- Corpus ID: 5940305
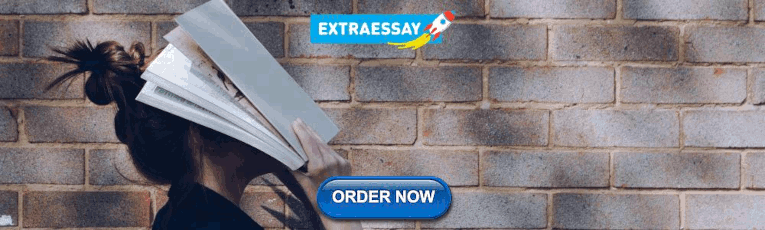
The Gender Similarities Hypothesis
- Janet Shibley
- Published 2005
Figures and Tables from this paper
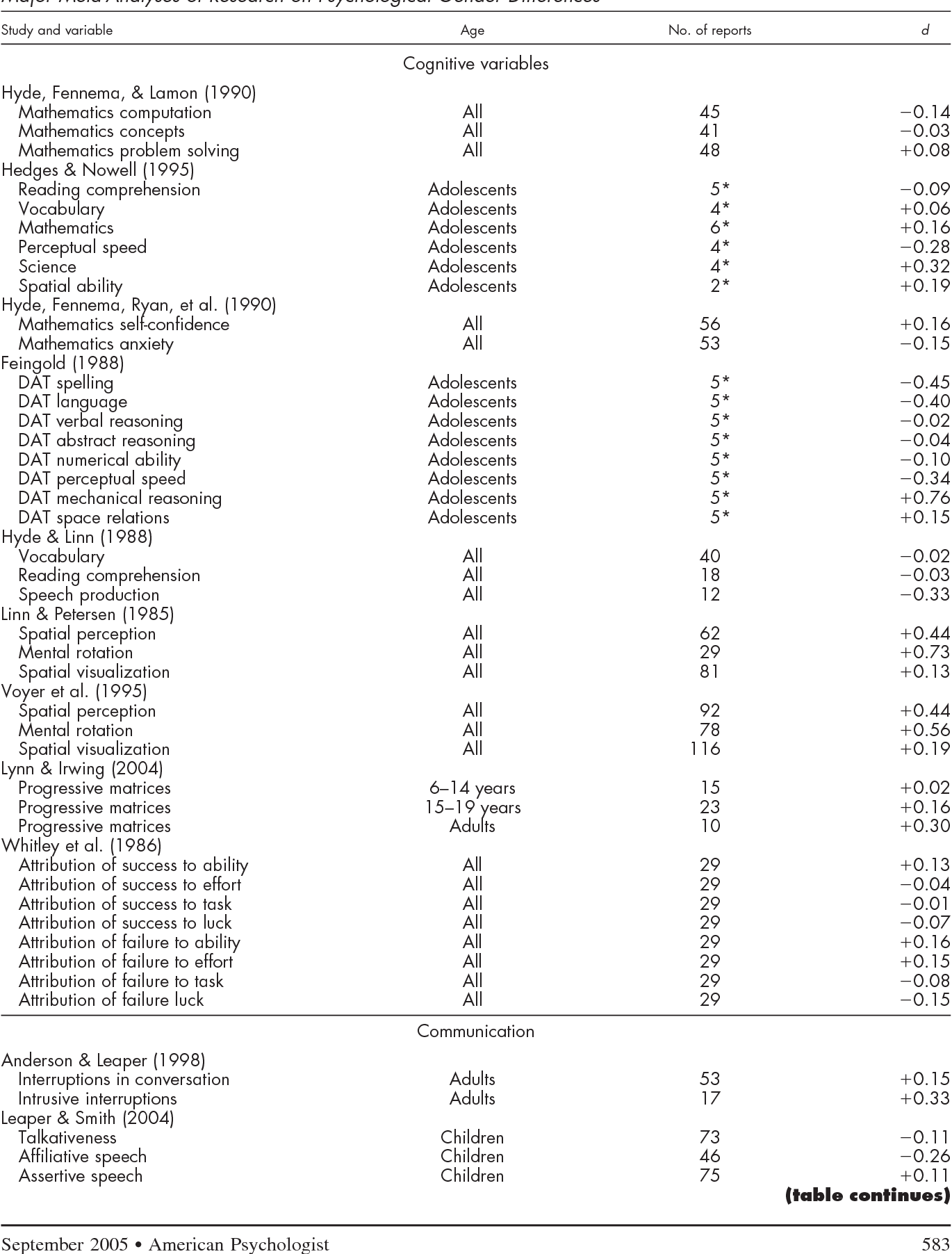
2,512 Citations
New directions in the study of gender similarities and differences.
- Highly Influenced
New Directions in the Study of Gender Similarities and
Gender differences in personality and social behavior, gender and media: content, uses, and impact, gender differences in personality and interests: when, where, and why, the intersection of gender, social class, and cultural context: a meta-analysis, lay beliefs about competition: scale development and gender differences, a feminist review of behavioral economic research on gender differences, what do true gender ratios and stereotype norms really tell us, understanding magnitude of sex/gender differences 1 understanding the magnitude of psychological differences between women and men requires seeing the forest and the trees, 97 references, magnitude of psychological gender differences. another side to the story..
- Highly Influential
Sex differences and similarities in job attribute preferences: a meta-analysis.
Gender differences in sexuality: a meta-analysis., estimating gender differences in the comprehension and preference of moral issues., gender differences in moral orientation: a meta-analysis., gender differences in personality: a meta-analysis., gender differences in computer-related attitudes and behavior: a meta-analysis, emotional arousal and gender differences in aggression: a meta‐analysis, gender and the evaluation of leaders: a meta-analysis., sex differences in aggression in real-world settings: a meta-analytic review.
