
An official website of the United States government
The .gov means it’s official. Federal government websites often end in .gov or .mil. Before sharing sensitive information, make sure you’re on a federal government site.
The site is secure. The https:// ensures that you are connecting to the official website and that any information you provide is encrypted and transmitted securely.
- Publications
- Account settings
- My Bibliography
- Collections
- Citation manager
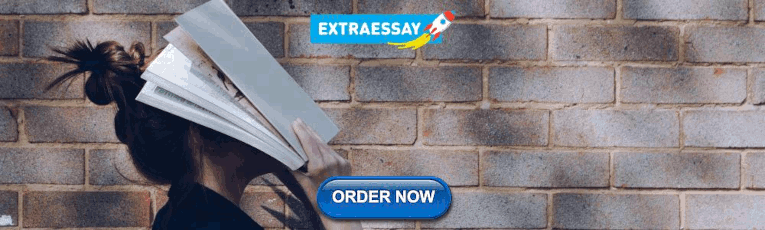
Save citation to file
Email citation, add to collections.
- Create a new collection
- Add to an existing collection
Add to My Bibliography
Your saved search, create a file for external citation management software, your rss feed.
- Search in PubMed
- Search in NLM Catalog
- Add to Search
AM last page. Quality criteria in qualitative and quantitative research
Affiliation.
- 1 Maastricht University.
- PMID: 23531762
- DOI: 10.1097/ACM.0b013e31828abf7f
PubMed Disclaimer
Similar articles
- AM last page: A guide to research paradigms relevant to medical education. Bergman E, de Feijter J, Frambach J, Godefrooij M, Slootweg I, Stalmeijer R, van der Zwet J. Bergman E, et al. Acad Med. 2012 Apr;87(4):545. doi: 10.1097/ACM.0b013e31824fbc8a. Acad Med. 2012. PMID: 22452919 No abstract available.
- AM last page. Understanding qualitative and quantitative research paradigms in academic medicine. Castillo-Page L, Bodilly S, Bunton SA. Castillo-Page L, et al. Acad Med. 2012 Mar;87(3):386. doi: 10.1097/ACM.0b013e318247c660. Acad Med. 2012. PMID: 22373638 No abstract available.
- AM last page: generalizability in medical education research. Artino AR Jr, Durning SJ, Boulet JR. Artino AR Jr, et al. Acad Med. 2011 Jul;86(7):917. doi: 10.1097/ACM.0b013e31821fb99e. Acad Med. 2011. PMID: 21715999 No abstract available.
- Mixing it but not mixed-up: mixed methods research in medical education (a critical narrative review). Maudsley G. Maudsley G. Med Teach. 2011;33(2):e92-104. doi: 10.3109/0142159X.2011.542523. Med Teach. 2011. PMID: 21275539 Review.
- [Qualitative methods in medical research--preconditions, potentials and limitations]. Malterud K. Malterud K. Tidsskr Nor Laegeforen. 2002 Oct 20;122(25):2468-72. Tidsskr Nor Laegeforen. 2002. PMID: 12448119 Review. Norwegian.
- Memes Adoption in Basic Medical Science Education as a Successful Learning Model: A Mixed Method Quasi-Experimental Study. Sharif A, Kasemy ZA, Rayan AH, Selim HMR, Aloshari SHA, Elkhamisy FAA. Sharif A, et al. Adv Med Educ Pract. 2024 May 29;15:487-500. doi: 10.2147/AMEP.S461757. eCollection 2024. Adv Med Educ Pract. 2024. PMID: 38826694 Free PMC article.
- Barriers and facilitators associated with the upscaling of the Transmural Trauma Care Model: a qualitative study. Ratter J, Wiertsema S, Ettahiri I, Mulder R, Grootjes A, Kee J, Donker M, Geleijn E, de Groot V, Ostelo RWJG, Bloemers FW, van Dongen JM. Ratter J, et al. BMC Health Serv Res. 2024 Feb 13;24(1):195. doi: 10.1186/s12913-024-10643-7. BMC Health Serv Res. 2024. PMID: 38350997 Free PMC article.
- How can the perceptions and experiences of medical educator stakeholders inform selection into medicine? An interpretative phenomenological pilot study. Lombard M, Poropat A, Alldridge L, Rogers GD. Lombard M, et al. MedEdPublish (2016). 2018 Dec 11;7:282. doi: 10.15694/mep.2018.0000282.1. eCollection 2018. MedEdPublish (2016). 2018. PMID: 38089195 Free PMC article.
- The impact of patients' social backgrounds assessment on nursing care: Qualitative research. Mizumoto J, Son D, Izumiya M, Horita S, Eto M. Mizumoto J, et al. J Gen Fam Med. 2023 Sep 22;24(6):332-342. doi: 10.1002/jgf2.650. eCollection 2023 Nov. J Gen Fam Med. 2023. PMID: 38025935 Free PMC article.
- Primary care-led weight-management intervention: qualitative insights into patient experiences at two-year follow-up. Spreckley M, de Lange J, Seidell J, Halberstadt J. Spreckley M, et al. Int J Qual Stud Health Well-being. 2023 Dec;18(1):2276576. doi: 10.1080/17482631.2023.2276576. Epub 2023 Nov 20. Int J Qual Stud Health Well-being. 2023. PMID: 38016037 Free PMC article.
- Search in MeSH
Related information
- Cited in Books
LinkOut - more resources
Full text sources.
- Ovid Technologies, Inc.
- Wolters Kluwer
Other Literature Sources
- scite Smart Citations
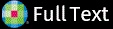
- Citation Manager
NCBI Literature Resources
MeSH PMC Bookshelf Disclaimer
The PubMed wordmark and PubMed logo are registered trademarks of the U.S. Department of Health and Human Services (HHS). Unauthorized use of these marks is strictly prohibited.
- Support & FAQ
AM last page. Quality criteria in qualitative and quantitative research
- Onderwijsontwikkeling & Onderwijsresearch
- SHE School of Health Professions Education
Research output : Contribution to journal › Article › Academic › peer-review
Original language | English |
---|---|
Pages (from-to) | 552 |
Journal | |
Volume | 88 |
Issue number | 4 |
DOIs | |
Publication status | Published - Apr 2013 |
- Education, Medical
- Qualitative Research
- Research Design
- Journal Article
Access to Document
- 10.1097/ACM.0b013e31828abf7f
T1 - AM last page. Quality criteria in qualitative and quantitative research
AU - Frambach, Janneke M
AU - van der Vleuten, Cees P M
AU - Durning, Steven J
PY - 2013/4
Y1 - 2013/4
KW - Education, Medical
KW - Humans
KW - Qualitative Research
KW - Research Design
KW - Journal Article
U2 - 10.1097/ACM.0b013e31828abf7f
DO - 10.1097/ACM.0b013e31828abf7f
M3 - Article
C2 - 23531762
SN - 1040-2446
JO - Academic Medicine
JF - Academic Medicine
AM last page. Quality criteria in qualitative and quantitative research.
Research output : Contribution to journal › Article › peer-review
Original language | English |
---|---|
Pages (from-to) | 552 |
Number of pages | 1 |
Journal | |
Volume | 88 |
Issue number | 4 |
State | Published - Apr 2013 |
Fingerprint
- quantitative research Social Sciences 100%
- qualitative research Social Sciences 78%
T1 - AM last page. Quality criteria in qualitative and quantitative research.
AU - Frambach, Janneke M.
AU - van der Vleuten, Cees P.M.
AU - Durning, Steven J.
PY - 2013/4
Y1 - 2013/4
UR - http://www.scopus.com/inward/record.url?scp=84878242017&partnerID=8YFLogxK
M3 - Article
C2 - 23531762
AN - SCOPUS:84878242017
JO - Unknown Journal
JF - Unknown Journal
Europe PMC requires Javascript to function effectively.
Either your web browser doesn't support Javascript or it is currently turned off. In the latter case, please turn on Javascript support in your web browser and reload this page.
- DOI: 10.1097/ACM.0b013e31828abf7f
- Corpus ID: 39554180
AM last page. Quality criteria in qualitative and quantitative research.
- J. Frambach , C. V. D. van der Vleuten , S. Durning
- Published in Academic medicine : journal… 1 April 2013
- Academic medicine : journal of the Association of American Medical Colleges
184 Citations
Exploring students’ perspectives on well-being and the change of united states medical licensing examination step 1 to pass/fail, assessment practices in continuing professional development activities in health professions: a scoping review, tools and instruments for needs assessment, monitoring and evaluation of health research capacity development activities at the individual and organizational level: a systematic review, elevating the behavioral and social sciences in premedical training: mcat2015, the meaning of feedback: medical students’ view, selection as a learning experience: an exploratory study, using focus groups in medical education research: amee guide no. 91, emergency physicians’ perceptions of critical appraisal skills: a qualitative study, standards for reporting qualitative research: a synthesis of recommendations, the introduction of advanced practice physiotherapy within dutch primary care is a quest for possibilities, added value, and mutual trust: a qualitative study amongst advanced practice physiotherapists and general practitioners.
- Highly Influenced
Related Papers
Showing 1 through 3 of 0 Related Papers
🇺🇦 make metadata, not war
AM Last Page: Quality Criteria in Qualitative and Quantitative Research
- J.M. Frambach
- C.P.M. van der Vleuten
- S.J. Durning
- Article / Letter to editor
- NCEBP 7: Effective primary care and public health
Similar works
Radboud Repository
This paper was published in Radboud Repository .
Having an issue?
Is data on this page outdated, violates copyrights or anything else? Report the problem now and we will take corresponding actions after reviewing your request.
Criteria for Good Qualitative Research: A Comprehensive Review
- Regular Article
- Open access
- Published: 18 September 2021
- Volume 31 , pages 679–689, ( 2022 )
Cite this article
You have full access to this open access article
- Drishti Yadav ORCID: orcid.org/0000-0002-2974-0323 1
90k Accesses
36 Citations
72 Altmetric
Explore all metrics
This review aims to synthesize a published set of evaluative criteria for good qualitative research. The aim is to shed light on existing standards for assessing the rigor of qualitative research encompassing a range of epistemological and ontological standpoints. Using a systematic search strategy, published journal articles that deliberate criteria for rigorous research were identified. Then, references of relevant articles were surveyed to find noteworthy, distinct, and well-defined pointers to good qualitative research. This review presents an investigative assessment of the pivotal features in qualitative research that can permit the readers to pass judgment on its quality and to condemn it as good research when objectively and adequately utilized. Overall, this review underlines the crux of qualitative research and accentuates the necessity to evaluate such research by the very tenets of its being. It also offers some prospects and recommendations to improve the quality of qualitative research. Based on the findings of this review, it is concluded that quality criteria are the aftereffect of socio-institutional procedures and existing paradigmatic conducts. Owing to the paradigmatic diversity of qualitative research, a single and specific set of quality criteria is neither feasible nor anticipated. Since qualitative research is not a cohesive discipline, researchers need to educate and familiarize themselves with applicable norms and decisive factors to evaluate qualitative research from within its theoretical and methodological framework of origin.
Similar content being viewed by others
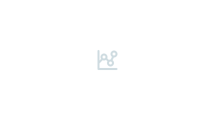
What is Qualitative in Qualitative Research
Qualitative Research: Ethical Considerations
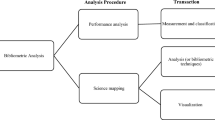
How to design bibliometric research: an overview and a framework proposal
Avoid common mistakes on your manuscript.
Introduction
“… It is important to regularly dialogue about what makes for good qualitative research” (Tracy, 2010 , p. 837)
To decide what represents good qualitative research is highly debatable. There are numerous methods that are contained within qualitative research and that are established on diverse philosophical perspectives. Bryman et al., ( 2008 , p. 262) suggest that “It is widely assumed that whereas quality criteria for quantitative research are well‐known and widely agreed, this is not the case for qualitative research.” Hence, the question “how to evaluate the quality of qualitative research” has been continuously debated. There are many areas of science and technology wherein these debates on the assessment of qualitative research have taken place. Examples include various areas of psychology: general psychology (Madill et al., 2000 ); counseling psychology (Morrow, 2005 ); and clinical psychology (Barker & Pistrang, 2005 ), and other disciplines of social sciences: social policy (Bryman et al., 2008 ); health research (Sparkes, 2001 ); business and management research (Johnson et al., 2006 ); information systems (Klein & Myers, 1999 ); and environmental studies (Reid & Gough, 2000 ). In the literature, these debates are enthused by the impression that the blanket application of criteria for good qualitative research developed around the positivist paradigm is improper. Such debates are based on the wide range of philosophical backgrounds within which qualitative research is conducted (e.g., Sandberg, 2000 ; Schwandt, 1996 ). The existence of methodological diversity led to the formulation of different sets of criteria applicable to qualitative research.
Among qualitative researchers, the dilemma of governing the measures to assess the quality of research is not a new phenomenon, especially when the virtuous triad of objectivity, reliability, and validity (Spencer et al., 2004 ) are not adequate. Occasionally, the criteria of quantitative research are used to evaluate qualitative research (Cohen & Crabtree, 2008 ; Lather, 2004 ). Indeed, Howe ( 2004 ) claims that the prevailing paradigm in educational research is scientifically based experimental research. Hypotheses and conjectures about the preeminence of quantitative research can weaken the worth and usefulness of qualitative research by neglecting the prominence of harmonizing match for purpose on research paradigm, the epistemological stance of the researcher, and the choice of methodology. Researchers have been reprimanded concerning this in “paradigmatic controversies, contradictions, and emerging confluences” (Lincoln & Guba, 2000 ).
In general, qualitative research tends to come from a very different paradigmatic stance and intrinsically demands distinctive and out-of-the-ordinary criteria for evaluating good research and varieties of research contributions that can be made. This review attempts to present a series of evaluative criteria for qualitative researchers, arguing that their choice of criteria needs to be compatible with the unique nature of the research in question (its methodology, aims, and assumptions). This review aims to assist researchers in identifying some of the indispensable features or markers of high-quality qualitative research. In a nutshell, the purpose of this systematic literature review is to analyze the existing knowledge on high-quality qualitative research and to verify the existence of research studies dealing with the critical assessment of qualitative research based on the concept of diverse paradigmatic stances. Contrary to the existing reviews, this review also suggests some critical directions to follow to improve the quality of qualitative research in different epistemological and ontological perspectives. This review is also intended to provide guidelines for the acceleration of future developments and dialogues among qualitative researchers in the context of assessing the qualitative research.
The rest of this review article is structured in the following fashion: Sect. Methods describes the method followed for performing this review. Section Criteria for Evaluating Qualitative Studies provides a comprehensive description of the criteria for evaluating qualitative studies. This section is followed by a summary of the strategies to improve the quality of qualitative research in Sect. Improving Quality: Strategies . Section How to Assess the Quality of the Research Findings? provides details on how to assess the quality of the research findings. After that, some of the quality checklists (as tools to evaluate quality) are discussed in Sect. Quality Checklists: Tools for Assessing the Quality . At last, the review ends with the concluding remarks presented in Sect. Conclusions, Future Directions and Outlook . Some prospects in qualitative research for enhancing its quality and usefulness in the social and techno-scientific research community are also presented in Sect. Conclusions, Future Directions and Outlook .
For this review, a comprehensive literature search was performed from many databases using generic search terms such as Qualitative Research , Criteria , etc . The following databases were chosen for the literature search based on the high number of results: IEEE Explore, ScienceDirect, PubMed, Google Scholar, and Web of Science. The following keywords (and their combinations using Boolean connectives OR/AND) were adopted for the literature search: qualitative research, criteria, quality, assessment, and validity. The synonyms for these keywords were collected and arranged in a logical structure (see Table 1 ). All publications in journals and conference proceedings later than 1950 till 2021 were considered for the search. Other articles extracted from the references of the papers identified in the electronic search were also included. A large number of publications on qualitative research were retrieved during the initial screening. Hence, to include the searches with the main focus on criteria for good qualitative research, an inclusion criterion was utilized in the search string.
From the selected databases, the search retrieved a total of 765 publications. Then, the duplicate records were removed. After that, based on the title and abstract, the remaining 426 publications were screened for their relevance by using the following inclusion and exclusion criteria (see Table 2 ). Publications focusing on evaluation criteria for good qualitative research were included, whereas those works which delivered theoretical concepts on qualitative research were excluded. Based on the screening and eligibility, 45 research articles were identified that offered explicit criteria for evaluating the quality of qualitative research and were found to be relevant to this review.
Figure 1 illustrates the complete review process in the form of PRISMA flow diagram. PRISMA, i.e., “preferred reporting items for systematic reviews and meta-analyses” is employed in systematic reviews to refine the quality of reporting.
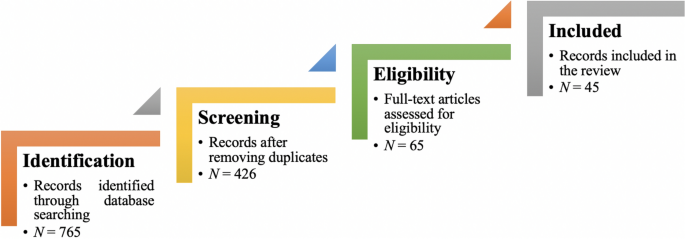
PRISMA flow diagram illustrating the search and inclusion process. N represents the number of records
Criteria for Evaluating Qualitative Studies
Fundamental criteria: general research quality.
Various researchers have put forward criteria for evaluating qualitative research, which have been summarized in Table 3 . Also, the criteria outlined in Table 4 effectively deliver the various approaches to evaluate and assess the quality of qualitative work. The entries in Table 4 are based on Tracy’s “Eight big‐tent criteria for excellent qualitative research” (Tracy, 2010 ). Tracy argues that high-quality qualitative work should formulate criteria focusing on the worthiness, relevance, timeliness, significance, morality, and practicality of the research topic, and the ethical stance of the research itself. Researchers have also suggested a series of questions as guiding principles to assess the quality of a qualitative study (Mays & Pope, 2020 ). Nassaji ( 2020 ) argues that good qualitative research should be robust, well informed, and thoroughly documented.
Qualitative Research: Interpretive Paradigms
All qualitative researchers follow highly abstract principles which bring together beliefs about ontology, epistemology, and methodology. These beliefs govern how the researcher perceives and acts. The net, which encompasses the researcher’s epistemological, ontological, and methodological premises, is referred to as a paradigm, or an interpretive structure, a “Basic set of beliefs that guides action” (Guba, 1990 ). Four major interpretive paradigms structure the qualitative research: positivist and postpositivist, constructivist interpretive, critical (Marxist, emancipatory), and feminist poststructural. The complexity of these four abstract paradigms increases at the level of concrete, specific interpretive communities. Table 5 presents these paradigms and their assumptions, including their criteria for evaluating research, and the typical form that an interpretive or theoretical statement assumes in each paradigm. Moreover, for evaluating qualitative research, quantitative conceptualizations of reliability and validity are proven to be incompatible (Horsburgh, 2003 ). In addition, a series of questions have been put forward in the literature to assist a reviewer (who is proficient in qualitative methods) for meticulous assessment and endorsement of qualitative research (Morse, 2003 ). Hammersley ( 2007 ) also suggests that guiding principles for qualitative research are advantageous, but methodological pluralism should not be simply acknowledged for all qualitative approaches. Seale ( 1999 ) also points out the significance of methodological cognizance in research studies.
Table 5 reflects that criteria for assessing the quality of qualitative research are the aftermath of socio-institutional practices and existing paradigmatic standpoints. Owing to the paradigmatic diversity of qualitative research, a single set of quality criteria is neither possible nor desirable. Hence, the researchers must be reflexive about the criteria they use in the various roles they play within their research community.
Improving Quality: Strategies
Another critical question is “How can the qualitative researchers ensure that the abovementioned quality criteria can be met?” Lincoln and Guba ( 1986 ) delineated several strategies to intensify each criteria of trustworthiness. Other researchers (Merriam & Tisdell, 2016 ; Shenton, 2004 ) also presented such strategies. A brief description of these strategies is shown in Table 6 .
It is worth mentioning that generalizability is also an integral part of qualitative research (Hays & McKibben, 2021 ). In general, the guiding principle pertaining to generalizability speaks about inducing and comprehending knowledge to synthesize interpretive components of an underlying context. Table 7 summarizes the main metasynthesis steps required to ascertain generalizability in qualitative research.
Figure 2 reflects the crucial components of a conceptual framework and their contribution to decisions regarding research design, implementation, and applications of results to future thinking, study, and practice (Johnson et al., 2020 ). The synergy and interrelationship of these components signifies their role to different stances of a qualitative research study.
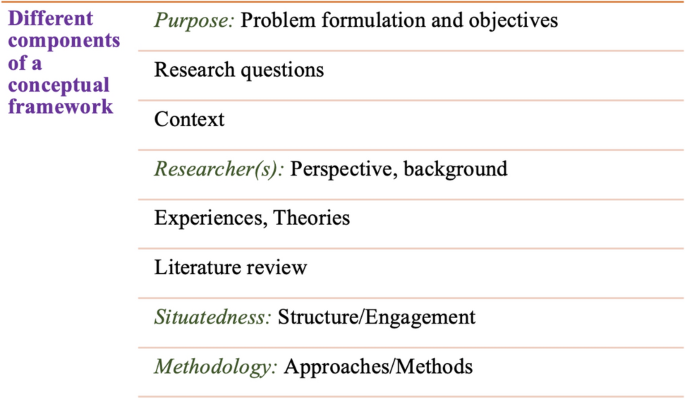
Essential elements of a conceptual framework
In a nutshell, to assess the rationale of a study, its conceptual framework and research question(s), quality criteria must take account of the following: lucid context for the problem statement in the introduction; well-articulated research problems and questions; precise conceptual framework; distinct research purpose; and clear presentation and investigation of the paradigms. These criteria would expedite the quality of qualitative research.
How to Assess the Quality of the Research Findings?
The inclusion of quotes or similar research data enhances the confirmability in the write-up of the findings. The use of expressions (for instance, “80% of all respondents agreed that” or “only one of the interviewees mentioned that”) may also quantify qualitative findings (Stenfors et al., 2020 ). On the other hand, the persuasive reason for “why this may not help in intensifying the research” has also been provided (Monrouxe & Rees, 2020 ). Further, the Discussion and Conclusion sections of an article also prove robust markers of high-quality qualitative research, as elucidated in Table 8 .
Quality Checklists: Tools for Assessing the Quality
Numerous checklists are available to speed up the assessment of the quality of qualitative research. However, if used uncritically and recklessly concerning the research context, these checklists may be counterproductive. I recommend that such lists and guiding principles may assist in pinpointing the markers of high-quality qualitative research. However, considering enormous variations in the authors’ theoretical and philosophical contexts, I would emphasize that high dependability on such checklists may say little about whether the findings can be applied in your setting. A combination of such checklists might be appropriate for novice researchers. Some of these checklists are listed below:
The most commonly used framework is Consolidated Criteria for Reporting Qualitative Research (COREQ) (Tong et al., 2007 ). This framework is recommended by some journals to be followed by the authors during article submission.
