- Reference Manager
- Simple TEXT file
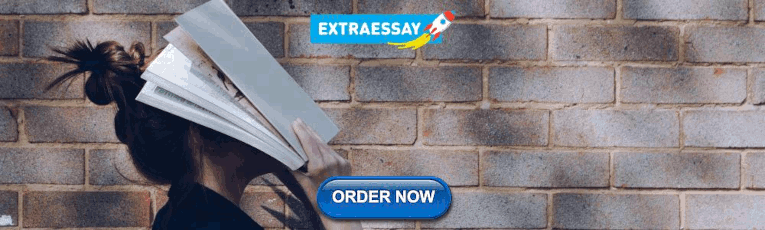
People also looked at
Review article, phishing attacks: a recent comprehensive study and a new anatomy.

- Cardiff School of Technologies, Cardiff Metropolitan University, Cardiff, United Kingdom
With the significant growth of internet usage, people increasingly share their personal information online. As a result, an enormous amount of personal information and financial transactions become vulnerable to cybercriminals. Phishing is an example of a highly effective form of cybercrime that enables criminals to deceive users and steal important data. Since the first reported phishing attack in 1990, it has been evolved into a more sophisticated attack vector. At present, phishing is considered one of the most frequent examples of fraud activity on the Internet. Phishing attacks can lead to severe losses for their victims including sensitive information, identity theft, companies, and government secrets. This article aims to evaluate these attacks by identifying the current state of phishing and reviewing existing phishing techniques. Studies have classified phishing attacks according to fundamental phishing mechanisms and countermeasures discarding the importance of the end-to-end lifecycle of phishing. This article proposes a new detailed anatomy of phishing which involves attack phases, attacker’s types, vulnerabilities, threats, targets, attack mediums, and attacking techniques. Moreover, the proposed anatomy will help readers understand the process lifecycle of a phishing attack which in turn will increase the awareness of these phishing attacks and the techniques being used; also, it helps in developing a holistic anti-phishing system. Furthermore, some precautionary countermeasures are investigated, and new strategies are suggested.
Introduction
The digital world is rapidly expanding and evolving, and likewise, as are cybercriminals who have relied on the illegal use of digital assets—especially personal information—for inflicting damage to individuals. One of the most threatening crimes of all internet users is that of ‘identity theft’ ( Ramanathan and Wechsler, 2012 ) which is defined as impersonating the person’s identity to steal and use their personal information (i.e., bank details, social security number, or credit card numbers, etc.) by an attacker for the individuals’ own gain not just for stealing money but also for committing other crimes ( Arachchilage and Love, 2014 ). Cyber criminals have also developed their methods for stealing their information, but social-engineering-based attacks remain their favorite approach. One of the social engineering crimes that allow the attacker to perform identity theft is called a phishing attack. Phishing has been one of the biggest concerns as many internet users fall victim to it. It is a social engineering attack wherein a phisher attempts to lure the users to obtain their sensitive information by illegally utilizing a public or trustworthy organization in an automated pattern so that the internet user trusts the message, and reveals the victim’s sensitive information to the attacker ( Jakobsson and Myers, 2006 ). In phishing attacks, phishers use social engineering techniques to redirect users to malicious websites after receiving an email and following an embedded link ( Gupta et al., 2015 ). Alternatively, attackers could exploit other mediums to execute their attacks such as Voice over IP (VoIP), Short Message Service (SMS) and, Instant Messaging (IM) ( Gupta et al., 2015 ). Phishers have also turned from sending mass-email messages, which target unspecified victims, into more selective phishing by sending their emails to specific victims, a technique called “spear-phishing.”
Cybercriminals usually exploit users with a lack of digital/cyber ethics or who are poorly trained in addition to technical vulnerabilities to reach their goals. Susceptibility to phishing varies between individuals according to their attributes and awareness level, therefore, in most attacks, phishers exploit human nature for hacking, instead of utilising sophisticated technologies. Even though the weakness in the information security chain is attributed to humans more than the technology, there is a lack of understanding about which ring in this chain is first penetrated. Studies found that certain personal characteristics make some persons more receptive to various lures ( Iuga et al., 2016 ; Ovelgönne et al., 2017 ; Crane, 2019 ). For example, individuals who usually obey authorities more than others are more likely to fall victim to a Business Email Compromise (BEC) that is pretending to be from a financial institution and requests immediate action by seeing it as a legitimate email ( Barracuda, 2020 ). Greediness is another human weakness that could be used by an attacker, for example, emails that offering either great discounts, free gift cards, and others ( Workman, 2008 ).
Various channels are used by the attacker to lure the victim through a scam or through an indirect manner to deliver a payload for gaining sensitive and personal information from the victim ( Ollmann, 2004 ). However, phishing attacks have already led to damaging losses and could affect the victim not only through a financial context but could also have other serious consequences such as loss of reputation, or compromise of national security ( Ollmann, 2004 ; Herley and Florêncio, 2008 ). Cybercrime damages have been expected to cost the world $6 trillion annually by 2021, up from $3 trillion in 2015 according to Cybersecurity Ventures ( Morgan, 2019 ). Phishing attacks are the most common type of cybersecurity breaches as stated by the official statistics from the cybersecurity breaches survey 2020 in the United Kingdom ( GOV.UK, 2020 ). Although these attacks affect organizations and individuals alike, the loss for the organizations is significant, which includes the cost for recovery, the loss of reputation, fines from information laws/regulations, and reduced productivity ( Medvet et al., 2008 ).
Phishing is a field of study that merges social psychology, technical systems, security subjects, and politics. Phishing attacks are more prevalent: a recent study ( Proofpoint, 2020 ) found that nearly 90% of organizations faced targeted phishing attacks in 2019. From which 88% experienced spear-phishing attacks, 83% faced voice phishing (Vishing), 86% dealt with social media attacks, 84% reported SMS/text phishing (SMishing), and 81% reported malicious USB drops. The 2018 Proofpoint 1 annual report ( Proofpoint, 2019a ) has stated that phishing attacks jumped from 76% in 2017 to 83% in 2018, where all phishing types happened more frequently than in 2017. The number of phishing attacks identified in the second quarter of 2019 was notably higher than the number recorded in the previous three quarters. While in the first quarter of 2020, this number was higher than it was in the previous one according to a report from Anti-Phishing Working Group (APWG 2 ) ( APWG, 2018 ) which confirms that phishing attacks are on the rise. These findings have shown that phishing attacks have increased continuously in recent years and have become more sophisticated and have gained more attention from cyber researchers and developers to detect and mitigate their impact. This article aims to determine the severity of the phishing problem by providing detailed insights into the phishing phenomenon in terms of phishing definitions, current statistics, anatomy, and potential countermeasures.
The rest of the article is organized as follows. Phishing Definitions provides a number of phishing definitions as well as some real-world examples of phishing. The evolution and development of phishing attacks are discussed in Developing a Phishing Campaign . What Attributes Make Some People More Susceptible to Phishing Attacks Than Others explores the susceptibility to these attacks. The proposed phishing anatomy and types of phishing attacks are elaborated in Proposed Phishing Anatomy . In Countermeasures , various anti-phishing countermeasures are discussed. The conclusions of this study are drawn in Conclusion .
Phishing Definitions
Various definitions for the term “phishing” have been proposed and discussed by experts, researchers, and cybersecurity institutions. Although there is no established definition for the term “phishing” due to its continuous evolution, this term has been defined in numerous ways based on its use and context. The process of tricking the recipient to take the attacker’s desired action is considered the de facto definition of phishing attacks in general. Some definitions name websites as the only possible medium to conduct attacks. The study ( Merwe et al., 2005 , p. 1) defines phishing as “a fraudulent activity that involves the creation of a replica of an existing web page to fool a user into submitting personal, financial, or password data.” The above definition describes phishing as an attempt to scam the user into revealing sensitive information such as bank details and credit card numbers, by sending malicious links to the user that leads to the fake web establishment. Others name emails as the only attack vector. For instance, PishTank (2006) defines phishing as “a fraudulent attempt, usually made through email, to steal your personal information.” A description for phishing stated by ( Kirda and Kruegel, 2005 , p.1) defines phishing as “a form of online identity theft that aims to steal sensitive information such as online banking passwords and credit card information from users.” Some definitions highlight the usage of combined social and technical skills. For instance, APWG defines phishing as “a criminal mechanism employing both social engineering and technical subterfuge to steal consumers’ personal identity data and financial account credentials” ( APWG, 2018 , p. 1). Moreover, the definition from the United States Computer Emergency Readiness Team (US-CERT) states phishing as “a form of social engineering that uses email or malicious websites (among other channels) to solicit personal information from an individual or company by posing as a trustworthy organization or entity” ( CISA, 2018 ). A detailed definition has been presented in ( Jakobsson and Myers, 2006 , p. 1), which describes phishing as “a form of social engineering in which an attacker, also known as a phisher, attempts to fraudulently retrieve legitimate users’ confidential or sensitive credentials by mimicking electronic communications from a trustworthy or public organization in an automated fashion. Such communications are most frequently done through emails that direct users to fraudulent websites that in turn collect the credentials in question.”
In order to understand the anatomy of the phishing attack, there is a necessity for a clear and detailed definition that underpins previous existent definitions. Since a phishing attack constitutes a mix of technical and social engineering tactics, a new definition (i.e., Anatomy) has been proposed in this article, which describes the complete process of a phishing attack. This provides a better understanding for the readers as it covers phishing attacks in depth from a range of perspectives. Various angles and this might help beginner readers or researchers in this field. To this end, we define phishing as a socio-technical attack, in which the attacker targets specific valuables by exploiting an existing vulnerability to pass a specific threat via a selected medium into the victim’s system, utilizing social engineering tricks or some other techniques to convince the victim into taking a specific action that causes various types of damages.
Figure 1 depicts the general process flow for a phishing attack that contains four phases; these phases are elaborated in Proposed Phishing Anatomy . However, as shown in Figure 1 , in most attacks, the phishing process is initiated by gathering information about the target. Then the phisher decides which attack method is to be used in the attack as initial steps within the planning phase. The second phase is the preparation phase, in which the phisher starts to search for vulnerabilities through which he could trap the victim. The phisher conducts his attack in the third phase and waits for a response from the victim. In turn, the attacker could collect the spoils in the valuables acquisition phase, which is the last step in the phishing process. To elaborate the above phishing process using an example, an attacker may send a fraudulent email to an internet user pretending to be from the victim’s bank, requesting the user to confirm the bank account details, or else the account may be suspended. The user may think this email is legitimate since it uses the same graphic elements, trademarks, and colors of their legitimate bank. Submitted information will then be directly transmitted to the phisher who will use it for different malicious purposes such as money withdrawal, blackmailing, or committing further frauds.
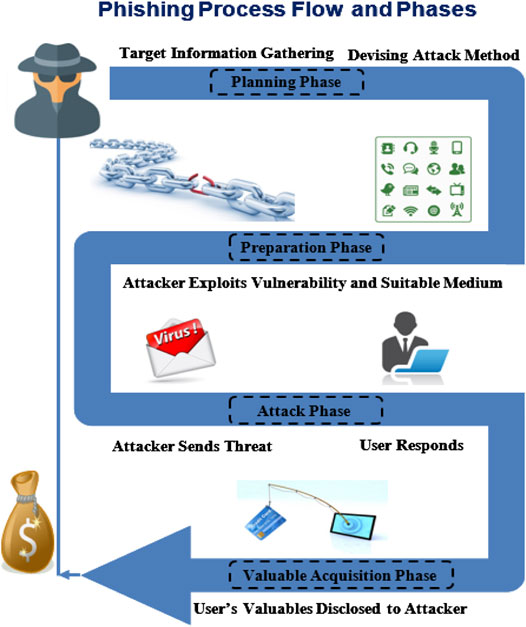
FIGURE 1 . General phishing attack process.
Real-World Phishing Examples
Some real-world examples of phishing attacks are discussed in this section to present the complexity of some recent phishing attacks. Figure 2 shows the screenshot of a suspicious phishing email that passed a University’s spam filters and reached the recipient mailbox. As shown in Figure 2 , the phisher uses the sense of importance or urgency in the subject through the word ‘important,’ so that the email can trigger a psychological reaction in the user to prompt them into clicking the button “View message.” The email contains a suspicious embedded button, indeed, when hovering over this embedded button, it does not match with Uniform Resource Locator (URL) in the status bar. Another clue in this example is that the sender's address is questionable and not known to the receiver. Clicking on the fake attachment button will result in either installation of a virus or worm onto the computer or handing over the user’s credentials by redirecting the victim onto a fake login page.
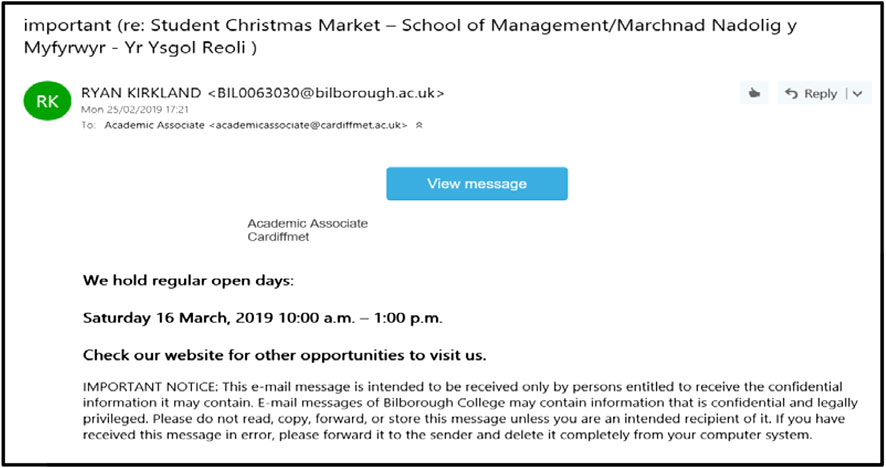
FIGURE 2 . Screenshot of a real suspicious phishing email received by the authors’ institution in February 2019.
More recently, phishers take advantage of the Coronavirus pandemic (COVID-19) to fool their prey. Many Coronavirus-themed scam messages sent by attackers exploited people’s fear of contracting COVID-19 and urgency to look for information related to Coronavirus (e.g., some of these attacks are related to Personal Protective Equipment (PPE) such as facemasks), the WHO stated that COVID-19 has created an Infodemic which is favorable for phishers ( Hewage, 2020 ). Cybercriminals also lured people to open attachments claiming that it contains information about people with Coronavirus within the local area.
Figure 3 shows an example of a phishing e-mail where the attacker claimed to be the recipient’s neighbor sending a message in which they pretended to be dying from the virus and threatening to infect the victim unless a ransom was paid ( Ksepersky, 2020 ).
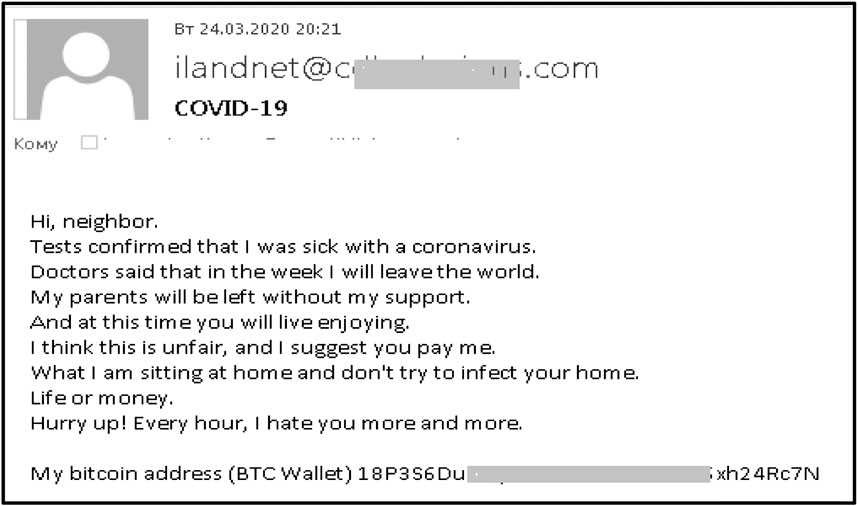
FIGURE 3 . Screenshot of a coronavirus related phishing email ( Ksepersky, 2020 ).
Another example is the phishing attack spotted by a security researcher at Akamai organization in January 2019. The attack attempted to use Google Translate to mask suspicious URLs, prefacing them with the legit-looking “ www.translate.google.com ” address to dupe users into logging in ( Rhett, 2019 ). That attack followed with Phishing scams asking for Netflix payment detail for example, or embedded in promoted tweets that redirect users to genuine-looking PayPal login pages. Although the tricky/bogus page was very well designed in the latter case, the lack of a Hypertext Transfer Protocol Secure (HTTPS) lock and misspellings in the URL were key red flags (or giveaways) that this was actually a phishing attempt ( Keck, 2018 ). Figure 4A shows a screenshot of a phishing email received by the Federal Trade Commission (FTC). The email promotes the user to update his payment method by clicking on a link, pretending that Netflix is having a problem with the user's billing information ( FTC, 2018 ).
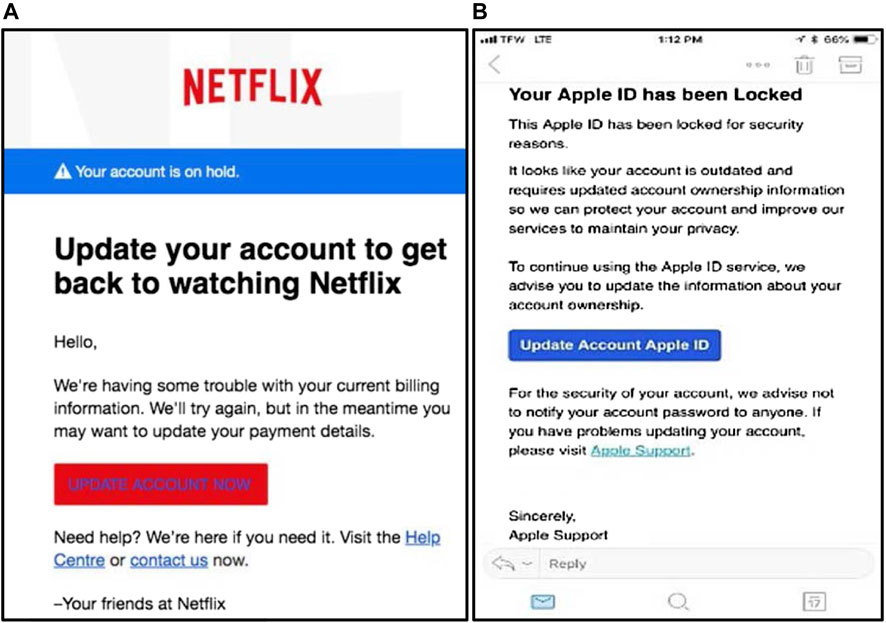
FIGURE 4 . Screenshot of the (A) Netflix scam email and (B) fraudulent text message (Apple) ( Keck, 2018 ; Rhett, 2019 )
Figure 4B shows a text message as another example of phishing that is difficult to spot as a fake text message ( Pompon et al., 2018 ). The text message shown appears to come from Apple asking the customer to update the victim’s account. A sense of urgency is used in the message as a lure to motivate the user to respond.
Developing a Phishing Campaign
Today, phishing is considered one of the most pressing cybersecurity threats for all internet users, regardless of their technical understanding and how cautious they are. These attacks are getting more sophisticated by the day and can cause severe losses to the victims. Although the attacker’s first motivation is stealing money, stolen sensitive data can be used for other malicious purposes such as infiltrating sensitive infrastructures for espionage purposes. Therefore, phishers keep on developing their techniques over time with the development of electronic media. The following sub-sections discuss phishing evolution and the latest statistics.
Historical Overview
Cybersecurity has been a major concern since the beginning of APRANET, which is considered to be the first wide-area packet-switching network with distributed control and one of the first networks to implement the TCP/IP protocol suite. The term “Phishing” which was also called carding or brand spoofing, was coined for the first time in 1996 when the hackers created randomized credit card numbers using an algorithm to steal users' passwords from America Online (AOL) ( Whitman and Mattord, 2012 ; Cui et al., 2017 ). Then phishers used instant messages or emails to reach users by posing as AOL employees to convince users to reveal their passwords. Attackers believed that requesting customers to update their account would be an effective way to disclose their sensitive information, thereafter, phishers started to target larger financial companies. The author in ( Ollmann, 2004 ) believes that the “ph” in phishing comes from the terminology “Phreaks” which was coined by John Draper, who was also known as Captain Crunch, and was used by early Internet criminals when they phreak telephone systems. Where the “f” in ‘fishing’ replaced with “ph” in “Phishing” as they both have the same meaning by phishing the passwords and sensitive information from the sea of internet users. Over time, phishers developed various and more advanced types of scams for launching their attack. Sometimes, the purpose of the attack is not limited to stealing sensitive information, but it could involve injecting viruses or downloading the malicious program into a victim's computer. Phishers make use of a trusted source (for instance a bank helpdesk) to deceive victims so that they disclose their sensitive information ( Ollmann, 2004 ).
Phishing attacks are rapidly evolving, and spoofing methods are continuously changing as a response to new corresponding countermeasures. Hackers take advantage of new tool-kits and technologies to exploit systems’ vulnerabilities and also use social engineering techniques to fool unsuspecting users. Therefore, phishing attacks continue to be one of the most successful cybercrime attacks.
The Latest Statistics of Phishing Attacks
Phishing attacks are becoming more common and they are significantly increasing in both sophistication and frequency. Lately, phishing attacks have appeared in various forms. Different channels and threats are exploited and used by the attackers to trap more victims. These channels could be social networks or VoIP, which could carry various types of threats such as malicious attachments, embedded links within an email, instant messages, scam calls, or other types. Criminals know that social engineering-based methods are effective and profitable; therefore, they keep focusing on social engineering attacks, as it is their favorite weapon, instead of concentrating on sophisticated techniques and toolkits. Phishing attacks have reached unprecedented levels especially with emerging technologies such as mobile and social media ( Marforio et al., 2015 ). For instance, from 2017 to 2020, phishing attacks have increased from 72 to 86% among businesses in the United Kingdom in which a large proportion of the attacks are originated from social media ( GOV.UK, 2020 ).
The APWG Phishing Activity Trends Report analyzes and measures the evolution, proliferation, and propagation of phishing attacks reported to the APWG. Figure 5 shows the growth in phishing attacks from 2015 to 2020 by quarters based on APWG annual reports ( APWG, 2020 ). As demonstrated in Figure 5 , in the third quarter of 2019, the number of phishing attacks rose to 266,387, which is the highest level in three years since late 2016. This was up 46% from the 182,465 for the second quarter, and almost double the 138,328 seen in the fourth quarter of 2018. The number of unique phishing e-mails reported to APWG in the same quarter was 118,260. Furthermore, it was found that the number of brands targeted by phishing campaigns was 1,283.
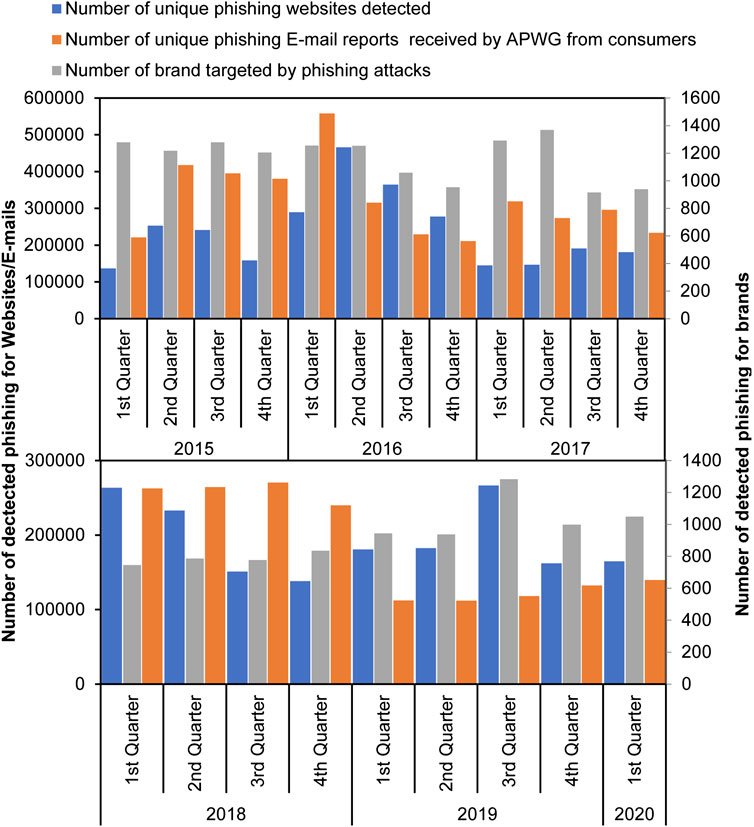
FIGURE 5 . The growth in phishing attacks 2015–2020 by quarters based on data collected from APWG annual reports.
Cybercriminals are always taking advantage of disasters and hot events for their own gains. With the beginning of the COVID-19 crisis, a variety of themed phishing and malware attacks have been launched by phishers against workers, healthcare facilities, and even the general public. A report from Microsoft ( Microsoft, 2020 ) showed that cyber-attacks related to COVID-19 had spiked to an unprecedented level in March, most of these scams are fake COVID-19 websites according to security company RiskIQ ( RISKIQ, 2020 ). However, the total number of phishing attacks observed by APWG in the first quarter of 2020 was 165,772, up from the 162,155 observed in the fourth quarter of 2019. The number of these unique phishing reports submitted to APWG during the first quarter of 2020 was 139,685, up from 132,553 in the fourth quarter of 2019, 122,359 in the third quarter of 2019, and 112,163 in the second quarter of 2019 ( APWG, 2020 ).
A study ( KeepnetLABS, 2018 ) confirmed that more than 91% of system breaches are caused by attacks initiated by email. Although cybercriminals use email as the main medium for leveraging their attacks, many organizations faced a high volume of different social engineering attacks in 2019 such as Social Media Attacks, Smishing Attacks, Vishing Attacks, USB-based Attacks (for example by hiding and delivering malware to smartphones via USB phone chargers and distributing malware-laden free USBs) ( Proofpoint, 2020 ). However, info-security professionals reported a higher frequency of all types of social engineering attacks year-on-year according to a report presented by Proofpoint. Spear phishing increased to 64% in 2018 from 53% in 2017, Vishing and/or SMishing increased to 49% from 45%, and USB attacks increased to 4% from 3%. The positive side shown in this study is that 59% of suspicious emails reported by end-users were classified as potential phishing, indicating that employees are being more security-aware, diligent, and thoughtful about the emails they receive ( Proofpoint, 2019a ). In all its forms, phishing can be one of the easiest cyber attacks to fall for. With the increasing levels of different phishing types, a survey was conducted by Proofpoint to identify the strengths and weaknesses of particular regions in terms of specific fundamental cybersecurity concepts. In this study, several questions were asked of 7,000 end-users about the identification of multiple terms like phishing, ransomware, SMishing, and Vishing across seven countries; the US, United Kingdom, France, Germany, Italy, Australia, and Japan. The response was different from country to country, where respondents from the United Kingdom recorded the highest knowledge with the term phishing at 70% and the same with the term ransomware at 60%. In contrast, the results showed that the United Kingdom recorded only 18% for each Vishing and SMishing ( Proofpoint, 2019a ), as shown in Table 1 .