- 14 Excerpts
Related Papers
Showing 1 through 3 of 0 Related Papers

An official website of the United States government
The .gov means it’s official. Federal government websites often end in .gov or .mil. Before sharing sensitive information, make sure you’re on a federal government site.
The site is secure. The https:// ensures that you are connecting to the official website and that any information you provide is encrypted and transmitted securely.
- Publications
- Account settings
- My Bibliography
- Collections
- Citation manager
Save citation to file
Email citation, add to collections.
- Create a new collection
- Add to an existing collection
Add to My Bibliography
Your saved search, create a file for external citation management software, your rss feed.
- Search in PubMed
- Search in NLM Catalog
- Add to Search
Gender similarities and differences
Affiliation.
- 1 Department of Psychology, University of Wisconsin, Madison, Wisconsin 53706; email: [email protected].
- PMID: 23808917
- DOI: 10.1146/annurev-psych-010213-115057
Whether men and women are fundamentally different or similar has been debated for more than a century. This review summarizes major theories designed to explain gender differences: evolutionary theories, cognitive social learning theory, sociocultural theory, and expectancy-value theory. The gender similarities hypothesis raises the possibility of theorizing gender similarities. Statistical methods for the analysis of gender differences and similarities are reviewed, including effect sizes, meta-analysis, taxometric analysis, and equivalence testing. Then, relying mainly on evidence from meta-analyses, gender differences are reviewed in cognitive performance (e.g., math performance), personality and social behaviors (e.g., temperament, emotions, aggression, and leadership), and psychological well-being. The evidence on gender differences in variance is summarized. The final sections explore applications of intersectionality and directions for future research.
PubMed Disclaimer
Similar articles
- Evaluating gender similarities and differences using metasynthesis. Zell E, Krizan Z, Teeter SR. Zell E, et al. Am Psychol. 2015 Jan;70(1):10-20. doi: 10.1037/a0038208. Am Psychol. 2015. PMID: 25581005
- Sex differences in aggression among children of low and high gender inequality backgrounds: a comparison of gender role and sexual selection theories. Nivette AE, Eisner M, Malti T, Ribeaud D. Nivette AE, et al. Aggress Behav. 2014 Sep-Oct;40(5):451-64. doi: 10.1002/ab.21530. Epub 2014 Feb 13. Aggress Behav. 2014. PMID: 24526324
- Sex differences in cooperation: a meta-analytic review of social dilemmas. Balliet D, Li NP, Macfarlan SJ, Van Vugt M. Balliet D, et al. Psychol Bull. 2011 Nov;137(6):881-909. doi: 10.1037/a0025354. Psychol Bull. 2011. PMID: 21910518 Review.
- The gender similarities hypothesis. Hyde JS. Hyde JS. Am Psychol. 2005 Sep;60(6):581-592. doi: 10.1037/0003-066X.60.6.581. Am Psychol. 2005. PMID: 16173891 Review.
- Gender similarities and differences in children's social behavior: finding personality in contextualized patterns of adaptation. Zakriski AL, Wright JC, Underwood MK. Zakriski AL, et al. J Pers Soc Psychol. 2005 May;88(5):844-55. doi: 10.1037/0022-3514.88.5.844. J Pers Soc Psychol. 2005. PMID: 15898879
- Unveiling gender differences in psychophysiological dynamics: support for a two-dimensional autonomic space approach. Menashri Sinai Y, Ma YXJ, Abba Daleski M, Gannot S, Bartsch RP, Gordon I. Menashri Sinai Y, et al. Front Hum Neurosci. 2024 Mar 13;18:1363891. doi: 10.3389/fnhum.2024.1363891. eCollection 2024. Front Hum Neurosci. 2024. PMID: 38545517 Free PMC article.
- Are many sex/gender differences really power differences? Galinsky AD, Turek A, Agarwal G, Anicich EM, Rucker DD, Bowles HR, Liberman N, Levin C, Magee JC. Galinsky AD, et al. PNAS Nexus. 2024 Feb 27;3(2):pgae025. doi: 10.1093/pnasnexus/pgae025. eCollection 2024 Feb. PNAS Nexus. 2024. PMID: 38415218 Free PMC article.
- Nonverbal cues to deception: insights from a mock crime scenario in a Chinese sample. Li H, Song H, Li M, Li H. Li H, et al. Front Psychol. 2024 Feb 9;15:1331653. doi: 10.3389/fpsyg.2024.1331653. eCollection 2024. Front Psychol. 2024. PMID: 38406306 Free PMC article.
- The relationship between food habits and physical activity and the IQ of primary school children. Khadem A, Nadery M, Noori S, Ghaffarian-Ensaf R, Djazayery A, Movahedi A. Khadem A, et al. J Health Popul Nutr. 2024 Feb 20;43(1):29. doi: 10.1186/s41043-024-00522-6. J Health Popul Nutr. 2024. PMID: 38378710 Free PMC article.
- Naive skepticism scale: development and validation tests applied to the chilean population. Ferrer-Urbina R, Ramírez Y, Mena-Chamorro P, Carmona-Halty M, Sepúlveda-Páez G. Ferrer-Urbina R, et al. Psicol Reflex Crit. 2024 Feb 20;37(1):6. doi: 10.1186/s41155-024-00288-0. Psicol Reflex Crit. 2024. PMID: 38376697 Free PMC article.
Publication types
- Search in MeSH
LinkOut - more resources
Full text sources.
- Ingenta plc
- Ovid Technologies, Inc.