Standards for Reporting Qualitative Research (SRQR) is another checklist that has been created particularly for medical education (O’Brien et al., 2014 ).
Also, Tracy ( 2010 ) and Critical Appraisal Skills Programme (CASP, 2021 ) offer criteria for qualitative research relevant across methods and approaches.
Further, researchers have also outlined different criteria as hallmarks of high-quality qualitative research. For instance, the “Road Trip Checklist” (Epp & Otnes, 2021 ) provides a quick reference to specific questions to address different elements of high-quality qualitative research.
Conclusions, Future Directions, and Outlook
This work presents a broad review of the criteria for good qualitative research. In addition, this article presents an exploratory analysis of the essential elements in qualitative research that can enable the readers of qualitative work to judge it as good research when objectively and adequately utilized. In this review, some of the essential markers that indicate high-quality qualitative research have been highlighted. I scope them narrowly to achieve rigor in qualitative research and note that they do not completely cover the broader considerations necessary for high-quality research. This review points out that a universal and versatile one-size-fits-all guideline for evaluating the quality of qualitative research does not exist. In other words, this review also emphasizes the non-existence of a set of common guidelines among qualitative researchers. In unison, this review reinforces that each qualitative approach should be treated uniquely on account of its own distinctive features for different epistemological and disciplinary positions. Owing to the sensitivity of the worth of qualitative research towards the specific context and the type of paradigmatic stance, researchers should themselves analyze what approaches can be and must be tailored to ensemble the distinct characteristics of the phenomenon under investigation. Although this article does not assert to put forward a magic bullet and to provide a one-stop solution for dealing with dilemmas about how, why, or whether to evaluate the “goodness” of qualitative research, it offers a platform to assist the researchers in improving their qualitative studies. This work provides an assembly of concerns to reflect on, a series of questions to ask, and multiple sets of criteria to look at, when attempting to determine the quality of qualitative research. Overall, this review underlines the crux of qualitative research and accentuates the need to evaluate such research by the very tenets of its being. Bringing together the vital arguments and delineating the requirements that good qualitative research should satisfy, this review strives to equip the researchers as well as reviewers to make well-versed judgment about the worth and significance of the qualitative research under scrutiny. In a nutshell, a comprehensive portrayal of the research process (from the context of research to the research objectives, research questions and design, speculative foundations, and from approaches of collecting data to analyzing the results, to deriving inferences) frequently proliferates the quality of a qualitative research.
Prospects : A Road Ahead for Qualitative Research
Irrefutably, qualitative research is a vivacious and evolving discipline wherein different epistemological and disciplinary positions have their own characteristics and importance. In addition, not surprisingly, owing to the sprouting and varied features of qualitative research, no consensus has been pulled off till date. Researchers have reflected various concerns and proposed several recommendations for editors and reviewers on conducting reviews of critical qualitative research (Levitt et al., 2021 ; McGinley et al., 2021 ). Following are some prospects and a few recommendations put forward towards the maturation of qualitative research and its quality evaluation:
In general, most of the manuscript and grant reviewers are not qualitative experts. Hence, it is more likely that they would prefer to adopt a broad set of criteria. However, researchers and reviewers need to keep in mind that it is inappropriate to utilize the same approaches and conducts among all qualitative research. Therefore, future work needs to focus on educating researchers and reviewers about the criteria to evaluate qualitative research from within the suitable theoretical and methodological context.
There is an urgent need to refurbish and augment critical assessment of some well-known and widely accepted tools (including checklists such as COREQ, SRQR) to interrogate their applicability on different aspects (along with their epistemological ramifications).
Efforts should be made towards creating more space for creativity, experimentation, and a dialogue between the diverse traditions of qualitative research. This would potentially help to avoid the enforcement of one's own set of quality criteria on the work carried out by others.
Moreover, journal reviewers need to be aware of various methodological practices and philosophical debates.
It is pivotal to highlight the expressions and considerations of qualitative researchers and bring them into a more open and transparent dialogue about assessing qualitative research in techno-scientific, academic, sociocultural, and political rooms.
Frequent debates on the use of evaluative criteria are required to solve some potentially resolved issues (including the applicability of a single set of criteria in multi-disciplinary aspects). Such debates would not only benefit the group of qualitative researchers themselves, but primarily assist in augmenting the well-being and vivacity of the entire discipline.
To conclude, I speculate that the criteria, and my perspective, may transfer to other methods, approaches, and contexts. I hope that they spark dialog and debate – about criteria for excellent qualitative research and the underpinnings of the discipline more broadly – and, therefore, help improve the quality of a qualitative study. Further, I anticipate that this review will assist the researchers to contemplate on the quality of their own research, to substantiate research design and help the reviewers to review qualitative research for journals. On a final note, I pinpoint the need to formulate a framework (encompassing the prerequisites of a qualitative study) by the cohesive efforts of qualitative researchers of different disciplines with different theoretic-paradigmatic origins. I believe that tailoring such a framework (of guiding principles) paves the way for qualitative researchers to consolidate the status of qualitative research in the wide-ranging open science debate. Dialogue on this issue across different approaches is crucial for the impending prospects of socio-techno-educational research.
Amin, M. E. K., Nørgaard, L. S., Cavaco, A. M., Witry, M. J., Hillman, L., Cernasev, A., & Desselle, S. P. (2020). Establishing trustworthiness and authenticity in qualitative pharmacy research. Research in Social and Administrative Pharmacy, 16 (10), 1472–1482.
Article Google Scholar
Barker, C., & Pistrang, N. (2005). Quality criteria under methodological pluralism: Implications for conducting and evaluating research. American Journal of Community Psychology, 35 (3–4), 201–212.
Bryman, A., Becker, S., & Sempik, J. (2008). Quality criteria for quantitative, qualitative and mixed methods research: A view from social policy. International Journal of Social Research Methodology, 11 (4), 261–276.
Caelli, K., Ray, L., & Mill, J. (2003). ‘Clear as mud’: Toward greater clarity in generic qualitative research. International Journal of Qualitative Methods, 2 (2), 1–13.
CASP (2021). CASP checklists. Retrieved May 2021 from https://casp-uk.net/casp-tools-checklists/
Cohen, D. J., & Crabtree, B. F. (2008). Evaluative criteria for qualitative research in health care: Controversies and recommendations. The Annals of Family Medicine, 6 (4), 331–339.
Denzin, N. K., & Lincoln, Y. S. (2005). Introduction: The discipline and practice of qualitative research. In N. K. Denzin & Y. S. Lincoln (Eds.), The sage handbook of qualitative research (pp. 1–32). Sage Publications Ltd.
Google Scholar
Elliott, R., Fischer, C. T., & Rennie, D. L. (1999). Evolving guidelines for publication of qualitative research studies in psychology and related fields. British Journal of Clinical Psychology, 38 (3), 215–229.
Epp, A. M., & Otnes, C. C. (2021). High-quality qualitative research: Getting into gear. Journal of Service Research . https://doi.org/10.1177/1094670520961445
Guba, E. G. (1990). The paradigm dialog. In Alternative paradigms conference, mar, 1989, Indiana u, school of education, San Francisco, ca, us . Sage Publications, Inc.
Hammersley, M. (2007). The issue of quality in qualitative research. International Journal of Research and Method in Education, 30 (3), 287–305.
Haven, T. L., Errington, T. M., Gleditsch, K. S., van Grootel, L., Jacobs, A. M., Kern, F. G., & Mokkink, L. B. (2020). Preregistering qualitative research: A Delphi study. International Journal of Qualitative Methods, 19 , 1609406920976417.
Hays, D. G., & McKibben, W. B. (2021). Promoting rigorous research: Generalizability and qualitative research. Journal of Counseling and Development, 99 (2), 178–188.
Horsburgh, D. (2003). Evaluation of qualitative research. Journal of Clinical Nursing, 12 (2), 307–312.
Howe, K. R. (2004). A critique of experimentalism. Qualitative Inquiry, 10 (1), 42–46.
Johnson, J. L., Adkins, D., & Chauvin, S. (2020). A review of the quality indicators of rigor in qualitative research. American Journal of Pharmaceutical Education, 84 (1), 7120.
Johnson, P., Buehring, A., Cassell, C., & Symon, G. (2006). Evaluating qualitative management research: Towards a contingent criteriology. International Journal of Management Reviews, 8 (3), 131–156.
Klein, H. K., & Myers, M. D. (1999). A set of principles for conducting and evaluating interpretive field studies in information systems. MIS Quarterly, 23 (1), 67–93.
Lather, P. (2004). This is your father’s paradigm: Government intrusion and the case of qualitative research in education. Qualitative Inquiry, 10 (1), 15–34.
Levitt, H. M., Morrill, Z., Collins, K. M., & Rizo, J. L. (2021). The methodological integrity of critical qualitative research: Principles to support design and research review. Journal of Counseling Psychology, 68 (3), 357.
Lincoln, Y. S., & Guba, E. G. (1986). But is it rigorous? Trustworthiness and authenticity in naturalistic evaluation. New Directions for Program Evaluation, 1986 (30), 73–84.
Lincoln, Y. S., & Guba, E. G. (2000). Paradigmatic controversies, contradictions and emerging confluences. In N. K. Denzin & Y. S. Lincoln (Eds.), Handbook of qualitative research (2nd ed., pp. 163–188). Sage Publications.
Madill, A., Jordan, A., & Shirley, C. (2000). Objectivity and reliability in qualitative analysis: Realist, contextualist and radical constructionist epistemologies. British Journal of Psychology, 91 (1), 1–20.
Mays, N., & Pope, C. (2020). Quality in qualitative research. Qualitative Research in Health Care . https://doi.org/10.1002/9781119410867.ch15
McGinley, S., Wei, W., Zhang, L., & Zheng, Y. (2021). The state of qualitative research in hospitality: A 5-year review 2014 to 2019. Cornell Hospitality Quarterly, 62 (1), 8–20.
Merriam, S., & Tisdell, E. (2016). Qualitative research: A guide to design and implementation. San Francisco, US.
Meyer, M., & Dykes, J. (2019). Criteria for rigor in visualization design study. IEEE Transactions on Visualization and Computer Graphics, 26 (1), 87–97.
Monrouxe, L. V., & Rees, C. E. (2020). When I say… quantification in qualitative research. Medical Education, 54 (3), 186–187.
Morrow, S. L. (2005). Quality and trustworthiness in qualitative research in counseling psychology. Journal of Counseling Psychology, 52 (2), 250.
Morse, J. M. (2003). A review committee’s guide for evaluating qualitative proposals. Qualitative Health Research, 13 (6), 833–851.
Nassaji, H. (2020). Good qualitative research. Language Teaching Research, 24 (4), 427–431.
O’Brien, B. C., Harris, I. B., Beckman, T. J., Reed, D. A., & Cook, D. A. (2014). Standards for reporting qualitative research: A synthesis of recommendations. Academic Medicine, 89 (9), 1245–1251.
O’Connor, C., & Joffe, H. (2020). Intercoder reliability in qualitative research: Debates and practical guidelines. International Journal of Qualitative Methods, 19 , 1609406919899220.
Reid, A., & Gough, S. (2000). Guidelines for reporting and evaluating qualitative research: What are the alternatives? Environmental Education Research, 6 (1), 59–91.
Rocco, T. S. (2010). Criteria for evaluating qualitative studies. Human Resource Development International . https://doi.org/10.1080/13678868.2010.501959
Sandberg, J. (2000). Understanding human competence at work: An interpretative approach. Academy of Management Journal, 43 (1), 9–25.
Schwandt, T. A. (1996). Farewell to criteriology. Qualitative Inquiry, 2 (1), 58–72.
Seale, C. (1999). Quality in qualitative research. Qualitative Inquiry, 5 (4), 465–478.
Shenton, A. K. (2004). Strategies for ensuring trustworthiness in qualitative research projects. Education for Information, 22 (2), 63–75.
Sparkes, A. C. (2001). Myth 94: Qualitative health researchers will agree about validity. Qualitative Health Research, 11 (4), 538–552.
Spencer, L., Ritchie, J., Lewis, J., & Dillon, L. (2004). Quality in qualitative evaluation: A framework for assessing research evidence.
Stenfors, T., Kajamaa, A., & Bennett, D. (2020). How to assess the quality of qualitative research. The Clinical Teacher, 17 (6), 596–599.
Taylor, E. W., Beck, J., & Ainsworth, E. (2001). Publishing qualitative adult education research: A peer review perspective. Studies in the Education of Adults, 33 (2), 163–179.
Tong, A., Sainsbury, P., & Craig, J. (2007). Consolidated criteria for reporting qualitative research (COREQ): A 32-item checklist for interviews and focus groups. International Journal for Quality in Health Care, 19 (6), 349–357.
Tracy, S. J. (2010). Qualitative quality: Eight “big-tent” criteria for excellent qualitative research. Qualitative Inquiry, 16 (10), 837–851.
Download references
Open access funding provided by TU Wien (TUW).
Author information
Authors and affiliations.
Faculty of Informatics, Technische Universität Wien, 1040, Vienna, Austria
Drishti Yadav
You can also search for this author in PubMed Google Scholar
Corresponding author
Correspondence to Drishti Yadav .
Ethics declarations
Conflict of interest.
The author declares no conflict of interest.
Additional information
Publisher's note.
Springer Nature remains neutral with regard to jurisdictional claims in published maps and institutional affiliations.
Rights and permissions
Open Access This article is licensed under a Creative Commons Attribution 4.0 International License, which permits use, sharing, adaptation, distribution and reproduction in any medium or format, as long as you give appropriate credit to the original author(s) and the source, provide a link to the Creative Commons licence, and indicate if changes were made. The images or other third party material in this article are included in the article's Creative Commons licence, unless indicated otherwise in a credit line to the material. If material is not included in the article's Creative Commons licence and your intended use is not permitted by statutory regulation or exceeds the permitted use, you will need to obtain permission directly from the copyright holder. To view a copy of this licence, visit http://creativecommons.org/licenses/by/4.0/ .
Reprints and permissions
About this article
Yadav, D. Criteria for Good Qualitative Research: A Comprehensive Review. Asia-Pacific Edu Res 31 , 679–689 (2022). https://doi.org/10.1007/s40299-021-00619-0
Download citation
Accepted : 28 August 2021
Published : 18 September 2021
Issue Date : December 2022
DOI : https://doi.org/10.1007/s40299-021-00619-0
Share this article
Anyone you share the following link with will be able to read this content:
Sorry, a shareable link is not currently available for this article.
Provided by the Springer Nature SharedIt content-sharing initiative
- Qualitative research
- Evaluative criteria
- Find a journal
- Publish with us
- Track your research
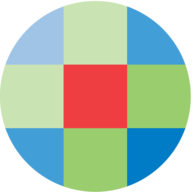
- Subscribe to journal Subscribe
- Get new issue alerts Get alerts
Secondary Logo
Journal logo.
Colleague's E-mail is Invalid
Your message has been successfully sent to your colleague.
Save my selection
Standards for Reporting Qualitative Research
A synthesis of recommendations.
O’Brien, Bridget C. PhD; Harris, Ilene B. PhD; Beckman, Thomas J. MD; Reed, Darcy A. MD, MPH; Cook, David A. MD, MHPE
Dr. O’Brien is assistant professor, Department of Medicine and Office of Research and Development in Medical Education, University of California, San Francisco, School of Medicine, San Francisco, California.
Dr. Harris is professor and head, Department of Medical Education, University of Illinois at Chicago College of Medicine, Chicago, Illinois.
Dr. Beckman is professor of medicine and medical education, Department of Medicine, Mayo Clinic College of Medicine, Rochester, Minnesota.
Dr. Reed is associate professor of medicine and medical education, Department of Medicine, Mayo Clinic College of Medicine, Rochester, Minnesota.
Dr. Cook is associate director, Mayo Clinic Online Learning, research chair, Mayo Multidisciplinary Simulation Center, and professor of medicine and medical education, Mayo Clinic College of Medicine, Rochester, Minnesota.
Funding/Support: This study was funded in part by a research review grant from the Society for Directors of Research in Medical Education.
Other disclosures: None reported.
Ethical approval: Reported as not applicable.
Disclaimer: The funding agency had no role in the study design, analysis, interpretation, writing of the manuscript, or decision to submit the manuscript for publication.
Supplemental digital content for this article is available at https://links.lww.com/ACADMED/A218 .
Correspondence should be addressed to Dr. O’Brien, Office of Research and Development in Medical Education, UCSF School of Medicine, Box 3202, 1855 Folsom St., Suite 200, San Francisco, CA 94143-3202; e-mail: [email protected] .
Purpose
Standards for reporting exist for many types of quantitative research, but currently none exist for the broad spectrum of qualitative research. The purpose of the present study was to formulate and define standards for reporting qualitative research while preserving the requisite flexibility to accommodate various paradigms, approaches, and methods.
Method
The authors identified guidelines, reporting standards, and critical appraisal criteria for qualitative research by searching PubMed, Web of Science, and Google through July 2013; reviewing the reference lists of retrieved sources; and contacting experts. Specifically, two authors reviewed a sample of sources to generate an initial set of items that were potentially important in reporting qualitative research. Through an iterative process of reviewing sources, modifying the set of items, and coding all sources for items, the authors prepared a near-final list of items and descriptions and sent this list to five external reviewers for feedback. The final items and descriptions included in the reporting standards reflect this feedback.
Results
The Standards for Reporting Qualitative Research (SRQR) consists of 21 items. The authors define and explain key elements of each item and provide examples from recently published articles to illustrate ways in which the standards can be met.
Conclusions
The SRQR aims to improve the transparency of all aspects of qualitative research by providing clear standards for reporting qualitative research. These standards will assist authors during manuscript preparation, editors and reviewers in evaluating a manuscript for potential publication, and readers when critically appraising, applying, and synthesizing study findings.
Qualitative research contributes to the literature in many disciplines by describing, interpreting, and generating theories about social interactions and individual experiences as they occur in natural, rather than experimental, situations. 1–3 Some recent examples include studies of professional dilemmas, 4 medical students’ early experiences of workplace learning, 5 patients’ experiences of disease and interventions, 6–8 and patients’ perspectives about incident disclosures. 9 The purpose of qualitative research is to understand the perspectives/experiences of individuals or groups and the contexts in which these perspectives or experiences are situated. 1 , 2 , 10
Qualitative research is increasingly common and valued in the medical and medical education literature. 1 , 10–13 However, the quality of such research can be difficult to evaluate because of incomplete reporting of key elements. 14 , 15 Quality is multifaceted and includes consideration of the importance of the research question, the rigor of the research methods, the appropriateness and salience of the inferences, and the clarity and completeness of reporting. 16 , 17 Although there is much debate about standards for methodological rigor in qualitative research, 13 , 14 , 18–20 there is widespread agreement about the need for clear and complete reporting. 14 , 21 , 22 Optimal reporting would enable editors, reviewers, other researchers, and practitioners to critically appraise qualitative studies and apply and synthesize the results. One important step in improving the quality of reporting is to formulate and define clear reporting standards.
Authors have proposed guidelines for the quality of qualitative research, including those in the fields of medical education, 23–25 clinical and health services research, 26–28 and general education research. 29 , 30 Yet in nearly all cases, the authors do not describe how the guidelines were created, and often fail to distinguish reporting quality from the other facets of quality (e.g., the research question or methods). Several authors suggest standards for reporting qualitative research, 15 , 20 , 29–33 but their articles focus on a subset of qualitative data collection methods (e.g., interviews), fail to explain how the authors developed the reporting criteria, narrowly construe qualitative research (e.g., thematic analysis) in ways that may exclude other approaches, and/or lack specific examples to help others see how the standards might be achieved. Thus, there remains a compelling need for defensible and broadly applicable standards for reporting qualitative research.
We designed and carried out the present study to formulate and define standards for reporting qualitative research through a rigorous synthesis of published articles and expert recommendations.
We formulated standards for reporting qualitative research by using a rigorous and systematic approach in which we reviewed previously proposed recommendations by experts in qualitative methods. Our research team consisted of two PhD researchers and one physician with formal training and experience in qualitative methods, and two physicians with experience, but no formal training, in qualitative methods.
We first identified previously proposed recommendations by searching PubMed, Web of Science, and Google using combinations of terms such as “qualitative methods,” “qualitative research,” “qualitative guidelines,” “qualitative standards,” and “critical appraisal” and by reviewing the reference lists of retrieved sources, reviewing the Equator Network, 22 and contacting experts. We conducted our first search in January 2007 and our last search in July 2013. Most recommendations were published in peer-reviewed journals, but some were available only on the Internet, and one was an interim draft from a national organization. We report the full set of the 40 sources reviewed in Supplemental Digital Appendix 1, found at https://links.lww.com/ACADMED/A218 .
Two of us (B.O., I.H.) reviewed an initial sample of sources to generate a comprehensive list of items that were potentially important in reporting qualitative research (Draft A). All of us then worked in pairs to review all sources and code the presence or absence of each item in a given source. From Draft A, we then distilled a shorter list (Draft B) by identifying core concepts and combining related items, taking into account the number of times each item appeared in these sources. We then compared the items in Draft B with material in the original sources to check for missing concepts, modify accordingly, and add explanatory definitions to create a prefinal list of items (Draft C).
We circulated Draft C to five experienced qualitative researchers (see the acknowledgments) for review. We asked them to note any omitted or redundant items and to suggest improvements to the wording to enhance clarity and relevance across a broad spectrum of qualitative inquiry. In response to their reviews, we consolidated some items and made minor revisions to the wording of labels and definitions to create the final set of reporting standards—the Standards for Reporting Qualitative Research (SRQR)—summarized in Table 1 .

To explicate how the final set of standards reflect the material in the original sources, two of us (B.O., D.A.C.) selected by consensus the 25 most complete sources of recommendations and identified which standards reflected the concepts found in each original source (see Table 2 ).

The SRQR is a list of 21 items that we consider essential for complete, transparent reporting of qualitative research (see Table 1 ). As explained above, we developed these items through a rigorous synthesis of prior recommendations and concepts from published sources (see Table 2 ; see also Supplemental Digital Appendix 1, found at https://links.lww.com/ACADMED/A218 ) and expert review. These 21 items provide a framework and recommendations for reporting qualitative studies. Given the wide range of qualitative approaches and methodologies, we attempted to select items with broad relevance.
The SRQR includes the article’s title and abstract (items 1 and 2); problem formulation and research question (items 3 and 4); research design and methods of data collection and analysis (items 5 through 15); results, interpretation, discussion, and integration (items 16 through 19); and other information (items 20 and 21). Supplemental Digital Appendix 2, found at https://links.lww.com/ACADMED/A218 , contains a detailed explanation of each item, along with examples from recently published qualitative studies. Below, we briefly describe the standards, with a particular focus on those unique to qualitative research.