TABLE 1 . Percentage of respondents understanding multiple cybersecurity terms from different countries.
On the other hand, a report by Wombat security reflects responses from more than 6,000 working adults about receiving fraudulent solicitation across six countries; the US, United Kingdom, Germany, France, Italy, and Australia ( Ksepersky, 2020 ). Respondents from the United Kingdom stated that they were recipients of fraudulent solicitations through the following sources: email 62%, phone call 27%, text message 16%, mailed letter 8%, social media 10%, and 17% confirmed that they been the victim of identity theft ( Ksepersky, 2020 ). However, the consequences of responding to phishing are serious and costly. For instance, the United Kingdom losses from financial fraud across payment cards, remote banking, and cheques totaled £768.8 million in 2016 ( Financial Fraud Action UK, 2017 ). Indeed, the losses resulting from phishing attacks are not limited to financial losses that might exceed millions of pounds, but also loss of customers and reputation. According to the 2020 state of phish report ( Proofpoint, 2020 ), damages from successful phishing attacks can range from lost productivity to cash outlay. The cost can include; lost hours from employees, remediation time for info security teams’ costs due to incident response, damage to reputation, lost intellectual property, direct monetary losses, compliance fines, lost customers, legal fees, etc.
There are many targets for phishing including end-user, business, financial services (i.e., banks, credit card companies, and PayPal), retail (i.e., eBay, Amazon) and, Internet Service Providers ( wombatsecurity.com, 2018 ). Affected organizations detected by Kaspersky Labs globally in the first quarter of 2020 are demonstrated in Figure 6 . As shown in the figure, online stores were at the top of the targeted list (18.12%) followed by global Internet portals (16.44%) and social networks in third place (13.07%) ( Ksepersky, 2020 ). While the most impersonated brands overall for the first quarter of 2020 were Apple, Netflix, Yahoo, WhatsApp, PayPal, Chase, Facebook, Microsoft eBay, and Amazon ( Checkpoint, 2020 ).
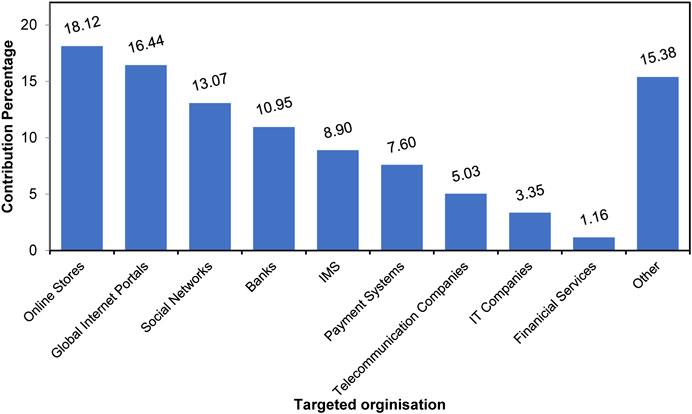
FIGURE 6 . Distribution of organizations affected by phishing attacks detected by Kaspersky in quarter one of 2020.
Phishing attacks can take a variety of forms to target people and steal sensitive information from them. Current data shows that phishing attacks are still effective, which indicates that the available existing countermeasures are not enough to detect and prevent these attacks especially on smart devices. The social engineering element of the phishing attack has been effective in bypassing the existing defenses to date. Therefore, it is essential to understand what makes people fall victim to phishing attacks. What Attributes Make Some People More Susceptible to Phishing Attacks Than Others discusses the human attributes that are exploited by the phishers.
What Attributes Make Some People More Susceptible to Phishing Attacks Than Others
Why do most existing defenses against phishing not work? What personal and contextual attributes make them more susceptible to phishing attacks than other users? Different studies have discussed those two questions and examined the factors affecting susceptibility to a phishing attack and the reasons behind why people get phished. Human nature is considered one of the most affecting factors in the process of phishing. Everyone is susceptible to phishing attacks because phishers play on an individual’s specific psychological/emotional triggers as well as technical vulnerabilities ( KeepnetLABS, 2018 ; Crane, 2019 ). For instance, individuals are likely to click on a link within an email when they see authority cues ( Furnell, 2007 ). In 2017, a report by PhishMe (2017) found that curiosity and urgency were the most common triggers that encourage people to respond to the attack, later these triggers were replaced by entertainment, social media, and reward/recognition as the top emotional motivators. However, in the context of a phishing attack, the psychological triggers often surpass people’s conscious decisions. For instance, when people are working under stress, they tend to make decisions without thinking of the possible consequences and options ( Lininger and Vines, 2005 ). Moreover, everyday stress can damage areas of the brain that weakens the control of their emotions ( Keinan, 1987 ). Several studies have addressed the association between susceptibility to phishing and demographic variables (e.g., age and gender) as an attempt to identify the reasons behind phishing success at different population groups. Although everyone is susceptible to phishing, studies showed that different age groups are more susceptible to certain lures than others are. For example, participants with an age range between 18 and 25 are more susceptible to phishing than other age groups ( Williams et al., 2018 ). The reason that younger adults are more likely to fall for phishing, is that younger adults are more trusting when it comes to online communication, and are also more likely to click on unsolicited e-mails ( Getsafeonline, 2017 ). Moreover, older participants are less susceptible because they tend to be less impulsive ( Arnsten et al., 2012 ). While some studies confirmed that women are more susceptible than men to phishing as they click on links in phishing emails and enter information into phishing websites more often than men do. The study published by Getsafeonline (2017) identifies a lack of technical know-how and experience among women than men as the main reason for this. In contrast, a survey conducted by antivirus company Avast found that men are more susceptible to smartphone malware attacks than women ( Ong, 2014 ). These findings confirmed the results from the study ( Hadlington, 2017 ) that found men are more susceptible to mobile phishing attacks than women. The main reason behind this according to Hadlington (2017) is that men are more comfortable and trusting when using mobile online services. The relationships between demographic characteristics of individualls and their ability to correctly detect a phishing attack have been studied in ( Iuga et al., 2016 ). The study showed that participants with high Personal Computer (PC) usage tend to identify phishing efforts more accurately and faster than other participants. Another study ( Hadlington, 2017 ) showed that internet addiction, attentional, and motor impulsivity were significant positive predictors for risky cybersecurity behaviors while a positive attitude toward cybersecurity in business was negatively related to risky cybersecurity behaviors. On the other hand, the trustworthiness of people in some web sites/platforms is one of the holes that the scammers or crackers exploit especially when it based on visual appearance that could fool the user ( Hadlington, 2017 ). For example, fraudsters take advantage of people’s trust in a website by replacing a letter from the legitimate site with a number such as goog1e.com instead of google.com . Another study ( Yeboah-Boateng and Amanor, 2014 ) demonstrates that although college students are unlikely to disclose personal information as a response to an email, nonetheless they could easily be tricked by other tactics, making them alarmingly susceptible to email phishing attacks. The reason for that is most college students do not have a basis in ICT especially in terms of security. Although security terms like viruses, online scams and worms are known by some end-users, these users could have no knowledge about Phishing, SMishing, and Vishing and others ( Lin et al., 2012 ). However, study ( Yeboah-Boateng and Amanor, 2014 ) shows that younger students are more susceptible than older students, and students who worked full-time were less likely to fall for phishing.
The study reported in ( Diaz et al., 2020 ) examines user click rates and demographics among undergraduates by sending phishing attacks to 1,350 randomly selected students. Students from various disciplines were involved in the test, from engineering and mathematics to arts and social sciences. The study observed that student susceptibility was affected by a range of factors such as phishing awareness, time spent on the computer, cyber training, age, academic year, and college affiliation. The most surprising finding is that those who have greater phishing knowledge are more susceptible to phishing scams. The authors consider two speculations for these unexpected findings. First, user’s awareness about phishing might have been increased with the continuous falling for phishing scams. Second, users who fell for the phish might have less knowledge about phishing than they claim. Other findings from this study agreed with findings from other studies that is, older students were more able to detect a phishing email, and engineering and IT majors had some of the lowest click rates as shown in Figure 7 , which shows that some academic disciplines are more susceptible to phishing than others ( Bailey et al., 2008 ).
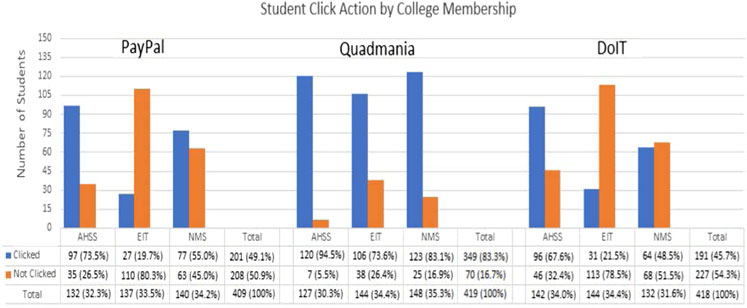
FIGURE 7 . The number of clicks on phishing emails by students in the College of Arts, Humanities, and Social Sciences (AHSS), the College of Engineering and Information Technology (EIT), and the College of Natural and Mathematical Sciences (NMS) at the University of Maryland, Baltimore County (UMBC) ( Diaz et al., 2020 ).
Psychological studies have also illustrated that the user’s ability to avoid phishing attacks affected by different factors such as browser security indicators and user's awareness of phishing. The author in ( Dhamija et al., 2006 ) conducted an experimental study using 22 participants to test the user’s ability to recognize phishing websites. The study shows that 90% of these participants became victims of phishing websites and 23% of them ignored security indexes such as the status and address bar. In 2015, another study was conducted for the same purpose, where a number of fake web pages was shown to the participants ( Alsharnouby et al., 2015 ). The results of this study showed that participants detected only 53% of phishing websites successfully. The authors also observed that the time spent on looking at browser elements affected the ability to detect phishing. Lack of knowledge or awareness and carelessness are common causes for making people fall for a phishing trap. Most people have unknowingly opened a suspicious attachment or clicked a fake link that could lead to different levels of compromise. Therefore, focusing on training and preparing users for dealing with such attacks are essential elements to minimize the impact of phishing attacks.
Given the above discussion, susceptibility to phishing varies according to different factors such as age, gender, education level, internet, and PC addiction, etc. Although for each person, there is a trigger that can be exploited by phishers, even people with high experience may fall prey to phishing due to the attack sophistication that makes it difficult to be recognized. Therefore, it is inequitable that the user has always been blamed for falling for these attacks, developers must improve the anti-phishing systems in a way that makes the attack invisible. Understanding the susceptibility of individuals to phishing attacks will help in better developing prevention and detection techniques and solutions.
Proposed Phishing Anatomy
Phishing process overview.
Generally, most of the phishing attacks start with an email ( Jagatic et al., 2007 ). The phishing mail could be sent randomly to potential users or it can be targeted to a specific group or individuals. Many other vectors can also be used to initiate the attack such as phone calls, instant messaging, or physical letters. However, phishing process steps have been discussed by many researchers due to the importance of understanding these steps in developing an anti-phishing solution. The author in the study ( Rouse, 2013 ) divides the phishing attack process into five phases which are planning, setup, attack, collection, and cash. A study ( Jakobsson and Myers, 2006 ) discusses the phishing process in detail and explained it as step-by-step phases. These phases include preparation for the attack, sending a malicious program using the selected vector, obtaining the user’s reaction to the attack, tricking a user to disclose their confidential information which will be transmitted to the phisher, and finally obtaining the targeted money. While the study ( Abad, 2005 ) describes a phishing attack in three phases: the early phase which includes initializing attack, creating the phishing email, and sending a phishing email to the victim. The second phase includes receiving an email by the victim and disclosing their information (in the case of the respondent) and the final phase in which the defrauding is successful. However, all phishing scams include three primary phases, the phisher requests sensitive valuables from the target, and the target gives away these valuables to a phisher, and phisher misuses these valuables for malicious purposes. These phases can be classified furthermore into its sub-processes according to phishing trends. Thus, a new anatomy for phishing attacks has been proposed in this article, which expands and integrates previous definitions to cover the full life cycle of a phishing attack. The proposed new anatomy, which consists of 4 phases, is shown in Figure 8 . This new anatomy provides a reference structure to look at phishing attacks in more detail and also to understand potential countermeasures to prevent them. The explanations for each phase and its components are presented as follows:
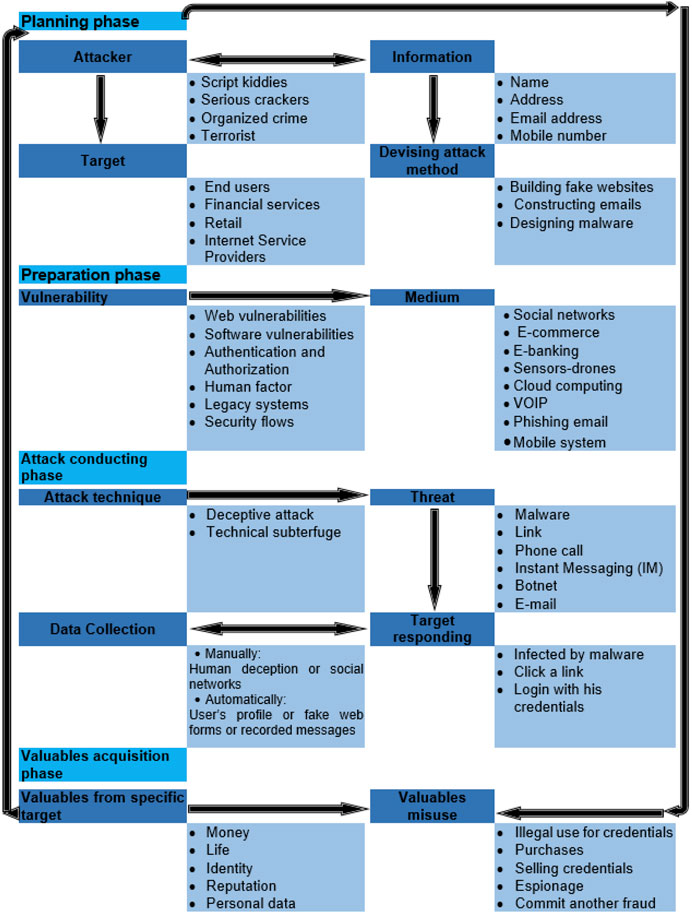
FIGURE 8 . The proposed anatomy of phishing was built upon the proposed phishing definition in this article, which concluded from our understanding of a phishing attack.
Figure 8 depicts the proposed anatomy of the phishing attack process, phases, and components drawn upon the proposed definition in this article. The proposed phishing anatomy explains in detail each phase of phishing phases including attackers and target types, examples about the information that could be collected by the attacker about the victim, and examples about attack methods. The anatomy, as shown in the figure, illustrates a set of vulnerabilities that the attacker can exploit and the mediums used to conduct the attack. Possible threats are also listed, as well as the data collection method for a further explanation and some examples about target responding types and types of spoils that the attacker could gain and how they can use the stolen valuables. This anatomy elaborates on phishing attacks in depth which helps people to better understand the complete phishing process (i.e., end to end Phishing life cycle) and boost awareness among readers. It also provides insights into potential solutions for phishing attacks we should focus on. Instead of always placing the user or human in an accusation ring as the only reason behind phishing success, developers must be focusing on solutions to mitigate the initiation of the attack by preventing the bait from reaching the user. For instance, to reach the target’s system, the threat has to pass through many layers of technology or defenses exploiting one or more vulnerabilities such as web and software vulnerabilities.
Planning Phase
This is the first stage of the attack, where a phisher makes a decision about the targets and starts gathering information about them (individuals or company). Phishers gather information about the victims to lure them based on psychological vulnerability. This information can be anything like name, e-mail addresses for individuals, or the customers of that company. Victims could also be selected randomly, by sending mass mailings or targeted by harvesting their information from social media, or any other source. Targets for phishing could be any user with a bank account and has a computer on the Internet. Phishers target businesses such as financial services, retail sectors such as eBay and Amazon, and internet service providers such as MSN/Hotmail, and Yahoo ( Ollmann, 2004 ; Ramzan and Wuest, 2007 ). This phase also includes devising attack methods such as building fake websites (sometimes phishers get a scam page that is already designed or used, designing malware, constructing phishing emails. The attacker can be categorized based on the attack motivation. There are four types of attackers as mentioned in studies ( Vishwanath, 2005 ; Okin, 2009 ; EDUCBA, 2017 ; APWG, 2020 ):
▪ Script kiddies: the term script kiddies represents an attacker with no technical background or knowledge about writing sophisticated programs or developing phishing tools but instead they use scripts developed by others in their phishing attack. Although the term comes from children that use available phishing kits to crack game codes by spreading malware using virus toolkits, it does not relate precisely to the actual age of the phisher. Script kiddies can get access to website administration privileges and commit a “Web cracking” attack. Moreover, they can use hacking tools to compromise remote computers so-called “botnet,” the single compromised computer called a “zombie computer.” These attackers are not limited to just sit back and enjoy phishing, they could cause serious damage such as stealing information or uploading Trojans or viruses. In February 2000, an attack launched by Canadian teen Mike Calce resulted in $1.7 million US Dollars (USD) damages from Distributed Denial of Service (DDoS) attacks on CNN, eBay, Dell, Yahoo, and Amazon ( Leyden, 2001 ).
▪ Serious Crackers: also known as Black Hats. These attackers can execute sophisticated attacks and develop worms and Trojans for their attack. They hijack people's accounts maliciously and steal credit card information, destroy important files, or sell compromised credentials for personal gains.
▪ Organized crime: this is the most organized and effective type of attacker and they can incur significant damage to victims. These people hire serious crackers for conducting phishing attacks. Moreover, they can thoroughly trash the victim's identity, and committing devastated frauds as they have the skills, tools, and manpower. An organized cybercrime group is a team of expert hackers who share their skills to build complex attacks and to launch phishing campaigns against individuals and organizations. These groups offer their work as ‘crime as a service’ and they can be hired by terrorist groups, organizations, or individuals.
▪ Terrorists: due to our dependency on the internet for most activities, terrorist groups can easily conduct acts of terror remotely which could have an adverse impact. These types of attacks are dangerous since they are not in fear of any aftermath, for instance going to jail. Terrorists could use the internet to the maximum effect to create fear and violence as it requires limited funds, resources, and efforts compared to, for example, buying bombs and weapons in a traditional attack. Often, terrorists use spear phishing to launch their attacks for different purposes such as inflicting damage, cyber espionage, gathering information, locating individuals, and other vandalism purposes. Cyber espionage has been used extensively by cyber terrorists to steal sensitive information on national security, commercial information, and trade secrets which can be used for terrorist activities. These types of crimes may target governments or organizations, or individuals.
Attack Preparation
After making a decision about the targets and gathering information about them, phishers start to set up the attack by scanning for the vulnerabilities to exploit. The following are some examples of vulnerabilities exploited by phishers. For example, the attacker might exploit buffer overflow vulnerability to take control of target applications, create a DoS attack, or compromise computers. Moreover, “zero-day” software vulnerabilities, which refer to newly discovered vulnerabilities in software programs or operating systems could be exploited directly before it is fixed ( Kayne, 2019 ). Another example is browser vulnerabilities, adding new features and updates to the browser might introduce new vulnerabilities to the browser software ( Ollmann, 2004 ). In 2005, attackers exploited a cross-domain vulnerability in Internet Explorer (IE) ( Symantic, 2019 ). The cross-domain used to separate content from different sources in Microsoft IE. Attackers exploited a flaw in the cross-domain that enables them to execute programs on a user's computer after running IE. According to US-CERT, hackers are actively exploiting this vulnerability. To carry out a phishing attack, attackers need a medium so that they can reach their target. Therefore, apart from planning the attack to exploit potential vulnerabilities, attackers choose the medium that will be used to deliver the threat to the victim and carry out the attack. These mediums could be the internet (social network, websites, emails, cloud computing, e-banking, mobile systems) or VoIP (phone call), or text messages. For example, one of the actively used mediums is Cloud Computing (CC). The CC has become one of the more promising technologies and has popularly replaced conventional computing technologies. Despite the considerable advantages produced by CC, the adoption of CC faces several controversial obstacles including privacy and security issues ( CVEdetails, 2005 ). Due to the fact that different customers could share the same recourses in the cloud, virtualization vulnerabilities may be exploited by a possible malicious customer to perform security attacks on other customers’ applications and data ( Zissis and Lekkas, 2012 ). For example, in September 2014, secret photos of some celebrities suddenly moved through the internet in one of the more terrible data breaches. The investigation revealed that the iCloud accounts of the celebrities were breached ( Lehman and Vajpayee, 2011 ). According to Proofpoint, in 2017, attackers used Microsoft SharePoint to infect hundreds of campaigns with malware through messages.
Attack Conducting Phase
This phase involves using attack techniques to deliver the threat to the victim as well as the victim’s interaction with the attack in terms of responding or not. After the victim's response, the system may be compromised by the attacker to collect user's information using techniques such as injecting client-side script into webpages ( Johnson, 2016 ). Phishers can compromise hosts without any technical knowledge by purchasing access from hackers ( Abad, 2005 ). A threat is a possible danger that that might exploit a vulnerability to compromise people’s security and privacy or cause possible harm to a computer system for malicious purposes. Threats could be malware, botnet, eavesdropping, unsolicited emails, and viral links. Several Phishing techniques are discussed in sub- Types and Techniques of Phishing Attacks .
Valuables Acquisition Phase
In this stage, the phisher collects information or valuables from victims and uses it illegally for purchasing, funding money without the user’s knowledge, or selling these credentials in the black market. Attackers target a wide range of valuables from their victims that range from money to people’s lives. For example, attacks on online medical systems may lead to loss of life. Victim’s data can be collected by phishers manually or through automated techniques ( Jakobsson et al., 2007 ).
The data collection can be conducted either during or after the victim’s interaction with the attacker. However, to collect data manually simple techniques are used wherein victims interact directly with the phisher depending on relationships within social networks or other human deception techniques ( Ollmann, 2004 ). Whereas in automated data collection, several techniques can be used such as fake web forms that are used in web spoofing ( Dhamija et al., 2006 ). Additionally, the victim’s public data such as the user’s profile in social networks can be used to collect the victim’s background information that is required to initialize social engineering attacks ( Wenyin et al., 2005 ). In VoIP attacks or phone attack techniques such as recorded messages are used to harvest user's data ( Huber et al., 2009 ).
Types and Techniques of Phishing Attacks
Phishers conduct their attack either by using psychological manipulation of individuals into disclosing personal information (i.e., deceptive attack as a form of social engineering) or using technical methods. Phishers, however, usually prefer deceptive attacks by exploiting human psychology rather than technical methods. Figure 9 illustrates the types of phishing and techniques used by phishers to conduct a phishing attack. Each type and technique is explained in subsequent sections and subsections.
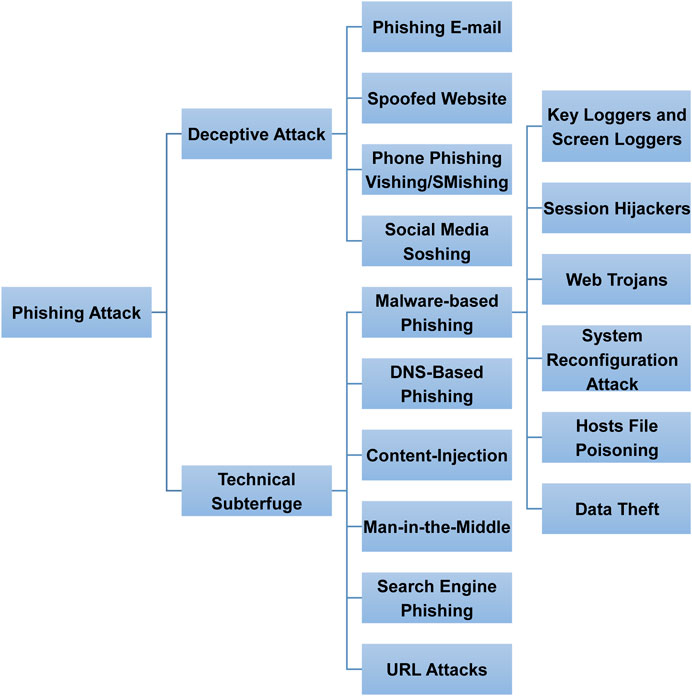
FIGURE 9 . Phishing attack types and techniques drawing upon existing phishing attacks.
Deceptive Phishing
Deceptive phishing is the most common type of phishing attack in which the attacker uses social engineering techniques to deceive victims. In this type of phishing, a phisher uses either social engineering tricks by making up scenarios (i.e., false account update, security upgrade), or technical methods (i.e., using legitimate trademarks, images, and logos) to lure the victim and convince them of the legitimacy of the forged email ( Jakobsson and Myers, 2006 ). By believing these scenarios, the user will fall prey and follow the given link, which leads to disclose his personal information to the phisher.
Deceptive phishing is performed through phishing emails; fake websites; phone phishing (Scam Call and IM); social media; and via many other mediums. The most common social phishing types are discussed below;
Phishing e-Mail
The most common threat derived by an attacker is deceiving people via email communications and this remains the most popular phishing type to date. A Phishing email or Spoofed email is a forged email sent from an untrusted source to thousands of victims randomly. These fake emails are claiming to be from a person or financial institution that the recipient trusts in order to convince recipients to take actions that lead them to disclose their sensitive information. A more organized phishing email that targets a particular group or individuals within the same organization is called spear phishing. In the above type, the attacker may gather information related to the victim such as name and address so that it appears to be credible emails from a trusted source ( Wang et al., 2008 ), and this is linked to the planning phase of the phishing anatomy proposed in this article. A more sophisticated form of spear phishing is called whaling, which targets high-rank people such as CEOs and CFOs. Some examples of spear-phishing attack victims in early 2016 are the phishing email that hacked the Clinton campaign chairman John Podesta’s Gmail account ( Parmar, 2012 ). Clone phishing is another type of email phishing, where the attacker clones a legitimate and previously delivered email by spoofing the email address and using information related to the recipient such as addresses from the legitimate email with replaced links or malicious attachments ( Krawchenko, 2016 ). The basic scenario for this attack is illustrated previously in Figure 4 and can be described in the following steps.
1. The phisher sets up a fraudulent email containing a link or an attachment (planning phase).
2. The phisher executes the attack by sending a phishing email to the potential victim using an appropriate medium (attack conducting phase).
3. The link (if clicked) directs the user to a fraudulent website, or to download malware in case of clicking the attachment (interaction phase).
4. The malicious website prompts users to provide confidential information or credentials, which are then collected by the attacker and used for fraudulent activities. (Valuables acquisition phase).
Often, the phisher does not use the credentials directly; instead, they resell the obtained credentials or information on a secondary market ( Jakobsson and Myers, 2006 ), for instance, script kiddies might sell the credentials on the dark web.
Spoofed Website
This is also called phishing websites, in which phishers forge a website that appears to be genuine and looks similar to the legitimate website. An unsuspicious user is redirected to this website after clicking a link embedded within an email or through an advertisement (clickjacking) or any other way. If the user continues to interact with the spoofed website, sensitive information will be disclosed and harvested by the phisher ( CSIOnsite, 2012 ).
Phone Phishing (Vishing and SMishing)
This type of phishing is conducted through phone calls or text messages, in which the attacker pretends to be someone the victim knows or any other trusted source the victim deals with. A user may receive a convincing security alert message from a bank convincing the victim to contact a given phone number with the aim to get the victim to share passwords or PIN numbers or any other Personally Identifiable Information (PII). The victim may be duped into clicking on an embedded link in the text message. The phisher then could take the credentials entered by the victim and use them to log in to the victims' instant messaging service to phish other people from the victim’s contact list. A phisher could also make use of Caller IDentification (CID) 3 spoofing to dupe the victim that the call is from a trusted source or by leveraging from an internet protocol private branch exchange (IP PBX) 4 tools which are open-source and software-based that support VoIP ( Aburrous et al., 2008 ). A new report from Fraud Watch International about phishing attack trends for 2019 anticipated an increase in SMishing where the text messages content is only viewable on a mobile device ( FraudWatchInternational, 2019 ).
Social Media Attack (Soshing, Social Media Phishing)
Social media is the new favorite medium for cybercriminals to conduct their phishing attacks. The threats of social media can be account hijacking, impersonation attacks, scams, and malware distributing. However, detecting and mitigating these threats requires a longer time than detecting traditional methods as social media exists outside of the network perimeter. For example, the nation-state threat actors conducted an extensive series of social media attacks on Microsoft in 2014. Multiple Twitter accounts were affected by these attacks and passwords and emails for dozens of Microsoft employees were revealed ( Ramzan, 2010 ). According to Kaspersky Lab’s, the number of phishing attempts to visit fraudulent social network pages in the first quarter of 2018 was more than 3.7 million attempts, of which 60% were fake Facebook pages ( Raggo, 2016 ).
The new report from predictive email defense company Vade Secure about phishers’ favorites for quarter 1 and quarter 2 of 2019, stated that Soshing primarily on Facebook and Instagram saw a 74.7% increase that is the highest quarter-over- quarter growth of any industry ( VadeSecure, 2021 ).
Technical Subterfuge
Technical subterfuge is the act of tricking individuals into disclosing their sensitive information through technical subterfuge by downloading malicious code into the victim's system. Technical subterfuge can be classified into the following types:
Malware-Based Phishing
As the name suggests, this is a type of phishing attack which is conducted by running malicious software on a user’s machine. The malware is downloaded to the victim’s machine, either by one of the social engineering tricks or technically by exploiting vulnerabilities in the security system (e.g., browser vulnerabilities) ( Jakobsson and Myers, 2006 ). Panda malware is one of the successful malware programs discovered by Fox-IT Company in 2016. This malware targets Windows Operating Systems (OS). It spreads through phishing campaigns and its main attack vectors include web injects, screenshots of user activity (up to 100 per mouse click), logging of keyboard input, Clipboard pastes (to grab passwords and paste them into form fields), and exploits to the Virtual Network Computing (VNC) desktop sharing system. In 2018, Panda malware expanded its targets to include cryptocurrency exchanges and social media sites ( F5Networks, 2018 ). There are many forms of Malware-based phishing attacks; some of them are discussed below:
Key Loggers and Screen Loggers
Loggers are the type of malware used by phishers and installed either through Trojan horse email attachments or through direct download to the user’s personal computer. This software monitors data and records user keystrokes and then sends it to the phisher. Phisher uses the key loggers to capture sensitive information related to victims, such as names, addresses, passwords, and other confidential data. Key loggers can also be used for non-phishing purposes such as to monitor a child's use of the internet. Key loggers can also be implemented in many other ways such as detecting URL changes and logs information as Browser Helper Object (BHO) that enables the attacker to take control of the features of all IE’s, monitoring keyboard and mouse input as a device driver and, monitoring users input and displays as a screen logger ( Jakobsson and Myers, 2006 ).
Viruses and Worms
A virus is a type of malware, which is a piece of code spreading in another application or program by making copies of itself in a self-automated manner ( Jakobsson and Myers, 2006 ; F5Networks, 2018 ). Worms are similar to viruses but they differ in the execution manner, as worms are executed by exploiting the operating systems vulnerability without the need to modify another program. Viruses transfer from one computer to another with the document that they are attached to, while worms transfer through the infected host file. Both viruses and worms can cause data and software damaging or Denial-of-Service (DoS) conditions ( F5Networks, 2018 ).
Spying software is a malicious code designed to track the websites visited by users in order to steal sensitive information and conduct a phishing attack. Spyware can be delivered through an email and, once it is installed on the computer, take control over the device and either change its settings or gather information such as passwords and credit card numbers or banking records which can be used for identity theft ( Jakobsson and Myers, 2006 ).
Adware is also known as advertising-supported software ( Jakobsson and Myers, 2006 ). Adware is a type of malware that shows the user an endless pop-up window with ads that could harm the performance of the device. Adware can be annoying but most of it is safe. Some of the adware could be used for malicious purposes such as tracking the internet sites the user visits or even recording the user's keystrokes ( cisco, 2018 ).
Ransomware is a type of malware that encrypts the user's data after they run an executable program on the device. In this type of attack, the decryption key is held until the user pays a ransom (cisco, 2018). Ransomware is responsible for tens of millions of dollars in extortion annually. Worse still, this is hard to detect with developing new variants, facilitating the evasion of many antivirus and intrusion detection systems ( Latto, 2020 ). Ransomware is usually delivered to the victim's device through phishing emails. According to a report ( PhishMe, 2016 ), 93% of all phishing emails contained encryption ransomware. Phishing, as a social engineering attack, convinces victims into executing actions without knowing about the malicious program.
A rootkit is a collection of programs, typically malicious, that enables access to a computer or computer network. These toolsets are used by intruders to hide their actions from system administrators by modifying the code of system calls and changing the functionality ( Belcic, 2020 ). The term “rootkit” has negative connotations through its association with malware, and it is used by the attacker to alert existing system tools to escape detection. These kits enable individuals with little or no knowledge to launch phishing exploits. It contains coding, mass emailing software (possibly with thousands of email addresses included), web development software, and graphic design tools. An example of rootkits is the Kernel kit. Kernel-Level Rootkits are created by replacing portions of the core operating system or adding new code via Loadable Kernel Modules in (Linux) or device drivers (in Windows) ( Jakobsson and Myers, 2006 ).
Session Hijackers
In this type, the attacker monitors the user’s activities by embedding malicious software within a browser component or via network sniffing. The monitoring aims to hijack the session, so that the attacker performs an unauthorized action with the hijacked session such as financial transferring, without the user's permission ( Jakobsson and Myers, 2006 ).
Web Trojans
Web Trojans are malicious programs that collect user’s credentials by popping up in a hidden way over the login screen ( Jakobsson and Myers, 2006 ). When the user enters the credentials, these programs capture and transmit the stolen credentials directly to the attacker ( Jakobsson et al., 2007 ).
Hosts File Poisoning
This is a way to trick a user into going to the phisher’s site by poisoning (changing) the host’s file. When the user types a particular website address in the URL bar, the web address will be translated into a numeric (IP) address before visiting the site. The attacker, to take the user to a fake website for phishing purposes, will modify this file (e.g., DNS cache). This type of phishing is hard to detect even by smart and perceptive users ( Ollmann, 2004 ).
System Reconfiguration Attack
In this format of the phishing attack, the phisher manipulates the settings on a user’s computer for malicious activities so that the information on this PC will be compromised. System reconfigurations can be changed using different methods such as reconfiguring the operating system and modifying the user’s Domain Name System (DNS) server address. The wireless evil twin is an example of a system reconfiguration attack in which all user’s traffic is monitored via a malicious wireless Access Point (AP) ( Jakobsson and Myers, 2006 ).
Data theft is an unauthorized accessing and stealing of confidential information for a business or individuals. Data theft can be performed by a phishing email that leads to the download of a malicious code to the user's computer which in turn steals confidential information stored in that computer directly ( Jakobsson and Myers, 2006 ). Stolen information such as passwords, social security numbers, credit card information, sensitive emails, and other personal data could be used directly by a phisher or indirectly by selling it for different purposes.
Domain Name System Based Phishing (Pharming)
Any form of phishing that interferes with the domain name system so that the user will be redirected to the malicious website by polluting the user's DNS cache with wrong information is called DNS-based phishing. Although the host’s file is not a part of the DNS, the host’s file poisoning is another form of DNS based phishing. On the other hand, by compromising the DNS server, the genuine IP addresses will be modified which results in taking the user unwillingly to a fake location. The user can fall prey to pharming even when clicking on a legitimate link because the website’s domain name system (DNS) could be hijacked by cybercriminals ( Jakobsson and Myers, 2006 ).
Content Injection Phishing
Content-Injection Phishing refers to inserting false content into a legitimate site. This malicious content could misdirect the user into fake websites, leading users into disclosing their sensitive information to the hacker or it can lead to downloading malware into the user's device ( Jakobsson and Myers, 2006 ). The malicious content could be injected into a legitimate site in three primary ways:
1. Hacker exploits a security vulnerability and compromises a web server.
2. Hacker exploits a Cross-Site Scripting (XSS) vulnerability that is a programming flaw that enables attackers to insert client-side scripts into web pages, which will be viewed by the visitors to the targeted site.
3. Hacker exploits Structured Query Language (SQL) injection vulnerability, which allows hackers to steal information from the website’s database by executing database commands on a remote server.
Man-In-The-Middle Phishing
The Man In The Middle attack (MITM) is a form of phishing, in which the phishers insert communications between two parties (i.e. the user and the legitimate website) and tries to obtain the information from both parties by intercepting the victim’s communications ( Ollmann, 2004 ). Such that the message is going to the attacker instead of going directly to the legitimate recipients. For a MITM, the attacker records the information and misuse it later. The MITM attack conducts by redirecting the user to a malicious server through several techniques such as Address Resolution Protocol (ARP) poisoning, DNS spoofing, Trojan key loggers, and URL Obfuscation ( Jakobsson and Myers, 2006 ).
Search Engine Phishing
In this phishing technique, the phisher creates malicious websites with attractive offers and use Search Engine Optimization (SEO) tactics to have them indexed legitimately such that it appears to the user when searching for products or services. This is also known as black hat SEO ( Jakobsson and Myers, 2006 ).
URL and HTML Obfuscation Attacks
In most of the phishing attacks, phishers aim to convince a user to click on a given link that connects the victim to a malicious phishing server instead of the destination server. This is the most popular technique used by today's phishers. This type of attack is performed by obfuscating the real link (URL) that the user intends to connect (an attempt from the attacker to make their web address look like the legitimate one). Bad Domain Names and Host Name Obfuscation are common methods used by attackers to fake an address ( Ollmann, 2004 ).
Countermeasures
A range of solutions are being discussed and proposed by the researchers to overcome the problems of phishing, but still, there is no single solution that can be trusted or capable of mitigating these attacks ( Hong, 2012 ; Boddy, 2018 ; Chanti and Chithralekha, 2020 ). The proposed phishing countermeasures in the literature can be categorized into three major defense strategies. The first line of defense is human-based solutions by educating end-users to recognize phishing and avoid taking the bait. The second line of defense is technical solutions that involve preventing the attack at early stages such as at the vulnerability level to prevent the threat from materializing at the user's device, which means decreasing the human exposure, and detecting the attack once it is launched through the network level or at the end-user device. This also includes applying specific techniques to track down the source of the attack (for example these could include identification of new domains registered that are closely matched with well-known domain names). The third line of defense is the use of law enforcement as a deterrent control. These approaches can be combined to create much stronger anti-phishing solutions. The above solutions are discussed in detail below.
Human Education (Improving User Awareness About Phishing)
Human education is by far an effective countermeasure to avoid and prevent phishing attacks. Awareness and human training are the first defense approach in the proposed methodology for fighting against phishing even though it does not assume complete protection ( Hong, 2012 ). End-user education reduces user's susceptibility to phishing attacks and compliments other technical solutions. According to the analysis carried out in ( Bailey et al., 2008 ), 95% of phishing attacks are caused due to human errors; nonetheless, existing phishing detection training is not enough for combating current sophisticated attacks. In the study presented by Khonji et al. (2013) , security experts contradict the effectiveness and usability of user education. Furthermore, some security experts claim that user education is not effective as security is not the main goal for users and users do not have a motivation to educate themselves about phishing ( Scaife et al., 2016 ), while others confirm that user education could be effective if designed properly ( Evers, 2006 ; Whitman and Mattord, 2012 ). Moreover, user training has been mentioned by many researchers as an effective way to protect users when they are using online services ( Dodge et al., 2007 ; Salem et al., 2010 ; Chanti and Chithralekha, 2020 ). To detect and avoid phishing emails, a combined training approach was proposed by authors in the study ( Salem et al., 2010 ). The proposed solution uses a combination of tools and human learning, wherein a security awareness program is introduced to the user as a first step. The second step is using an intelligent system that detects the attacks at the email level. After that, the emails are classified by a fuzzy logic-based expert system. The main critic of this method is that the study chooses only limited characteristics of the emails as distinguishing features ( Kumaraguru et al., 2010 ; CybintCyberSolutions, 2018 ). Moreover, the majority of phishing training programs focus on how to recognize and avoid phishing emails and websites while other threatening phishing types receive less attention such as voice phishing and malware or adware phishing. The authors in ( Salem et al., 2010 ) found that the most used solutions in educating people are not useful if they ignore the notifications/warnings about fake websites. Training users should involve three major directions: the first one is awareness training through holding seminars or online courses for both employees within organizations or individuals. The second one is using mock phishing attacks to attack people to test users’ vulnerability and allow them to assess their own knowledge about phishing. However, only 38% of global organizations claim they are prepared to handle a sophisticated cyber-attack ( Kumaraguru et al., 2010 ). Wombat Security’s State of the Phish™ Report 2018 showed that approximately two-fifths of American companies use computer-based online awareness training and simulated phishing attacks as educating tools on a monthly basis, while just 15% of United Kingdom firms do so ( CybintCyberSolutions, 2018 ). The third direction is educating people by developing games to teach people about phishing. The game developer should take into consideration different aspects before designing the game such as audience age and gender, because people's susceptibility to phishing is varying. Authors in the study ( Sheng et al., 2007 ) developed a game to train users so that they can identify phishing attacks called Anti-Phishing Phil that teaches about phishing web pages, and then tests users about the efficiency and effectiveness of the game. The results from the study showed that the game participants improve their ability to identify phishing by 61% indicating that interactive games might turn out to be a joyful way of educating people. Although, user’s education and training can be very effective to mitigate security threats, phishing is becoming more complex and cybercriminals can fool even the security experts by creating convincing spear phishing emails via social media. Therefore, individual users and employees must have at least basic knowledge about dealing with suspicious emails and report it to IT staff and specific authorities. In addition, phishers change their strategies continuously, which makes it harder for organizations, especially small/medium enterprises to afford the cost of their employee education. With millions of people logging on to their social media accounts every day, social media phishing is phishers' favorite medium to deceive their victims. For example, phishers are taking advantage of the pervasiveness of Facebook to set up creative phishing attacks utilizing the Facebook Login feature that enables the phisher to compromise all the user's accounts with the same credentials (VadeSecure). Some countermeasures are taken by Social networks to reduce suspicious activities on social media such as Two-Factor authentication for logging in, that is required by Facebook, and machine-learning techniques used by Snapchat to detect and prevent suspicious links sent within the app ( Corrata, 2018 ). However, countermeasures to control Soshing and phone phishing attacks might include:
• Install anti-virus, anti-spam software as a first action and keep it up to date to detect and prevent any unauthorized access.
• Educate yourself about recent information on phishing, the latest trends, and countermeasures.
• Never click on hyperlinks attached to a suspicious email, post, tweet, direct message.
• Never trust social media, do not give any sensitive information over the phone or non-trusted account. Do not accept friend requests from people you do not know.
• Use a unique password for each account.
Training and educating users is an effective anti-phishing countermeasure and has already shown promising initial results. The main downside of this solution is that it demands high costs ( Dodge et al., 2007 ). Moreover, this solution requires basic knowledge in computer security among trained users.
Technical Solutions
The proposed technical solutions for detecting and blocking phishing attacks can be divided into two major approaches: non-content based solutions and content-based solutions ( Le et al., 2006 ; Bin et al., 2010 ; Boddy, 2018 ). Both approaches are briefly described in this section. Non-content based methods include blacklists and whitelists that classify the fake emails or webpages based on the information that is not part of the email or the webpage such as URL and domain name features ( Dodge et al., 2007 ; Ma et al., 2009 ; Bin et al., 2010 ; Salem et al., 2010 ). Stopping the phishing sites using blacklist and whitelist approaches, wherein a list of known URLs and sites is maintained, the website under scrutiny is checked against such a list in order to be classified as a phishing or legitimate site. The downside of this approach is that it will not identify all phishing websites. Because once a phishing site is taken down, the phisher can easily register a new domain ( Miyamoto et al., 2009 ). Content-based methods classify the page or the email relying on the information within its content such as texts, images, and also HTML, java scripts, and Cascading Style Sheets (CSS) codes ( Zhang et al., 2007 ; Maurer and Herzner, 2012 ). Content-based solutions involve Machine Learning (ML), heuristics, visual similarity, and image processing methods ( Miyamoto et al., 2009 ; Chanti and Chithralekha, 2020 ). and finally, multifaceted methods, which apply a combination of the previous approaches to detect and prevent phishing attacks ( Afroz and Greenstadt, 2009 ). For email filtering, ML techniques are commonly used for example in 2007, the first email phishing filter was developed by authors in ( Fette et al., 2007 ). This technique uses a set of features such as URLs that use different domain names. Spam filtering techniques ( Cormack et al., 2011 ) and statistical classifiers ( Bergholz et al., 2010 ) are also used to identify a phishing email. Authentication and verification technologies are also used in spam email filtering as an alternative to heuristics methods. For example, the Sender Policy Framework (SPF) verifies whether a sender is valid when accepting mail from a remote mail server or email client ( Deshmukh and raddha Popat, 2017 ).
The technical solutions for Anti-phishing are available at different levels of the delivery chain such as mail servers and clients, Internet Service Providers (ISPs), and web browser tools. Drawing from the proposed anatomy for phishing attacks in Proposed Phishing Anatomy , authors categorize technical solutions into the following approaches:
1. Techniques to detect the attack after it has been launched. Such as by scanning the web to find fake websites. For example, content-based phishing detection approaches are heavily deployed on the Internet. The features from the website elements such as Image, URL, and text content are analyzed using Rule-based approaches and Machine Learning that examine the presence of special characters (@), IP addresses instead of the domain name, prefix/suffix, HTTPS in domain part and other features ( Jeeva and Rajsingh, 2016 ). Fuzzy Logic (FL) has also been used as an anti-phishing model to help classify websites into legitimate or ‘phishy’ as this model deals with intervals rather than specific numeric values ( Aburrous et al., 2008 ).
2. Techniques to prevent the attack from reaching the user's system. Phishing prevention is an important step to defend against phishing by blocking a user from seeing and dealing with the attack. In email phishing, anti-spam software tools can block suspicious emails. Phishers usually send a genuine look-alike email that dupes the user to open an attachment or click on a link. Some of these emails pass the spam filter because phishers use misspelled words. Therefore, techniques that detect fake emails by checking the spelling and grammar correction are increasingly used, so that it can prevent the email from reaching the user's mailbox. Authors in the study ( Fette et al., 2007 ) have developed a new classification algorithm based on the Random Forest algorithm after exploring email phishing utilizing the C4.5 decision tree generator algorithm. The developed method is called "Phishing Identification by Learning on Features of Email Received" (PILFER), which can classify phishing email depending on various features such as IP based URLs, the number of links in the HTML part(s) of an email, the number of domains, the number of dots, nonmatching URLs, and availability of JavaScripts. The developed method showed high accuracy in detecting phishing emails ( Afroz and Greenstadt, 2009 ).
3. Corrective techniques that can take down the compromised website, by requesting the website's Internet Service Provider (ISP) to shut down the fake website in order to prevent more users from falling victims to phishing ( Moore and Clayton, 2007 ; Chanti and Chithralekha, 2020 ). ISPs are responsible for taking down fake websites. Removing the compromised and illegal websites is a complex process; many entities are involved in this process from private companies, self-regulatory bodies, government agencies, volunteer organizations, law enforcement, and service providers. Usually, illegal websites are taken down by Takedown Orders, which are issued by courts or in some jurisdictions by law enforcement. On the other hand, these can be voluntarily taken down by the providers themselves as a result of issued takedown notices ( Moore and Clayton, 2007 ; Hutchings et al., 2016 ). According to PHISHLABS ( PhishLabs, 2019 ) report, taking down phishing sites is helpful but it is not completely effective as these sites can still be alive for days stealing customers' credentials before detecting the attack.
4. Warning tools or security indicators that embedded into the web browser to inform the user after detecting the attack. For example, eBay Toolbar and Account Guard ( eBay Toolbar and Account Guard, 2009 ) protect customer’s eBay and PayPal passwords respectively by alerting the users about the authenticity of the sites that users try to type the password in. Numerous anti-phishing solutions rely mainly on warnings that are displayed on the security toolbar. In addition, some toolbars block suspicious sites to warn about it such as McAfee and Netscape. A study presented in ( Robichaux and Ganger, 2006 ) conducted a test to evaluate the performance of eight anti-phishing solutions, including Microsoft Internet Explorer 7, EarthLink, eBay, McAfee, GeoTrust, Google using Firefox, Netscape, and Netcraft. These tools are warning and blocking tools that allow legitimate sites while block and warn about known phishing sites. The study also found that Internet Explorer and Netcraft Toolbar showed the most effective results than other anti-phishing tools. However, security toolbars are still failing to avoid people falling victim to phishing despite these toolbars improving internet security in general ( Abu-Nimeh and Nair, 2008 ).
5. Authentication ( Moore and Clayton, 2007 ) and authorization ( Hutchings et al., 2016 ) techniques that provide protection from phishing by verifying the identity of the legitimate person. This prevents phishers from accessing a protected resource and conducting their attack. There are three types of authentication; single-factor authentication requires only username and password. The second type is two-factor authentication that requires additional information in addition to the username and password such as an OTP (One-Time Password) which is sent to the user’s email id or phone. The third type is multi-factor authentication using more than one form of identity (i.e., a combination of something you know, something you are, and something you have). Some widely used methods in the authorization process are API authorization and OAuth 2.0 that allow the previously generated API to access the system.
However, the progressive increase in phishing attacks shows that previous methods do not provide the required protection against most existing phishing attacks. Because no single solution or technology could prevent all phishing attacks. An effective anti-phishing solution should be based on a combination of technical solutions and increased user awareness ( Boddy, 2018 ).
Solutions Provided by Legislations as a Deterrent Control
A cyber-attack is considered a crime when an individual intentionally accesses personal information on a computer without permission, even if the individual does not steal information or damage the system ( Mince-Didier, 2020 ). Since the sole objective of almost all phishing attacks is to obtain sensitive information by knowingly intending to commit identity theft, and while there are currently no federal laws in the United States aimed specifically at phishing, therefore, phishing crimes are usually covered under identity theft laws. Phishing is considered a crime even if the victim does not actually fall for the phishing scam, the punishments depend on circumstances and usually include jail, fines, restitution, probation ( Nathan, 2020 ). Phishing attacks are causing different levels of damages to the victims such as financial and reputational losses. Therefore, law enforcement authorities should track down these attacks in order to punish the criminal as with real-world crimes. As a complement to technical solutions and human education, the support provided by applicable laws and regulations can play a vital role as a deterrent control. Increasingly authorities around the world have created several regulations in order to mitigate the increase of phishing attacks and their impact. The first anti-phishing laws were enacted by the United States, where the FTC in the US added the phishing attacks to the computer crime list in January 2004. A year later, the ‘‘Anti-Phishing Act’’ was introduced in the US Congress in March 2005 ( Mohammad et al., 2014 ). Meanwhile, in the United Kingdom, the law legislation is gradually conforming to address phishing and other forms of cyber-crime. In 2006, the United Kingdom government improved the Computer Misuse Act 1990 intending to bring it up to date with developments in computer crime and to increase penalties for breach enacted penalties of up to 10 years ( eBay Toolbar and Account Guard, 2009 ; PhishLabs, 2019 ). In this regard, a student in the United Kingdom who made hundreds of thousands of pounds blackmailing pornography website users was jailed in April 2019 for six years and five months. According to the National Crime Agency (NCA), this attacker was the most prolific cybercriminal to be sentenced in the United Kingdom ( Casciani, 2019 ). Moreover, the organizations bear part of the responsibility in protecting personal information as stated in the Data Protection Act 2018 and EU General Data Protection Regulation (GDPR). Phishing websites also can be taken down through Law enforcement agencies' conduct. In the United Kingdom, websites can be taken down by the National Crime Agency (NCA), which includes the National Cyber Crime Unit, and by the City of London Police, which includes the Police Intellectual Property Crime Unit (PIPCU) and the National Fraud Intelligence Bureau (NFIB) ( Hutchings et al., 2016 ).
However, anti-phishing law enforcement is still facing numerous challenges and limitations. Firstly, after perpetrating the phishing attack, the phisher can vanish in cyberspace making it difficult to prove the guilt attributed to the offender and to recover the damages caused by the attack, limiting the effectiveness of the law enforcement role. Secondly, even if the attacker’s identity is disclosed in the case of international attackers, it will be difficult to bring this attacker to justice because of the differences in countries' legislations (e.g., exchange treaties). Also, the attack could be conducted within a short time span, for instance, the average lifetime for a phishing web site is about 54 h as stated by the APWG, therefore, there must be a quick response from the government and the authorities to detect, control and identify the perpetrators of the attack ( Ollmann, 2004 ).
Phishing attacks remain one of the major threats to individuals and organizations to date. As highlighted in the article, this is mainly driven by human involvement in the phishing cycle. Often phishers exploit human vulnerabilities in addition to favoring technological conditions (i.e., technical vulnerabilities). It has been identified that age, gender, internet addiction, user stress, and many other attributes affect the susceptibility to phishing between people. In addition to traditional phishing channels (e.g., email and web), new types of phishing mediums such as voice and SMS phishing are on the increase. Furthermore, the use of social media-based phishing has increased in use in parallel with the growth of social media. Concomitantly, phishing has developed beyond obtaining sensitive information and financial crimes to cyber terrorism, hacktivism, damaging reputations, espionage, and nation-state attacks. Research has been conducted to identify the motivations and techniques and countermeasures to these new crimes, however, there is no single solution for the phishing problem due to the heterogeneous nature of the attack vector. This article has investigated problems presented by phishing and proposed a new anatomy, which describes the complete life cycle of phishing attacks. This anatomy provides a wider outlook for phishing attacks and provides an accurate definition covering end-to-end exclusion and realization of the attack.
Although human education is the most effective defense for phishing, it is difficult to remove the threat completely due to the sophistication of the attacks and social engineering elements. Although, continual security awareness training is the key to avoid phishing attacks and to reduce its impact, developing efficient anti-phishing techniques that prevent users from being exposed to the attack is an essential step in mitigating these attacks. To this end, this article discussed the importance of developing anti-phishing techniques that detect/block the attack. Furthermore, the importance of techniques to determine the source of the attack could provide a stronger anti-phishing solution as discussed in this article.
Furthermore, this article identified the importance of law enforcement as a deterrent mechanism. Further investigations and research are necessary as discussed below.
1. Further research is necessary to study and investigate susceptibility to phishing among users, which would assist in designing stronger and self-learning anti-phishing security systems.
2. Research on social media-based phishing, Voice Phishing, and SMS Phishing is sparse and these emerging threats are predicted to be significantly increased over the next years.
3. Laws and legislations that apply for phishing are still at their infant stage, in fact, there are no specific phishing laws in many countries. Most of the phishing attacks are covered under traditional criminal laws such as identity theft and computer crimes. Therefore, drafting of specific laws for phishing is an important step in mitigating these attacks in a time where these crimes are becoming more common.
4. Determining the source of the attack before the end of the phishing lifecycle and enforcing law legislation on the offender could help in restricting phishing attacks drastically and would benefit from further research.
It can be observed that the mediums used for phishing attacks have changed from traditional emails to social media-based phishing. There is a clear lag between sophisticated phishing attacks and existing countermeasures. The emerging countermeasures should be multidimensional to tackle both human and technical elements of the attack. This article provides valuable information about current phishing attacks and countermeasures whilst the proposed anatomy provides a clear taxonomy to understand the complete life cycle of phishing.
Author Contributions
This work is by our PhD student ZA supported by her Supervisory Team.
Conflict of Interest
The authors declare that the research was conducted in the absence of any commercial or financial relationships that could be construed as a potential conflict of interest.
AOL America Online
APWG Anti Phishing Working Group Advanced
APRANET Advanced Research Projects Agency Network.
ARP address resolution protocol.
BHO Browser Helper Object
BEC business email compromise
COVID-19 Coronavirus disease 2019
CSS cascading style sheets
DDoS distributed denial of service
DNS Domain Name System
DoS Denial of Service
FTC Federal Trade Commission
FL Fuzzy Logic
HTTPS Hypertext Transfer Protocol Secure
IE Internet Explorer
ICT Information and Communications Technology
IM Instant Message
IT Information Technology
IP Internet Protocol
MITM Man-in-the-Middle
NCA National Crime Agency
NFIB National Fraud Intelligence Bureau
PIPCU Police Intellectual Property Crime Unit
OS Operating Systems
PBX Private Branch Exchange
SMishing Text Message Phishing
SPF Sender Policy Framework
SMTP Simple Mail Transfer Protocol
SMS Short Message Service
Soshing Social Media Phishing
SQL structured query language
URL Uniform Resource Locator
UK United Kingdom
US United States
USB Universal Serial Bus
US-CERT United States Computer Emergency Readiness Team.
Vishing Voice Phishing
VNC Virtual Network Computing
VoIP Voice over Internet Protocol
XSS Cross-Site Scripting
1 Proofpoint is “a leading cybersecurity company that protects organizations’ greatest assets and biggest risks: their people. With an integrated suite of cloud-based solutions”( Proofpoint, 2019b ).
2 APWG Is “the international coalition unifying the global response to cybercrime across industry, government and law-enforcement sectors and NGO communities” ( APWG, 2020 ).
3 CalleR ID is “a telephone facility that displays a caller’s phone number on the recipient's phone device before the call is answered” ( Techpedia, 2021 ).
4 An IPPBX is “a telephone switching system within an enterprise that switches calls between VoIP users on local lines while allowing all users to share a certain number of external phone lines” ( Margaret, 2008 ).
Abad, C. (2005). The economy of phishing: a survey of the operations of the phishing market. First Monday 10, 1–11. doi:10.5210/fm.v10i9.1272
CrossRef Full Text | Google Scholar
Abu-Nimeh, S., and Nair, S. (2008). “Bypassing security toolbars and phishing filters via dns poisoning,” in IEEE GLOBECOM 2008–2008 IEEE global telecommunications conference , New Orleans, LA , November 30–December 2, 2008 ( IEEE) , 1–6. doi:10.1109/GLOCOM.2008.ECP.386
Aburrous, M., Hossain, M. A., Thabatah, F., and Dahal, K. (2008). “Intelligent phishing website detection system using fuzzy techniques,” in 2008 3rd international conference on information and communication technologies: from theory to applications (New York, NY: IEEE , 1–6. doi:10.1109/ICTTA.2008.4530019
Afroz, S., and Greenstadt, R. (2009). “Phishzoo: an automated web phishing detection approach based on profiling and fuzzy matching,” in Proceeding 5th IEEE international conference semantic computing (ICSC) , 1–11.
Google Scholar
Alsharnouby, M., Alaca, F., and Chiasson, S. (2015). Why phishing still works: user strategies for combating phishing attacks. Int. J. Human-Computer Stud. 82, 69–82. doi:10.1016/j.ijhcs.2015.05.005
APWG (2018). Phishing activity trends report 3rd quarter 2018 . US. 1–11.
APWG (2020). APWG phishing attack trends reports. 2020 anti-phishing work. Group, Inc Available at: https://apwg.org/trendsreports/ (Accessed September 20, 2020).
Arachchilage, N. A. G., and Love, S. (2014). Security awareness of computer users: a phishing threat avoidance perspective. Comput. Hum. Behav. 38, 304–312. doi:10.1016/j.chb.2014.05.046
Arnsten, B. A., Mazure, C. M., and April, R. S. (2012). Everyday stress can shut down the brain’s chief command center. Sci. Am. 306, 1–6. Available at: https://www.scientificamerican.com/article/this-is-your-brain-in-meltdown/ (Accessed October 15, 2019).
Bailey, J. L., Mitchell, R. B., and Jensen, B. k. (2008). “Analysis of student vulnerabilities to phishing,” in 14th americas conference on information systems, AMCIS 2008 , 75–84. Available at: https://aisel.aisnet.org/amcis2008/271 .
Barracuda (2020). Business email compromise (BEC). Available at: https://www.barracuda.com/glossary/business-email-compromise (Accessed November 15, 2020).
Belcic, I. (2020). Rootkits defined: what they do, how they work, and how to remove them. Available at: https://www.avast.com/c-rootkit (Accessed November 7, 2020).
Bergholz, A., De Beer, J., Glahn, S., Moens, M.-F., Paaß, G., and Strobel, S. (2010). New filtering approaches for phishing email. JCS 18, 7–35. doi:10.3233/JCS-2010-0371
Bin, S., Qiaoyan, W., and Xiaoying, L. (2010). “A DNS based anti-phishing approach.” in 2010 second international conference on networks security, wireless communications and trusted computing , Wuhan, China , April 24–25, 2010 . ( IEEE ), 262–265. doi:10.1109/NSWCTC.2010.196
Boddy, M. (2018). Phishing 2.0: the new evolution in cybercrime. Comput. Fraud Secur. 2018, 8–10. doi:10.1016/S1361-3723(18)30108-8
Casciani, D. (2019). Zain Qaiser: student jailed for blackmailing porn users worldwide. Available at: https://www.bbc.co.uk/news/uk-47800378 (Accessed April 9, 2019).
Chanti, S., and Chithralekha, T. (2020). Classification of anti-phishing solutions. SN Comput. Sci. 1, 11. doi:10.1007/s42979-019-0011-2
Checkpoint (2020). Check point research’s Q1 2020 brand phishing report. Available at: https://www.checkpoint.com/press/2020/apple-is-most-imitated-brand-for-phishing-attempts-check-point-researchs-q1-2020-brand-phishing-report/ (Accessed August 6, 2020).
cisco (2018). What is the difference: viruses, worms, Trojans, and bots? Available at: https://www.cisco.com/c/en/us/about/security-center/virus-differences.html (Accessed January 20, 2020).
CISA (2018). What is phishing. Available at: https://www.us-cert.gov/report-phishing (Accessed June 10, 2019).
Cormack, G. V., Smucker, M. D., and Clarke, C. L. A. (2011). Efficient and effective spam filtering and re-ranking for large web datasets. Inf. Retrieval 14, 441–465. doi:10.1007/s10791-011-9162-z
Corrata (2018). The rising threat of social media phishing attacks. Available at: https://corrata.com/the-rising-threat-of-social-media-phishing-attacks/%0D (Accessed October 29, 2019).
Crane, C. (2019). The dirty dozen: the 12 most costly phishing attack examples. Available at: https://www.thesslstore.com/blog/the-dirty-dozen-the-12-most-costly-phishing-attack-examples/#:∼:text=At some level%2C everyone is susceptible to phishing,outright trick you into performing a particular task (Accessed August 2, 2020).
CSI Onsite (2012). Phishing. Available at: http://csionsite.com/2012/phishing/ (Accessed May 8, 2019).
Cui, Q., Jourdan, G.-V., Bochmann, G. V., Couturier, R., and Onut, I.-V. (2017). Tracking phishing attacks over time. Proc. 26th Int. Conf. World Wide Web - WWW ’17 , Republic and Canton of Geneva, Switzerland: International World Wide Web Conferences Steering Committee . 667–676. doi:10.1145/3038912.3052654
CVEdetails (2005). Vulnerability in microsoft internet explorer. Available at: https://www.cvedetails.com/cve/CVE-2005-4089/ (Accessed August 20, 2019).
Cybint Cyber Solutions (2018). 13 alarming cyber security facts and stats. Available at: https://www.cybintsolutions.com/cyber-security-facts-stats/ (Accessed July 20, 2019).
Deshmukh, M., and raddha Popat, S. (2017). Different techniques for detection of phishing attack. Int. J. Eng. Sci. Comput. 7, 10201–10204. Available at: http://ijesc.org/ .
Dhamija, R., Tygar, J. D., and Hearst, M. (2006). “Why phishing works,” in Proceedings of the SIGCHI conference on human factors in computing systems - CHI ’06 , Montréal Québec, Canada , (New York, NY: ACM Press ), 581. doi:10.1145/1124772.1124861
Diaz, A., Sherman, A. T., and Joshi, A. (2020). Phishing in an academic community: a study of user susceptibility and behavior. Cryptologia 44, 53–67. doi:10.1080/01611194.2019.1623343
Dodge, R. C., Carver, C., and Ferguson, A. J. (2007). Phishing for user security awareness. Comput. Security 26, 73–80. doi:10.1016/j.cose.2006.10.009
eBay Toolbar and Account Guard (2009). Available at: https://download.cnet.com/eBay-Toolbar/3000-12512_4-10153544.html (Accessed August 7, 2020).
EDUCBA (2017). Hackers vs crackers: easy to understand exclusive difference. Available at: https://www.educba.com/hackers-vs-crackers/ (Accessed July 17, 2019).
Evers, J. (2006). Security expert: user education is pointless. Available at: https://www.cnet.com/news/security-expert-user-education-is-pointless/ (Accessed June 25, 2019).
F5Networks (2018). Panda malware broadens targets to cryptocurrency exchanges and social media. Available at: https://www.f5.com/labs/articles/threat-intelligence/panda-malware-broadens-targets-to-cryptocurrency-exchanges-and-social-media (Accessed April 23, 2019).
Fette, I., Sadeh, N., and Tomasic, A. (2007). “Learning to detect phishing emails,” in Proceedings of the 16th international conference on world wide web - WWW ’07 , Banff Alberta, Canada , (New York, NY: ACM Press) , 649–656. doi:10.1145/1242572.1242660
Financial Fraud Action UK (2017). Fraud the facts 2017: the definitive overview of payment industry fraud. London. Available at: https://www.financialfraudaction.org.uk/fraudfacts17/assets/fraud_the_facts.pdf .
Fraud Watch International (2019). Phishing attack trends for 2019. Available at: https://fraudwatchinternational.com/phishing/phishing-attack-trends-for-2019/ (Accessed October 29, 2019).
FTC (2018). Netflix scam email. Available at: https://www.ftc.gov/tips-advice/business-center/small-businesses/cybersecurity/phishing (Accessed May 8, 2019).
Furnell, S. (2007). An assessment of website password practices). Comput. Secur. 26, 445–451. doi:10.1016/j.cose.2007.09.001
Getsafeonline (2017). Caught on the net. Available at: https://www.getsafeonline.org/news/caught-on-the-net/%0D (Accessed August 1, 2020).
GOV.UK (2020). Cyber security breaches survey 2020. Available at: https://www.gov.uk/government/publications/cyber-security-breaches-survey-2020/cyber-security-breaches-survey-2020 (Accessed August 6, 2020).
Gupta, P., Srinivasan, B., Balasubramaniyan, V., and Ahamad, M. (2015). “Phoneypot: data-driven understanding of telephony threats,” in Proceedings 2015 network and distributed system security symposium , (Reston, VA: Internet Society ), 8–11. doi:10.14722/ndss.2015.23176
Hadlington, L. (2017). Human factors in cybersecurity; examining the link between internet addiction, impulsivity, attitudes towards cybersecurity, and risky cybersecurity behaviours. Heliyon 3, e00346-18. doi:10.1016/j.heliyon.2017.e00346
Herley, C., and Florêncio, D. (2008). “A profitless endeavor,” in New security paradigms workshop (NSPW ’08) , New Hampshire, United States , October 25–28, 2021 , 1–12. doi:10.1145/1595676.1595686
Hewage, C. (2020). Coronavirus pandemic has unleashed a wave of cyber attacks – here’s how to protect yourself. Conversat . Available at: https://theconversation.com/coronavirus-pandemic-has-unleashed-a-wave-of-cyber-attacks-heres-how-to-protect-yourself-135057 (Accessed November 16, 2020).
Hong, J. (2012). The state of phishing attacks. Commun. ACM 55, 74–81. doi:10.1145/2063176.2063197
Huber, M., Kowalski, S., Nohlberg, M., and Tjoa, S. (2009). “Towards automating social engineering using social networking sites,” in 2009 international conference on computational science and engineering , Vancouver, BC , August 29–31, 2009 ( IEEE , 117–124. doi:10.1109/CSE.2009.205
Hutchings, A., Clayton, R., and Anderson, R. (2016). “Taking down websites to prevent crime,” in 2016 APWG symposium on electronic crime research (eCrime) ( IEEE ), 1–10. doi:10.1109/ECRIME.2016.7487947
Iuga, C., Nurse, J. R. C., and Erola, A. (2016). Baiting the hook: factors impacting susceptibility to phishing attacks. Hum. Cent. Comput. Inf. Sci. 6, 8. doi:10.1186/s13673-016-0065-2
Jagatic, T. N., Johnson, N. A., Jakobsson, M., and Menczer, F. (2007). Social phishing. Commun. ACM 50, 94–100. doi:10.1145/1290958.1290968
Jakobsson, M., and Myers, S. (2006). Phishing and countermeasures: understanding the increasing problems of electronic identity theft . New Jersey: John Wiley and Sons .
Jakobsson, M., Tsow, A., Shah, A., Blevis, E., and Lim, Y. K. (2007). “What instills trust? A qualitative study of phishing,” in Lecture notes in computer science (including subseries lecture notes in artificial intelligence and lecture notes in bioinformatics) , (Berlin, Heidelberg: Springer ), 356–361. doi:10.1007/978-3-540-77366-5_32
Jeeva, S. C., and Rajsingh, E. B. (2016). Intelligent phishing url detection using association rule mining. Hum. Cent. Comput. Inf. Sci. 6, 10. doi:10.1186/s13673-016-0064-3
Johnson, A. (2016). Almost 600 accounts breached in “celebgate” nude photo hack, FBI says. Available at: http://www.cnbc.com/id/102747765 (Accessed: February 17, 2020).
Kayne, R. (2019). What are script kiddies? Wisegeek. Available at: https://www.wisegeek.com/what-are-script-kiddies.htm V V February 19, 2020).
Keck, C. (2018). FTC warns of sketchy Netflix phishing scam asking for payment details. Available at: https://gizmodo.com/ftc-warns-of-sketchy-netflix-phishing-scam-asking-for-p-1831372416 (Accessed April 23, 2019).
Keepnet LABS (2018). Statistical analysis of 126,000 phishing simulations carried out in 128 companies around the world. USA, France. Available at: www.keepnetlabs.com .
Keinan, G. (1987). Decision making under stress: scanning of alternatives under controllable and uncontrollable threats. J. Personal. Soc. Psychol. 52, 639–644. doi:10.1037/0022-3514.52.3.639
Khonji, M., Iraqi, Y., and Jones, A. (2013). Phishing detection: a literature survey. IEEE Commun. Surv. Tutorials 15, 2091–2121. doi:10.1109/SURV.2013.032213.00009
Kirda, E., and Kruegel, C. (2005). Protecting users against phishing attacks with AntiPhish. Proc. - Int. Comput. Softw. Appl. Conf. 1, 517–524. doi:10.1109/COMPSAC.2005.126
Krawchenko, K. (2016). The phishing email that hacked the account of John Podesta. CBSNEWS Available at: https://www.cbsnews.com/news/the-phishing-email-that-hacked-the-account-of-john-podesta/ (Accessed April 13, 2019).
Ksepersky (2020). Spam and phishing in Q1 2020. Available at: https://securelist.com/spam-and-phishing-in-q1-2020/97091/ (Accessed July 27, 2020).
Kumaraguru, P., Sheng, S., Acquisti, A., Cranor, L. F., and Hong, J. (2010). Teaching Johnny not to fall for phish. ACM Trans. Internet Technol. 10, 1–31. doi:10.1145/1754393.1754396
Latto, N. (2020). What is adware and how can you prevent it? Avast. Available at: https://www.avast.com/c-adware (Accessed May 8, 2020).
Le, D., Fu, X., and Hogrefe, D. (2006). A review of mobility support paradigms for the internet. IEEE Commun. Surv. Tutorials 8, 38–51. doi:10.1109/COMST.2006.323441
Lehman, T. J., and Vajpayee, S. (2011). “We’ve looked at clouds from both sides now,” in 2011 annual SRII global conference , San Jose, CA , March 20–April 2, 2011 , ( IEEE , 342–348. doi:10.1109/SRII.2011.46
Leyden, J. (2001). Virus toolkits are s’kiddie menace. Regist . Available at: https://www.theregister.co.uk/2001/02/21/virus_toolkits_are_skiddie_menace/%0D (Accessed June 15, 2019).
Lin, J., Sadeh, N., Amini, S., Lindqvist, J., Hong, J. I., and Zhang, J. (2012). “Expectation and purpose,” in Proceedings of the 2012 ACM conference on ubiquitous computing - UbiComp ’12 (New York, New York, USA: ACM Press ), 1625. doi:10.1145/2370216.2370290
Lininger, R., and Vines, D. R. (2005). Phishing: cutting the identity theft line. Print book . Indiana: Wiley Publishing, Inc .
Ma, J., Saul, L. K., Savage, S., and Voelker, G. M. (2009). “Identifying suspicious URLs.” in Proceedings of the 26th annual international conference on machine learning - ICML ’09 (New York, NY: ACM Press ), 1–8. doi:10.1145/1553374.1553462
Marforio, C., Masti, R. J., Soriente, C., Kostiainen, K., and Capkun, S. (2015). Personalized security indicators to detect application phishing attacks in mobile platforms. Available at: http://arxiv.org/abs/1502.06824 .
Margaret, R. I. P. (2008). PBX (private branch exchange). Available at: https://searchunifiedcommunications.techtarget.com/definition/IP-PBX (Accessed June 19, 2019).
Maurer, M.-E., and Herzner, D. (2012). Using visual website similarity for phishing detection and reporting. 1625–1630. doi:10.1145/2212776.2223683
Medvet, E., Kirda, E., and Kruegel, C. (2008). “Visual-similarity-based phishing detection,” in Proceedings of the 4th international conference on Security and privacy in communication netowrks - SecureComm ’08 (New York, NY: ACM Press ), 1. doi:10.1145/1460877.1460905
Merwe, A. v. d., Marianne, L., and Marek, D. (2005). “Characteristics and responsibilities involved in a Phishing attack, in WISICT ’05: proceedings of the 4th international symposium on information and communication technologies . Trinity College Dublin , 249–254.
Microsoft (2020). Exploiting a crisis: how cybercriminals behaved during the outbreak. Available at: https://www.microsoft.com/security/blog/2020/06/16/exploiting-a-crisis-how-cybercriminals-behaved-during-the-outbreak/ (Accessed August 1, 2020).
Mince-Didier, A. (2020). Hacking a computer or computer network. Available at: https://www.criminaldefenselawyer.com/resources/hacking-computer.html (Accessed August 7, 2020).
Miyamoto, D., Hazeyama, H., and Kadobayashi, Y. (2009). “An evaluation of machine learning-based methods for detection of phishing sites,” in international conference on neural information processing ICONIP 2008: advances in neuro-information processing lecture notes in computer science . Editors M. Köppen, N. Kasabov, and G. Coghill (Berlin, Heidelberg: Springer Berlin Heidelberg ), 539–546. doi:10.1007/978-3-642-02490-0_66
Mohammad, R. M., Thabtah, F., and McCluskey, L. (2014). Predicting phishing websites based on self-structuring neural network. Neural Comput. Applic 25, 443–458. doi:10.1007/s00521-013-1490-z
Moore, T., and Clayton, R. (2007). “Examining the impact of website take-down on phishing,” in Proceedings of the anti-phishing working groups 2nd annual eCrime researchers summit on - eCrime ’07 (New York, NY: ACM Press ), 1–13. doi:10.1145/1299015.1299016
Morgan, S. (2019). 2019 official annual cybercrime report. USA, UK, Canada. Available at: https://www.herjavecgroup.com/wp-content/uploads/2018/12/CV-HG-2019-Official-Annual-Cybercrime-Report.pdf .
Nathan, G. (2020). What is phishing? + laws, charges & statute of limitations. Available at: https://www.federalcharges.com/phishing-laws-charges/ (Accessed August 7, 2020).
Okin, S. (2009). From script kiddies to organised cybercrime. Available at: https://comsecglobal.com/from-script-kiddies-to-organised-cybercrime-things-are-getting-nasty-out-there/ (Accessed August 12, 2019).
Ollmann, G. (2004). The phishing guide understanding & preventing phishing attacks abstract. USA. Available at: http://www.ngsconsulting.com .
Ong, S. (2014). Avast survey shows men more susceptible to mobile malware. Available at: https://www.mirekusoft.com/avast-survey-shows-men-more-susceptible-to-mobile-malware/ (Accessed November 5, 2020).
Ovelgönne, M., Dumitraş, T., Prakash, B. A., Subrahmanian, V. S., and Wang, B. (2017). Understanding the relationship between human behavior and susceptibility to cyber attacks. ACM Trans. Intell. Syst. Technol. 8, 1–25. doi:10.1080/00207284.1985.11491413
Parmar, B. (2012). Protecting against spear-phishing. Computer Fraud Security , 2012, 8–11. doi:10.1016/S1361-3723(12)70007-6
Phish Labs (2019). 2019 phishing trends and intelligence report the growing social engineering threat. Available at: https://info.phishlabs.com/hubfs/2019 PTI Report/2019 Phishing Trends and Intelligence Report.pdf .
PhishMe (2016). Q1 2016 malware review. Available at: WWW.PHISHME.COM .
PhishMe (2017). Human phishing defense enterprise phishing resiliency and defense report 2017 analysis of susceptibility, resiliency and defense against simulated and real phishing attacks. Available at: https://cofense.com/wp-content/uploads/2017/11/Enterprise-Phishing-Resiliency-and-Defense-Report-2017.pdf .
PishTank (2006). What is phishing. Available at: http://www.phishtank.com/what_is_phishing.php?view=website&annotated=true (Accessed June 19, 2019).
Pompon, A. R., Walkowski, D., and Boddy, S. (2018). Phishing and Fraud Report attacks peak during the holidays. US .
Proofpoint (2019a). State of the phish 2019 report. Sport Mark. Q. 14, 4. doi:10.1038/sj.jp.7211019
Proofpoint (2019b). What is Proofpoint. Available at: https://www.proofpoint.com/us/company/about (Accessed September 25, 2019).
Proofpoint (2020). 2020 state of the phish. Available at: https://www.proofpoint.com/sites/default/files/gtd-pfpt-us-tr-state-of-the-phish-2020.pdf .
Raggo, M. (2016). Anatomy of a social media attack. Available at: https://www.darkreading.com/analytics/anatomy-of-a-social-media-attack/a/d-id/1326680 (Accessed March 14, 2019).
Ramanathan, V., and Wechsler, H. (2012). PhishGILLNET-phishing detection methodology using probabilistic latent semantic analysis, AdaBoost, and co-training. EURASIP J. Info. Secur. 2012, 1–22. doi:10.1186/1687-417X-2012-1
Ramzan, Z. (2010). “Phishing attacks and countermeasures,” in Handbook of Information and communication security (Berlin, Heidelberg: Springer Berlin Heidelberg ), 433–448. doi:10.1007/978-3-642-04117-4_23
Ramzan, Z., and Wuest, C. (2007). “Phishing Attacks: analyzing trends in 2006,” in Fourth conference on email and anti-Spam (Mountain View , ( California, United States ).
Rhett, J. (2019). Don’t fall for this new Google translate phishing attack. Available at: https://www.gizmodo.co.uk/2019/02/dont-fall-for-this-new-google-translate-phishing-attack/ (Accessed April 23, 2019). doi:10.5040/9781350073272
RISKIQ (2020). Investigate | COVID-19 cybercrime weekly update. Available at: https://www.riskiq.com/blog/analyst/covid19-cybercrime-update/%0D (Accessed August 1, 2020).
Robichaux, P., and Ganger, D. L. (2006). Gone phishing: evaluating anti-phishing tools for windows. Available at: http://www.3sharp.com/projects/antiphishing/gonephishing.pdf .
Rouse, M. (2013). Phishing defintion. Available at: https://searchsecurity.techtarget.com/definition/phishing (Accessed April 10, 2019).
Salem, O., Hossain, A., and Kamala, M. (2010). “Awareness program and AI based tool to reduce risk of phishing attacks,” in 2010 10th IEEE international conference on computer and information technology (IEEE) , Bradford, United Kingdom , June 29–July 1, 2010, 2001 ( IEEE ), 1418–1423. doi:10.1109/CIT.2010.254
Scaife, N., Carter, H., Traynor, P., and Butler, K. R. B. (2016). “Crypto lock (and drop it): stopping ransomware attacks on user data,” in 2016 IEEE 36th international conference on distributed computing systems (ICDCS) ( IEEE , 303–312. doi:10.1109/ICDCS.2016.46
Sheng, S., Magnien, B., Kumaraguru, P., Acquisti, A., Cranor, L. F., Hong, J., et al. (2007). “Anti-Phishing Phil: the design and evaluation of a game that teaches people not to fall for phish,” in Proceedings of the 3rd symposium on usable privacy and security - SOUPS ’07 (New York, NY: ACM Press ), 88–99. doi:10.1145/1280680.1280692
Symantic, (2019). Internet security threat report volume 24|February 2019 . USA.
Techpedia (2021). Caller ID. Available at: https://www.techopedia.com/definition/24222/caller-id (Accessed June 19, 2019).
VadeSecure (2021). Phishers favorites 2019. Available at: https://www.vadesecure.com/en/ (Accessed October 29, 2019).
Vishwanath, A. (2005). “Spear phishing: the tip of the spear used by cyber terrorists,” in deconstruction machines (United States: University of Minnesota Press ), 469–484. doi:10.4018/978-1-5225-0156-5.ch023
Wang, X., Zhang, R., Yang, X., Jiang, X., and Wijesekera, D. (2008). “Voice pharming attack and the trust of VoIP,” in Proceedings of the 4th international conference on security and privacy in communication networks, SecureComm’08 , 1–11. doi:10.1145/1460877.1460908
Wenyin, L., Huang, G., Xiaoyue, L., Min, Z., and Deng, X. (2005). “Detection of phishing webpages based on visual similarity,” in 14th international world wide web conference, WWW2005 , Chiba, Japan , May 10–14, 2005 , 1060–1061. doi:10.1145/1062745.1062868
Whitman, M. E., and Mattord, H. J. (2012). Principles of information security. Course Technol. 1–617. doi:10.1016/B978-0-12-381972-7.00002-6
Williams, E. J., Hinds, J., and Joinson, A. N. (2018). Exploring susceptibility to phishing in the workplace. Int. J. Human-Computer Stud. 120, 1–13. doi:10.1016/j.ijhcs.2018.06.004
wombatsecurity.com (2018). Wombat security user risk report. USA. Available at: https://info.wombatsecurity.com/hubfs/WombatProofpoint-UserRiskSurveyReport2018_US.pdf .
Workman, M. (2008). Wisecrackers: a theory-grounded investigation of phishing and pretext social engineering threats to information security. J. Am. Soc. Inf. Sci. 59 (4), 662–674. doi:10.1002/asi.20779
Yeboah-Boateng, E. O., and Amanor, P. M. (2014). Phishing , SMiShing & vishing: an assessment of threats against mobile devices. J. Emerg. Trends Comput. Inf. Sci. 5 (4), 297–307.
Zhang, Y., Hong, J. I., and Cranor, L. F. (2007). “Cantina,” in Proceedings of the 16th international conference on World Wide Web - WWW ’07 (New York, NY: ACM Press ), 639. doi:10.1145/1242572.1242659
Zissis, D., and Lekkas, D. (2012). Addressing cloud computing security issues. Future Generat. Comput. Syst. 28, 583–592. doi:10.1016/j.future.2010.12.006
Keywords: phishing anatomy, precautionary countermeasures, phishing targets, phishing attack mediums, phishing attacks, attack phases, phishing techniques
Citation: Alkhalil Z, Hewage C, Nawaf L and Khan I (2021) Phishing Attacks: A Recent Comprehensive Study and a New Anatomy. Front. Comput. Sci. 3:563060. doi: 10.3389/fcomp.2021.563060
Received: 17 May 2020; Accepted: 18 January 2021; Published: 09 March 2021.
Reviewed by:
Copyright © 2021 Alkhalil, Hewage, Nawaf and Khan. This is an open-access article distributed under the terms of the Creative Commons Attribution License (CC BY). The use, distribution or reproduction in other forums is permitted, provided the original author(s) and the copyright owner(s) are credited and that the original publication in this journal is cited, in accordance with accepted academic practice. No use, distribution or reproduction is permitted which does not comply with these terms.
*Correspondence: Chaminda Hewage, [email protected]
This article is part of the Research Topic
2021 Editor's Pick: Computer Science

