially correlated high dimensional data are prevalent in the big data era. In order to predict and learn the complex relationship among the multiple time series, high dimensional modeling has gained importance in various fields such as control theory, statistics, economics, finance, genetics and neuroscience. This dissertation studies a number of high dimensional statistical problems involving different classes of mixing processes. 9. Random forest robustness, variable importance, and tree aggregationRandom forest methodology is a nonparametric, machine learning approach capable of strong performance in regression and classification problems involving complex data sets. In addition to making predictions, random forests can be used to assess the relative importance of feature variables. This dissertation explores three topics related to random forests: tree aggregation, variable importance, and robustness. 10. Climate Data Computing: Optimal Interpolation, Averaging, Visualization and DeliveryThis dissertation solves two important problems in the modern analysis of big climate data. The first is the efficient visualization and fast delivery of big climate data, and the second is a computationally extensive principal component analysis (PCA) using spherical harmonics on the Earth’s surface. The second problem creates a way to supply the data for the technology developed in the first. These two problems are computationally difficult, such as the representation of higher order spherical harmonics Y400, which is critical for upscaling weather data to almost infinitely fine spatial resolution. I hope you enjoyed learning about these compelling machine learning dissertations. Editor’s note: Interested in more data science research? Check out the Research Frontiers track at ODSC Europe this September 17-19 or the ODSC West Research Frontiers track this October 27-30.  Daniel Gutierrez, ODSCDaniel D. Gutierrez is a practicing data scientist who’s been working with data long before the field came in vogue. As a technology journalist, he enjoys keeping a pulse on this fast-paced industry. Daniel is also an educator having taught data science, machine learning and R classes at the university level. He has authored four computer industry books on database and data science technology, including his most recent title, “Machine Learning and Data Science: An Introduction to Statistical Learning Methods with R.” Daniel holds a BS in Mathematics and Computer Science from UCLA.  Podcast: World Models – A Deep Dive With Andre FrancaPodcast Modeling posted by ODSC Team Jul 2, 2024 Learn about cutting-edge developments in AI and data science from the experts who know them best...  Unveiling the Future: Data Science and AI in Financial MarketsPodcast Business + Management posted by ODSC Team Jul 2, 2024 In the rapidly evolving world of financial markets, the integration of artificial intelligence (AI) and data...  The Evolution of Retrieval Systems in AIModeling posted by ODSC Team Jul 2, 2024 In a recent episode of the ODSC Ai X Podcast, Pasquale Antonante, co-founder and CTO of...  We have 84 big data PhD Projects, Programmes & ScholarshipsAll disciplines All locations Institution All Institutions All PhD Types All Funding big data PhD Projects, Programmes & ScholarshipsData quality and cleaning in big data, phd research project. PhD Research Projects are advertised opportunities to examine a pre-defined topic or answer a stated research question. Some projects may also provide scope for you to propose your own ideas and approaches. Self-Funded PhD Students OnlyThis project does not have funding attached. You will need to have your own means of paying fees and living costs and / or seek separate funding from student finance, charities or trusts. Big data analytics and visualization for personalized Type 2 Diabetes treatment and management supportUsing big data approaches to enhance fluid stewardship in intensive care unit, funded phd project (european/uk students only). This project has funding attached for UK and EU students, though the amount may depend on your nationality. Non-EU students may still be able to apply for the project provided they can find separate funding. You should check the project and department details for more information. Modelling the Impact of Diagnostic Pathways in Cancer and Cardiovascular Disease - University of Swansea (part of Health Data Research UK’s Big Data for Complex Disease Driver Programme)Funded phd project (uk students only). This research project has funding attached. It is only available to UK citizens or those who have been resident in the UK for a period of 3 years or more. Some projects, which are funded by charities or by the universities themselves may have more stringent restrictions. Big data and machine learning for urban energy and sustainability assessment and designBig data modelling the knowledge economy, context-aware dynamic encoding for efficient big data processing, funded phd project (students worldwide). This project has funding attached, subject to eligibility criteria. Applications for the project are welcome from all suitably qualified candidates, but its funding may be restricted to a limited set of nationalities. You should check the project and department details for more information. AI to the rescue of climate change, modelling air quality for cleaner urban planningRepurposing and enriching cardiovascular risk prediction model to identify people at risk of cancer – ucl (part of health data research uk’s big data for complex disease driver programme), ontology and rule-based reasoning for intelligent manufacturing digital twin, fully funded 3-year ph.d. position in transportation engineering at the university of canterbury, new zealand, predicting cognitive and neurodegenerative outcomes from big data using automated retinal imaging analysis systems, leveraging electronic health records: bayesian joint longitudinal and survival modelling of bivariate longitudinal trajectories for two disease outcomes, perioperative brain state monitoring algorithms based on eeg big data and deep learning technology, responsible decentralised ai and big data analytics. FindAPhD. Copyright 2005-2024 All rights reserved. Unknown ( change ) Have you got time to answer some quick questions about PhD study? Select your nearest cityYou haven’t completed your profile yet. To get the most out of FindAPhD, finish your profile and receive these benefits: - Monthly chance to win one of ten £10 Amazon vouchers ; winners will be notified every month.*
- The latest PhD projects delivered straight to your inbox
- Access to our £6,000 scholarship competition
- Weekly newsletter with funding opportunities, research proposal tips and much more
- Early access to our physical and virtual postgraduate study fairs
Or begin browsing FindAPhD.com or begin browsing FindAPhD.com *Offer only available for the duration of your active subscription, and subject to change. You MUST claim your prize within 72 hours, if not we will redraw. 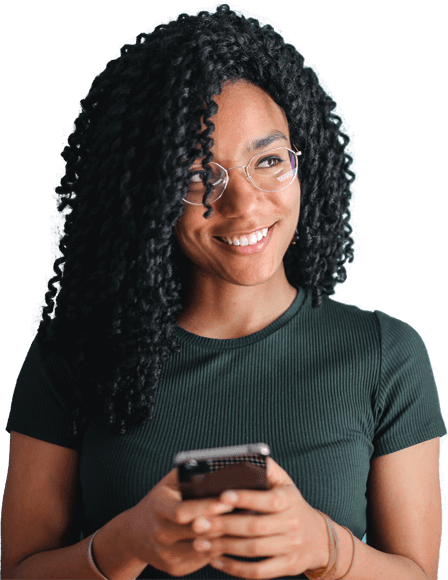 Do you want hassle-free information and advice?Create your FindAPhD account and sign up to our newsletter: - Find out about funding opportunities and application tips
- Receive weekly advice, student stories and the latest PhD news
- Hear about our upcoming study fairs
- Save your favourite projects, track enquiries and get personalised subject updates
 Create your accountLooking to list your PhD opportunities? Log in here . Filtering Results  BIG DATA MASTER THESISWhat is a master’s thesis? The master thesis is an innovative scholarship with the assistance and guidelines of the research professors and advisors. The master thesis and doctoral dissertation are more or less similar with a small difference such as the master thesis is shorter than the dissertation and is narrowly concentrated on the topic. We have well-experienced research experts in the field of big data . Using their experience below we have stated the notable big data master thesis topics as the reference for the research scholars. 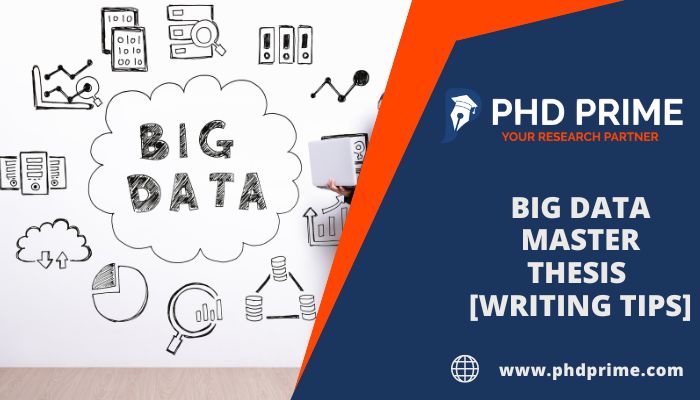 Latest Thesis and Research Topics in Big Data- Predictive analysis
- Big data analysis
- Big data maturity model
- Internet of things
- Big data virtualization
- SQL on Hadoop
- It is an interesting topic for master thesis and further research in big data using recent and classical research
- It is considered the real-time application result for business intelligence and big data
- It has applications in the areas such as customer service, finance, etc.