Titles, abstracts, and introductory material. Reporting standards for titles, abstracts, and introductory material (problem formulation, research question) in qualitative research are very similar to those for quantitative research, except that the results reported in the abstract are narrative rather than numerical, and authors rarely present a specific hypothesis. 29 , 30
Research design and methods. Reporting on research design and methods of data collection and analysis highlights several distinctive features of qualitative research. Many of the criteria we reviewed focus not only on identifying and describing all aspects of the methods (e.g., approach, researcher characteristics and role, sampling strategy, context, data collection and analysis) but also on justifying each choice. 13 , 14 This ensures that authors make their assumptions and decisions transparent to readers. This standard is less commonly expected in quantitative research, perhaps because most quantitative researchers share positivist assumptions and generally agree about standards for rigor of various study designs and sampling techniques. 14 Just as quantitative reporting standards encourage authors to describe how they implemented methods such as randomization and measurement validity, several qualitative reporting criteria recommend that authors describe how they implemented a presumably familiar technique in their study rather than simply mentioning the technique. 10 , 14 , 32 For example, authors often state that data collection occurred until saturation, with no mention of how they defined and recognized saturation. Similarly, authors often mention an “iterative process,” with minimal description of the nature of the iterations. The SRQR emphasizes the importance of explaining and elaborating on these important processes. Nearly all of the original sources recommended describing the characteristics and role of the researcher (i.e., reflexivity). Members of the research team often form relationships with participants, and analytic processes are highly interpretive in most qualitative research. Therefore, reviewers and readers must understand how these relationships and the researchers’ perspectives and assumptions influenced data collection and interpretation. 15 , 23 , 26 , 34
Results. Reporting of qualitative research results should identify the main analytic findings. Often, these findings involve interpretation and contextualization, which represent a departure from the tradition in quantitative studies of objectively reporting results. The presentation of results often varies with the specific qualitative approach and methodology; thus, rigid rules for reporting qualitative findings are inappropriate. However, authors should provide evidence (e.g., examples, quotes, or text excerpts) to substantiate the main analytic findings. 20 , 29
Discussion. The discussion of qualitative results will generally include connections to existing literature and/or theoretical or conceptual frameworks, the scope and boundaries of the results (transferability), and study limitations. 10–12 , 28 In some qualitative traditions, the results and discussion may not have distinct boundaries; we recommend that authors include the substance of each item regardless of the section in which it appears.
The purpose of the SRQR is to improve the quality of reporting of qualitative research studies. We hope that these 21 recommended reporting standards will assist authors during manuscript preparation, editors and reviewers in evaluating a manuscript for potential publication, and readers when critically appraising, applying, and synthesizing study findings. As with other reporting guidelines, 35–37 we anticipate that the SRQR will evolve as it is applied and evaluated in practice. We welcome suggestions for refinement.
Qualitative studies explore “how?” and “why?” questions related to social or human problems or phenomena. 10 , 38 Purposes of qualitative studies include understanding meaning from participants’ perspectives (How do they interpret or make sense of an event, situation, or action?); understanding the nature and influence of the context surrounding events or actions; generating theories about new or poorly understood events, situations, or actions; and understanding the processes that led to a desired (or undesired) outcome. 38 Many different approaches (e.g., ethnography, phenomenology, discourse analysis, case study, grounded theory) and methodologies (e.g., interviews, focus groups, observation, analysis of documents) may be used in qualitative research, each with its own assumptions and traditions. 1 , 2 A strength of many qualitative approaches and methodologies is the opportunity for flexibility and adaptability throughout the data collection and analysis process. We endeavored to maintain that flexibility by intentionally defining items to avoid favoring one approach or method over others. As such, we trust that the SRQR will support all approaches and methods of qualitative research by making reports more explicit and transparent, while still allowing investigators the flexibility to use the study design and reporting format most appropriate to their study. It may be helpful, in the future, to develop approach-specific extensions of the SRQR, as has been done for guidelines in quantitative research (e.g., the CONSORT extensions). 37
Limitations, strengths, and boundaries
We deliberately avoided recommendations that define methodological rigor, and therefore it would be inappropriate to use the SRQR to judge the quality of research methods and findings. Many of the original sources from which we derived the SRQR were intended as criteria for methodological rigor or critical appraisal rather than reporting; for these, we inferred the information that would be needed to evaluate the criterion. Occasionally, we found conflicting recommendations in the literature (e.g., recommending specific techniques such as multiple coders or member checking to demonstrate trustworthiness); we resolved these conflicting recommendations through selection of the most frequent recommendations and by consensus among ourselves.
Some qualitative researchers have described the limitations of checklists as a means to improve methodological rigor. 13 We nonetheless believe that a checklist for reporting standards will help to enhance the transparency of qualitative research studies and thereby advance the field. 29 , 39
Strengths of this work include the grounding in previously published criteria, the diversity of experience and perspectives among us, and critical review by experts in three countries.
Implications and application
Similar to other reporting guidelines, 35–37 the SRQR may be viewed as a starting point for defining reporting standards in qualitative research. Although our personal experience lies in health professions education, the SRQR is based on sources originating in diverse health care and non-health-care fields. We intentionally crafted the SRQR to include various paradigms, approaches, and methodologies used in qualitative research. The elaborations offered in Supplemental Digital Appendix 2 (see https://links.lww.com/ACADMED/A218 ) should provide sufficient description and examples to enable both novice and experienced researchers to use these standards. Thus, the SRQR should apply broadly across disciplines, methodologies, topics, study participants, and users.
The SRQR items reflect information essential for inclusion in a qualitative research report, but should not be viewed as prescribing a rigid format or standardized content. Individual study needs, author preferences, and journal requirements may necessitate a different sequence or organization than that shown in Table 1 . Journal word restrictions may prevent a full exposition of each item, and the relative importance of a given item will vary by study. Thus, although all 21 standards would ideally be reflected in any given report, authors should prioritize attention to those items that are most relevant to the given study, findings, context, and readership.
Application of the SRQR need not be limited to the writing phase of a given study. These standards can assist researchers in planning qualitative studies and in the careful documentation of processes and decisions made throughout the study. By considering these recommendations early on, researchers may be more likely to identify the paradigm and approach most appropriate to their research, consider and use strategies for ensuring trustworthiness, and keep track of procedures and decisions.
Journal editors can facilitate the review process by providing the SRQR to reviewers and applying its standards, thus establishing more explicit expectations for qualitative studies. Although the recommendations do not address or advocate specific approaches, methods, or quality standards, they do help reviewers identify information that is missing from manuscripts.
As authors and editors apply the SRQR, readers will have more complete information about a given study, thus facilitating judgments about the trustworthiness, relevance, and transferability of findings to their own context and/or to related literature. Complete reporting will also facilitate meaningful synthesis of qualitative results across studies. 40 We anticipate that such transparency will, over time, help to identify previously unappreciated gaps in the rigor and relevance of research findings. Investigators, editors, and educators can then work to remedy these deficiencies and, thereby, enhance the overall quality of qualitative research.
Acknowledgments: The authors thank Margaret Bearman, PhD, Calvin Chou, MD, PhD, Karen Hauer, MD, Ayelet Kuper, MD, DPhil, Arianne Teherani, PhD, and participants in the UCSF weekly educational scholarship works-in-progress group (ESCape) for critically reviewing the Standards for Reporting Qualitative Research.
References Cited Only in Table 2
Supplemental digital content.
- ACADMED_89_9_2014_05_22_OBRIEN_1301196_SDC1.pdf; [PDF] (385 KB)
- + Favorites
- View in Gallery
Readers Of this Article Also Read
The distinctions between theory, theoretical framework, and conceptual framework, summary of instructions for authors, common qualitative methodologies and research designs in health professions..., the problem and power of professionalism: a critical analysis of medical..., boyer's expanded definitions of scholarship, the standards for assessing....
Criteria for Quality in Quantitative and Qualitative Writing Research
Good writing research is characterized by evidence that is trustworthy, applicable to multiple practical settings, consistent and transparent about its position—regardless of whether a qualitative or a quantitative approach is used. Qualitative and quantitative writing research both require standards for good evidence, even though the articulation of criteria in the two approaches is different. Below, we provide a description of high quality writing research practices. While individual descriptors might not apply equally to all approaches, editors and authors can refer to these guidelines in assessing the quality of chapters.
Techniques for Quality in Quantitative Writing Research | Quality Criteria in Quantitative Writing Research | Quality Principles | Quality Criteria in Qualitative Writing Research | Techniques for Quality in Qualitative Writing Research |
The extent to which observed effects can be attributed to the independent variable | Truth Value of Evidence |
The extent to which study findings are trustworthy and believable to others ), methods ( ), researchers ( ) and theories ( ). ). | ||
The extent to which results can be generalized from the research sample to the population . | Applicability of Evidence |
The extent to which findings can be transferred or applied in different settings e.g. ). | ||
The extent to which results are consistent if the study would be replicated | Consistency of Evidence |
The extent to which findings are consistent in relation to the contexts in which they were generated ). | ||
The extent to which personal biases are removed and value-free information is gathered | Ethical Treatment of Evidence |
The extent to which findings are based on the study's participants and settings and not researchers' biases |
Please also consult the series statement of ethical practices , its language policy , and the WAC Clearinghouse peer review process .
Suggestions for further reading:
- Levitt, H. M. (2019). Reporting qualitative research in psychology . APA.
- Cooper, H. (2019). Reporting quantitative research in psychology . APA.
This overview of quality criteria for the International Exchanges on the Study of Writing book series uses a similar layout and is informed by the article, "AM Last Page: Quality Criteria in Qualitative and Quantitative Research" by Janneke M. Frambach, Cees P. M. van der Vleuten, and Steven J.Durning, which was published in Academic Medicine (volume 88, issue 4, page 552) in April 2013. With the first author’s permission, we have adapted the table for research in writing studies. We also acknowledge revision comments for this document offered by Rebecca Babcock ( https://www.utpb.edu/directory/faculty -staff/babcock_r) and Ruth Villalón ( https://orcid.org/0000-0003-1600-8026 ).

An official website of the United States government
The .gov means it’s official. Federal government websites often end in .gov or .mil. Before sharing sensitive information, make sure you’re on a federal government site.
The site is secure. The https:// ensures that you are connecting to the official website and that any information you provide is encrypted and transmitted securely.
- Publications
- Account settings
Preview improvements coming to the PMC website in October 2024. Learn More or Try it out now .
- Advanced Search
- Journal List
- BMC Med Ethics

Research across the disciplines: a road map for quality criteria in empirical ethics research
Marcel mertz.
1 Institute for History of Medicine and Medical Ethics, Research Unit Ethics, University of Cologne, Herderstr. 54, D-50931 Cologne, Germany
2 Institute for Ethics, History and Philosophy of Medicine, Hannover Medical School, Carl-Neuberg-Str. 1, D-30625 Hannover, Germany
Julia Inthorn
3 Department of Medical Ethics and History of Medicine, University Medical Center Göttingen, Humboldtallee 36, D-37073 Göttingen, Germany
Günter Renz
4 Protestant Academy Bad Boll, Bad Boll, Akademieweg 11, D-73087 Bad Boll, Germany
Lillian Geza Rothenberger
5 Formerly at: Institute of Ethics and History in Medicine, Centre for Medicine, Society and Prevention, University of Tübingen, Gartenstr 47, D-72074 Tübingen, Germany
Sabine Salloch
6 Institute for Medical Ethics and History of Medicine, NRW Junior Research Group “Medical Ethics at the End of Life: Norm and Empiricism”, Ruhr University Bochum, Malakowturm, Markstr 258a, D-44799 Bochum, Germany
Jan Schildmann
Sabine wöhlke, silke schicktanz.
Research in the field of Empirical Ethics (EE) uses a broad variety of empirical methodologies, such as surveys, interviews and observation, developed in disciplines such as sociology, anthropology, and psychology. Whereas these empirical disciplines see themselves as purely descriptive, EE also aims at normative reflection. Currently there is literature about the quality of empirical research in ethics, but little or no reflection on specific methodological aspects that must be considered when conducting interdisciplinary empirical ethics. Furthermore, poor methodology in an EE study results in misleading ethical analyses, evaluations or recommendations. This not only deprives the study of scientific and social value, but also risks ethical misjudgement.
While empirical and normative-ethical research projects have quality criteria in their own right, we focus on the specific quality criteria for EE research. We develop a tentative list of quality criteria – a “road map” – tailored to interdisciplinary research in EE, to guide assessments of research quality. These quality criteria fall into the categories of primary research question, theoretical framework and methods , relevance , interdisciplinary research practice and research ethics and scientific ethos.
EE research is an important and innovative development in bioethics. However, a lack of standards has led to concerns about and even rejection of EE by various scholars. Our suggested orientation list of criteria, presented in the form of reflective questions, cannot be considered definitive, but serves as a tool to provoke systematic reflection during the planning and composition of an EE research study. These criteria need to be tested in different EE research settings and further refined.
Background a
Empirical ethics.
For roughly two decades there have been debates in bioethics about the question of how to address the challenge of best practice in interdisciplinary methodology. Empirical research in bioethics, principally using the methods of social sciences [ 1 , 2 ] b , has considerably increased during this period (e.g. [ 3 ]).
Generally speaking, this debate comes under the label of what is known as “empirical ethics” (abbreviated “EE”, e.g. [ 4 - 8 ]); some authors prefer to talk about “empirically informed ethics” or sometimes “evidence-based ethics” (e.g. [ 9 - 13 ]). This field calls for more empirical research, mainly from sociology, psychology or anthropology, and/or more consideration of empirical research results in normative bioethics c . Whereas empirical disciplines aim to be purely descriptive, however, EE has a strong normative objective: empirical research in EE is not an end in itself, but a required step towards a normative conclusion or statement with regard to empirical analysis, leading to a combination of empirical research with ethical analysis and argument.
Research problem
The widespread use of EE highlights the importance question above: what is the best practice for applying empirical methodologies in such an interdisciplinary setting? This interdisciplinary challenge is still not solved, and proponents of EE can self-critically assume that the quality of EE studies is often unsatisfactory. This problem can be tackled by two strategies: either focusing only on one particular methodology, or trying to establish standards for ‘good’ EE research. The advantage of the latter is obvious: methodologies are highly dependent on theoretical assumptions, and there is no such thing as one true theory, neither in empirical research nor in ethics. It therefore seems most appropriate to focus on best practice instead of perfecting one particular methodology in EE.
The lack of standards for assessing and safeguarding quality is not only a problem for scientific quality per se , but also an ethical problem: poor methodology in EE may give rise to misleading ethical analyses, evaluations or recommendations c , not only depriving the study of scientific and social value, but also risking ethical misjudgement. Improving the quality of EE is therefore an ethical necessity in itself.
Aims & premises
This article aims to provide a “road map” (see below) to assist researchers in conducting EE research, and also to initiate a more focused debate within bioethics about how to improve the quality of EE research. Our contribution should be understood primarily as a heuristic approach. As the discussion on quality criteria for EE research is rather new and touches on a number of complex topics within interdisciplinary research we would see our article as a first and provisional suggestion in this respect. We will discuss four domains of quality criteria and provide a tentative list of questions to be considered by researchers when engaging in EE research. Each formal quality criterion will therefore be guided by practical questions which illustrate its reflective and methodological purpose.
In this paper we will focus mainly on providing and discussing the abstract criteria, but will refrain from citing detailed examples for each criterion because of length limitations. While different application fields for quality criteria can be imagined (such as journal peer review, assessment of research proposals, or the planning of individual research projects), it should be noted that the criteria we present are only designed for guiding EE research (and, partially and indirectly, for reporting on it, since the reported study is what peer reviewers and readers of scientific literature ultimately see).
We start from the premise – supported by our own research, and corroborated by several authors in the debate e.g. [ 6 , 8 , 14 - 18 ] – that empirical research is vital for the vast majority of normative ethical research. Here we focus on “applied ethics”, that is, research concerning analyses, evaluations and recommendations in ethically sensitive fields such as medicine and clinical research, genetics and neuroscience, and also economics and the media.
As far as empirical research is concerned, we limit our claims here to socio-empirical research, i.e. studies based on methodologies from the social sciences . As to the normative-ethical aspect of EE research, we primarily refer to normative-ethical research based on philosophical methods. While theological methods are also important and valuable, we did not assess them in the context of this work.
Definition of empirical ethics research
As a descriptive definition of EE could not claim to define “empirical ethics” for all instances in which this term is used for this, (see e.g. [ 11 , 15 , 19 ]) we will confine ourselves to a stipulative definition covering the various ways of conducting EE research (e.g. [ 6 , 14 , 17 , 20 , 21 ]).
EE research, as we understand it, is normatively oriented bioethical or medical ethical research that directly integrates empirical research e . Key elements of this kind of study are therefore that it encompasses (i) empirical research as well as (ii) normative argument or analysis, and (iii) attempts to integrate them in such a way that knowledge is produced which would not have been possible without combining them. Concerning (iii), we proceed on the assumption that descriptive and normative statements can and should be analytically distinguished from each other in order to evaluate their validity [ 22 - 24 ]. Some proponents of EE, e.g. those taking a phenomenological or hermeneutical approach (e.g. [ 7 , 25 - 27 ]), would assume that descriptive and normative statements are inevitably inseparable and indistinguishable. However, in the context of the current article, we exclude from our analysis approaches to EE research which are mainly hermeneutically or historically oriented. We believe that they can fruitfully contribute to EE research, but these approaches are in need of specific quality criteria that go beyond the scope of this paper. Nonetheless, the development of quality criteria or best practice standards might also be relevant for these approaches.
The above-mentioned integration of empirical research and a normative-ethical argument makes interdisciplinary work inevitable. It implies collaboration between researchers trained in different fields and methodologies. While it is theoretically possible for interdisciplinary research to be carried out by a single researcher skilled in more than one academic field, most EE research will benefit from interdisciplinary research teams (e.g. [ 8 ]). This is because the skills needed for applying both sound empirical research methods and thorough normative analysis and argument are seldom possessed by a single researcher.
Working in teams also offers the opportunity to overcome methodological biases, penchants for particular research approaches and intellectual myopia in terms of background assumptions. For example, in qualitative research (e.g. interviews, observations), intersubjective exchange during the interpretation process is a necessary precondition for enhancing the validity of the results. It also seems unlikely, on the basis of the criteria we are about to present, that such interdisciplinary (team-) work can be done (fully) independent of other team members, based on a strict division of labour between empirical researchers and ethicists. For all these reasons, in our further analysis we proceed on the assumption that EE research should best be carried out in an interdisciplinary research team.
“Road map” analogy
We propose to use the analogy of a “road map” in order to structure the different criteria in our paper, applying the metaphor of moving through a (not yet familiar) landscape for the conduct of EE research. According to this metaphor, the following criteria can be understood as “landmarks”, indicating what paths to take, how fast to go, and where to expect a rocky road or a dead end.
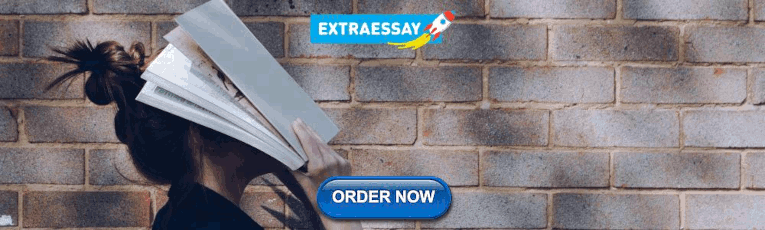
Mapping landmarks of quality and drafting a road map
To survey specific “landmarks” of quality in EE research, and to draft a corresponding “road map”, our procedure consists of the following main steps (Figure 1 Search and analysis strategy , provides a graphical overview of the search and analysis strategy the working groups used during the project):

Search and analysis strategy.
(i) to analyse selected empirical quantitative and qualitative studies as well as theoretical ethics studies about living organ donation (“bottom-up-strategy”) regarding their use of empirical data and ethical concepts, and if they reflected upon that relationship;
(ii) to study, present and critically discuss already established quality criteria for each of the following three branches of relevant criteria, viz. a) empirical/social science research, b) philosophical/normative-ethical research, and c) EE research (“top-down-strategy”);
(iii) to consider, present and critically discuss research ethics criteria for each of the three branches, in the light of our experience in EE research and knowledge of the EE debate;
(iv) to develop a consensus among the authors;
(v) to refine the different branches and reduce complexity for publication; and
(vi) to draft a tentative checklist of questions which operationalises criteria pertinent to EE research.
Our search and analysis strategy included first (i) a bottom-up analysis of 10 publications, dealing explicitly and/or implicitly with ethical and empirical issues of living organ donation. This field was used as a focused case study to allow a comparison of quantitative (n = 3 [ 28 - 30 ]) as well as qualitative (n = 3 [ 31 - 33 ]) empirical studies and theoretical ethics publications (n = 4 [ 34 - 37 ]).
This bottom-up detailed analysis revealed that firstly, the relevance of empirical data for ethical analysis is often not made explicit, secondly, empirical studies tend to be crypto-normative in their conclusions (“crypto-normative” for us implies that implicit evaluations and ethical conclusions are made, but the evaluative step is not explicated), and thirdly, theoretical studies often refer to empirical data, but rarely critically reflect the empirical methodology, or often tend to apply empirical data in a positivistic manner.
For step (ii), we applied a more top-down strategy by summarising existing literature in quality criteria in the three relevant fields: empirical research in social sciences, philosophy/normative ethics research, and EE research. Thus, we composed three subgroups in our Empirical Ethics Working Group (comprising about 13 active members at that time; see endnote (i) for further information about the working group). Each group conducted a review of the methodological literature in the relevant area. We also included explicit recommendations for quality research drafted by scholarly societies (e.g. of psychology or sociology).
Literature was searched with a narrative / selective search strategy (see e.g. [ 38 ]). This strategy was developed due to difficulties and inappropriate results when trying a systematic literature search by using specific search terms, given the interdisciplinary nature of our topic. As a consequence, we decided to broaden our approach by using literature found via PubMed, Philpapers and Google Scholar, via manual search of scientific journals, as well as via expert opinions generated from the members of our working group and their connections to the respective scientific community.
The subgroup of trained philosophers (MM, with non-authors JD and UM; see Acknowledgments ) analysing the criteria within philosophy often had to extract informal and implicitly given criteria (apart from criteria directly related to standards of argument, e.g. logic). The principal subcategories of criteria for philosophical, normative-ethical research are the criteria of good argument (as discussed in informal and formal logic (see e.g. [ 39 , 40 ])), the use of specific philosophical methods (theories, approaches) with their respective quality criteria (e.g. [ 41 ]), and criteria of good ethical judgement and/or decision-making (as discussed in models and methods of decision-making (e.g. [ 42 ]) (see Figure 2 , Specific criteria of quality ).

Specific criteria of quality.