- Advanced Search
Human Factors in Phishing Attacks: A Systematic Literature Review

Universitá di Bari Aldo Moro
Sapienza Universitá di Roma

- 18 citation
New Citation Alert added!
This alert has been successfully added and will be sent to:
You will be notified whenever a record that you have chosen has been cited.
To manage your alert preferences, click on the button below.
New Citation Alert!
Please log in to your account
- Publisher Site
ACM Computing Surveys

Phishing is the fraudulent attempt to obtain sensitive information by disguising oneself as a trustworthy entity in digital communication. It is a type of cyber attack often successful because users are not aware of their vulnerabilities or are unable to understand the risks. This article presents a systematic literature review conducted to draw a “big picture” of the most important research works performed on human factors and phishing. The analysis of the retrieved publications, framed along the research questions addressed in the systematic literature review, helps in understanding how human factors should be considered to defend against phishing attacks. Future research directions are also highlighted.
Index Terms
Human-centered computing
Human computer interaction (HCI)
Security and privacy
Human and societal aspects of security and privacy
Intrusion/anomaly detection and malware mitigation
Social engineering attacks
Recommendations
Mitigating phishing attacks: an overview.
Social engineering is the process of getting a person to provide a service or complete a task that may give away private or confidential information. Phishing is the most common type of social engineering. In phishing, an attacker poses as a trustworthy ...
Defending against phishing attacks: taxonomy of methods, current issues and future directions
Internet technology is so pervasive today, for example, from online social networking to online banking, it has made people's lives more comfortable. Due the growth of Internet technology, security threats to systems and networks are relentlessly ...
Fighting against phishing attacks: state of the art and future challenges
In the last few years, phishing scams have rapidly grown posing huge threat to global Internet security. Today, phishing attack is one of the most common and serious threats over Internet where cyber attackers try to steal user's personal or financial ...
Login options
Check if you have access through your login credentials or your institution to get full access on this article.
Full Access
- Information
- Contributors
Published in

University of Sydney, Australia
Copyright © 2021 Association for Computing Machinery.
Permission to make digital or hard copies of all or part of this work for personal or classroom use is granted without fee provided that copies are not made or distributed for profit or commercial advantage and that copies bear this notice and the full citation on the first page. Copyrights for components of this work owned by others than ACM must be honored. Abstracting with credit is permitted. To copy otherwise, or republish, to post on servers or to redistribute to lists, requires prior specific permission and/or a fee. Request permissions from [email protected] .
In-Cooperation
Association for Computing Machinery
New York, NY, United States
Publication History
- Published: 4 October 2021
- Accepted: 1 June 2021
- Revised: 1 May 2021
- Received: 1 July 2020
Permissions
Request permissions about this article.
Check for updates
Author tags.
- human factors
- cybersecurity
Funding Sources
Other metrics.
- Bibliometrics
- Citations 18
Article Metrics
- 18 Total Citations View Citations
- 3,415 Total Downloads
- Downloads (Last 12 months) 1,175
- Downloads (Last 6 weeks) 162
View or Download as a PDF file.
View online with eReader.
Digital Edition
View this article in digital edition.
HTML Format
View this article in HTML Format .
Share this Publication link
https://dl.acm.org/doi/10.1145/3469886
Share on Social Media
- 0 References
Export Citations
- Please download or close your previous search result export first before starting a new bulk export. Preview is not available. By clicking download, a status dialog will open to start the export process. The process may take a few minutes but once it finishes a file will be downloadable from your browser. You may continue to browse the DL while the export process is in progress. Download
- Download citation
- Copy citation
We are preparing your search results for download ...
We will inform you here when the file is ready.
Your file of search results citations is now ready.
Your search export query has expired. Please try again.
Information
- Author Services
Initiatives
You are accessing a machine-readable page. In order to be human-readable, please install an RSS reader.
All articles published by MDPI are made immediately available worldwide under an open access license. No special permission is required to reuse all or part of the article published by MDPI, including figures and tables. For articles published under an open access Creative Common CC BY license, any part of the article may be reused without permission provided that the original article is clearly cited. For more information, please refer to https://www.mdpi.com/openaccess .
Feature papers represent the most advanced research with significant potential for high impact in the field. A Feature Paper should be a substantial original Article that involves several techniques or approaches, provides an outlook for future research directions and describes possible research applications.
Feature papers are submitted upon individual invitation or recommendation by the scientific editors and must receive positive feedback from the reviewers.
Editor’s Choice articles are based on recommendations by the scientific editors of MDPI journals from around the world. Editors select a small number of articles recently published in the journal that they believe will be particularly interesting to readers, or important in the respective research area. The aim is to provide a snapshot of some of the most exciting work published in the various research areas of the journal.
Original Submission Date Received: .
- Active Journals
- Find a Journal
- Proceedings Series
- For Authors
- For Reviewers
- For Editors
- For Librarians
- For Publishers
- For Societies
- For Conference Organizers
- Open Access Policy
- Institutional Open Access Program
- Special Issues Guidelines
- Editorial Process
- Research and Publication Ethics
- Article Processing Charges
- Testimonials
- Preprints.org
- SciProfiles
- Encyclopedia
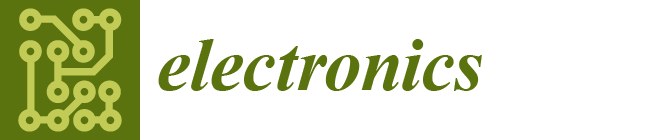
Article Menu
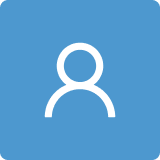
- Subscribe SciFeed
- Google Scholar
- on Google Scholar
- Table of Contents
Find support for a specific problem in the support section of our website.
Please let us know what you think of our products and services.
Visit our dedicated information section to learn more about MDPI.
JSmol Viewer
A systematic review on deep-learning-based phishing email detection.
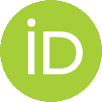
1. Introduction
1.1. our contribution, 1.2. organization of the document, 2. methodology, 2.1. research question and search strategy, 2.2. study selection, 2.3. data extraction and analysis, 2.4. quality assessment, 2.5. inclusion and exclusion criteria, 2.5.1. inclusion criteria.
- The paper must contain empirical results on deep-learning-based phishing detection.
- The paper must be published in the English language.
2.5.2. Exclusion Criteria
- The paper is not available in full-text format.
- The paper is not related to the research question.
- The paper is a duplicate publication. The paper is a review article or a meta-analysis.
- The paper is a conference abstract or poster presentation.
- The paper is a book, book chapter, or thesis.
- The paper is of low quality, as determined by the QATQS.
3. Literature Survey and Findings
3.1. research papers published in 2017 and before, 3.2. research papers published in 2018, 3.3. research papers published in 2019, 3.4. research papers published in 2020, 3.5. research papers published in 2021, 3.6. research papers published in 2022, 3.7. research papers published in 2023, 4. results and analysis, 4.1. findings of data analysis, 4.2. limitations found, 4.3. future direction, 4.3.1. privacy preservation, 4.3.2. increasing dataset size and optimizing feature selection, 4.3.3. broader email content analysis, 4.3.4. handling modern phishing techniques, 4.3.5. handling concept drift, 4.3.6. consideration of additional factors, 4.3.7. comparison with state-of-the-art techniques, 4.3.8. hyperparameter optimization and more deep learning architectures, 4.3.9. real-time dataset and processing, 4.3.10. exploration of other machine learning techniques, 4.3.11. incorporating additional data sources, 4.3.12. enriching the dataset, 4.3.13. exploring attackers’ behavior and modus operandi, 4.3.14. testing on other domains, 5. conclusions, author contributions, data availability statement, conflicts of interest.
- Alshingiti, Z.; Alaqel, R.; Al-Muhtadi, J.; Haq, Q.E.U.; Saleem, K.; Faheem, M.H. A Deep Learning-Based Phishing Detection System Using CNN, LSTM, and LSTM-CNN. Electronics 2023 , 12 , 232. [ Google Scholar ] [ CrossRef ]
- Tsohou, A.; Diamantopoulou, V.; Gritzalis, S.; Lambrinoudakis, C. Cyber insurance: State of the art, trends and future directions. Int. J. Inf. Secur. 2023 , 22 , 737–748. [ Google Scholar ] [ CrossRef ]
- Sheng, S.; Wardman, B.; Warner, G.; Cranor, L.; Hong, J.; Zhang, C. An Empirical Analysis of Phishing Blacklists. In Proceedings of the Sixth Conference on Email and Anti-Spam, Mountain View, CA, USA, 16–17 July 2009. [ Google Scholar ]
- Edge, M.E.; Sampaio, P.R.F. A survey of signature based methods for financial fraud detection. Comput. Secur. 2009 , 28 , 381–394. [ Google Scholar ] [ CrossRef ]
- Safi, A.; Singh, S. A systematic literature review on phishing website detection techniques. J. King Saud Univ. Comput. Inf. Sci. 2023 , 35 , 590–611. [ Google Scholar ] [ CrossRef ]
- Aldawood, H.; Skinner, G. An Advanced Taxonomy for Social Engineering Attacks. Int. J. Comput. Appl. 2020 , 177 , 1–11. [ Google Scholar ] [ CrossRef ]
- Aleroud, A.; Zhou, L. Phishing environments, techniques, and countermeasures: A survey. Comput. Secur. 2017 , 68 , 160–196. [ Google Scholar ] [ CrossRef ]
- Kocher, G.; Kumar, G. Machine learning and deep learning methods for intrusion detection systems: Recent developments and challenges. Soft Comput. 2021 , 25 , 9731–9763. [ Google Scholar ] [ CrossRef ]
- Chen, D.; Wawrzynski, P.; Lv, Z. Cyber security in smart cities: A review of deep learning-based applications and case studies. Sustain. Cities Soc. 2021 , 66 , 102655. [ Google Scholar ] [ CrossRef ]
- Adebowale, M.A.; Lwin, K.T.; Hossain, M.A. Deep learning with convolutional neural network and long short-term memory for phishing detection. In Proceedings of the 2019 13th International Conference on Software, Knowledge, Information Management and Applications (SKIMA), Island of Ulkulhas, Maldives, 26–28 August 2019; pp. 1–8. [ Google Scholar ]
- Thomas, B.; Ciliska, D.; Dobbins, M.; Micucci, S. A Process for Systematically Reviewing the Literature: Providing the Research Evidence for Public Health Nursing Interventions. Worldviews Evid.-Based Nurs. 2004 , 1 , 176–184. [ Google Scholar ] [ CrossRef ]
- Nosseir, A.; Nagati, K.; Taj-Eddin, I. Intelligent word-based spam filter detection using multi-neural networks. Int. J. Comput. Sci. Issues (IJCSI) 2013 , 10 Pt 1 , 17. [ Google Scholar ]
- Almomani, A.; Gupta, B.B.; Wan, T.C.; Altaher, A.; Manickam, S. Phishing dynamic evolving neural fuzzy framework for online detection zero-day phishing email. Indian J. Sci. Technol. 2013 , 6 , 3960–3964. [ Google Scholar ] [ CrossRef ]
- Hamid, I.R.A.; Abawajy, J.; Kim, T.H. Using feature selection and classification scheme for automating phishing email detection. Stud. Inform. Control. 2013 , 22 , 61–70. [ Google Scholar ] [ CrossRef ]
- Jameel, N.G.M.; George, L.E. Detection of phishing emails using feed forward neural network. Int. J. Comput. Appl. 2013 , 77 , 10–15. [ Google Scholar ]
- Soni, A.N. Spam-e-mail-detection-using-advanced-deep-convolution-neuralnetwork-algorithms. J. Innov. Dev. Pharm. Tech. Sci. 2019 , 2 , 74–80. [ Google Scholar ]
- Zhang, N.; Yuan, Y. Phishing Detection Using Neural Network. Available online: http://cs229.stanford.edu/proj2012/ZhangYuan-PhishingDetectionUsingNeuralNetwork.pdf (accessed on 1 October 2023).
- Kufandirimbwa, O.; Gotora, R. Spam detection using artificial neural networks (perceptron learning rule). Online J. Phys. Environ. Sci. Res. 2012 , 1 , 22–29. [ Google Scholar ]
- Abu-Nimeh, S.; Nappa, D.; Wang, X.; Nair, S. A comparison of machine learning techniques for phishing detection. In Proceedings of the Anti-Phishing Working Groups 2nd Annual eCrime Researchers Summit, Pittsburgh, PA, USA, 4–5 October 2007; pp. 60–69. [ Google Scholar ]
- Chandan, C.J.; Chheda, H.P.; Gosar, D.M.; Shah, H.R.; Bhave, P.U. A Machine learning approach for detection of phished websites using neural networks. Int. J. Recent Innov. Trends Comput. Commun. 2014 , 2 , 42054209. [ Google Scholar ]
- Alkaht, I.J.; Al Khatib, B. Filtering SPAM Using Several Stages Neural Networks. Int. Rev. Comput. Softw. (IRECOS) 2016 , 11 , 123–132. [ Google Scholar ] [ CrossRef ]
- Coyotes, C.; Mohan, V.S.; Naveen, J.; Vinayakumar, R.; Soman, K.P.; Verma, A.D.R. ARES: Automatic rogue email spotter. In Proceedings of the 1st AntiPhishing Shared Pilot at 4th ACM International Workshop on Security and Privacy Analytics (IWSPA), Tempe, AZ, USA, 1–11 March 2018. [ Google Scholar ]
- Smadi, S.; Aslam, N.; Zhang, L. Detection of online phishing email using dynamic evolving neural network based on reinforcement learning. Decis. Support Syst. 2018 , 107 , 88–102. [ Google Scholar ] [ CrossRef ]
- Hiransha, M.; Unnithan, N.A.; Vinayakumar, R.; Soman, K.; Verma, A.D.R. Deep learning based phishing e-mail detection. In Proceedings of the 1st AntiPhishing Shared Pilot at 4th ACM International Workshop Security Privacy Analytics (IWSPA), Tempe, AZ, USA, 1–11 March 2018; pp. 1–5. [ Google Scholar ]
- Barushka, A.; Hajek, P. Spam filtering using integrated distribution-based balancing approach and regularized deep neural networks. Appl. Intell. 2018 , 48 , 3538–3556. [ Google Scholar ] [ CrossRef ]
- Fang, Y.; Zhang, C.; Huang, C.; Liu, L.; Yang, Y. Phishing Email Detection Using Improved RCNN Model With Multilevel Vectors and Attention Mechanism. IEEE Access 2019 , 7 , 56329–56340. [ Google Scholar ] [ CrossRef ]
- Harikrishnan, N.B.; Vinayakumar, R.; Soman, K.P.; Poornachandran, P. Time split based pre-processing with a data-driven approach for malicious url detection. Cybersecur. Secur. Inf. Syst. Chall. Solut. Smart Environ. 2019 , 43–65. [ Google Scholar ] [ CrossRef ]
- Ali, W.; Ahmed, A.A. Hybrid intelligent phishing website prediction using deep neural networks with genetic algorithm-based feature selection and weighting. IET Inf. Secur. 2019 , 13 , 659–669. [ Google Scholar ] [ CrossRef ]
- Oña, D.; Zapata, L.; Fuertes, W.; Rodríguez, G.; Benavides, E.; Toulkeridis, T. Phishing attacks: Detecting and preventing infected e-mails using machine learning methods. In Proceedings of the 2019 3rd Cyber Security in Networking Conference (CSNet), IEEE, Quito, Ecuador, 23–25 October 2019; pp. 161–163. [ Google Scholar ]
- Nguyen, M.; Nguyen, T.; Nguyen, T.H. A deep learning model with hierarchical lstms and supervised attention for anti-phishing. CEUR Workshop Proc. 2018 , 2124 , 29–38. [ Google Scholar ]
- Wei, B.; Hamad, R.A.; Yang, L.; He, X.; Wang, H.; Gao, B.; Woo, W.L. A deep-learning-driven light-weight phishing detection sensor. Sensors 2019 , 19 , 4258. [ Google Scholar ] [ CrossRef ] [ PubMed ]
- Vinayakumar, R.; Soman, K.P.; Poornachandran, P.; Akarsh, S.; Elhoseny, M. Deep learning framework for cyber threat situational awareness based on email and url data analysis. In Cybersecurity and Secure Information Systems: Challenges and Solutions in Smart Environments ; Springer: Berlin/Heidelberg, Germany, 2019; pp. 87–124. [ Google Scholar ]
- Yang, P.; Zhao, G.; Zeng, P. Phishing Website Detection Based on Multidimensional Features Driven by Deep Learning. IEEE Access 2019 , 7 , 15196–15209. [ Google Scholar ] [ CrossRef ]
- Saha, I.; Sarma, D.; Chakma, R.J.; Alam, M.N.; Sultana, A.; Hossain, S. Phishing attacks detection using deep learning approach. In Proceedings of the 2020 Third International Conference on Smart Systems and Inventive Technology (ICSSIT), IEEE, Tirunelveli, India, 20–22 August 2020; pp. 1180–1185. [ Google Scholar ]
- Thapa, C.; Tang, J.W.; Abuadbba, A.; Gao, Y.; Camtepe, S.; Nepal, S.; Almashor, M.; Zheng, Y. Evaluation of Federated Learning in Phishing Email Detection. Sensors 2023 , 23 , 4346. [ Google Scholar ] [ CrossRef ] [ PubMed ]
- Adebowale, M.A.; Lwin, K.T.; Hossain, M.A. Intelligent phishing detection scheme using deep learning algorithms. J. Enterp. Inf. Manag. 2020 , 36 , 747–766. [ Google Scholar ] [ CrossRef ]
- Alotaibi, R.; Al-Turaiki, I.; Alakeel, F. Mitigating email phishing attacks using convolutional neural networks. In Proceedings of the 2020 3rd International Conference on Computer Applications & Information Security (ICCAIS), IEEE, Riyadh, Saudi Arabia, 19–21 March 2020; pp. 1–6. [ Google Scholar ]
- Baccouche, A.; Ahmed, S.; Sierra-Sosa, D.; Elmaghraby, A. Malicious text identification: Deep learning from public comments and emails. Information 2020 , 11 , 312. [ Google Scholar ] [ CrossRef ]
- Soon, G.K.; On, C.K.; Rusli, N.M.; Fun, T.S.; Alfred, R.; Guan, T.T. March. Comparison of simple feedforward neural network, recurrent neural network and ensemble neural networks in phishing detection. J. Phys. Conf. Ser. 2020 , 1502 , 012033. [ Google Scholar ] [ CrossRef ]
- Alauthman, M. Botnet Spam E-Mail Detection Using Deep Recurrent Neural Network. Int. J. Emerg. Trends Eng. Res. 2020 , 8 , 1979–1986. [ Google Scholar ] [ CrossRef ]
- Eryılmaz, E.E.; Şahin, D.Ö.; Kılıç, E. Filtering turkish spam using LSTM from deep learning techniques. In Proceedings of the 2020 8th International Symposium on Digital Forensics and Security, ISDFS, IEEE, Beirut, Lebanon, 1–2 June 2020; pp. 1–6. [ Google Scholar ]
- Halgaš, L.; Agrafiotis, I.; Nurse, J.R. Catching the Phish: Detecting phishing attacks using recurrent neural networks (RNNs). In Proceedings of the Information Security Applications: 20th International Conference, WISA 2019, Jeju Island, Republic of Korea, 21–24 August 2019; pp. 219–233. [ Google Scholar ]
- Isik, S.; Kurt, Z.; Anagun, Y.; Ozkan, K. Spam E-mail Classification Recurrent Neural Networks for Spam E-mail Classification on an Agglutinative Language. Int. J. Intell. Syst. Appl. Eng. 2020 , 8 , 221–227. [ Google Scholar ] [ CrossRef ]
- AlEroud, A.; Karabatis, G. Bypassing detection of URL-based phishing attacks using generative adversarial deep neural networks. In Proceedings of the Sixth International Workshop on Security and Privacy Analytics, New Orleans, LA, USA, 18 March 2020; pp. 53–60. [ Google Scholar ]
- Castillo, E.; Dhaduvai, S.; Liu, P.; Thakur, K.S.; Dalton, A.; Strzalkowski, T. Email threat detection using distinct neural network approaches. In Proceedings of the First International Workshop on Social Threats in Online Conversations: Understanding and Management, Marseille, France, 11–16 May 2020; pp. 48–55. [ Google Scholar ]
- Kumar, A.; Chatterjee, J.M.; Díaz, V.G. A novel hybrid approach of SVM combined with NLP and probabilistic neural network for email phishing. Int. J. Electr. Comput. Eng. (IJECE) 2020 , 10 , 486–493. [ Google Scholar ] [ CrossRef ]
- Opara, C.; Wei, B.; Chen, Y. HTMLPhish: Enabling phishing web page detection by applying deep learning techniques on HTML analysis. In Proceedings of the 2020 International Joint Conference on Neural Networks (IJCNN), Glasgow, UK, 19–24 July 2020; pp. 1–8. [ Google Scholar ]
- AbdulNabi, I.; Yaseen, Q. Spam Email Detection Using Deep Learning Techniques. Procedia Comput. Sci. 2021 , 184 , 853–858. [ Google Scholar ] [ CrossRef ]
- Otter, D.W.; Medina, J.R.; Kalita, J.K. A Survey of the Usages of Deep Learning for Natural Language Processing. IEEE Trans. Neural Networks Learn. Syst. 2020 , 32 , 604–624. [ Google Scholar ] [ CrossRef ]
- Alhogail, A.; Alsabih, A. Applying machine learning and natural language processing to detect phishing email. Comput. Secur. 2021 , 110 , 102414. [ Google Scholar ] [ CrossRef ]
- Bagui, S.; Nandi, D.; Bagui, S.; White, R.J. Machine learning and deep learning for phishing email classification using one-hot encoding. J. Comput. Sci. 2021 , 17 , 610–623. [ Google Scholar ] [ CrossRef ]
- Lee, J.; Tang, F.; Ye, P.; Abbasi, F.; Hay, P.; Divakaran, D.M. D-Fence: A flexible, efficient, and comprehensive phishing email detection system. In Proceedings of the 2021 IEEE European Symposium on Security and Privacy (EuroS&P), IEEE, Vienna, Austria, 7–11 September 2021; pp. 578–597. [ Google Scholar ]
- Manaswini, M.; Srinivasu, D.N. Phishing Email Detection Model using Improved Recurrent Convolutional Neural Networks and Multilevel Vectors. Ann. Rom. Soc. Cell Biol. 2021 , 25 , 16674–16681. [ Google Scholar ]
- Ghaleb, S.A.A.; Mohamad, M.; Fadzli, S.A.; Ghanem, W.A.H.M. Training Neural Networks by Enhance Grasshopper Optimization Algorithm for Spam Detection System. IEEE Access 2021 , 9 , 116768–116813. [ Google Scholar ] [ CrossRef ]
- Eckhardt, R.; Bagui, S. Convolutional Neural Networks and Long Short Term Memory for Phishing Email Classification. Int. J. Comput. Sci. Inf. Secur. 2021 , 19 , 27–35. [ Google Scholar ]
- Sheneamer, A. Comparison of Deep and Traditional Learning Methods for Email Spam Filtering. Int. J. Adv. Comput. Sci. Appl. 2021 , 12 , 560–565. [ Google Scholar ] [ CrossRef ]
- Dubey, K.A.; Ganesh, K.B.; Gowtham, V.; Balakrishnan, M.D. Phishing email detection. Int. J. Emerg. Technol. Comput. Sci. Electron. (IJETCSE) 2021 , 28 , 1–4. [ Google Scholar ]
- Samarthrao, K.V.; Rohokale, V.M. Enhancement of email spam detection using improved deep learning algorithms for cyber security. J. Comput. Secur. 2022 , 30 , 231–264. [ Google Scholar ] [ CrossRef ]
- Dewis, M.; Viana, T. Phish Responder: A Hybrid Machine Learning Approach to Detect Phishing and Spam Emails. Appl. Syst. Innov. 2022 , 5 , 73. [ Google Scholar ] [ CrossRef ]
- Khan, S.A.; Iqbal, K.; Mohammad, N.; Akbar, R.; Ali, S.S.A.; Siddiqui, A.A. A Novel Fuzzy-Logic-Based Multi-Criteria Metric for Performance Evaluation of Spam Email Detection Algorithms. Appl. Sci. 2022 , 12 , 7043. [ Google Scholar ] [ CrossRef ]
- Malhotra, P.; Malik, S. Spam Email Detection Using Machine Learning and Deep Learning Techniques. In Proceedings of the International Conference on Innovative Computing & Communication (ICICC), Delhi, India, 24 June 2022. [ Google Scholar ] [ CrossRef ]
- Korkmaz, M.; Koçyiğit, E.; Şahingöz, Ö.; Diri, B. A Hybrid Phishing Detection System by Using Deep Learning-Based URL and Content Analysis. Elektron. Ir Elektrotechnika 2022 , 28 , 80–89. [ Google Scholar ] [ CrossRef ]
- Zhu, E.; Yuan, Q.; Chen, Z.; Li, X.; Fang, X. CCBLA: A Lightweight Phishing Detection Model Based on CNN, BiLSTM, and Attention Mechanism. Cogn. Comput. 2022 , 15 , 1320–1333. [ Google Scholar ] [ CrossRef ]
- Nooraee, M.; Ghaffari, H. Optimization and Improvement of Spam Email Detection Using Deep Learning Approaches. J. Comput. Robot. 2022 , 15 , 61–70. [ Google Scholar ]
- Prosun, P.R.K.; Alam, K.S.; Bhowmik, S. Improved Spam Email Filtering Architecture Using Several Feature Extraction Techniques. In Proceedings of the International Conference on Big Data, IoT, and Machine Learning: BIM 2021, Cox’s Bazar, Bangladesh, 23–25 September 2021; Springer: Singapore, 2021; pp. 665–675. [ Google Scholar ]
- Jafar, M.T.; Al-Fawa’reh, M.; Barhoush, M.; Alshira’H, M.H. Enhanced Analysis Approach to Detect Phishing Attacks During COVID-19 Crisis. Cybern. Inf. Technol. 2022 , 22 , 60–76. [ Google Scholar ] [ CrossRef ]
- Do, N.Q.; Selamat, A.; Krejcar, O.; Herrera-Viedma, E.; Fujita, H. Deep Learning for Phishing Detection: Taxonomy, Current Challenges and Future Directions. IEEE Access 2022 , 10 , 36429–36463. [ Google Scholar ] [ CrossRef ]
- Zhou, M.-G.; Liu, Z.-P.; Yin, H.-L.; Li, C.-L.; Xu, T.-K.; Chen, Z.-B. Quantum Neural Network for Quantum Neural Computing. Research 2023 , 6 , 0134. [ Google Scholar ] [ CrossRef ] [ PubMed ]
- Rafat, K.F.; Xin, Q.; Javed, A.R.; Jalil, Z.; Ahmad, R.Z. Evading obscure communication from spam emails. Math. Biosci. Eng. 2021 , 19 , 1926–1943. [ Google Scholar ] [ CrossRef ] [ PubMed ]
- Rathee, D.; Mann, S. Detection of E-Mail Phishing Attacks – using Machine Learning and Deep Learning. Int. J. Comput. Appl. 2022 , 183 , 1–7. [ Google Scholar ] [ CrossRef ]
- Mughaid, A.; AlZu’bi, S.; Hnaif, A.; Taamneh, S.; Alnajjar, A.; Abu Elsoud, E. An intelligent cyber security phishing detection system using deep learning techniques. Clust. Comput. 2022 , 25 , 3819–3828. [ Google Scholar ] [ CrossRef ] [ PubMed ]
- Butt, U.A.; Amin, R.; Aldabbas, H.; Mohan, S.; Alouffi, B.; Ahmadian, A. Cloud-based email phishing attack using machine and deep learning algorithm. Complex Intell. Syst. 2022 , 9 , 3043–3070. [ Google Scholar ] [ CrossRef ]
- Logavarshini, G.; Yogalakshmi, S. E-Mail Spam Classification Via Deep Learning and Natural Language Processing. Int. J. Res. Publ. Rev. 2022 , 2582 , 7421. [ Google Scholar ]
- Ghaleb, S.A.A.; Mohamad, M.; Ghanem, W.A.H.M.; Nasser, A.B.; Ghetas, M.; Abdullahi, A.M.; Saleh, S.A.M.; Arshad, H.; Omolara, A.E.; Abiodun, O.I. Feature Selection by Multiobjective Optimization: Application to Spam Detection System by Neural Networks and Grasshopper Optimization Algorithm. IEEE Access 2022 , 10 , 98475–98489. [ Google Scholar ] [ CrossRef ]
- Babu, D.K. Phishing Detection in Emails Using Multi-Convolutional Neural Network Fusion. Ph.D. Thesis, National College of Ireland, Dublin, Ireland, 2022. [ Google Scholar ]
- Shmalko, M.; Abuadbba, A.; Gaire, R.; Wu, T.; Paik, H.Y.; Nepal, S. Profiler: Profile-Based Model to Detect Phishing Emails. arXiv 2022 , arXiv:2208.08745. [ Google Scholar ]
- Muralidharan, T.; Nissim, N. Improving malicious email detection through novel designated deep-learning architectures utilizing entire email. Neural Networks 2023 , 157 , 257–279. [ Google Scholar ] [ CrossRef ]
- Bountakas, P.; Xenakis, C. HELPHED: Hybrid Ensemble Learning PHishing Email Detection. J. Netw. Comput. Appl. 2023 , 210 , 103545. [ Google Scholar ] [ CrossRef ]
- Wen, T.; Xiao, Y.; Wang, A.; Wang, H. A novel hybrid feature fusion model for detecting phishing scam on Ethereum using deep neural network. Expert Syst. Appl. 2023 , 211 , 118463. [ Google Scholar ] [ CrossRef ]
- Liu, Z.-P.; Zhou, M.-G.; Liu, W.-B.; Li, C.-L.; Gu, J.; Yin, H.-L.; Chen, Z.-B. Automated machine learning for secure key rate in discrete-modulated continuous-variable quantum key distribution. Opt. Express 2022 , 30 , 15024–15036. [ Google Scholar ] [ CrossRef ] [ PubMed ]
Click here to enlarge figure
Ref | Method | Data | Result | Innovations | Limitations |
---|---|---|---|---|---|
[ ] | CNN, MLP, RNN | Self-generated emails dataset | Accuracy: 93.1% | Highlighted issues related to imbalance data | Highly imbalanced nature of the dataset |
[ ] | NN | SpanAssian | Accuracy: 99.07% | Provided guidelines to improve offline data | Needed to enrich the offline dataset to enhance model performance |
[ ] | CEN-Deepspam | Self-generated emails dataset | Accuracy: 95.5% | Larger dataset could improve accuracy | Additional dataset required to validate the result |
[ ] | DBB-RDNN-ReL | Enron, SpamAssassin, SMS Spam Colection | Accuracy: 96.1% | DBBRDNN-ReL model outperformed compared to other models | Slow processing |
Ref | Method | Data | Result | Innovations | Limitations |
---|---|---|---|---|---|
[ ] | THEMIS | Enron and SpamAssassin | Accuracy: 99.85% | Utilized unbalanced dataset | Limited to detecting phishing emails with header |
[ ] | NB, DT, AB, RF, DNN, RNN, CNN | PhishTank | Accuracy: 88.5% | Tf-idf presentation is better than feature hashing and embedding | Limited real-time dataset |
[ ] | DNN | UCI phishing websites | Accuracy: 95% | Hybrid model performs better for classification | Feature selection requires longer time |
[ ] | NN | Debian and PhishTank | Accuracy: 93.9% | Better accuracy | Limited use of deep learning |
[ ] | LSTM | Data-no-header and data-full-header | Accuracy: 89.34% | - | Low effectiveness |
[ ] | Multi-spatial CNN | Self-generated emails dataset | Accuracy: 86.63% | 30% reduction in the execution time | Did not compare model’s performance with other state-of-the-art methods |
[ ] | CNN, RNN, CNN-RNN, CNN-LSTM | Spam dataset. URL dataset | Recall: 99% | Better performance in detecting malware | Performance could be improved by adding sub-modules |
[ ] | CNN, RNN, LSTM, CNN-RNN | Self-generated emails dataset | Accuracy: 98.99% | High accuracy and low FPR | Focused on a single type of phishing attack |
Ref | Method | Data | Result | Innovations | Limitations |
---|---|---|---|---|---|
[ ] | IPDS | URLs | Accuracy: 93.28% | Novel approach to differentiate phishing and legitimate URLs | Ensuring the availability of the dataset would be challenging |
[ ] | CNN | PhishingCorpus and SpamAssasin | Accuracy: 99.42% | Used a huge dataset to detect phishing emails | Used a smaller dataset |
[ ] | Multi-label LSTM | Self-generated emails dataset | Accuracy: 92.7% | Used combined dataset | No comparison of the results |
[ ] | GRU-RNN+SVM | Spambase dataset | Accuracy: 98.7% | Claimed higher accuracy | Limited to one dataset |
[ ] | LSTM+Keras | 800 Turkish emails dataset | Accuracy: 100% | Proposed hybrid model | Limited dataset |
[ ] | RNNs | SA-JN and En-JN datasets | Accuracy: 98.91% and 96.74% | Outperformed state-of-the-art systems | Unrealistically hard |
[ ] | ANN, LSTM, and BILSTM | Self-generated Turkish emails dataset | Accuracy: 100% | Highest accuracy | Focused on the Turkish language only |
[ ] | GAN-based | PhishTank and MillerSmiles | TPR: 97% | Has used actual phishing dataset | Controlled environment |
[ ] | ML, DL, NLP | Rnron, APWG | Accuracy: 93% | - | Limited dataset |
[ ] | SVM combined with NLP and PNN | Self-generated emails dataset | Accuracy: 89% | Probabilistic NN would be more accurate in phishing detection | Only works on a small phishing dataset |
[ ] | CNN | HTML documents | Accuracy: 93% | Automatic phishing web page detection | Limited to HTML document analysis |
Ref | Method | Data | Result | Innovations | Limitations |
---|---|---|---|---|---|
[ ] | GCN+NLP | Self-generated email body text dataset | Accuracy: 98.2% | Enhance phishing detection on the email body text | Tested only English corpus |
[ ] | CNN and LSTM | Self-generated emails dataset | Accuracy: 96.34% | CNN with word embedding is most accurate | Tested only English corpus |
[ ] | D-Fence | Self-generated emails dataset | Accuracy: 99% | D-Fence maintained a high detection rate | Relied on multiple modules |
[ ] | Themis | Self-generated emails dataset | Accuracy: 99.87% | Combined email head and body | Focused only on analyzing the email structure |
[ ] | MLP | SpamBase, SpamAssassin, UK-2011 Webspam | Accuracy: 98.1% | Used several dataset and features | Spam detection study is inadequate |
[ ] | CNN and LSTM | Two datasets | Accuracy: 98.3% | Adam optimizer outperformed the SGD optimizer | Comparison limited to textual data classification |
[ ] | CNN | Self-generated emails dataset | Accuracy: 96.52% | Automated features extraction | Limited datasets |
Ref | Method | Data | Result | Innovations | Limitations |
---|---|---|---|---|---|
[ ] | Fitness-oriented, Levy improvement-based Dragonfly | N/A | Accuracy: 14.93% | Better performance than DT, KNN, and SVM | Misclassification existed |
[ ] | DL+NLP | Text-based and numerical-based datasets | Accuracy: 99% (text-based) and 94% (numerical-based) | Phish Responder better than other models | Limited data used; no explanation on the dataset employed |
[ ] | ML and DL | N/A | Accuracy: 98.5% | BiLSTM classifier performed better | Dataset did not contain variety of spam emails |
[ ] | TshPhish | PhishTank | Accuracy: 98.37% | Improved feature selection through evolutionary algorithms | Low recall rate |
[ ] | CCBLA | Two datasets | Accuracy: 99.85% | Combined CNN, bi-directional LSTM, and attention mechanism | Huge time consumption |
[ ] | LSTM and Glove word embedding | Two datasets | Accuracy: 98.39% and 99.49% | Used multiple datasets | Limited to one language |
[ ] | ML-based voting model | N/A | Accuracy: 98% | Used various feature retrieval algorithms | Lack of benchmark datasets |
[ ] | GRU-based Phishing URL detection | Phishing URLs | Accuracy: 98.30% | Highly accurate classifier | Limited detection of phishing attacks during COVID-19 |
[ ] | Deep learning | N/A | Accuracy: 92% | Incorporated less explored DL techniques | No details of empirical analysis |
[ ] | ML and DL | Spamassassin | Precision: 95.26%, recall: 97.18%, F1-score: 96% | Focused on the limitations of ML and DL algorithms | Broader email content analysis |
[ ] | DL | Email text | Accuracy: 88–100% | - | Cannot effectively handle modern phishing techniques |
[ ] | RCNN | Email Structure | N/A | Examined emails at multiple levels, including the header, body, character, and words | Limited to detecting phishing emails with header |
[ ] | Multiobjective optimization | SpamBase, SpamAssassin, and UK-2011 datasets | Accuracy: 97.5%, 98.3%, and 96.4% | - | Limited to detecting spam |
Ref | Method | Data | Result | Innovations | Limitations |
---|---|---|---|---|---|
[ ] | Deep ensemble learning | Email segments | AUC of 0.993 and TPR of 5% | Higher AUC result | Focus on privacy preservation in future work. |
[ ] | HELPHED | Imbalanced | F1-score: 99.42% | Superior result in the imbalance dataset | Focused on the detection and did not address prevention or mitigation of attacks. The dataset was imbalanced. |
[ ] | LBPS | Ethereum data | F1-score: 97.86% | Phishing scam account detection model | Tested the LBPS model only on Ethereum data. |
The statements, opinions and data contained in all publications are solely those of the individual author(s) and contributor(s) and not of MDPI and/or the editor(s). MDPI and/or the editor(s) disclaim responsibility for any injury to people or property resulting from any ideas, methods, instructions or products referred to in the content. |
Share and Cite
Thakur, K.; Ali, M.L.; Obaidat, M.A.; Kamruzzaman, A. A Systematic Review on Deep-Learning-Based Phishing Email Detection. Electronics 2023 , 12 , 4545. https://doi.org/10.3390/electronics12214545
Thakur K, Ali ML, Obaidat MA, Kamruzzaman A. A Systematic Review on Deep-Learning-Based Phishing Email Detection. Electronics . 2023; 12(21):4545. https://doi.org/10.3390/electronics12214545
Thakur, Kutub, Md Liakat Ali, Muath A. Obaidat, and Abu Kamruzzaman. 2023. "A Systematic Review on Deep-Learning-Based Phishing Email Detection" Electronics 12, no. 21: 4545. https://doi.org/10.3390/electronics12214545
Article Metrics
Article access statistics, further information, mdpi initiatives, follow mdpi.
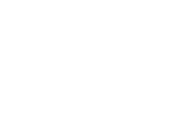
Subscribe to receive issue release notifications and newsletters from MDPI journals

- SUGGESTED TOPICS
- The Magazine
- Newsletters
- Managing Yourself
- Managing Teams
- Work-life Balance
- The Big Idea
- Data & Visuals
- Reading Lists
- Case Selections
- HBR Learning
- Topic Feeds
- Account Settings
- Email Preferences
AI Will Increase the Quantity — and Quality — of Phishing Scams
- Fredrik Heiding,
- Bruce Schneier,
- Arun Vishwanath

How businesses can prepare now.
Gen AI tools are rapidly making these emails more advanced, harder to spot, and significantly more dangerous. Recent research showed that 60% of participants fell victim to artificial intelligence (AI)-automated phishing, which is comparable to the success rates of non-AI-phishing messages created by human experts. Companies need to: 1) understand the asymmetrical capabilities of AI-enhanced phishing, 2) determine the company or division’s phishing threat severity level, and 3) confirm their current phishing awareness routines.
Anyone who has worked at a major organization has likely had to do training on how to spot a phishing attack — the deceptive messages that pretend to be from legitimate sources and aim to trick users into giving away personal information or clicking on harmful links. Phishing emails often exploit sensitive timings and play on a sense of urgency, such as urging the user to update a password. But unfortunately for both companies and employees, gen AI tools are rapidly making these emails more advanced, harder to spot, and significantly more dangerous.