- It explores the fundamental details of the customers
- It is used to discover huge volume data sets to recognize the hidden patterns
- It deploys in the process of regulation and organizes the capabilities of big data
- It is beneficial in measuring the big data maturity
- The data partition has two types such as soft and hard
- There are different algorithms in data mining and big data
- It is used to divide the data into various sets using some techniques
- IoT devices are used to extract the connectivity among the devices and it is sued to sense the data
- IoT and big data work with the concurrence
- The tools in virtualization are used to regulate the process of data analytics
- The process produces some structures in the big data system
- It is used for the implementation process of the Hadoop platform with the accumulation of SQL-style querying systems
- It divides the work among Mapreduce such as the raw form of HDFS clusters and normal HDFS clusters
The above-mentioned are the notable trends in the contemporary big data period. The research scholars should select their big data master thesis topics from the latest research trends. For more research references in big data, the research scholars can reach us. Now, it’s time to discuss the several functions of the master thesis . Let us start with the importance of a master thesis. Is a master thesis important?The thesis is considered as the most important work because it is the innovative expertise of the researchers so it is particularly important. The master thesis is a chance for the researchers to show what the ability of the researcher is. The specialization of a master’s degree is to write the thesis and do projects in the degree stage . In the following, our research experts have explained the originality of the master thesis. Does a master’s thesis have to be original?The research students select their thesis topic on their own because that is directly relevant to their degree. The research topic from the students is the only essential part of the thesis and it is parallel to the common research papers. It is not essential to add any raw data on the topic if the process doesn’t take place. The aforementioned is about the significant features of the master thesis which is useful for the research scholars to develop their research in big data master thesis topics . In the following, our research experts have described the details of the specifications of the topic in the thesis. Finding topic - The research questions arise from various discussions and seminars. So, the researchers should collect their ideas to create an innovative research
- Researchers should focus on novel research ideas to provide the finest research topic and recreate the past research with various technologies
- Research students can offer innovative thesis topics for the research
Can you get a PhD without a master’s thesis?The master’s research program may have additional requirements for the researchers who are incomplete in their thesis work . The students who have completed their thesis are acceptable with their capstone project. Can you fail your master thesis? If the student fails a master’s thesis means it doesn’t mean that the student didn’t write the thesis. The failure takes place due to the student’s efforts in the thesis. The students can request the research supervisors verify the research thesis . This might help students that why they failed in their thesis. How many references should a master’s thesis have?In general, the master thesis should showcase a minimum of one secondary resource for every thousand words . This may vary in the doctoral thesis that every 150 words should have a secondary source. The research scholars can choose our research experts to start their research projects in big data. Additionally, our research experts offer the whole guidance for the big data master thesis topics and the guidance which starts from topic selection to the research code implementation process. For your reference, we have listed down the guideline for writing a thesis. Tips to write a thesis- The journal articles used to set the destination
- It helps to spot the research relevant to the topic
- Gaze the confirmation in the paper
- The article pages up to 25 and in some cases, it ends in 15 pages
- Set the deadline and allocate to work reach the aim
- The assignment writing will carry over until the researcher ends it
- Select the time and work according to that time allocation
As a result of this page, we believe that you have received some knowledge about the big data master thesis topics . Our research experts assist with your big data Dissertation such as the significant uses of methods, modifications in the protocols, appropriate research implementation, etc. So, the research scholar can reach us to reach better heights in research.  Opening Hours- Mon-Sat 09.00 am – 6.30 pm
- Lunch Time 12.30 pm – 01.30 pm
- Break Time 04.00 pm – 04.30 pm
- 18 years service excellence
- 40+ country reach
- 36+ university mou
- 194+ college mou
- 6000+ happy customers
- 100+ employees
- 240+ writers
- 60+ developers
- 45+ researchers
- 540+ Journal tieup
Payment Options Our Clients Social Links  Opening Time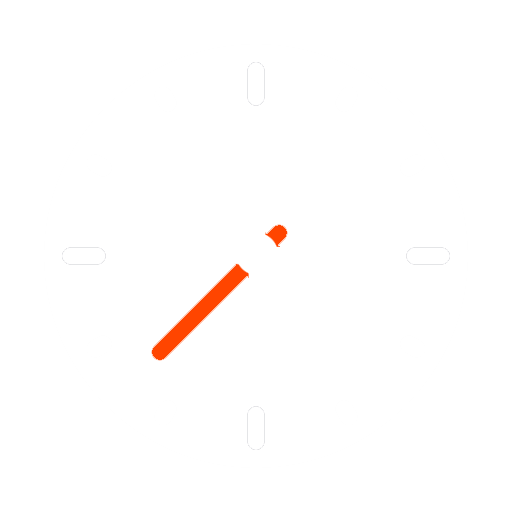 Closing Time- We follow Indian time zone
 - How It Works
- PhD thesis writing
- Master thesis writing
- Bachelor thesis writing
- Dissertation writing service
- Dissertation abstract writing
- Thesis proposal writing
- Thesis editing service
- Thesis proofreading service
- Thesis formatting service
- Coursework writing service
- Research paper writing service
- Architecture thesis writing
- Computer science thesis writing
- Engineering thesis writing
- History thesis writing
- MBA thesis writing
- Nursing dissertation writing
- Psychology dissertation writing
- Sociology thesis writing
- Statistics dissertation writing
- Buy dissertation online
- Write my dissertation
- Cheap thesis
- Cheap dissertation
- Custom dissertation
- Dissertation help
- Pay for thesis
- Pay for dissertation
- Senior thesis
- Write my thesis
214 Best Big Data Research Topics for Your Thesis Paper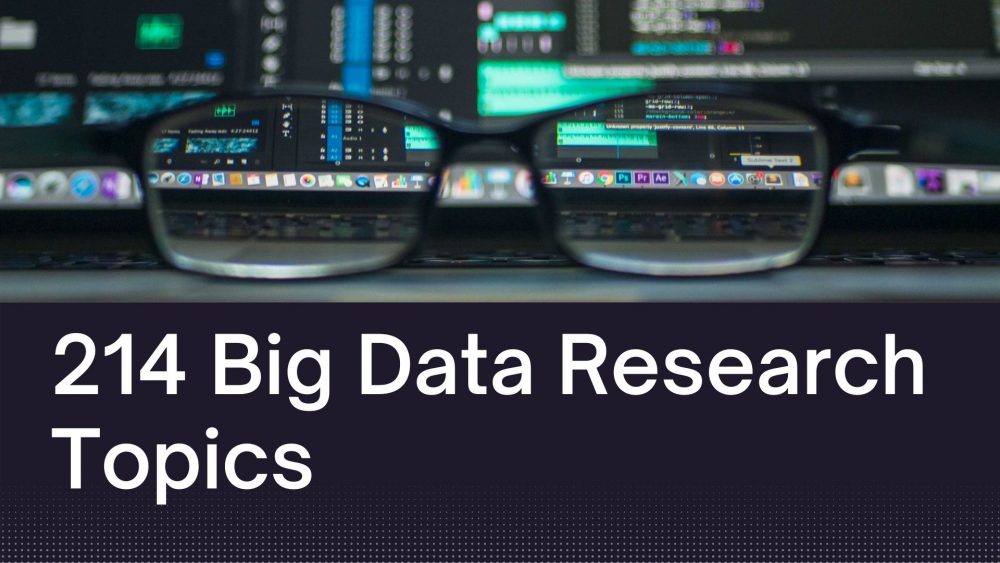 Finding an ideal big data research topic can take you a long time. Big data, IoT, and robotics have evolved. The future generations will be immersed in major technologies that will make work easier. Work that was done by 10 people will now be done by one person or a machine. This is amazing because, in as much as there will be job loss, more jobs will be created. It is a win-win for everyone. Big data is a major topic that is being embraced globally. Data science and analytics are helping institutions, governments, and the private sector. We will share with you the best big data research topics. On top of that, we can offer you the best writing tips to ensure you prosper well in your academics. As students in the university, you need to do proper research to get top grades. Hence, you can consult us if in need of research paper writing services. Big Data Analytics Research Topics for your Research ProjectAre you looking for an ideal big data analytics research topic? Once you choose a topic, consult your professor to evaluate whether it is a great topic. This will help you to get good grades. - Which are the best tools and software for big data processing?