The subgroup on quality criteria for empirical research in social sciences (LGR, GR) had to differentiate the literature search of journals and monographs into general criteria (such as adequacy of the research process, transparency, good scientific and ethical conduct) and specific criteria for quantitative methods (e.g. [ 43 ]), qualitative methods (e.g. [ 44 - 48 ]) or mixed-methods approaches (e.g. [ 49 - 51 ]) (see Figure 2 ).
The subgroup working on quality criteria for EE research (JS, SaS) performed a selective literature review in relevant bioethics journals and books focusing on theoretical and methodological contributions to EE research. While a number of conceptual accounts to EE were identified, the issue of quality standards was only rarely addressed [ 52 , 53 ]. However, parts of the conceptual considerations as identified in the literature could be translated into criteria relevant to EE research [ 54 , 55 ]. In addition, the researchers drew from current EE research addressing ‘end of life issues’ which is performed in an interdisciplinary research group of medical ethicists with a disciplinary background in philosophy, sociology and medicine.
Following these procedures, the findings of each subgroup were discussed within the whole working group. Our next step was to systematise the criteria identified by clustering them into main categories according to their field (empirical, philosophical, EE research), as well as into subcategories (e.g. different categories for qualitative and quantitative empirical research, or different categories for criteria related to logic/argumentation theory and philosophical approaches). The clustering was based on the literature search (inductive strategy), as well as on our own theoretical estimation of aspects relevant for assessing the quality of scientific work (deductive strategy). The summarising process was supported by mind mapping software to track modifications, deletions, additions or re-locations of criteria. The main and sub-categories in this mind map were generated either inductively on the basis of the literature or deductively by own reasoning against the backdrop of scientific experience and theoretical knowledge.
Finally, we derived three overarching standards of scientific research, which were subdivided into formal, cognitive and ethical norms (see below Three peaks that dominate the scenery ) based especially on the philosophy of science. The actual quality criteria were then seen as specific expressions of these overarching standards.
In step (ii), issues concerning research ethics already found in the reviewed literature were added to the mind map as further criteria. Additional literature was also reviewed [ 56 - 59 ].
A consensus round was initiated for step (iii) where each criterion was again critically discussed with a view to identify possible redundancies. Consensus was reached with the results of the argumentation in the discussion, which was most often accompanied by a final, explicit request if there were any dissenting votes regarding the result. Active members of the working group who were not able to participate at the consensus round (about 3 out of 13 members) had the opportunity to show assent or dissent on the basis of the sent draft of the mind map; there was no crucial dissent that led to a substantial revision of the mind map.
The next step (iv) consisted of focusing on those criteria that were seen as only specific to and coherent with EE research, excluding those relating to broadly empirical research in social sciences and philosophy. With this aim in mind, the working group agreed to divide specific EE criteria into four domains (see also Figure 1 ), effectively reducing the amount of criteria in the mind map of about 200 (all branches, research ethics included) to about 50. In these four domains, the formal and cognitive norms relevant to all kinds of scientific research were specified and adjusted to the particular field of EE research (“ primary research question, theoretical framework & methods” and “ relevance” ). Furthermore, the specific interdisciplinary nature of EE research was addressed ( “interdisciplinary research practice” ), and issues of research ethics which are pertinent to EE research ( “research ethics & scientific ethos” ) were considered.
As a result, four new subgroups were established that had to summarise, systematise and elucidate the according criteria on the basis of the already found literature of the three aforementioned subgroups, as well as propose additional criteria if found necessary. The members of these new subgroups also became the authors of the paper at hand and were assigned to the four domains as following: a) primary research question, theoretical framework & methods (JI, SiS, SW); b) relevance (MM); c) interdisciplinary research practice (JS, SaS); and d) research ethics & scientific ethos (LGR, GR).
This work also led to the last step (v), the drafting of a refined list which allows the “road map” below to be used as a checklist to guide EE research. For clarity, the criteria included in this list are presented in tabular form. These tables (see below) contain each criterion, operationalised into questions. We decided that it was heuristically more effective to ask questions rather than to consider statements, and to conceive these questions as a pragmatic aid to guide scholars and help them reflect on their own research. Nevertheless, the questions that operationalise criteria should not be understood as simple “yes/no” queries – instead they should function as reflective and critical questions designed to assess certain quality-related aspects of EE research. Each subgroup proposed their phrasing of the questions to the whole author group to achieve consensus on the final phrasing.
This checklist idea is not new. It is already well established in other research fields, e.g. in medicine for guideline recommendations (GRADE [ 60 ]; SIGN [ 61 ]), quantitative randomised medical trials (CONSORT [ 62 ]) and observational epidemiological trials (STROBE [ 63 ]), where they are used to check evidence and/or the quality of (the reporting of) trials. Although normative or especially ethical aspects are rarely explicitly mentioned in these checklists, they include implicit normative items such as asking for ethical approval, informed consent, funding or possible sources of bias. Critical appraisal is more and more coming up on the agenda of evidence based medicine ([ 64 ] see also [ 65 ]).
In analogy, we thought it necessary to render explicit ethical questions that are implicit in EE research. Looking for the best fitting form of presentation, our working group came to the consensus that we would try to adapt the checklist format, as we thought it will be most helpful in order to display the suggested criteria in a clear, feasible way.
The road map
An aerial view: spotting hills and valleys.
As is perhaps obvious, the first criteria that have to be considered are philosophical quality criteria, and social sciences quality criteria. This idea is already mentioned in the EE literature (e.g. [ 54 , 66 ]).
However, even if (ideally) one had knowledge of both sets of criteria, and had the relevant skills to apply them, distinctive features of the quality of EE research would still be missed. This is due to the interdisciplinarity of EE research, and specifically to the complexity that the necessary methodological combination of the two sets of criteria requires. This therefore goes far beyond the need for cooperation between disciplines. Additional criteria reflect, in particular, the combination of normative-ethical and empirical research.
In the following, the “basic” philosophical and social science quality criteria will not again be summarised, as they have already been discussed in various contexts. We presuppose that quality work in EE research includes consideration of these already established criteria. Instead our major focus will be on criteria that are either (i) specific to EE research (i.e., not directly relevant to other ethical or social sciences research), or (ii) also useful in other research settings, but especially important for EE research.
Three peaks that dominate the scenery: “good science” criteria
There are three kinds of standards that dominate all research (including normative-ethical research, empirical research, and combinations of the two), as they are interconnected by norms which are generally concerned with “good” scholarship. They are based on a common-sense definition of what good science is: formal norms (scientific writing), cognitive norms (general methodology) and ethical norms (research ethics). All quality criteria ultimately derive their normative power from the same general norms and can thus be understood (more or less) as specific expressions of these norms.
Looking for an interdisciplinary highway
a) Setting up the road signs: designing a primary research question and selecting a theoretical framework and corresponding methods
EE research often faces the problem that the ethical and empirical aspects motivating our research are intertwined [ 66 ]. Special attention must therefore be paid to the design and development of the research question. The empirical and normative-ethical aspects of the research question have to be separated clearly without being split into two separate research approaches [ 22 ]; at the same time, the relationship between them has to be elucidated [ 23 ]. This reflective intermediate step is relevant because it shows why they are part of the same research, and cannot be dealt with sufficiently by two (or more) separate research projects. The inherent link between the parts should be considered on several levels: the theoretical assumptions [ 67 ], the relevance of empirical knowledge and data to the ethical question and vice versa [ 15 ], the chosen methodology [ 66 , 68 ], the type of result/data envisaged, and the way the result can inform further EE research, both empirical and ethical [ 69 ] (see Table 1 ).
Criteria related to primary research question and selecting a theoretical framework and corresponding methods
(even if the ethical motivation is more prominent than the ethical research question) | • Can an explicit distinction be made between the empirical and ethical research questions? (e.g. a distinction between interviewing patients about their wishes and the ethical weight given to patient autonomy) [ , ] |
• How dependent is the empirical research question on particular ethical background assumptions? (e.g. justification for the selection of a target group for a questionnaire: why do we think their opinion is ethically relevant?) [ ] | |
• How is the ethical research question dependent on empirical or socio-theoretical background assumptions? (e.g. ethical considerations of vulnerability of a particular group such as pregnant women: what are the underlying anthropological or psychological considerations? Are there any hidden gender-related stereotypes?) [ ] | |
• What are the explicit and implicit research interests and motivations of the EE researchers? (e.g. is research with dying patients motivated by curiosity or the moral attempt to empower them? Is the researcher motivated to identify possible conflicts of interest or might the research serve mainly to produce more social acceptance of a technology?) [ , ] | |
• What kind of epistemic research interest motivates the researcher to combine ethical and empirical research? (e.g. explaining whether the aim is the evaluation of established ethical practice, or of measures taken to improve ethical practice; or whether the aim is ethical theory-building, norm-construction, or legitimization/critique or a particular practice) [ ] | |
• How can a theoretical framework be developed; what are the main limitations of the chosen theoretical framework? (e.g. premises and limitations of a principle of autonomy, when analysing macro-social interactions) [ ], see contributions in [ ] | |
• Were potential ambiguities of central concepts considered within the theoretical framework? (e.g. to which extent is the concept of ‘identity’ used differently in current philosophy and in sociology when wanting to analyse the discourse of identity changes by neuroenhancement empirically and its ethical implications) [ , ] | |
• How does the chosen medico-theoretical framework (e.g. concept of disease/health) fit into the ethical-normative framework? (e.g. does a science-positivistic concept of disease fit into a Kantian or hermeneutic approach of ethics?) [ ] | |
• How does the chosen sociological-, cultural- or philosophical framework (e.g. concept of personal identity) fit into an ethical normative framework (e.g. approach to a cosmopolitical ethics of justice?) [ ] | |
• Are the chosen empirical methods compatible with the combined theoretical framework? (e.g. are interviews with doctors as experts compatible with a liberal, autonomy-driven approach that claims to empower patients?) | |
• What is the advantage of the chosen method in comparison to other available methods? (e.g. why and when to choose a deductive approach in applied ethics to assess ethical problems of a new technology and not an inductive, or hermeneutic one?) [ , ] | |
• Are the chosen methodological approaches appropriate for the envisaged combined research question? (e.g. does the empirical method of interviewing parents generate results relevant for the ethical question of whether parents should be allowed to influence the genetic make-up of their children?) |
In theory-guided research, the research questions as well as the chosen methodology normally depend on a particular theoretical framework. The underlying assumptions and theoretical background of both the ethical and the empirical parts of the research should be made explicit and transparent [ 22 , 67 ] (see Table 1 ). This includes theoretical work on different levels. Firstly, when combining empirical and ethical approaches, we need to reflect on the compatibility of the theories used in each part. The combination of two theoretical approaches needs to be consistent. For example, the empirical discourse analysis of communication structures cannot simply be transformed into ethical questions of individual responsibility for decisions. A more appropriate theoretical ethical approach would be one that ascribes a high level of normative relevance to communication and social interrelations. An analysis of the core concepts of the theoretical approaches makes it possible to test whether these approaches are compatible [ 73 ]. These core concepts include ideas such as human agency, the relationship between social and individual levels of agency, concepts of causality, the relevance and concept of gender, to name just a few examples [ 71 , 74 ].
Theoretical compatibility is also a point to consider when it comes to the question of how empirical and ethical research inform each other. Connected to this, the selection of both the empirical methods and the theoretical line of ethical argument (in short, the theoretical method) is crucial. Both methods need to be compatible with the combined theoretical framework of the research, but should also reflect how the results of each part can inform the other part [ 66 , 68 ]. For example – and this is far from being an exhaustive list –, discursive ethical approaches have strong links with argumentative, discursive methods of surveying opinion, while liberal-utilitarian ethical approaches tend to accept opinion polls and quantitative surveys. Critical reflection on the chosen methods and on their limitations for the combined argumentation is a must for all EE.
b) “Driving only when necessary”: demonstrating relevance
Even if an EE study is sufficiently transparent with regard to its primary research question and methods, it can still be unnecessary , or more specifically, not valuable [ 22 ]. From an epistemic, ethical and even economic point of view (due to limited research resources), one can claim that an interdisciplinary approach should only be favoured if it is likely to lead to new insights, or to broader or more nuanced insights than those gained from intradisciplinary research. One should also bear in mind that it is ethically problematic to expose research participants (e.g. patients as interviewees) to stress if the research has low relevance (see also section d).
Relevance should be understood on two levels, as epistemic relevance on the one hand and societal relevance on the other. Scholars conducting EE research must be able to demonstrate the value of their planned project in at least one sense, and ideally in both (see Table 2 ).
Criteria related to relevance
• Will the study possibly produce knowledge that could not be generated by relying on traditional disciplinary methodologies? (e.g. overcoming too separated empirical research and separate philosophical discussion) [ , ] | |
• Does the study aim to increase our knowledge, and if so, with regard to what? (e.g. does the study contribute to a balance between theoretically generated norms and empirically found norms? E.g. Does it revise/improve the impact of ethical guidelines?) [ - , ] | |
• Does the study aim to give input on an ongoing controversy, or does it provide a new perspective on it? (e.g. clarifying if relatives are able to give substitute judgment for incapacitated patients or not, or e.g. if post-trial access should be compelling on the basis of new evidence of consequences when post-trial access is not given etc.) [ ], partly [ ] | |
• Does the study aim to offer substantial arguments for or criticism of established ethical positions? (e.g. is a contribution to theory modification or to a refinement of the application of a theory expected? Are descriptive presuppositions of an ethical position, such as anthropological, sociological or psychological assumptions, criticised?) [ , , ] | |
• Does the study aim to contribute to the development or refinement of scientific methods, especially methods of EE research, and if so, how? (e.g. pilot testing of a jointly developed research instrument, identifying the need of developing new or refined forms of interactions between researchers) [ , ] | |
• Does the study aim to offer potential for innovation, and if so, what kind of innovation? (e.g. is it a contribution to theory-building expected? Will the study generate new instruments for ethical decision-making?) [ , ] | |
• Does the study aim to contribute to another scientific and/or ethical discourse? (e.g. does it contribute to social sciences discourses?) [partly [ ] | |
• Does the study clearly states to whom it is addressed, and who will benefit from its results? (e.g. are the addresses and/or beneficiaries physicians, nurses, social scientists, ethicists or especially empirical ethicists? Are policy-makers or persons in a management position addressed? [ ] | |
• Does the study aim to improve ethical decision-making? (e.g. will it produce evidence that was absent, or will it give guidance regarding the specification of accepted norms or regarding the interpretation of institutional or legal rules?) [ , , ], partly [ ] | |
• Does the study aim to raise awareness (among actors, institutions or society) of particular ethical problems? (e.g. does the study identify new ethical problems, or does it highlight specific aspects of a known ethical problem that was not yet addressed sufficiently in practice?) [ , ] | |
• Does the study aim to lead to a shift in structures and/or decision-making processes (in relevant institutions)? (e.g. establishing new guidelines or building new forms of committees for ethical review) [ ], partly [ ] | |
• Does the study aim to establish minimum ethical standards (in relevant institutions or professions)? (e.g. creating new informed consent procedures for specific patient groups?) | |
• Does the study aim to contribute to or stimulate public debate? (e.g. about physician-assisted suicide, rationing in health care, public health funds etc.) | |
• Does the study aim to contribute to or stimulate a process of legislation? (e.g. proposing alteration of legal norms) | |
• Does the study aim to articulate the need for reforms (in a certain institution or system of society)? (e.g. by evaluating current practices.) [ ] | |
• Does the study aim to articulate new ethically pertinent ecological or economic problems? (e.g. costs related to a broad implementation of the use of social robots in elderly care) [ ] |
The main issue regarding epistemic relevance (e.g. [ 56 - 58 , 79 ]) is whether the study makes a contribution to academic ethics, for example by adding new knowledge (e.g. developing a sound ethical argumentation for the topic in question), contributing to an ongoing controversy (e.g. providing a new perspective), or criticizing established positions on a theoretical or applied level. In other words, it needs to be clear what knowledge gains the research will provide in terms of the development, modification or application of theory.
Another way to ensure scientific relevance, especially in EE research, is to develop or refine scientific methods. Potential for innovation (e.g. constructing a new theory or new instruments for ethical decision-making) should also be regarded as part of epistemic relevance. If there is a contribution to other disciplines (e.g. to social science debates on agency or social dependency), this also fulfils the criterion of epistemic relevance. Finally, the target group of a study, e.g. the future beneficiaries of the results and conclusions of this particular EE research, should be clearly stated. This gives guidance for research planning, and for the analysis of the results.
As far as societal or practical relevance (e.g. [ 58 , 59 , 79 ]) is concerned, EE research should be able to show what it contributes to the improvement of ethical praxis. As much ethics research is funded by public money, it might even be argued that there is a moral obligation to generate not only scientifically valuable knowledge, but also knowledge that benefits society or certain groups within it, e.g. specific vulnerable group. This assumption relies on a normative understanding of ethics as a discipline aimed at providing orientation knowledge, which is needed to detect problems or to indirectly or directly improve praxis. So scholars should try to demonstrate the societal/practical relevance of their EE research whenever feasible.
Examples of this kind of relevance could be improvements to ethical decision-making (e.g. an empirically tested model of ethical decision-making), raising awareness of ethical problems and challenges (e.g. showing that without regulated antimicrobial stewardship, there is a high risk of antibiotics increasingly losing their effectiveness), a shift in structures and decision-making processes (e.g. not asking relatives what they want, but what the patient would have wanted), or the establishment of minimum ethical standards in the institutions or professions related to the praxis under consideration (e.g. formulation and implementation of guidelines). Furthermore, societal relevance is present when the study initiates or simply provides a stimulus for public debate, or leads to/assists the process of legislation. Articulating a need for reforms or new ethically pertinent ecological or economic problems – or articulating existing problems in a new, enlightening way – is also of societal relevance (e.g. [ 80 ]).
We are not arguing that all EE research has to demonstrate societal or practical relevance. EE research can be relevant even if it is only relevant for scientific/scholarly reasons. Nonetheless, as stated above, since ethical research seems (to us) to be ultimately guided by a practical interest in ameliorating (some) social practices, scholars need to think about potential societal relevance when planning or evaluating their own EE research (compare also [ 24 ]).
c) “Enabling car sharing”: guaranteeing interdisciplinary research practice
Quality criteria for interdisciplinary cooperation in EE research encompass different stages of the research process, including drafting, data gathering, data analysis and conclusions [ 36 , 37 ]. For the different stages, the following points are of particular importance (see Table 3 ).
Criteria related to interdisciplinary research practice
• What form of interdisciplinary collaboration serves the needs of an EE study? (e.g. how strong and how often should collaborators interact? Is it necessary to have face-to-face-meetings? Who has to be involved in which step of the research? Is there reflection on the potentials and the limitations of the kind of collaboration?) [ ] | |
• How can the participating researchers be adequately selected? (e.g. Which disciplines/methods are actually needed?) | |
• How can an appropriate task schedule be developed? (e.g. at which point in time is empirical data to be gathered) | |
• Which agreements must be reached with regard to interdisciplinary communication? (e.g. consideration of terms used, explanation of professional jargon and development of a “common language”) [ ] | |
• How can competencies and responsibilities be reasonably distributed within the research team? (e.g. despite their varying competencies, will all the interdisciplinary researchers remain actively involved in the research process? Who is accountable for what?) | |
• How can research questions be developed jointly? (e.g. regarding different interests and disciplinary perspectives, or regarding the goal of the study) | |
• How can the literature search be carried out? (e.g. having to acknowledge empirical-ethical studies from one’s own thematic field as well as to acknowledge both empirical and ethical work from different disciplines in diverse types of publication) | |
• How is the joint development or modification of a research instrument carried out? (e.g. Is there a process that allows for dissent and argument in developing or modifying a research instrument?) | |
• Is there normative-ethical reflection on the empirical research process? (e.g. can implicit normativity be revealed that is related to a theoretical background (“social constructivism”)?) | |
• Is there a mutually critical appraisal by normative and empirical sciences with regard to data gathering? (e.g. what constitutes “good” data for the EE study) [ ] | |
• How do normative and empirical aspects interrelate with regard to analysis and deliberation? | |
◦ Is the analysis of the empirical data influenced by normative theories, concepts, or standpoints? (e.g. by a specific account of patient autonomy) | |
• Is the normative deliberation influenced by the requirements of the empirical data analysis? (e.g. by standardization of data) [ ] | |
• How do normative and empirical aspects interrelate with regard to the study’s conclusions? | |
◦ Are the ethical conclusions actually linked with normative premises? (e.g. avoiding an is/ought fallacy) [ , ] | |
◦ Are the empirical conclusions supported by the data, or is there a bias in the empirical results based on the normative conclusions? (e.g. avoiding a normativist fallacy or “wishful thinking”, deducing broad conclusions from fine-grained data, under- or overrating of empirical data, ignoring of empirical evidence that would criticize normative conclusions etc.) [ , ] | |
• Is there a critical evaluation of the results? (e.g. addressing methodological critique with regard to interdisciplinary cooperation, or indication of limitations) |
In the planning stage of an EE study, the reflection on adequate forms of interdisciplinary collaboration should include consideration of not only its potential benefits, but also its limitations. Limitations are often the result of different professional jargons and terms [ 3 ]. It is therefore advisable to clarify terminology at the beginning of the collaboration. Furthermore, a clear distribution of competences and responsibilities within the research team is needed. A further challenge in the planning of an EE study is posed by the literature research, which has to consider journals, books, and databases from diverse fields [ 84 ]. During data gathering , it is important to reflect the potential bias produced by normative or descriptive assumptions [ 54 ] . One should also be aware of possible biases when selecting published empirical studies (e.g. [ 85 ]).
During data analysis , researchers should explicitly discuss the relevance of ethical theories, concepts, or standpoints for the empirical data analysis, as well as possible reductionist tendencies associated with the requirements of particular methods, e.g. the standardization of question wording and statistical analysis in quantitative surveys [ 81 ]. A final crucial question is how much the empirical results contribute to the normative judgement [ 16 , 24 ]. Do they help to “justify” a particular norm, and if so, based on which ethical theoretical consideration? Or do they have an impact on the level of practical application, when public acceptance seems crucial if a rule is to be applied in a particular way? It is also desirable to consider whether the interpretation of the empirical data (e.g. approval or criticism of a particular group’s view) was led by a particular ethical view [ 82 ].
d) “Driving responsibly”: observing research ethics & scientific ethos
Of course EE research is liable to general principles of research ethics in general and bioethics in particular. Important topics are avoiding harm to the participants [ 86 , 87 ], confidentiality and data safety [ 86 , 88 , 89 ], informed consent [ 86 , 90 , 91 ], management of research data [ 89 , 92 ].