- FH Fredrik Heiding is a research fellow in computer science at Harvard John A. Paulson School of Engineering and Applied Sciences and a teaching fellow for the Generative AI for Business Leaders course at the Harvard Business School. He researches how to mitigate AI-enabled cyberattacks via technical innovations, organizational strategies, and national security policies. Fredrik also works with the World Economic Forum’s Cybercrime Center to improve cybersecurity standards of AI-based cyber defense.
- Bruce Schneier is an internationally renowned security technologist, called a “security guru’ by the Economist. He is the New York Times best-selling author of 14 books — including A Hacker’s Mind — as well as hundreds of articles, essays, and academic papers. His influential newsletter Crypto-Gram and blog Schneier on Security are read by over 250,000 people. Schneier is a fellow at the Berkman-Klein Center for Internet and Society at Harvard University, a Lecturer in Public Policy at the Harvard Kennedy School, a board member of the Electronic Frontier Foundation and AccessNow, and an advisory board member of EPIC and VerifiedVoting.org. He is the Chief of Security Architecture at Inrupt, Inc.
- AV Arun Vishwanath , PhD, MBA, is a distinguished scholar and practitioner at the forefront of addressing cybersecurity’s “people problem” who has contributed commentary Wired , CNN, and The Washington Post . A former fellow at Harvard University’s Berkman Klein Center, he is the founder of the Cyber Hygiene Academy and serves as a distinguished expert for the NSA’s Science of Security & Privacy directorate. He is the author of the book The Weakest Link , published by MIT Press.
Partner Center
- Open access
- Published: 09 August 2020
Don’t click: towards an effective anti-phishing training. A comparative literature review
- Daniel Jampen 1 ,
- Gürkan Gür ORCID: orcid.org/0000-0002-3105-4904 1 ,
- Thomas Sutter ORCID: orcid.org/0000-0003-2649-3299 1 &
- Bernhard Tellenbach ORCID: orcid.org/0000-0002-5008-1107 1
Human-centric Computing and Information Sciences volume 10 , Article number: 33 ( 2020 ) Cite this article
31k Accesses
44 Citations
14 Altmetric
Metrics details
Email is of critical importance as a communication channel for both business and personal matters. Unfortunately, it is also often exploited for phishing attacks. To defend against such threats, many organizations have begun to provide anti-phishing training programs to their employees. A central question in the development of such programs is how they can be designed sustainably and effectively to minimize the vulnerability of employees to phishing attacks. In this paper, we survey and categorize works that consider different elements of such programs via a clearly laid-out methodology, and identify key findings in the technical literature. Overall, we find that researchers agree on the answers to many relevant questions regarding the utility and effectiveness of anti-phishing training. However, we identified influencing factors, such as the impact of age on the success of anti-phishing training programs, for which mixed findings are available. Finally, based on our comprehensive analysis, we describe how a well-founded anti-phishing training program should be designed and parameterized with a set of proposed research directions.
Introduction
The security threat posed by email-based phishing campaigns targeted at employees is a well-known problem experienced by many organizations. Attacks are reported each year, and a reduction in the number of such attacks is unlikely to occur in the near future (see Fig. 1 ). A common type of phishing attack involves an attacker attempting to trick victims into clicking on links sent via email. Such links redirect victims to websites that are carefully designed to mimic those of legitimate organizations with the goal of convincing users to provide their personal information and credentials. Attackers then use the phished data to execute their schemes further. Phishing attacks may be used to obtain access to an organization’s internal servers and steal company secrets or to steal victims’ personal information, such as credit card details [ 1 ]. In this publication, we focus on email-based phishing attacks, as this is currently the most commonly used channel and poses a significant threat to both individuals and companies globally [ 2 ]. Therefore, in this paper, the term phishing always refers to email-based phishing. Phishing is a lucrative criminal activity that is seldom prosecuted. Moreover, take-down measures are often ineffective, as the landing pages used in phishing attacks transmit the stolen data before they can be shut down [ 3 ]. As depicted in Fig. 1 , the amount of global phishing attacks is still huge despite more efforts in combatting them. Failing to address or ignoring the threat posed by phishing can result in detrimental consequences for any company. The 2015 Sony Inc. hack is an example of a successful phishing campaign and demonstrates the extent of the damage that such an attack can cause [ 4 ]. In this case, according to the New York Times , the damage was in the order of hundreds of millions of US dollars [ 5 ].
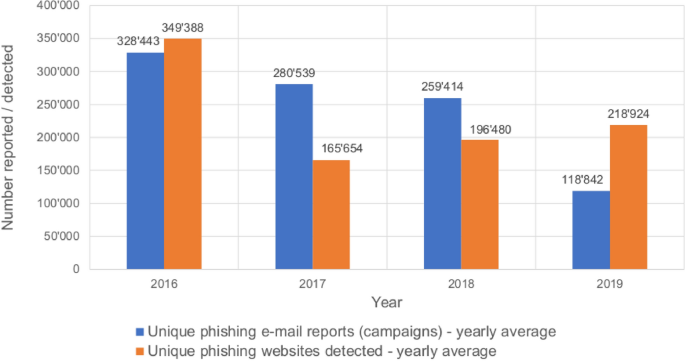
Amount of global phishing attacks reported by APWG [ 7 , 8 , 9 , 10 ]
To increase the perceived legitimacy of phishing emails, attackers often adjust their campaigns according to current events. For example, shortly after the publication of the results of the 2016 United States (US) election, Russian hackers began sending emails with malicious zip files attached from spoofed Harvard University email addresses allegedly explaining “Why American Elections are Flawed” [ 6 ]. Thus, phishing attacks can be very organized and sophisticated, with the potential to cause extensive damage to the targeted party and maximize the gains for the attackers. The damage caused by phishing attacks can only be estimated, as not all incidents are reported, and the overall damage caused can be challenging to quantify [ 2 ]. Nevertheless, Hong et al. [ 11 ] reported that the direct loss caused by phishing in the U.S. varies from 61 million to 3 billion USD per year. However, these figures do not reflect the whole picture, as substantial indirect costs are also incurred of post-attack disruption to the ordinary course of business. In addition, phishing attacks are often used as a starting point for other detrimental cyber-attacks [ 4 ]. As stated in the 2019 Ninth Annual Cost of Cybercrime Study published by Accenture Security, attackers often begin by targeting the human layer, which is the weakest link in corporate electronic security [ 2 ]. In 2013, the Wall Street Journal published an article estimating the annual cost of cybercrimes in the U.S. at 100 billion USD [ 12 ]. Similarly, based on the rapid global digitalization of consumers’ lives and enterprise records, Juniper Research estimated the costs resulting from data breaches in 2015, reaching 500 billion USD globally [ 13 ]. The recent 2019 Official Annual Cybercrime Report from Cybersecurity Ventures, discusses costs of up to 3 trillion USD globally for 2015 and estimates that this figure will double by 2021 [ 14 ]. Despite the variety in terms of the figures estimated, the resulting picture is clear: As a security threat, phishing has to be taken seriously, as it can cause both direct and indirect costs and can open the door to other, even costlier, attacks [ 15 ].
Although there exist various technical solutions intended to prevent phishing emails from reaching their targets, such systems are not perfect and cannot filter out all malicious emails [ 16 ]. Attackers (i.e., phishers), have invariably found means of circumventing newly implemented protection mechanisms in the long run [ 17 ]. In that regard, techniques based on Machine Learning (ML) have yielded promising results compared to other solutions, as, in some cases, they have almost completely defeated zero-hour phishing attacks and have demonstrated very high true-positive detection rates [ 18 ]. Nevertheless, ML does not represent a “silver bullet” against phishing as there are practical challenges that remain to be overcome, such as how these systems should be trained or the threat of adversarial use of ML. As ML is still not an entirely bullet-proof technique, the phishing problem continues to pose a threat [ 19 , 20 ].
Therefore, an essential part of any institution’s anti-phishing strategy is to take a proactive stance by educating its users so that they can identify phishing emails themselves and act accordingly. There are various suggestions concerning how this goal can be accomplished, including offering dedicated courses or simulating encounters with phishing emails, with such scenarios often developed by an institution’s own security staff. As research results show, however, it is unclear how successful any of these methods are (see “ Impact of anti-phishing training ” section for a detailed discussion). Thus, considerable academic attention has recently been paid to how anti-phishing education can be improved and how the utility of this proactive approach can be maximized. However, sources in the literature are occasionally not consistent among themselves concerning specific factors and their impact. This phenomenon results in a situation in which it is challenging for practitioners to create efficient anti-phishing training programs based on academic findings. Additionally, to make the identification of research gaps easier, researchers need to make additional efforts to grasp an overview of the current state of the art. Given the lack of consensus in the literature, it remains challenging to create an effective anti-phishing training program.
Research contributions
Designing an effective anti-phishing strategy involves considering multiple factors, such as how , when and at what frequency users should undergo training. In this work, the term training is used to refer to a process (e.g., a course), intended to improve a person’s awareness and knowledge of phishing, which in turn has a potential impact on his or her ability to detect and respond to phishing attempts. Such training can involve different instruments or media, such as computer-based simulations, videos, and leaflets or other printed materials.
To address the challenges associated with training employees to avoid such attacks, we identify relevant factors that should be considered in a company’s anti-phishing training program, then provide a comprehensive survey of relevant research results and, based on these findings, present a proposal for an ideal anti-phishing training program. The research questions we address within this survey are:
What are the relevant factors of an effective anti-phishing training?
Are there any controversial reports of anti-phishing training effects in the academic work of the last 17 years?
What are the implications of current research findings for designing effective anti-phishing training programs?
This effort is crucial, as insights into anti-phishing training and into how an effective training program can be developed are instrumental in improving defense against phishing attacks. Moreover, a training program serves to reduce potential damage and increase the overall security of organizations. Current research indicates that factors such as the selected training method, how feedback should be provided to users, how training materials should be designed and how retraining intervals should be organized are relevant and thus have direct impact on the success of an anti-phishing program [ 21 , 22 , 23 , 24 , 25 , 26 ]. Considering these findings, this paper makes the following contributions:
It identifies relevant academic works on anti-phishing training (“ Methodology ” section);
It defines multiple categories, each covering one or several of the identified core areas by examining and categorizing the surveyed works (“ Categories ” section);
It concisely presents the most important findings of each study and their implications for an envisaged training program (“ Literature analysis ” section);
It proposes an effective anti-phishing training program based on the performed analysis (“ Discussion ” section).
The next section provides essential background information on phishing. We briefly discuss what phishing is and what can be done to address it. In “ Methodology ” section, we describe the methodology applied in the identification and categorization of phishing studies. We then present a comparative literature analysis, which includes a detailed discussion of findings from a wide range of research works in “ Literature analysis ” section. The discussion in “ Discussion ” section further elaborates on those findings to indicate how they can be used to improve the design and execution of an effective anti-phishing training program. “ Discussion ” section also describes how anti-phishing training tools intended to support the features required for such programs should be developed. Then, " Conclusion " section presents our conclusions and key findings, followed by future research directions in “ Future research directions ” section.
The term phishing refers to attempts by attackers to trick victims into performing a specific action. The objective of such an action could be manifold: it may aim to make the users click on an email attachment, download and execute a file from the Internet. It may also trick them to execute an action on an online platform or to unknowingly provide confidential information such as login or bank details [ 1 , 27 , 28 ]. Often, attackers first attempt to gain the trust of their victims and then abuse that trust to lure users into accessing fraudulent or hacked websites. These websites are carefully designed not to make users suspicious and, for example, contain forms that relay entered data directly to the attacker, provide malicious files to download, or contain exploits that infect the victims’ devices with malware [ 11 ]. Such malware can then be used for a wide range of attacks, such as infecting the target with ransomware or engaging in industrial espionage.
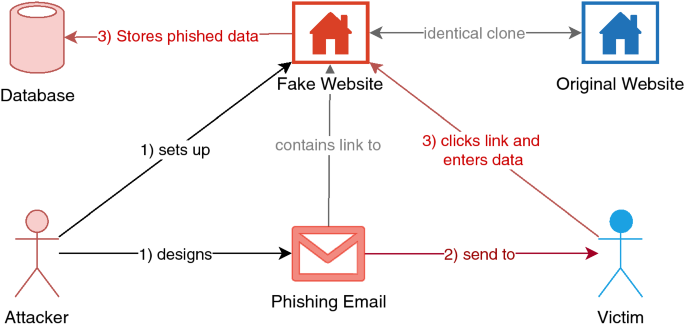
Example of an email based phishing attack
Figure 2 presents an example of an email-based phishing attack [ 29 ]. First, attackers identify an existing website containing a form requesting the data they wish to obtain. They then set up a phishing website by cloning the existing one, design an email containing a link to the phishing website (step 1), and send the email(s) to the phishing victim(s) (step 2). In the event of a successful attack, the victim thinks the email is legitimate; he or she then clicks the link and provides an attack with the desired data (step 3).
One key element of an institution’s anti-phishing campaign is the education of its employees. Currently, several techniques are used to achieve this goal. The most prominent of these are providing informative material concerning phishing, offering dedicated computer-based or “offline” anti-phishing training courses, and developing a phishing simulation that provides anti-phishing training materials if a link is clicked [ 21 , 22 , 25 , 30 ]. All of these strategies have different pros and cons, which should be considered from a cost-benefit perspective. In particular, the resources required (e.g., money) are often a decisive limiting factor. For instance, according to research based on responses provided by 500 companies with between 1000 and 5000 employees in 2017, the cost of user security education that includes anti-phishing training has reached approximately 290 K USD per year for large enterprises [ 31 ]. Therefore, we believe that determining the most effective training program is critical in overcoming such concerns and improving cybersecurity. However, please note that addressing the cost-effectiveness of training programs is beyond the scope of this survey.
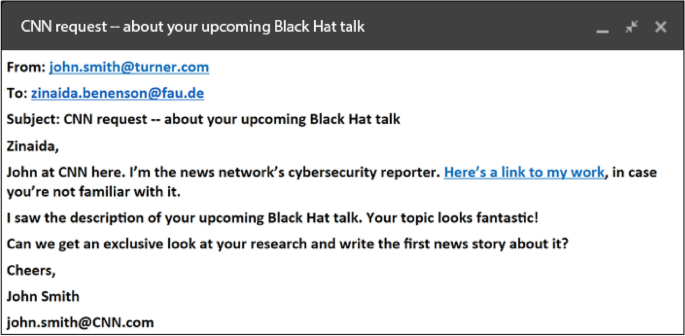
Sample spear-phishing email abusing a user’s curiosity by the fact that the content fits the target’s actual behavior, as the receiver was about to give a talk at BlackHat USA 2016 [ 32 ]
Besides generic phishing , in which many potential victims are targeted, spear phishing describes a specially tailored phishing attack against one victim or a small group thereof [ 33 ]. Attackers research their targets and abuse the acquired information to design phishing emails for each victim. As, in such cases, the contents of a phishing email will then reflect the target’s current activities, the likelihood of the attack succeeding will probably increase. An example of a spear phishing email exploiting the current activities of its target is presented in Fig. 3 .
- Phishing countermeasures
Phishing countermeasures can be applied at several stages during an attack. Considering the general attack model in Fig. 2 , a technical filtering solution could be deployed in Step 2 (e.g., [ 20 ]). Such solutions process and analyze all incoming email messages and, based on rules or ML, classify them as either phishing or legitimate . ML filtering techniques have become state of the art and the classification of phishing website (e.g., [ 34 , 35 , 36 , 37 , 38 ]) can be used for blacklists. Such approaches can prevent a phishing message from reaching the target user, but attackers can use ML techniques as well (e.g., [ 39 ]) for bypassing such AI detection systems. Furthermore, ML based countermeasures can be further adapted and optimized for different operational environments to improve performance and combat implementation challenges. A recent example is [ 40 ] where the authors have implemented an anti-phishing virtual network function at the edge of the network with embedded robust machine learning techniques for phishing detection.
Alternatively, education of users is a proactive method. In other words, users themselves could be educated to identify phishing scams (e.g., [ 41 ]). By creating awareness of phishing attacks and training users to be able to identify them, this method can prevent employees from falling victim to phishing scams and therefore prevent possible information leaks. Additionally, web filtering software or a specific firewall could be used to analyze all of the websites visited by an employee (Step 3 in Fig. 2 ) and attempt to prevent access to sites with malicious intent (e.g., [ 42 ]). This would again prevent users from inadvertently leaking information. A more active approach is the take-down of phishing websites by third parties such as the law enforcement agencies (LEAs) or the hosting services to prevent potential victims from accessing such websites (e.g., [ 3 ]). According to Hong et al. [ 11 ], the duration of the entire take-down process averages approximately 62 h. An important requirement for an efficient mitigation effort is multi-agency participation (e.g. Internet users, brand enterprises, browser manufacturers and authorities) with uniform data sharing format and unobstructed sharing channels for common phishing reporting. One way to achieve this is with multi-party phishing data sharing platform based on blockchains [ 43 ]. In summary, the methods listed in Table 1 are available to counteract phishing.
When attempting to address phishing attacks, security should always be implemented using multiple layers of defense (defense-in-depth), as each layer has its strengths and weaknesses [ 45 ]. In that regard, each layer should be considered breakable, as no bullet-proof solution against phishing currently exists. Therefore, a combination of the layers, as mentioned earlier, would be an approach to the problem. An essential aspect of such a defense strategy would be to educate employees and strengthen their ability to identify phishing attacks. This requirement raises the critical question of how anti-phishing training programs and tools should be designed and implemented, which constitutes the primary rationale for the contributions made by this paper.
Methodology
In this section, we describe how the literature for this survey was selected. We explain the methods used for searching, filtering, and selecting the literature. Moreover, we introduce a categorization system for academic anti-phishing training papers and use this system in “ Literature analysis ” section to categorize the selected papers.
During this study, we carried out two iterations of our literature selection process. We conducted the first iteration in November 2018, and it includes articles from 2003 to 2018. It contains the main corpus of our research. In April 2020, we executed the second iteration of our selection with the scope of articles from 2019 and 2020 during the peer review process of our paper. We chose articles between 2019 and 2020 for the completeness of our survey and because we wanted to include the latest state-of-the-art articles when it is published.
The literature related to anti-phishing training is extensive. It covers areas ranging from technical approaches for exploiting a weakness of a given email client with phishing purposes to user education in general. In this work, we analyze a comprehensive set of publications related to factors relevant to anti-phishing training and the success thereof. There are several types of training, such as the use of using videos, web-based courses, informational material such as leaflets/flyers, or simulated phishing attacks. No specific type of training is favored in this publication.
To this end, “ Identifying relevant sources ” section provides information concerning how the works included in this survey were chosen (i.e., what criteria they had to meet). In “ Categories ” section, we accordingly construct categories based on the identified literature, which we later utilize to classify the surveyed papers.
Identifying relevant sources
To render it as reproducible and as clear as possible, we have divided the identification process into multiple steps. This section explains each step in detail, while Table 2 shows how those steps were used to narrow the relevant literature down to the works included in this survey.
Prior to the first step, in which the search keywords were defined and the initial set of publications was obtained, potential electronic sources were evaluated. Querying the search engines of IEEE Footnote 1 , ACM Footnote 2 , ScienceDirect Footnote 3 , Wiley Footnote 4 and GS Footnote 5 with the keyword “anti-phishing training” (including the quotation marks) returned the following number of publications: GS : 406, IEEExplore : 2, ACM Digital Library : 3, ScienceDirect : 11, Wiley Online Library : 5. Cross-checking the publications returned by each search engine indicated that the search engine that returned the most results, GS, already included the publications found by the other engines. As stated in the GS About page [ 46 ], this engine provides a service that allows users to search the databases of many publishers from one location as reflected in the results of our initial search engine test. Therefore, GS was selected as the literature search engine for this survey.
In Step 1 of the first iteration, which is the start of the literature identification process, GS was used to obtain a set of publications for potential inclusion in this survey. In the initial search engine test, the keywords “anti-phishing training” has been used. As this query returned only 406 publications, we expanded the search by using a more general keyword: “phishing”. We ensure that the publications remain relevant with a 15-year date filter (2003 to 2018) that we added to the query. This search returned 37,300 results, of which GS returned the first 1000 publications, sorted by relevance. GS ranks the list of results by weighting the full text of each document, where it was published, by whom it was written, and how often as well as how recently it has been cited in other literature [ 46 ]. As an additional check, the other search engines queried in the initial search test were also queried using the new keyword, whereupon IEEExplore returned 1040 publications, ACM Digital Library 1148, ScienceDirect 2678 and Wiley Online Library 1241. As GS does not provide a way to export search results, the tool Publish or Perish was used to perform this task [ 47 ].
Step 2 involved the application of a generic filter to the data set obtained in Step 1 to focus on scholarly publications with complete identifier data. In that regard, we removed publications for which GS could not identify a publisher or source. Moreover, we applied a filter that removed patents.
In Step 3 , we used the publication title as the primary indicator of whether it is relevant to the focus of this paper. First, we conducted a keyword search with the following keywords: Awareness, training, phishing, susceptibility, and behavior. In case one of the keywords was found within the title, we directly selected it for Step 4. If we did not find any of the keywords, we examined the abstract and the conclusion of the paper. If the abstract or the conclusion of the paper had a focus of anti-phishing training, we selected it for Step 4. The remaining steps constituted a progressive identification process (elimination according to various attributes such as being peer-reviewed and the level of relevance) for the list of publications.
In Step 4 , we applied an attribute-based approach to filtering to the list from Step 3 for quality control in terms of peer reviewing and experiment design. We applied the following quality criteria in this step:
Peer-reviewed: To ensure the quality and reliability of our survey’s conclusions, only peer-reviewed papers are considered
Target study group size: Works presenting conclusions based on a low participant number n in their studies ( \(n<20\) ) are not included (see Table 3 for further details)
Control group: All publications that involve actual participant training have to make use of a control group to verify their findings against participants who do not undergo any training
Language: Only publications in English are considered
The final step, Step 5 , requires the most effort, as it is in this stage that the list of publications identified in Step 4 are thoroughly evaluated and selected due to their merit. In this step, each remaining paper was examined, read, and reviewed to determine whether it offers relevant contributions to the focus of the present study.
For the second iteration, we applied the same steps, but for the time between 2019 to 2020. Finally, the merger of the two result sets provided us the surveyed paper base.
Limitations of survey methodology Identifying studies for potential inclusion in a literature survey is a process wherein limits and boundaries have to be set carefully. Depending on the adopted data collection guidelines, a comprehensive overview of the existing literature should be provided in survey work, although such an overview is usually not exhaustive in terms of coverage. For our work, GS was used as the search engine of choice. Since the engine already returned many relevant publications (e.g., 37,300 results for 2003–2018 period), no additional searches (e.g., backward/forward citation searches) were performed. Such complementary methods may have led to the identification of additional articles; thus, some relevant articles may have been omitted due to the methodology in the present study, which was based on keyword searches. However, considering the vast body of articles evaluated and the fact that the rating system of GS considers both relevance and impact, we believe that this work presents a comprehensive study and contributes to research on anti-phishing training.
We divide the selected publications into multiple categories, each of which covers one or multiple core area(s), which were identified by examining those works. A paper can appear in multiple categories should its results cover more than one area/factor. For a better overview of the approach to categorization described in the following sections, all papers, along with their corresponding categories, are presented in Table 4 . We compare the results of a reviewed paper with those of other studies in the same category. Therefore, the objective of the comparative analysis conducted in this paper is to identify contradictory findings and evaluate consistency with other findings and conclusions.
Research results concerning phishing mostly address either attack success rates or training effects . For example, a work stating that emails containing links in a specific format are more successful would fall into the former group while another describing an educational game and the effects thereof on the participants would belong to the latter. In our categorization, the second and third categories in Table 4 include papers concerning attack success rate, while the others address training effects. Both of these groups are crucial since they render the inherent factors on anti-phishing training design and effectiveness by jointly illustrating aspects of susceptibility, attack success, and training efficiency.
Our classification does not cover all possible relevant features of an anti-phishing campaign. In the context of this survey, we identify the dominant ones and restrict the analysis to them in the interests of concision and clarity. We list all categories and provide brief descriptions of the data that are covered by them:
Training impact contains data concerning the training effects after exposing users to anti-phishing training. The key questions are related to the benefits of training: Is educating users a viable approach? Does it help at all, or should the focus of IT personnel be on other phishing defense strategies?
Target group impact contains findings regarding which users exhibit a better or worse ability to identify phishing threats and whether this ability changes as a result of anti-phishing training. This data can be used to find weak links within an organization and direct increased training efforts towards strengthening them.
Email content and structure includes data related to the design and structure of phishing emails. The essential questions concern the visual appearance of such emails and how it is designed, the content and how (URLs) can make it easier or more difficult for users to determine the legitimacy of a phishing email.
Feedback contains information concerning the design of the web pages for phishing attacks and the phishing training material. Moreover, it contains findings of possible ways to present educational material and how effective these are.
Knowledge retention includes findings of how long the knowledge gained through educational measures is retained, how effective it is, and in which intervals users should be retrained.
Literature analysis
In this section, we present the analysis of surveyed works structured into the categories defined in our methodology. In each category, we employ a consistent approach to structuring our review of the literature: A discussion of related works follows a short introductory section; after that, a concise analysis of the findings of each study is performed to identify any common traits and to draw conclusions. This presentation pattern is intended to make the literature review and analysis more accessible for the reader.
Impact of anti-phishing training
A fundamental question concerning the design and structure of training programs is whether or not they have a measurable impact on employee behavior with regard to phishing emails. In the following survey segment, we first discuss those studies that report a positive effect of anti-phishing training measures. We then conclude the survey by reviewing literary sources that report mixed results. As an important note, the list of papers obtained through the selection process does not contain any papers that report negative results only.
There is a large body of publications that confirm a decreased likelihood that users will fall victim to phishing messages after educating them with general anti-phishing material or via embedded training. The latter is a training method that is seamlessly integrated into a user’s typical workflow. Embedded training usually works along the following lines: A training system sends artificial phishing emails to a set of trainees. The trainees have to identify and report those emails when they process their emails during a typical workday. In contrast to controlled training environments, embedded training occurs under realistic settings in which trainees might be affected by factors such as distractions, stress, and a lack of focus. Should a trainee click on the link in the phishing email, he or she will fail the training and receive some kind of education (see “ Feedback ” section).
In [ 104 ], Neupane et al. conducted a multi-modal neurophysiological study regarding phishing detection and malware warnings. In regards to the participants’ trainability, the authors found that their users were paying attention to the information provided and made active efforts while performing the assigned tasks. Based on these results, the authors conclude that the participants in their study did not ignore provided training materials and that training is indeed a valuable approach to address phishing. In the same vein, Halevi et al. confirmed that awareness, which often increased due to training, helped their participants not to fall victim to phishing as the subjects were more concerned about protecting themselves [ 107 ]. In [ 101 ], Greene et al. examined long-term, operationally-situated data that was captured during embedded phishing awareness training exercises held throughout four and a half years at a U.S. government institution. Apart from an improved phishing detection rate, the authors also observed new competition due to the gamification of the phishing awareness training exercises over the years. Participants would attempt to beat their colleagues and be the first to identify the phishing emails, which possibly improved the training results further. Doge et al. [ 71 ] report similar success when using embedded training. In an experiment with three groups of approximately 300 participants each, the first group was exposed to embedded training, the second group received a notification after falling victim to a phishing email, and the third was the control group which was not exposed to training. Their results indicate that over a period of 10 days, there was no significant difference in terms of susceptibility among the three groups. However, over a more extended period (63 days in this experiment), training was found to result in significant improvements for the participants’ clicking behavior. Of the participants who received training, 24.5% failed the experiment. Of those participants who received feedback alone, 32.08% failed, and, in the group that received neither feedback nor training, 47.5% failed.
A more recent work by Gordon et al. is a retrospective study of employee susceptibility at six US health care institutions. In this multicenter study, phishing simulations (95 campaigns) were run from 2011 to 2018, with 3 million phishing emails sent to employees of those organizations. Overall click rates varied by institution but were notably high: on average, around 400,000 (14%) of simulated emails were clicked on by employees. in their work, repeated phishing campaigns were associated with reduced odds of clicking on subsequent phishing emails. In models adjusted for several potential confounders, including year, the institutional campaign number, institution, and email category, the odds of clicking on a phishing email were 0.511 lower for 6 to 10 campaigns at an institution and 0.335 lower for more than 10 campaigns at an institution. They also found that there were important institutional differences in click rates, as well as differences in click rates between email category and season. Other papers included in this study that report the embedded training method having a positive effect are [ 21 , 22 , 24 , 41 , 82 , 99 , 109 , 110 ].
Papers that report mixed results but that are partially in favor of a positive effect of anti-phishing training are [ 50 , 55 , 72 , 76 , 80 , 126 ]. In [ 76 ], Orunsolu et al. examined the effectiveness of the security tips provided by a Nigerian bank to their customers as a form of education. These messages provide information on how users can identify online scams and which actions users should avoid. The authors’ findings showed that most participants were unable to reliably identify a phishing email despite having been exposed to the security tips. After this test, the authors performed a course-based training session, and, in the follow-up test, participants exhibited an increased success rate in identifying phishing threats. In [ 55 ], Caputo et al. obtained mixed results in their study regarding the impact of anti-phishing training. They found that the phishing detection rate of members of two groups, whom they referred to as “all clickers” and “non-clickers” did not improve at all, as they always (11%) or never (22%) clicked, regardless of the applied training method. Additionally, the authors grouped the remaining 67% into a group they called “inexplicable.” Users in this group seem to click or not click on phishing links randomly. Nevertheless, the authors note that phishing messages that are not detected by technological solutions are often identified as a result of company personnel reporting an email as being suspicious. According to Caputo et al., providing a reporting feature should be considered as a possible additional layer in a company’s phishing defense system, mainly, as early reports provide meaningful benefits for members of an organization’s incident response team. Karakasiliotis et al. [ 126 ] conducted a study to assess end-user awareness of social engineering and phishing. They conclude that a need for increased security awareness is evident but designing a generalized approach to achieving such awareness could be a complicated process due to the technical unfamiliarity of users or behavioral differences among them.
Vishwanath et al. [ 80 ] developed a methodology for determining why so many users fall victim to phishing and why this seems to occur on a random basis. They report that a user’s susceptibility to phishing depends on multiple factors, only one of which can be trained using the embedded training technique. However, using the method developed by the authors, security officers can identify the weak links within their organizations; in addition, it enables them to determine how much training an employee requires and to set the focus of the training. Siadati et al. [ 50 ] found that training participants using persuasive phishing emails significantly improves their average resilience to such emails. In contrast, training involving emails that were not considered to be very persuasive had little impact on the phishing susceptibility of the investigated users. Finally, Moody et al. [ 72 ] report that even with education, users are still overconfident in their ability to detect phishing messages. This overconfidence can, however, be diminished through education.
As multiple research studies show, an increased ability to correctly handle phishing emails after receiving anti-phishing training is well supported in the scientific community. However, Caputo et al. identified two groups of users who were not affected by the applied training: those who clicked all links and those who never clicked. However, the authors did not address how these groups should be educated.
While most studies have attempted to answer the question of whether training makes trainees less susceptible to phishing attempts, little information is available concerning how such training changes their behavior regarding benign emails. Three notable exceptions are [ 30 , 75 , 102 ]. In [ 75 ], Kumaraguru et al. report that embedded anti-phishing training does not affect users’ willingness to click on links in benign emails [ 75 ]. However, this is in contrast with the findings of Sheng et al., who report that some users stopped clicking on legitimate links in emails when the design of the provided training materials did not take such behavior into account. Unfortunately, the authors did not identify the type of design that could achieve this outcome [ 30 ]. The finding of Sheng et al. is confirmed by Yang et al. [ 102 ], which confirms that this issue should be given special attention.
Another intriguing issue is raised by a literature survey conducted by Khonjii et al. [ 122 ]. They conclude that user education has a positive impact, but they criticize the fact that none of the reviewed studies evaluates whether such improvement is still meaningful when considering different technical phishing-detection solutions. If there are solutions that can filter all but those emails with which users struggle to identify, training would not provide any benefits even after appropriate anti-phishing training.
In summary, these mostly positive results indicate that anti-phishing training indeed has a positive impact. However, training design, especially complementing embedded training with standard training sessions and even individualization of training, might also play an important role.
Target group impact
Findings in this category feature works that are related to user-specific properties. For example, they may note that users working in technical jobs are as likely to fall victim to phishing as others. Such insights are critical for identifying groups of users who are more susceptible to phishing. Employees in such groups could accordingly receive additional training or receive different types of training to mitigate possible attacks.
In this part, we focus on the properties presented in Table 5 , and we use it as a guide for the discussion of the works considered in this section. More specifically, we first discuss all of the works included in the column titled Has impact and then those included in the No impact column. Within a column, we start with the papers listed for the first parameter and then continue row by row. However, as most papers present findings concerning more than one parameter and discussing the same paper in multiple places makes little sense, we also discuss the findings related to other parameters on the first mention of a source. As a consequence, when we follow the order of the parameters, only papers that have not yet been introduced will be discussed.
Papers reporting impact In [ 75 ], Kumaraguru et al. report on an experiment in a university setting. They find that participants between the ages of 18 to 25 are consistently more vulnerable than other age groups. Sheng et al. confirm the same finding in [ 75 ] regarding this age group. Furthermore, Sheng et al. state that the results of their roleplaying online survey instrument-based study involving 1001 participants suggest that women are more susceptible to phishing, probably because they have undergone less technical training [ 30 ].
Another study that points in the same direction as Sheng et al. is by Jagatic et al. [ 56 ]. The authors tested students and found that a phishing mail was slightly more likely to be successful when the sender was of the opposite gender to the receiver. In [ 78 ], Iuga et al. consider relationships between the demographic characteristics of individuals and their ability to correctly identify a phishing attack, as well as the impact of time-related factors. Their results suggest that gender and the number of years of computer usage experience have a statistically significant impact on the phishing detection rate; the same can be observed for the psychological anchoring effect.
Halevi et al. [ 107 ] studied the impact of gender, awareness of cyber-risks, and personal traits on spear-phishing susceptibility. They used a combination of a questionnaire and a real-world phishing simulation and found that women are more likely to respond to spear-phishing messages about winning a prize than men and that people who are more aware of cyber-risks are less susceptible to such attacks. Concerning personality traits, Halevi et al. found that less suspicious/aware online users are more likely to fall victim to phishing and that conscientiousness can be targeted by attackers to gain a higher phishing response rate. They suggest that, based on their findings, a user-targeted approach to phishing defense may be required.
Flores et al. [ 100 ] conducted a study with a focus on targeted phishing attacks. Their results contradict the previously discussed findings concerning the impact of gender as they found that women are less susceptible to phishing attacks. Furthermore, the authors report that an individual’s trust and risk behavior significantly affected his or her actual behavior during the phishing experiment. Specifically, computer experience at work and willingness to help showed a significant correlation with the participant’s phishing susceptibility.
Hong et al. [ 105 ] aimed to identify user profiles that can be used to predict when phishing attacks will be successful. They sought to identify attributes that make some individuals more vulnerable to phishing attacks than others. Their results suggest that gender, trust, and personality are among those attributes.
Another user-specific property is the technical background of a person and the degree to which his or her job is technical. Butavicius et al. conducted two experiments: In the first, they did not tell the participants to be aware of phishing emails, whereas, in the second, they did [ 125 ]. They found that computer-savvy participants were more vulnerable to phishing attacks; however, this was only found to be the case in the informed experiment. In the non-informed experiment, they performed similarly to the other participants. Also, by comparing their results with those of a prior personality test [ 139 ], Butavicius et al. found that participants in the non-informed experiment performed better in terms of detecting phishing emails when they had more extroverted or open personalities. The same was found for less impulsive people in the non-informed experiment. The authors inferred that those participants who probably deliberated over a phishing email appeared to demonstrate better performance in detecting phishing emails [ 125 ]. More support for the impact of a person’s technical knowledge comes from Flores et al. [ 70 ], who investigated the correlation among selected psychological and demographic factors.
Furthermore, to assess the impact of national culture on these correlations, they performed an experiment involving 2099 employees of nine organizations in Sweden, the USA, and India. It was found that general information security awareness, formal information security training, and computer experience showed a positive correlation with phishing resilience. However, the authors also observed that the behavior demonstrated in response to phishing differs among Swedish, US, and Indian employees.
Parsons et al. [ 88 ] present another interesting finding concerning the impact of the participant’s technical knowledge. They report that whether or not participants are aware that they are participating in a phishing study might have a significant impact on the outcome of such a study. Participants who were informed that they were participating in a phishing study demonstrated significantly better performance in terms of identifying phishing emails and took longer to make decisions. Intriguingly, participants who had formal training in information systems (technicality) performed more poorly overall.
The impact of trust in a sender’s email address was the subject of research conducted by Moody et al. [ 72 ]. Their results show that users’ susceptibility tends to increase when the sender of a possibly fraudulent email is known and reduces if the sender is unknown. The results indicate that users are more likely to click on a link in an email should they believe that the sender is deceitful. This behavior could be caused by the users’ desire to discover the true intentions of the sender. Also, the authors state that users who frequently browse the Internet are more likely to click on links in emails than others.
Alseadoon et al. performed a simulated phishing attempt and applied the detection deception model [ 137 ] developed by Wright et al. to determine which individuals are more susceptible to phishing. The authors of this study conclude that users who have less email experience and high levels of submissiveness are more likely to fall victim to phishing [ 83 ]. Harrison et al. [ 84 ] observed that individual factors such as knowledge and experience with email increase resilience to phishing attacks. The focus was on the characteristics of phishing emails, users’ knowledge of and experience with phishing, and how these factors interact and influence how users cognitively process phishing emails. It was found that phishing susceptibility can be predicted by a particular combination of a user paying little attention to some aspects of an email and a high degree of elaboration on the part of the phishing message.
However, email experience, especially in the form of personal email habits and processing strategies, might also have a negative impact. Vishwanath et al. [ 85 ] compared the causes and consequences of email habits and cognitive processing. The results of their simulated phishing attack indicate that the cumulative effects of heuristic processing and email habits were the main factors affecting the phishing susceptibility, as they were found to cause a fourfold increase in a user falling victim to a phishing attempt and, therefore, nullify any advantage offered by systematic processing [ 85 ].
According to the study conducted by Workman [ 73 ], people who are more trusting and obedient to authority are more susceptible to social engineering. Furthermore, the author found that people with higher normative, effective, and continuance commitments are more likely to fall victim to phishing attacks. Normative commitment refers to the formation of implied obligations to others. Continuance commitment refers to becoming emotionally invested in a decision, and affective commitment means that people model the behaviors of other groups, role models, or important persons.
One parameter that is mentioned in many studies is the impact of people’s level of awareness. In [ 77 ], the authors conducted a phishing exercise in an academic environment as part of an ongoing information security awareness project. They found that educational and awareness activities pertaining to email environments are critical in managing the increased threat of identity theft. Another study pointing in a similar direction is that of [ 86 ], in which the authors use signal detection theory to measure vulnerability to phishing attacks, including variation in performance across task conditions. They found that phishing-related decisions are sensitive to individuals’ response bias, confidence, detection ability, and perception of consequences (awareness). Specifically, higher sensitivity was found to be positively correlated with confidence, while greater willingness to treat emails as legitimate was negatively correlated with the perceived consequences of participants’ actions and positively correlated with confidence.
Arachchilage et al. [ 112 ] developed a new game design that educates users about phishing. Their study results showed a significant improvement in participants’ phishing avoidance behavior in the second test assessment conducted by the authors. The findings suggest that participants’ threat perception, safeguard effectiveness, self-efficacy, perceived severity of a potential threat, and perceived susceptibility elements positively impact threat avoidance behavior, whereas safeguard cost had a negative impact.
Abbasi et al. [ 74 ] confirm that awareness is an important factor, but only one of many. Nearly two-thirds of the users in their study fell victim to the phishing mail created by the authors. A cluster analysis of the collected data, which was obtained via questionnaire and phishing simulation, found that, among other factors, over-confidence, a low awareness level, and a high level of trust in technology on the part of the user were detrimental.
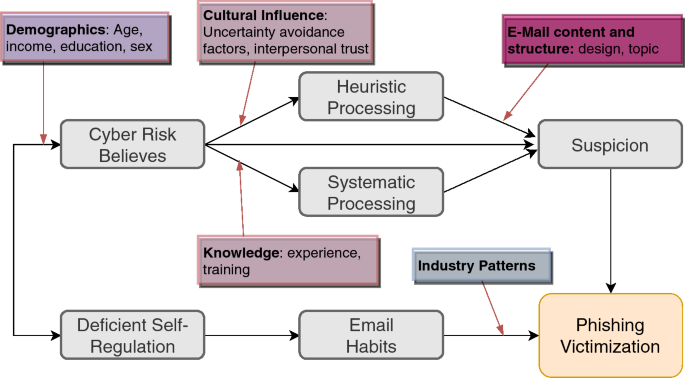
The Suspicion Cognition Automaticity Model (SCAM) [ 80 ]
In [ 80 ], Vishwanath et al., the authors found that a user’s awareness level is of similar importance. They observed that research related to human factors and their impact on phishing victimization generally identifies two main sets of factors: The first set is the victim’s cognitive processing schema, which is influenced by his or her awareness of the safety of engaging in certain online activities. The second set of factors is the behavior rituals developed by a user based on the work cultures experienced and/or the types of communication devices used. Based on these findings, the authors developed the Suspicion Cognition Aromaticity Model (SCAM) model, which is presented in Fig. 4 . It describes the likelihood of such victimization of an employee based on the following five parameters: individual beliefs concerning cyber-risk, both heuristic and systematic patterns exhibited while processing an email, deficient self-regulation, and developed email habits. As the SCAM was developed to include all of these parameters, it uses experiential, dispositional, behavioral, and cognitive factors to provide a more comprehensive explanation of the phishing victimization process. Vishwanath presented a proposal concerning how to apply the SCAM for practical use at Blackhat 2016, where he presented how one can calculate the Cyber Risk Index (CRI).
Vishwanath et al. propose a questionnaire with 40 questions, the result of which is used as an input for the algorithm in Fig. 5 . First, the algorithm asks the user about his or her cyber-risk beliefs. If these beliefs are faulty, they will have to be changed. If they are reasonable, the user’s phishing email identification heuristics are checked. Should a user exhibit poor heuristics, the algorithm will suggest teaching better heuristics. However, should a user apply good heuristics but process emails inadequately, anti-phishing training should be applied. Moreover, even if an employee passes all of these checks, he or she may still fail to identify a phishing email due to bad habits, which would also have to be remedied.
In their multi-modal neuro-physiological study, Neupane et al. [ 104 ] found in their multi-modal neuro-physiological study that their participant’s personality traits, specifically attention control, directly impacted their phishing detection accuracy. The authors conclude that users may better detect phishing attacks if they could, in addition to undergoing phishing awareness training, be trained to exercise attention control. The authors note, however, that further work is necessary to understand the effect of such interventional training on the user’s performance in phishing detection tasks.
In [ 127 ], Butavicius et al. point in a similar direction by reporting that the participants in their study who were less impulsive in terms of decision-making were more likely to consider the links in phishing emails as being dangerous. Based on that observation, the authors state that a lower level of cognitive impulsivity could protect against spear phishing. In addition, they found that lower cognitive impulsivity did not adversely influence the participant’s judgment of genuine emails.
The study conducted by Welk et al. confirm the results of the study by Butavicius et al. related to impulsivity [ 106 ]. Welk et al. aimed to determine how individual differences relate to performance on a phishing task by having undergraduate students complete a questionnaire and an email task in which they had to discriminate between legitimate emails and phishing attempts. The results indicated that certain trust, personality and impulsivity predictors were linked with accuracy in terms of detecting phishing attempts: personality characteristics that support reserved behavior, low impulsivity and distrust decreased phishing susceptibility in an email-based decision-making task.
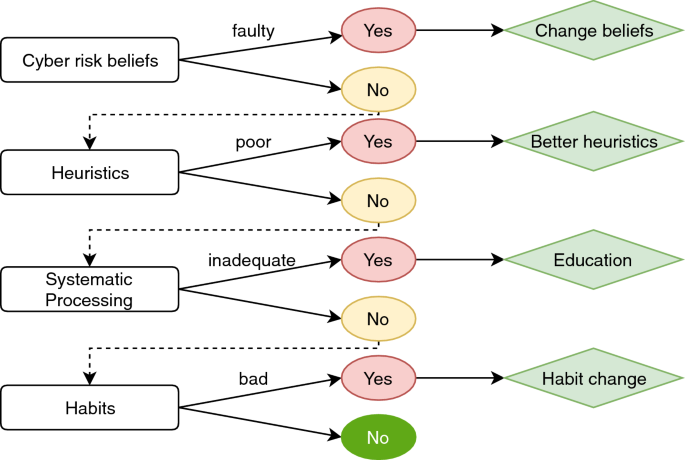
Algorithm developed by Vishwanath et al., which was presented at Blackhat 2016 and determines why users potentially fall victim to phishing and what training should focus on
Papers reporting no impact [ 99 ], Zielinska et al. conducted a questionnaire-based study with 96 participants recruited from Amazon Mechanical Turk. The study did not find any differences in age or gender in terms of susceptibility to phishing.
Similar findings were obtained in a study conducted by Kumaraguru et al. [ 21 ] that analyzed data from 42 participants and a study by Mohebzada et al. [ 48 ] involving 10,000 participants. However, instead of age and gender, [ 48 ] report that awareness is a critical parameter, as 10% of the users investigated in their study fell victim to phishing. Benenson et al. investigated whether there is a statistical correlation between the following factors and the click rate on phishing links:
Gender of the sender and receiver
Subjects are friends on Facebook
Sender has a publicly available Facebook profile
Receiver has knowledge that emails can be spoofed
Receiver knows that clicking on links in emails can be dangerous
Their results show no significant statistical correlation between these factors and the clicking behavior [ 54 ]. Karumbaiah et al. [ 25 ] found the same for gender but also the personal traits of trust and perceived internet risk.
Another adverse finding concerning user properties has been reported by Leukfeldt et al. [ 49 ]. Their study shows that frequently engaging in online activities such as participating in chat rooms, gaming, actively using forums or engaging in high-visibility social networking is not correlated with an individual’s susceptibility to phishing; furthermore, operating system or browser affinity was not found to be correlated with their phishing susceptibility as well.
The last no-impact findings concern the parameter of the technical complexity of an individual’s job. In [ 82 ], Kumaraguru et al. find that employees working in technical and non-technical jobs exhibit similar susceptibility to phishing. This is confirmed by [ 81 ], who states that even educated users can fall victim to phishing as their detection ability alone may not be enough to prevent an attack. The authors also argue that contextual factors indirectly influence phishing susceptibility. They conclude that individuals fall victim to phishing attempts due to their lack of cognitive involvement rather than an inability to detect phishing.
Table 5 summarizes what the surveyed body of work reports regarding the impact or lack thereof user-specific properties. One key observation is that, with the exceptions of the properties age, gender, frequent engagement of online activities, and job technicality, the answer to the question of whether or not a parameter has an impact on susceptibility to phishing attacks seems quite clear. However, especially for properties that were discussed by only one of the publications, the observation should be taken with a grain of salt.
As many parameters have been identified as impacting susceptibility to phishing attacks and given that there may be many more, an efficient approach could be to start training all employees using the same framework. In a subsequent step, a training regime (i.e., differentiation) could then be developed based on their response to training and progress using models such as the SCAM or the CRI proposed by Vishwanath et al. [ 80 ].
Email content and structure
This section covers essential aspects one should consider when designing and populating a phishing email to use in anti-phishing training exercises. Such aspects could the email’s visual appearance, how the link Uniform Resource Locator (URL) is masked, or the content’s context (see Fig. 7 ). Multiple studies have investigated how these properties influence the success rate of phishing emails. Analyzing these results enables the creation of synthetic phishing emails with varying levels of difficulty in terms of detection.
Siadati et al. [ 50 ] conducted a study on how the content of a phishing email impacts its success. They investigated which topics were more appealing to the participants in their study, as well as whether more persuasive content influences the outcome of phishing attempts. The results clearly show that persuasive emails do, indeed increase the success rate. Moreover, the following five email topics were identified as the most effective (see Fig. 6 for more details): shipping, order, received fax email template #1, received fax email template #2 and complaints. In Fig. 6 , the thinner bar shows the click-through rates of individual groups, while the thicker bar shows the range for a click-through rate of individual campaigns. Red dots show the weighted average click-throughs over the campaigns. On the other end of the spectrum, topics such as celebrity, sports, or newsletter are the least ineffective ones yielding meager click-through rates. The top-five most effective topics have more than twice the click-through rates than the immediately following topics. Caputo et al. observed no statistically significant difference in the clicking rate when exposing participants to email contents that the authors divided into the following categories: other gain , other loss , individual gain and individual loss [ 55 ]. For example, an email from the category other loss would state that another individual would suffer a financial loss should the recipient not click on the link provided.
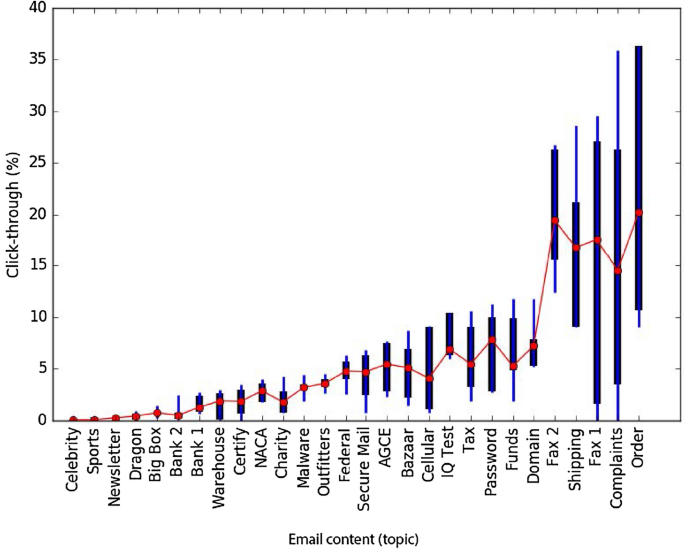
Click-through value ranges for the phishing emails used in [ 50 ]
Harrison et al. [ 87 ] studied how perceptions of social presence in a phishing attack influence the victimization rate. In their experiment, their participants were subject to a simulated phishing attack in which the amount of social presence in the email used was varied. Their results show that richness cues in the email were heuristically rather than systematically processed and that these cues significantly increased the likelihood of successful victimization. The authors, therefore, conclude that the rich information in phishing emails triggered perceptions of social presence and that the resulting heuristic evaluation increased the chances of victimization. Additionally, it appeared that once triggered, the perceived social presence of a phishing email not only reduced the users’ considerations of mediation but also indirectly increased the persuasiveness of the email.
In [ 136 ], Parsons et al. concluded that the participants in their study developed personal approaches to the categorization of emails. They tended to treat emails as if they were important, regardless of their actual legitimacy. For instance, emails from banks or government institutions were more likely to be considered as important and therefore treated as legitimate. Additionally, the authors conclude that the participants were more likely to fall victim to phishing emails if their content threatened a potential financial loss on the part of the receiver. A similar result was presented by Butavicius et al. [ 127 ], who found that the most effective social engineering strategy for influencing a user’s judgment of a link was authority, while the least effective was social proof. Their participants were unable to reliably distinguish between spear phishing and legitimate emails when the emails contained a reference to an authority figure. Thus, the authors concluded that, in terms of judging an email’s legitimacy, the link destinations were unrelated to the actual content of an email. The study conducted by Jansen et al. investigated judgmental heuristics employed by users in evaluating the authenticity of messages [ 129 ]. Their participants’ opinions about the validity of a website relied heavily on the presence of safety signs, such as a closed padlock symbol (presumably, however, they were unaware of how easily such a symbol can be faked). The study conducted by Dhamija et al. confirms this behavior [ 111 ].
In [ 130 ], Parsons et al. attempted to determine the best cues for identifying phishing emails and whether users actually use them. The authors surveyed studies related to this question, compiled a list of cues identified therein, and organized their findings into categories, as shown in Table 6 . Because they found that all of the studies investigated were based on participants self-reporting how they used these cues to distinguish between phishing and genuine emails, the authors performed experiments to measure the impact thereof empirically. They identified content consistency, link legitimacy, email personalization, and spelling as the best indicators. However, their results indicate that users often make their decisions based on poor indicators; for example, their participants were influenced by the visual presentation of the email used. If the phishing email was visually more appealing (e.g., a professional-looking logo was present), they tended to make more accurate decisions concerning its legitimacy compared to emails with a poor visual presentation. Additionally, the authors found that participants were influenced by the urgent tone of an email, as they seemed to perform the worst in that case.
Similarly, Benenson et al. [ 32 ] studied why users click on the links provided in phishing emails. The results indicate the following reasons: 34% of users stated that they opened emails due to curiosity concerning their content—For example, the content may have been related to the actual behavior or activities of the recipient, such as a link to photographs of a party. 27% of users opened emails to determine their validity. 17% of users opened as they claimed to know the sender of the email, even though the addresses were generated with a random name selector. 16% of the participants opened because they trusted the technical solutions in place to keep them safe. Figure 3 shows a tricky combination of content fits actual behavior or activities and curiosity .
The study [ 79 ], which focused on students, found an increased phishing success rate when emails that are as similar as possible to the original were used. Additionally, more users fell victim to phishing when the linked page was an identical clone of the expected original website. According to Afroz et al. [ 124 ], most users will consider a website and will provide the requested information, if what they see does not contradict their expectations. The authors’ analysis revealed that over 90% of users use a website’s appearance as an indication of its authenticity. The goal of an attacker would, therefore, be to design a phishing website in such a way that it is as close in appearance to the original as possible.
There are also works focusing on the features of URLs embedded in phishing emails (see Fig. 7 —items ➃ and ➄ ). Canova et al. [ 128 ] defined multiple categories, each of which includes several URL spoofing tricks; these are listed in Table 7 . Their results indicate that URL categories 1, 2, and 7 were the easiest to identify, where types 5 and 6 were the most difficult to spot and, therefore, the most successful. Subsequently, they published a follow-up study adding the results of a retention test conducted 5 months after the initial training. The attack using well-hidden typos (category 6) was again the most successful, where over 60% of the participants were unable to identify the message as phishing. Furthermore, the authors report that including keywords such as “secure” in an URL and sub-domain tricks (see category 3) confused the participants the most [ 128 ]. Andric et al. [ 79 ] found that users demonstrated superior performance in terms of identifying phishing URLs and fake websites when they knew the correct URL and the protocol used by the original website.
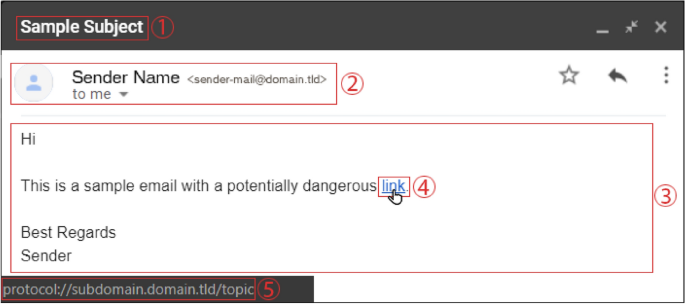