- Evaluate the security issues that face big data.
- An analysis of large-scale data for social networks globally.
- The influence of big data storage systems.
- The best platforms for big data computing.
- The relation between business intelligence and big data analytics.
- The importance of semantics and visualization of big data.
- Analysis of big data technologies for businesses.
- The common methods used for machine learning in big data.
- The difference between self-turning and symmetrical spectral clustering.
- The importance of information-based clustering.
- Evaluate the hierarchical clustering and density-based clustering application.
- How is data mining used to analyze transaction data?
- The major importance of dependency modeling.
- The influence of probabilistic classification in data mining.
Interesting Big Data Analytics TopicsWho said big data had to be boring? Here are some interesting big data analytics topics that you can try. They are based on how some phenomena are done to make the world a better place. - Discuss the privacy issues in big data.
- Evaluate the storage systems of scalable in big data.
- The best big data processing software and tools.
- Data mining tools and techniques are popularly used.
- Evaluate the scalable architectures for parallel data processing.
- The major natural language processing methods.
- Which are the best big data tools and deployment platforms?
- The best algorithms for data visualization.
- Analyze the anomaly detection in cloud servers
- The scrutiny normally done for the recruitment of big data job profiles.
- The malicious user detection in big data collection.
- Learning long-term dependencies via the Fourier recurrent units.
- Nomadic computing for big data analytics.
- The elementary estimators for graphical models.
- The memory-efficient kernel approximation.
Big Data Latest Research TopicsDo you know the latest research topics at the moment? These 15 topics will help you to dive into interesting research. You may even build on research done by other scholars. - Evaluate the data mining process.
- The influence of the various dimension reduction methods and techniques.
- The best data classification methods.
- The simple linear regression modeling methods.
- Evaluate the logistic regression modeling.
- What are the commonly used theorems?
- The influence of cluster analysis methods in big data.
- The importance of smoothing methods analysis in big data.
- How is fraud detection done through AI?
- Analyze the use of GIS and spatial data.
- How important is artificial intelligence in the modern world?
- What is agile data science?
- Analyze the behavioral analytics process.
- Semantic analytics distribution.
- How is domain knowledge important in data analysis?
Big Data Debate TopicsIf you want to prosper in the field of big data, you need to try even hard topics. These big data debate topics are interesting and will help you to get a better understanding. - The difference between big data analytics and traditional data analytics methods.
- Why do you think the organization should think beyond the Hadoop hype?
- Does the size of the data matter more than how recent the data is?
- Is it true that bigger data are not always better?
- The debate of privacy and personalization in maintaining ethics in big data.
- The relation between data science and privacy.
- Do you think data science is a rebranding of statistics?
- Who delivers better results between data scientists and domain experts?
- According to your view, is data science dead?
- Do you think analytics teams need to be centralized or decentralized?
- The best methods to resource an analytics team.
- The best business case for investing in analytics.
- The societal implications of the use of predictive analytics within Education.
- Is there a need for greater control to prevent experimentation on social media users without their consent?
- How is the government using big data; for the improvement of public statistics or to control the population?
University Dissertation Topics on Big DataAre you doing your Masters or Ph.D. and wondering the best dissertation topic or thesis to do? Why not try any of these? They are interesting and based on various phenomena. While doing the research ensure you relate the phenomenon with the current modern society. - The machine learning algorithms are used for fall recognition.
- The divergence and convergence of the internet of things.
- The reliable data movements using bandwidth provision strategies.
- How is big data analytics using artificial neural networks in cloud gaming?
- How is Twitter accounts classification done using network-based features?
- How is online anomaly detection done in the cloud collaborative environment?
- Evaluate the public transportation insights provided by big data.
- Evaluate the paradigm for cancer patients using the nursing EHR to predict the outcome.
- Discuss the current data lossless compression in the smart grid.
- How does online advertising traffic prediction helps in boosting businesses?
- How is the hyperspectral classification done using the multiple kernel learning paradigm?
- The analysis of large data sets downloaded from websites.
- How does social media data help advertising companies globally?
- Which are the systems recognizing and enforcing ownership of data records?
- The alternate possibilities emerging for edge computing.
The Best Big Data Analysis Research Topics and EssaysThere are a lot of issues that are associated with big data. Here are some of the research topics that you can use in your essays. These topics are ideal whether in high school or college. - The various errors and uncertainty in making data decisions.
- The application of big data on tourism.
- The automation innovation with big data or related technology
- The business models of big data ecosystems.
- Privacy awareness in the era of big data and machine learning.
- The data privacy for big automotive data.
- How is traffic managed in defined data center networks?
- Big data analytics for fault detection.
- The need for machine learning with big data.
- The innovative big data processing used in health care institutions.
- The money normalization and extraction from texts.
- How is text categorization done in AI?
- The opportunistic development of data-driven interactive applications.
- The use of data science and big data towards personalized medicine.
- The programming and optimization of big data applications.
The Latest Big Data Research Topics for your Research ProposalDoing a research proposal can be hard at first unless you choose an ideal topic. If you are just diving into the big data field, you can use any of these topics to get a deeper understanding. - The data-centric network of things.
- Big data management using artificial intelligence supply chain.
- The big data analytics for maintenance.
- The high confidence network predictions for big biological data.
- The performance optimization techniques and tools for data-intensive computation platforms.
- The predictive modeling in the legal context.
- Analysis of large data sets in life sciences.
- How to understand the mobility and transport modal disparities sing emerging data sources?
- How do you think data analytics can support asset management decisions?
- An analysis of travel patterns for cellular network data.
- The data-driven strategic planning for citywide building retrofitting.
- How is money normalization done in data analytics?
- Major techniques used in data mining.
- The big data adaptation and analytics of cloud computing.
- The predictive data maintenance for fault diagnosis.
Interesting Research Topics on A/B Testing In Big DataA/B testing topics are different from the normal big data topics. However, you use an almost similar methodology to find the reasons behind the issues. These topics are interesting and will help you to get a deeper understanding. - How is ultra-targeted marketing done?
- The transition of A/B testing from digital to offline.
- How can big data and A/B testing be done to win an election?
- Evaluate the use of A/B testing on big data
- Evaluate A/B testing as a randomized control experiment.
- How does A/B testing work?
- The mistakes to avoid while conducting the A/B testing.
- The most ideal time to use A/B testing.
- The best way to interpret results for an A/B test.
- The major principles of A/B tests.
- Evaluate the cluster randomization in big data
- The best way to analyze A/B test results and the statistical significance.
- How is A/B testing used in boosting businesses?
- The importance of data analysis in conversion research
- The importance of A/B testing in data science.
Amazing Research Topics on Big Data and Local GovernmentsGovernments are now using big data to make the lives of the citizens better. This is in the government and the various institutions. They are based on real-life experiences and making the world better. - Assess the benefits and barriers of big data in the public sector.
- The best approach to smart city data ecosystems.
- The big analytics used for policymaking.
- Evaluate the smart technology and emergence algorithm bureaucracy.
- Evaluate the use of citizen scoring in public services.
- An analysis of the government administrative data globally.
- The public values are found in the era of big data.
- Public engagement on local government data use.
- Data analytics use in policymaking.
- How are algorithms used in public sector decision-making?
- The democratic governance in the big data era.
- The best business model innovation to be used in sustainable organizations.
- How does the government use the collected data from various sources?
- The role of big data for smart cities.
- How does big data play a role in policymaking?
Easy Research Topics on Big DataWho said big data topics had to be hard? Here are some of the easiest research topics. They are based on data management, research, and data retention. Pick one and try it! - Who uses big data analytics?