Our discussions within our broader research group revealed, that in addition to that some aspects are of special importance in the field of EE research:
In an interdisciplinary setting where researchers can have different institutional backgrounds and differ in the personal motivations for their research, potentially competing interests should be disclosed and critically discussed. There should be explicit reflection about the interdisciplinary setting and the division of labour (see e.g. [ 93 ]). This, we think, must include a readiness to critically reflect upon a possible inclination to design the empirical part of the research in such a way that the results may favour the researcher’s own ethical position. For example, one should ask: am I especially critical or joyful when it comes to this issue? Do I tend to exaggerate the issue in my research questions? Which results of the empirical research would I predict and which would I wish to see? Here the interdisciplinary context offers an excellent opportunity for an open discourse (see Table 4 ).
Criteria related to research ethics & scientific ethos
• Which personal (e.g. cultural, philosophical, theological) presumptions concerning ethics may bias the EE research process significantly and how can they be adequately managed? (e.g. inclination to a emotivist meta-ethics, a neopositivist philosophy of science, a postmodernist account of society etc.) [ ] | |
• Do different standards exist in the various disciplines involved, and if so, have they been critically and respectfully discussed among the EE research team to find the most appropriate ethical standard? (e.g. is waiving of consent allowed, is assent sufficient, how to establish informed consent in emotional difficult situations at the end of life etc.) [ - ] | |
• Is the EE research team aware of a possible confidence bonus, and have strategies been developed to deal with this phenomenon carefully? (e.g. making transparent which goals and which limitations the own study will have and informing research participants and partners accordingly) | |
• Is the EE research team aware of the (implicit) ethical impact of the way results are presented? (e.g. was the potential for stigmatization or discrimination considered when choosing the wording and emphasis of particular results?) [ ], partly [ ] | |
• Has the EE research team made sure that the way the results are presented reduces the potential for misinterpretation by third parties such as politicians and special interest groups? (e.g. by changing perspectives when re-reading results and revising the wording etc.) | |
• Has the risk–benefit ratio for the EE research project been discussed in terms of its possible consequences for the people/society of the (near/more distant) future? (e.g. does lay considerable burden on study participants for a relatively low practical or epistemic output?) [ ] | |
• Has the research team overlooked any negative consequences that could be detected in advance and therefore avoided? (e.g. acknowledging non-scientific partners when publishing, the handling of emotional distress of participants in interview studies with sensible questions, supervision of researchers involved in asking sensible questions etc.) |
With regard to so-called informed consent , different standards may obtain in different disciplines such as medicine [ 94 , 95 ], psychology [ 96 , 97 ] or social sciences [ 98 - 100 ]. However, we think that it is the task of the interdisciplinary research team to openly and ethically consider the possibilities and to choose the most appropriate format – which may exceed the legal standard. Regardless of consent by the participants, the definitive ethical responsibility remains with the researcher – especially as the EE researcher may be faced with a confidence bonus granted by the research participants just because she/he is an ethicist (an “ethical misconception” analogous to the “therapeutic misconception” in some clinical research). It is the researcher’s duty to deal with this bonus very carefully.
EE research can be misinterpreted by politicians and special interest groups. Therefore, we suggest that researchers should reflect on the following questions: have we ensured that the results cannot easily be misunderstood or misused? Might the EE study have unanticipated negative consequences that could be detected in advance and therefore avoided? Although it seems clear that we cannot anticipate every kind of negative consequences, the EE researcher may have a particular responsibility to carefully reflect on the outcomes of her/his own research beyond the short time frame of the study, since EE might have a strong influence on public policies, e.g. in health care or biopolitics.
Limitations and conclusions
Limitations.
We assume that it is theoretically acceptable to start from the analytical premise that there is genuine ethical research on one side and genuine empirical–descriptive research on the other, and that these have to be paired up with each other through interdisciplinary research practices. We reject, on the other hand, a stance that denies the need for, the possibility of, or the value of strong interdisciplinary collaboration between empirical and ethical research (e.g. being afraid of naturalistic fallacies) to gain a valid applied ethical conclusion f .
Our research is strongly influenced by experience and in-depth analysis of current EE research in the context of Western bioethics and medical ethics. It does not encompass other possible epistemic approaches such as revealing “concealed” normativity in empirical research, or more institutional aspects of a combination of ethical and empirical disciplines. Our “road map” is therefore restricted to this field. Whether this “road map” can be of use in other ethical disciplines, such as economic/business ethics or ecological ethics, has to be explored elsewhere.
Because our literature search strategy was selective, there are of course limitations concerning the completeness of reviewed literature. There is a good case to believe that further criteria could be mentioned when including additional literature. But discussing quality criteria for EE research in a single short publication necessitates condensation and simplification; we therefore understand this paper as an attempt to encourage more intensive meta-ethical and methodological discussion within the EE field.
The proposed criteria in this paper need to be tested in EE research practice. It is likely that the deliberate use of these criteria to guide and report on individual research will lead to the refinement, addition or removal of some criteria.
Conclusions
EE is a highly interdisciplinary and dynamic research field with specific methodological aspects. Because of its genuinely interdisciplinary nature, a reflection on methodological quality is necessarily more complex than in traditional intradisciplinary ethical or empirical research. However, contributions addressing the specific challenges of EE research are so far rare. We have therefore tried, in this article, to give an overview of basic criteria which have to be considered to arrive at EE studies of good scientific quality; they may also inform the assessment of research protocols or proposals. We assume that many of the suggested criteria are self-evident, perhaps even common-sense – but the important thing here is the argument that they should be used together as a whole .
However, our analysis also points out conflicts that may occur between different quality criteria, especially those concerning the empirical part of the research on the one hand and the ethical part on the other. While researchers may not always be able to overcome such conflicts, it is paramount to at least address these different requirements when publishing EE research and to defend the chosen approach in the light of this conflict.
We have argued that empirical research in EE is not an end in itself, but vital for reaching applied normative conclusions. As such EE research is usually interdisciplinary, engaging in sound EE research requires more than merely maintaining the quality of normative argument and empirical method.
Thus criteria for determining the quality of genuinely interdisciplinary aspects of EE research, methodological as well as ethical, are required. We have proposed several criteria of this kind under the headings Primary Research Question, Theoretical Framework and Methods , Relevance , Interdisciplinary Research Practice and Research Ethics and Scientific Ethos . Although these criteria cannot (yet) be considered definitive, they provide a starting point for reflection on the topic. The criteria need to be tested in real EE research practice and evaluation, and are likely to require further refinement.
a The following paper has been jointly produced by core members of the Empirical Ethics Working Group (coordinator until the end of 2013: Prof. Dr Silke Schicktanz; current coordinators: Jan Schildmann and Marcel Mertz) of the German Academy for Ethics in Medicine (AEM; see http://www.aem-online.de ). The working group was founded in 2007, with the objective of bringing together researchers interested in the challenges of empirical research in bioethics, and particularly its relevance to and connection with normative-ethical research. The working group became highly interdisciplinary, consisting of philosophers, theologians, medical ethicists, social scientists, physicians, and humanities scholars.
b Researchers in the field of EE use a broad variety of empirical research approaches, which overlap with the methods used in empirical disciplines such as the social sciences, psychology, ethnography and anthropology (see e.g. [ 16 , 101 ]).
c Though sometimes results from empirical research in bioethics, without any normative conclusions, are also categorised under this label (see e.g. [ 55 , 102 , 103 ]).
d This is especially important for attempts to ethically improve clinical practice guidelines, ethical guidelines or clinical support services by relying on empirical data, as the situation concerning quality criteria is similarly unsatisfactory (e.g. [ 77 , 104 ]).
e Studies that explicitly rely on (vast) empirical research (results) for their ethical argumentation, but do not engage in empirical research themselves, should also be considered when discussing issues of quality in EE research. We decided, though, to focus here solely on EE studies that incorporate genuine empirical research.
f Though we do not think that EE research is generally burdened with the problem of avoiding the naturalistic fallacy, or that it usually illegitimately crosses the is/ought gap (see [ 23 ]).
Abbreviations
EE: Empirical ethics.
Competing interests
Financial competing interest: None to declare. Non-financial competing interest: All the authors of this paper are committed to EE research and will therefore not generally assess such efforts in a negative way. Moreover, they have all engaged in EE research and have an interest in pursuing such research further.
Authors’ contributions
MM: drafted and revised the manuscript in general, drafted section “Driving only when necessary”, coordinated the writing process, and finalised the manuscript. JI: drafted section “Setting up the road signs”, and revised the manuscript. GR: drafted section “Driving responsibly”, made substantial contributions to limitations section, and revised the manuscript. LGR: drafted section “Driving responsibly”, made substantial contributions to limitations section, and revised the manuscript. SaS: drafted section “Enabling car sharing”, made substantial contributions to conclusions section, and revised the manuscript. JS: drafted section “Enabling car sharing”, made substantial contributions to conclusions section, and revised the manuscript. SW: drafted section “Setting up the road signs”, and revised the manuscript. SiS: drafted section “Setting up the road signs”, revised and finalised the manuscript; initiated and coordinated the Empirical Ethics Working Group (until the end of 2013). All the authors were involved in the conception and design of the material presented, and read and approved the final manuscript.
Pre-publication history
The pre-publication history for this paper can be accessed here:
http://www.biomedcentral.com/1472-6939/15/17/prepub
Acknowledgements
We would like to express our thanks to other members of our working group who enriched our discussions substantially: Monika Bobbert, Ruth Denkhaus, Julia Dietrich (JD), Jochen Fehling, Uta Mueller (UM) and Daniel Strech. We also thank both the reviewers for their insightful remarks.
- Haimes E. What can the social sciences contribute to the study of ethics? Theoretical, empirical and substantive considerations. Bioethics. 2002; 16 (2):89–113. doi: 10.1111/1467-8519.00273. [ PubMed ] [ CrossRef ] [ Google Scholar ]
- Hedgecoe AM. Critical bioethics: beyond the social science critique of applied ethics. Bioethics. 2004; 18 (2):120–143. doi: 10.1111/j.1467-8519.2004.00385.x. [ PubMed ] [ CrossRef ] [ Google Scholar ]
- Borry P, Schotsmans P, Dierickx K. The birth of the empirical turn in bioethics. Bioethics. 2005; 19 (1):49–71. doi: 10.1111/j.1467-8519.2005.00424.x. [ PubMed ] [ CrossRef ] [ Google Scholar ]
- Salloch S, Schildmann J, Vollmann J. In: Empirische Medizinethik. Konzepte, Methoden und Ergebnisse. Vollmann J, Schildmann J, editor. Münster: LIT Verlag; 2011. Empirische Medizinethik: Eine Übersicht zu Begriff und Konzepten; pp. 11–24. [ Google Scholar ]
- Hope T. Empirical medical ethics. J Med Ethics. 1999; 25 :219–220. doi: 10.1136/jme.25.3.219. [ PMC free article ] [ PubMed ] [ CrossRef ] [ Google Scholar ]
- Molewijk B, Stiggelbout AM, Otten W, Dupuis HM, Kievit J. Empirical data and moral theory. A plea for integrated empirical ethics. Med Health Care Philos. 2004; 7 :55–69. [ PubMed ] [ Google Scholar ]
- Widdershoven G, Van der Scheer L. In: Empirical ethics in psychiatry. Widdershoven G, McMillan J, Hope T, Van der Scheer L, editor. New York: Oxford University Press; 2008. Theory and methodology of empirical ethics: a pragmatic hermeneutic perspective; pp. 23–36. [ Google Scholar ]
- McMillan J, Hope T. In: Empirical ethics in psychiatry. Widdershoven G, McMillan J, Hope T, Van der Scheer L, editor. New York: Oxford University Press; 2008. The possibility of empirical psychiatric ethics; pp. 9–22. [ Google Scholar ]
- Tyson JE, Stoll BJ. Evidence-based ethics and the care and outcome of extremely premature infants. Clin Perinatol. 2003; 30 :363–387. doi: 10.1016/S0095-5108(03)00028-9. [ PubMed ] [ CrossRef ] [ Google Scholar ]
- Strech D. Evidence-based ethics – What it should be and what it shouldn’t. BMC Med Ethics. 2008; 9 :16. doi: 10.1186/1472-6939-9-16. [ PMC free article ] [ PubMed ] [ CrossRef ] [ Google Scholar ]
- Mertz M. Zur Möglichkeit einer evidenzbasierten Klinischen Ethik. Philosophische Untersuchungen zur Verwendung von Empirie und Evidenz in der (Medizin-)Ethik. GRIN Verlag: München/Ravensburg; 2009. [ Google Scholar ]
- Kalichman M. Evidence-Based Research Ethics. Am J Bioeth. 2009; 9 (6–7):85–87. [ PMC free article ] [ PubMed ] [ Google Scholar ]
- Salloch S. Evidenzbasierte Ethik“? – Über hypothetische und kategorische Handlungsnormen in der Medizin. Ethik Med. 2012; 24 :5–17. doi: 10.1007/s00481-011-0153-9. [ CrossRef ] [ Google Scholar ]
- Borry P, Schotsmans P, Dierickx K. What is the role of empirical research in bioethical reflection and decision-making? An ethical analysis. Med Health Care Philos. 2004; 7 :41–53. [ PubMed ] [ Google Scholar ]
- Musschenga AW. Empirical ethics, context-sensitivity, and contextualism. J Med Philos. 2005; 30 (5):467–490. doi: 10.1080/03605310500253030. [ PubMed ] [ CrossRef ] [ Google Scholar ]
- Sulmasy DP, Sugarman J. In: Methods in Medical Ethics. 2. Sugarman J, Sulmasy DP, editor. Washington D.C: Georgetown University Press; 2010. The Many Methods of Medical Ethics (Or, Thirteen Ways of Looking at a Blackbird) pp. 3–20. [ Google Scholar ]
- Kon AA. The Role of Empirical Research in Bioethics. Am J Bioeth. 2009; 9 (6–7):59–65. [ PMC free article ] [ PubMed ] [ Google Scholar ]
- Birnbacher D. Ethics and social science: which kind of cooperation? Ethical Theory Moral Pract. 1999; 2 :319–336. doi: 10.1023/A:1009903815157. [ PubMed ] [ CrossRef ] [ Google Scholar ]
- Schicktanz S, Schildmann J. Medizinethik und Empirie – Standortbestimmungen eines spannungsreichen Verhältnisses. Ethik Med. 2009; 21 (3):183–186. doi: 10.1007/s00481-009-0026-7. [ CrossRef ] [ Google Scholar ]
- Ives J, Draper H. Appropriate methodologies for empirical bioethics: It’s all relative. Bioethics. 2009; 23 (4):249–258. doi: 10.1111/j.1467-8519.2009.01715.x. [ PubMed ] [ CrossRef ] [ Google Scholar ]
- Reiter-Theil S. What does empirical research contribute to medical ethics? A methodological discussion using exemplar studies. Camb Q Healthc Ethics. 2012; 21 (4):425–435. doi: 10.1017/S0963180112000205. [ PubMed ] [ CrossRef ] [ Google Scholar ]
- Düwell M. Wofür braucht die Medizinethik empirische Methoden? Eine normativ-ethische Untersuchung. Ethik Med. 2009; 21 :201–211. doi: 10.1007/s00481-009-0019-6. [ CrossRef ] [ Google Scholar ]
- De Vries R, Gordijn B. Empirical ethics and its alleged meta-ethical fallacies. Bioethics. 2009; 23 (4):193–201. doi: 10.1111/j.1467-8519.2009.01710.x. [ PubMed ] [ CrossRef ] [ Google Scholar ]
- Dunn M, Sheehan M, Hope T, Parker M. Towards Methodological Innovation in Empirical Ethics Research. Camb Q Healthc Ethics. 2012; 21 :466–480. doi: 10.1017/S0963180112000242. [ PubMed ] [ CrossRef ] [ Google Scholar ]
- Lindseth A, Norberg A. A Phenomenological Hermeneutical Method for Researching Lived Experience. Scand J Caring Sci. 2004; 18 :145–153. doi: 10.1111/j.1471-6712.2004.00258.x. [ PubMed ] [ CrossRef ] [ Google Scholar ]
- Ebbesen M, Pedersen B. Using empirical research to formulate normative ethical principles in biomedicine. Med Health Care Philos. 2007; 10 :33–48. doi: 10.1007/s11019-006-9011-9. [ PubMed ] [ CrossRef ] [ Google Scholar ]
- Rehmann-Sutter C, Porz R, Scully JL. How to relate the empirical to the normative. Toward a phenomenologically informed hermeneutic approach to bioethics. Camb Q Healthc Ethics. 2012; 21 :436–447. doi: 10.1017/S0963180112000217. [ PubMed ] [ CrossRef ] [ Google Scholar ]
- Strong RW. Living-donor liver transplantation: an overview. J Hepato-Biliary-Pancreat Surg. 2006; 13 (5):370–377. doi: 10.1007/s00534-005-1076-y. [ PubMed ] [ CrossRef ] [ Google Scholar ]
- Decker O, Winter M, Brähler E, Beutel M. Between commodification and altruism: gender imbalance and attitudes towards organ donation. A representative survey of German. Journal of Gender studies. 2006; 17 (3):251–255. [ Google Scholar ]
- Schicktanz S, Rieger JW, Lüttenberg B. Geschlechterunterschiede bei der Lebendnierentransplantation: Ein Vergleich bei globalen, mitteleuropäischen und deutschen Daten und deren ethische Relevanz. Transplantationsmedizin. 2006; 19 :83–90. [ Google Scholar ]
- Kranenburg LW, Richards M, Zuidema WC, Weimar W, Hilhorst MT, Ijzermans JN, Passchier J, Busschbach JJ. Avoiding the issue: Patients’ (non)communication with potential living kidney donors. Patient Educ Couns. 2009; 74 :39–44. doi: 10.1016/j.pec.2008.07.028. [ PubMed ] [ CrossRef ] [ Google Scholar ]
- Gill P, Lowes L. Gift exchange and organ donation: Donor and recipient experiences of live related kidney transplantation. Int J Nurs Stud. 2008; 45 :1607–1617. doi: 10.1016/j.ijnurstu.2008.03.004. [ PubMed ] [ CrossRef ] [ Google Scholar ]
- Andersen MH. Follow-up interviews of 12 living kidney donors one year after open donor nephrectomy. Clin Transplant. 2007; 21 :702–709. [ PubMed ] [ Google Scholar ]
- Truog R. The Ethics of Organ Donation by Living Donors. N Engl J Med. 2005; 353 :444–446. doi: 10.1056/NEJMp058155. [ PubMed ] [ CrossRef ] [ Google Scholar ]
- Eibach U. Organspende von Lebenden: Auch unter Freunden ein Akt der „Nächstenliebe“? Zeitschrift für Medizinische Ethik. 1999; 45 :217–231. [ Google Scholar ]
- Paul N. In: Ethik der Lebendorganspende. Rittner C, Paul N, editor. Mainz: Akademie der Wissenschaften und der Literatur; 2005. Zu den Grenzen des Altruismus in der Lebendorganspende; pp. 205–215. [ Google Scholar ]
- Gutmann T. In: Organlebendspende in Europa. Gutmann T, Schroth U, editor. Berlin: Springer; 2002. Die Ethik der Lebendspende; pp. 107–122. [ Google Scholar ]
- Ressing M, Blettner M, Klug SJ. Systematic literature reviews and meta-analyses. Part 6 of a Series on Evaluation of Scientific Publications. Dtsch Arztebl Int. 2009; 106 (27):456–463. [ PMC free article ] [ PubMed ] [ Google Scholar ]
- Fogelin RJ, Sinnott-Armstrong W. Understanding Arguments. An Introduction to Informal Logic. Belmont, CA: Wadsworth; 2005. [ Google Scholar ]
- Toulmin SE. The Uses of Arguments. Cambridge: Cambridge University Press; 1993. [ Google Scholar ]
- Wuchterl K. Methoden der Gegenwartsphilosophie. Stuttgart: Paul Haupt; 1999. [ Google Scholar ]
- Dietrich J. In: Umweltkonflikte verstehen und bewerten: Ethische Urteilsbildung im Natur und Umweltschutz. Eser U, Mueller A, editor. München: Oekom-Verlag; 2006. Zur Methode ethischer Urteilsbildung in der Umweltethik; pp. 177–193. [ Google Scholar ]
- Bortz J, Döring N. Forschungsmethoden und Evaluation für Human- und Sozialwissenschaftler. Berlin/Heidelberg: Springer-Verlag; 2002. [ Google Scholar ]
- Lamnek S. Qualitative Sozialforschung. Lehrbuch. Psychologie Verlagsunion: Weinheim; 2005. [ Google Scholar ]
- Steinke I. In: Qualitative Forschung – Ein Handbuch. Flick U, Von Kardoff E, Steinke I, editor. Reinbek: Rowohlt Verlag; 2000. Gütekriterien qualitativer Forschung; pp. 319–331. [ Google Scholar ]
- Seale C. The Quality of Qualitative Research. London: SAGE Publications Ltd.; 1999. [ Google Scholar ]
- Bourgeault I, Dingwall R, De Vries R. Qualitative Methods in Health Research. London: SAGE Publications Ltd.; 2011. [ Google Scholar ]
- Green J, Thorogood N. Qualitative Methods for Health Research. 2. London: SAGE Publications Ltd.; 2009. [ Google Scholar ]
- Bergman MM. Mixed Methods Research. London: SAGE Publications Ltd.; 2009. [ Google Scholar ]
- Denzin N, Lincoln YS. The SAGE Handbook of Qualitative Research. 4. London: SAGE Publications Ltd.; 2012. [ Google Scholar ]
- Inthorn J. Quantitative und qualitative Forschungsmethoden in den Sozialwissenschaften. Eine philosophische Analyse der Argumente des Methodenstreits. Munich: PhD thesis. Munich School of Philosophy; 2010. [ Google Scholar ]
- Taylor HA, Hull SC, Kass NE. In: Methods in Medical Ethics. 2. Sugarman J, Sulmasy DP, editor. Washington: Georgetown University Press; 2010. Qualitative Methods; pp. 193–214. [ Google Scholar ]
- Pearlman RA, Starks HE. In: Methods in Medical Ethics. 2. Sugarman J, Sulmasy DP, editor. Washington: Georgetown University Press; 2010. Quantitative Surveys; pp. 233–250. [ Google Scholar ]
- Hurst S. What ‘empirical turn in bioethics’? Bioethics. 2010; 24 :439–444. doi: 10.1111/j.1467-8519.2009.01720.x. [ PubMed ] [ CrossRef ] [ Google Scholar ]
- Salloch S, Schildmann J, Vollmann J. Empirical research in medical ethics: How conceptual accounts on normative-empirical collaboration may improve research practice. BMC Med Ethics. 2012; 13 :5. doi: 10.1186/1472-6939-13-5. [ PMC free article ] [ PubMed ] [ CrossRef ] [ Google Scholar ]
- Forschungsgemeinschaft D. Sicherung guter wissenschaftlicher Praxis. Denkschrift. Wiley-VCH: Weinheim; 1998. [ Google Scholar ]
- Fuchs M. In: Forschungsethik. Eine Einführung. Fuchs M, Heinemann T, Heinrichs B, Hübner D, Kipper J, Rottländer K, Runkel T, Spranger TM, Vermeulen V, Völker-Albert M, editor. Stuttgart/Weimar: J.B. Metzler; 2010. Gute wissenschaftliche Praxis; pp. 41–55. [ Google Scholar ]
- Emanuel EJ, Wendler D, Grady C. In: The Oxford Textbook of Clinical Research Ethics. Emanuel EJ, Grady C, Crouch RA, Lie RK, Miller FG, Wendler D, editor. New York: Oxford University Press; 2008. An ethical framework for biomedical research; pp. 123–135. [ Google Scholar ]
- Heinemann T. In: Forschungsethik. Eine Einführung. Fuchs M, Heinemann T, Heinrichs B, Hübner D, Kipper J, Rottländer K, Runkel T, Spranger TM, Vermeulen V, Völker-Albert M, editor. Stuttgart/Weimar: J.B. Metzler; 2010. Forschung und Gesellschaft; pp. 98–119. [ Google Scholar ]
- Schünemann HJ, Wiercioch W, Etxeandia I, Falavigna M, Santesso N, Mustafa R, Ventresca V, Brignardello-Petersen R, Laisaar K-T, Kowalski S, Baldeh T, Zhang Y, Raid U, Neumann I, Norris SL, Thornton J, Harbour R, Treweek S, Guyatt G, Alonso-Coello P, Reinap M, Brožek J, Oxman A, Akl EA. Guidelines 2.0: systematic development of a comprehensive checklist for a successful guideline enterprise. CMAJ. 2013. http://cebgrade.mcmaster.ca/guidelinechecklistprintable.pdf . [ PMC free article ] [ PubMed ]
- Scottish Intercollegiate Guidelines Network: SIGN 50. A guideline developer’s handbook. Revised edition 2011. Edinburgh. 2011. http://sign.ac.uk/guidelines/fulltext/50/compchecklist.html .