Example of a sample phishing email. ➀ : Email subject, ➁ : sender name and address, ➂ : content area (can contain HTML code), ➃ : link with hidden URL using HTML, ➄ : actual URL of the link shown on mouse-over
There are also contradictory results in the literature concerning the effect of email content and structure. Harrison et al. [ 84 ] designed multiple phishing emails, to which they added typographical/spelling errors. They found that all their efforts went completely unnoticed and subsequently did not affect either processing or susceptibility to phishing.
In order to maximize the effectiveness of a phishing email, we could use a combination of the previously described study approaches. For example, the use of an extremely persuasive topic such as shipping or order , an email that looks identical to a regular email and an URL using spoofing tricks that fall into category 5 or 6 redirecting to a clone of the expected website is promising. Multiple studies found that the success rate of phishing attempts improves when emails that are very similar to the original one are used. However, the study of Harrison et al. [ 84 ] found that spelling errors have no impact. This may be because people do not spot them. According to Rawlinson et al. [ 140 ], the human brain can read words with scrambled characters because it generally processes word features through a classification/identification scheme. The brain can recognize a word as long as the beginning, and the end of the word remains intact, and the middle part of the word still contains the correct letter features, although they can be arranged independently of their correct position. This leads to the assumption that the visual appearance of an email is more important than the words used, with the limitation that the topic and content must still match that expected of an email. To provide a concise overview, Table 8 summarizes all of the findings described in this section.
This category covers the design of learning materials, when or how educational documents are presented to participants, and how a training program should be designed. Potential approaches to education could include courses, repeatedly sending educational material to target users, or attempting actually to phish users and presenting the relevant training material thereafter. The latter method is referred to as embedded training in this work. This section is organized as follows: First, we present results regarding how the training itself should occur (e.g., if courses are a more effective form of training than just providing informative material via email). In the second part, we analyze publications studying the training materials themselves (e.g., how the documents should be structured or whether more graphics should be used than text).
a. Form of the training: Based on their results, Kumaraguru et al. [ 82 ] suggest that users learn more effectively when the training materials are presented after the users have fallen victim to a simulated attack. The authors refer to this educational method as embedded training . Additionally, they also measured the average time the users spent reading the provided training materials. Participants in the embedded training group spent 97 s on average, whereas the non-embedded group spent 37 s. This result is reflected in data collected from recurring phishing tests: The adoption of the embedded approach results in an improved training effect. Al-Daeef et al. [ 23 ] also confirmed this finding by observing that users make better decisions concerning phishing emails after having to experience embedded training. Also, Kumaraguru et al. [ 21 ] did not observe a significant difference in phishing detection performance between the participants receiving non-embedded training and the members of the control group.
Offering personalized training is instrumental in increasing the effectiveness of the anti-phishing training program. The literature survey in [ 24 ] highlights the benefits of ongoing, embedded anti-phishing training for employees as such education will not be as detached from a user’s reality as, for instance, a dedicated course would. Schroeder’s suggestion is to implement training on a per-user basis with different difficulty levels. The author notes that incorporating personalized spaced repetition provides added benefits for employees, as they receive the impression that the training has been customized to their needs. The participants would feel more engaged by the customized materials since they knew that the training was designed to provide them the ability to succeed. Mapping the learning tasks to each level would allow each participant to progress at his or her own pace. Users might stretch themselves to reach a higher level than they would in the absence of a personalized program.
Carella et al. [ 22 ] confirmed that embedded training substantially outperforms no-training and in-class training situations. However, the authors stated that in-class training has the most significant short-term impact. The high short-term training effect of in-class education was also observed by Karumbaiah et al. [ 25 ], who, in their research, concluded that users exposed to a high-quality anti-phishing training video were less likely to click on phishing links during a subsequent 30-min experiment than those exposed to other training methods.
The phishing type against which users should be trained also impacts the effectiveness of embedded training. Caputo et al. [ 55 ] studied embedded training for spear phishing and obtained mixed results. They concluded that the training was not as effective against spear-phishing as it was against general phishing. The authors speculated that the participants might have perceived the provided information as “not credible, relevant or interesting”.
How phishing education is presented to users has a significant impact on how they react to it. Wang et al. [ 102 ] extended an email client with a phishing warning bar, which would warn the user should he or she receive suspicious emails. However, the results showed that many users did not notice the warning sign and fell victim to the phishing attempt. Akhawe et al. [ 121 ] conducted a large-scale study to investigate the impact of warning messages further and found that such messages can indeed be effective in practice. The authors evaluated browser telemetry data obtained from Mozilla and Google and reached the following conclusion: When malware or phishing warnings were shown, only a quarter of the users ignored the warnings and continued to open the website. If, however, the Secure Socket Layer (SSL) warning page was displayed, more than 70% of users clicked through. The authors concluded that the experience of a user for a specific warning message has a significant impact on the click-through rate. According to Engelman et al., such warning messages must be designed such that they actively interrupt the user’s primary task, only pop up if necessary and require the user actually to read the message; besides, to be efficient, they should display clear and understandable choices [ 103 ].
b. Educational material: Kumaraguru et al. investigated whether users provided with text- or comic-based training materials exhibit different learning results in [ 75 ]. The participant group provided with the comic-based materials achieved better results than with standard training methods. The authors subsequently improved their training materials even further by developing a game called “Anti-Phishing Phil”. Their results show that participants who played the game performed better in terms of identifying phishing URLs [ 57 ]. In a similar vein, Sheng et al. [ 30 ] studied and tested several anti-phishing materials, finding that there is no significant difference between the training effect of the materials as long as users are provided with at least one of them. A similar result was obtained by Jensen et al. [ 26 ], who concluded that training materials consisting of only text were as effective as those featuring a text-plus-graphics presentation method. Harrison et al. [ 84 ] suggest focusing the training on “refining the quality of initial attention to the email”, such as by teaching users to focus on a few key elements of an email (e.g., the existence of hyperlinks or verifying the sender’s email address). Greene et al. [ 101 ] analyzed the data of a 4.5-year-long embedded training-based phishing awareness program. They found that the people who clicked on the simulated phishing messages tend to overestimate the technological phishing detection system of their company. Therefore, they advise that companies should consider explicitly informing their employees that no technological solution is completely infallible. Promising training effects were identified in the results obtained by Siadati et al. [ 50 ], who developed a web-based interactive email client in which participants had to identify a certain number of suspicious elements to complete their training.
In their study, Kirlappos and Sasse [ 108 ] proposed that the way in which security education is designed should be revised. Their results show that materials provided to employees are largely ignored because they focus on indicators that users potentially do not understand or trust. Therefore, the authors propose offering different modules when implementing a training program, as they conclude that awareness, education, and training are three distinct steps in improving a user’s security competence.
Zikai et al. show in [ 117 ] an interactive form of awareness training with a role-playing game. In their study, they compared their game to similar approaches and state that users learn the concepts of phishing better with playing their game than watching video material.
The effectiveness of anti-phishing training based on the embedded model has been successfully verified in the past. Ideally, such training should be designed on a per-participant basis as an ongoing process within an organization, starting with an in-class training seminar. Various types of training materials have been investigated, with mixed results being obtained (see Table 9 ), while one of the works considered that providing materials, regardless of their type, was the most important factor [ 30 ].
Knowledge retention
This section covers works that investigate the impact of the anti-phishing training program over time. It presents findings related to the question of whether a single training session is sufficient or whether recurring training sessions at certain frequencies are required to achieve and maintain a decreased likelihood of employees falling victim to phishing attacks.
There are various findings that support the view that an effective anti-phishing training program should consist of multiple recurring training sessions [ 21 , 24 , 55 , 75 ]. However, findings regarding how long participants retain the knowledge obtained during training or how long the intervals before potential re-training sessions should be, differ. On three occasions, Kumaraguru et al. concluded that users can retain learned content for at least 1 week [ 57 , 82 , 109 ]. On a similar time-scale, Jackson et al. [ 110 ] showed that users retained their anti-phishing knowledge up to 16 days after undergoing their first training session. Another study published by these authors titled “School of Phish: A Real-World Evaluation of Anti-Phishing Training”, confirmed knowledge retention even after 28 days [ 75 ].
The considered studies overwhelmingly conclude that training should be designed as an ongoing and integrated process. Employees should be able to train in a way that feels natural for them; for example, training could be integrated into their routine work activities. It was found in [ 23 , 24 ] that, through ongoing anti-phishing training, click rates were reduced from 58 percent to single-digit percentages after the first training iteration. In [ 24 ], Schroeder further advises choosing the training intervals on an individual basis per user depending on his or her educational progress. These intervals should, however, be determined in such a way that they do not annoy employees by resulting in excessively frequent scheduled training sessions; however, each user should be trained at least four times a year. In a similar vein, Canova et al. [ 128 ] found a significant decrease in the performance of the participants in their retention study after 5 months.
As summarized in Table 10 , all of the works considered in this survey agree on the notion that recurring training sessions must be scheduled to ensure that the learned anti-phishing knowledge is not forgotten. Unfortunately, the findings regarding knowledge retention are not as clear. They suggest that the retention period is between 7 days and 5 months. Therefore, one should train all users at least once every 5 months even with an optimistic view on knowledge retention.
Our literature analysis showed that anti-phishing training has a significant impact on user susceptibility to phishing attacks. It is, therefore, evident that any organization should have a valid and well-founded anti-phishing training program. However, a key question lingers: what should such a program look like?
Our comparative analysis of related works showed that the parameters and values listed in Table 11 are reasonably certain to have a positive impact when they are taken into consideration in a program’s design.
Based on these parameters, we first discuss how such training should look alike. After that, we consider the implications for tools that can be used to implement or facilitate anti-phishing training. Finally, we conclude this section with a brief look at the current state of anti-phishing training tools.
Aspects of a well-founded anti-phishing training program
The reviewed body of work leaves little doubt that everyone is susceptible to phishing, to at least some degree. Therefore, every organization should have an anti-phishing training program. A valid and well-founded anti-phishing training program should start with a “kick-off course,” as training sessions organized as courses produce the highest short-term training effect. After this initial step, the participants of the program should be trained through embedded training . However, the reviewed literature suggests (see " Target group impact " section) that user-specific parameters such as age, gender, technical expertise, and personal traits have an impact on phishing susceptibility and on the type of training that yields the best results. A method such as the CRI could be used to determine how much and what type of training an employee needs.
Concerning embedded training, the training material must be displayed as soon as a mistake is made; for example, just after clicking a link in a phishing email. Alternatively, the presentation of the training material could be delayed until some additional steps are taken, for example, after credentials have been entered on a fake company login page. However, these cases are challenging, as, if the user clicks but does not enter his/her credentials, a training action might still be required if the phishing attempt could have been recognized based on the email content and link. The training material itself should provide information on why the user is being presented with that and how he or she could have recognized this instance of a phishing attempt. Moreover, if an employee does not click on the phishing email but does not report it either, he/she should receive training materials on how to report phishing emails and why this step is essential.
As shown in Table 11 , according to the research findings in the literature, the email topics leading to the highest click rates are shipping , orders and received fax . However, this situation does not mean that only such emails should be used. According to the context and training goals, it is also appropriate to use other topics or a mixture thereof, with those that have a higher impact being weighted more than others (see Table 8 ).
Each employee will most certainly have a different knowledge state before training. A possible solution for this problem could be to create multiple difficulty levels and allow users to progress through those individually. These levels would contain different sets of emails and landing pages, with their difficulties being adjusted based on the findings presented in “ Email content and structure ” section. If employees continuously exhibit the correct anti-phishing behavior, we could upgrade them directly to the next level. Alternatively, we could send them an email describing their success and offering the possibility of proceeding to the next level if desired.
To ensure that the gained anti-phishing knowledge is retained, a program should be designed as an ongoing process that is integrated into users’ daily workflow and mimics actual attacks as closely as possible. Each user should be exposed to such training at least once every 5 months, but preferably four times a year. However, as the results regarding the ideal intervals between re-training sessions differ, one could experiment as suggested by Schroeder et al. [ 24 ]: The intervals should be chosen such that the users do not get annoyed with excessively frequent retesting but still fulfill the requirements set by management.
Organizational aspect, perception and ethics
Although our conclusions may give the impression that intensive anti-phishing training should be implemented in any organization, there is also the organizational aspect of anti-phishing training regarding how such training might be perceived/taken up in different organizations.
Since organizations differ in their settings, security, and organizational cultures, the impact of a well-founded training programme may vary across companies. If, for instance, a company with a flat organizational structure and a very liberal work culture implemented embedded anti-phishing training, its employees could perceive the training as an observation tool. In contrast, in a bank, where each action taken by an employee might already be monitored, the likelihood of such impressions might be lower.
In any case, embedded training may increase the pressure on employees as, for various reasons, they may not wish to fail the training procedure. Therefore, they might feel constantly tested or pressured by their employers, which could have an impact on their health and/or work performance. Thus, any security training, including phishing training, should be varied according to the needs, market pressures, modernization goals, prerequisites, and budget of a firm.
Additionally, it is crucial to consider the “security fatigue effect”, referring to the situation in which people (e.g., a company’s employees) become overwhelmed by and tired of the barrage of installed security warnings and regulations [ 141 ]. They are basically “drowned” in the ongoing flow of advice concerning how they should stay safe and keep constantly alert. With regards to anti-phishing training, each company has to consider for itself whether its employees are able to handle additional training or whether they would become overwhelmed. If, for example, a company already has security training programs in place, adding an anti-phishing training program might prove counterproductive in terms of security fatigue, and the desired security improvement effect might therefore not materialize.
Psychological aspects of training design
The psychology of end-users, the subjects to be trained to achieve higher phishing-awareness, and attackers should be considered when devising effective anti-phishing training programs [ 142 , 143 ].
Regarding the end-users, the literature review in " Literature analysis " section provides ample evidence that the exploitation of peculiarities of the human psyche is an important factor for a successful phishing attack. Examples of such peculiarities are the almost blind trust in authority figures [ 127 ] and security symbols [ 129 ] or the lower attention to phishing in emails with an urgent tone [ 130 ]. One way to address this is to design training programs that do not only teach users how to recognize phishing emails but aim at altering the user’s problematic cognitive processes. Other end-user related psychological aspects are the different perception of training if it is detached from the user’s reality [ 24 ] or the personal relevance of the training material. The former can be addressed with embedded training and the latter by adjusting the training method and material to different psychological profiles. However, the reviewed literature does not offer much guidance on how these could be done.
Regarding the attackers, a better understanding of the psychology of attackers is crucial in modeling adversary behavior and identifying the implicit factors that determine how deception and phishing strategies are employed in phishing emails in the first place [ 74 , 144 ]. Currently, the psychology of criminal behaviors is usually neglected in the field of cybersecurity [ 143 ]. Nevertheless, target-adversary interactions and how they are driven as part of adversary strategies are important in facilitating realistic phishing simulations and, consequently, training tools. Therefore, researching the application and exploitation of psychological aspects in the design and development of anti-phishing training seems promising.
Implications for anti-phishing training tools
To achieve the training objectives described above, the proposed parameters in Table 11 should be reflected in the design and capabilities of anti-phishing training tools. In addition, those tools should entail practical functions which enable the exploitation of these findings. More specifically, such tools should consider the following aspects:
Progression system: As each user must have the ability to progress at his or her own pace, the tool has to support some level-based progression system. The user is to be moved to a higher level automatically as soon as he or she has mastered the current one. Similarly, he or she could be placed at a lower level should he or she continuously fail to identify attacks at the present level of difficulty. For initial level positioning and training selection, the system could implement the CRI survey questions [ 80 ] and apply the algorithm developed by Vishwanath et al. to identify the appropriate training focus. Furthermore, to identify personality traits and cognitive processes that potentially amplify a user’s susceptibility to phishing, a generic psychological questionnaire could be used. The level and progression of such users could then be adjusted according to the impact severity of their traits.
Emails: As users should be exposed to simulated phishing attacks of different difficulties, email templates must be assignable to levels, or the tool should have a mechanism by which to automatically adjust the given template to a specific level. Such adjustments might include changing the URLs used, adding safety signs to the content like a closed padlock [ 129 ] or tweaking the other parameters described in " Email content and structure " section. Another desirable automation is to mutate the URLs used in a campaign to reflect different URL categories (see Table 6 ). To minimize the administrative efforts required, the system should be able to autonomously manage the URLs on the company’s internal Domain Name System (DNS) server.
To further reduce the work time that an administrator has to devote to creating templates, a tool could additionally offer community template pools that enable users to exchange templates. This solution would allow it to exploit the power of numbers (i.e., to offer support mechanisms by which to share the workload among the members of a community). Another approach to improve efficiency is to share the load to create email templates for typical user groups in the academic community. However, a major challenge is to determine who should operate and manage this platform, as well as how quality control for such a collaborative infrastructure should be implemented. Similar to the personality trait-based adjustments made in other parts of the training design, the emails should be adjusted based on the user’s specific traits. For example, people who tend to overlook details can be trained with emails that are only slightly different from genuine messages.
Automatic population of user database: The system should feature an active connection to the central employee database of the company. This allows the automatic population of users during deployment or in the event of changes due to new requirements or role changes. The automatic inclusion of new employees is a particularly important requirement in terms of addressing vulnerabilities as fast as possible.
Feedback pages: The feedback page has to be adjusted according to the level a user is currently on. This means that it has to match the difficulty level of the simulated phishing email sent to that user. For example, on easier levels, it should directly show the user how he or she could have identified the phishing email. For advanced levels, first, a fake website with a login form could be displayed; then, if the user enters his or her login details, the feedback page with the training material could be displayed. If information about the personality traits of the users is available, the system could display feedback pages that are more suitable for a specific user’s cognitive processing and the ability to assimilate information. For instance, if a user reacts better to graphical content, comic-based feedback pages could be shown.
Retraining system: To support the knowledge retention of employees, the tool should automatically schedule retraining sessions for all users. This should be done in a way that depends upon the user’s current level, and it should feature some time randomization to prevent, for example, having all the phishing emails sent at 8 a.m. Additionally, similar to the progression system , if a generic psychological questionnaire is administered before the start of the training, the retraining-scheduling algorithm could take the user’s psychological and cognitive traits into account as well. For instance, the retraining interval could be longer for users who exhibit traits that result in lower susceptibility.
Phishing email reporting system: If users spot a simulated phishing email in their inboxes, they must be provided with the ability to report this email as an instance of phishing. To support the principle of embedded training, which proposes training users in their working environments, this should be the same mechanism employees use to report real phishing threats. One such mechanism is forwarding the email to a special company email address; another is clicking on a “report email as phishing” button in the email client. Since offering the latter has become quite common in many email clients (e.g., the “Report phishing” drop-down menu option in Gmail, the “Report Message” add-in button for Microsoft Outlook or the “Report Spam” add-on for Thunderbird), the effort required to implement a reporting mechanism and integrate it into the daily routine of a company or its employees is probably acceptable. If a user neither falls for the phishing email nor reports it within the first 24 h, it is likely that it was missed, ignored, or not processed by the user, due to reasons such as being out of the office or having a day off. The period of 24 hours has been reported by [ 75 ] and confirmed by the observation made by Mohebzada et al. that many users fell for their phishing attempt even though their campaign was only active for 18 h. The results of [ 48 , 75 ] are summarized in Fig. 8 .
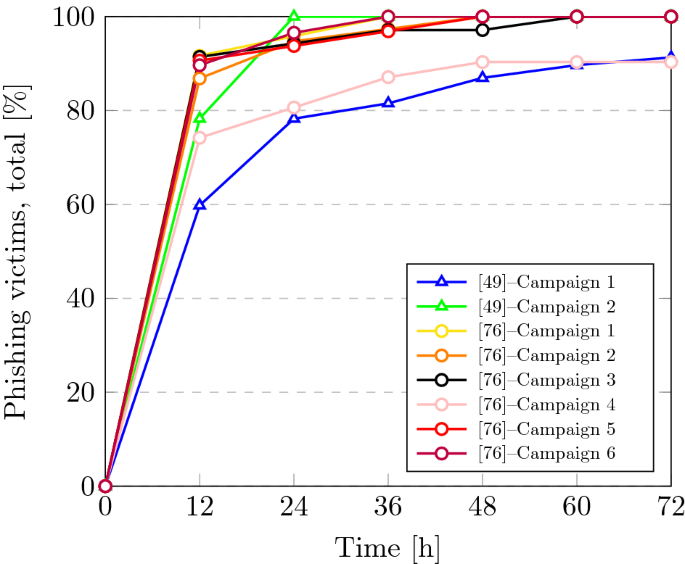
Cumulative percentage in hours of all victims clicking on a phishing link after receiving the phishing email. Each line shows the data for an independent phishing experiment reported in publication X, which is cited as [X]
However, one challenge related to such reporting systems is analyzing the reported emails and providing feedback. If a company does not have the staff and processes in place to react to such reports and provide feedback, employees might lose interest in reporting phishing emails [ 138 ]. This lack of interest would, in turn, make measuring the impact of training efforts difficult. While there is some practical advice available from renowned sources (e.g., from the SANS Institute [ 145 ]) on how to design a good reporting process, it remains unclear which advice is backed by science and which is not. A literature review with a focus on the design of such a reporting system would be needed to shed more light on this question.
Privacy: Another requirement is the support of adequate privacy features. Although they are not related to the core performance of an anti-phishing training effort, such features are crucial for any practical tool to protect its user’s privacy. To this end, statistics and tracking mechanisms should work with pseudonyms. Structural measures such as the isolation of analytics from the sending component in these tools are also necessary. Different aggregation and anonymization schemes for creating reports, such as k-anonymity and differential privacy, should be integrated into the tool [ 146 ].
Automated optimization of training parameters: Using data from multiple institutions, companies and sectors may also provide opportunities for synergistic gains, as analyzing the impact of different factors on training effectiveness could be made more streamlined and generic. Data sharing among stakeholders enables large-scale and long-term analysis with which the impact of different factors on training effectiveness can be measured. The results could then be used to automatically and continuously fine-tune the training parameters of the participating stakeholders.
Anti-phishing training tools and available features
There are intrinsic links among the factors that determine the success of a phishing attack, the effectiveness of anti-phishing training and the construction and operation of an anti-phishing training tool. To get an idea whether or not today’s anti-phishing training tools come with the functionality required to implement a training as outlined in the previous two subsections, we searched the Internet for such tools. More specifically, for each desired functionality or feature (e.g., a level-based approach to training where the training level is automatically determined and adjusted based on the user’s feedback), we attempted to identify at least one commercial or non-commercial tool that offered it. In summary, we found that the currently available tools lack at least one of the desired features. However, since our findings are based on information that could be found using search engines and/or by browsing the respective webpages of each product only, we might have overlooked tools for which this information is not available through these channels. A more detailed summary of our most important finding regarding the aspects discussed in the vious subsection can be found below.
Progression system: We could not identify any tool that supports an individualized automated user progress tracking and level system. Automated tracking and modification of training intensity based on user feedback, personality traits, psychological processes, and progress have not been implemented in any anti-phishing training tool. Furthermore, scientific algorithms that could help to select targets and/or determine why an employee fails to identify phishing threats (e.g., the CRI) have not been implemented.
Emails: While most of the available tools support template mechanisms, they all lack the ability to categorize templates based on detection difficulty. Additionally, none offers a mechanism that can automatically alter a template to increase or reduce its detection difficulty. The shortcomings of existing tools include the lack of functionality by which to manage the URLs automatically used in training emails and mutations thereof in a company’s DNS infrastructure. Some tools ship with templates mimicking emails of well-known Internet companies, such as Google or Amazon. Others offer a version of a template exchange platform based on Github, but we did not identify a tool with a directly-integrated platform usable for everyone.
Automatic population of user database: Most available tools support manual user imports, for instance via comma-separated values (csv) files or through a Lightweight Directory Access Protocol (LDAP) connection. These features must be extended to automatically pull new users from an organization’s central user database and start their training.
Feedback pages: Most tools offer the ability to upload HTML content that will be presented to the user when he or she clicks on the link in the phishing email. This mechanism could be used to upload educational material. However, as most tools are using campaigns to send out phishing emails, such a page can only be defined on the campaign level. An ideal solution would require individual landing pages matching the sent phishing email and therefore matching the level a user is currently on; in addition, these landing pages should be displayed in a form that is adjusted to a user’s psychological and personality traits. We could not find any available tool that offered such a feature.
Retraining system: As most tools use a campaign system, retraining cannot be applied as proposed in our analysis. After each campaign, an administrator would have to analyze the results of each user and manually schedule the follow-up training sessions.
Phishing email reporting system: Some of the tools we looked at offer a way for users to report an email as an instance of phishing. However, this mechanism is usually connected to the anti-phishing training system only and does not relay information about real threats to the company’s email filtering solution.
Automated optimization of training parameters: Most tools assess and create reports on the performance of users and create a report about it. However, we could not identify any tool that continuously analyzes the impact of training parameters, such as training frequency or email types, on training effectiveness. Therefore, we could not find any available tool which makes recommendations on how to modify the training parameters for enhancing the training gains.
Phishing is a growing security issue for both institutions and individuals. Although there are various mitigation techniques, proactive anti-phishing training is an important building block of any multi-level phishing defense strategy. In this paper, we identified various factors that influence the effectiveness of such training efforts. Building on our analysis of the research literature, we outlined how an effective anti-phishing training program should be designed and implemented. Based on the weak coherence between our empirical findings and currently used anti-phishing training solutions, we believe that this contribution addresses a crucial technical gap.
In our discussion, we outlined several implications of our findings concerning the design and capabilities of anti-phishing tools. Significant design aspects and capabilities in this regard are automated operation and individualization with continuous assessment/optimization of the configuration of training parameters. This is crucial, as our literature analysis showed that research results concerning some of the parameters are inconclusive or even contradictory, indicating that these parameters require further investigation. Moreover, an effective anti-phishing training tool should have community functions to facilitate cooperation and load-balancing among disparate anti-phishing efforts (e.g., shared email templates or co-designed training curricula.)
Based on our survey and analysis of relevant sources in the technical literature, we found that, despite the various advanced capabilities that tools currently available in the anti-phishing domain offer, such tools only support a limited subset of the potential factors identified as necessary to yield the desired training effects. Therefore, we believe that our work does have a high practical value in terms of contributing to the development of more complete training solutions with a more significant impact to reduce phishing susceptibility on the part of users. We are convinced that greater awareness of phishing techniques and means of addressing them increases overall security and peace of mind.
Future research directions
Our survey points out that two key research directions: First, the factors on anti-phishing training effectiveness deserve further research with more extensive and diverse experiments in higher numbers focusing on the gray areas, i.e., where contradictory results are available in the current body of work. Second, phishing awareness training, as done today, has several limitations. First of all, this includes a lack of consideration of scientific results that are available in the scientific outputs. Furthermore, a lack of individualization of training limits the efficiency of training. Thus, how to customize training based on trainee profiles is another research topic.
Once the training is done, another requirement becomes evident, leading to another research direction: how to measure the training effect. This is accompanied by the lack of ensuring long-term training benefits. More studies are necessary to show the long-term effects of anti-phishing training and make the results of these studies comparable. To this end, we will conduct further iterations of our survey in the future to see how the research in this field has progressed over the years and to compare new findings.
Availability of data and materials
Not applicable.
IEEExplore: https://ieeexplore.ieee.org .
ACM Digital Library: https://dl.acm.org .
ScienceDirect: https://www.sciencedirect.com .
Wiley Online Library: https://onlinelibrary.wiley.com .
GS: https://scholar.google.com .
Infosec: phishing definition, prevention, and examples (2019). https://resources.infosecinstitute.com/category/enterprise/phishing/
Bissell K, LaSalle RM, Cin PD (2019) Accenture’s ninth annual cost of cybercrime study: unlocking the value of improved cybersecurity protection. https://www.accenture.com/us-en/insights/security/cost-cybercrime-study
Nero PJ, Wardman B, Copes H, Warner G (2011) Phishing: crime that pays. In: 2011 eCrime researchers summit, pp 1–10
Bisson D (2015) Sony hackers used phishing emails to breach company networks. https://www.tripwire.com/state-of-security/latest-security-news/sony-hackers-used-phishing-emails-to-breach-company-networks/ . Accessed 26 Dec 2017
Sanger DE, Benner K (2018) U.S. accuses North Korea of plot to hurt economy as spy is charged in Sony hack. The New York Times, Chap, U.S. Accessed 29 Oct 2018
Franceschi-Bicchierai L (2016) Russian hackers launch targeted cyberattacks hours after trump’s win. https://motherboard.vice.com/en_us/article/nz79gb/russian-hackers-launch-targeted-cyberattacks-hours-after-trumps-win . Accessed 26 Dec 2017
Aaron G (2020) APWG phishing activity trends 4th quarter report 2019. https://docs.apwg.org/reports/apwg_trends_report_q4_2019.pdf . Accessed 04 Jan 2020
Aaron G (2019) APWG phishing activity trends 4th quarter report 2018. https://docs.apwg.org//reports/apwg_trends_report_q4_2018.pdf . Accessed 04 Jan 2020
Aaron G (2018) APWG phishing activity trends 4th quarter report 2017. https://docs.apwg.org//reports/apwg_trends_report_q4_2017.pdf . Accessed 04 Jan 2020
Aaron G (2017) APWG phishing activity trends 4th quarter report 2016. https://docs.apwg.org//reports/apwg_trends_report_q4_2016.pdf . Accessed 04 Jan 2020
Hong J (2012) The state of phishing attacks. Commun ACM 55(1):74–81
Google Scholar
Gorman S (2013) Annual U.S. cybercrime costs estimated at \$100 billion. Wall Street J. Accessed 22 Mar 2017
Morrow S (2019) Juniper research—the future of cybercrime & security research report. https://www.juniperresearch.com/document-library/white-papers/the-future-of-cybercrime-white-paper
Cybersecurity ventures: 2019 official annual cybercrime report (2019). https://www.herjavecgroup.com/the-2019-official-annual-cybercrime-report/
CNBC: Xoom says $30.8 mln transferred fraudulently to overseas accounts (2015). https://www.cnbc.com/2015/01/06/xoom-says-308-mln-transferred-fraudulently-to-overseas-accounts.html
Dou Z, Khalil I, Khreishah A, Al-Fuqaha A, Guizani M (2017) Systematization of knowledge (SoK): a systematic review of software-based web phishing detection. IEEE Commun Surv Tutor 19(4):2797–2819
Gupta BB, Tewari A, Jain AK, Agrawal DP (2017) Fighting against phishing attacks: state of the art and future challenges. Neural Comput Appl 28(12):3629–3654
Deeb Al-Mo AA, Wan T-C, Tat-Chee K, Altaher A, Ramadass S, Manasrah A, Melhiml LB, Anbar M (2011) An online model on evolving phishing e-mail detection and classification method. J Appl Sci 11(18):3301–3307
Angelov P, Filev DP, Kasabov N (2010) Evolving intelligent systems: methodology and applications. Wiley, Hoboken
Fette I, Sadeh N, Tomasic A (2007) Learning to detect phishing emails. In: Proceedings of the 16th international conference on world wide web. WWW ’07, ACM, New York, pp 649–656
Kumaraguru P, Rhee Y, Sheng S, Hasan S, Acquisti A, Cranor LF, Hong J (2007) Getting users to pay attention to anti-phishing education: evaluation of retention and transfer. In: Proceedings of the anti-phishing working groups 2nd annual eCrime researchers summit, ACM, Pittsburgh, Pennsylvania, pp 70–81
Carella A, Kotsoev M, Truta TM (2017) Impact of security awareness training on phishing click-through rates. In: 2017 IEEE international conference on Big Data (Big Data), pp 4458–4466
Al-Daeef MM, Basir N, Hukins M (2017) Security awareness training: a review. In: Proceedings of the world congress on engineering 2017, vol 1
Schroeder J (2017) Persistent training. In: Advanced persistent training, Apress, Berkeley, pp 25–32
Karumbaiah S, Wright RT, Durcikova A, Jensen ML (2016) Phishing training: a preliminary look at the effects of different types of training. WISP 2016 proceedings. 11
Jensen ML, Dinger M, Wright RT, Thatcher JB (2017) Training to mitigate phishing attacks using mindfulness techniques. J Manage Inf Syst 34(2):597–626
SANS: SANS security awareness—phishing (2019). https://www.sans.org/security-awareness-training/ouch-newsletter/2015/phishing
MITRE: MITRE attack framework—initial access (2019). https://attack.mitre.org/tactics/TA0001/
Yue C, Wang H (2010) Bogusbiter: a transparent protection against phishing attacks. ACM Trans Internet Technol 10(2):6–1631
Sheng S, Holbrook M, Kumaraguru P, Cranor LF, Downs J (2010) Who falls for phish?: a demographic analysis of phishing susceptibility and effectiveness of interventions. In: Proceedings of the SIGCHI conference on human factors in computing systems, pp 373–382
Seals T (2017) Cost of user security training tops \$290K per year. Infosecurity magazine. https://www.infosecurity-magazine.com/news/cost-of-user-security-training . Accessed 15 Sept 2017
Benenson Z, Gassmann F, Landwirth R (2016) Exploiting curiosity and context: how to make people click on a dangerous link despite their security awareness. BlackHat USA
Stembert N, Padmos A, Bargh MS, Choenni S, Jansen F (2015) A study of preventing email (Spear) phishing by enabling human intelligence. In: 2015 European intelligence and security informatics conference, pp 113–120
Vrbančič G, Fister I, Podgorelec V (2018) Swarm intelligence approaches for parameter setting of deep learning neural network: case study on phishing websites classification. In: Proceedings of the 8th international conference on web intelligence, mining and semantics. Association for Computing Machinery, New York. https://doi.org/10.1145/3227609.3227655
Tian K, Jan STK, Hu H, Yao D, Wang G (2018) Needle in a haystack: tracking down elite phishing domains in the wild. In: Proceedings of the internet measurement conference 2018. IMC ’18, Association for Computing Machinery, New York, pp 429–442. https://doi.org/10.1145/3278532.3278569
Sirigineedi SS, Soni J, Upadhyay H (2020) Learning-based models to detect runtime phishing activities using urls. In: Proceedings of the 2020 the 4th international conference on compute and data analysis. ICCDA 2020, Association for Computing Machinery, New York, pp 102–106. https://doi.org/10.1145/3388142.3388170
Tyagi I, Shad J, Sharma S, Gaur S, Kaur G (2018) A novel machine learning approach to detect phishing websites. In: 2018 5th international conference on signal processing and integrated networks (SPIN), pp 425–430
Sahingoz OK, Buber E, Demir O, Diri B (2019) Machine learning based phishing detection from urls. Expert Syst Appl 117:345–357. https://doi.org/10.1016/j.eswa.2018.09.029
Article Google Scholar
Bahnsen Alejandro C, Ivan Torroledo LDC, Villegas S (2018) Deepphish: simulating malicious ai. In: 2018 APWG symposium on electronic crime research (eCrime), pp 1–8
Pham C, Nguyen LAT, Tran NH, Huh E, Hong CS (2018) Phishing-aware: a neuro-fuzzy approach for anti-phishing on fog networks. IEEE Trans Netw Serv Manage 15(3):1076–1089
Mayhorn CB, Nyeste PG (2012) Training users to counteract phishing. Work 41(Supplement 1):3549–3552
Alnajim A, Munro M (2009) An approach to the implementation of the anti-phishing tool for phishing websites detection. In: 2009 international conference on intelligent networking and collaborative systems, IEEE, pp 105–112
Liu D, Wang W, Wang Y, Tan Y (2019) Phishledger: a decentralized phishing data sharing mechanism. In: Proceedings of the 2019 international electronics communication conference. IECC ’19. Association for Computing Machinery, New York, pp 84–89. https://doi.org/10.1145/3343147.3343154
Hutchings A, Clayton R, Anderson R (2016) Taking down websites to prevent crime. In: 2016 APWG symposium on electronic crime research (eCrime), pp 1–10
Whitman ME (2003) Enemy at the gate: threats to information security. Commun ACM 46(8):91–95
Google: about Google Scholar (2019). https://scholar.google.ch/intl/en/scholar/about.html . Accessed 24 Apr 2019
Harzing A-W (2019) Publish or Perish. https://harzing.com/resources/publish-or-perish . Accessed 24 Apr 2019
Mohebzada JG, Zarka AE, Bhojani AH, Darwish A (2012) Phishing in a university community: two large scale phishing experiments. In: 2012 international conference on innovations in information technology (IIT), pp 249–254
Leukfeldt E (2014) Phishing for suitable targets in the netherlands: routine activity theory and phishing victimization. Cyberpsychol Behav Soc Netw 17:551–555
Siadati H, Palka S, Siegel A, McCoy D (2017) Measuring the effectiveness of embedded phishing exercises. In: 10th USENIX workshop on cyber security experimentation and test (CSET 17). https://www.usenix.org/node/205854
Gordon WJ, Wright A, Aiyagari R, Corbo L, Glynn RJ, Kadakia J, Kufahl J, Mazzone C, Noga J, Parkulo M, Sanford B, Scheib P, Landman AB (2019) Assessment of employee susceptibility to phishing attacks at us health care institutions. JAMA Netw Open 2(3):190393–190393. https://doi.org/10.1001/jamanetworkopen.2019.0393
Taib R, Yu K, Berkovsky S, Wiggins M, Bayl-Smith P (2019) Social engineering and organisational dependencies in phishing attacks. In: Lamas D, Loizides F, Nacke L, Petrie H, Winckler M, Zaphiris P (eds) Human–computer interaction—INTERACT 2019. Springer, Cham, pp 564–584
Baillon A, de Bruin J, Emirmahmutoglu A, van de Veer E, van Dijk B (2019) Informing, simulating experience, or both: a field experiment on phishing risks. PLoS ONE 14(12):1–15. https://doi.org/10.1371/journal.pone.0224216
Benenson Z, Gassmann F, Landwirth R (2017) Unpacking spear phishing susceptibility. In: Brenner M, Rohloff K, Bonneau J, Miller A, Ryan PYA, Teague V, Bracciali A, Sala M, Pintore F, Jakobsson M (eds) Financial cryptography and data security. Lecture notes in computer science. Springer, Cham, pp 610–627
Caputo DD, Pfleeger SL, Freeman JD, Johnson ME (2014) Going spear phishing: exploring embedded training and awareness. IEEE Secur Priv 12(1):28–38
Jagatic TN, Johnson NA, Jakobsson M, Menczer F (2007) Social phishing. Commun ACM 50(10):94–100
Kumaraguru P, Sheng S, Acquisti A, Cranor LF, Hong J (2010) Teaching Johnny not to fall for phish. ACM Trans Internet Technol 10(2):7–1731
Dodge RC, Carver C, Ferguson AJ (2007) Phishing for user security awareness. Comput Secur 26(1):73–80
Li W, Lee J, Purl J, Greitzer F, Yousefi B, Laskey K (2020) Experimental investigation of demographic factors related to phishing susceptibility. In: Hawaii international conference on system sciences. http://hdl.handle.net/10125/64015 . Accessed 01 Apr 2020
Burns AJ, Johnson ME, Caputo DD (2019) Spear phishing in a barrel: insights from a targeted phishing campaign. J Organ Comput Electron Commer 29(1):24–39. https://doi.org/10.1080/10919392.2019.1552745
Gordon WJ, Wright A, Glynn RJ, Kadakia J, Mazzone C, Leinbach E, Landman A (2019) Evaluation of a mandatory phishing training program for high-risk employees at a US healthcare system. J Am Med Inform Assoc 26(6):547–552
Steves, MP, Greene KK, Theofanos MF (2019) A phish scale: rating human phishing message detection difficulty. In: Workshop on usable security (USEC)
Ikhsan MG, Ramli K (2019) Measuring the information security awareness level of government employees through phishing assessment. In: 2019 34th international technical conference on circuits/systems, computers and communications (ITC-CSCC)
Higashino M, Kawato T, Ohmori M, Kawamura T (2019) An anti-phishing training system for security awareness and education considering prevention of information leakage. In: 2019 5th international conference on information management (ICIM), pp 82–86
Rastenis J, Ramanauskaitė S, Janulevičius J, Čenys A (2019) Credulity to phishing attacks: Aareal-world study of personnel with higher education. In: 2019 Open conference of electrical, electronic and information sciences (eStream)
Diaz A, Sherman AT, Joshi A (2020) Phishing in an academic community: a study of user susceptibility and behavior. Cryptologia 44(1):53–67
Kim B, Lee D-Y, Kim B (2019) Deterrent effects of punishment and training on insider security threats: a field experiment on phishing attacks. Behav Inf Technol 0(0), 1–20
Canfield CI, Fischhoff B, Davis A (2019) Better beware: comparing metacognition for phishing and legitimate emails. Metacogn Learn 14(3):343–362
Xiong A, Proctor RW, Yang W, Li N (2019) Embedding training within warnings improves skills of identifying phishing webpages. Hum Factors 61(4):577–595
Flores WR, Holm H, Nohlberg M, Ekstedt M (2015) Investigating personal determinants of phishing and the effect of national culture. Inf Comput Secur 23(2):178–199
Dodge R, Coronges K, Rovira E (2012) Empirical benefits of training to phishing susceptibility. In: Gritzalis D, Furnell S, Theoharidou M (eds) Information security and privacy research, vol 376. Springer, Berlin, pp 457–464
Moody G, Galletta D, Walker J, Dunn B (2011) Which phish get caught? An exploratory study of individual susceptibility to phishing. In: International conference on information systems 2011, ICIS 2011, vol 3
Workman M (2008) Wisecrackers: a theory-grounded investigation of phishing and pretext social engineering threats to information security. J Am Soc Inf Sci Technol 59(4):662–674
Abbasi A, Zahedi FM, Chen Y (2016) Phishing susceptibility: the good, the bad, and the ugly. In: 2016 IEEE conference on intelligence and security informatics (ISI), pp 169–174
Kumaraguru P, Cranshaw J, Acquisti A, Cranor L, Hong J, Blair MA, Pham T (2009) School of phish: a real-world evaluation of anti-phishing training. In: Proceedings of the 5th symposium on usable privacy and security. SOUPS ’09, ACM, New York, NY, USA, pp 3–1312
Orunsolu AA, Sodiya AS, Akinwale AT, Olajuwon BI, Alaran MA, Bamgboye OO, Afolabi OA (2017) An empirical evaluation of security tips in phishing prevention: a case study of Nigerian banks. Int J Electron Inf Eng 6(1):25–39
Steyn T, Kruger HA, Drevin L (2007) Identity theft—empirical evidence from a phishing exercise. In: Venter H, Eloff M, Labuschagne L, Eloff J, von Solms R (eds) New approaches for security, privacy and trust in complex environments. Springer, Boston, pp 193–203
Iuga C, Nurse JRC, Erola A (2016) Baiting the hook: factors impacting susceptibility to phishing attacks. Hum-centric Comput Inf Sci 6(1):8
Andrić J, Oreški D, Kišasondi T (2016) Analysis of phishing attacks against students. In: 2016 39th international convention on information and communication technology, electronics and microelectronics (MIPRO), pp 1423–1429
Vishwanath A, Harrison B, Ng YJ (2016) Suspicion, cognition, and automaticity model of phishing susceptibility. Commun Res 45(8):1146–1166
Vishwanath A, Herath T, Chen R, Wang J, Rao HR (2011) Why do people get phished? Testing individual differences in phishing vulnerability within an integrated, information processing model. Decis Support Syst 51(3):576–586
Kumaraguru P, Sheng S, Acquisti A, Cranor LF, Hong J (2008) Lessons from a real world evaluation of anti-phishing training. In: 2008 eCrime researchers summit, pp 1–12
Alseadoon I, Chan T, Foo E, Nieto J (2012) Who is more susceptible to phishing emails? A Saudi Arabian study. In: ACIS 2012: proceedings of the 23rd Australasian conference on information systems
Harrison B, Svetieva E, Vishwanath A (2016) Individual processing of phishing emails: how attention and elaboration protect against phishing. Online Inf Rev 40(2):265–281
Vishwanath A (2015) Examining the distinct antecedents of e-mail habits and its influence on the outcomes of a phishing attack. J Comput Mediat Commun 20(5):570–584
Canfield CI, Fischhoff B, Davis A (2016) Quantifying phishing susceptibility for detection and behavior decisions. Hum Factors 58(8):1158–1172
Harrison B, Vishwanath A, Ng YJ, Rao R (2015) Examining the impact of presence on individual phishing victimization. In: 2015 48th Hawaii international conference on system sciences, pp 3483–3489
Parsons K, McCormac A, Pattinson M, Butavicius M, Jerram C (2013) Phishing for the truth: a scenario-based experiment of users’ behavioural response to emails. In: Janczewski LJ, Wolfe HB, Shenoi S (eds) Security and privacy protection in information processing systems. Springer, Berlin, pp 366–378
Petelka J, Zou Y, Schaub F (2019) Put your warning where your link is: improving and evaluating email phishing warnings. In: Proceedings of the 2019 CHI conference on human factors in computing systems. CHI ’19. Association for computing machinery, New York, NY, USA
Tian CA, Jensen ML (2019) Effects of emotional appeals on phishing susceptibility. In: Proceedings of the 14th Pre-ICIS workshop on information security and privacy
Lee HS, Jeong DN, Lee SI, Lee SH, Kim KH, Lee HY, Cho HJ, Choi SW, Ko T (2019) Result and effectiveness of malicious e-mail response training in a hospital. Stud Health Technol Inform. https://doi.org/10.3233/shti190732
Hermogenes MGG, Capariño ET (2019) Evaluating internet security awareness and practices of bulsu-sc students. In: Proceedings of the 2019 7th international conference on information and education technology. ICIET 2019, Association for Computing Machinery, New York, NY, pp 62–66
Anawar S, Kunasegaran DL, Mas’ud MZ, Zakaria NA (2019) Analysis of phishing susceptibility in a workplace: a big-five personality perspectives. J Eng Sci Technol 14(5):2865–2882
Musuva P, Chepken C, Getao K (2019) A naturalistic methodology for assessing susceptibility to social engineering through phishing. Afr J Inf Syst 11:2
Jones HS, Towse JN, Race N, Harrison T (2019) Email fraud: the search for psychological predictors of susceptibility. PLoS ONE 14(1):0209684–0209684. https://doi.org/10.1371/journal.pone.0209684
Williams EJ, Polage D (2019) How persuasive is phishing email? the role of authentic design, influence and current events in email judgements. Behav Inf Technol 38(2):184–197
Shakela V, Jazri H (2019) Assessment of spear phishing user experience and awareness: an evaluation framework model of spear phishing exposure level (spel) in the namibian financial industry. In: 2019 international conference on advances in big data, computing and data communication systems (icABCD), pp 1–5
Lin T, Capecci DE, Ellis DM, Rocha HA, Dommaraju S, Oliveira DS, Ebner NC (2019) Susceptibility to spear-phishing emails: effects of internet user demographics and email content. ACM Trans Comput Hum Interact 26(5):1–28
Zielinska OA, Tembe R, Hong KW, Ge X, Murphy-Hill E, Mayhorn CB (2014) One phish, two phish, how to avoid the internet phish: analysis of training strategies to detect phishing emails. Proc Hum Factors Ergon Soc Annu Meet 58(1):1466–1470
Flores WR, Holm H, Svensson G, Ericsson G (2014) Using phishing experiments and scenario-based surveys to understand security behaviours in practice. Inf Manage Comput Secur 22(4):393–406
Greene K, Steves M, Theofanos M, Kostick J (2018) User context: an explanatory variable in phishing susceptibility. In: Proceedings 2018 workshop on usable security. Internet Society, San Diego, CA
Yang W, Xiong A, Chen J, Proctor RW, Li N (2017) Use of phishing training to improve security warning compliance: evidence from a field experiment. In: Proceedings of the hot topics in science of security: symposium and bootcamp. HoTSoS. ACM, New York, pp 52–61
Egelman S, Cranor L, Hong J (2008) You’ve been warned: an empirical study of the effectiveness of web browser phishing warnings. In: SIGCHI conference on human factors in computing systems, pp 1065–1074
Neupane A, Rahman ML, Saxena N, Hirshfield L (2015) A multi-modal neuro-physiological study of phishing detection and malware warnings. In: Proceedings of the 22nd ACM SIGSAC conference on computer and communications security—CCS ’15. ACM Press, Denver, Colorado, pp 479–491
Hong KW, Kelley CM, Tembe R, Murphy-Hill E, Mayhorn CB (2013) Keeping up with the joneses: assessing phishing susceptibility in an email task. Proc Hum Factors Ergon Soc Annu Meet 57(1):1012–1016
Welk AK, Hong KW, Zielinska OA, Tembe R, Murphy-Hill E, Mayhorn CB (2015) Will the “phisher-men” reel you in?: assessing individual differences in a phishing detection task. Int J Cyber Behav Psychol Learn 5(4):1–17
Halevi T, Memon N, Nov O (2015) Spear-phishing in the wild: a real-world study of personality, phishing self-efficacy and vulnerability to spear-phishing attacks. SSRN Electron J. Accessed 29 Apr 2019
Kirlappos I, Sasse MA (2012) Security education against phishing: a modest proposal for a major rethink. IEEE Secur Priv 10(2):24–32
Kumaraguru P, Rhee Y, Acquisti A, Cranor LF, Hong J, Nunge E (2007) Protecting people from phishing: the design and evaluation of an embedded training email system. In: Proceedings of the SIGCHI conference on human factors in computing systems, pp 905–914
Jackson C, Simon D, Tan D, Barth A (2017) An evaluation of extended validation and picture-in-picture phishing attacks. Microsoft Research (2007). Accessed 19 Dec 2017
Dhamija R, Tygar JD, Hearst M (2006) Why phishing works. In: Proceedings of the SIGCHI conference on human factors in computing systems. CHI ’06, ACM, New York, pp 581–590
Arachchilage NAG User-centred security education: a game design to thwart phishing attacks. arXiv:1511.03459 [cs]. Accessed 29 Apr 2019
Lemay DJ, Basnet RB, Doleck T (2020) Examining the relationship between threat and coping appraisal in phishing detection among college students. J Internet Serv Inf Secur. 10(1):38–49
Bin Othman Mustafa MS, Kabir MN, Ernawan F, Jing W (2019) An enhanced model for increasing awareness of vocational students against phishing attacks. In: 2019 IEEE international conference on automatic control and intelligent systems (I2CACIS), pp 10–14
Li Y, Xiong K, Li X (2019) Understanding user behaviors when phishing attacks occur. In: 2019 IEEE international conference on intelligence and security informatics (ISI), p 222
Baral G, Arachchilage NAG (2019) Building confidence not to be phished through a gamified approach: conceptualising user’s self-efficacy in phishing threat avoidance behaviour. In: 2019 cybersecurity and cyberforensics conference (CCC), pp 102–110
Wen ZA, Lin Z, Chen R, Andersen E (2019) What.hack: engaging anti-phishing training through a role-playing phishing simulation game. In: Proceedings of the 2019 CHI conference on human factors in computing systems. CHI ’19. Association for Computing Machinery, New York
Yu K, Taib R, Butavicius MA, Parsons K, Chen F (2019) Mouse behavior as an index of phishing awareness. In: Lamas D, Loizides F, Nacke L, Petrie H, Winckler M, Zaphiris P (eds) Human–computer interaction—INTERACT 2019. Springer, Cham, pp 539–548
Pfeffel K, Ulsamer P, Müller NH (2019) Where the user does look when reading phishing mails—an eye-tracking study. In: Zaphiris P, Ioannou A (eds) Learning and collaboration technologies designing learning experiences. Springer, Cham, pp 277–287
Patel P, Sarno DM, Lewis JE, Shoss M, Neider MB, Bohil CJ (2019) Perceptual representation of spam and phishing emails. Appl Cogn Psychol 33(6):1296–1304
Akhawe D, Felt AP (2013) Alice in Warningland: a large-scale field study of browser security warning effectiveness. In: USENIX security symposium, vol 13
Khonji M, Iraqi Y, Jones A (2013) Phishing detection: a literature survey. IEEE Commun Surv Tutor 15(4):2091–2121
Purkait S (2012) Phishing counter measures and their effectiveness—literature review. Inf Manage Comput Secur 20(5):382–420
Afroz S, Greenstadt R (2011) PhishZoo: detecting phishing websites by looking at them. In: 2011 IEEE fifth international conference on semantic computing, pp 368–375
Pattinson M, Jerram C, Parsons K, McCormac A, Butavicius M (2012) Why do some people manage phishing e-mails better than others? Inf Manage Comput Secur 20(1):18–28
Karakasiliotis A, Furnell SM, Papadaki M (2006) Assessing end-user awareness of social engineering and phishing. In: Proceedings of 7th Australian information warfare and security conference. Accessed 29 Apr 2019
Butavicius M, Parsons K, Pattinson M, McCormac A (2016) Breaching the human firewall: social engineering in phishing and spear-phishing emails. arXiv e-prints, 1606–00887. arXiv:1606.00887
Canova G, Volkamer M, Bergmann C, Borza R (2014) NoPhish: an anti-phishing education app. In: Security and trust management. Lecture notes in computer science, Springer, Cham, pp 188–192
Jansen J, Leukfeldt R (2015) How people help fraudsters steal their money: an analysis of 600 online banking fraud cases. In: 2015 workshop on socio-technical aspects in security and trust, pp 24–31
Parsons K, Butavicius M, Pattinson M, Calic D, Mccormac A, Jerram C (2015) Do users focus on the correct cues to differentiate between phishing and genuine emails? Australasian Conference on Information Systems. arXiv:1605.04717
Turner CMB, Turner CF (2019) Analyzing the impact of experiential pedagogy in teaching socio-cybersecurity: cybersecurity across the curriculum. J Comput Sci Coll 34(5):12–22
Takata T, Ogura K (2019) Confront phishing attacks—from a perspective of security education. In: 2019 IEEE 10th international conference on awareness science and technology (iCAST), pp 1–4
House D, Raja MK (2019) Phishing: message appraisal and the exploration of fear and self-confidence. Behav Inf Technol. https://doi.org/10.1080/0144929X.2019.1657180
Eaton V, Cordova J, Greer T, Smith L (2019) A comparison of perceptions of cs majors and non-cs majors regarding email security. J Comput Sci Coll 34(3):31–37
Sommestad T, Karlzén H (2019) A meta-analysis of field experiments on phishing susceptibility. In: 2019 APWG symposium on electronic crime research (eCrime), pp 1–14
Parsons K, McCormac A, Pattinson M, Butavicius M, Jerram C (2015) The design of phishing studies: challenges for researchers. Comput Secur 52:194–206
Wright R, Chakraborty S, Basoglu A, Marett K (2010) Where did they go right? understanding the deception in phishing communications. Group Decis Negot 19(4):391–416
Williams EJ, Hinds J, Joinson AN (2018) Exploring susceptibility to phishing in the workplace. Int J Hum Comput Stud 120:1–13
John OP, Srivastava S (1999) The big five trait taxonomy: history, measurement, and theoretical perspectives. In: Pervin LA, John OP (eds) Handbook of personality: theory and research, 2nd edn. Guilford Press, New York, pp 102–138
Rawlinson G (2007) The significance of letter position in word recognition. IEEE Aerosp Electron Syst Mag 22(1):26–27
Stanton B, Theofanos MF, Prettyman SS, Furman S (2016) Security fatigue. IT Prof 18(5):26–32
Crossler RE, Johnston AC, Lowry PB, Hu Q, Warkentin M, Baskerville R (2013) Future directions for behavioral information security research. Comput Secur 32:90–101
Rajivan P, Gonzalez C (2018) Creative persuasion: a study on adversarial behaviors and strategies in phishing attacks. Front Psychol 9:135
Nguyen TH, Yang R, Azaria A, Kraus S, Tambe M (2013) Analyzing the effectiveness of adversary modeling in security games. In: Proceedings of the twenty-seventh AAAI conference on artificial intelligence. AAAI’13, AAAI Press, Bellevue, Washington, pp 718–724
Polley S (2017) ComBAT phishing with email automation. SANS Institute Information Security Reading Room 29. https://www.sans.org/reading-room/whitepapers/email/combat-phishing-email-automation-38025
Dwork C (2008) Differential privacy: a survey of results. In: Agrawal M, Du D, Duan Z, Li A (eds) Theory and applications of models of computation. Lecture notes in computer science. Springer, Berlin, pp 1–19
MATH Google Scholar
Download references
Acknowledgements
Author information, authors and affiliations.
Institute of Applied Information Technology (InIT), Zurich University of Applied Sciences (ZHAW), Winterthur, 8401, Switzerland
Daniel Jampen, Gürkan Gür, Thomas Sutter & Bernhard Tellenbach
You can also search for this author in PubMed Google Scholar
Contributions
DJ and BT did the initial survey design. DJ carried out most of the survey work with TS, GG and BT helping out at a later stage of the survey work. GG and BT supervised the drafting of the manuscript and contributed heavily to its design and revisions. All authors read and approved the final manuscript.
Corresponding author
Correspondence to Daniel Jampen .
Ethics declarations
Competing interests.
The authors declare that they have no competing interests.
Additional information
Publisher's note.
Springer Nature remains neutral with regard to jurisdictional claims in published maps and institutional affiliations.
Rights and permissions
Open Access This article is licensed under a Creative Commons Attribution 4.0 International License, which permits use, sharing, adaptation, distribution and reproduction in any medium or format, as long as you give appropriate credit to the original author(s) and the source, provide a link to the Creative Commons licence, and indicate if changes were made. The images or other third party material in this article are included in the article's Creative Commons licence, unless indicated otherwise in a credit line to the material. If material is not included in the article's Creative Commons licence and your intended use is not permitted by statutory regulation or exceeds the permitted use, you will need to obtain permission directly from the copyright holder. To view a copy of this licence, visit http://creativecommons.org/licenses/by/4.0/ .
Reprints and permissions
About this article
Cite this article.
Jampen, D., Gür, G., Sutter, T. et al. Don’t click: towards an effective anti-phishing training. A comparative literature review. Hum. Cent. Comput. Inf. Sci. 10 , 33 (2020). https://doi.org/10.1186/s13673-020-00237-7
Download citation
Received : 03 December 2019
Accepted : 15 June 2020
Published : 09 August 2020
DOI : https://doi.org/10.1186/s13673-020-00237-7
Share this article
Anyone you share the following link with will be able to read this content:
Sorry, a shareable link is not currently available for this article.
Provided by the Springer Nature SharedIt content-sharing initiative
- Anti-phishing training
- Security awareness
- Security training tools
- Machine learning
Phishing – challenges and solutions
- January 2018
- Computer Fraud & Security 2018(1):15-20
- 2018(1):15-20
- This person is not on ResearchGate, or hasn't claimed this research yet.