- Evaluate structure machine learning.
- Explain the whole deep learning process.
- Which are the best ways to manage platforms for enterprise analytics?
- Which are the new technologies used in data management?
- What is the importance of data retention?
- The best way to work with images is when doing research.
- The best way to promote research outreach is through data management.
- The best way to source and manage external data.
- Does machine learning improve the quality of data?
- Describe the security technologies that can be used in data protection.
- Evaluate token-based authentication and its importance.
- How can poor data security lead to the loss of information?
- How to determine secure data.
- What is the importance of centralized key management?
Unique IoT and Big Data Research TopicsInternet of Things has evolved and many devices are now using it. There are smart devices, smart cities, smart locks, and much more. Things can now be controlled by the touch of a button. - Evaluate the 5G networks and IoT.
- Analyze the use of Artificial intelligence in the modern world.
- How do ultra-power IoT technologies work?
- Evaluate the adaptive systems and models at runtime.
- How have smart cities and smart environments improved the living space?
- The importance of the IoT-based supply chains.
- How does smart agriculture influence water management?
- The internet applications naming and identifiers.
- How does the smart grid influence energy management?
- Which are the best design principles for IoT application development?
- The best human-device interactions for the Internet of Things.
- The relation between urban dynamics and crowdsourcing services.
- The best wireless sensor network for IoT security.
- The best intrusion detection in IoT.
- The importance of big data on the Internet of Things.
Big Data Database Research Topics You Should TryBig data is broad and interesting. These big data database research topics will put you in a better place in your research. You also get to evaluate the roles of various phenomena. - The best cloud computing platforms for big data analytics.
- The parallel programming techniques for big data processing.
- The importance of big data models and algorithms in research.
- Evaluate the role of big data analytics for smart healthcare.
- How is big data analytics used in business intelligence?
- The best machine learning methods for big data.
- Evaluate the Hadoop programming in big data analytics.
- What is privacy-preserving to big data analytics?
- The best tools for massive big data processing
- IoT deployment in Governments and Internet service providers.
- How will IoT be used for future internet architectures?
- How does big data close the gap between research and implementation?
- What are the cross-layer attacks in IoT?
- The influence of big data and smart city planning in society.
- Why do you think user access control is important?
Big Data Scala Research TopicsScala is a programming language that is used in data management. It is closely related to other data programming languages. Here are some of the best scala questions that you can research. - Which are the most used languages in big data?
- How is scala used in big data research?
- Is scala better than Java in big data?
- How is scala a concise programming language?
- How does the scala language stream process in real-time?
- Which are the various libraries for data science and data analysis?
- How does scala allow imperative programming in data collection?
- Evaluate how scala includes a useful REPL for interaction.
- Evaluate scala’s IDE support.
- The data catalog reference model.
- Evaluate the basics of data management and its influence on research.
- Discuss the behavioral analytics process.
- What can you term as the experience economy?
- The difference between agile data science and scala language.
- Explain the graph analytics process.
Independent Research Topics for Big DataThese independent research topics for big data are based on the various technologies and how they are related. Big data will greatly be important for modern society. - The biggest investment is in big data analysis.
- How are multi-cloud and hybrid settings deep roots?
- Why do you think machine learning will be in focus for a long while?
- Discuss in-memory computing.
- What is the difference between edge computing and in-memory computing?
- The relation between the Internet of things and big data.
- How will digital transformation make the world a better place?
- How does data analysis help in social network optimization?
- How will complex big data be essential for future enterprises?
- Compare the various big data frameworks.
- The best way to gather and monitor traffic information using the CCTV images
- Evaluate the hierarchical structure of groups and clusters in the decision tree.
- Which are the 3D mapping techniques for live streaming data.
- How does machine learning help to improve data analysis?
- Evaluate DataStream management in task allocation.
- How is big data provisioned through edge computing?
- The model-based clustering of texts.
- The best ways to manage big data.
- The use of machine learning in big data.
Is Your Big Data Thesis Giving You Problems?These are some of the best topics that you can use to prosper in your studies. Not only are they easy to research but also reflect on real-time issues. Whether in University or college, you need to put enough effort into your studies to prosper. However, if you have time constraints, we can provide professional writing help. Are you looking for online expert writers? Look no further, we will provide quality work at a cheap price. 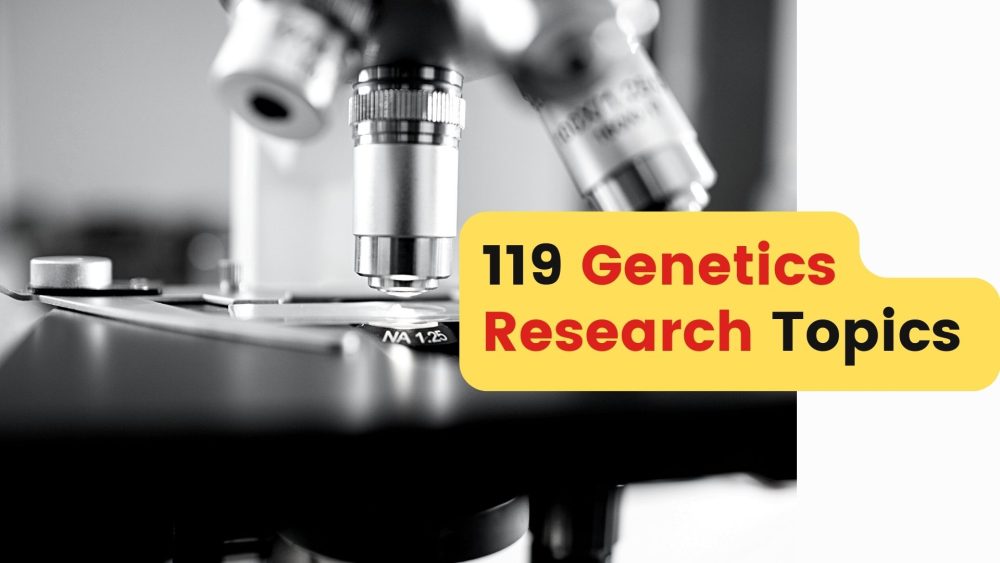 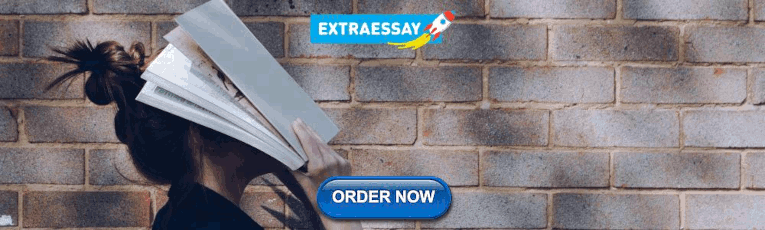 Leave a Reply Cancel replyYour email address will not be published. Required fields are marked * Comment * Error message Name * Error message Email * Error message Save my name, email, and website in this browser for the next time I comment. As Putin continues killing civilians, bombing kindergartens, and threatening WWIII, Ukraine fights for the world's peaceful future. Ukraine Live Updates Beware of fake email, SMS and WhatsApp messages: check before clicking. Read more 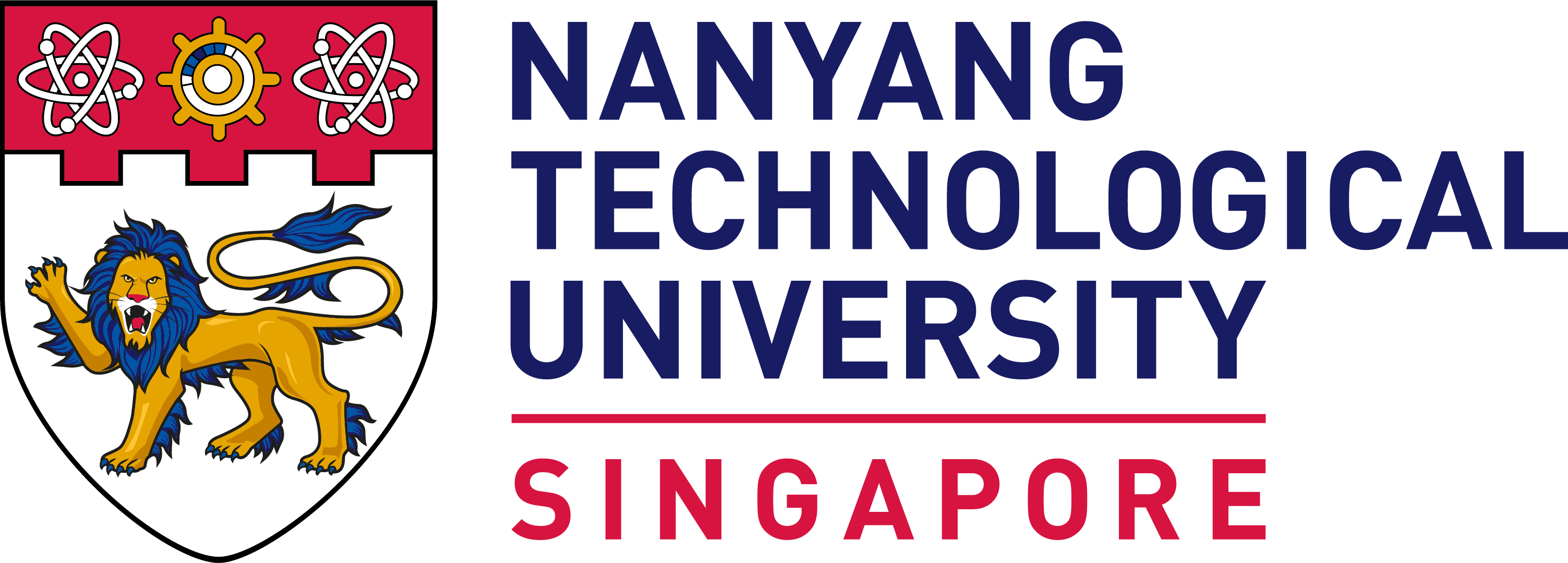 College of Computing and Data ScienceHow can we help you.  Financial Matters Student Exchange Student Life Overseas exchanges Course finder Alumni events Alumni stories Professional development Alumni discounts Research Focus/enri-thumbnails/careeropportunities1f0caf1c-a12d-479c-be7c-3c04e085c617.tmb-mega-menu.jpg?Culture=en&sfvrsn=d7261e3b_1) Research Hub Academic partnersResearch collaborations. 7e6fdc03-9018-4d08-9a98-8a21acbc37ba.tmb-mega-menu.jpg?Culture=en&sfvrsn=7deaf618_1) Information for Suppliers Suppliers User Guide for AribaCcds outstanding phd thesis award 2024, congratulations to the following phd graduates for their achievement, for contributions to building generalizable solutions that can enhance the capabilities and applicability of aiot systems..  