Other Literature Sources
- scite Smart Citations
- Citation Manager
NCBI Literature Resources
MeSH PMC Bookshelf Disclaimer
The PubMed wordmark and PubMed logo are registered trademarks of the U.S. Department of Health and Human Services (HHS). Unauthorized use of these marks is strictly prohibited.
- A-Z Publications
Annual Review of Psychology
Volume 65, 2014, review article, gender similarities and differences.
- Janet Shibley Hyde 1
- View Affiliations Hide Affiliations Affiliations: Department of Psychology, University of Wisconsin, Madison, Wisconsin 53706; email: [email protected]
- Vol. 65:373-398 (Volume publication date January 2014) https://doi.org/10.1146/annurev-psych-010213-115057
- First published as a Review in Advance on June 26, 2013
- © Annual Reviews
Whether men and women are fundamentally different or similar has been debated for more than a century. This review summarizes major theories designed to explain gender differences: evolutionary theories, cognitive social learning theory, sociocultural theory, and expectancy-value theory. The gender similarities hypothesis raises the possibility of theorizing gender similarities. Statistical methods for the analysis of gender differences and similarities are reviewed, including effect sizes, meta-analysis, taxometric analysis, and equivalence testing. Then, relying mainly on evidence from meta-analyses, gender differences are reviewed in cognitive performance (e.g., math performance), personality and social behaviors (e.g., temperament, emotions, aggression, and leadership), and psychological well-being. The evidence on gender differences in variance is summarized. The final sections explore applications of intersectionality and directions for future research.
Article metrics loading...
Full text loading...
Data & Media loading...
- Article Type: Review Article
Most Read This Month
Most cited most cited rss feed, job burnout, executive functions, social cognitive theory: an agentic perspective, on happiness and human potentials: a review of research on hedonic and eudaimonic well-being, sources of method bias in social science research and recommendations on how to control it, mediation analysis, missing data analysis: making it work in the real world, grounded cognition, personality structure: emergence of the five-factor model, motivational beliefs, values, and goals.
Publication Date: 03 Jan 2014
Online Option
Sign in to access your institutional or personal subscription or get immediate access to your online copy - available in PDF and ePub formats
Gender Similarities and Differences
Annual Review of Psychology, Vol. 65, pp. 373-398, 2014
Posted: 8 Jan 2014
Janet Shibley Hyde
University of Wisconsin - Madison - Department of Psychology
Date Written: January 2014
Whether men and women are fundamentally different or similar has been debated for more than a century. This review summarizes major theories designed to explain gender differences: evolutionary theories, cognitive social learning theory, sociocultural theory, and expectancy-value theory. The gender similarities hypothesis raises the possibility of theorizing gender similarities. Statistical methods for the analysis of gender differences and similarities are reviewed, including effect sizes, meta-analysis, taxometric analysis, and equivalence testing. Then, relying mainly on evidence from meta-analyses, gender differences are reviewed in cognitive performance (e.g., math performance), personality and social behaviors (e.g., temperament, emotions, aggression, and leadership), and psychological well-being. The evidence on gender differences in variance is summarized. The final sections explore applications of intersectionality and directions for future research.
Suggested Citation: Suggested Citation
Janet Shibley Hyde (Contact Author)
University of wisconsin - madison - department of psychology ( email ).
Madison, WI 53706 United States
Do you have a job opening that you would like to promote on SSRN?
Paper statistics, related ejournals, behavioral & experimental finance (editor's choice) ejournal.
Subscribe to this free journal for more curated articles on this topic
Psychological Anthropology eJournal
Subscribe to this fee journal for more curated articles on this topic
Feminist Theory & Philosophy eJournal
Social & personality psychology ejournal, social psychology ejournal, sociology of gender ejournal, annual review of psychology, anthropology of kinship, gender, the body & sexuality ejournal.
Thank you for visiting nature.com. You are using a browser version with limited support for CSS. To obtain the best experience, we recommend you use a more up to date browser (or turn off compatibility mode in Internet Explorer). In the meantime, to ensure continued support, we are displaying the site without styles and JavaScript.
- View all journals
- Explore content
- About the journal
- Publish with us
- Sign up for alerts
- Open access
- Published: 08 November 2019
Gender similarities in the brain during mathematics development
- Alyssa J. Kersey ORCID: orcid.org/0000-0001-6685-5982 1 , 2 ,
- Kelsey D. Csumitta ORCID: orcid.org/0000-0001-9488-7981 1 &
- Jessica F. Cantlon ORCID: orcid.org/0000-0003-0207-5615 1 , 3
npj Science of Learning volume 4 , Article number: 19 ( 2019 ) Cite this article
37k Accesses
26 Citations
565 Altmetric
Metrics details
- Human behaviour
- Intelligence
Some scientists and public figures have hypothesized that women and men differ in their pursuit of careers in science, technology, engineering, and mathematics (STEM) owing to biological differences in mathematics aptitude. However, little evidence supports such claims. Some studies of children and adults show gender differences in mathematics performance but in those studies it is impossible to disentangle intrinsic, biological differences from sociocultural influences. To investigate the early biology of mathematics and gender, we tested for gender differences in the neural processes of mathematics in young children. We measured 3–10-year-old children’s neural development with functional magnetic resonance imaging (fMRI) during naturalistic viewing of mathematics education videos. We implemented both frequentist and Bayesian analyses that quantify gender similarities and differences in neural processes. Across all analyses girls and boys showed significant gender similarities in neural functioning, indicating that boys and girls engage the same neural system during mathematics development.