- Cleveland State University
Abstract and Figures

Discover the world's research
- 25+ million members
- 160+ million publication pages
- 2.3+ billion citations

- Karim Ouazzane

- Rosyzie Anna Awg Haji Mohd Apong

- Manu J Pillai
- Yongyun Cho
- Wosah Peace Nmachi
- Curtis Briddick

- James Nicholson
- Peace Nmachi Wosah
- Qublai Khan Ali Mirza

- Medha Wyawahare
- Rutuja Nagdekar
- Atharva Naik

- Sarah Jamjoom

- COMPUT SECUR
- Nina Marshall
- Daniel Sturman

- Neeranjan Chitare

- J Appl Math

- Ahmad Gbara

- Elizabeth Nunge

- Tom Jagatic
- Nathaniel A. Johnson

- Tracey Caldwell

- Recruit researchers
- Join for free
- Login Email Tip: Most researchers use their institutional email address as their ResearchGate login Password Forgot password? Keep me logged in Log in or Continue with Google Welcome back! Please log in. Email · Hint Tip: Most researchers use their institutional email address as their ResearchGate login Password Forgot password? Keep me logged in Log in or Continue with Google No account? Sign up
Help | Advanced Search
Computer Science > Cryptography and Security
Title: next generation of phishing attacks using ai powered browsers.
Abstract: The increase in the number of phishing demands innovative solutions to safeguard users from phishing attacks. This study explores the development and utilization of a real-time browser extension integrated with machine learning model to improve the detection of phishing websites. The results showed that the model had an accuracy of 98.32%, precision of 98.62%, recall of 97.86%, and an F1-score of 98.24%. When compared to other algorithms like Support Vector Machine, Naïve Bayes, Decision Tree, XGBoost, and K Nearest Neighbor, the Random Forest algorithm stood out for its effectiveness in detecting phishing attacks. The zero-day phishing attack detection testing over a 15-day period revealed the model's capability to identify previously unseen threats and thus achieving an overall accuracy rate of 99.11%. Furthermore, the model showed better performance when compared to conventional security measures like Google Safe Browsing. The model had successfully detected phishing URLs that evaded detection by Google safe browsing. This research shows how using machine learning in real-time browser extensions can defend against phishing attacks. It gives useful information about cybersecurity and helps make the internet safer for everyone.
Comments: | 6 pages, 10 Figures |
Subjects: | Cryptography and Security (cs.CR) |
Cite as: | [cs.CR] |
(or [cs.CR] for this version) | |
Focus to learn more arXiv-issued DOI via DataCite |
Submission history
Access paper:.
- Other Formats

References & Citations
- Google Scholar
- Semantic Scholar
BibTeX formatted citation

Bibliographic and Citation Tools
Code, data and media associated with this article, recommenders and search tools.
- Institution
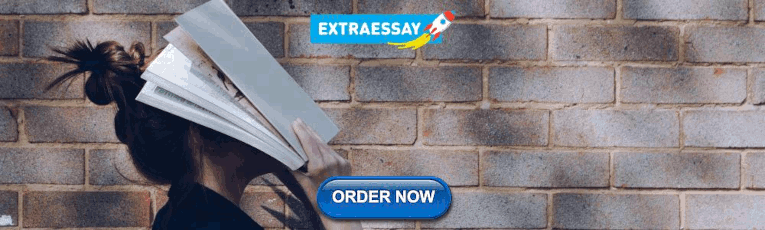
arXivLabs: experimental projects with community collaborators
arXivLabs is a framework that allows collaborators to develop and share new arXiv features directly on our website.
Both individuals and organizations that work with arXivLabs have embraced and accepted our values of openness, community, excellence, and user data privacy. arXiv is committed to these values and only works with partners that adhere to them.
Have an idea for a project that will add value for arXiv's community? Learn more about arXivLabs .