Dr XU Huatao Building Generalizable Deep Learning Solutions for Mobile Sensing This thesis signifies a significant leap in mobile sensing with deep learning. It introduces LIMU-BERT, a pioneering sensor foundation model adaptable to various applications, and integrates it into UniHAR, a universal learning framework that trains models across domains using physics-informed data augmentation. A notable innovation is 'Penetrative AI,' the first-ever application of Large Language Models (LLMs) like ChatGPT for processing IoT sensor signals. This breakthrough enables LLMs to interact with the physical world, laying the groundwork for generalizable IoT solutions. The thesis's excellence is recognized by the SenSys 2021 best paper runner-up award and the GetMobile 2022 research highlight. Its models have gained nationwide adoption in Eleme, China's second-largest food delivery service. It also has sparked widespread discussion on social media. Altogether, this work substantially enriches the mobile sensing field, expanding both the scope and effectiveness of AIoT systems in practical applications. for contributions to advancing graph deep learning through innovative benchmarks, neural network architectures, and scalable frameworks Dr DWIVEDI Vijay Prakash Deep Learning for Graph Structured Data This thesis marks a significant advancement in deep learning for graph-structured data which are ubiquitous in domains such as drug discovery, social networks, medicine and transportation. Addressing the inadequacies of traditional deep learning approaches for such data, the thesis introduces comprehensive benchmarks for assessing Graph Neural Networks (GNNs) across varied domains. A key contribution is the extension of Transformer networks, fundamental to ChatGPT, to graph domains, integrating graph-based inductive biases and positional encodings, thereby enhancing expressivity and generalization. His work also proposes novel techniques for learning distinct structural and positional representations in GNNs, boosting model capacities. Further, he develops scalable Graph Transformers that can adapt to massive graphs with billions of edge connections, employing efficient local and global graph representations and fast neighborhood sampling. Overall, this thesis paves the way for the application of GNNs in complex real-world relational data scenarios, significantly contributing to the field of graph representation learning. for contributions to systems addressing efficiency and practicality issues of ML model tuning, training, scheduling, and deployment in large-scale clusters. Dr HU Qinghao Building Efficient and Practical Machine Learning Systems Emerging ML technologies have empowered transformative applications, such as ChatGPT and Stable Diffusion. These breakthroughs heavily rely on advanced system support, encompassing training frameworks and cluster schedulers. However, as ML workloads proliferate and billion-scale models surface, current systems fail to handle them efficiently. Qinghao’s thesis focuses on addressing efficiency and practicality issues with ML-tailored system designs. His research expands along two lines: (1) Efficiency. He pioneers system optimizations for both cluster and job levels. His ground-breaking work is the first to facilitate hyperparameter tuning for large models such as GPT-3. Through novel model scaling, fusion, and interleaving, he achieves two orders of magnitude acceleration. (2) Practicality. Most existing work targets excellent system performance while ignoring its complexity and usability. Qinghao first attains the state-of-the-art performance under the non-intrusive design principle in cluster scheduling systems. Besides, he crafts a unified framework to achieve transparent, performant and lightweight systems. Prof Loy Chen Change (Jury Chair) Assoc Prof Tang Xueyan Assoc Prof Lam Siew Kei Assoc Prof Zhang Hanwang Related stories Team NTU Celebrates Third Place Win in Online Component of ISC24 Student Cluster Competition at Hamburg, Germany Global Essay Competition Winners at the 53rd St. Gallen Symposium 2024 Three Innovative Application of AI Awards from AAAI 2024a57ab68f-1049-4be2-a83a-d3821af9aeee.tmb-listing.jpg?Culture=en&sfvrsn=e59ee71c_1) SCSE Team wins Distinguished Paper Award at Artificial Intelligence System with Confidential Computing (AISCC 2024) 2023 Google PhD Fellowship SCSE graduate Dr Emadeldeen Eldele received the 3rd Prize in the 2023 IEEE Engineering in Medicine and Biology Prize Paper Award- Our Promise
- Our Achievements
- Our Mission
- Proposal Writing
- System Development
- Paper Writing
- Paper Publish
- Synopsis Writing
- Thesis Writing
- Assignments
- Survey Paper
- Conference Paper
- Journal Paper
- Empirical Paper
- Journal Support
- PhD Thesis on Big Data Analytics
PhD Thesis on Big Data Analytics is a thesis link where PhD scholars can hold their unique thesis in the latest trend. In big data analytics, thesis completion is a big thing for PhD beginners. Since it is a complex process due to the variety of data analysis, in this case, our PhD thesis on Big Data Analytics is the best solution for it. To deal with the BIG DATA, you will need our BIG SERVICE. It translates our insights to the research scholars over 15+ years…. Assets of our PhD Thesis on Big Data analytics- R&D team with enthusiastic techies update all latest research tendencies
- Skilled research analysts with in-depth knowledge in every aspect of big data
- Striving to break all prior researches with our innovative approaches
- Self-motivated technical writers to write your thesis within your time boundary
- As an illustration, access to all academic databases
We served the 1000+ thesis for PhD scholars in the field of Big Data without any error. You just plan the format of your thesis with your university or supervisor. If you do not have the format, no problem, we can customize your thesis according to our best structure. From our experience, we will know that every PhD scholar will trap their thesis due to its page length and reference count matters. Without good exposure, one couldn’t satisfy both constraints, especially in big data. Are you trapping with your thesis? Bind with us; we will be your healing agent… Understand our Big Data analyticsBig data can probably define in the 3V’s, but today we will find the big data is a 10v’s. At the present time, it is described in all the fields. Besides, it will change the entire world into smart cities. As a matter of fact, most of the scholars will prefer big data. Now, let’s check those terms (10v’s) in big data, - Variability
- Vulnerability
- Visualization
Our Big Data Analytics tools for your researchConsidering the current trend in big data, we will make the team for it. In addition, our experts have 18 years of field practice in several trends. Furthermore, our project experts are experts and skill in all the data tools. So we are capable of working on both the big data models and tools offering PhD thesis on big data analytics . For example, - Hadoop with AWS
- Microsoft Azure
- Google Cloud Platform
Our momentous data processing tools are,- Apache Hadoop
- Spark Apache
- Apache Storm
- Apache SAMOA
Here fewer auspicious thesis topics in big data are enlisted,- Elastic cloud/fog design
- Best practices for data acquisition, fusion, and cleaning
- Big data visualization
- New programming model beyond Hadoop/MapReduce, STORM
- Large scale recommender system
- Statistical, Neural, Reinforcement, and multi-learning strategies
- Automated data extraction and semantic processing
- Data security using blockchain technology
- Interoperability of heterogeneous data in the cloud platform
- Big data analytics in industrial environments