Similar content being viewed by others
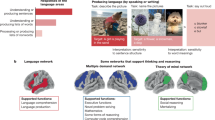
Language is primarily a tool for communication rather than thought
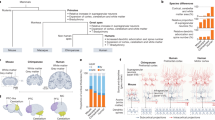
A molecular and cellular perspective on human brain evolution and tempo
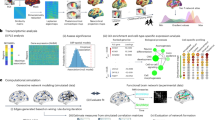
A shifting role of thalamocortical connectivity in the emergence of cortical functional organization
Introduction.
Limited evidence for intrinsic, biological gender differences in mathematics ability has fueled debate about the underrepresentation of girls and women in STEM fields (science, technology, engineering, and mathematics). Some have suggested that girls and women are underrepresented in careers in STEM owing to biological differences. 1 Biological sex differences manifest in aspects of brain function, particularly those related to neuroendocrinology, 2 , 3 , 4 but many measures indicate that neural variability is a continuum wherein the brains of males and females reflect one heterogenous population rather than two distinct groups. 4 In the domain of mathematics, the evidence for biologically based gender differences is weak because when gender differences are observed, the studies fail to differentiate intrinsic biological factors from sociocultural ones. 5 , 6 , 7 , 8 , 9 Moreover, behavioral studies often find no gender differences in mathematical cognition in early childhood, and there are no prior functional neuroimaging studies of biological gender differences in mathematical cognition during early childhood. 5 , 8 , 9 , 10 , 11 , 12 , 13 In order to understand the origins of mathematics ability, and whether there are any gender differences, it is important to ask whether boys and girls begin development with biological differences in mathematical processing. Here, we combine frequentist and Bayesian statistical approaches to test for gender similarities and differences in the neural processing of mathematics during early childhood. We use “gender” instead of “sex” throughout this manuscript, which accords with the relevant literature, 12 , 13 , 14 and because we collected parental report of children’s gender and did not measure their chromosomes.
Although evidence for behavioral gender differences in mathematics is weak in older children, adolescents, and adults, it is important to consider when and how any differences might emerge. One possibility is that despite established gender similarities on behavioral tasks in early childhood, 5 , 10 , 12 , 13 the underlying biological or neural processes could differ between boys and girls. For example, boys’ and girls’ incorrect responses could result from different neural processes (e.g., inefficient recruitment of math processing regions vs inefficient response selection mechanisms). Boys’ and girls’ error rates could yield the same levels on behavioral tests of mathematics but the biology that underlies the errors in each gender group could differ. Alternatively, boys and girls may show significant, widespread biological similarities in the neural processes of mathematics during early childhood. This outcome would be consistent with yet untested claims that boys and girls share a core biology for mathematical cognition.
To compare the neural processes underlying mathematics development, we used functional magnetic resonance imaging (fMRI) to measure neural activity in 3–10-year-old children while they watched video clips that targeted early childhood mathematics skills (e.g., counting, addition; see Methods for more details). In total, 104 children (55 girls) participated in one of three natural viewing tasks (2 published studies 15 , 16 + 1 unpublished study, under review). Data were combined across natural viewing tasks by normalizing each subject to a within-task adult baseline using intersubject correlations. 17 This approach yields 80% power to achieve a medium-effect size of d = 0.55, p < 0.05 for independent samples t tests.
Intersubject correlations were conducted by comparing each child with every other child and comparing each child with every adult within a comparison group (63 total adults, 25 women, who watched one set of video clips). This resulted in an index of ‘neural maturity’ (child-to-adult intersubject correlations) and an index of ‘neural similarity’ (child-to-child intersubject correlations). The majority of analyses focus on similarities and differences in ‘neural maturity’, which indicates how well-developed and adult-like each child’s brain is during mathematical processing. 15 , 16 Neural maturity was calculated by conducting intersubject correlations 17 of the neural timecourses across the entire video between children and adults in every voxel of the brain (see Methods for details). Thus, it assesses the degree to which children’s neural activity resembled that of adults who watched the same video and allows the data to be combined across studies in a meaningful way. Within-child comparisons of neural maturity calculated to women vs men revealed that children’s neural maturity did not statistically differ based on the gender of the adult comparison group (see Methods for details). Therefore, for each child, their measure of neural maturity is averaged across all adults who watched the same video.
Girls’ and boys’ neural maturity were statistically compared across five whole-brain analyses which test for differences in mean neural maturity, similarities in mean neural maturity, differences in variance of neural maturity, and differences in the rate of mathematics development. First, we conducted frequentist statistical tests of differences (two-sided, independent samples t tests) and similarities in neural maturity. Similarities in mean neural maturity were assessed using statistical equivalence statistics. Testing for statistical equivalence is critical for evaluating gender similarities because a null result from a t test only suggests that there is not enough evidence to conclude that a difference exists—to address this we conducted a statistical test of similarity, Schuirmann’s Test of Equivalence. 18 This test uses two one-sided t tests to determine the likelihood that the mean difference between two groups falls within a specified similarity range (consistent with previous work that tested for similarities and differences in SAT scores, we used a similarity range of 2/3 of a standard deviation 19 ). Complementary to this approach, we conducted a Bayes Factor analysis, which also allows for the interpretation of both significant differences and significant similarities. The Bayes Factor analysis weighs the evidence for an alternative hypothesis against the evidence for the null hypothesis by taking the ratio of the posterior probabilities for the two hypotheses (the Bayes Factor). Bayes Factors > 3 indicate substantial, interpretable evidence for the corresponding hypothesis, and Bayes Factors < 3 suggest that the evidence is only anecdotal. Following previous work, 13 the prior for the alternative hypothesis of gender differences was the default Cauchy distribution centered on the prior for the null hypothesis with a width of 0.707. The prior for the null hypothesis was 0. To test for differences in neural variance between boys and girls across the whole brain, we used Levene’s Test of Variance. Some have claimed that differences in the upper and lower tails of the distributions drive gender differences. 20 Variance thus is an important measure because previous work shows that gender differences in variance can exist even when mean performance is the same. 10 , 21 Following the whole-brain analyses, we present more-detailed region-of-interest analyses on regions of the number processing network (bilateral intraparietal sulcus, bilateral inferior frontal gyrus, and anterior cingulate cortex), which were defined from an independent functional localizer scan. In a final analysis, we calculated intersubject correlations across children to obtain measures of within-gender and between-gender neural similarity. We then directly compared children’s neural similarity as calculated with children of the same and of the different gender. If gender differences in neural activity have a biological categorical origin rooted in childhood, these categorical differences should be evident in the brain. In contrast, if gender differences in neural activity do not originate from categorical differences in early childhood, there should instead be widespread similarities.