- Schulz KF, Altman DG, Moher D. CONSORT Group. CONSORT 2010 Statement. Updated Guidelines for Reporting Parallel Group Randomised Trials. PLoS Med. 2010; 7 (3):e1000251. doi: 10.1371/journal.pmed.1000251. http://www.consort-statement.org/consort-statement/overview0/#checklist . [ PMC free article ] [ PubMed ] [ CrossRef ] [ Google Scholar ]
- Von Elm E, Altman DG, Egger M, Pocock SJ, Gøtzsche PC, Vandenbroucke JP. The Strengthening the Reporting of Observational Studies in Epidemiology (STROBE) statement: guidelines for reporting observational studies . PLoS Med. 2007; 4 :e296. doi: 10.1371/journal.pmed.0040296. http://www.strobe-statement.org/fileadmin/Strobe/uploads/checklists/STROBE_checklist_v4_combined.pdf . [ PMC free article ] [ PubMed ] [ CrossRef ] [ Google Scholar ]
- Young JM, Solomon MJ. How to critically appraise an article. Nat Rev Gastroenterol Hepatol. 2009; 6 :82–91. doi: 10.1038/ncpgasthep1331. [ PubMed ] [ CrossRef ] [ Google Scholar ]
- The Critical Appraisal Company. http://www.criticalappraisal.com .
- Graumann S, Lindemann G. Medizin als gesellschaftliche Praxis, sozialwissenschaftliche Empirie und ethische Reflexion: ein Vorschlag für eine soziologisch aufgeklärte Medizinethik. Ethik Med. 2009; 21 :235–245. doi: 10.1007/s00481-009-0021-z. [ CrossRef ] [ Google Scholar ]
- Hirschauer S, Kalthoff H. Lindemann G (eds.): Theoretische Empirie. Zur Relevanz qualitativer Forschung. Frankfurt am Main. Berlin: Suhrkamp; 2008. [ Google Scholar ]
- Kelle U. Integration qualitativer und quantitativer Methoden in der empirischen Sozialforschung. Theoretische Grundlagen und methodologische Konzepte, 2. ed. VS Verlag für Sozialwissenschaften: Wiesbaden; 2008. [ Google Scholar ]
- De Vries R. How can we help? From “Sociology in” to “Sociology of” Bioethics. J Law Med Ethics. 2003; 32 (2):279–292. [ PubMed ] [ Google Scholar ]
- Schicktanz S, Schweda M, Wynne B. The ethics of ‘public understanding of ethics’ – Why and how bioethics expertise should include public and patients’ Voices. Med Health Care Philos. 2011; 15 (2):129–139. [ PMC free article ] [ PubMed ] [ Google Scholar ]
- Taylor C. Quellen des Selbst, Frankfurt am Main: Suhrkamp. 1994.
- Anderson J. In: The Contingent Nature of Life. 39. Düwell M, Rehmann-Sutter C, Mieth D, editor. New York: International Library of Ethics, Law, and the New Medicine; 2008. Neuro-Prosthetics, the Extended Mind, and Respect for Persons with Disability; pp. 259–274. [ Google Scholar ]
- Khushf G. Why Bioethics needs the Philosophy of Medicine: Some Implications of Reflection on Concepts of Health and Disease. Theor Med. 1997; 18 :145–163. doi: 10.1023/A:1005730108133. [ PubMed ] [ CrossRef ] [ Google Scholar ]
- Appiah KA. The ethics of identity. University Press: Princeton; 2005. [ Google Scholar ]
- Strech D. Evidenz und Ethik. Kritische Analysen zur Evidenz-basierten Medizin und empirischen Ethik. Berlin: Lit Verlag; 2008. [ Google Scholar ]
- Weaver GR, Trevino LK. Normative and Empirical Buisness Ethics: Separation, Marriage of Convenience, or Marriage of Necessity? Bus Ethics Q. 1994; 4 (2):129–143. doi: 10.2307/3857485. [ CrossRef ] [ Google Scholar ]
- Reiter-Theil S, Mertz M, Schürmann J, Stingelin Giles N, Meyer-Zehnder B. Evidence – competence – discourse: the theoretical framework of the multi-centre clinical ethics support project METAP. Bioethics. 2011; 25 (7):403–412. doi: 10.1111/j.1467-8519.2011.01915.x. [ PubMed ] [ CrossRef ] [ Google Scholar ]
- Leget C, Borry P, De Vries R. Nobody tosses a dwarf!The relation between the empirical and the normative reexamined. Bioethics. 2009; 23 :226–235. doi: 10.1111/j.1467-8519.2009.01711.x. [ PMC free article ] [ PubMed ] [ CrossRef ] [ Google Scholar ]
- Lenk H. Einführung in die Angewandte Ethik. Verantwortlichkeit und Gewissen. W. Kohlhammer GmbH: Stuttgart/Berlin/Köln; 1997. [ Google Scholar ]
- Wöhlke S. In: Public Engagement in Organ Donation. Schicktanz S, editor. Randhawa. Lengerich: Pabst Science Publishers; The morality of giving and receiving living kidneys: Empirical findings on opinions of affected patients. in press. [ Google Scholar ]
- Ashcroft RE. Constructing empirical bioethics: Foucauldian reflections on the empirical turn in bioethics research. Health Care Anal. 2003; 11 :3–13. doi: 10.1023/A:1025329811812. [ PubMed ] [ CrossRef ] [ Google Scholar ]
- Salloch S, Vollmann J, Schildmann J. Ethics by opinion poll? The functions of attitudes research for normative deliberations in medical ethics. J Med Ethics. doi:10.1136/medethics-2012-101253. [ PubMed ]
- Parker M. Two concepts of empirical ethics. Bioethics. 2009; 23 (4):202–213. doi: 10.1111/j.1467-8519.2009.01708.x. [ PubMed ] [ CrossRef ] [ Google Scholar ]
- Strech D, Synofzik M, Marckmann G. Systematic reviews of empirical bioethics. J Med Ethics. 2008; 34 :472–477. doi: 10.1136/jme.2007.021709. [ PubMed ] [ CrossRef ] [ Google Scholar ]
- Solbakk JH. Use and abuse of empirical knowledge in contemporary bioethics. A critical analysis of empirical arguments employed in the controversy surrounding studies of maternal-fetal HIV-transmission and HIV-prevention in developing countries. Med Health Care Philos. 2004; 7 :5–16. [ PubMed ] [ Google Scholar ]
- Israel M, Hay I. Research Ethics for Social Scientists. London: Sage Publications; 2006. [ Google Scholar ]
- Beauchamp TL, Childress JF. Principles of Biomedical Ethics. 6. New York: Oxford University Press; 2009. [ Google Scholar ]
- Armstrong PW, Califf RM. Data and safety monitoring boards: academic credit where credit is due? JAMA. 2013; 310 (15):1563–1564. doi: 10.1001/jama.2013.280383. [ PubMed ] [ CrossRef ] [ Google Scholar ]
- National Academy of Sciences. Committee on Ensuring the Utility and Integrity of Research Data in a Digital Age; Committee on Science, Engineering, and Public Policy (COSEPUP); Policy and Global Affairs (PGA); Institute of Medicine (IOM): Ensuring the Integrity, accessibility, and Stewardship of research data in the digital age. Washington, D.C: The National Academies Press; 2009. http://www.nap.edu/openbook.php?record_id=12615 . [ PubMed ] [ Google Scholar ]
- Faden RR, Beauchamp TL. A History and Theory of Informed Consent. Oxford: Oxford University Press; 1986. [ Google Scholar ]
- Annas GJ, Grodin MA. In: The Oxford Textbook of Clinical Research Ethics. Emanuel EJ, Grady C, Crouch RA, Lie RK, Miller FG, Wendler D, editor. New York: Oxford University Press; 2008. The Nuremberg Code; pp. 136–140. [ Google Scholar ]
- National Academy of Sciences, National Academy of Engineering. Committee on Science, Engineering, and Public Policy (COSEPUP); Institute of Medicine (IOM); Policy and Global Affairs (PGA): On being a scientist. A guide to responsible conduct in research. Third. Washington, D.C: The National Academies Press; 2009. http://www.nap.edu/openbook.php?record_id=12192 . [ PubMed ] [ Google Scholar ]
- Institute of Medicine (IOM) Conflicts of Interest in Medical Research, Education, and Practice. Washington D.C: National Academic Press; 2009. [ Google Scholar ]
- European Medicines Agency (EMA) ICH Topic E6 (R1) Guideline for Good Clinical Practice. http://www.emea.europa.eu/docs/en_GB/document_library/Scientific_guideline/2009/09/WC500002874.pdf .
- World Medical Association (WMA) WMA-Declaration of Helsinki – Ethical Principles for Medical Research Involving Human Subjects. http://www.wma.net/en/30publications/10policies/b3/ [ PubMed ]
- German Psychological Society (DGP) and the Association of German Professional Psychologists (BDP) Ethical Principles of the German Psychological Society and the Association of German Professional Psychologists. http://www.bdp-verband.org/bdp/verband/clips/ethic.pdf .
- Ethics Committee of the British Psychological Society. Code of Ethics and Conduct. Guidance. 2009. http://www.bps.org.uk/sites/default/files/documents/code_of_ethics_and_conduct.pdf .
- National Committees for Research Ethics in Norway. Guidelines for Research Ethics in the Social Sciences, Law and the Humanities. http://graduateschool.nd.edu/assets/21765/guidelinesresearchethicsinthesocialscienceslawhumanities.pdf .
- Respect Project. RESPECT Code of Practice for Socio-Economic Research. http://www.respectproject.org/code/respect_code.pdf .
- Canadian Institutes of Health Research. Natural Sciences and Engineering Research Council of Canada, and Social Sciences and Humanities Research Council of Canada: Tri-Council Policy Statement: Ethical Conduct for Research Involving Humans. http://www.pre.ethics.gc.ca .
- Jacoby L, Siminoff L. Empirical Methods for Bioethics. Oxford: A Primer. Elsevier JAI; 2008. [ Google Scholar ]
- Miller FG, Wendler D. The relevance of empirical research in bioethics. Schizophr Bull. 2006; 32 :37–41. [ PMC free article ] [ PubMed ] [ Google Scholar ]
- Nilstun T, Melltorp G, Hermeren G. Surveys on attitudes to active euthanasia and the difficulty of drawing normative conclusions. Scand J Public Health. 2000; 28 :111–116. doi: 10.1177/140349480002800206. [ PubMed ] [ CrossRef ] [ Google Scholar ]
- Strech D, Schildmann J. Quality of ethical guidelines and ethical content in clinical guidelines: the example of end-of-life decision-making. J Med Ethics. 2011; 37 (7):390–396. doi: 10.1136/jme.2010.040121. [ PubMed ] [ CrossRef ] [ Google Scholar ]
- 1-icon/ui/arrow_right Amsterdam Public Health
- 1-icon/ui/arrow_right Home
- 1-icon/ui/arrow_right Research Lifecycle
More APH...
- 1-icon/ui/arrow_right About
- 1-icon/ui/arrow_right News
- 1-icon/ui/arrow_right Events
- 1-icon/ui/arrow_right Research information
- 1-icon/ui/arrow_right Our strenghts
- Amsterdam Public Health
- Research Lifecycle
- Research information
- Our strenghts
- Proposal Writing
- Study Preparation
- Methods & Data Collection
- Process & Analyze Data
- Writing & Publication
- Archiving & Open Data
- Knowledge Utilization
- Supervision
Introduction qualitative research
- Set-up & Conduct
Qualitative research
Introduction.
- Research design
The field of qualitative research is very broad, with many different theoretical approaches and research methods. This guideline aims to offer you a short introduction to this field and to make you attentive towards some important aspects. Many references for further reading are offered, as well as several examples of qualitative research.
Overall, qualitative studies mostly aim to answer questions about the ‘what’, ‘how’ of ‘why’ of a phenomenon, instead of questions on ‘how many’ or ‘how much’. These studies are mainly about the nature of a phenomenon and perceptions or experiences of respondents. Qualitative research methods are particularly appropriate if you want to study the meaning of interactions, processes, behaviours, feelings, attitudes and experiences or if you want to give a detailed in-depth description of a situation or a case.
It is strongly advised to read this complete guideline before starting a qualitative study, because an important feature of qualitative research is that it is an iterative process. This means that data collection and analysis take place simultaneously and the researcher can also go back to the previous phase. The analysis of the material starts as soon as the first data have been collected. In a way, this analysis guides subsequent data collection. The researcher starts with a few global insights or sensitizing concepts that provide an initial frame for the initiation of the search process. After having become acquainted with the field, these insights or preliminary concepts can become important focus points for the research questions, and finally focused work can be undertaken using the specific research questions.
The novice qualitative researcher, with little prior knowledge of social science theory, is advised to read Green & Thorogood (2010). They give an introduction to qualitative methods in health research and describe the designing, conducting and writing stage: Green, J. & Thorogood N. (2010) Qualitative Methods for Health Research. Third edition. London: Sage Publications.
Differences between qualitative and quantitative research
For researchers familiar with quantitative research, it is important to realize there are some fundamental differences between qualitative and quantitative research. For an overview of different research paradigms, see this figure.
Mixed methods research
In mixed methods research , qualitative and quantitative research is combined. For example, qualitative research can be used to gain more insight into a relatively unknown phenomenon or topic and this can be used to develop a survey to quantify the results. Or qualitative research can help to get a better understanding of quantitative results. According to Creswell and Clark (2011) mixed methods ‘focuses on collecting, analyzing, and mixing both quantitative and qualitative data in a single study or series of studies. Its central premise is that the use of quantitative and qualitative approaches in combination provides a better understanding of research problems than either approach alone.’
Masks Strongly Recommended but Not Required in Maryland, Starting Immediately
Due to the downward trend in respiratory viruses in Maryland, masking is no longer required but remains strongly recommended in Johns Hopkins Medicine clinical locations in Maryland. Read more .
- Vaccines
- Masking Guidelines
- Visitor Guidelines
Institute for Excellence in Education
Committed to Leading the Way in Medical and Biomedical Education
The mission of the Institute for Excellence in Education (IEE) is to promote, value and advance the educational mission of the School of Medicine while enhancing the school's leadership role in medical and biomedical education. To guide this mission, IEE has identified four guiding principles or "pillars" into which all of our decisions, work and programs are aligned.
For the 2023-2024 academic year, the IEE is highlighting the theme of “Successful Education: Thriving Learners, Flourishing Educators.”
About the IEE
Educator resources, partner with us, join our mailing list, explore the pillars of our mission.
Improving Teaching
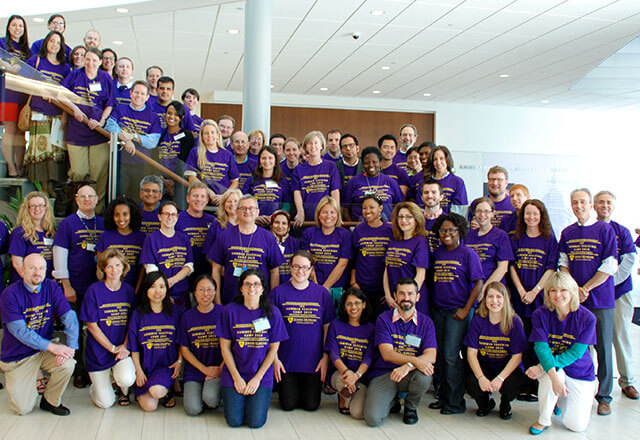
Inspiring and Supporting Research, Scholarship and Innovation in Education
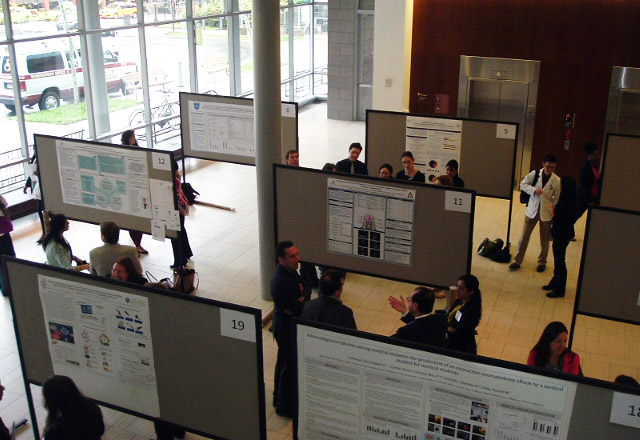
Valuing and Recognizing Teachers and Educators
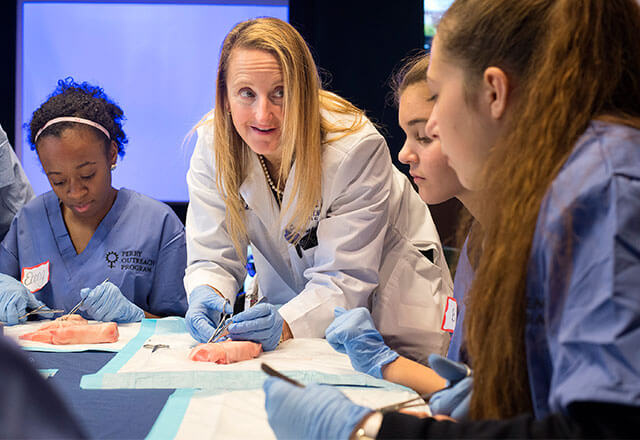
Fostering a Community of Educators
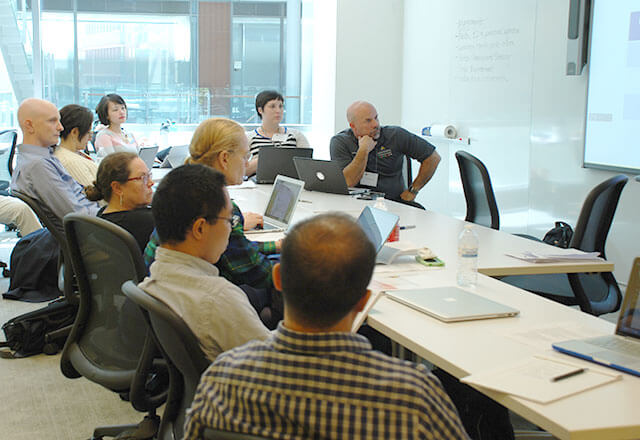
IEE Calls to Action
Funding opportunities.
Apply for grant funding through our small grants program , the Faculty Education Scholars Program , or submit a proposal to be considered for IEE Education Shark Tank.
Explore programs
Nominations
The IEE Outstanding Educator Awards are designed to recognize and celebrate our outstanding educators and acknowledge great achievements in education. The awards are open to any full- or part-time faculty members of the School of Medicine, and are peer nominated, reviewed and selected.
Make a nomination
Present Your Scholarship
Submit a scholarship or innovation abstract to be considered for the upcoming IEE Education Conference and Celebration. We invite you to submit abstracts in all areas of medical, biomedical, nursing or public health education.
Celebrating 10 Years of the IEE
The Institute for Excellence in Education of the Johns Hopkins University School of Medicine celebrated its 10th anniversary!
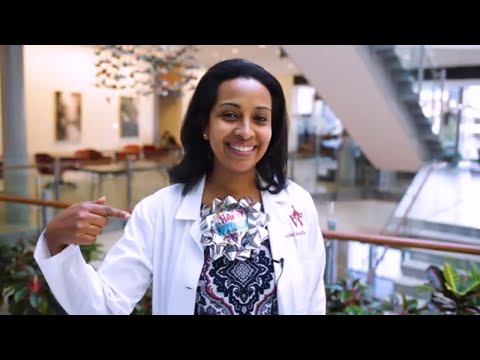
Educator Domains, Competencies and Metrics
The Educator Domains, Competencies and Metrics were developed by a diverse committee of Hopkins educator faculty, leaders and educators. We hope these tools will be useful to faculty as they plan their educational careers; mentors, coaches and advisors as they help guide faculty; leadership as they plan and build programs; and those evaluating educators to give recognition for the work done.
It is also our hope that the Johns Hopkins University School of Medicine Foundational Principles for Teaching and Education will be inspiring to Johns Hopkins leaders, educators and learners.
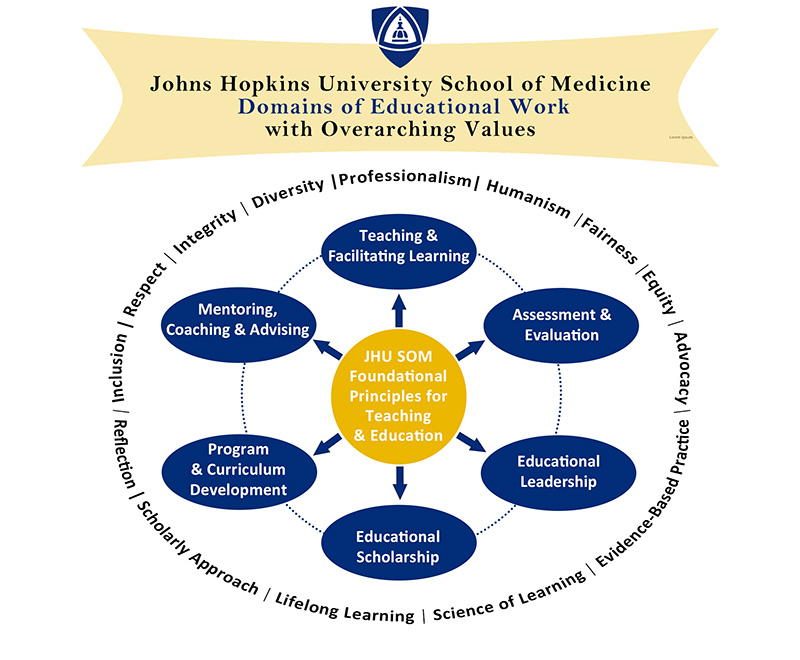
Foundations of Teaching and Learning Course
Foundations of educational scholarship course, foundations of curriculum development concepts, foundations of assessment and evaluation, foundations of educational leadership, upcoming events.