To sum up, start your PhD thesis with us to save your precious time because you deserve it… A little progress each day adds up to big results… MILESTONE 1: Research ProposalFinalize journal (indexing). Before sit down to research proposal writing, we need to decide exact journals. For e.g. SCI, SCI-E, ISI, SCOPUS. Research Subject SelectionAs a doctoral student, subject selection is a big problem. Phdservices.org has the team of world class experts who experience in assisting all subjects. When you decide to work in networking, we assign our experts in your specific area for assistance. Research Topic SelectionWe helping you with right and perfect topic selection, which sound interesting to the other fellows of your committee. For e.g. if your interest in networking, the research topic is VANET / MANET / any other Literature Survey WritingTo ensure the novelty of research, we find research gaps in 50+ latest benchmark papers (IEEE, Springer, Elsevier, MDPI, Hindawi, etc.) Case Study WritingAfter literature survey, we get the main issue/problem that your research topic will aim to resolve and elegant writing support to identify relevance of the issue. Problem StatementBased on the research gaps finding and importance of your research, we conclude the appropriate and specific problem statement. Writing Research ProposalWriting a good research proposal has need of lot of time. We only span a few to cover all major aspects (reference papers collection, deficiency finding, drawing system architecture, highlights novelty) MILESTONE 2: System DevelopmentFix implementation plan. We prepare a clear project implementation plan that narrates your proposal in step-by step and it contains Software and OS specification. We recommend you very suitable tools/software that fit for your concept. Tools/Plan ApprovalWe get the approval for implementation tool, software, programing language and finally implementation plan to start development process. Pseudocode DescriptionOur source code is original since we write the code after pseudocodes, algorithm writing and mathematical equation derivations. Develop Proposal IdeaWe implement our novel idea in step-by-step process that given in implementation plan. We can help scholars in implementation. Comparison/ExperimentsWe perform the comparison between proposed and existing schemes in both quantitative and qualitative manner since it is most crucial part of any journal paper. Graphs, Results, Analysis TableWe evaluate and analyze the project results by plotting graphs, numerical results computation, and broader discussion of quantitative results in table. Project DeliverablesFor every project order, we deliver the following: reference papers, source codes screenshots, project video, installation and running procedures. MILESTONE 3: Paper WritingChoosing right format. We intend to write a paper in customized layout. If you are interesting in any specific journal, we ready to support you. Otherwise we prepare in IEEE transaction level. Collecting Reliable ResourcesBefore paper writing, we collect reliable resources such as 50+ journal papers, magazines, news, encyclopedia (books), benchmark datasets, and online resources. Writing Rough DraftWe create an outline of a paper at first and then writing under each heading and sub-headings. It consists of novel idea and resources Proofreading & FormattingWe must proofread and formatting a paper to fix typesetting errors, and avoiding misspelled words, misplaced punctuation marks, and so on Native English WritingWe check the communication of a paper by rewriting with native English writers who accomplish their English literature in University of Oxford. Scrutinizing Paper QualityWe examine the paper quality by top-experts who can easily fix the issues in journal paper writing and also confirm the level of journal paper (SCI, Scopus or Normal). Plagiarism CheckingWe at phdservices.org is 100% guarantee for original journal paper writing. We never use previously published works. MILESTONE 4: Paper PublicationFinding apt journal. We play crucial role in this step since this is very important for scholar’s future. Our experts will help you in choosing high Impact Factor (SJR) journals for publishing. Lay Paper to SubmitWe organize your paper for journal submission, which covers the preparation of Authors Biography, Cover Letter, Highlights of Novelty, and Suggested Reviewers. Paper SubmissionWe upload paper with submit all prerequisites that are required in journal. We completely remove frustration in paper publishing. Paper Status TrackingWe track your paper status and answering the questions raise before review process and also we giving you frequent updates for your paper received from journal. Revising Paper PreciselyWhen we receive decision for revising paper, we get ready to prepare the point-point response to address all reviewers query and resubmit it to catch final acceptance. Get Accept & e-ProofingWe receive final mail for acceptance confirmation letter and editors send e-proofing and licensing to ensure the originality. Publishing PaperPaper published in online and we inform you with paper title, authors information, journal name volume, issue number, page number, and DOI link MILESTONE 5: Thesis WritingIdentifying university format. We pay special attention for your thesis writing and our 100+ thesis writers are proficient and clear in writing thesis for all university formats. Gathering Adequate ResourcesWe collect primary and adequate resources for writing well-structured thesis using published research articles, 150+ reputed reference papers, writing plan, and so on. Writing Thesis (Preliminary)We write thesis in chapter-by-chapter without any empirical mistakes and we completely provide plagiarism-free thesis. Skimming & ReadingSkimming involve reading the thesis and looking abstract, conclusions, sections, & sub-sections, paragraphs, sentences & words and writing thesis chorological order of papers. Fixing Crosscutting IssuesThis step is tricky when write thesis by amateurs. Proofreading and formatting is made by our world class thesis writers who avoid verbose, and brainstorming for significant writing. Organize Thesis ChaptersWe organize thesis chapters by completing the following: elaborate chapter, structuring chapters, flow of writing, citations correction, etc. Writing Thesis (Final Version)We attention to details of importance of thesis contribution, well-illustrated literature review, sharp and broad results and discussion and relevant applications study. How PhDservices.org deal with significant issues ?1. novel ideas. Novelty is essential for a PhD degree. Our experts are bringing quality of being novel ideas in the particular research area. It can be only determined by after thorough literature search (state-of-the-art works published in IEEE, Springer, Elsevier, ACM, ScienceDirect, Inderscience, and so on). SCI and SCOPUS journals reviewers and editors will always demand “Novelty” for each publishing work. Our experts have in-depth knowledge in all major and sub-research fields to introduce New Methods and Ideas. MAKING NOVEL IDEAS IS THE ONLY WAY OF WINNING PHD. 2. Plagiarism-FreeTo improve the quality and originality of works, we are strictly avoiding plagiarism since plagiarism is not allowed and acceptable for any type journals (SCI, SCI-E, or Scopus) in editorial and reviewer point of view. We have software named as “Anti-Plagiarism Software” that examines the similarity score for documents with good accuracy. We consist of various plagiarism tools like Viper, Turnitin, Students and scholars can get your work in Zero Tolerance to Plagiarism. DONT WORRY ABOUT PHD, WE WILL TAKE CARE OF EVERYTHING. 3. Confidential InfoWe intended to keep your personal and technical information in secret and it is a basic worry for all scholars. - Technical Info: We never share your technical details to any other scholar since we know the importance of time and resources that are giving us by scholars.
- Personal Info: We restricted to access scholars personal details by our experts. Our organization leading team will have your basic and necessary info for scholars.