Frequentist and Bayesian comparisons of neural maturity
We used intersubject correlations to compare girls’ and boys’ temporal patterns of neural activity across the whole brain during the educational videos. First, we compared girls’ and boys’ overall neural maturity using frequentist statistics. A whole-brain t test revealed no differences in neural maturity between groups (threshold = t (102) ≥ 2.36, voxel-wise p ≤ 0.01, cluster corrected to p < 0.05, cluster threshold = 29 voxels). Next, gender similarities were assessed using Schuirmann’s Test of Equivalence. 18 Findings of statistical equivalence would suggest that children’s neural processing of mathematics comprises one heterogenous group rather than two distinct gender groups. In fact, girls and boys showed statistically equivalent levels of neural maturity throughout the brain (Fig. 1a , light purple; minimum t(102) ≥ 2.36, maximum one-sided p ≤ 0.01), suggesting that the neural processing of mathematics develops at similar rates in boys and girls. In terms of differences in neural variance, Levene’s Test of Variance revealed one small region of right posterior parietal cortex where groups differed in variance but not in mean activation, with girls showing greater variance than boys (TAL peak: 18, −64, 43; F(1,102) ≥ 6.89, voxel-wise p ≤ 0.01, cluster corrected to p < 0.05, cluster threshold = 11 voxels, Supplementary Fig. 1 ). This variance cluster was small (15 voxels) and girls exhibited more variance than boys at equal neural amplitudes to boys, which did not result in a mean difference between groups in this region.
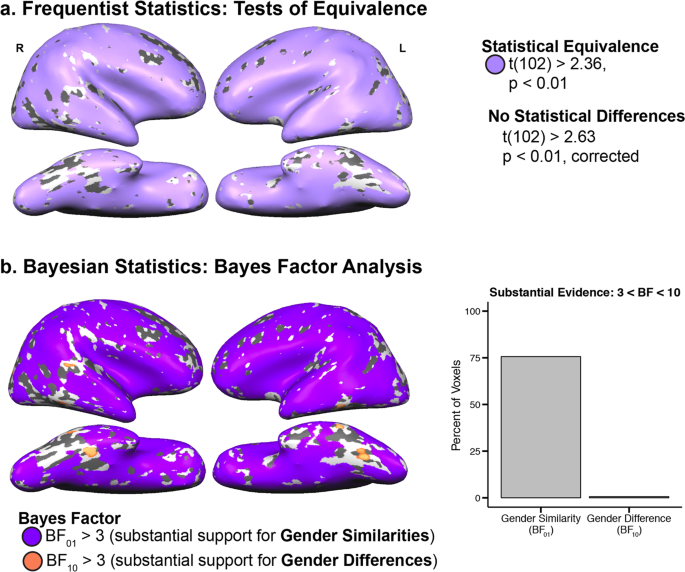
Whole-brain analyses. a Frequentist statistical tests of gender differences and similarities (light purple) of neural maturity. Using frequentist analyses ( t tests), there are no regions showing significant gender differences at the standard threshold. b Whole-brain Bayes Factor analysis showing substantial evidence of gender differences (orange) and gender similarities (dark purple) of neural maturity. The plot to the right shows the percent of voxels across the brain that show substantial support for gender similarities and differences. The regions that show evidence of gender similarities are consistent across frequentist and Bayesian approaches
The pattern of large-scale statistical similarities between boys and girls from the frequentist analyses was replicated in the Bayesian analysis. In each voxel of the brain, the weight of evidence for the null and alternative hypotheses were indexed by the Bayes factor (B 01 for the null hypothesis of gender similarities, B 10 for the alternative hypothesis of gender differences). Bayes factors suggesting that the data provide substantial support of the hypotheses are displayed in Fig. 1b (B 01 > 3, in purple indicating gender similarities, B 10 > 3 in orange indicating greater neural maturity in girls; no cortical regions showed substantial support for greater neural maturity in boys). Less than 1% of voxels (0.8%) showed substantial or strong (B 10 > 10) evidence of gender differences (Fig. 1b ; 23.6% voxels did not substantially support either similarities or differences in the Bayesian analysis).
Similarities in math processing networks
Importantly, across all three natural viewing tasks, children engaged numerical processes in the brain. Children showed number-selective neural activation in the intraparietal sulci (IPS) during the mathematics content in the educational videos (Fig. 2 ) consistent with previous fMRI research on numerical cognition in children and adults. 15 , 16 Boys and girls showed equivalent mathematics-related neural responses (see Fig. 2 ). This is evidence that children engaged mathematical neural processes during the educational videos, and that boys and girls did so equally.