An official website of the United States government
The .gov means it’s official. Federal government websites often end in .gov or .mil. Before sharing sensitive information, make sure you’re on a federal government site.
The site is secure. The https:// ensures that you are connecting to the official website and that any information you provide is encrypted and transmitted securely.
- Publications
- Account settings
Preview improvements coming to the PMC website in October 2024. Learn More or Try it out now .
- Advanced Search
- Journal List
- Springer Nature - PMC COVID-19 Collection

Prevention and mitigation measures against phishing emails: a sequential schema model
Yumi e. suzuki.
1 School of Criminal Justice, Wichita State University, 1845 Fairmount St., Wichita, KS 67260-0135 USA
Sergio A. Salinas Monroy
2 School of Computing, Wichita State University, Wichita, USA
Phishing emails have permeated our digital communication, taking advantage of vulnerabilities that the information technology system poses to users. Given the potential for further cybersecurity incidents, theft of personally identifiable information, and damage to organizations’ assets, cybersecurity professionals have implemented various mitigation practices to combat phishing emails. This paper categorizes current mitigation practices in relation to a sequential schema adopted from the situational crime prevention approach, so as to enable a more organized and strategic assessment of human and environmental vulnerabilities. Our model could be useful for cybersecurity professionals to further advance mitigation measures as an incident progresses and for criminologists and other academic researchers to reduce the severity of subsequent criminal incidents.
Introduction
As early as March 9, 2020, the U.S. Secret Service ( 2020 ) was releasing statements alerting the public about well-crafted phishing emails related to the coronavirus. Opportunistic criminals have continued to engage in email scams, aimed especially at healthcare and pharmaceutical providers, involving information and supplies related to the coronavirus (FinCEN 2020 ). Although phishing emails have existed since the advent of the email communication system, significant increases in phishing campaigns amid the pandemic, observed by FinCEN and other federal agencies, such as the U.S. Department of Homeland Security (DHS) and the Cybersecurity and Infrastructure Security Agency (CISA), have indicated the need for strategic vigilance at the individual and organizational levels. Individuals are gatekeepers to personally identifiable information (PII) 1 and potentially sensitive data that could impact an organization’s assets. A report by Proofpoint ( 2021a ) documents how successful phishing attacks in 2020 resulted in the loss of data, credential and account compromise, ransomware infection, and other malware infections.
The FBI’s Internet Crime Complaint Center defines phishing as “the use of unsolicited email…purportedly from a legitimate company requesting personal, financial, and/or login credentials” (FBI 2021a , p. 28). As of February 23, 2020, 23 U.S. states and Guam had laws prohibiting phishing, whereas the remaining states address this crime under the categories of computer crime, fraudulent or deceptive practices, or identity theft (National Conference of State Legislatures 2020 ). Phishing is the most frequently reported internet crime to the FBI (FBI 2021a ), and phishing attacks are rising globally (Oest et al. 2018 ). Webmail and software-as-a-service users (31.4%) and financial institutions (19.2%) were the top two major targets during the third quarter of 2020 (Anti-Phishing Working Group 2020 ), and the resulting monetary losses suffered by businesses and consumers are growing (FBI 2021a ). Phishing emails were the most common type of breach, and they involved organized groups in over half of all cases (Verizon 2020 ). Phishing emails targeting specific individuals in an organization can result in business email compromise (BEC) that ends with significant financial losses (Better Business Bureau [BBB] 2019 ; FBI 2021a ).
Given the wide-ranging and severe threats that phishing emails pose to multiple sectors of organizations, cybersecurity professionals are at the forefront of the fight against persistent phishers and their evolving methods. Meanwhile, criminologists with expertise in cybercrime have made modest advancements in the conceptualization and application of theoretical frameworks suitable for addressing phishing emails. Cybercrime is a reasonably new addition to the field of criminology/criminal justice, and it is an inherently international and interdisciplinary field of study (Payne and Hadzhidimova 2020 ). Many of the prevention and mitigation practices that cybersecurity professionals have proposed or adopted to thwart other types of attacks are often based on the situational crime prevention (SCP) approach (Willison and Siponen 2009 ) or compatible with it. By adopting and adjusting the crime script model from SCP, this paper configures techniques drawn from current cybersecurity practices to prevent and mitigate phishing attacks.
Increased investments in cybersecurity training by many organizations reveal the critical role of human vulnerabilities for which the organization’s infrastructure alone cannot compensate (Steves et al. 2020 ). Although organizations can purchase anti-phishing software or phishing awareness training from various vendors, gaining a systematic understanding of phishing approaches based on SCP may complement existing anti-phishing mechanisms by revealing both human and environmental (i.e., organizational) vulnerabilities.
Beginning with the broader impact of phishing emails, we describe the current state of phishing email defenses. We then discuss in detail the proposed model and its application for combating phishing attacks, with descriptions of prevention and mitigation measures.
The broader impact of phishing emails
According to HP-Bromium ( 2020 ), most malware was delivered by email during the fourth quarter of 2020. Malware can be disguised as an attachment or a URL in phishing emails, and malware payloads may include remote access Trojans, downloaders, keyloggers (Proofpoint 2021a ), and ransomware (Greenman et al. 2021 ). Kratikal ( 2020 ), a network security company, observed that almost all phishing emails (97%) received by their customers during 2020 contained ransomware. A recent alert from the FBI ( 2021b ) sent to cybersecurity professionals and system administrators highlighted an increase in ransomware attacks on educational institutions, initiated by compromising Remote Desktop Protocol (RDP) credentials or phishing emails. Over one-quarter of organizations infected with ransomware in 2020 paid the ransom; of these, 60% regained access to data or systems after the first payment, whereas 32% of them had to pay an additional ransom (Proofpoint 2021a ).
The deployment of ransomware in industrial control systems (ICS), such as manufacturing plants or the power grid, has grown steadily in incidence and sophistication over the last few years. Brubaker et al ( 2020 ) reported what appeared to be the first malware designed for an ICS and delivered through a phishing email in 2020. Other security companies have also noted this trend (FortiNet Guard Labs 2020 ). Ransomware deployed via malware in phishing emails that targets ICS is of particular concern due to the potentially catastrophic consequences for critical infrastructure.
For example, when the Colonial Pipeline Company announced its operational halt due to a ransomware attack on May 8, 2021, gasoline supplies throughout the East Coast were disrupted (Congressional Research Service 2021 ), bringing cybersecurity of ICS to the attention of federal agencies and the U.S. Congress. Although the ransomware attack at Colonial Pipeline appears to have been deployed to its networks via a compromised password (Fung and Sands 2021 ), the culprit in the attack, DarkSide, has previously used phishing emails and RDP to infiltrate targeted systems (FBI and CISA 2021 ). In response to this incident, on May 27, 2021, the Transportation Security Administration issued a directive to critical pipeline owners and operators regarding specific cybersecurity requirements (DHS 2021a ), followed by an additional directive requiring measures against ransomware attacks and other cybersecurity issues (DHS 2021b ). Because of a series of high-profile ransomware attacks in addition to the one that impacted Colonial Pipeline, the U.S. Department of Justice (DOJ) and the DHS announced on July 15, 2021, the launch of a one-stop ransomware resource website (DOJ 2021 ). Furthermore, CISA and the FBI released a joint advisory on July 20, 2021, alerting ICS stakeholders regarding detailed information on the past intrusion campaigns targeting ICS (CISA 2021a ).
Another common consequence of phishing email attacks is identity theft (Finklea 2014 ). In addition to Verizon’s data breach report (2020), which showed that organized criminals were involved in more than half of the breaches, a myriad of federal cases has corroborated the nexus between identity theft and organized crime (e.g., DOJ 2014 , 2017 ; FBI, n.d.). The FBI ( 2006 ) has indicated explicitly that its identity theft investigations target organized groups and criminal enterprises to maximize efficient use of resources. The allocation of law enforcement resources and efforts to the identity theft–organized crime nexus may be timely, given the increasing trend of identity theft as reported by the Federal Trade Commission ( 2021 ) and the resurgence of schemes taking advantage of the pandemic, such as government benefit frauds.
Additionally, experts in identity crime services have increasingly recognized this nexus (Green et al. 2020 ). Collaboration among law enforcement, cybersecurity professionals, academic researchers, and victim service providers may offer a more comprehensive picture of the role of phishing emails in identity theft, opportunities to assess existing investigative tools and technologies, and strategies for protecting PII at both individual and organizational levels. Button and Cross ( 2017 ) pointed out that fraud and scams have not been a high priority for law enforcement, thereby perhaps generating misconceptions about the severity of these crimes and their actual impact on victims. They also lamented a lack of literature addressing fraud prevention measures. Since phishers typically have almost nine hours between the first victim’s visit to a fraudulent website and anti-phishing mechanisms’ detection of their phishing site as malicious, and an additional 12 hours until the last unsuspecting victim visits the malicious site (Oest et al. 2020b ), strategic efforts to minimize the potential damage are essential for both individuals and organizations. In collaboration with concerned professionals, academic researchers could conduct innovative studies on identity theft or resulting fraud to advance knowledge and best practices among the professional community and raise awareness about potential harms to the public.
Current understanding of phishing attacks
Current phishing attack prevention techniques focus mainly on preventing phishing emails from reaching the users’ inboxes and on discouraging users from accessing phishing websites. These approaches can be classified into email filters, blocking of phishing websites, and user training.
Email filters to prevent phishing emails have been extensively studied and are widely used by email service providers (Karim et al. 2019 ). Email filters are software applications that run on email servers. Their objective is to inspect email messages addressed to users within an organization and to classify them as legitimate or malicious messages. Legitimate messages are forwarded to the addressed users; malicious messages can be deleted, sent to the addressed users’ spam folder, or stored by the email server for further analysis. Email filters prevent users from clicking on potentially malicious URLs in the email messages or engaging in email conversations with the attacker by keeping those users from ever receiving the message.
Email filters use multiple strategies to classify email messages (El Aassal et al. 2020 ). They initially attempt to determine whether the email message was sent from a legitimate source by checking the sender’s email address against a phishing or spam blacklist. The email filter also verifies that the public key from the sender’s email server matches its IP address and domain name. It does so by using the Sender Policy Framework (SPF) and DomainKeys Identified Mails (DKIM) protocols.
After establishing the email sender's legitimacy, the email filter then inspects URLs within the body of the email message. The filter first checks the URLs against widely used blacklists maintained by the Internet community and security companies (Oest et al. 2020a ). If the URL is on the blacklist, the email is classified as malicious. If it does not appear on any blacklist, the filter can proceed to run one of various phishing URL detectors. These detectors employ heuristics and artificial intelligence to determine whether the URL’s features are consistent with those of previously identified phishing URLs.
Although the email filtering techniques described above successfully classify many phishing emails as malicious messages, attackers can still evade the filters by carefully crafting their messages (Hu and Wang 2018 ). For example, attackers may use legitimate email accounts from various email service providers to bypass the sender legitimacy check. They can also evade the URL check by hosting their phishing websites on previously compromised domains of legitimate organizations. Furthermore, they may send phishing emails that contain no URLs at all, hoping instead to engage victims in correspondence and persuade them to act on behalf of the attacker. Lastly, all email filters have a certain false-negative rate that results in some malicious messages being classified as legitimate.
A second way for system administrators to protect their users from phishing attacks is by blocking the domains known to host phishing websites. In this approach, users are prevented from accessing any domain that appears on one of the widely used blacklists. Even if a malicious email with a URL pointing to a phishing website bypasses the email filter, users are restrained from opening the website, thereby protecting them from falling victim to a phishing scam.
To prevent users from accessing a domain, system administrators can configure their firewalls to block all outgoing connections to the domain's IP address or the domain. They can also configure their domain name system (DNS), which translates URLs to IP addresses for users, to return a default IP address when a user requests access to a blacklisted domain.
The third technique employed to prevent phishing attacks is to educate users about phishing attacks, either by providing reminders of potential phishing attempts in incoming emails or by offering on-site or online training. System administrators can install software that displays alert messages on emails sent from outside the organization (Thompson et al. 2019 ). Such alerts have demonstrated some effectiveness (Xiong et al. 2017 ). Reminders briefly explaining what phishing is to users have also been described as a promising practice (Reinheimer et al. 2020 ).
Direct training through workshops is another way to educate users about the danger of phishing and how to spot examples. As users become aware of phishing emails and how they work, they are more likely to notice them in their inboxes. This approach has had substantial success, but research has shown that a small proportion of users remain vulnerable to phishing scams even after training (Singh et al. 2019 ).
Although the above techniques constitute an essential first line of defense against phishing attacks, they ignore the subsequent steps of a phishing attack, that is, what happens after a criminal manages to use phishing emails and websites to steal users’ credentials and PII. Once attackers have access to the organization’s networks via the stolen credentials, they perform actions that can cause considerable harm to victims, including intellectual property theft, access to bank accounts using stolen personal information, and installing ransomware.
In the following discussion, we adapt some of the tools of SCP to highlight how some cybersecurity best practices can be used to protect organizations against all steps of phishing attacks.
Applying the situational crime prevention approach to phishing emails
The situational crime prevention approach is rooted primarily in the rational choice perspective of an individual’s assessment for crime commission based on perceived rewards and risks (Clarke 1983 ; Cornish and Clarke 1987 ), as well as in routine activity approach of three elements of a direct-contact predatory crime (Cohen and Felson 1979 ). Specifically, SCP takes into consideration the offender’s decision in weighing the costs and benefits of offending as well as the convergence of a motivated offender, a suitable target, and the absence of a capable guardian as necessary prerequisites for a crime to occur (Bossler 2020 ). SCP yields crime prevention measures targeting a specific type of crime by systematically manipulating the environment “to reduce the opportunities for crime and increase its risks as perceived by a wide range of offenders” (Clarke 1983 , p. 225). Thus, it seeks to decrease the prospects of crime by limiting the opportunities to commit crime (or situational components of crime) and impacting perceptions and decisions about offending (Clarke 1983 ; Ekblom 2017 ; Smith and Clarke 2012 ). These can be done by physically blocking or increasing the effort in obtaining crime opportunities, by increasing the actual (or perception of the) risks of crime, and by limiting the rewards associated with the crime. SCP also can, in some situations, reduce the factors making a criminal decision more likely and remind potential offenders about the rules of behavior (Cornish and Clarke 2003 ). Rather than focusing on the criminal justice system to sanction illegal behavior, SCP relies on organizations to reduce crime opportunities by making their environments less conducive to committing crimes (Clarke 1997 ).
The original eight-technique classification scheme of SCP, published in 1980 (Hough et al. 1980 ), offered potential mechanisms and techniques applicable in certain situations (Smith and Clarke 2012 ). Later, a 12-technique classification scheme focusing on the prevention concepts of effort, risk, and reward was introduced (Clarke 1992 ), followed by a 16-technique scheme with a new classification category of guilt or shame (Clarke and Homel 1997 ). In response to Wortley’s ( 2001 ) critique of SCP and his proposal of situational precipitation strategies with four control mechanisms (related to prompts, pressures, permissibility, and provocations), Cornish and Clarke ( 2003 ) presented a revised and updated classification scheme, which included five techniques, each of which is categorized under five prevention mechanisms. Acknowledging “the importance of addressing the interaction between the offender and his or her environment” and “the general value of situational crime prevention as a way of controlling crime by trying to understand and manage aspects of this interaction” (Cornish and Clarke 2003 , p. 50), the latest classification scheme incorporates reducing provocations and removing excuses as additional prevention mechanisms to the existing three mechanisms from the two previous versions (i.e., Clarke 1992 ; Clarke and Homel 1997 ), namely increasing the effort, increasing the risks, and reducing the rewards for committing a crime.
Although limited in scope and application, SCP has been utilized to reduce the risk of various types of cybercrimes. Hartel et al ( 2011 ) demonstrated that SCP techniques apply to crimes occurring in cyberspace just as to more traditional crimes offline. SCP has been used to address information security (Hinduja and Kooi 2013 ; Willison and Backhouse 2006 ; Willison and Siponen 2009 ), cybersecurity (Back and LaPrade 2020 ), cyber frauds and scams (Button and Cross 2017 ), and cyberstalking (Reyns 2010 ).
Unlike other forms of cybercrime, in which motives are known and victim types are consistent, phishers are likely to have varying goals, motivations, and victim types. 2 For example, a phisher may seek to steal PII from a government agency only to subsequently steal highly sensitive information. Similarly, a phisher may enter an individual victim’s system to steal credentials, but may go further by demanding a ransom in exchange for releasing the victim’s system and data. Thus, our model purposely covers phishing emails regardless of perceived motives, goals, or victim types, as a phishing email is both a specific crime and a mechanism by which to commit additional crimes. Furthermore, phishers’ true motives may be unknown and their goals may change as they swim through the target systems. However, the utility of two aspects of the SCP approach, bounded rationality and crime scripts, may provide potentially sustainable applications to the phishing email defense.
Bounded rationality, developed by Simon ( 1955 , 1957 ), considers “the cognitive limitations of [goal-oriented] decision makers in attempting to achieve those goals” (Jones 1999 , p. 299). In the case of phishers, these limitations may include time, ability or skills, knowledge, and resources available to accomplish varying goals of phishing attacks. Parker ( 1998 ) proposed that key characteristics of cybercriminals include skills, knowledge, resources, authority, and motives (SKRAM). Understanding criminals’ exhibited skills used to penetrate the system, their knowledge of the phishing ecosystem from the onset of attacks to the end of the phishing lifecycle, the resources they use to launch phishing attacks, and in some cases their possession of a privileged or authorized access (usually obtained via the physical theft of credentials or by abusing rights to access certain applications or files) may help devise specific mitigation measures as phishers make procedural decisions with bounded rationality.
Another critical component of SCP’s evolving applicability to versatile crimes is the use of crime scripts. A crime script, or an event schema, “organizes our knowledge about how to understand and enact commonplace behavioral processes or routines” (Cornish 1994 , p. 158). Specifically, a script helps cybersecurity professionals to examine a sequence of events at each stage of crime commission and evaluate suitable safeguards (Willison and Backhouse 2006 ; Willison and Siponen 2009 ). Detailed prevention or mitigation measures can thus be identified and then implemented at each stage to halt the progression of criminal events.
With these two advantages of SCP in mind, we have identified prevention and mitigation measures to counter phishing attacks. Consistent with the purpose of SCP, our list of these measures should be viewed as a worksheet for implementing and improving phishing email prevention/mitigation practice and policy in an organization, not as an exhaustive set of options. Thus, our model should not replace the cybersecurity framework of practices specified by the U.S. National Institute of Standards and Technology (NIST 2018 ) 3 or other organizations, such as the International Organization for Standardization. Rather, our model is specific to one particular security issue (i.e., phishing emails) and can complement the current behavior-based frameworks 4 to achieve an increased awareness of situational vulnerabilities. As phishers’ tactics evolve, our prevention and mitigation measures must do so as well.
Prevention/mitigation points and measures
The first and second columns of Table Table1 1 present a crime script in which the scene function (first column) shows a generic progression of a crime (adopted from Cornish 1994 ), followed by the script action (second column) that demonstrates a phishing attack sequence. The third column, situational control, has a broadly defined objective that corresponds to a tactic to accomplish the objective. The last column details prevention or mitigation measures matching situational control’s objectives and tactics. At any point in the script action, the element of the phisher’s bounded rationality may become known to cybersecurity professionals. For example, high-tech phishers may have the skills, knowledge, and resources to acquire the phishing infrastructure needed to launch wide-scale attacks, which are commonly initiated by launching deceptive websites, sending emails to potential victims, and downloading the stolen information (Oest et al. 2020b ). System administrators, in turn, could strengthen network security and guardianship provided by the organization. Similarly, the process of phishers gaining authorized access to specific files or programs may be reverse-engineered to reveal a potential source of a security breach. Knowledge of what constraints phishers are willing or unwilling to overcome can be valuable information facilitating an assessment of the durability of the organization’s existing cybersecurity. Below, we further explain mitigation measures that correspond to the progression of phishing attacks and the situational objective of each measure.
Mitigation points for phishing attacks
Scene function | Script action | Situational control | Mitigation measures | |
---|---|---|---|---|
Objective | Tactic | |||
Preparation | Scour online for emails Obtain email addresses | (1) Increase the effort of a successful phishing attack. | Limit presence in OSINT | Limit personal info available to the public |
Set up phishing infrastructure | Block subscription to unknown websites | |||
Entry | Trigger phishing infrastructure to send emails | Restricted access by users | Avoid publishing personal or business email addresses online Avoid using business emails for personal subscriptions | |
Protected access for users | Email filter Automatic spam folder Honey accounts Disable compromised credentials | |||
Precondition | Wait for email response Wait for URL clicks Wait for attachment to open | (2) Clarify users’ responsibility | Promote acceptable behavior | IT training Promote organizational email policy Develop credential disclosure policy Award programs for good email practice |
Awareness/reminders | Banner alerting potential scams Banner alerting emails from outside organization Flag suspicious URLs in emails Display sender’s true email address Display “reply to” | |||
Instrumental pre-condition Instrumental initiation | Collect credentials Enter target network Locate PII | 3) Increase the probability of detecting a phishing attack | Better guardianship | Email reply tracking IP-based monitoring Monitor email exchanges and login attempts Domain verification |
User authentication | Strong passwords MF authentication | |||
Instrumental actualization | Access PII | (4) Limit phishers’ ability to find sensitive information | Access control | Privileged access Multiple-person sign off on access to data Limited access to users in local network or VPN |
Doing | Extract PII | Network security | Network segregation Firewalls Intrusion detection/prevention systems | |
Database security | Data encryption | |||
Data backup | ||||
Automatic OS and software updates | ||||
Post-condition | Exit the system | (5) Discourage similar attacks | No public disclosure of exploited vulnerabilities VPN access to IT | |
Exit | Close remote connection |
Situational control objective 1: increase the effort of a successful phishing attack
When phishers scour online sources for emails or obtain compromised emails, a phishing kit can be used to launch phishing attacks. With the requisite phishing infrastructure in place, massive phishing emails are on the way with a simple click. Ways to counter phishers’ efforts to gain access to PII and the organization’s sensitive information include limiting one’s presence in the publicly available data that could be used for open-source intelligence (OSINT), restricting access by users, and protecting access for users.
Limit presence in OSINT
One of the key measures taken by individuals or organizations is to limit publicly available information, thereby reducing the chance that phishers will acquire contact information to launch phishing attacks or conduct personalized phishing scams (BBB 2019). OSINT, therefore, can be used for or against phishing. For example, phishers may collect information from public and social networking sites to exploit potential victims. The United Kingdom’s National Cyber Security Centre (NCSC 2018 ) recommends examining the information available on the organization’s website and social media. System administrators may assess the level of publicly available organizational information, particularly contact information, to determine what is truly necessary. Likewise, system administrators may consider blocking subscriptions to unknown websites to reduce presence in OSINT.
Restricted access by users
Potential victims can safeguard themselves against phishers by restricting their publicly available information, such as personal or business email addresses or any other information that should not be in phishers’ hands. Avoiding the use of business emails for private correspondence can also protect against unwanted access. Subscribing to online services using business emails may potentially invite phishers into the organization’s system.
Protected access for users
Other measures to increase the effort of a successful phishing attack include filtering emails and utilizing an automatic spam folder (NCSC 2018 ), which most email service providers offer as a default setting. The accuracy of filtering emails may vary; however, content-based filtering featuring 27 items extracted from emails resulted in successful phishing rates of less than 1% (Bergholz et al. 2010 ). Compromised credentials should immediately be disabled to mitigate further damage.
System administrators can create so-called honey email accounts at the organizational level to detect phishers and deflect them away from legitimate email accounts. Honey email accounts are intentionally designed to allow attackers to compromise their credentials (Akiyama et al. 2018 ; Gajek and Sadeghi 2007 ; Lazarov et al. 2016 ; Peng et al. 2019 ). System administrators can fill the honey accounts with seemingly real email traffic to lure phishers into spending an excessive amount of time looking for valuable information. By wasting the phisher’s time, honey accounts attempt to reduce their time spent on legitimate accounts.
Situational control objective 2: clarify the user’s responsibility
While phishers wait for email responses, URL clicks, or attachments to open, users can be the best defense against further attacks by being vigilant against phishers’ tactics. Users may appreciate having situational controls to assist their guardianship, allowing them to be more aware of their role in, and their responsibility for, detecting and minimizing the impact of phishing emails.
Promote acceptable behavior
Among over 2,500 manufacturers and other businesses surveyed, 42% indicated that they did not have or were not sure if they had policies and procedures in place to protect their data and intellectual property (Travelers Risk Control 2016 ). If they do not already offer it, organizations may consider requiring regular information technology (IT) training that includes awareness of phishing schemes and encourages compliance with email and credential disclosure policies. Reinheimer et al ( 2020 ) reported that security awareness training yielded successful identification of phishing and legitimate emails even four months after the training.
In addition to regular training, organizations may consider offering award programs for good email practice. For example, a top employee with no record of phishing compromise and the greatest number of phishing emails reported to the IT team may be given priority parking for a month or some other prized privileges.
Awareness/situational reminders
Equally crucial to promoting acceptable behavior among users are situational reminders of potential phishing attempts (NCSC 2018 ). Banner alerts for potential scams or emails outside organizations may become more frequent practice than in the previous decade. Flagging suspicious URLs in emails can further alert the users to the sender's likely nefarious intent, thus dissuading them from attempting to access the URLs. Users may also be reminded of the importance of examining the sender’s valid email address and the “reply to” field to see if any discrepancy exists, which could be a sign of phishing.
Situational control objective 3: increase the probability of detecting a phishing attack
As phishers gain access to credentials and enter the organization’s network to locate PII, organizations that provide specific guardianship and user authentication via IT mechanisms may be in a better position to offer a strong defense against further damage. In this sense, system administrators are in the optimal position to devise suitable mechanisms to detect any phishing attempts.
Better guardianship
Organizations can offer better guardianship by tracking email replies and monitoring the IP addresses involved. Many phishers fill the “from” field in their emails with a legitimate-looking address. 5 However, they often do not control the address listed in the “from” field. To receive the replies from their victims, phishers fill the “reply to” field with an address that they control. By checking for discrepancies between the “from” and “reply to” addresses, system administrators can detect phishing emails.
In this context, we must note that some legitimate emails may have different addresses in the “from” and “reply to” fields. For example, when a personal assistant sends emails on behalf of her client, an additional method of evaluation is needed to determine whether an email is legitimate. The IP address used by users to access their email accounts is roughly composed of two parts; the first part identifies the Internet service provider (ISP), and the second part identifies the user within the ISP. Although the part of the IP address that identifies the user changes continually, the part that identifies the ISP remains constant. Since users often employ the same set of ISPs to access their accounts (e.g., their home ISP, their mobile ISP, and their work ISP), by monitoring the ISPs that people use to login to their email accounts, it is possible to identify suspicious logins from different ISPs that may indicate that a remote attacker has compromised an account. Consequently, ISPs can maintain a regular update on IP address blacklisting, such as a DNS-based Blackhole List (DNSBL) (Bhadane and Mane 2017 ; Gupta et al. 2018 ).
In addition to monitoring suspicious email exchanges and logins for potential phishing attempts, system administrators can also attempt to identify phishing emails by verifying the signature of the email provider who sent them. The CISA (n.d.) suggests SPF and DKIM in detecting unauthorized emails. SPF enables the recipient to know which mail servers are used from the sender’s domain, which in turn shows the DNS “which servers are allowed to send email on behalf of a domain” (Bhadane and Mane 2017 , p. 21).
When a legitimate email provider implements DKIM, it signs outgoing emails with its private key, which is a secret value that only the email provider knows. The email provider receiving the signed email message can use standard cryptographic techniques to verify the signature's authenticity. Phishing emails that spoof the “from” address with the address of a provider with DKIM can be easily detected with this technique. An attacker could conceivably set up an email server with DKIM for the sole purpose of sending phishing emails; however, such servers are eventually added to a blacklist that the system administrator should keep up to date. Additionally, CISA (n.d.) has pointed out that DMARC (Domain-based Message Authentication, Reporting & Conformance) “provides the strongest protection against spoofed email, ensuring that unauthenticated messages are rejected at the mail server” (p. 2) if the DMARC reject policy is in place.
User authentication
As the major line of defense, user authentication assigns an identifier, such as a login name, and verifies the user through an authentication process, which is typically accomplished by associating the user with passwords (Stallings 2020 ). Users should, therefore, devise strong passwords or passphrases, which may deter phishers' efforts to launch a successful attack (NCSC 2018 ). Verification of the user can also be accomplished by combining something that only the user knows (e.g., passwords), possesses (e.g., a code), or exhibits as an inherent bodily feature (e.g., fingerprints, retina/iris patterns) (CISA 2020 ; Stallings 2020 ). The multifactor authentication of knowledge, possession, and inherence relevant to the user is recommended as an essential tool (CISA 2020 ) in place of the previously utilized two-factor authentication.
Situational control objective 4: limit phishers’ ability to find sensitive information
When phishers attempt to access PII, their ability to locate it or sensitive information belonging to the organization will be reduced if the appropriate procedures are in place. Access control 6 and network and database security may be appropriate additional defenses against extraction of PII.
Access control
Granting users access only to needed files and programs while restricting access to sensitive information to those with specially assigned privileges may minimize the risk of penetration by adversaries (CISA 2020 ). Requiring multiple people to sign off on granting access to sensitive data may also be prudent in safeguarding the organization’s assets. Limiting users’ access to local networks or virtual private networks (VPNs) may offer further safeguards against phishers.
Network security
Network segregation separates sensitive servers from publicly accessible ones. Sensitive network assets can be separated from the rest of the network by placing firewalls between them and other servers. In extreme cases, they can be completely disconnected from the rest of the network. Servers that must be accessed both by Internet users and sensitive servers can be placed in a special network compartment called a demilitarized zone (DMZ) (Stouffer et al. 2011 ; Tracy et al. 2002 ). Servers in the DMZ can connect to users on the Internet through a firewall and to sensitive servers through a separate firewall. Firewalls provide a “controlled link” between the network and the Internet, as well as a “single choke point” where security protocol can be implemented (Stallings 2020 , p. 155). When network segregation is in place, phishers must defeat several network security barriers before they can access sensitive servers (National Security Agency 2010 ).
An intrusion detection system (IDS) and an intrusion prevention system (IPS) may provide additional layers of security by sending system administrators real-time alerts of an unauthorized user’s attempts to access the system and by blocking unauthorized and malicious activity (Stallings 2020 ).
Database security
If a phisher accesses sensitive data, the system administrator can protect it by using encryption. Encryption programs take the original data, called the plaintext, and a secret key as input and output seemingly random blocks of data, called the ciphertext. To recover the plaintext, a decryption program takes the ciphertext and the secret key as input and outputs the plaintext. The secret key is known only to the system administrator or to legitimate users who own the plaintext. To recover the plaintext, a phisher must steal both the ciphertext and the secret key from a legitimate user. Therefore, encryption adds a security layer to sensitive data (Stallings 2006 ). It is also important for system administrators to backup data and enable automatic updates for all operating systems and software as basic cybersecurity practices (CISA 2021b ).
Situational control objective 5: discourage similar attacks
Once the phisher has exited the system, it may be appropriate to share the general characteristics of phishing attacks as a lessons-learned report to reduce similar future attacks. However, system administrators may wish to consider security and liability issues before revealing the specifics of exploited vulnerabilities (Cichonski et al. 2012 ). For example, revealing the actual content of a malicious attachment might offer potential phishers clues to further exploit the vulnerability before security patches are deployed. Likewise, a nondisclosure agreement may prohibit system administrators from disclosing the details of a phishing attack that has impacted the confidentiality of the organization’s sensitive information (Cichonski et al. 2012 ). Accessing the IT site for the latest security requirements or trends via VPN only may further ensure that the organization’s information infrastructure remains protected from those who have no business accessing its IT-related information.
Although the potential to be compromised by phishing emails is always present due to human and environmental vulnerabilities, mitigation measures at various sequential points as described above should be sufficient to assess current cybersecurity practices and guide the selection and implementation of any additional security protocols.
Phishing emails may appear to be merely a nuisance; however, their potential blow to an individual’s PII and an organization’s information infrastructure and assets can be costly. In this paper, we have presented a series of mitigation points for phishing attacks and corresponding countermeasures as an incident progresses, with the goal of gaining a systematic understanding of phishing emails by revealing both human and environmental vulnerabilities. The application of a sequential schema from the situational crime prevention approach also facilitates a holistic understanding of phishing emails from cybersecurity and criminological perspectives. For cybersecurity professionals, our model may offer an additional tool to assess existing security measures with a particular focus on appropriate mitigation measures as a security incident progresses. Criminologists and other academic researchers may further advance the utility of the situational crime prevention approach in analyzing and preventing other criminal events, especially those commonly connected to phishing emails, such as identity theft and other identity-based fraud. The mounting evidence of increased volumes of phishing emails, subsequent cybersecurity issues, monetary losses for organizations, and criminal organizations’ involvement in identity theft stemming from human and environmental vulnerabilities should drive all concerned stakeholders to engage in the greatly needed theory-driven and empirically based research that will help us address a host of vital issues surrounding phishing attacks.
Acknowledgements
The authors would like to thank Bruce Barron and Martha Smith for their helpful comments on earlier drafts of this paper. The authors also express their appreciation to the anonymous reviewers for their valuable suggestions.
Declarations
On behalf of all authors, the corresponding author states that there is no conflict of interest.
1 Throughout this paper, PII refers to any information identified with or identifiable as relating to a particular individual, such as credentials for online accounts, medical or financial information, IP addresses, phone numbers, and facial images to unlock smartphones (Nanduru 2021 )
2 Both monetary motives and the pursuit of data theft, including intellectual property and other espionage purposes, have been reported in 2020 (FireEye Mandiant Services 2021 ). For example, a cyberespionage group, Iron Liberty, has used phishing emails to access ICS (Secureworks 2019 ). A more well-known example of varying motives of phishers was observed in the 2016 phishing of an email account owned by John Podesta, who was the campaign chairman for Hillary Clinton (Gupta et al. 2018 ), so as to influence a U.S. presidential election. As these examples illustrate, phishers target individual victims, businesses, and organizations (FBI 2021a ) as well as governments (HP-Bronium 2020 ). The preferred method of infiltrating the target systems via phishing emails appears consistent, as indicated by a recent threat report (Proofpoint 2021b )
3 Our model shares some similarities with the NIST framework of identify, protect, detect, respond, and recover. For example, under the protect category, implementing access control and awareness training is suggested; both of these measures are included in our model. Although the NIST cybersecurity framework was originally developed for critical infrastructure, it can be implemented by other organizations in any sector that rely on IT, ICS, and the IoT (NIST 2018). NIST also makes available the Computer Security Incident Handling Guide (Cichonski et al. 2012 ).
4 In addition to frameworks proposed by NIST and other organizations, the MITRE ATT&CK cyber adversary behavioral model is widely used by cybersecurity professionals and organizations, including CISA. This model includes attack tactics, goals, and techniques to accomplish tactics in phases (Strom et al. 2020 ). For example, attackers may use phishing as initial access, followed by command and scripting interpreter as execution, access token manipulation as privilege escalation, and two-factor authentication interception as credential access (see Strom et al. 2020 , p. 6 for the matrix of techniques).
5 The CAN-SPAM (Controlling the Assault of Non-Solicited Pornography and Marketing) Act of 2003 stipulates a working return email address and a way to opt out of receiving future messages from commercial emailers (Rustad 2019 ). Providing false and fraudulent email addresses, domain names, or IP addresses can be used by the government or any service providers (Rustad 2019 ) to lodge a claim against commercial phishers under the CAN-SPAM Act, but not against phishers without legitimate affiliations with commercial entities.
6 Although some elements of access control may correspond to the concept of target hardening in SCP, consistent with cybersecurity and information security practices, we use access control to refer to “the ability to limit and control the access to host systems and applications via communications links” (Stallings 2020 , p. 11). See also Hinduja and Kooi ( 2013 ) for examples of target hardening and access control in the information security field.
Publisher's Note
Springer Nature remains neutral with regard to jurisdictional claims in published maps and institutional affiliations.
Contributor Information
Yumi E. Suzuki, Email: [email protected] .
Sergio A. Salinas Monroy, Email: [email protected] .
- Akiyama M, Yagi T, Hariu T, Kadobayashi Y. HoneyCirculator: Distributing credential honeytoken for introspection of web-based attack cycle. International Journal of Information Security. 2018; 17 (2):135–151. doi: 10.1007/s10207-017-0361-5. [ CrossRef ] [ Google Scholar ]
- Anti-Phishing Working Group. 2020. Phishing activity trends report: 3rd quarter 2020 . https://docs.apwg.org/reports/apwg_trends_report_q3_2020.pdf . Accessed 3 Feb 2021.
- Back S, LaPrade J. Cyber-situational crime prevention and the breadth of cybercrimes among higher education institutions. International Journal of Cybersecurity Intelligence and Cybercrime. 2020; 3 (2):25–47. doi: 10.52306/RGWS2555. [ CrossRef ] [ Google Scholar ]
- Bergholz A, De Beer J, Glahn S, Moens MF, Paab G, Strobel S. New filtering approaches for phishing email. Journal of Computer Security. 2010; 18 :7–35. doi: 10.3233/JSC-2010-0371. [ CrossRef ] [ Google Scholar ]
- Better Business Bureau. 2019. Is that email really from “the boss?” The explosion of business email compromise (BEC) scams . https://www.bbb.org/article/news-releases . Accessed 8 Mar 2021.
- Bhadane A, Mane SB. State of research on phishing and recent trends of attacks. i-Manager’s Journal on Computer Science. 2017; 5 (4):14–35. [ Google Scholar ]
- Bossler A. Contributions of criminological theory to the understanding of cybercrime offending and victimization. In: Leukfeldt R, Holt TJ, editors. The human factor of cybercrime. Abingdon-on-Thames: Routledge; 2020. pp. 29–59. [ Google Scholar ]
- Brubaker, N., Zafra, D. K., Lunden, K., Proska, K. and Hildebrandt, C. 2020 Financially motivated actors are expanding access into OT: Analysis of kill lists that include OT processes used with seven malware families, 2020 . https://www.fireeye.com/blog/threat-research/2020/07/financially-motivated-actors-are-expanding-access-into-ot.html . Accessed 2 Feb 2021.
- Button M, Cross C. Cyber frauds, scams and their victims. Abingdon-on-Thames: Routledge; 2017. [ Google Scholar ]
- Cichonski, P., Millar, T., Grance, T. and Scarfone, K. 2012. Computer security incident handling guide: Recommendations of the National Institute of Standards and Technology . U.S. Department of Commerce. Special publication 800-61, Revision 2.
- Clarke RV. Situational crime prevention: Its theoretical basis and practical scope. Crime and Justice. 1983; 4 :225–256. doi: 10.1086/449090. [ CrossRef ] [ Google Scholar ]
- Clarke RV. Introduction. In: Clarke RV, editor. Situational crime prevention: Successful case studies. Guilderland: Harrow and Heston; 1992. pp. 3–36. [ Google Scholar ]
- Clarke RV. Introduction. In: Clarke RV, editor. Situational crime prevention: Successful case studies. Guilderland: Harrow and Heston; 1997. pp. 1–43. [ Google Scholar ]
- Clarke RV, Homel R. A revised classification of situational crime prevention techniques. In: Lab SP, editor. Crime prevention at a crossroads. Greenbelt: Academy of Criminal Justice Sciences and Anderson; 1997. pp. 17–27. [ Google Scholar ]
- Cohen LE, Felson M. Social change and crime rate trends: A routine activity approach. American Sociological Review. 1979; 44 (August):588–608. doi: 10.2307/2094589. [ CrossRef ] [ Google Scholar ]
- Congressional Research Service. 2021. Colonial Pipeline: The DarkSide strikes . https://crsreports.congress.gov . Accessed 12 July 2021.
- Cornish D. The procedural analysis of offending and its relevance for situational prevention. In: Clarke R, editor. Crime prevention studies. New York: Criminal Justice Press; 1994. pp. 151–196. [ Google Scholar ]
- Cornish DB, Clarke RV. Understanding crime displacement: An application of rational choice theory. Criminology. 1987; 25 (4):933–948. doi: 10.1111/j.1745-9125.1987.tb00826.x. [ CrossRef ] [ Google Scholar ]
- Cornish DB, Clarke RV. Opportunities, precipitators and criminal decisions: A reply to Wortley’s critique of situational crime prevention. Crime Prevention Studies. 2003; 16 :41–96. [ Google Scholar ]
- Cybersecurity & Infrastructure Security Agency. 2020. Cyber essentials toolkit chapter 4: Your surroundings. https://www.cisa.gov/sites/default/files/publications/cyber%20Essentials%20Toolkit%204%2020200818_508.pdf . Accessed 18 Nov 2020.
- Cybersecurity & Infrastructure Security Agency. 2021a. Significant historical cyber-intrusion campaigns targeting ICS. https://us-cert.cisa.gov/ncas/current-activity/2021/07/20/significant-historical-cyber-intrusion-campaigns-taregting-ics . Accessed 20 Jul 2021.
- Cybersecurity & Infrastructure Security Agency. 2021b. Cyber essentials starter kit: The basics for building a culture of cyber readiness. https://www.cisa.gov/Cyber-Essentials . Accessed 8 Mar 2021.
- Cybersecurity & Infrastructure Security Agency. n.d. CISA Insights: Enhance email & web security. https://www.cisa.gov/sites/default/files/publications/CISAInsights-Cyber-EnhanceEmailandWebSecurity_S508C-a.pdf . Accessed 17 Nov 2020.
- Ekblom P. Crime, situational prevention and technology: The nature of opportunity and how it evolves. In: McGuire MR, Holt TJ, editors. The Routledge handbook of technology, crime and justice. London: Routledge; 2017. pp. 353–374. [ Google Scholar ]
- El Aassal A, Baki S, Das A, Verma RM. An in-depth benchmarking and evaluation of phishing detection research for security needs. IEEE Access. 2020; 8 :22170–22192. doi: 10.1109/ACCESS.2020.2969780. [ CrossRef ] [ Google Scholar ]
- Federal Bureau of Investigation. 2006. Financial crimes report to the public . U.S. Department of Justice. https://fbi.gov/file-repository/stats-services-publications-fcs_report2006-financial-crimes-report-to-the-public-2006-pdf/view Accessed 8 Apr 2020.
- Federal Bureau of Investigation. 2021a. Internet crime report 2020 . https://www.ic3.gov/Media/PDF/AnnualReport/2020_IC3Report.pdf Accessed 17 Mar 2021.
- Federal Bureau of Investigation. 2021b. Increase in PYSA ransomware targeting education institutions . Alert number CP-000142-MW. https://www.ic3.gov/Media/News/2021/210316.pdf . Accessed 7 Apr 2021.
- Federal Bureau of Investigation. n.d. Scams and safety . https://fbi.gov/scams-and-safety/common-scams-and-crimes/identity-theft . Accessed 11 Feb 2021.
- Federal Bureau of Investigation and Cybersecurity & Infrastructure Security Agency. 2021. DarkSide ransomware: Best practices for preventing business disruption from ransomware attacks . https://us-cert.cisa.gov/sites/default/files/publications/AA21-131A_Darkside_Ransomware.pdf . Accessed 12 July 2021.
- Federal Trade Commission. 2021. Consumer sentinel network: Databook 2020 . https://www.ftc.gov/data . Accessed 1 Mar 2021.
- FinCEN. 2020. Advisory on cybercrime and cyber-enabled crime exploiting the Coronavirus disease 2019 (COVID-19) pandemic . FIN-2020-A005.
- Finklea, K. 2014. Identity theft: Trends and issues . Congressional Research Service.
- FireEye Mandiant Services. 2021. M-Trends 2021 . https://www.fireeye.com/current-Threats/annual-threat-report/mtrends.html . Accessed 14 July 2021.
- FortiNet Guard Labs. 2020 EKANS ransomware targeting OT ICS systems . https://www.fortinet.com/blog/threat-research/ekans-ransomware-targeting-ot-ics-systems . Accessed 9 Feb 2021.
- Fung, B. and Sands, G. 2021. Ransomware attackers used compromised password to access Colonial Pipeline network . https://www.cnn.com/2021/06/04/politics/colonial-pipeline-ransomware-attack-password/index.html . Accessed 12 Jul 2021.
- Gajek, S. and Sadeghi, A. R. (2007) A forensic framework for tracing phishers. In IFIP International Summer School on the Future of Identity in the Information Society (pp. 23–35). Springer.
- Green B, Gies S, Bobnis A, Piquero NL, Piquero AR, Velasquez E. Exploring identity-based crime victimizations: Assessing threats and victim services among a sample of professionals. Deviant Behavior. 2020 doi: 10.1080/01639625.2020.1720938. [ CrossRef ] [ Google Scholar ]
- Greenman C, Johnson R, Esplin D. Cyberattacks in higher education at an epidemic level. Fraud Magazine. 2021; 36 (1):12–15. [ Google Scholar ]
- Gupta BB, Arachchilage NAG, Psannis KE. Defending against phishing attacks: Taxonomy of methods, current issues and future directions. Telecommunication Systems. 2018; 67 :247–267. doi: 10.1007/s11235-017-0334-z. [ CrossRef ] [ Google Scholar ]
- Hartel P, Junger M, Wieringa R. Cyber-crime science = crime science +information security. London: University of Twente; 2011. [ Google Scholar ]
- Hinduja S, Kooi B. Curtailing cyber and information security vulnerabilities through situational crime prevention. Security Journal. 2013; 26 :383–402. doi: 10.1057/sj.2013.25. [ CrossRef ] [ Google Scholar ]
- HP-Bromium. 2020. Threat insights report, Q4–2020 . https://threatresearch.ext.hp.com/wp-content/uploads/2021/03/HP_Bromium_Threat_Insights_Report_Q4_2020.pdf . Accessed 17 Mar 2021.
- Hu, H. and Wang, G. 2018. End-to-end measurements of email spoofing attacks . In Proceedings of the 27th USENIX Security Symposium. https://www.usenix.org/system/files/conference/usenixsecurity18/sec18-hu.pdf . Accessed 2 Mar 2019.
- Hough JM, Clarke RVG, Mayhew P. Introduction. In: Clarke RVG, Mayhew P, editors. Designing out crime. London: Her Majesty’s Stationery Office; 1980. pp. 1–17. [ Google Scholar ]
- Jones BD. Bounded rationality. Annual Review of Political Science. 1999; 2 :297–321. doi: 10.1146/annurev.polisci.2.1.297. [ CrossRef ] [ Google Scholar ]
- Karim A, Azam S, Shanmugam B, Kannoorpatti K, Alazab M. A comprehensive survey for intelligent spam email detection. IEEE Access. 2019; 7 :168261–168295. doi: 10.1109/ACCESS.2019.2954791. [ CrossRef ] [ Google Scholar ]
- Kratikal. 2020 Staggering phishing statistics in 2020 . https://www.kratikal.com/blog/Staggering-phishing-statistics-in-2020/ . Accessed 10 Feb 2021.
- Lazarov, M., Onaolapo, J. and Stringhini, G. 2016. Honey sheets: What happens to leaked Google spreadsheets? In Proceeding of the 9th Workshop on Cyber SecurityExperimentation and Test. https://www.usenix.org/system/files/conference/cset16/cset16-paper-lazarov.pdf . Accessed 1 Sep 2019.
- Nanduru, B. 2021. Take it personally: Ten tips for protecting your personally identifiable information . National Cybersecurity Alliance. https://staysafeonline.org/blog/ten-tips-for-protecting-your-pii/ . Accessed 23 Aug 2020.
- National Conference of State Legislatures. 2020. State laws addressing “phishing.” https://www.ncsl.org/research/telecommunications-and-information-technology/state-phishing-laws.aspx . Accessed 11 Feb 2021.
- National Cyber Security Centre. 2018 Phishing attacks: Defending your organization . https://ncsc.gov.uk/guidance/phishing . Accessed 15 Oct 2020.
- National Institute of Standards and Technology. 2018. Framework for improving critical infrastructure cybersecurity . Version 1.1. https://nvlpubs.nist.gov/nistpubs/CSWP/NIST.CSWP.04162018.pdf . Accessed 17 Jul 2021.
- National Security Agency. 2010. Defense in depth. https://apps.nsa.gov/iaarchive/library/ia-guidance/archive/defense-in-depth.cfm . Accessed 11 Mar 2019.
- Oest, A., Safei, Y., Doupe, A., Ahn, G. J., Wardman, B. and Warner, G. 2018. Inside a phisher’s mind: Understanding the anti-phishing ecosystem through phishing kit analysis. In Proceedings of the 2018 APWG Symposium on Electronic Crime Research. https://docs.apwg.org/ecrimeresearch/2018/5349207.pdf . Accessed 1 June 2020.
- Oest, A., Safaei, Y., Zhang, P., Wardman, B., Tyers, K., Shoshitaishvili, Y. and Doupé, A. 2020a. Phishtime: Continuous longitudinal measurement of the effectiveness of anti-phishing blacklists . In Proceedings of the 29th USENIX Security Symposium. https://www.usenix.org/system/files/sec20-oest-phishtime.pdf . Accessed 1 Nov 2020.
- Oest, A., Zhang, P., Wardman, B., Nunes, E., Burgis, J., Zand, A., Thomas, K., Doupe, A. and Ahn, G.J. 2020b. Sunrise to sunset: Analyzing the end-to-end life cycle and effectiveness of phishing attacks at scale . In Proceedings of the 29th USENIX Security Symposium. https://www.usenix.org/conference/usenixsecurity20/presentation/oest-sunrise . Accessed 10 Oct 2020.
- Parker DB. Fighting computer crime: A new framework for protecting information. New York: Wiley; 1998. [ Google Scholar ]
- Payne BK, Hadzhidimova L. Disciplinary and interdisciplinary trends in cybercrime research: An examination. International Journal of Cyber Criminology. 2020; 14 (1):81–105. [ Google Scholar ]
- Peng, P., Xu, C., Quinn, L., Hu, H., Viswanath, B. and Wang, G. (2019) What happens after you leak your password: Understanding credential sharing on phishing sites . In: Proceedings of the 2019 ACM Asia Conference on Computer and Communications Security. https://people.cs.vt.edu/pengp17/papers/asiaccs19.pdf . Accessed 1 June 2020.
- Proofpoint. 2021a 2021 State of the phish: An in-depth look at user awareness, vulnerability and resilience . https://www.proofpoint.com . Accessed 11 Feb 2021.
- Proofpoint. 2021b. Q4 2020 threat report: A quarterly analysis of cybersecurity trends, tactics and themes. https://www.proofpoint.com/us/blog/threat-insight/q4-2020-threat-report-quarterly-analysis-cybersecurity-trends-tactics-and-themes . Accessed 25 Feb 2021.
- Reinheimer, B., Aldag, L., Mayer, P., Mossano, M., Duezguen, R., Lofthouse, B., von Landesberger, T. and Volkamer, M. 2020. An investigation of phishing awareness and education over time: When and how to best remind users . In: Proceedings of the 16th USENIX Symposium on Usable Privacy and Security. https://www.usenix.org/system/files/soups2020-reinheimer_0.pdf . Accessed 10 Oct 2020.
- Reyns BW. A situational crime prevention approach to cyberstalking victimization: Preventive tactics for Internet users and online place managers. Crime Prevention and Community Safety. 2010; 12 :99–118. doi: 10.1057/cpcs.2009.22. [ CrossRef ] [ Google Scholar ]
- Rustad ML. Global Internet law in a nutshell. 4. St. Paul: West Academic Publishing; 2019. [ Google Scholar ]
- Secureworks. 2019. Resurgent Iron Liberty targeting energy sector . Counter Threat Unit Research Team. https://www.secureworks.com/research/resurgent-iron-liberty-targeting-energy-sector . Accessed 14 July 2021.
- Simon HA. A behavioral model of rational choice. Quarterly Journal of Economics. 1955; 69 (1):99–118. doi: 10.2307/1884852. [ CrossRef ] [ Google Scholar ]
- Simon HA. Models of man. New Yok: Wiley; 1957. [ Google Scholar ]
- Singh, K., Aggarwal, P., Rajivan, P. and Gonzalez, C. 2019. Training to detect phishing emails: Effects of the frequency of experienced phishing emails . In Proceedings of the Human Factors and Ergonomics Society Annual Meeting (vol. 63, no. 1, pp. 453–457). Sage.
- Smith MJ, Clarke RV. Situational crime prevention: Classifying techniques using “good enough” theory. In: Welsh BC, Farrington DP, editors. Oxford Handbook of crime prevention. Oxford: Oxford University Press; 2012. pp. 291–315. [ Google Scholar ]
- Stallings W. Cryptography and network security. 4. London: Pearson; 2006. [ Google Scholar ]
- Stallings W. Information privacy engineering and privacy by design: Understanding privacy threats, technology, and regulations based on standards and best practices. Boston: Addison-Wesley; 2020. [ Google Scholar ]
- Steves M, Greene K, Theofanos M. Categorizing human phishing difficulty: A Phish Scale. Journal of Cybersecurity. 2020 doi: 10.1093/cybsec/tyaa009. [ CrossRef ] [ Google Scholar ]
- Stouffer, K., Falco, J. and Scarfone, K. (2011) Guide to industrial control systems (ICS) security . NIST Special Publication 800–82. https://nvlpubs.nist.gov/nistpubs/SpecialPublications/NIST.SP.800-82rl.pdf . Accessed 12 Dec 2020.
- Strom, B. E., Applebaum, A., Miller, D. P., Nickels, K. C., Pennington, A. G. and Thomas, C. B. (2020) MITRE ATT&CK: Design and philosophy . MITRE Corporation.
- Thompson, C., Shelton, M., Stark, E., Walker, M., Schechter, E. and Felt, A. P. 2019. The web's identity crisis: understanding the effectiveness of website identity indicators . In Proceedings of the 28th USENIX Security Symposium. https://www.usenix.org/system/files/sec19-thompson.pdf . Accessed 12 Dec 2020.
- Tracy, M., Jansen, W. and McLarnon, M. 2002. Guidelines on securing public web servers . NIST Special Publication 800–44. Version 2.
- Travelers Risk Control. 2016. Where is your supply chain the most vulnerable? [Infographic]. Travelers Insurance. https://www.travelers.com/resources/supply-chain-management/where-is-your-supply-chain-most-vulnerable . Accessed 7 Apr 2020.
- U.S. Department of Homeland Security. 2021. DHS announces new cybersecurity requirements for critical pipeline owners and operators. https://www.dhs.gov/news/2021/05/27/hds-announces-new-cybersecurity-requirements-critical-pipeline-owners-and-operators . Accessed 20 July 2021.
- U.S. Department of Homeland Security. 2021b. DHS announces new cybersecurity requirements for critical pipeline owners and operators. https://www.dhs.gov/news/2021/07/20/hds-announces-new-cybersecurity-requirements-critical-pipeline-owners-and-operators . Accessed 20 July 2021.
- U.S. Department of Justice. 2014. Leader of identity theft ring sentenced for stealing more than 600 identities and causing more than $1 million in losses. Office of Public Affairs. https://www.justice.gov/opa/pr/leader-identity-theft-ring-sentenced-stealing-more-600-identities-and-causing-more-1-million . Accessed 3 Mar 2020.
- U.S. Department of Justice. 2017. Russian cyber-criminal sentenced to 14 years in prison for role in organized cybercrime ring responsible for $50 million in online identity theft and $9 million bank fraud conspiracy. Office of Public Affairs. https://www.justice.gov/opa/pr/russian-cyber-criminal-sentenced-14-years-prison-role-organized-cybercrime-ring-responsible . Accessed 3 Mar 2020.
- U.S. Department of Justice. 2021. U.S. government launches first one-stop ransomware resource at StopRansomware.gov. Office of Public Affairs. https://www.justice.gov/opa/pr/us-government-launches-first-one-stop-ransomware-resource-stopransomwaregov . Accessed 15 July 2021.
- U.S. Secret Service. 2020. Secret Service issues COVID-19 (Coronavirus)phishing alert [Press release]. https://www.secretservice.gov/press/releases/2020/03/secret-service-issues-covid-19-coronavirus-phishing-alert . Accessed 7 Apr 2020.
- Verizon. 2020. Data breach investigations report. https://enterprise.verizon.com/resources/rejports/2020/2020-data-breach-investigations-report.pdf . Accessed 8 Dec 2020.
- Willison R, Backhouse J. Opportunities for computer crime: Considering systems risk from a criminological perspective. European Journal of Information Systems. 2006; 15 :403–414. doi: 10.1057/palgrave.ejis.3000592. [ CrossRef ] [ Google Scholar ]
- Willison R, Siponen M. Overcoming the insider: Reducing employee computer crime through situational crime prevention. Communication of the ACM. 2009; 52 (9):133–137. doi: 10.1145/1562164.1562198. [ CrossRef ] [ Google Scholar ]
- Wortley R. A classification of techniques for controlling situational precipitators of crime. Security Journal. 2001; 14 (4):63–82. doi: 10.1057/palgrave.sj.8340098. [ CrossRef ] [ Google Scholar ]
- Xiong A, Proctor RW, Yang W, Li N. Is domain highlighting actually helpful in identifying phishing web pages? Human Factors. 2017; 59 (4):640–660. doi: 10.1177/0018720816684064. [ PubMed ] [ CrossRef ] [ Google Scholar ]
UTA researchers work to prevent AI phishing scams
Friday, Jun 21, 2024 • Brian Lopez : contact
A team of researchers at the University of Texas at Arlington has developed software that prevents artificial intelligence (AI) chatbots such as ChatGPT from creating phishing websites — a growing concern as cybercriminals have been utilizing the technology for designing scams.
Created by Shirin Nilizadeh, assistant professor in the Department of Computer Science and Engineering, and her doctoral students Sayak Saha Roy and Poojitha Thota, the software allows AI chatbots to better detect and reject instruction prompts entered by users that could be used to create phishing websites.
Currently, AI chatbots have some inbuilt detection capabilities, but Dr. Nilizadeh said her team has found loopholes that could easily bypass them and exploit the chatbots to create these attacks. With the emergence of AI chatbots, launching online scams has become highly accessible, even for attackers with minimal technical skills. Now, one does not need coding expertise to create a website, as AI can build one almost instantly.
“These tools are very powerful, and we are showing how they can be misused by attackers,” Nilizadeh said.
To develop their tool, the group initially identified various instruction prompts that could be used to create phishing websites, Saha Roy said. Leveraging this knowledge, they successfully trained their software to recognize and react to those specific keywords and patterns, enhancing its ability to detect and block such malicious prompts from being executed by the chatbots.
The team's work has captured significant attention within the cybersecurity industry, highlighted by their recent publication at the IEEE Symposium on Security and Privacy (IEEE S&P 2024), the premier conference in the field. In May, the researchers not only shared their findings but also received the prestigious Distinguished Paper Award, further underscoring the impact of their research.
“I want people to be receptive to our work and see the risk,” Saha Roy said. “It starts with the security community and trickles down from there.”
The researchers have reached out to the major tech companies that drive these chatbots, including Google and OpenAI, aiming to integrate their findings into broader AI security strategies. Both Saha Roy and Thota expressed a strong commitment to their research's implications for cybersecurity.
“I’m really happy that I was able to work on this important research,” Thota added. “I’m also looking forward to sharing this work with our colleagues in the cybersecurity space and finding ways to further our work.”
Opinion An epidemic of scientific fakery threatens to overwhelm publishers
More than 10,000 scientific papers were retracted last year as “paper mills” exploit the system.
Adam Marcus, editorial director for primary care at Medscape, and Ivan Oransky, editor in chief of the Transmitter and a journalism professor at New York University, are the co-founders of Retraction Watch.
A record number of retractions — more than 10,000 scientific papers in 2023 . Nineteen academic journals shut down recently after being overrun by fake research from paper mills. A single researcher with more than 200 retractions .
The numbers don’t lie: Scientific publishing has a problem, and it’s getting worse. Vigilance against fraudulent or defective research has always been necessary, but in recent years the sheer amount of suspect material has threatened to overwhelm publishers.
We were not the first to write about scientific fraud and problems in academic publishing when we launched Retraction Watch in 2010 with the aim of covering the subject regularly. Back then, coverage of such issues was episodic, and it was routine to have a year or more between major scandals worthy of national or even international attention, such as the spectacular blowup a decade ago of the Italian surgeon Paolo Macchiarini, whose misdeeds (involving the implantation of artificial windpipes) resulted in a prison sentence in Europe and a Netflix docuseries .
Today, reporters jockey for scoops about scientific malfeasance that regularly grace the front pages of major news outlets around the world. In the space of a year, the presidents of Harvard and Stanford have both stepped down amid allegations of research misconduct or plagiarism, and the Dana-Farber Cancer Institute has requested half a dozen retractions and many more corrections.
But it is not just high-profile institutions in the crosshairs. In the past few months, deans of two other universities have resigned following our coverage of allegations regarding problems in their work .
As for the epidemic of retracted papers: The 10,000-plus retractions last year were largely the result of paper mill activity overwhelming a single publisher, Hindawi, a subsidiary of Wiley. Paper mills — shadowy companies that operate in places such as China and Latvia and advertise their services on social media — sell entire papers, authorship slots or citations to a researcher’s work to inflate its seeming importance.
The total number of retractions represents about 1 in 500 published papers , up from about 1 in 5,000 two decades ago. And while many of these papers are on esoteric topics, they are undoubtedly an undercount of the amount of problematic research that exists.
So what’s happening? Is fraud on the rise? Or is something finally being done to police it?
Although only a hugely time-consuming and expensive audit of literature over the decades could determine whether fraud is indeed becoming more common, research misconduct clearly is being industrialized on an unprecedented scale.
Paper mills in particular are seizing on the opportunities that a “publish or perish” academic culture provides. Publishers have been aware of, but largely ignored, these schemes for at least a decade , but their thirst for growth and profits — around $2.2 billion last year for Elsevier alone — has led them to tolerate, and even incentivize, such activity.
Mills appear to have exploited a blind spot for major publishers: highly profitable but poorly monitored special issues, which have helped bloat the number of papers they release each year to nearly 3 million . Many of these articles — including some on truly bizarre ideas, such as linking aerobics and dance training to geology — are never cited by other researchers and possibly read by no one. (And, thanks to the rise of generative AI, an increasing percentage likely were not written by humans.)
Only recently, after more mainstream journalists took an interest in paper mills and related dubious endeavors, did Elsevier and other titans such as Springer Nature and Wiley begin acknowledging their existence, while claiming victim status instead of admitting they were complicit in creating business models and incentives that promoted such behavior. In the meantime, paper mills have been bribing journal editors to publish their clients’ work. That and other misconduct continues to plague the literature.
Thankfully, a small army of volunteer sleuths have made it their mission to alert the world to problematic papers. Such people are at risk, however, of being sued by the targets of their scrutiny when they make their findings public. And yet public scrutiny seems to be the only way to prod publishers to pay attention.
The public may believe scientific fraud and sloppiness happens because, say, Big Pharma pushes corrupt studies in their thirst for profits. Cases like that of Cassava Sciences, whose experimental drug for Alzheimer’s disease has been dogged by allegations of fraudulent data, help reinforce that perception.
Meanwhile, some critics accuse scientists of bending the truth to win government grants. That seems to be a theme of angry debates over the origins of the pandemic-causing coronavirus . It’s also true that producing findings that make funders and supporters happy can be a recipe for success.
But in reality, we see far fewer retractions of papers reporting such work than we do of more purely academic studies. Research sponsored by pharmaceutical firms is closely vetted by regulators, whose scrutiny of the data tends to give would-be fakers pause. (An entirely different but no less significant issue is drug companies’ habit of burying studies that don’t reflect well on the products they’re testing.)
The much more important reason researchers cut corners and cheat is bureaucratic: rankings. University rankings such as Times Higher Education’s rely heavily on the number of citations gained by work produced by the institution’s researchers. Yet accumulating citations can be relatively easy to game. The more researchers publish, the more they can be cited — and given current business models, in which authors pay journals anywhere from hundreds of dollars to more than $10,000 to publish their papers and make them available without a subscription, the more publishers earn. When universities move up the rankings, the more top-tier students and faculty they attract — along with more funding.
Finding and flagging fraudulent work is an essential job. But it is akin to building more and more sewage treatment plants at the mouths of rivers to prevent ocean pollution. Building more “plants” is urgently needed, but so is changing the incentives — in this case, publish or perish — so that waste doesn’t get into the river far upstream.
Here are three fixes that could have far-reaching impact. First, give government agencies such as the Office of Research Integrity, which has oversight of research funded by the National Institutes of Health, more teeth and better funding. Sen. Bill Cassidy (R-La.) proposed doing that just last month. Second, the too-easily-gamed practice of counting a paper’s citations by other researchers as a metric of quality should be abandoned. Lastly, scientific journals — however painful this might be — should retire the pay-for-play business model that, by charging researchers to publish their work, has the effect of putting the veneer of legitimacy up for sale.
Science is being polluted, and science must fight back.
About guest opinion submissions
The Washington Post accepts opinion articles on any topic. We welcome submissions on local, national and international issues. We publish work that varies in length and format, including multimedia. Submit a guest opinion or read our guide to writing an opinion article .