CONFIDENTIALITY AND PRIVACY OF INFORMATION HELD IS OF VITAL IMPORTANCE AT PHDSERVICES.ORG. WE HONEST FOR ALL CUSTOMERS. 4. PublicationMost of the PhD consultancy services will end their services in Paper Writing, but our PhDservices.org is different from others by giving guarantee for both paper writing and publication in reputed journals. With our 18+ year of experience in delivering PhD services, we meet all requirements of journals (reviewers, editors, and editor-in-chief) for rapid publications. From the beginning of paper writing, we lay our smart works. PUBLICATION IS A ROOT FOR PHD DEGREE. WE LIKE A FRUIT FOR GIVING SWEET FEELING FOR ALL SCHOLARS. 5. No DuplicationAfter completion of your work, it does not available in our library i.e. we erased after completion of your PhD work so we avoid of giving duplicate contents for scholars. This step makes our experts to bringing new ideas, applications, methodologies and algorithms. Our work is more standard, quality and universal. Everything we make it as a new for all scholars. INNOVATION IS THE ABILITY TO SEE THE ORIGINALITY. EXPLORATION IS OUR ENGINE THAT DRIVES INNOVATION SO LET’S ALL GO EXPLORING. Client ReviewsI ordered a research proposal in the research area of Wireless Communications and it was as very good as I can catch it. I had wishes to complete implementation using latest software/tools and I had no idea of where to order it. My friend suggested this place and it delivers what I expect. It really good platform to get all PhD services and I have used it many times because of reasonable price, best customer services, and high quality. My colleague recommended this service to me and I’m delighted their services. They guide me a lot and given worthy contents for my research paper. I’m never disappointed at any kind of service. Till I’m work with professional writers and getting lot of opportunities. - Christopher Once I am entered this organization I was just felt relax because lots of my colleagues and family relations were suggested to use this service and I received best thesis writing. I recommend phdservices.org. They have professional writers for all type of writing (proposal, paper, thesis, assignment) support at affordable price. You guys did a great job saved more money and time. I will keep working with you and I recommend to others also. These experts are fast, knowledgeable, and dedicated to work under a short deadline. I had get good conference paper in short span. Guys! You are the great and real experts for paper writing since it exactly matches with my demand. I will approach again. I am fully satisfied with thesis writing. Thank you for your faultless service and soon I come back again. Trusted customer service that you offer for me. I don’t have any cons to say. I was at the edge of my doctorate graduation since my thesis is totally unconnected chapters. You people did a magic and I get my complete thesis!!! - Abdul Mohammed Good family environment with collaboration, and lot of hardworking team who actually share their knowledge by offering PhD Services. I enjoyed huge when working with PhD services. I was asked several questions about my system development and I had wondered of smooth, dedication and caring. I had not provided any specific requirements for my proposal work, but you guys are very awesome because I’m received proper proposal. Thank you! - Bhanuprasad I was read my entire research proposal and I liked concept suits for my research issues. Thank you so much for your efforts. - Ghulam Nabi I am extremely happy with your project development support and source codes are easily understanding and executed. Hi!!! You guys supported me a lot. Thank you and I am 100% satisfied with publication service. - Abhimanyu I had found this as a wonderful platform for scholars so I highly recommend this service to all. I ordered thesis proposal and they covered everything. Thank you so much!!! Related PagesPhd Thesis On Network Communication Phd Projects In Information Forensics Security Buy Phd Thesis Online Phd Thesis On Electronics Phd Projects In Iot Internet Of Things Phd Projects In Information Security Phd Thesis On Data Mining Phd Thesis On Image Processing Phd Projects In Information Technology Best Phd Thesis Writing Service Phd Projects In Iot Mqtt Phd Projects In Internet Computing Phd Thesis Consultants In India Phd Research Topics In Iot Phd Thesis On Internet Of Things  - UTHEALTH HOME
- INSIDE THE UNIVERSITY Secured Page
- Prospective Students
- New Students
- Current Students
- Faculty & Staff
- Continuing Education
- MSBMI Intranet
Search UTHealth HoustonDecoding disease mechanisms: unraveling large-scale biological data for novel therapeutic target discovery. Author: Yan Chu (2024) Advisory Committee: W. Jim Zheng, PhD PhD thesis: McWilliams School of Biomedical Informatics at UTHealth Houston. This dissertation leverages large biological datasets and data-driven research to enhance our understanding of complex human diseases, deploying a unified narrative across three interconnected bioinformatics studies. Each study targets a different biological level, including genes, proteins, cells, and tissues, to uncover disease mechanisms and speed up the discovery of potential therapeutic targets. The first study addresses gene-level analysis in the context of bladder cancer. It integrates gene expression data from lab models with clinical survival data to identify new therapeutic targets. This research pinpoints the IL6/JAK/STAT3 signaling pathway as a promising target for treating SMARCB1-deficient bladder cancer, demonstrating how combining various data types can lead to significant discoveries in cancer therapeutic targets. Moving to the protein level, the second study focuses on Alzheimer’s disease and explores how alternative splicing, a process by which a single gene can lead to multiple protein forms, affects protein structure and disease development. Employing the cuttingedge AlphaFold 2 technology alongside robust statistical analyses, this study identifies specific changes in the Tau protein that are potentially linked to Alzheimer's. The findings suggest new paths for understanding and treating the disease, emphasizing the importance of structural protein studies in biomedical research. The third study broadens the scope to cellular and tissue levels, utilizing advanced statistical and explainable artificial intelligence (AI) models to analyze spatial transcriptomics and single-cell RNA sequencing data. At the cellular level, the use of AI helps decode complex gene-gene interactions related to Alzheimer's disease from intricate neural network analyses, paving the way for novel therapeutic approaches. Extending these techniques to tissue level, the research examines how aging impacts gene expression in acute pancreatitis. By integrating spatial and single-cell data, it identifies key gene expressions linked to aging effects, enhancing our understanding of age-related changes in disease processes. In conclusion, this dissertation employs sophisticated bioinformatics methodologies across multiple studies to provide new insights into the mechanisms of human diseases at the gene, protein, cell, and tissue levels. It showcases the effective use of diverse data types for cancer therapy development, employs high-performance computing to reveal the impacts of protein splicing on disease, and utilizes explainable AI to unravel complex gene interactions. Collectively, these efforts advance the field of bioinformatics and open up new avenues for the discovery of therapeutic targets and strategies, illustrating the transformative potential of integrating advanced computational tools in biomedical research. The OECD: Better policies for better lives Select a languageThe Organisation for Economic Co-operation and Development (OECD) is an international organisation that works to build better policies for better lives. We draw on more than 60 years of experience and insights to shape policies that foster prosperity and opportunity, underpinned by equality and well-being. We work closely with policy makers, stakeholders and citizens to establish evidence-based international standards and to find solutions to social, economic and environmental challenges. From improving economic performance and strengthening policies to fight climate change to bolstering education and fighting international tax evasion, the OECD is a unique forum and knowledge hub for data, analysis and best practices in public policy. Our core aim is to provide advice on international standard-setting – and help countries forge a path towards stronger, fairer and cleaner societies. Mathias Cormann Secretary-General | OECD  "The OECD is a force for good in the world. All of us have a collective responsibility to use it to its full potential. Our core purpose, under our Convention, is to preserve individual liberty and to increase the economic and social well-being of our people. Our essential mission of the past – to promote stronger, cleaner, fairer economic growth and to raise employment and living standards – remains the critically important mission for the future." Get to know the OECD- Our history The OECD is an intergovernmental organisation founded in 1961 to advise governments on how to deliver better policies for better lives. Learn more
- How we work The OECD informs policies and creates global standards through multi-stakeholder collaboration and intensive peer learning. Learn more
- Organisational structure The Council, more than 300 committees and the Secretariat work together to determine the output of the OECD. Learn more
- Members and partners The OECD’s member countries and partners work on key global policy challenges to help drive and anchor reform around the world. Learn more
- Budget Member countries’ national contributions and voluntary contributions help to support the OECD’s programme of work. Learn more
- Locations The OECD has two main sites: one in its Paris Headquarters and another one right next to Paris in Boulogne-Billancourt. Learn more
Engage with usNewslettersGet the latest from the OECD on a range of topics straight to your inbox  Learn about working at the OECD and discover our latest job vacancies  Strategic documents - Trust in Global Cooperation: The vision for the OECD for the next decade
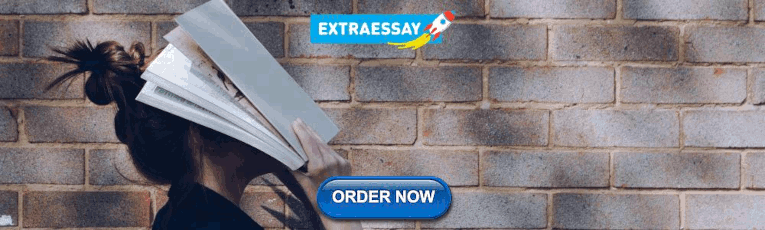 |
IMAGES
VIDEO
COMMENTS
The Big Data Analytics PhD program consists of at least 72 credit hours of course work beyond the Bachelor's degree, of which a minimum of 42 hours of formal course work, exclusive of independent study, and 15 credit hours of dissertation research (STA 7980) are required. The program requires 15 hours of elective courses.