Number and math selectivity in the intraparietal sulci (IPS). Individual data for boys are shown in blue and for girls are shown in red. Error bars represent ±1 standard error of the mean. a Percent signal change for number clips vs non-number clips in Study 1—redrawn data 15 (RIPS: t(25) = 4.01, p = 0.0005, 95% CI = 0.19–0.60, Cohen’s d = 0.79; LIPS: t(25) = 1.51, p = 0.26, 95% CI = −0.09–0.33, Cohen’s d = 0.23). b Preference for math clips vs non-math clips in Study 2—redrawn data 16 (RIPS: t(34) = 6.86, p < 0.0001, 95% CI = 0.12–0.23, Cohen’s d = 1.16; LIPS: t(34) = 4.12, p = 0.0002, 95% CI = 0.05–0.15, Cohen’s d = 0.70). c Slope of % signal change across increasing counting vs alphabet sequences in Study 3 (higher slope = greater sensitivity to sequence; RIPS: t(42) = 3.21, p = 0.003, 95% CI = 0.35–1.56, Cohen’s d = 0.49; LIPS: t(42) = 2.55, p = 0.014, 95% CI = 0.14–1.17, Cohen’s d = 0.39). Independent t tests suggest no differences between girls and boys (max t value = 0.84, min p value = 0.41) and Bayes Factor analyses suggest anecdotal to substantial evidence for gender similarities (BF 10 for gender differences: 0.30–0.47; BF 01 for gender similarities: 2.14–3.25). Although, samples for each individual study are small, effects are consistent with the larger patterns reported in the following analyses
Next, we compared the rate of mathematics development in boys and girls. Ninety-seven children completed the Test of Early Mathematics Ability to evaluate their mathematics skills (TEMA-3 22 ; n = 50 girls, 3.12–8.96 years; 47 boys, 3.33–9.08 years). Math ability was statistically equivalent across children and did not show gender differences in mean ability or variance (Fig. 3a ; t test: t(95) = 0.57, p = 0.57, girls’ mean = 33.62, boys’ mean = 35.96, 95% CI = −10.42–5.74; Tests of Equivalence: t 1 (95) = 3.84, p < 0.001, t 2 (95) = −2.70, p = 0.004; Test of Variance: F(1,95) = 0.29, p = 0.59, girls’ sd = 20.19, boys’ sd = 19.87; descriptively there were more girls than boys in the upper tail of the distribution: 14 girls and 10 boys). Nor did the relation between gender and math ability change across age (Fig. 3a ; Regression of TEMA-3 on gender and age: R 2 = 0.79, F(3,93) = 118.5, p < 0.0001, Gender: b = 1.22, t(93) = 0.163, p = 0.871; Age: b = 11.74, t = 13.97, p < 0.0001; Gender × Age Interaction: b = 0.26, t = 0.21, p = 0.84). These behavioral data were previously included as part of a larger behavioral study that showed no differences and statistical equivalence in math ability in this age group. 12
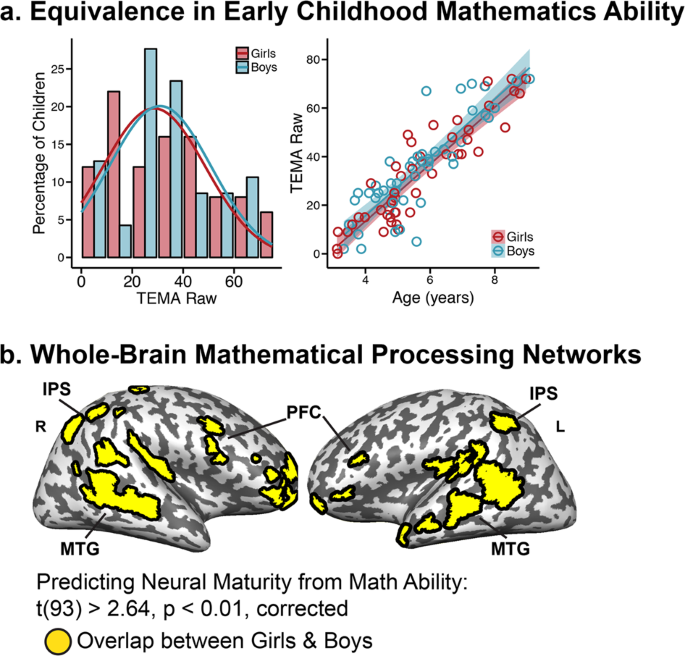
Gender similarities in math ability. a Left: distributions of TEMA-3 scores for girls (red) and boys (blue). Right: TEMA-3 scores increase with age for girls (red) and boys (blue), shaded regions around the line represent ±1 standard error of the mean. b Regions where boys and girls showed a relation between neural maturity and math ability. Importantly, no cortical regions showed an interaction between math ability and gender. Abbreviations: IPS = intraparietal sulcus, PFC = prefrontal cortex, MTG = middle temporal gyrus, R = right, L = left
We then identified mathematical processing networks by testing for regions that showed higher neural maturity in children with stronger math skills. Math ability, gender, and the interaction between math ability and gender were entered as predictors of neural maturity in a whole-brain regression. This regression revealed that math ability predicted neural maturity in both gender groups in the IPS, prefrontal cortex, and middle temporal gyrus (Fig. 3b ; t(93) ≥ 2.63 voxel-wise p ≤ 0.01, cluster corrected to p < 0.05, girls: r(48) ≥ 0.36 with cluster threshold of 109 voxels, boys: r(45) ≥ 0.37 with cluster threshold of 69 voxels, see Supplementary Fig. 2 for separate maps). These regions are consistent with those that show a correlation between neural maturity and math ability when collapsed across gender (r(95) ≥ 0.26, p ≤ 0.01, corrected, Supplementary Fig. 2 ). No cortical regions showed significant interactions between math ability and gender (t(93) ≤ 2.63, threshold: voxel-wise p ≤ 0.01, cluster corrected to p < 0.05, threshold = 110 voxels), indicating that the relation between math ability and neural maturity does not depend on gender. In other words, mathematical processing networks develop at the same rate for girls and boys.