Medical and Biomedical Education Grand Rounds David Asai, PhD 4:00 - 5:00 pm Basic Sciences Building
26 JUN 2024
Medical and Biomedical Education Grand Rounds Cornelius James, MD 4:00 pm - 5:00 pm Armstrong Medical Education Building Strauch Auditorium
25 JUL 2024
Summer Teaching Camp 9:00 PM - 5:00 PM Armstrong Medical Education Building REGISTRATION OPEN
Our Community of Educators
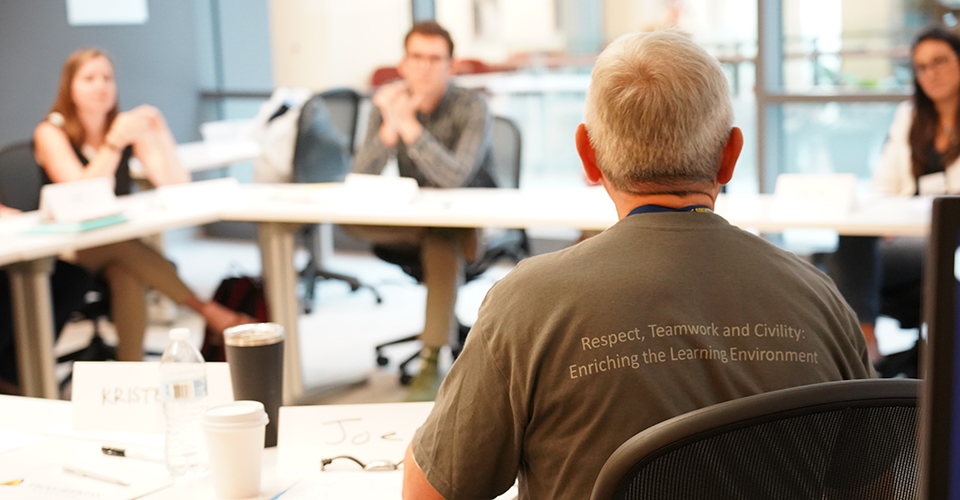
- Open access
- Published: 24 June 2024
Development of a quantitative index system for evaluating the quality of electronic medical records in disease risk intelligent prediction
- Jiayin Zhou 1 na1 ,
- Jie Hao 1 na1 ,
- Mingkun Tang 1 ,
- Haixia Sun 1 ,
- Jiayang Wang 2 ,
- Jiao Li 1 &
- Qing Qian 1
BMC Medical Informatics and Decision Making volume 24 , Article number: 178 ( 2024 ) Cite this article
14 Accesses
Metrics details
This study aimed to develop and validate a quantitative index system for evaluating the data quality of Electronic Medical Records (EMR) in disease risk prediction using Machine Learning (ML).
Materials and methods
The index system was developed in four steps: (1) a preliminary index system was outlined based on literature review; (2) we utilized the Delphi method to structure the indicators at all levels; (3) the weights of these indicators were determined using the Analytic Hierarchy Process (AHP) method; and (4) the developed index system was empirically validated using real-world EMR data in a ML-based disease risk prediction task.
The synthesis of review findings and the expert consultations led to the formulation of a three-level index system with four first-level, 11 second-level, and 33 third-level indicators. The weights of these indicators were obtained through the AHP method. Results from the empirical analysis illustrated a positive relationship between the scores assigned by the proposed index system and the predictive performances of the datasets.
The proposed index system for evaluating EMR data quality is grounded in extensive literature analysis and expert consultation. Moreover, the system’s high reliability and suitability has been affirmed through empirical validation.
The novel index system offers a robust framework for assessing the quality and suitability of EMR data in ML-based disease risk predictions. It can serve as a guide in building EMR databases, improving EMR data quality control, and generating reliable real-world evidence.
Peer Review reports
Introduction
The onset of the digital health era has led to a paradigm shift in health management, transitioning from a focus on reactive treatment to proactive prevention [ 1 ]. Disease risk intelligent prediction has become a vital strategy in proactive health management, aiming to identify potential risk factors and prevent the progression of diseases. By harnessing the capabilities of Artificial Intelligence (AI) technologies and Machine Learning (ML) approaches, healthcare professionals can gain valuable insights into diseases, enabling the development of more effective preventive treatment plans [ 2 , 3 ].
Johnson [ 4 ] applied four different ML-based models to predict subsequent deaths or cardiovascular events in a cohort of 6,892 patients. The study found that the ML-based model had superior discrimination ability compared to traditional coronary Computed Tomography (CT) scores in identifying patients at risk of adverse cardiovascular events. Electronic medical records (EMR) data, as a valuable real-world data source, plays a critical role in disease risk prediction using ML techniques [ 4 ]. An EMR refers to a digital version of a patient’s medical record, encompassing medical history, medications, test results, and other relevant information [ 5 , 6 ]. Healthcare providers commonly utilize EMRs to document and track patient information, enabling comprehensive decision-making regarding patient care. Furthermore, clinical researchers can leverage de-identified EMR data to study disease patterns, develop novel treatments, and advance medical knowledge. The integration of ML with EMRs has recently shown significant improvements in predicting patient outcomes, such as identifying individuals with suspected coronary artery disease [ 7 ] or forecasting the likelihood of open-heart surgery [ 8 ]. These advancements highlight the potential of ML in enhancing the efficiency of clinical decision-making [ 9 ].
Nevertheless, several studies have raised concerns about the quality of EMRs in clinical research, emphasizing issues such as lack of data standardization, incomplete or missing clinical data, and discrepancies in data types and element representations [ 10 , 11 ]. Ensuring the quality of EMR data is crucial, as it forms the bedrock for effective utilization of EMRs. High-quality EMR data not only supplies robust evidence, but also accelerates the clinical research process, shortens its timeline, and reduces associated risks. Therefore, controlling and evaluating EMR data quality are pivotal in upholding the overall quality and integrity of clinical research.
Despite numerous studies investigating the assessment of EMR data quality in clinical research, it is noteworthy that the body of literature evaluating EMR data quality is growing [ 12 , 13 ]. However, publicly published clinical studies employing ML techniques and utilizing EMR data frequently overlook data quality or implement methods lacking expert knowledge or evidential support. While methods for data quality evaluation have been described in the informatics literature, researchers without specialized knowledge in this field may find difficulty choosing the appropriate evaluation method in line with the available data and research problems [ 14 ]. Furthermore, the existing quality assessment framework primarily relies on qualitative approaches, making objective measurement of quality and suability challenging.
In this paper, we aim to develop and validate a quantitative index system for evaluating the quality of EMR in disease risk prediction using ML. The proposed index system is intended to provide guidance for utilizing EMR data in research, enhance the quality of EMR data within a Hospital Information System (HIS), and facilitate the implementation of clinical decision-making research based on EMR data. By applying the proposed index system, researchers and healthcare professionals can make knowledgeable decisions regarding the use of EMR data for ML-based disease prediction research, ultimately improving patient care and advancing medical knowledge.
In this paper, we present the development of a quantitative index system, depicted in Fig. 1 , designed to ensure the quality control of EMR data in disease prediction models. The development process incorporated the use of the Delphi method and the analytic hierarchy process (AHP). In addition, an empirical study was undertaken to validate the effectiveness of the developed index system using real-world EMR data in disease risk intelligent prediction.
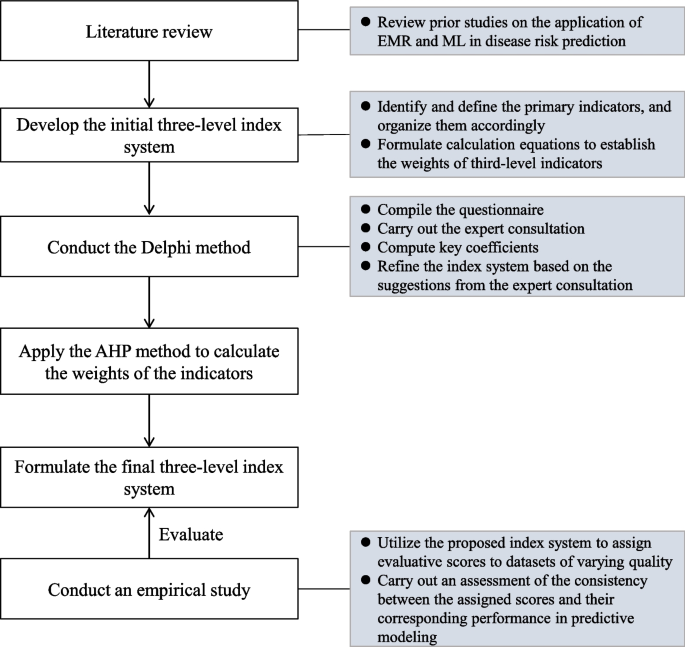
Workflow of the study
Sketching the preliminary index system
Preliminary indicator identification, definition, and organization.
The initial set of indicators was determined through a comprehensive literature review of studies published before September 27, 2021, obtained from the PubMed database. The search query used was “(machine learning) AND (electronic medical records) AND (disease prediction)”, which resulted in 549 papers. The inclusion criteria required that the research data be related to EMR or HIS and that disease risk was predicted using ML techniques. Review articles and papers deemed to have low relevance were excluded, leading to the removal of 225 papers based on the fulfillment of the exclusion criteria after reading abstracts.
Further screening was conducted by reading the full papers to eliminate studies that did not involve EMR or HIS data or utilized disease prediction methods other than machine learning. Additionally, 18 relevant papers were included by examining the reference lists of the selected studies. Ultimately, a total of 229 papers were retained for the development of the preliminary index system. The detailed process of paper screening is illustrated in Fig. 2 .
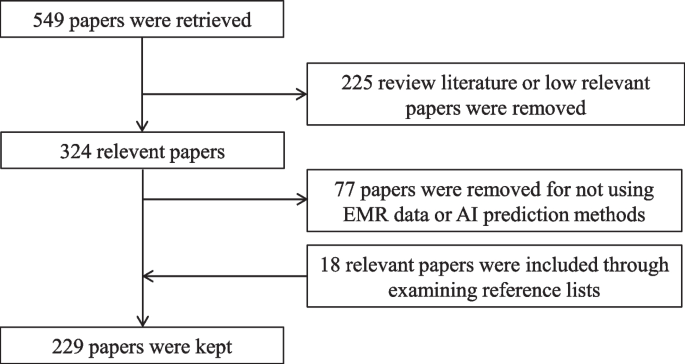
Flowchart of paper screening
Upon analyzing the review results, we formulated an initial multi-level index system consisting of four first-level, 11 second-level, and 33 third-level indicators. The first-level indicators represent broad dimensions of data quality, while the second-level indicators correspond to the general dimensions specifically for EMR data quality. The third-level indicators capture specific dimensions relevant to EMR-based disease prediction models.
Calculation methods
We utilized the AHP method to determine the weights of the first- and second-level indicators in the three-level index system. The weights of the third-level indicators were calculated using percentages or binary values according to their definitions. The calculation formulas of these third-level indicators will be assessed in the forthcoming Delphi consultation.
Developing a three-level index system using the Delphi method
Questionnaire compilation and expert consultation.
We conducted a Delphi consultation to gather feedback from experts based on the preliminary index system. The consultation questionnaire, provided in Additional file 1 , consists of four parts: experts' basic information (see Table S1), familiarity and judgment basis with AI-based disease prediction (see Table S2-S3), evaluation tables for the preliminary index system (see Table S4-S6), and an evaluation table for the calculation formulas of the third-level indicators (see Table S7). The importance of the preliminary indicators was measured using a 5-point Likert scale, ranging from “very unimportant” to “very important”. To ensure the extensibility of the preliminary index system, three additional options were included: delete, modify, and new indicator(s). For the calculation formulas part, experts were asked to provide a yes or no response, and if the answer was no, a suggestion for modification was requested.
A total of twenty experts specializing in healthcare/EMR data governance and medical AI were selected for the Delphi consultation. The inclusion criteria for the selection were as follows: (1) holding a Ph.D. degree or being a senior technical associate; (2) possessing more than two years of research experience in related fields; (3) being familiar with the construction and evaluation of EMR data; and (4) being able to give feedback in a timely manner. We conducted a single-round consultation since the nature of our consulting panel was relatively small and homogeneous [ 15 ].
Key coefficients and statistical analyses
To achieve relatively consistent and reliable feedback from the questionnaire, we calculated four metrics: the experts' positive coefficients, expert authority coefficients (Cr), coefficient of variation (CV), and Kendall's coefficient of concordance. The experts' positive coefficients were determined based on the response rate to the questionnaire. A response rate of 70% or higher is considered satisfactory [ 16 ]. The Cr was calculated as the average of the familiarity coefficient (Cs) and the judgment coefficient (Ca), reflecting the reliability of the expert consultation. A Cr value of 0.7 or above is considered acceptable. The CV measures the consistency of indicators on the same level. A CV value less than 0.25 is expected, indicating a high level of consistency [ 17 ]. Kendall's coefficient of concordance evaluates the overall consistency of all indicators in the system. It ranges from zero to one, with a value greater than 0.2 considered acceptable [ 18 ]. All statistical analyses were performed using Microsoft Excel/IBM SPSS 25.0.
Using the AHP method for weight assignment
We applied the AHP method to determine the weights of indicators at each level, which is a well-known technique in multiple criteria decision-making [ 19 ]. AHP enables the quantification of criteria and opinions that are difficult to measure numerically, and its outcomes are free from subjective influence due to its use of pairwise comparisons and eigenvalues [ 20 ].
In this study, our AHP method was conducted in three steps. First, we obtained the importance ratings of experts for each indicator. Then, we averaged these ratings for each indicator and performed pairwise comparisons among indicators at the same level that belong to the same upper level. This step allowed us to construct multiple judgment matrices based on their ratios.
Second, we calculated the eigenvectors of each indicator by normalizing the judgement matrix. A larger eigenvector for an indicator represents a higher relative importance. The relative weights of indicators at the same level were determined by standardizing the eigenvectors. For the first-level indicators, their relative weights were equal to their absolute weights. For the second- and third-level indicators, their absolute weights were calculated by multiplying their relative weights with the absolute weight of the upper level.
Third, we performed a consistency test using the consistency ratio (CR) to evaluate the consistency of the judgment matrices. A CR below 0.1 indicated that the judgment matrices were consistent and that the obtained weights were considered valid [ 21 ]. The steps of the AHP method are illustrated in Fig. 3 .
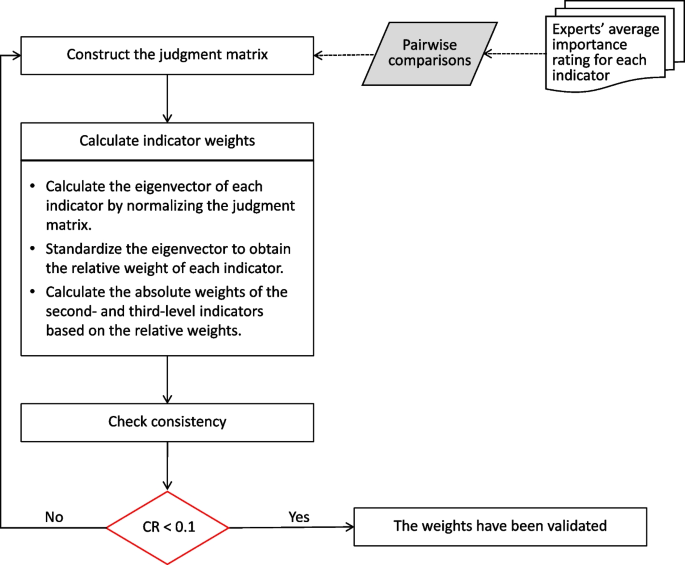
Flowchart of the AHP method
Evaluating the index system through prediction tasks
To further validate the suitability of the proposed index system, an empirical study was conducted using real-world EMR data for disease risk prediction.
Dataset construction
To ensure a fair assessment, we opted to generate multiple datasets from a single EMR data resource. The chosen data resource needed to be large-scale, open-access, and regularly updated. Once the data resource was identified, we constructed several datasets with varying sample types but maintaining the same set of attributes.
For each dataset, we computed the scores of 33 third-level indicators using the established calculation formulas. The weights of the proposed index system were applied to obtain weighted scores for all indicators within each dataset. The overall score of a dataset was subsequently computed by summing the scores of the first-level indicators.
Predictive modeling
In the context of disease risk prediction, we considered three widely used ML models: logistic regression (LR), support vector machine (SVM), and random forest (RF). LR is a traditional classification algorithm used to estimate the probability of an event occurring [ 22 ]. SVM, a nonlinear classifier, employs a kernel function to transform input data into a higher-dimensional space, making it effective in handling complex relationships and nonlinear patterns [ 23 ]. RF is an ensemble method that combines the predictions from multiple decision trees. It has shown great success in disease risk prediction tasks by reducing overfitting and improving predictive accuracy [ 24 ]. For our analysis, we used the scikit-learn python library [ 25 ] to implement LR, SVM, and RF.
Reliability analysis
In our study, we conducted reliability analysis to examine the relationship between the scores obtained from our constructed datasets and the performance of predictive models. we applied Pearson correlation for assessing linear relationships [ 26 ] and Spearman correlation for nonlinearity [ 27 ]. The Pearson correlation coefficient was calculated using the formula:
Here, \({x}_{i}\) and \({y}_{i}\) represent individual data points from the two respective datasets, while \(\overline{x }\) and \(\overline{{\text{y}} }\) denote the mean values of these datasets. A Pearson correlation coefficient near 1 or -1 indicates a strong linear relationship between dataset scores and model performance, whereas a value close to 0 suggests a very weak linear relationship.
Similarly, the Spearman correlation coefficient was calculated using the formula:
Here, \({d}_{i}\) represents the difference in rank between the two datasets for the i -th observation, and n denotes the total number of observations. A Spearman correlation coefficient near 1 or -1 indicates a strong nonlinear relationship, while a value close to 0 suggests a very weak relationship.
In both analyses, statistical significance was established with a p-value less than 0.05. This finding indicates a significant correlation between the scores of our constructed datasets and the performance of predictive models. Thus, this statistically significant outcome supports the reliability of our proposed index system in evaluating the data quality of EMR for intelligent disease risk prediction.
The characteristics of the experts
In the Delphi consultation, a total of twenty experts were invited, of which 17 actively participated, yielding a response rate of 85.0%. Out of the 17 experts, 16 provided feedback that met the credibility criteria for a Delphi study, resulting in an effective response rate of 94.1%. These response rates reflect a high degree of expert engagement.
Most of the participating experts were male, held Ph.D. degrees, and specialized in medical informatics or medical AI. Over half of the experts were aged between 40 and 50 years, and 62.5% had between 10 and 20 years of work experience. Moreover, 68.7% of the experts occupied senior associate positions or higher. For detailed information, see Table S8 in Additional file 3 .
The key coefficients of the Delphi method
The degree of expert authority (Cr) is defined by two factors: the expert's familiarity with the consultation content (Cs) and the basis of expert judgment (Ca). Of the 16 participating experts, 7 were found to be very familiar with the content, while 9 were relatively familiar. This indicates an overall sound understanding of the field among the experts. Only two experts exhibited a low judgment basis, suggesting that the majority of the experts were well-equipped to offer informed judgment. Details of expert familiarity and judgment basis can be found in Table S9 in Additional file 3 .
Cr was calculated to be 0.89, with Cs and Ca values of 0.88 and 0.90, respectively. These values indicate a high level of expert authority and reliability in the consultation results. The CV values for the first-level indicators were less than 0.16, for the second-level indicators were less than 0.20, and for the third-level indicators were no more than 0.25. These low CV values indicate a high level of consistency among experts' scores for the preliminary indicators at each level. Kendall's coefficients of concordance for all three levels were greater than 0.30, indicating a substantial level of agreement among the experts. Additionally, the p-values for the preliminary second- and third-level indicators were very small, further confirming the consistency of experts' scores for each preliminary indicator. Overall, the results demonstrate a high level of consistency and reliability in the experts' assessments for each preliminary indicator.
The final weighted three-level index system
Experts' comments focused on changes to the definition of indicators. After further discussions with experts, all preliminary indicators were included in the final weighted three-level index system, as shown in Table 1 . No new indicators were added to the system. The index system comprises four first-level indicators, 11 second-level indicators, and 33 third-level indicators, with the weights determined using the AHP method and percentages.
The first-level indicators represent a series of data quality characteristics that determine the suitability of EMR data for disease risk intelligent prediction research. The second-level indicators provide a concrete representation or evaluation of the first-level indicators, making it easier for users to understand their extension or evaluation. The third-level indicators further specify the second-level indicators, providing clear quality requirements for different levels of granularity in the EMR dataset, such as data records, data elements, and data element values. This facilitates users in understanding the evaluation needs and contents more clearly. For detailed information on the indicators, please see Additional file 2 .
Data preprocessing
In this empirical study, the MIMIC-III clinical database was chosen as the representative real-world EMR data resource. MIMIC-III Footnote 1 is an extensive and freely accessible database that contains comprehensive health-related data from more than 46,000 patients admitted to intensive care unit (ICU) at the Beth Israel Deaconess Medical Center between 2001 and 2012 [ 28 ]. For this study, we utilized MIMIC-III v1.4, which is the latest version released in 2016 [ 29 ] and ensures effective control over EMR data.
Sepsis is a leading cause of mortality among ICU patients, highlighting the importance of accurate sepsis risk prediction for precise treatments in the ICU [ 30 ]. Hence, we selected sepsis as the disease prediction task using the MIMIC-III database. Potential predictors were extracted from the records of vital signs, routine blood examinations [ 31 ], liver function tests [ 32 ] and demographic information. The outcome variable for the prediction task is the occurrence of sepsis. Furthermore, we obtained five different populations of ICU patients with a high risk of sepsis from the MIMIC-III database. The number of patients in each population, categorized as elderly (> 80 years old), long-stay (> 30 days of length of stay, LLOS), ischemic stroke, acute renal failure (ARF), and cirrhosis (CIR), is presented in Table S10 in Additional file 3 .