The Big Data Analytics PhD program consists of at least 72 credit hours of course work beyond the Bachelor's degree, of which a minimum of 42 hours of formal course work, exclusive of independent study, and 15 credit hours of dissertation research (STA 7980) are required. The program requires 15 hours of elective courses.
The Evolution of Big Data and Its Business Applications. Doctor of Philosophy (Information Science), May 2018, 113 pp., 12 tables, 10 figures, references, 203 titles. The arrival of the Big Data era has become a major topic of discussion in many sectors because of the premises of big data utilizations and its impact on decisionmaking. It is an -
Thesis Title: Investigating the Impact of Big Data Analytics on Supply Chain Operations: Case Studies from the UK Private Sector A thesis submitted for the degree of Doctor of Philosophy By Ruaa Hasan Brunel Business School Brunel University London 2021 . 2 | P a g e
2. implementation strategies of big data analytics can result in selecting the wrong technologies and skillsets (Matthias et al., 2017). Big data technology enables organizational leaders to have the ability to access, diagnose, and integrate the necessary information gathered through various data sources.
This doctoral thesis examines the rise of Big Data as a complex sociotechnical phenomenon. How is it that the notion of Big Data could gain so much traction, becoming the subject of ... more reflexive engagement with the potential pitfalls of Big Data-based technologies. The thesis objective is to contribute to a better understanding of the ...
The PhD-DS program will prepare you for research spanning big data analytics, artificial intelligence, and data-driven decision-making by exploring each stage of the data science life cycle in depth from an applied and a theoretical perspective. ... A PhD dissertation is a scholarly documentation of research that makes an original contribution ...
Optimizing Big Data Analytics Frameworks in Geographically Distributed Datacenters by Shuhao Liu A thesis submitted in conformity with the requirements for the degree of Doctor of Philosophy The Edward S. Rogers Sr. Department of Electrical and Computer Engineering University of Toronto
Program Overview. The Doctor of Computer Science with a concentration in Big Data Analytics (DCS-BDA) is a 100-credit-hour terminal degree in computer science. The program includes 40 credit hours of core management courses, 20 credit hours of big data analytics concentration courses, 4 credit hours of symposium-related courses, and 36 credit ...
Computational and Data Sciences (PhD) Dissertations. Below is a selection of dissertations from the Doctor of Philosophy in Computational and Data Sciences program in Schmid College that have been included in Chapman University Digital Commons. Additional dissertations from years prior to 2019 are available through the Leatherby Libraries ...
Cloud-based Machine Learning Architecture for Big Data Analysis Rezvan Pakdel MSC 113220306 Thesis submitted for the degree of Doctor of Philosophy
High-dimensional data are now widely collected in many areas to make scienti c discoveries or build complicated predictive models. The high dimensionality of such data requires analyses to have greater exibility in modeling while ensuring the re-producibility of discoveries. This thesis contains three self-contained chapters that
The document discusses the challenges of writing a PhD thesis on big data. Some of the primary challenges include the vast and evolving nature of big data, the large volume of available data that can be overwhelming to analyze, and integrating multiple disciplines like computer science, statistics, and domain-specific knowledge. Additionally, the methodology for big data research requires a ...
Hadi, Mohammed Safa (2020) Network Optimisation using Big Data Analytics. PhD thesis, University of Leeds. Abstract. Interdisciplinary research is fuelling a paradigm shift to endow technology-based services with a personalised dimension. The main contributors for such innovatory change are the surge in data production rate, the proliferation ...
2014. The explosion in the amount of data, called "data deluge", is forcing to redefine many scientific and technological fields, with the affirmation in any environment of Big Data as a potential source of data. Official statistics institutions a few years ago started to open up to external data sources such as administrative data.
This dissertation explores three topics related to random forests: tree aggregation, variable importance, and robustness. 10. Climate Data Computing: Optimal Interpolation, Averaging, Visualization and Delivery. This dissertation solves two important problems in the modern analysis of big climate data.
Big Data PhD Program Handbook 2 The College of Graduate Studies offers several thesis and dissertation Workshops each term. Students are highly encouraged to attend these workshops early in the dissertation process to fully understand the above policies and procedures.
PhD Thesis on Big Data Analytics. Big data is denoted as the variety, volume, and velocity of information assets in high form along with processes such as decision-making, innovations in information processing, and the cost-effective process. The objectives of big data analytics are deployed with advanced analytics techniques through various ...
FUTUREDATA4EU - TRAINING FUTURE BIG DATA EXPERTS FOR EUROPE (MSCA COFUND EUROPEAN PROJECT): FULLY FUNDED PHD POSITIONS IN ITALY. University of Bologna. Applications open - 53 PhD Positions in Big Data Research and Related Areas, in Leading Universities in Italy. The call for applications is available in the program web page here.
Findings manifested that big data analytics was a game changer for university libraries. BDA transformed library services, and enhanced information services. The study also revealed that awareness and expertise, technical issues, and data integrity and security were the major challenges that causes barriers for the adoption of BDA based ...
Commonly described with certain V's, which define the characteristics of big data (volume, variety, velocity, veracity, variability and value). It is seen as a management approach where the aim is to manage, process and analyze different V's to enchase organizations value creation, for example through decision-making.
The Big Data Analytics PhD program consists of at least 72 credit hours of course work beyond the Bachelor's degree, of which a minimum of 42 hours of formal course work, exclusive of independent study, and 15 credit hours of dissertation research (STA 7980) are required. The program requires 15 hours of elective courses.
Predictive analysis. It is an interesting topic for master thesis and further research in big data using recent and classical research. It is considered the real-time application result for business intelligence and big data. Big data analysis. It has applications in the areas such as customer service, finance, etc.
These 15 topics will help you to dive into interesting research. You may even build on research done by other scholars. Evaluate the data mining process. The influence of the various dimension reduction methods and techniques. The best data classification methods. The simple linear regression modeling methods.
Overall, this thesis paves the way for the application of GNNs in complex real-world relational data scenarios, significantly contributing to the field of graph representation learning. for contributions to systems addressing efficiency and practicality issues of ML model tuning, training, scheduling, and deployment in large-scale clusters.
In big data analytics, thesis completion is a big thing for PhD beginners. Since it is a complex process due to the variety of data analysis, in this case, our PhD thesis on Big Data Analytics is the best solution for it. To deal with the BIG DATA, you will need our BIG SERVICE. It translates our insights to the research scholars over 15+ years ...
Advisory Committee: W. Jim Zheng, PhD. PhD thesis: McWilliams School of Biomedical Informatics at UTHealth Houston. ABSTRACT. This dissertation leverages large biological datasets and data-driven research to enhance our understanding of complex human diseases, deploying a unified narrative across three interconnected bioinformatics studies.
From improving economic performance and strengthening policies to fight climate change to bolstering education and fighting international tax evasion, the OECD is a unique forum and knowledge hub for data, analysis and best practices in public policy.