To visualize patterns of gender similarities, neural maturity was extracted from an independently-defined number processing network 15 consisting of bilateral IPS, bilateral inferior frontal gyrus, and anterior cingulate cortex (number > face, shape, and word matching; Fig. 4a ; t(17) ≥ 4.04, FDR corrected to p < 0.05). In accord with the whole-brain analyses, these regions showed statistical equivalence, not statistical differences, and no differences in variance (Fig. 4b ; t tests: max t(102) = 1.08, p = 0.28; equivalence-tests: min t 1 (102) = 3.82, p = 0.0001; min absolute value of t 2 (102) = 2.33, p = 0.01; see Supplementary Table 1 for full statistics). Regression analyses revealed that math ability predicted neural maturity throughout the number processing network, particularly in the IPS, but did not interact with gender (interaction predictors: max t(93) = 1.42, p = 0.16, Supplementary Table 1 ). This shows that within key number processing regions of the brain, girls and boys show the same degree of development in mathematical processing.
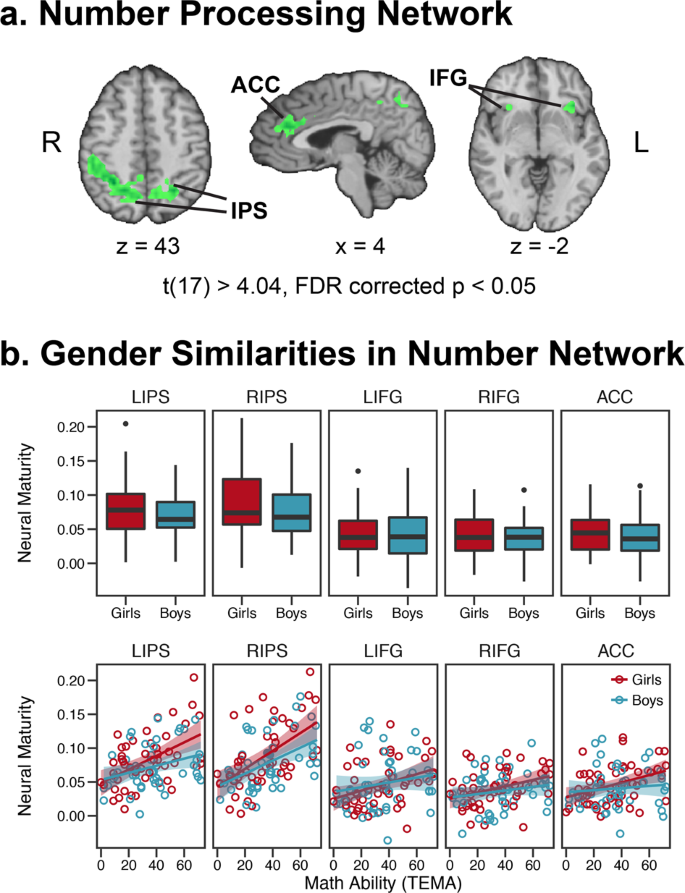
Region-of-interest analyses. a Number processing network identified from an independent localizer (number matching > face, shape, & word matching). b Mean neural maturity in the number network (top) and relation between neural maturity and math ability in the number network (bottom). Boxplot center line identifies the median, the upper whiskers extend from the 75th percentile to the 75th percentile + 1.5 interquartile range, the lower whiskers extend from the 25th percentile to the 25th–1.5 interquartile range. Outliers are those data points beyond the whisker ranges. Shaded regions around the lines in the scatterplots indicate ±1 standard error. Abbreviations: IPS = intraparietal sulcus, ACC = anterior cingulate cortex, IFG = inferior frontal gyrus, R = right, L = left
Whole-brain child-to-child similarity
Finally, we examined neural similarity in children of the same versus different genders. Intersubject correlations were calculated between children, resulting in maps of same-gender neural similarity (comparing girls with girls and boys with boys) and different-gender neural similarity (comparing girls with boys and boys with girls). To determine whether there were differences between same-gender versus different-gender similarity, each child’s whole-brain different-gender similarity map was subtracted from their same-gender similarity map. These difference maps were then subjected to a one-sample t test vs 0. This whole-brain t test revealed no regions that showed a difference in neural activity (threshold: t(103) ≥ 2.62, voxel-wise p ≤ 0.01, corrected to p < 0.05 with a cluster threshold of 19 voxels). Figure 5 shows average neural similarity calculated to children of the same gender (yellow), a different gender (dark green), and the overlap of those maps (light green; r(500) ≥ 0.115, p ≤ 0.01). The regions that showed strong neural similarity between children were identical for statistical comparisons of the same gender and different genders. This again indicates that children’s patterns of neural activity reflect one heterogenous group, rather than two distinct groups based on gender.