19 Types of Phishing Attacks
Understand what phishing is and how to recognize different types of phishing attacks.
- Get A Threat Assessment
- Read the Outbreak Alerts Report

Different Types of Phishing Attacks
Phishing involves an attacker trying to trick someone into providing sensitive account or other login information online. All the different types of phishing are designed to take advantage of the fact that so many people do business over the internet. This makes phishing one of the most prevalent cybersecurity threats around, rivaling distributed denial-of-service (DDoS) attacks , data breaches , and many kinds of malware .
Knowing the different types of phishing attacks can equip you to protect your organization from each.
1. Spear phishing
Spear phishing involves targeting a specific individual in an organization to try to steal their login credentials . The attacker often first gathers information about the person before starting the attack, such as their name, position, and contact details.
Example of spear phishing
An attacker tried to target an employee of NTL World , which is a part of the Virgin Media company, using spear phishing. The attacker claimed that the victim needed to sign a new employee handbook. This was designed to lure them into clicking a link where they would have been asked to submit private information.
Vishing , which is short for "voice phishing," is when someone uses the phone to try to steal information. The attacker may pretend to be a trusted friend or relative or to represent them.
Example of vishing
In 2019, there was a vishing campaign that targeted members of the UK’s parliament and their staffers. The attack was part of an assault that involved at least 21 million spam emails targeting UK lawmakers.
3. Email phishing
In an email phishing scam, the attacker sends an email that looks legitimate, designed to trick the recipient into entering information in reply or on a site that the hacker can use to steal or sell their data.
Example of email phishing
Hackers used LinkedIn to grab contact information from employees at Sony and targeted them with an email phishing campaign. They got away with over 100 terabytes of data.
4. HTTPS phishing
An HTTPS phishing attack is carried out by sending the victim an email with a link to a fake website. The site may then be used to fool the victim into entering their private information.
Example of HTTPS phishing
Hacker group Scarlet Widow searches for the employee emails of companies and then targets them with HTTPS phishing. When the user gets a mostly empty email, they click on the little link that is there, taking the first step into Scarlet Widow's web.
5. Pharming
In a pharming attack, the victim gets malicious code installed on their computer. This code then sends the victim to a fake website designed to gather their login credentials.
Example of pharming
In 2007, a complex pharming attack went after at least 50 financial institutions across the world. Users were directed to false websites and instructed to enter sensitive information.
6. Pop-up phishing
Pop-up phishing often uses a pop-up about a problem with your computer’s security or some other issue to trick you into clicking. You are then directed to download a file, which ends up being malware, or to call what is supposed to be a support center.
Example of pop-up phishing
Users have sometimes received pop-ups saying they can qualify for AppleCare renewal , which would supposedly avail them of extended protection for their Apple devices. However, the offer is fake.
7. Evil twin phishing
In an evil twin attack, the hacker sets up a false Wi-Fi network that looks real. If someone logs in to it and enters sensitive details, the hacker captures their info.
Example of evil twin phishing
A Russian military agency called GRU was recently charged with executing evil twin attacks using fake access points . The access points were made to look like they provided connections to real networks when in reality they led users to sites that stole their credentials or downloaded malware onto their computers.
8. Watering hole phishing
In a watering hole phishing attack , a hacker figures out a site a group of users tends to visit. They then use it to infect the users’ computers in an attempt to penetrate the network.
Example of watering hole phishing
In 2012, the U.S. Council on Foreign Relations was targeted by a watering hole attack . The assault aimed to take advantage of the high-profile users that were frequenting the site, as well as the login credentials they could provide. The attack achieved some success, particularly using a vulnerability within Internet Explorer.
A whaling attack is a phishing attack that targets a senior executive. These individuals often have deep access to sensitive areas of the network, so a successful attack can result in access to valuable info.
Example of whaling
A founder of Levitas, an Australian hedge fund was the target of a whaling attack that led the individual to a fake connection using a fraudulent Zoom link. After following the link, they had malware installed on their system, and the company lost $800.000 .
10. Clone phishing
A clone phishing attack involves a hacker making an identical copy of a message the recipient already received. They may include something like “resending this” and put a malicious link in the email.
Example of clone phishing
In a recent attack, a hacker copied the information from a previous email and used the same name as a legitimate contact that had messaged the victim about a deal. The hacker pretended to be a CEO named Giles Garcia and referenced the email Mr. Garcia had previously sent. The hacker then proceeded to pretend to carry on the previous conversation with the target, as if they really were Giles Garcia.
11. Deceptive phishing
Deceptive phishers use deceptive technology to pretend they are with a real company to inform the targets they are already experiencing a cyberattack. The users then click on a malicious link, infecting their computer.
Example of deceptive phishing
Users were sent emails that came from the address [email protected] and had “Apple Support” in the sender information. The message claimed that the victim’s Apple ID had been blocked. They were then prompted to validate their accounts by entering information the hacker would use to crack it.
12. Social engineering
Social engineering attacks pressure someone into revealing sensitive information by manipulating them psychologically.
Example of social engineering
A hacker pretended to be a representative of Chase Bank while saying that the action was needed on the target’s debit or ATM card. The attacker was trying to pressure the victim into divulging their information by leveraging their fear of not being able to access their money in their Chase account.
13. Angler phishing
Anglers use fake social media posts to get people to provide login info or download malware.
Example of angler phishing
Hackers pretended to represent Domino's Pizza on Twitter, fielding the concerns and comments of customers. Once they engaged with a customer, they would use their situation to try to get their personal information—using the guise of trying to get them a refund or a reward.
14. Smishing
Smishing is phishing through some form of a text message or SMS.
Example of smishing
Hackers pretended to be from American Express and sent text messages to their victims telling them they needed to tend to their accounts. The message said it was urgent, and if the victim clicked, they would be taken to a fake site where they would enter their personal information.
15. Man-in-the-middle (MiTM) attacks
With a man-in-the-middle attack , the hacker gets in “the middle” of two parties and tries to steal information exchanged between them, such as account credentials.
Example of man-in-the-middle attack
In 2017, Equifax, the popular credit score company, was targeted by man-in-the-middle attacks that victimized users who used the Equifax app without using HTTPS, which is a secure way to browse the internet. As the users accessed their accounts, the hackers intercepted their transmissions, stealing their login credentials.
16. Website spoofing
With website spoofing, a hacker creates a fake website that looks legitimate. When you use the site to log in to an account, your info is collected by the attacker.
Example of website spoofing
Hackers made a fake Amazon website that looked nearly identical to the real Amazon.com but had a different Uniform Resource Locator (URL) . All other details, including fonts and images, looked legitimate. Attackers were hoping that users would put in their username and password.
17. Domain spoofing
Domain spoofing, also referred to as DNS spoofing, is when a hacker imitates the domain of a company—either using email or a fake website—to lure people into entering sensitive information. To prevent domain spoofing , you should double-check the source of every link and email.
Example of domain spoofing
An attacker would execute a domain spoofing attack by creating a fraudulent domain made to look like a real LinkedIn site, for example. When users go to the site and enter any information, it is sent straight to hackers who could use it or sell it to someone else.
18. Image phishing
Image phishing uses images with malicious files in them meant to help a hacker steal your account info or infect your computer.
Example of image phishing
Hackers have made use of AdGholas to hide malicious code written in JavaScript inside images and HTML files. When someone clicked on an image generated by AdGholas, malware would be downloaded onto their computer that could be used to phish for their personal information.
19. Search engine phishing
A search engine phishing attack involves an attacker making fake products that look attractive. When these pop up in a search engine, the target is asked to enter sensitive information before purchasing, which then goes to a hacker.
Example of search engine phishing
In 2020, Google said that they found 25 billion spam pages every day, like the one put up by hackers pretending to be from the travel company Booking.com . An ad would pop up in users’ search results that looked like it was from booking.com and included the site’s address and the kind of wording users would expect from a real ad by the company. After users clicked, they were prompted to enter sensitive login information that was then transmitted to hackers.
Get a Cybersecurity Threat Assessment
Know your vulnerabilities - get the facts about your security risk and at no cost.
Phishing FAQs
What is whaling, what is smishing, what is website spoofing, what is deceptive phishing.
Deceptive phishers use deceptive technology to pretend they are with a real company to inform the targets they are already experiencing a cyberattack.
Cybersecurity Resources
- Cybersecurity
- Types of Cyber Attacks
- IT vs OT Cybersecurity
- AI Cybersecurity
- Cyber Threat Intelligence
- Cybersecurity Management
- Network Security
- Data Security
- Email Security
- Endpoint Security
- Web Security
- Enterprise Security
- Cybersecurity Mesh
Quick Links
- Fortinet Products
- Fortinet Demos
- Analyst Reports
Speak with an Expert
Please fill out the form and a knowledgeable representative will get in touch with you soon.
By clicking submit you agree to the Fortinet Terms and Conditions & Privacy Policy .
A comprehensive survey of phishing: mediums, intended targets, attack and defence techniques and a novel taxonomy
- Regular Contribution
- Published: 19 October 2023
- Volume 23 , pages 819–848, ( 2024 )
Cite this article
- Richa Goenka 1 ,
- Meenu Chawla 1 &
- Namita Tiwari 1
1228 Accesses
3 Citations
Explore all metrics
The recent surge in phishing incidents in the post-COVID era poses a serious threat towards the social and economic well-being of users. The escalation in dependency upon the internet for meeting daily chores has made them vulnerable to falling prey to the ever-evolving menace of phishing. The objective of this article is: to explore different tactics and motivational factors behind phishing, identify the communication mediums through which phishing is circulated and perform a detailed review along with a comparison of the various surveys in this domain. Another objective is to determine the open research challenges in this genre and to identify the scope of research in the future. An extensive literature survey is performed, which includes articles from eminent online research databases. Barring a few initial articles related to phishing, the articles published in Science Citation/Scopus-indexed journals and survey/review articles published in the last ten years are considered. Highly cited works are given preference. The search query returned numerous articles, which were narrowed by title screening. Further screening of articles was performed by reading the abstract and eliminating the articles related to user-oriented phishing interventions. Eventually, 25 survey articles were shortlisted to be surveyed. This article is an effort to provide a novel taxonomy of phishing to academia that would assist in identifying the sections where phishing countermeasures are inadequate.
This is a preview of subscription content, log in via an institution to check access.
Access this article
Price includes VAT (Russian Federation)
Instant access to the full article PDF.
Rent this article via DeepDyve
Institutional subscriptions
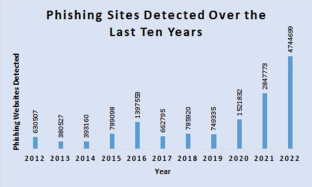
Similar content being viewed by others
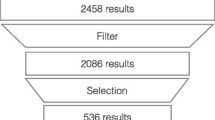
Achieving a consensual definition of phishing based on a systematic review of the literature
Awareness of Phishing Attacks in the Public Sector: Review Types and Technical Approaches
Unpacking Spear Phishing Susceptibility
Data availability.
Since this work is a survey, no datasets were created or analysed. Hence, data sharing is not applicable.
Williams, E.J., Hinds, J., Joinson, A.N.: Exploring susceptibility to phishing in the workplace. Int. J. Hum. Comput. Stud. 120 , 1–13 (2018)
Article Google Scholar
Maroofi, S., Korczyński, M., Hölzel, A., Duda, A.: Adoption of email anti-spoofing schemes: a large scale analysis. IEEE Trans. Netw. Serv. Manag. 18 (3), 3184–3196 (2021)
Pandey, N., Pal, A., et al.: Impact of digital surge during COVID-19 pandemic: a viewpoint on research and practice. Int. J. Inf. Manag. 55 , 102171 (2020)
Beech, F.M..: Covid-19 pushes up internet use 70% and streaming more than 12%, first figures reveal. https://www.forbes.com/sites/markbeech/2020/03/25/covid-19-pushes-up-internet-use-70-streaming-more-than-12-first-figures-reveal/?sh=1e813ced3104 , (May 2020). Accessed June 2022
Akala, A.: More big employers are talking about permanent work-from-home positions. https://www.cnbc.com/2020/05/01/major-companies-talking-about-permanent-work-from-home-positions.html . Accessed June 2022
BBC News. Twitter allows staff to work from home “forever”. https://www.bbc.com/news/technology-52628119 (2020). Accessed June 2022
APWG. Phishing activity trends report-4th quarter (2022). https://apwg.org/trendsreports/ . Accessed July 2023
Abroshan, H., Devos, J., Poels, G., Laermans, E.: Covid-19 and phishing: effects of human emotions, behavior, and demographics on the success of phishing attempts during the pandemic. IEEE Access 9 , 121916–121929 (2021)
Lallie, H.S., Shepherd, L.A., Nurse, J.R.C., Erola, A., Epiphaniou, G., Maple, C., Bellekens, X.: Cyber security in the age of Covid-19: a timeline and analysis of cyber-crime and cyber-attacks during the pandemic. Comput. Secur. 105 , 102248 (2021)
BNP Media T. Kelly: How hackers are using Covid-19 to find new phishing victims. https://www.securitymagazine.com/articles/92666-how-hackers-are-using-covid-19-to-find-new-phishing-victims . Accessed June 2022
Cision: Phishing in a pandemic: 1 in 4 Americans received a Covid-19 related phishing email. https://www.prnewswire.com/news-releases/phishing-in-a-pandemic-1-in-4-americans-received-a-covid-19-related-phishing-email-301134037.html (2021). Accessed June 2022
Security Boulevard: Phishing statistics: the 29 latest phishing stats to know in 2020. https://securityboulevard.com/2020/04/phishing-statistics-the-29-latest-phishing-stats-to-know-in-2020/ . Accessed June 2022
APWG: Phishing activity trends report-1st quarter 2020. https://docs.apwg.org/reports/apwg_trends_report_q1_2020.pdf?_ga=2.30422460.2018635328.1665064249-1448730527.1654753557 &_gl=1*a4rx10*_ga*MTQ0ODczMDUyNy4xNjU0NzUzNTU3*_ga_55RF0RHXSR*MTY2NTA2NDI0OC4xNS4xLjE2NjUwNjQ1MDYuMC4wLjA . Accessed April 2022
Stu Sjouwerman: Q1 2020 coronavirus-related phishing email attacks are up 600%. https://blog.knowbe4.com/q1-2020-coronavirus-related-phishing-email-attacks-are-up-600 . Accessed 15 Jan 2022
FBI: Internet crime report. https://www.ic3.gov/Media/PDF/AnnualReport/2020_IC3Report.pdf . Accessed 16 Feb 2022
Jakobsson, M., Myers, S.: Phishing and Countermeasures: Understanding the Increasing Problem of Electronic Identity Theft. Wiley, New York (2006)
Book Google Scholar
Ollmann, G.: The phishing guide understanding & preventing phishing attacks. NGS Software Insight Security Research (2004)
Ramzan, Z.: Phishing Attacks and Countermeasures, pp. 433–448. Springer, Berlin (2010)
Google Scholar
Khonji, M., Iraqi, Y., Jones, A.: Phishing detection: a literature survey. IEEE Commun. Surv. Tutor. 15 (4), 2091–2121 (2013)
Almomani, A., Gupta, B.B., Atawneh, S., Meulenberg, A., Almomani, E.: A survey of phishing email filtering techniques. IEEE Commun. Surv. Tutor. 15 (4), 2070–2090 (2013)
Mohammad, R.M., Thabtah, F., McCluskey, L.: Tutorial and critical analysis of phishing websites methods. Comput. Sci. Rev. 17 , 1–24 (2015)
Article MathSciNet Google Scholar
Tewari, A., Jain, A.K., Gupta, B.B.: Recent survey of various defense mechanisms against phishing attacks. J. Inf. Priv. Secur. 12 (1), 3–13 (2016)
Varshney, G., Misra, M., Atrey, P.K.: A survey and classification of web phishing detection schemes. Secur. Commun. Netw. 9 (18), 6266–6284 (2016)
Aleroud, A., Zhou, L.: Phishing environments, techniques, and countermeasures: A survey. Comput. Secur. 68 , 160–196 (2017)
Gupta, B.B., Tewari, A., Jain, A.K., Agrawal, D.P.: Fighting against phishing attacks: state of the art and future challenges. Neural Comput. Appl. 28 (12), 3629–3654 (2017)
Dou, Z., Khalil, I., Khreishah, A., Al-Fuqaha, A., Guizani, M.: Systematization of knowledge (SOK): a systematic review of software-based web phishing detection. IEEE Commun. Surv. Tutor. 19 (4), 2797–2819 (2017)
Chiew, K.L., Yong, K.S.C., Tan, C.L.: A survey of phishing attacks: their types, vectors and technical approaches. Expert Syst. Appl. 106 , 1–20 (2018)
Qabajeh, I., Thabtah, F., Chiclana, F.: A recent review of conventional vs. automated cybersecurity anti-phishing techniques. Comput. Sci. Rev. 29 , 44–55 (2018)
Das, A., Baki, S., El Aassal, A., Verma, R., Dunbar, A.: Sok: a comprehensive reexamination of phishing research from the security perspective. IEEE Commun. Surv. Tutor. 22 (1), 671–708 (2019)
Akinyelu, A.A.: Machine learning and nature inspired based phishing detection: a literature survey. Int. J. Artif. Intell. Tools 28 (05), 1930002 (2019)
Alabdan, R.: Phishing attacks survey: types, vectors, and technical approaches. Future Internet 12 (10), 168 (2020)
Gangavarapu, T., Jaidhar, C.D., Chanduka, B.: Applicability of machine learning in spam and phishing email filtering: review and approaches. Artif. Intell. Rev. 53 (7), 5019–5081 (2020)
Vijayalakshmi, M., Shalinie, S.M., Yang, M.H., Meenakshi, U.R.: Web phishing detection techniques: a survey on the state-of-the-art, taxonomy and future directions. IET Netw. 9 (5), 235–246 (2020)
Lee, J., Lee, Y., Lee, D., Kwon, H., Shin, D.: Classification of attack types and analysis of attack methods for profiling phishing mail attack groups. IEEE Access 9 , 80866–80872 (2021)
Alkhalil, Z., Hewage, C., Nawaf, L., Khan, I.: Phishing attacks: a recent comprehensive study and a new anatomy. Front. Comput. Sci. 3 , 563060 (2021)
Jain, A.K., Gupta, B.B.: A survey of phishing attack techniques, defence mechanisms and open research challenges. Enterp. Inf. Syst. 16 (4), 527–565 (2022)
Al-Qahtani, A.F., Cresci, S.: The COVID-19 scamdemic: a survey of phishing attacks and their countermeasures during COVID-19. IET Inf. Secur. 16 (5), 324–345 (2022)
Basit, A., Zafar, M., Liu, X., Javed, A.R., Jalil, Z., Kifayat, K.: A comprehensive survey of ai-enabled phishing attacks detection techniques. Telecommun. Syst. 76 (1), 139–154 (2021)
Salloum, S., Gaber, T., Vadera, S., Sharan, K.: A systematic literature review on phishing email detection using natural language processing techniques. IEEE Access (2022)
Abdillah, R., Shukur, Z., Mohd, M., Murah, M.Z.: A systematic literature review on phishing classification techniques . IEEE Access (2022)
Rekouche, K.: Early phishing. arXiv preprint arXiv:1106.4692 (2011)
BBC News: Twitter hack: staff tricked by phone spear-phishing scam. https://www.bbc.com/news/technology-53607374 . Accessed Jan 2022
Twitter: An update on our security incident. https://blog.twitter.com/en_us/topics/company/2020/an-update-on-our-security-incident (2020). Accessed Jan 2022
CNBC: How this scammer used phishing emails to steal over \$100 million from google and facebook. https://www.cnbc.com/2019/03/27/phishing-email-scam-stole-100-million-from-facebook-and-google.html (2019). Accessed Jan 2022
Reuters: Austria’s facc, hit by cyber fraud, fires CEO. https://www.reuters.com/article/us-facc-ceo-idUSKCN0YG0ZF (2016). Accessed Jan 2022
SecurityIntelligence: Ibm uncovers global phishing campaign targeting the covid-19 vaccine cold chain. https://securityintelligence.com/posts/ibm-uncovers-global-phishing-covid-19-vaccine-cold-chain/ . Accessed Jan 2022
Weider, D.Yu., Nargundkar, S., Tiruthani, N.: A phishing vulnerability analysis of web based systems. In: 2008 IEEE Symposium on Computers and Communications, pp. 326–331. IEEE (2008)
Nazah, S., Huda, S., Abawajy, J., Hassan, M.M.: Evolution of dark web threat analysis and detection: a systematic approach. IEEE Access 8 , 171796–171819 (2020)
Bates, R.A.: Tracking lone wolf terrorists. J. Public Prof. Sociol. 8 (1), 6 (2016)
Weimann, G.: Going dark: terrorism on the dark web. Stud. Conf. Terror. 39 (3), 195–206 (2016)
E-ISAC and SANS: Analysis of the cyber attack on the Ukrainian power grid. https://media.kasperskycontenthub.com/wp-content/uploads/sites/43/2016/05/20081514/E-ISAC_SANS_Ukraine_DUC_5.pdf . Accessed Jan 2022
Verizon: Dbir:data breach investigations report. https://www.verizon.com/business/resources/Tcd0/reports/dbir/2022-data-breach-investigations-report-dbir.pdf (2022). Accessed Dec 2022
SOPHOS: The state of ransomware 2022. https://assets.sophos.com/X24WTUEQ/at/4zpw59pnkpxxnhfhgj9bxgj9/sophos-state-of-ransomware-2022-wp.pdf . Accessed Oct 2022
Hull, G., John, H., Arief, B.: Ransomware deployment methods and analysis: views from a predictive model and human responses. Crime Sci. 8 (1), 1–22 (2019)
Damopoulos, D., Kambourakis, G., Gritzalis, S.: From keyloggers to touchloggers: take the rough with the smooth. Comput. Secur. 32 , 102–114 (2013)
Statista: Number of smartphone subscriptions worldwide from 2016 to 2021, with forecasts from 2022 to 2027. https://www.statista.com/statistics/330695/number-of-smartphone-users-worldwide/ (2022). Accessed June 2022
APWG: Phishing activity trends report-2nd quarter 2022. https://docs.apwg.org/reports/apwg_trends_report_q2_2022.pdf?_ga=2.45552807.263073049.1665252062-1448730527.1654753557 &_gl=1*14k5jc7*_ga*MTQ0ODczMDUyNy4xNjU0NzUzNTU3*_ga_55RF0RHXSR*MTY2NTI1ODU1NS4xOS4xLjE2NjUyNTg1NTkuMC4wLjA . Accessed April 2022
Diksha Goel and Ankit Kumar Jain: Mobile phishing attacks and defence mechanisms: state of art and open research challenges. Comput. Secur. 73 , 519–544 (2018)
Jain, A.K., Debnath, N., Jain, A.K.: APuML: an efficient approach to detect mobile phishing webpages using machine learning. Wirel. Pers. Commun. 125 (4), 3227–3248 (2022)
Shahriar, H., Klintic, T., Clincy, V., et al.: Mobile phishing attacks and mitigation techniques. J. Inf. Secur. 6 (03), 206 (2015)
Felt, A.P., Wagner, D.: Phishing on mobile devices (2011)
Business2Community: SMS marketing: texting your way to success. https://www.business2community.com/digital-marketing/sms-marketing-texting-your-way-to-success-02388639 . Accessed June 2022
Mishra, S., Soni, D.: Smishing detector: a security model to detect smishing through SMS content analysis and URL behavior analysis. Futur. Gener. Comput. Syst. 108 , 803–815 (2020)
Jakobsson, M.: The human factor in phishing. Privacy Security of Consumer Information (2007)
Singh, H.P., Singh, S., Singh, J., Khan, S.A.: VoIP: state of art for global connectivity—a critical review. J. Netw. Comput. Appl. 37 , 365–379 (2014)
Mustafa, H., Wenyuan, X., Sadeghi, A.-R., Schulz, S.: End-to-end detection of caller id spoofing attacks. IEEE Trans. Depend. Secure Comput. 15 (3), 423–436 (2016)
DENSO WAVE INCORPORATED. History of QR code. https://www.qrcode.com/en/history/ . Accessed Dec 2021
Lin, P.-Y., Chen, Y.-H.: High payload secret hiding technology for QR codes. EURASIP J. Image Video Process. 2017 (1), 1–8 (2017)
Dabrowski, A., Krombholz, K., Ullrich, J., Weippl, E.R.: QR inception: barcode-in-barcode attacks. In: Proceedings of the 4th ACM Workshop on Security and Privacy in Smartphones & Mobile Devices, pp. 3–10 (2014)
Vidas, T., Owusu, E., Wang, S., Zeng, C., Cranor, L.F., Christin, N.: Qrishing: the susceptibility of smartphone users to QR code phishing attacks. In: International Conference on Financial Cryptography and Data Security, pp. 52–69. Springer (2013)
Focardi, R., Luccio, F.L., Wahsheh, H.A.M.: Security threats and solutions for two-dimensional barcodes: a comparative study. In: Computer and Network Security Essentials, pp. 207–219. Springer (2018)
Verizon: Dbir:data breach investigations report. https://www.verizon.com/business/resources/reports/2020-data-breach-investigations-report.pdf (2020). Accessed Dec 2021
PhishLabs: Social media attacks doubled in 2021 according to latest phishlabs report. https://www.phishlabs.com/news/social-media-attacks-doubled-in-2021-according-to-latest-phishlabs-report/ (2022). Accessed 3 Sept 2022
Jagatic, T.N., Johnson, N.A., Jakobsson, M., Menczer, F.: Social phishing. Commun. ACM 50 (10), 94–100 (2007)
Cisco: Cybersecurity threat trends: phishing, crypto top the list. https://learn-umbrella.cisco.com/ebook-library/2021-cyber-security-threat-trends-phishing-crypto-top-the-list (2021). Accessed 3 Sept 2022
Avanan: 1h cyber attack report. https://www.avanan.com/hubfs/Content/Collateral/1H-Cyber-Attack-Report.pdf (2021). Accessed 3 Sept 2022
Statista: Number of internet and social media users worldwide as of July 2022. https://www.statista.com/statistics/617136/digital-population-worldwide/ (2022). Accessed 3 Sept 2022
Statista: 16% of all facebook accounts are fake or duplicates. https://www.statista.com/chart/20685/duplicate-and-false-facebook-accounts/ (2020). Accessed 20 Aug 2022
Song, Y., Yang, C., Gu, G.: Who is peeping at your passwords at starbucks? To catch an evil twin access point. In: 2010 IEEE/IFIP International Conference on Dependable Systems & Networks (DSN), pp. 323–332. IEEE (2010)
Atzori, L., Iera, A., Morabito, G.: The internet of things: a survey. Comput. Netw. 54 (15), 2787–2805 (2010)
Sharma, R., Mahapatra, R. P., Sharma, N.: The internet of things and its applications in cyber security. In: A Handbook of Internet of Things in Biomedical and Cyber Physical System, pp. 87–108 (2020)
Nirmal, K., Janet, B., Kumar, R.: Analyzing and eliminating phishing threats in IoT, network and other web applications using iterative intersection. Peer-to-Peer Netw. Appl. 14 , 2327–2339 (2021)
Tewari, A., Gupta, B.B.: Security, privacy and trust of different layers in internet-of-things (IoTs) framework. Future Gener. Comput. Syst. 108 , 909–920 (2020)
PaloAlto Networks. 2020 unit 42 IoT threat report. https://start.paloaltonetworks.com/unit-42-iot-threat-report . Accessed July 2023
Caputo, D.D., Pfleeger, S.L., Freeman, J.D., Johnson, M.E.: Going spear phishing: exploring embedded training and awareness. IEEE Secur. Priv. 12 (1), 28–38 (2013)
Parmar, B.: Protecting against spear-phishing. Comput. Fraud Secur. 2012 (1), 8–11 (2012)
Wang, J., Herath, T., Chen, R., Vishwanath, A., Rao, H.R.: Research article phishing susceptibility: an investigation into the processing of a targeted spear phishing email. IEEE Trans. Prof. Commun. 55 (4), 345–362 (2012)
Symantec: Istr:internet security threat report. https://docs.broadcom.com/doc/istr-24-2019-en (2019). Accessed 23 Apr 2022
Kwak, Y., Lee, S., Damiano, A., Vishwanath, A.: Why do users not report spear phishing emails? Telemat. Inf. 48 , 101343 (2020)
Al-Musib, N.S., Al-Serhani, F.M., Humayun, M., Jhanjhi, N.Z.: Business email compromise (BEC) attacks. Mater. Today Proc. (2021)
FBI. Public service announcement. https://www.ic3.gov/Media/Y2022/PSA220504 (2022). Accessed 23 Apr 2022
FBI. Public service announcement. https://www.ic3.gov/Media/Y2022/PSA220216 (2022). Accessed 23 Apr 2022
Le Page, S., Jourdan, G.-V.: Victim or attacker? A multi-dataset domain classification of phishing attacks. In: 2019 17th International Conference on Privacy, Security and Trust (PST), pp. 1–10. IEEE (2019)
Corona, I., Biggio, B., Contini, M., Piras, L., Corda, R., Mereu, M., Mureddu, G., Ariu, D., Roli, F.: Deltaphish: detecting phishing webpages in compromised websites. In: European Symposium on Research in Computer Security, pp. 370–388. Springer (2017)
Moore, T., Clayton, R.: Examining the impact of website take-down on phishing. In: Proceedings of the anti-phishing working groups 2nd annual eCrime researchers summit, pp. 1–13 (2007)
PhishLabs. Most phishing attacks use compromised domains and free hosting. https://www.phishlabs.com/blog/most-phishing-attacks-use-compromised-domains-and-free-hosting/ (2021). Accessed 14 Feb 2022
Pope, M.B., Warkentin, M., Mutchler, L.A., Luo, X.R.: The domain name system-past, present, and future. Commun. Assoc. Inf. Syst. 30 (1), 21 (2012)
Kim, H., Huh, J.H.: Detecting DNS-poisoning-based phishing attacks from their network performance characteristics. Electron. Lett. 47 (11), 656–658 (2011)
Perdisci, R., Antonakakis, M., Luo, X., Lee, W.: WSEC DNS: protecting recursive DNS resolvers from poisoning attacks. In: 2009 IEEE/IFIP International Conference on Dependable Systems & Networks, pp. 3–12. IEEE (2009)
Schiller, C.A., Binkley, J., Harley, D., Evron, G., Bradley, T., Willems, C., Cross, M.: Botnets overview. In: Botnets, pp. 29–75. Syngress (2007)
Vural, I., Venter, H.: Detecting mobile spam botnets using artificial immune systems. In: IFIP International Conference on Digital Forensics, pp. 183–192. Springer (2011)
Negash, N., Che, X.: An overview of modern botnets. Inf. Secur. J. Glob. Perspect. 24 (4–6), 127–132 (2015)
Milletary, J., CERT Coordination Center.: Technical trends in phishing attacks. Retrieved December 1(2007):3 (2005)
Gupta, S., Gupta, B.B.: Cross-site scripting (XSS) attacks and defense mechanisms: classification and state-of-the-art. Int. J. Syst. Assur. Eng. Manag. 8 (1), 512–530 (2017)
Ruderman, J.: The same origin policy. http://www.mozilla.org/projects/security/components/same-origin.html (2001)
Patchstack. State of wordpress security in 2021. https://patchstack.com/wp-content/uploads/2022/03/Patchstack-%E2%80%93-State-Of-WordPress-Security-In-2021.pdf (2022). Accessed 15 May 2022
Nagar, N., Suman, U.: Prevention, detection, and recovery of CSRF attack in online banking system. In: Online banking security measures and data protection, pp. 172–188. IGI Global (2017)
Zhang, J., Hu, H., Huo, S.: A browser-based cross site request forgery detection model. J. Phys. Conf. Ser. 1738 , 012073 (2021)
Gelernter, N., Herzberg, A.: Tell me about yourself: the malicious captcha attack. In: Proceedings of the 25th International Conference on World Wide Web, pp. 999–1008 (2016)
Yalçın, N., Köse, U.: What is search engine optimization: Seo? Procedia Soc. Behav. Sci. 9 , 487–493 (2010)
Chaudhry, J.A., Chaudhry, S.A., Rittenhouse, R.G.: Phishing attacks and defenses. Int. J. Secur. Appl. 10 (1), 247–256 (2016)
Nagunwa, T.: Behind identity theft and fraud in cyberspace: the current landscape of phishing vectors. Int. J. Cyber-Secur. Digit. Forensics IJCSDF 3 (1), 72–83 (2014)
van der Toorn, O., Müller, M., Dickinson, S., Hesselman, C., Sperotto, A., van Rijswijk-Deij, R.: Addressing the challenges of modern DNS a comprehensive tutorial. Comput. Sci. Rev. 45 , 100469 (2022)
Wang, Y.-M., Beck, D., Wang, J., Verbowski, C., Daniels, B.: Strider typo-patrol: discovery and analysis of systematic typo-squatting. SRUTI 6 (31–36), 2–2 (2006)
Spaulding, J., Nyang, D., Mohaisen, A.: Understanding the effectiveness of typosquatting techniques. In: Proceedings of the fifth ACM/IEEE Workshop on Hot Topics in Web Systems and Technologies, pp. 1–8 (2017)
Moore, T., Edelman, B.: Measuring the perpetrators and funders of typosquatting. In: International Conference on Financial Cryptography and Data Security, pp. 175–191. Springer (2010)
Dinaburg, A.: Bitsquatting: Dns hijacking without exploitation (2011)
Nikiforakis, N., Balduzzi, M., Desmet, L., Piessens, F., Joosen, W.: Soundsquatting: uncovering the use of homophones in domain squatting. In: International Conference on Information Security, pp. 291–308. Springer (2014)
Holgers, T., Watson, D.E., Gribble, S.D.: Cutting through the confusion: a measurement study of homograph attacks. In: USENIX Annual Technical Conference, General Track, pp. 261–266 (2006)
Kintis, P., Miramirkhani, N., Lever, C., Chen, Y., Romero-Gómez, R., Pitropakis, N., Nikiforakis, N., Antonakakis, M.: Hiding in plain sight: a longitudinal study of combosquatting abuse. In: Proceedings of the 2017 ACM SIGSAC Conference on Computer and Communications Security, pp. 569–586 (2017)
Zeng, Y., Zang, T., Zhang, Y., Chen, X., Wang, Y.: A comprehensive measurement study of domain-squatting abuse. In: ICC 2019-2019 IEEE International Conference on Communications (ICC), pp. 1–6. IEEE (2019)
Nikiforakis, N., Van Acker, S., Meert, W., Desmet, L., Piessens, F., Joosen, W.: Bitsquatting: exploiting bit-flips for fun, or profit? In: Proceedings of the 22nd international conference on World Wide Web, pp. 989–998 (2013)
Rader, M., Rahman, S.: Exploring historical and emerging phishing techniques and mitigating the associated security risks. arXiv preprint arXiv:1512.00082 (2015)
Skolka, P., Staicu, C.-A., Pradel, M.: Anything to hide? Studying minified and obfuscated code in the web. In: The World Wide Web Conference, pp. 1735–1746 (2019)
APWG. Phishing activity trends report-4th quarter 2020. https://docs.apwg.org/reports/apwg_trends_report_q4_2020.pdf?_ga=2.52213802.263073049.1665252062-1448730527.1654753557 &_gl=1*1imdh26*_ga*MTQ0ODczMDUyNy4xNjU0NzUzNTU3*_ga_55RF0RHXSR*MTY2NTI1MjA2MS4xOC4wLjE2NjUyNTIzNTMuMC4wLjA (2021). Accessed April 2022
Sarker, S., Jueckstock, J., Kapravelos, A.: Hiding in plain site: detecting javascript obfuscation through concealed browser api usage. In: Proceedings of the ACM Internet Measurement Conference, pp. 648–661 (2020)
Romano, A., Lehmann, D., Pradel, M., Wang, W.: Wobfuscator: Obfuscating javascript malware via opportunistic translation to webassembly. In: Proceedings of the 2022 IEEE Symposium on Security and Privacy (S &P 2022), pp. 1101–1116 (2022)
Bagchi, K., Udo, G.: An analysis of the growth of computer and internet security breaches. Commun. Assoc. Inf. Syst. 12 (1), 46 (2003)
Loughran, D.T., Salih, M.K., Subburaj, V.H.: All about SQL injection attacks. J. Colloq. Inf. Syst. Secur. Educ. 6 , 24–24 (2018)
Patil, D.R., Patil, J.B.: Survey on malicious web pages detection techniques. Int. J. u-and e-Serv. Sci. Technol. 8 (5), 195–206 (2015)
Conti, M., Dragoni, N., Lesyk, V.: A survey of man in the middle attacks. IEEE Commun. Surv. Tutor. 18 (3), 2027–2051 (2016)
Sahani, R., Randhawa, S.: Clickjacking: Beware of clicking. Wirel. Pers. Commun. 121 (4), 2845–2855 (2021)
Shahriar, H., Devendran, V.K.: Classification of clickjacking attacks and detection techniques. Inf. Secur. J. A Glob. Perspect. 23 (4–6), 137–147 (2014)
Sinha, R., Uppal, D., Singh, D., Rathi, R.: Clickjacking: existing defenses and some novel approaches. In: 2014 International Conference on Signal Propagation and Computer Technology (ICSPCT 2014), pp 396–401. IEEE (2014)
Akhawe, D., He, W., Li, Z., Moazzezi, R., Song, D.: Clickjacking revisited: a perceptual view of \(\{\) UI \(\}\) security. In: 8th USENIX workshop on offensive technologies (WOOT 14) (2014)
Stone, P.: Next generation clickjacking. BlackHat Europe (2010)
Huang, L.-S., Moshchuk, A., Wang, H.J., Schecter, S., Jackson, C.: Clickjacking: attacks and defenses. In: 21st USENIX Security Symposium (USENIX Security 12), pp. 413–428 (2012)
Gupta, B.B., Yadav, K., Razzak, I., Psannis, K., Castiglione, A., Chang, X.: A novel approach for phishing URLs detection using lexical based machine learning in a real-time environment. Comput. Commun. 175 , 47–57 (2021)
Jain, A.K., Gupta, B.B.: A machine learning based approach for phishing detection using hyperlinks information. J. Amb. Intell. Hum. Comput. 10 (5), 2015–2028 (2019)
Ramana, A.V., Rao, K.L., Rao, R.S.: Stop-phish: an intelligent phishing detection method using feature selection ensemble. Soc. Netw. Anal. Min. 11 (1), 1–9 (2021)
Rao, R.S., Pais, A.R., Anand, P.: A heuristic technique to detect phishing websites using TWSVM classifier. Neural Comput. Appl. 33 (11), 5733–5752 (2021)
Rao, R.S., Pais, A.R.: Jail-phish: an improved search engine based phishing detection system. Comput. Secur. 83 , 246–267 (2019)
Suri, R.K., Tomar, D.S., Sahu, D.R.: An approach to perceive tabnabbing attack. Int. J. Sci. Technol. Res. 1 (6), 90–94 (2012)
Raskin, A.: Tabnabbing: a new type of phishing attack. línea. http://www.azarask.in/blog/post/a-new-type-of-phishing-attack/ . [Último acceso: 10 12 2013] (2010)
Kolsek, M.: Session fixation vulnerability in web-based applications. ACROS Security. http://www.acrossecurity.com/papers/sessionfixation.pdf (2002)
Kaspersky. Phishing-kit market: what’s inside “off-the-shelf” phishing packages. https://securelist.com/phishing-kit-market-whats-inside-off-the-shelf-phishing-packages/106149/ (2022). Accessed 25 Aug 2022
Kaspersky. How scammers are creating thousands of fake pages using phishing kits. https://usa.kaspersky.com/about/press-releases/2022_quick-cheap-and-dangerous-how-scammers-are-creating-thousands-of-fake-pages-using-phishing-kits (2022). Accessed 25 Aug 2022
Bahnsen, A.C., Torroledo, I., Camacho, L.D., Villegas, S.: Deepphish: simulating malicious AI. In: 2018 APWG Symposium on Electronic Crime Research (eCrime), pp. 1–8 (2018)
Group-IB. https://www.group-ib.com/media-center/press-releases/phishing-kits-2022/ (2023). Accessed Sep 2023
CNBC. https://www.cnbc.com/2023/01/07/phishing-attacks-are-increasing-and-getting-more-sophisticated.html (2023). Accessed July 2023
Dupuis, M., Geiger, T., Slayton, M., Dewing, F.: The use and non-use of cybersecurity tools among consumers: do they want help? In: Proceedings of the 20th Annual SIG Conference on Information Technology Education, pp. 81–86 (2019)
SECTRIO. https://sectrio.com/iot-security-reports/2023-ot-iot-threat-landscape-report/ (2023). Accessed July 2023
Proofpoint. https://www.proofpoint.com/sites/default/files/threat-reports/pfpt-us-tr-state-of-the-phish-2023.pdf (2023). Accessed Sep 2023
Check Point. https://www.avanan.com/hubfs/2022-Defender-Report/WP_Avanan_Keeping_Your_Emails_Secure_Who_Does_It_Best.pdf (2022). Accessed Sep 2023
Abnormal Society. https://cdn2.assets-servd.host/gifted-zorilla/production/files/Read-Alert-Data-Shows-28-of-BEC-Attacks-Opened-by-Employees.pdf?dm=1675457683 (2023). Accessed Sep 2023
AKAMAI. https://www.akamai.com/blog/security/over-25-percent-of-malicious-javascript-is-being-obfuscated (2021). Accessed July 2023
AKAMAI. https://www.akamai.com/blog/security/web-application-and-api-protection-from-sql-injection-to-magecart (2020). Accessed July 2023
COMPARITECH. https://www.comparitech.com/blog/information-security/botnet-statistics/ (2022). Accessed July 2023
ZedNET. https://www.zdnet.com/article/clickjacking-scripts-found-on-613-popular-sites-academics-say// (2019). Accessed July 2023
F5 Labs. https://www.f5.com/content/dam/f5-labs-v2/article/articles/threats/22--2020-oct-dec/20201110_2020_phishing_report/F5Labs-2020-Phishing-and-Fraud-Report.pdf (2020). Accessed July 2023
CheckPoint. https://blog.checkpoint.com/security/social-networks-most-likely-to-be-imitated-by-criminal-groups-with-linkedin-now-accounting-for-half-of-all-phishing-attempts-worldwide/ (2022). Accessed July 2023
Rao, R.S., Pais, A.R.: An enhanced blacklist method to detect phishing websites. In: International Conference on Information Systems Security, pp. 323–333. Springer (2017)
Bell, S., Komisarczuk, P.: An analysis of phishing blacklists: Google safe browsing, openphish, and phishtank. In: Proceedings of the Australasian Computer Science Week Multiconference, pp. 1–11 (2020)
Prakash, P., Kumar, M., Kompella, R R., Gupta, M.: Phishnet: predictive blacklisting to detect phishing attacks. In: 2010 Proceedings IEEE INFOCOM, pp. 1–5. IEEE (2010)
Han, W., Cao, Y., Bertino, E., Yong, J.: Using automated individual white-list to protect web digital identities. Expert Syst. Appl. 39 (15), 11861–11869 (2012)
Jain, A.K., Gupta, B.B.: A novel approach to protect against phishing attacks at client side using auto-updated white-list. EURASIP J. Inf. Secur. 2016 (1), 1–11 (2016)
Azeez, N.A., Misra, S., Margaret, I.A., Fernandez-Sanz, L., et al.: Adopting automated whitelist approach for detecting phishing attacks. Comput. Secur. 108 , 102328 (2021)
Sheng, S., Wardman, B., Warner, G., Hong, J., Zhang, C.: An empirical analysis of phishing blacklists. Lorrie Cranor (2009)
Sahingoz, O.K., Buber, E., Demir, O., Diri, B.: Machine learning based phishing detection from URLs. Expert Syst. Appl. 117 , 345–357 (2019)
Jain, A.K., Gupta, B.B.: Phish-safe: URL features-based phishing detection system using machine learning. In: Cyber Security, pp. 467–474. Springer (2018)
Ali, M.S., Jain, A.K.: Efficient feature selection approach for detection of phishing URL of Covid-19 era. In: International Conference on Cyber Security, Privacy and Networking, pp. 45–56. Springer (2021)
Jain, A.K., Parashar, S., Katare, P., Sharma, I.: Phishskape: a content based approach to escape phishing attacks. Procedia Comput. Sci. 171 , 1102–1109 (2020)
Rao, R.S., Umarekar, A., Pais, A.R.: Application of word embedding and machine learning in detecting phishing websites. Telecommun. Syst. 1–13 (2022)
Varshney, G., Misra, M., Atrey, P.K.: A phish detector using lightweight search features. Comput. Secur. 62 , 213–228 (2016)
Jain, A.K., Gupta, B.B.: Two-level authentication approach to protect from phishing attacks in real time. J. Amb. Intell. Hum. Comput. 9 (6), 1783–1796 (2018)
Gupta, B.B., Jain, A.K.: Phishing attack detection using a search engine and heuristics-based technique. J. Inf. Technol. Res. JITR 13 (2), 94–109 (2020)
Jain, A.K., Gupta, B.B.: Towards detection of phishing websites on client-side using machine learning based approach. Telecommun. Syst. 68 (4), 687–700 (2018)
Rao, R.S., Pais, A.R.: Detection of phishing websites using an efficient feature-based machine learning framework. Neural Comput. Appl. 31 (8), 3851–3873 (2019)
Jain, A.K., Gupta, B.B., Kaur, K., Bhutani, P., Alhalabi, W., Almomani, A.: A content and URL analysis-based efficient approach to detect smishing SMS in intelligent systems. Int. J. Intell. Syst. 37 (12), 11117–11141 (2022)
Mao, J., Tian, W., Li, P., Wei, T., Liang, Z.: Phishing-alarm: robust and efficient phishing detection via page component similarity. IEEE Access 5 , 17020–17030 (2017)
Chen, J.-L., Ma, Y.-W., Huang, K.-L.: Intelligent visual similarity-based phishing websites detection. Symmetry 12 (10), 1681 (2020)
Routhu Srinivasa Rao and Alwyn Roshan Pais: Two level filtering mechanism to detect phishing sites using lightweight visual similarity approach. J. Ambient. Intell. Humaniz. Comput. 11 (9), 3853–3872 (2020)
Ahmet Selman Bozkir and Murat Aydos: Logosense: a companion hog based logo detection scheme for phishing web page and e-mail brand recognition. Comput. Secur. 95 , 101855 (2020)
Phishtank. https://phishtank.org/ . Accessed July 2023
Ahrefs. https://ahrefs.com/ . Accessed July 2023
Similarweb. https://www.similarweb.com/ . Accessed July 2023
Majestic million. https://majestic.com/reports/majestic-million . Accessed July 2023
https://github.com/ebubekirbbr/pdd/tree/master/input . Accessed July 2023
https://www.unb.ca/cic/datasets/url-2016.html . Accessed July 2023
Vrbančič, G.: Phishing websites dataset. Mendeley Data (2020)
Jpcert/cc. https://github.com/JPCERTCC/phishurl-list/ . Accessed July 2023
Lee, L.-H., Lee, K.-C., Chen, H.-H., Tseng, Y.-H.: Poster: Proactive blacklist update for anti-phishing. In: Proceedings of the 2014 ACM SIGSAC Conference on Computer and Communications Security, pp. 1448–1450 (2014)
Barraclough, P.A., Fehringer, G., Woodward, J.: Intelligent cyber-phishing detection for online. Comput. Secur. 104 , 102123 (2021)
Karim, A., Shahroz, M., Mustofa, K., Belhaouari, S.B., Joga, S.R.K.: Phishing detection system through hybrid machine learning based on URL. IEEE Access 11 , 36805–36822 (2023)
Download references
No funding was received for conducting this study and the authors have no financial or proprietary interests in any material discussed in this article.
Author information
Authors and affiliations.
CSE Department, Maulana Azad National Institute of Technology, Bhopal, M.P., India
Richa Goenka, Meenu Chawla & Namita Tiwari
You can also search for this author in PubMed Google Scholar
Contributions
RG wrote the whole manuscript. MC and NT provided valuable inputs and reviewed the manuscript.
Corresponding author
Correspondence to Richa Goenka .
Ethics declarations
Conflict of interest.
The authors declare no competing interests.
Additional information
Publisher's note.
Springer Nature remains neutral with regard to jurisdictional claims in published maps and institutional affiliations.
Rights and permissions
Springer Nature or its licensor (e.g. a society or other partner) holds exclusive rights to this article under a publishing agreement with the author(s) or other rightsholder(s); author self-archiving of the accepted manuscript version of this article is solely governed by the terms of such publishing agreement and applicable law.
Reprints and permissions
About this article
Goenka, R., Chawla, M. & Tiwari, N. A comprehensive survey of phishing: mediums, intended targets, attack and defence techniques and a novel taxonomy. Int. J. Inf. Secur. 23 , 819–848 (2024). https://doi.org/10.1007/s10207-023-00768-x
Download citation
Published : 19 October 2023
Issue Date : April 2024
DOI : https://doi.org/10.1007/s10207-023-00768-x
Share this article
Anyone you share the following link with will be able to read this content:
Sorry, a shareable link is not currently available for this article.
Provided by the Springer Nature SharedIt content-sharing initiative
- Phishing techniques
- Phishing circulation mediums
- Intended targets
- Phishing countermeasures
- Find a journal
- Publish with us
- Track your research
Content has been added to your Folio
Risiken für die Privatsphäre
Worldwide 2023 Email Phishing Statistics and Examples
Explore the need for going beyond built-in Microsoft 365 and Google Workspace™ security based on email threats detected in 2023.
By: Trend Micro June 20, 2024 Read time: ( words)
Save to Folio
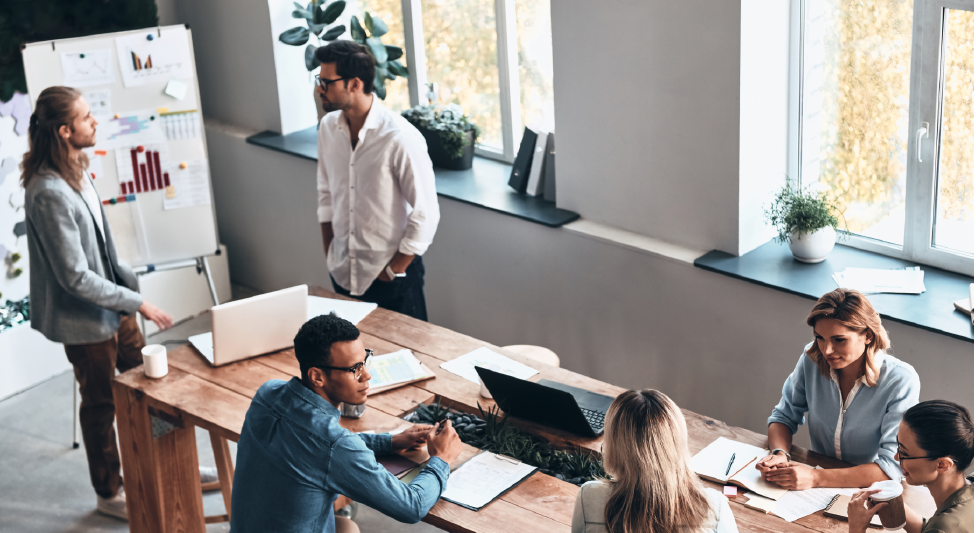
Remote and hybrid work environments have become the new norm. The fact that email become increasingly integral to your business operations has led malicious actors to favor email as an attack vector. In 2023, Trend Vision One™ – Email and Collaboration Security discovered more than 45 million high-risk email threats , in addition to those detected by built-in Microsoft 365 and Google Workspace security. As adversaries become stealthier and more organized, it’s more important than ever to take your organizational defenses beyond native security.
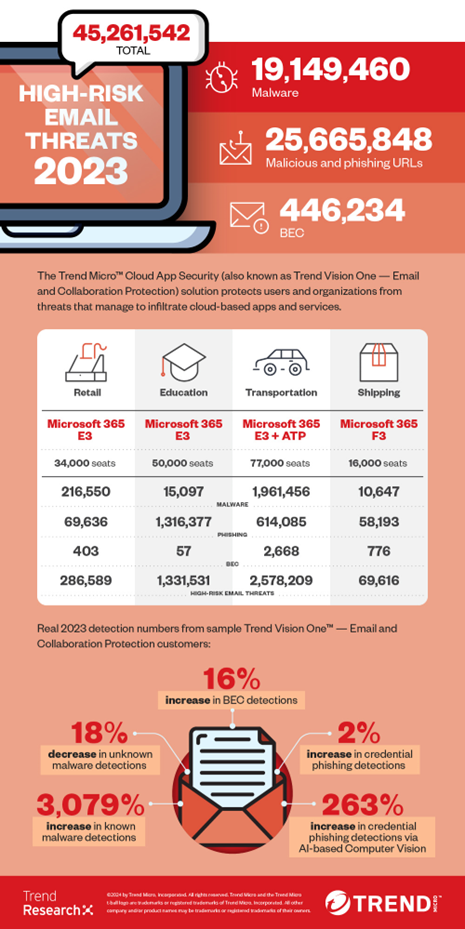
With flexible and remote workforces continue to cause gaps in cloud security to be exploited, cybercriminals are leveraging blind spots in email services’ built-in security. In fact, 46% of all threats blocked by Trend Micro in 2023 were email threats.
Unfortunately, built-in security for popular email services, like Microsoft 365 and Google Workspace, is simply not enough to stop malicious emails from infiltrating enterprises. Tools like Email and Collaboration Security have become a vital part of your defense strategy, as this security platform supplements built-in security and acts as a second layer of defense that can catch highly evasive and complex threats.
Malware attacks surged, known malware detections dropped
Trend detected and blocked 19.1 million malware files in 2023. This represents a 349% rise when compared to 2022. The number of known malware threats also spiked to 16 million , indicating a massive 3,079% surge. Nevertheless, it is important to mention that the number of unknown malware files to 3 million, representing a 18% decline .
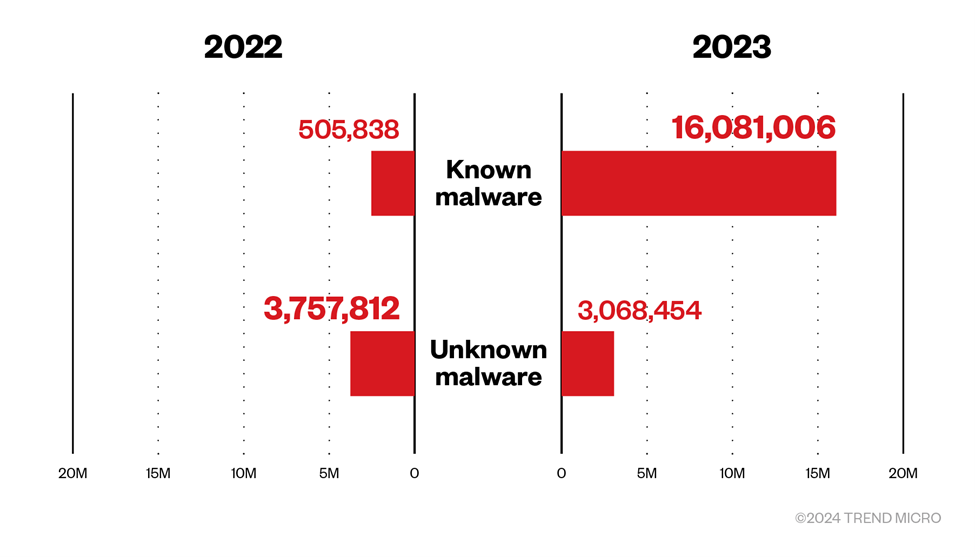
A mid-2022 measure implemented by Microsoft continues contribute to these drop in detections. During this period, Microsoft took action to prevent the execution of macro programs in Microsoft 365 documents, particularly those obtained from the internet or received as email attachments. Over the past eight years, the prevailing method for initial access has been through Microsoft 365 documents containing malicious macros, commonly distributed to targets via email.
Phishing remains a common attack method
According to a report by security company Egress, 94% of organizations have fallen victim to phishing attacks in 2023. This accounts for the 40% increase in phishing incidents from 2022, where we detected and blocked a total of over 14 million attacks.
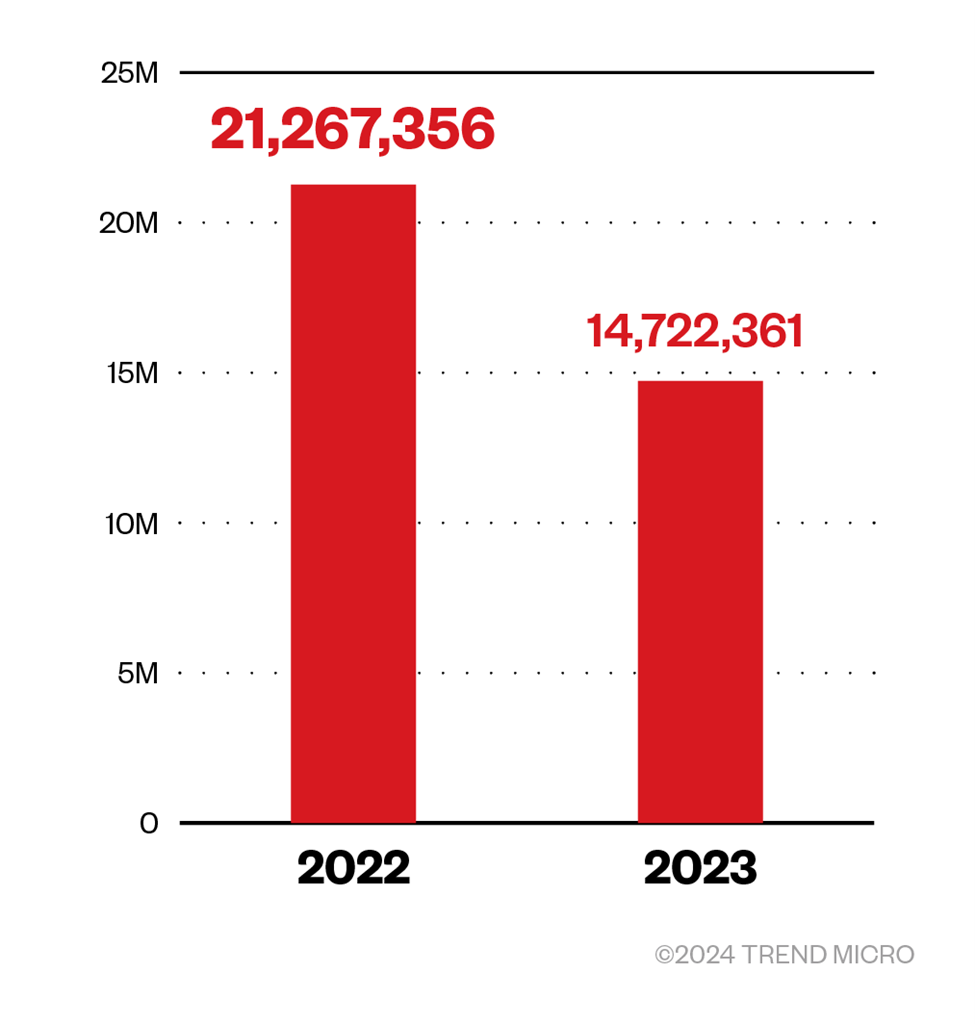
We also observed a 45% decrease in phishing attacks detected via spam count in 2023, with close to 8 million total detections.
It’s important to note that Email and Collaboration Security detection efforts count phishing links within attachments as potential malware, which explains the drop in phishing detections. Cybercriminals are now opting to incorporate phishing links inside email attachments versus directly in the email body for obfuscation purposes.
Phishing attacks aimed at stealing info and data, also known as credential phishing, saw a 17% growth in 2023, with nearly 7 million detections . Trend saw minimal growth for known credential phishing detections at 5%, while unknown credential phishing detections leaped a significant 29%.
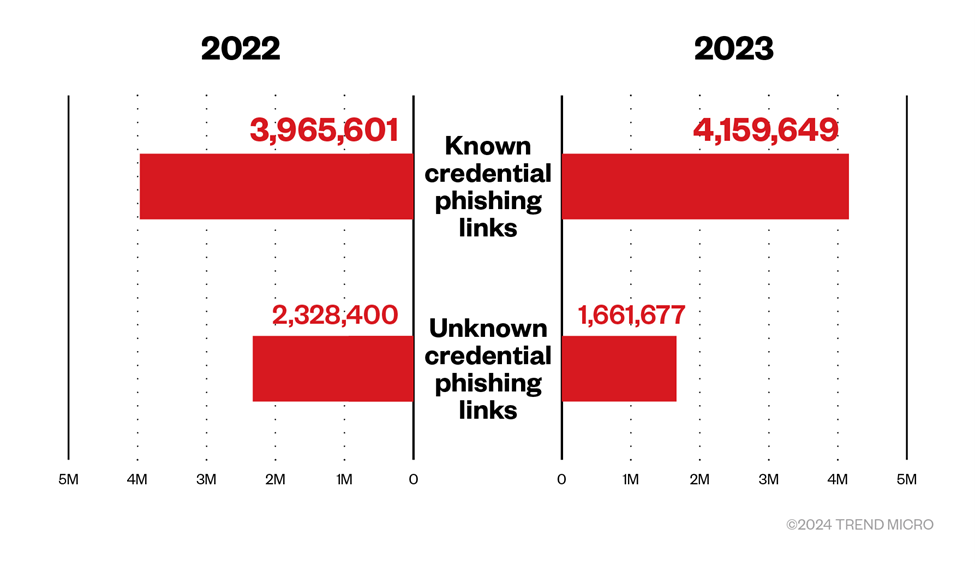
However, 870,555 credential phishing links were discovered via Computer Vision, an image analysis and machine learning (ML) technology that detects credential phishing emails by checking site content like branded elements and login forms. Our findings represent a whopping 263% increase compared to 2022.
BEC continues to be a lucrative attack option
Trend™ Research disclosed that business email compromise (BEC) detections rose to 446,234 in 2023, a growth of 16% . The number of BEC attacks that were detected via Trend Micro™ Writing Style DNA reached 166,034, while 280,191 were detected through the anti-spam engine, representing a 13% increase.
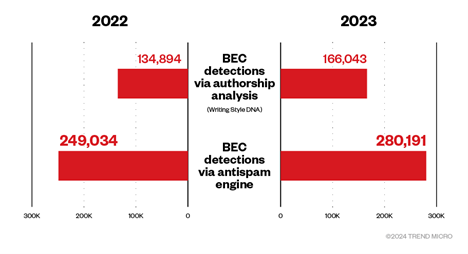
Cybercriminals continued to evolve their tactics to take advantage of new work setups. Trend Research determined that BEC actors mostly impersonated executives or high-ranking management personnel by spoofing general employees’ names. With arrival of sophisticated chat AI tools are expected to make cybercriminals more adept at these type of spoofs.
Visibility across the enterprise is paramount in the new normal of remote and hybrid work environments. You need to continually discover, assess, and mitigate risk across your digital attack surface to keep your users secure and the business out of the headlines.
To gain comprehensive visibility, cybersecurity leaders should leverage a SaaS-based platform that supplements the built-in security features in email platforms like Microsoft 365 and Google Workspace.
SaaS-based solutions like Cloud App Security are easy to set up, use sophisticated techniques like ML, and are a part of our Trend Vision One ™. This single modern cloud-native security operations platform delivers key capabilities like power purpose-built XDR, attack surface management, and zero-trust capabilities so you can move faster than your adversaries and protect business operations.
Learn more about the facts and figures of email threats for 2022 as well as mitigation strategies in our exclusive email threat landscape report: Cybercriminal Tactics, Techniques That Organizations Need to Know .
Trend Micro
Research, News, and Perspectives
Related Articles
- Behind the Great Wall: Void Arachne Targets Chinese-Speaking Users With the Winos 4.0 C&C Framework
- Bedrohungsbekämpfung nimmt an Reife zu
- Not Just Another 100% Score: MITRE ENGENUITY ATT&CK
Testen Sie die Services 30 Tage lang kostenlos
- Kostenlose Probeversion
- --> --> -->
- Berichte zu Bedrohungen
- DevOps Resource Center
- CISO Resource Center
- Partner suchen
- Support-Portal für Unternehmen
- Kostenlose Testversionen
- Karriere bei Trend Micro
- Veranstaltungshinweise
- Trust Center
Hauptniederlassung DACH
Trend Micro - Germany (DE)
Parkring 29 85748 Garching Deutschland
Telefon: +49 (0)89 8393 29700
Land/Region auswählen
Nord-, Mittel- und Südamerika
Naher osten und afrika.
- Naher Osten und Nordafrika
- Belgien (België)
- Tschechische Republik
- Deutschland, Österreich, Schweiz
- Niederlande
- Norwegen (Norge)
- Polen (Polska)
- Finnland (Suomi)
- Schweden (Sverige)
- Türkei (Türkiye)
- Vereinigtes Königreich
Beyond the trans/cis binary: introducing new terms will enrich gender research
- Florence Ashley 0 ,
- Shari Brightly-Brown 1 &
- G. Nic Rider 2
Florence Ashley (they/them) is an assistant professor at the Faculty of Law, University of Alberta and in the John Dossetor Health Ethics Centre, University of Alberta, Edmonton, Canada.
You can also search for this author in PubMed Google Scholar
Shari Brightly-Brown (they/them) is a postdoctoral fellow at the Institute for Sexual and Gender Health and in the National Center for Gender Health, University of Minnesota Medical School in Minneapolis.
G. Nic Rider (they/them) is an assistant professor at the Institute for Sexual and Gender Health and director of the National Center for Gender Health, University of Minnesota Medical School in Minneapolis.
Illustration: Sophi Gullbrants
You have full access to this article via your institution.
“Are you transgender?” Participating in a study for their public-health class, neither Alex nor Luna knew how to answer. Alex uses they/them pronouns and identifies as agender. They are also among a growing number of young people who have been raised in a gender-neutral manner: their parents did not refer to them as a boy or a girl until they were old enough to choose for themselves. Whatever genitals Alex was born with is not common knowledge. If you are agender and were never assigned a gender, does that make you transgender?
As for Luna, today she identifies as a woman, which aligns with the gender she was assigned at birth. But this is a recent development: Luna identified as a boy for as long as she can remember and, after coming out as trans, lived openly as one throughout her childhood and adolescence. As a woman who has detransitioned, she often feels that she has more in common with transgender women than with cisgender ones, whose gender identity corresponds to the gender they were assigned at birth. Although Luna doesn’t call herself transgender, she fears that answering ‘no’ to the study’s question means that her gender trajectory and experiences will be erased.
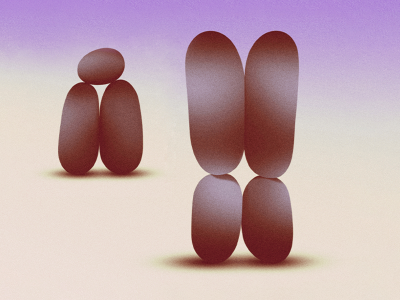
Collection: Sex and gender in science
The difficulties Alex and Luna experienced might seem unusual. But many individuals find themselves unmoored from binary terms such as male and female, or cis and trans 1 . In the United States, an estimated 9.2% of secondary-school students don’t wholly identify with the gender they were assigned at birth 2 , yet only 1.8% anonymously answer ‘yes’ when asked whether they are transgender 3 . These identities are not trivial. How people identify shapes not only their experiences of marginalization, but also their bodies — be it by influencing their smoking habits, whether they exercise, what they eat or whether they undergo hormone therapy or transition-related surgeries.
Human experiences are inevitably richer than the categories we carve out for them. But finding the right concepts and language to describe their diversity is an essential part of the scientific endeavour. It helps researchers to capture the experiences of participants more accurately, enhances analytical clarity and contributes to people feeling included and respected. Scientists need terms that are flexible enough to capture the nuances of people’s experience, that leave space for language to evolve and that are nonetheless pragmatic enough to be used in research.
Encompassing diversity
The term ‘gender modality’ could enable researchers to broaden their horizons.
A person’s gender identity is their sense of gender at any given time. By contrast, gender modality refers to how a person’s gender identity relates to the gender they were assigned at birth (see go.nature.com/3x34784 ). It is a mode or way of being one’s gender.
The best-known gender modalities are ‘cisgender’ and ‘transgender’, but the term allows for other possibilities, such as ‘agender’, which includes those who do not identify with any gender, and ‘detrans’ or ‘retrans’ for people who have ceased, shifted or reversed their gender transition. The term also makes space for gender modalities specific to intersex individuals, gender-questioning people, people with dissociative identity disorder and people with culture-specific identities (see ‘Many ways of being’). Gender modality serves a similar purpose to sexual orientation, which describes a facet of human existence and makes space for orientations beyond gay and straight.
Many ways of being
‘Gender modality’ refers to how a person’s gender identity relates to the gender they were assigned at birth, and includes options other than cisgender and transgender. This is not an exhaustive list.
Agender: people who do not identify with any gender.
Cisgender: people whose gender identity corresponds to the gender they were assigned at birth.
Closeted trans people: individuals whose gender identity does not correspond to the gender they were assigned at birth, but who do not share their gender identity publicly.
Culture-specific identities: individuals can have identities, such as Two-Spirit identities in North American Indigenous communities and hijra on the Indian subcontinent, that might not align with Western concepts of gender and sexuality. People with these identities might not consider themselves cis or trans because of the Western philosophies that underpin these terms.
Detrans/retrans: People who have ceased, shifted or reversed their gender transition.
Gender questioning: people who are unsure of their gender identity and are in the process of working it out.
Intersex: people who were born or who endogenously developed sexual traits that differ from typical expectations of female and male bodies. Some intersex people do not consider themselves to be cis or trans.
People with dissociative identity disorder whose alters have distinct gender identities: people with this condition, also known as plural people, can have several identities, known as alters or headmates, that have distinct gender identities. These alters can have different gender modalities.
Raised in a gender-neutral manner: people who were raised without being referred to as a boy/he or girl/she until they were old enough to express their gender identity.
Transgender: people whose gender identity does not correspond to the gender they were assigned at birth.
The term gender modality was coined in 2019 by one of us (F.A.) in response to frustrations felt as a trans bioethicist and jurist with the limits of existing language (see go.nature.com/3x34784 ). The term has since been used by transgender communities, clinicians and policymakers to describe the realities of trans communities and the heterogeneity of trans experiences. It is increasingly being used in trans health research 4 – 6 and has been included in official documentation by the government agency Statistics Canada (see go.nature.com/3wvzczw ); in educational materials by the US non-profit organization Planned Parenthood (see go.nature.com/4arxzuw ); and in decisions by the Supreme Court of Canada.
In our view, researchers can use gender modality to improve their work in three important ways.
First, scientists can expand the gamut of gender modalities included in questionnaires given to participants, to capture a broader range of experiences than those represented by the binary of cis and trans. Formulating new categories, adapted to the study design, will enhance the validity of the research 7 , 8 . It could also improve response rates and reduce the likelihood of people dropping out.
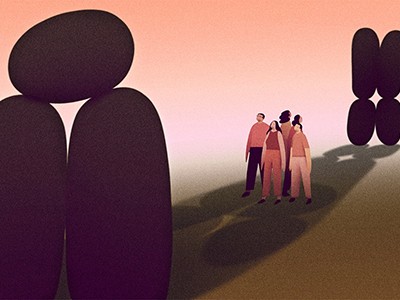
We need more-nuanced approaches to exploring sex and gender in research
Researchers who exclude possibilities beyond cis and trans typically rely on several tacit assumptions about bodies and social experiences. For instance, they might assume that participants whose gender identity corresponds to their gender assigned at birth are cisgender men and women — which would be incorrect for people, like Alex, who were raised in a wholly gender-neutral manner. Or they could assume that a person’s gender identity hasn’t changed across their lifespan and that they can infer certain facts about the participant’s body or medical history from their response — which would be incorrect for those, like Luna, who have detransitioned.
Other false assumptions could include the idea that every culture understands gender in a similar manner, that gender assigned at birth straightforwardly reflects anatomy at birth, that everyone has only one gender identity and that everyone can identify and communicate their gender identity.
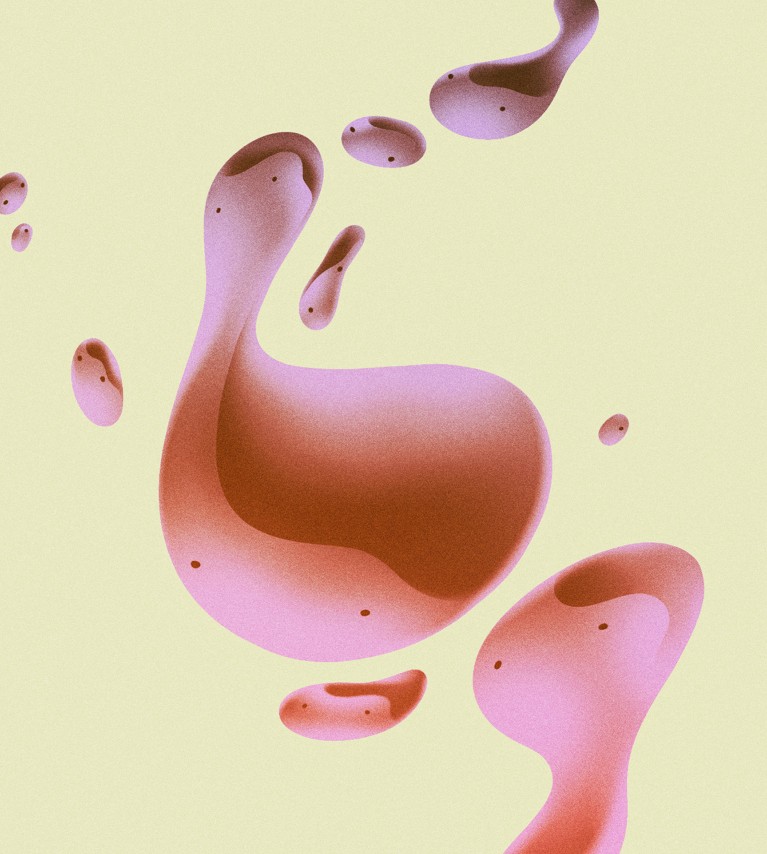
This is not to say that every study should include every possible gender modality. The guiding principles are feasibility, necessity and respect. Sometimes, there are too few participants in a subgroup for researchers to analyse them separately. Moreover, some gender modalities could be irrelevant to the study question. For instance, it might not be necessary to include a detrans gender modality in a study if researchers are specifically interested in health outcomes among trans people. The point is to avoid uncritically assuming that the cis/trans binary suffices. It might, or it might not.
Capturing the human experience
The second way in which researchers can use gender modality to improve their work is by using it to refine how they phrase questions or discuss results.
By reflecting on gender modality, researchers can better ensure that participants feel respected, and can avoid assigning gender modalities that conflict with participants’ identities. Recognizing gender modalities beyond cis and trans is a matter of justice 9 . In some studies, offering write-in opportunities can help participants to feel respected despite the nuances of their experiences not being captured. But it could be as simple as using ‘gender modality’ instead of ‘gender identity’ or ‘transgender status’ in a table heading, because the last two terms can be seen as inaccurate or marginalizing.
Unlike terms such as transgender status, gender modality places cis, trans and other gender-expansive people on an equal footing. It reflects a universalizing conception of gender diversity 10 , in which everyone has a gender modality and being trans, detrans or cis comprises just some of the options in the vast expanse of human experience. This is important, given the long history of trans and gender non-conforming people being treated as deviant or abnormal.
Finally, researchers can use gender modality to think more meticulously about what it is that they are really trying to capture in their study.
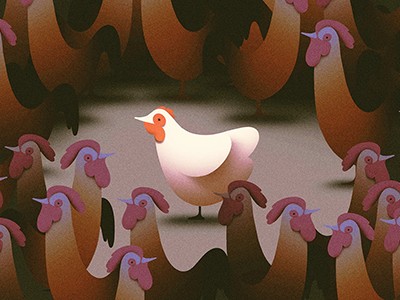
Male–female comparisons are powerful in biomedical research — don’t abandon them
Linguistic gaps abound when it comes to our ability to describe trans people’s experiences. For instance, discrimination against trans people is often described as discrimination on the basis of gender identity. Although this shorthand might be workable, it is not entirely accurate. If a trans woman is fired for being trans, should we say that her gender identity was targeted when she has the same gender identity as cis women? Although her gender identity was part of the equation, it would be more accurate to say that she was discriminated against on the basis of her gender modality 11 . Gender modality, not gender identity, is what distinguishes trans women from cis women.
Gender modality can help researchers to describe participant experiences with improved accuracy by shaping how they phrase their study questions or frame the discussion section of their papers. For example, asking whether participants experienced discrimination because of their gender identity can cause confusion, which could be dispelled by asking whether they experienced discrimination because they are trans, detrans or another gender modality. Used thoughtfully, gender modality is a means of achieving improved clarity, accuracy and understanding. A researcher’s first question should always be: ‘What am I really trying to work out?’
Fluid and flexible language
Gender modality is not a panacea. Rather, it is one piece in the toolbox of those who engage in research involving human participants, whether in the medical, biological or social sciences. Its power lies in what people make of it. Our hope is that researchers and others will play with it, stretching it and exploring its full potential. Rather than foreclosing the evolution of language, gender modality welcomes it.
Not everyone is male or female. Not everyone is cis or trans. The sooner we make space for these truths, the better. And inviting scientists to adopt the concept of gender modality will hopefully foster research that better reflects the intricacies and nuances of our increasingly gender-expansive world.
The first step in science should never be to assume that something is correct. It should be to engage with the world in front of us — in all its magnificent complexity. Researching gender should begin with critically engaging with current language and concepts. Thoughtfulness, flexibility, curiosity and empathy are what science needs.
Nature 630 , 293-295 (2024)
doi: https://doi.org/10.1038/d41586-024-01719-9
Beischel, W. J., Schudson, Z. C., Hoskin, R. A. & van Anders, S. M. Psychol. Sex. Orientat. Gend. Divers. 10 , 355–372 (2023).
Article Google Scholar
Kidd, K. M. et al. Pediatrics 147 , e2020049823 (2021).
Article PubMed Google Scholar
Johns, M. M. et al. Morb. Mortal. Wkly Rep. 68 , 67–71 (2019).
Rioux, C. et al. J. Epidemiol. Commun. Health 76 , 764–768 (2022).
Hayes, L. et al. Am. J. Public Health 113 , 1153–1156 (2023).
Streed, C. G. Jr et al. Circulation 144 , e136–e148 (2021).
Adams, N. et al. Transgend. Health 2 , 165–175 (2017).
Marshall, Z., Kaposy, C., Brunger, F. & Welch, V. Bull. Appl. Transgend. Stud. 1 , 187–210 (2022).
Beauchamp, T. L. & Childress, J. F. Principles of Biomedical Ethics 8th edn (Oxford Univ. Press, 2019).
Google Scholar
Sedgwick, E. K. Epistemology of the Close t (Univ. California Press, 2008).
Katri, I. Univ . PA J. Law Soc. Change 20 , 51–79 (2017).
Download references
Competing Interests
The authors declare no competing interests.
Related Articles
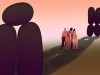
Let’s talk about (biological) sex
- Public health
The global refugee crisis is above all a human tragedy — but it affects wildlife, too
Correspondence 18 JUN 24
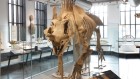
Why museums should repatriate fossils
Comment 18 JUN 24
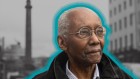
My pivot from grain scientist to slave-trade historian
Career Q&A 14 JUN 24
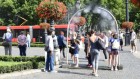
How climate change is hitting Europe: three graphics reveal health impacts
News 18 JUN 24
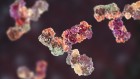
What causes long COVID? Case builds for rogue antibodies
News 13 JUN 24
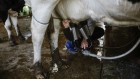
Huge amounts of bird-flu virus found in raw milk of infected cows
News 05 JUN 24
Endowed Chair in Macular Degeneration Research
Dallas, Texas (US)
The University of Texas Southwestern Medical Center (UT Southwestern Medical Center)
Postdoctoral Fellow
Postdoc positions on ERC projects – cellular stress responses, proteostasis and autophagy
Frankfurt am Main, Hessen (DE)
Goethe University (GU) Frankfurt am Main - Institute of Molecular Systems Medicine
ZJU 100 Young Professor
Promising young scholars who can independently establish and develop a research direction.
Hangzhou, Zhejiang, China
Zhejiang University
Qiushi Chair Professor
Distinguished scholars with notable achievements and extensive international influence.
Research Postdoctoral Fellow - MD
Houston, Texas (US)
Baylor College of Medicine (BCM)
Sign up for the Nature Briefing newsletter — what matters in science, free to your inbox daily.
Quick links
- Explore articles by subject
- Guide to authors
- Editorial policies
We've detected unusual activity from your computer network
To continue, please click the box below to let us know you're not a robot.
Why did this happen?
Please make sure your browser supports JavaScript and cookies and that you are not blocking them from loading. For more information you can review our Terms of Service and Cookie Policy .
For inquiries related to this message please contact our support team and provide the reference ID below.
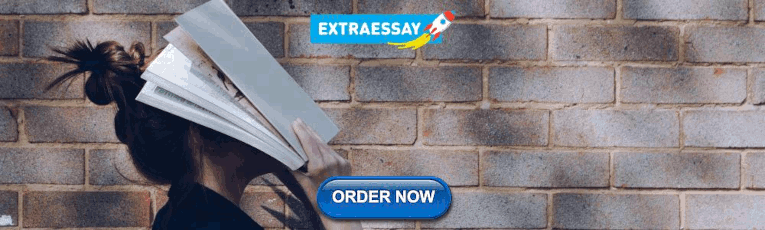
IMAGES
VIDEO
COMMENTS
With the significant growth of internet usage, people increasingly share their personal information online. As a result, an enormous amount of personal information and financial transactions become vulnerable to cybercriminals. Phishing is an example of a highly effective form of cybercrime that enables criminals to deceive users and steal important data. Since the first reported phishing ...
The paper presents a systematic literature review featuring 248 articles (from the beginning of 2018 until March 2023) across the main digital libraries to identify, (1) the existing mitigation strategies against phishing attacks, and the underlying technologies considered in the development of these strategies; (2) the most considered phishing ...
These phishing attacks come in the form of a request, urgent, important, seeking attention and often requiring some form of payment . According to research some industries are more targeted than others, for example, public administration services had the most breaches from social engineering, followed by other professional services .
Research article. First published online May 17, 2021. The Scams Among Us: Who Falls Prey and Why ... This was the most intense and extensive phishing attack in the company's history (Kumaran & Lugani, 2020). Millions of other COVID-19 scams 1 were circulating the globe, including ones that asked for donations, offered COVID-19 treatments, or ...
That is, the landscape of research on COVID‐19 phishing attacks and their countermeasures is made of a majority of studies aimed at investigating attacks, with only a relative minority of works that proposed specific solutions to them. The analysis of the literature that investigated attacks revealed that scholars already explored different ...
Phishing is an example of a highly. effective form of cybercrime that enables criminals to deceive users and steal important. data. Since the first reported phishing attack in 1990, it has been ...
Section 2 presents the literature survey focusing on deep learning, machine learning, hybrid learning, and scenario-based phishing attack detection techniques and presents the comparison of these techniques. Section 3 presents a discussion on various approaches used in literature. Section 4 present the current and future challenges.
According to the Anti-Phishing Working Group (APWG) report in the 3rd Quarter of 2020, the number of phishing attacks has grown since March, and 28,093 unique phishing sites have been detected ...
Phishing attacks are on the increase. The fact that our ways of living, studying and working have drastically changed as a result of the COVID pandemic (i.e., almost everything being done online) has created many new cyber security concerns. In particular, with the move to remote working, the number of phishing emails threatening employees has increased. The 2020 Phishing Attack Landscape ...
Phishing is the fraudulent attempt to obtain sensitive information by disguising oneself as a trustworthy entity in digital communication. ... Tejaswini Herath, Rui Chen, Arun Vishwanath, and H. Raghav Rao. 2012. Research article phishing susceptibility: An investigation into the processing of a targeted spear phishing email. IEEE Transactions ...
1. This systematic literature review aims to provide a comprehensive overview of the current state of research on the use of deep learning techniques for phishing detection. 2. The review explores the various deep learning techniques used for phishing detection, their effectiveness, and areas for future research. 3.
(Phishing methods detection). 244 research articles were collected from the first decade of 2000 up to date. However, in the first review, it was found that more recent works already covered previous studies taking as global categories detection methods as List, Heuristically, Machine learning and it was also necessary to consider a great ...
Research article. First published online March 11, 2022. Phishing—A Cyber Fraud: The Types, Implications and Governance ... Thus, in order to combat phishing attacks, internet users should be educated on security concerns, the influence of social engineering and anti-phishing knowledge. This paper presents a literature review of phishing, a ...
Recent research showed that 60% of participants fell victim to artificial intelligence (AI)-automated phishing, which is comparable to the success rates of non-AI-phishing messages created by ...
ing and phishing prevention in large organizations. 2) Supportive results for several previous research findings with improved ecological validity. 3) Contradicting findings that challenge the conclusions of previous research studies and popular industry practices. 4) Large-scale evaluation of crowd-sourced phishing re-
The security threat posed by email-based phishing campaigns targeted at employees is a well-known problem experienced by many organizations. Attacks are reported each year, and a reduction in the number of such attacks is unlikely to occur in the near future (see Fig. 1).A common type of phishing attack involves an attacker attempting to trick victims into clicking on links sent via email.
Phishing is a major threat to all Internet users and is difficult to trace or. defend against since it does not present itself as obviously malicious in nature. In today's society, everything is ...
It is a known fact that cybercriminals often manipulate people to steal sensitive information. However, there has been a lack of research in investigating cybercriminals' strategies and the evolution of strategies when crafting phishing emails to entice people to perform a variety of malicious tasks such as clicking on fraudulent links.
There was a 61% increase in the rate of phishing attacks in the six months ending October 2022 compared to the previous year. The attacks are also getting more sophisticated, and are spreading ...
The increase in the number of phishing demands innovative solutions to safeguard users from phishing attacks. This study explores the development and utilization of a real-time browser extension integrated with machine learning model to improve the detection of phishing websites. The results showed that the model had an accuracy of 98.32%, precision of 98.62%, recall of 97.86%, and an F1-score ...
Cyberattacks are hitting research institutions — with devastating effects. Hackers are targeting universities and research institutes with ransomware, leaving staff and students without the ...
The broader impact of phishing emails. According to HP-Bromium (), most malware was delivered by email during the fourth quarter of 2020.Malware can be disguised as an attachment or a URL in phishing emails, and malware payloads may include remote access Trojans, downloaders, keyloggers (Proofpoint 2021a), and ransomware (Greenman et al. 2021). ...
Emergence of AI chatbots is making it easier to create phishing scams . Friday, Jun 21, 2024 • Brian Lopez : contact A team of researchers at the University of Texas at Arlington has developed software that prevents artificial intelligence (AI) chatbots such as ChatGPT from creating phishing websites — a growing concern as cybercriminals have been utilizing the technology for designing scams.
Opinion. An epidemic of scientific fakery threatens to overwhelm publishers. More than 10,000 scientific papers were retracted last year as "paper mills" exploit the system. By Adam Marcus ...
In recent times, a phishing attack has become one of the most prominent attacks faced by internet users, governments, and service-providing organizations. In a phishing attack, the attacker(s) collects the client's sensitive data (i.e., user account login details, credit/debit card numbers, etc.) by using spoofed emails or fake websites. Phishing websites are common entry points of online ...
Phishing involves an attacker trying to trick someone into providing sensitive account or other login information online. All the different types of phishing are designed to take advantage of the fact that so many people do business over the internet. This makes phishing one of the most prevalent cybersecurity threats around, rivaling distributed denial-of-service (DDoS) attacks, data breaches ...
The recent surge in phishing incidents in the post-COVID era poses a serious threat towards the social and economic well-being of users. The escalation in dependency upon the internet for meeting daily chores has made them vulnerable to falling prey to the ever-evolving menace of phishing. The objective of this article is: to explore different tactics and motivational factors behind phishing ...
Trend detected and blocked 19.1 million malware files in 2023. This represents a 349% rise when compared to 2022. The number of known malware threats also spiked to 16 million, indicating a massive 3,079% surge. Nevertheless, it is important to mention that the number of unknown malware files to 3 million, representing a 18% decline.
The term 'gender modality' could enable researchers to broaden their horizons. A person's gender identity is their sense of gender at any given time. By contrast, gender modality refers to ...
Ilya Sutskever, the famed researcher who co-founded OpenAI, took part in the 2023 board ouster of Sam Altman as chief executive officer, before changing course and helping engineer Altman's ...