Scoring datasets
According to the proposed index system, we evaluated the five datasets and assigned scores to each indicator based on their respective weights in the system. The detailed list of scores of divergent indicators for each dataset can be found in Table S11 in Additional file 3 . In Table 2 , we present the scores of first-level indicators. It is important to note that the scores for the operability indicator were consistent across all five datasets, with a value of 0.251. This is because these datasets were obtained from a single resource.
When considering the overall scores, the LLOS dataset achieved the highest score of 0.966, indicating a higher level of quality, while the ARF dataset obtained the lowest score of 0.907. These scores provide an assessment of the datasets' suitability and quality for disease risk prediction using the proposed index system.
Experimental results
Additional data processing was conducted to prepare the datasets for training ML models. To address the missing values, median imputation was applied to predictors with a small proportion of missing values in each dataset. To mitigate potential bias arising from imbalanced datasets, we applied undersampling on the majority class to achieve a balanced ratio of 1:1. Each dataset was then randomly split into 80% for training and 20% for testing. To ensure fairness in model comparison, the predictors were normalized, and a tenfold cross-validation was performed during the training process.
Regarding model hyperparameters, the LR model applied the 'liblinear' solver method. The SVM model utilized a Radial Basis Function kernel, with a regularization parameter (C) set to 1.0, and the gamma value was set to 'scale'. For the RF model, it was constructed with 10 trees (n_estimators = 10), a maximum tree depth of 7 (max_depth = 7), and optimal feature selection (max_features = ‘auto’).
The evaluation of model performance was based on accuracy (ACC), precision, and area under the curve (AUC). Accuracy represents the proportion of correct predictions made by a model among all predictions. Precision measures the proportion of true positive predictions among all positive predictions made by a model. AUC, also known as the area under the receiver operating characteristic curve, is a metric used to evaluate the performance of binary classification models [ 33 ].
Table 3 displays the model performance on the five datasets. Among the three models, LLOS achieved the highest performance across all three evaluation metrics. On the other hand, ARF had the lowest performance in most cases, except for precision in the LR model.
Association analysis
The relationships between the scores of datasets and the performance metrics of the models were analyzed as follows. First, a normality test was conducted on each pair of scores. If the scores passed the normality test, a Pearson correlation analysis was performed. Otherwise, a Spearman correlation analysis was conducted. Table 4 shows that all correlations, except for LR-Precision, were strongly positive and statistically significant. The SVM-Precision correlation showed the strongest effect among them.
We have developed a quantitative evaluation index system to assess the suitability of EMRs in disease risk intelligent prediction research. The proposed index system was validated through an empirical study using MIMIC-III datasets for predicting sepsis. Three popular ML models were performed, and the predictive results demonstrated that datasets with higher scores achieved better performance across three ML models. Our result is consistent with a previous study that showed the impact of data quality on prediction performance [ 34 ]. Additionally, the association analyses revealed a strong positive relationship between the scores of datasets and the combination of the ML model and evaluation metric. These findings confirm that the proposed index system was effective in evaluating the quality of EMR data in disease risk prediction using ML techniques.
Compared to the general framework for evaluating EMR data quality, our proposed index system was constructed by incorporating both the quality characteristics of EMR data and the specific research activities in ML-based disease risk prediction. It differs from the frameworks developed by Johnson [ 35 ] and Lv [ 36 ], which focused on summarizing literature on general medical data rather than specifically on EMR data. Although Weiskopf [ 37 ] specified EMR data as a required condition for a literature search, they did not explicitly address the situation of using EMR data in their development. The proposed index system considers not only the practical foundation of EMR data but also the data processing operations and operational objectives of EMRs at different stages of prediction model construction. This approach makes the evaluation index system more focused on its research purpose and enhances its explanatory power.
Another significant contribution of the proposed index system is the quantitative evaluation of EMR data quality in disease risk prediction. This provides researchers with guidance or standards for quantifying the EMR datasets for specific research purposes. Most current EMR data quality evaluation systems for clinical research rely on qualitative indicators [ 38 ]. Qualitative indicators are often based on typical cases, statements, and supporting materials, which may lack objectivity. While the study of Weiskopf [ 37 ] incorporated quantitative evaluation, it still relied on subjective scoring of each dimension by experts to calculate the mean value. The evaluation model proposed by Zan [ 39 ] utilized objective measurement indicators, but it primarily focused on binary classification and only included first-level indicators.
The proposed three-level index system was developed using a combination of qualitative and quantitative approaches. The naming and definition of all three levels of indicators were constructed through an extensive literature review and expert consultation. The first-level indicators correspond to the core qualitative aspects of evaluating EMR data quality in ML-based disease risk prediction. The second-level indicators serve as a refinement of the first-level qualitative indicators. The third-level indicators are quantitative in nature and can be obtained through objective quantitative calculations, such as assessing the coverage bias of the outcome variables in the integrity of the third-level indicators. Through the AHP method, the weights of the first- and second-level indicators can be obtained by the weights of third-level indicators in a hierarchical way.
Our study has several limitations. First, the calculation of the weights for the third-level indicators was based on simple percentages. This calculation method may neglect variations in the importance of different indicators. Second, the empirical study was conducted using the MIMIC-3 v1.4 database. Although the MIMIC-3 dataset is a widely used resource in research, the use of a single data resource may restrict the generalizability of our findings. Certain indicators for comparing data resources may be hard to validate without diverse EMR data resources. Hence, future validation studies using another data resource should be conducted to ensure the robustness of the proposed index system.
In this paper, we developed a quantitative three-level index system, which included four first-level, 11 second-level, and 33 third-level indicators, to evaluate the EMR data quality in ML-based disease risk prediction. The reliability of the proposed index system has been verified through an empirical study with real-world data.
The proposed index system can benefit both EMR users for research and data managers. For EMR users for research, the proposed index system could provide them with a measurement for the suitability of EMR data in ML-based disease risk predictions. For EMR data managers, it could guide the direction of EMR database construction and improve the EMR data quality control. Eventually, we hope that the proposed index system can promote the generation of real-world evidence from reliable real-world EMR data.
Availability of data and materials
All data generated or analyzed during this study are included in this published article and its additional files.
https://physionet.org/content/mimiciii/1.4/
Abbreviations
Artificial intelligence
Analytic hierarchy process
Acute renal failure
Area under curve
Judgment coefficient
Consistency ratio
Expert authority coefficients
Familiarity coefficient
Computed tomography
Coefficient of variation
- Electronic medical record
Hospital information system
Intensive care units
> 30 Days of length of stay
Logistic regression
- Machine learning
Support vector machine
Random forest
Waldman SA, Terzic A. Healthcare evolves from reactive to proactive. Clin Pharmacol Ther. 2019;105(1):10.
Article PubMed Google Scholar
Razzak MI, Imran M, Xu G. Big data analytics for preventive medicine. Neural Comput Appl. 2020;32:4417–51.
Davenport T, Kalakota R. The potential for artificial intelligence in healthcare. Future Healthc J. 2019;6(2):94–8.
Article PubMed PubMed Central Google Scholar
Dzau VJ, Balatbat CA. Health and societal implications of medical and technological advances. Sci Transl Med. 2018;10(463):eaau4778.
Institute of Medicine. The Computer-Based Patient Record: An Essential Technology for Health Care. Washington DC: National Academy Press; 1997.
Google Scholar
Ambinder EP. Electronic health records. Journal of oncology practice. 2005;1(2):57.
Motwani M, Dey D, Berman DS, et al. Machine learning for prediction of all-cause mortality in patients with suspected coronary artery disease: a 5-year multi centre prospective registry analysis. Eur Heart J. 2017;38(7):500–7.
PubMed Google Scholar
Allyn J, Allou N, Augustin P, et al. A comparison of a machine learning model with EuroSCORE II in predicting mortality after elective cardiac surgery: a decision curve analysis. PLoS ONE. 2017;12(1): e0169772.
Geissbuhler A, Miller RA. Clinical application of the UMLS in a computerized order entry and decision-support system. Proc AMIA Symp. 1998;1998:320–4.
Field D, Sansone SA. A special issue on data standards. OMICS. 2006;10(2):84–93.
Article CAS Google Scholar
Mead CN. Data interchange standards in healthcare IT–computable semantic interoperability: now possible but still difficult, do we really need a better mousetrap? J Healthc Inf Manag. 2006;20(1):71–8.
Reimer AP, Milinovich A, Madigan EA. Data quality assessment framework to assess electronic medical record data for use in research. Int J Med Inform. 2016;90:40–7.
Johnson SG, Speedie S, Simon G, et al. Application of an ontology for characterizing data quality for a secondary use of EHR data. Appl Clin Inform. 2016;7(1):69–88.
Ozonze O, Scott PJ, Hopgood AA. Automating electronic health record data quality assessment. J Med Syst. 2023;47(1):23.
Strasser A. Delphi method variants in IS research: a taxonomy proposal. In: PAC15 2016 Proceedings. 2016. https://aisel.aisnet.org/pacis2016/224 . Accessed 5 July 2023.
Babbie ER. The Practice of Social Research. Mason, OH: CENGAGE Learning Custom Publishing; 2014.
Bryman A. Social Research Methods. London, England: Oxford University Press; 2015.
Ruan Y, Song S, Yin Z, et al. Comprehensive evaluation of military training-induced fatigue among soldiers in China: A Delphi consensus study. Front Public Health. 2022;10:1004910.
Shim JP. Bibliographical research on the analytic hierarchy process (AHP). Socio-Econ Plann Sci. 1989;23(3):161–7.
Article Google Scholar
Ho W. Integrated analytic hierarchy process and its applications–A literature review. Eur J Oper Res. 2008;186(1):211–28.
Lane EF, Verdini WA. A consistency test for AHP decision makers. Decis Sci. 1989;20(3):575–90.
Johnson AE, Pollard TJ, Mark RG. “MIMIC-III clinical database (version 1.4),” PhysioNet. 2016; https://doi.org/10.13026/C2XW26 .
Nusinovici S, Tham YC, Yan MYC, et al. Logistic regression was as good as machine learning for predicting major chronic diseases. J Clin Epidemiol. 2020;122:56–69.
Watanabe T, Kessler D, Scott C, et al. Disease prediction based on functional connectomes using a scalable and spatially-informed support vector machine. Neuroimage. 2014;96:183–202.
Yang L, Wu H, Jin X, et al. Study of cardiovascular disease prediction model based on random forest in eastern China. Sci Rep. 2020;10(1):5245.
Article CAS PubMed PubMed Central Google Scholar
Pedregosa F, Varoquaux G, Gramfort A, et al. Scikit-learn: Machine learning in Python. J Machine Learn Res. 2011;12:2825–30.
Cohen I, Huang Y, Chen J, et al. Pearson correlation coefficient. In: Noise reduction in speech processing. Heidelberg: Springer; 2009:1–4.
Hauke J, Kossowski T. Comparison of values of Pearson’s and Spearman’s correlation coefficients on the same sets of data. Quaestion Geograph. 2011;30(2):87–93.
Johnson AEW, Pollard TJ, Shen L, et al. MIMIC-III, a freely accessible critical care database. Scientific data. 2016;3(1):1–9.
Mayr FB, Yende S, Angus DC. Epidemiology of severe sepsis. Virulence. 2014;5(1):4–11.
Lan P, Wang SJ, Shi QC, et al. Comparison of the predictive value of scoring systems on the prognosis of cirrhotic patients with suspected infection. Medicine. 2018;97(28): e11230.
Lan P, Pan K, Wang S, et al. High serum iron level is associated with increased mortality in patients with sepsis. Sci Rep. 2018;8(1):11072.
Saito T, Rehmsmeier M. Precrec: fast and accurate precision-recall and ROC curve calculations in R. Bioinformatics. 2017;33(1):145–7.
Article CAS PubMed Google Scholar
Ferencek A, Kljajić BM. Data quality assessment in product failure prediction models. J Decis Syst. 2020;29(Suppl 1):79–86.
Johnson SG, Speedie S, Simon G, et al. A data quality ontology for the secondary use of EHR data. AMIA Annu Symp Proc. 2015;2015:1937–46.
PubMed PubMed Central Google Scholar
Tian Q, Chen Y, Han Z, et al. Research on evaluation indexes of clinical data quality. J Med Inform. 2020;41(10):9–17.
Weiskopf NG, Bakken S, Hripcsak G, et al. A data quality assessment guideline for electronic health record data reuse. EGEMS (Wash DC). 2017;5(1):14.
Kahn MG, Callahan TJ, Barnard J, et al. A harmonized data quality assessment terminology and framework for the secondary use of electronic health record data. EGEMS (Wash DC). 2016;4(1):1244.
Cai L, Zhu Y. The challenges of data quality and data quality assessment in the big data era. Data Sci J. 2015;14:2–2.
Download references
Acknowledgements
The authors would like to thank all those who participated in the expert consultation.
This work was supported by the Chinese Academy of Medical Sciences Initiative for Innovative Medicine (Grant No. 2021-I2M-1–057 and Grant No. 2021-I2M-1–056), National Key Research and Development Program of China (Grant No. 2022YFC3601001), and National Social Science Fund of China (Grant No. 21BTQ069).
Author information
Jiayin Zhou and Jie Hao contributed equally to this work.
Authors and Affiliations
Institute of Medical Information, Chinese Academy of Medical Sciences & Peking Union Medical College, Beijing, People’s Republic of China
Jiayin Zhou, Jie Hao, Mingkun Tang, Haixia Sun, Jiao Li & Qing Qian
Chinese Academy of Medical Sciences & Peking Union Medical College, Beijing, People’s Republic of China
Jiayang Wang
You can also search for this author in PubMed Google Scholar
Contributions
All authors contributed to this study. QQ led and designed the study. HS designed the study and structured the manuscript. JZ drafted and revised the manuscript. JH drafted, revised the manuscript, and provided assistance with experiment interpretation. JW and MT conducted the empirical study. JL provided critical revision. All authors read and approved the final manuscript.
Corresponding author
Correspondence to Qing Qian .
Ethics declarations
Ethics approval and consent to participate.
Ethics approval was not deemed necessary for this study by the Ethics Committee at the Institute of Medical Information & Library, Chinese Academy of Medical Sciences, in accordance with national guidelines and local legislation. Written informed consent was obtained from all participants. All methods were performed in accordance with the relevant guidelines and regulations. This study was conducted in compliance with the Declaration of the Helsinki.
Consent for publication
Not applicable.
Competing interests
The authors declare no competing interests.
Additional information
Publisher’s note.
Springer Nature remains neutral with regard to jurisdictional claims in published maps and institutional affiliations.
Supplementary Information
Supplementary material 1., supplementary material 2., supplementary material 3. , rights and permissions.
Open Access This article is licensed under a Creative Commons Attribution 4.0 International License, which permits use, sharing, adaptation, distribution and reproduction in any medium or format, as long as you give appropriate credit to the original author(s) and the source, provide a link to the Creative Commons licence, and indicate if changes were made. The images or other third party material in this article are included in the article's Creative Commons licence, unless indicated otherwise in a credit line to the material. If material is not included in the article's Creative Commons licence and your intended use is not permitted by statutory regulation or exceeds the permitted use, you will need to obtain permission directly from the copyright holder. To view a copy of this licence, visit http://creativecommons.org/licenses/by/4.0/ . The Creative Commons Public Domain Dedication waiver ( http://creativecommons.org/publicdomain/zero/1.0/ ) applies to the data made available in this article, unless otherwise stated in a credit line to the data.
Reprints and permissions
About this article
Cite this article.
Zhou, J., Hao, J., Tang, M. et al. Development of a quantitative index system for evaluating the quality of electronic medical records in disease risk intelligent prediction. BMC Med Inform Decis Mak 24 , 178 (2024). https://doi.org/10.1186/s12911-024-02533-z
Download citation
Received : 05 July 2023
Accepted : 13 May 2024
Published : 24 June 2024
DOI : https://doi.org/10.1186/s12911-024-02533-z
Share this article
Anyone you share the following link with will be able to read this content:
Sorry, a shareable link is not currently available for this article.
Provided by the Springer Nature SharedIt content-sharing initiative
- Quality control
- Data management
- Disease risk prediction
BMC Medical Informatics and Decision Making
ISSN: 1472-6947
- General enquiries: [email protected]
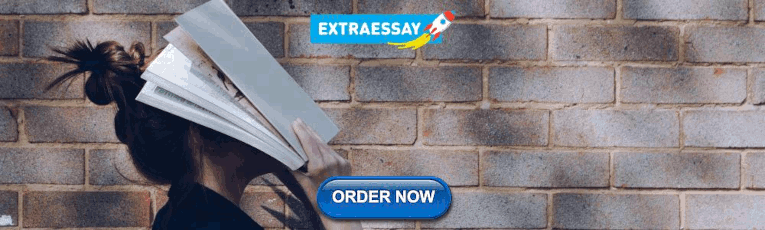
COMMENTS
Quality criteria in qualitative and quantitative research. ... Quality criteria in qualitative and quantitative research Acad Med. 2013 Apr;88(4):552. doi: 10.1097/ACM.0b013e31828abf7f. Authors Janneke M Frambach 1 , Cees P M van der Vleuten, Steven J Durning. Affiliation 1 Maastricht University. PMID ...
However, while qualitative and quantitative research share similar standards for good evidence (quality criteria), the conception and operationalization of these quality criteria differ between the two. Below, we provide an overview of these criteria and a number of techniques that researchers can use to meet them. In
Different quality criteria for qualitative and quantitative research share common principles: trustworthiness, applicability, consistency, and neutrality (Frambach et al., 2013). In qualitative ...
Quality criteria in qualitative and quantitative research. AU - Frambach, Janneke M. AU - van der Vleuten, Cees P M. AU - Durning, Steven J. PY - 2013/4. Y1 - 2013/4. KW - Education, Medical. KW - Humans. KW - Qualitative Research. KW - Research Design. KW - Journal Article.
Quality criteria in qualitative and quantitative research.'. Together they form a unique fingerprint. ... Quality criteria in qualitative and quantitative research. AU - Frambach, Janneke M. AU - van der Vleuten, Cees P.M. AU - Durning, Steven J. PY - 2013/4. Y1 - 2013/4.
Quality criteria in qualitative and quantitative research. ... Quality criteria in qualitative and quantitative research. Frambach JM, van der Vleuten CP, Durning SJ. Author information. Affiliations. All authors. 1. Maastricht University. ... Frambach JM, 0000-0003-1527-6539;
AM Last Page Quality Criteria in Qualitative and Quantitative Research. Frambach, Janneke M. MA, MSc, PhD; student; van der Vleuten, Cees P.M. PhD; Durning, Steven J ...
Quality criteria in qualitative and quantitative research. ... Quality criteria in qualitative and quantitative research.}, author={Janneke M. Frambach and Cees P. M. van der Vleuten and Steven J. Durning}, journal={Academic medicine : journal of the Association of American Medical Colleges}, year={2013}, volume={88 4}, pages={ 552 }, url ...
Use your RU credentials (u/z-number and password) to log in with SURFconext to upload a file for processing by the repository team.
AM Last Page: Quality Criteria in Qualitative and Quantitative Research. Authors. J.M. Frambach; C.P.M. van der Vleuten; S.J. Durning; Publication date 1 January 2013. Publisher. Abstract Item does not contain fulltex. ... Discover our research outputs and cite our work. Follow us:
Within the psychology literature, Elliot, Fischer, and Rennie (1999) proposed seven common guidelines for evaluation of quantitative and qualitative work, as well as seven "evolving" criteria specific to qualitative research. The common criteria address explicating the context, purpose, and worth of the study; using appropriate methods and ...
Fundamental Criteria: General Research Quality. Various researchers have put forward criteria for evaluating qualitative research, which have been summarized in Table 3.Also, the criteria outlined in Table 4 effectively deliver the various approaches to evaluate and assess the quality of qualitative work. The entries in Table 4 are based on Tracy's "Eight big‐tent criteria for excellent ...
methodology of qualitative research. Unfortunately, the qualitative community has faced sev-eral complicating issues that challenge the development of a set of criteria we all support. Numbers-based quantitative research emanating from a positivist paradigm still domi-nates public understandings of what equates with scientific validity (Cheek ...
quality of qualitative research: (1) qualitative research (QUAL) should be judged according to the same criteria as. quantitative research (QUANT); (2) qualitative research (QUAL) should be judged using its own criteria (Lincoln and Guba. 1985); and. ermined criteria for judging qualitative criteria (QUAL)
bility to accommodate various paradigms, approaches, and methods. Method The authors identified guidelines, reporting standards, and critical appraisal criteria for qualitative research by searching PubMed, Web of Science, and Google through July 2013; reviewing the reference lists of retrieved sources; and contacting experts. Specifically, two authors reviewed a sample of sources to generate ...
This overview of quality criteria for the International Exchanges on the Study of Writing book series uses a similar layout and is informed by the article, "AM Last Page: Quality Criteria in Qualitative and Quantitative Research" by Janneke M. Frambach, Cees P. M. van der Vleuten, and Steven J.Durning, which was published in Academic Medicine ...
In general, qualitative data analysis does not seek to quantify data. The results are described in descriptive, and possi. ly in a visual manner, supported with quotes or images from the data. Qualitative data. analyses is also characterized by its cyclical and iterative process. Data analysis often takes place alongside data collection t.
The subgroup on quality criteria for empirical research in social sciences (LGR, GR) had to differentiate the literature search of journals and monographs into general criteria (such as adequacy of the research process, transparency, good scientific and ethical conduct) and specific criteria for quantitative methods (e.g. ), qualitative methods ...
Frambach, J. et al. (2013) AM Last Page: Quality Criteria in Qualitative and Quantitative Research. Academic Medicine, 88, 4, 552. While qualitative and quantitative research share similar standards for good evidence (quality criteria), the conception and operationalization of these quality criteria differ between the two.
Janneke M. Frambach's 40 research works with 755 citations and 7,608 reads, including: Learning in Tension: A Case Study Examining What Internal Medicine Residents Learn in the Ambulatory Care Setting
The mission of the Institute for Excellence in Education (IEE) is to promote, value and advance the educational mission of the School of Medicine while enhancing the school's leadership role in medical and biomedical education. To guide this mission, IEE has identified four guiding principles or "pillars" into which all of our decisions, work ...
AM Last Page: Quality Criteria in Qualitative and Quantitative Research Janneke M. Frambach, MA, MSc, PhD student, Cees P. van der Vleuten, PhD, professor of education, Maastricht University, Steven J. Durning, MD, PhD, professor of medicine and pathology, Uniformed Services University of the Health Sciences. Suggestions for further reading:
This study aimed to develop and validate a quantitative index system for evaluating the data quality of Electronic Medical Records (EMR) in disease risk prediction using Machine Learning (ML). The index system was developed in four steps: (1) a preliminary index system was outlined based on literature review; (2) we utilized the Delphi method to structure the indicators at all levels; (3) the ...