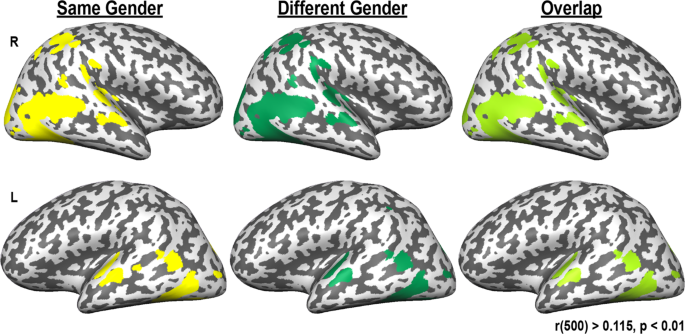
Child-to-child neural saimilarity. Average neural similarity (r(500) ≥ 0.115, p ≤ 0.01) when calculated across children of the same gender (yellow, column 1) and children of different genders (green, column 2). The third column shows the overlap of the first two columns in light green. Importantly, regions are identical across neural similarity calculated to children of the same gender and to children of different genders
Across multiple neural analyses, we show that girls’ and boys’ brains function similarly during mathematical processing. We saw no evidence of gender differences in neural responses to mathematics content, neural responses during educational video viewing, or rates of neural development for mathematical processing in early childhood, and in fact we found statistical equivalence between boys and girls throughout the brain. Tests of statistical equivalence and a Bayes Factor analysis show gender similarities throughout the number processing network. Furthermore, boys’ and girls’ math abilities related to the rate of neural mathematics development in the same brain regions, and neural similarity was consistent across children of the same and of different genders.
Our results are consistent with the ‘Gender Similarities Hypothesis’, which argues that boys and girls function similarly in most areas of cognition. 10 , 23 In particular, gender similarities in early childhood mathematics show, as proposed in the domain of spatial cognition, 14 that gender differences in STEM fields in adults are not derived from intrinsic differences in children’s brains but likely from a complex environmental origin.
Any test of cognitive ability that shows gender differences faces the difficulty of disentangling biological factors from social ones. For instance, 4- to 7-year-old boys show an advantage over girls in tests of spatial skills, but parents also report more-spatial play with their boys compared with their girls, 14 suggesting a possible sociocultural influence on gender differences in spatial cognition. Similarly, in math and science, teachers tend to show differential distributions of time spent encouraging students, praising students, and explaining concepts to students, with boys receiving more time than girls. 24 , 25 , 26 , 27 This is important because teachers’ perceptions of children’s math ability predicts later math achievement scores. 28 Parents’ expectations about their children’s success also correlate with children’s own self-concepts of their abilities and their performance on math tasks. 29 , 30 A strong sociocultural influence on early childhood math achievement makes it difficult to tease apart intrinsic gender differences from sociocultural factors in older children and adults. 8 , 9
Given the broad similarities between boys and girls, gender differences observed in STEM performance during adolescence or adulthood are unlikely to originate from early childhood differences in the brain or cognition. Although gender differences in STEM may emerge later in development or from interactions between STEM training and sexually dimorphic behaviors (e.g., differences in hormone levels following puberty), 6 , 31 , 32 the findings of widespread gender similarities in boys’ and girls’ brains do not support claims of biological gender differences in childhood. Instead, the data show that the neural functions underlying mathematical cognition are similar between genders and represent one heterogeneous population rather than two categorical groups.
Participants
In total, 104 typically-developing 3- to 10-year-old children (55 girls) and 63 adults (35 women) participated in one of three studies. Age was statistically equivalent between girls and boys (t 1 (102) = 3.32, p = 0.0006, t 2 (102) = 3.43, p = 0.0004), and there were no differences in age variability, reflecting an even distribution of age across-gender groups (F(1,102) = 0.07, p = 0.79, girls’ sd = 1.65, boys’ sd = 1.63). Informed written consent was obtained from adult participants and parents of children, and informed written assent was obtained for children 7 years and older. All protocols were approved by the University of Rochester Research Subjects Review Board.
fMRI paradigms
Twenty-six 4- to 10-year-old children (15 girls; girls’ mean age = 6.93 years, boys’ mean age = 7.13 years; range = 4.32–10.80 years) and 20 adults (13 women; women’s mean age = 20.52 years, men’s mean age = 20.98 years; range = 18.9–25.4 years) successfully participated in Study 1. This paradigm consisted of a 20.3-min video containing clips from children’s educational television shows. Clips ranged from 12 to 176 s in length and were edited into a continuous movie. These data have been previously reported 15 as the “natural viewing” task.
Thirty-five 4- to 8-year-old children (17 girls; girls’ mean age = 6.61 years, boys’ mean age = 6.35 years; range = 4.08–8.67 years) and 23 adults (12 women; women’s mean age = 22.13 years, men’s mean age = 22.65 years; range = 18.44–28.09 years) successfully participated in Study 2. The 11.6-min video contained clips from children’s educational television shows. Clips ranged from 12.5 to 32.4 s in length and were edited into a continuous movie. These data have been previously reported 16 as the “natural viewing” task.
Forty-three 3- to 5-year-old children (23 girls; girls’ mean age = 4.54 years, boys’ mean age = 4.71 years; range = 3.12–5.96 years) and 20 adults (10 women; women’s mean age = 23.43 years, men’s mean age = 24.17 years; range = 20.15–31.55 years) successfully participated in Study 3. In this study, participants listened to pre-recorded audio tracks of someone counting or saying the alphabet. Short clips from child-friendly cartoons were presented on the screen during the sequences. Audio tracks were removed from the cartoons and were replaced with quieter, child-friendly instrumental music. Cartoon tracks were matched across sequences. Sequences were presented in 70 s blocks of 60 items presented at a rate of one item every 1.1–1.2 s. Fifteen blocks were presented throughout the experimental paradigm and were separated by 4-s of blackscreen. The scan began and ended with 12 s of blackscreen resulting in a total scan time of 19.2 min.
Number Localizer
Eighteen children from Study 1 (11 girls, 7 boys; girls’ mean age = 6.78 years, boys’ mean age = 7.47 years; range = 4.32–10.8 years) completed a traditional fMRI paradigm in which they compared pairs of stimuli (isolated images of faces, numbers, words or shapes) that were presented on the left and right sides of the screen. Participants reported whether they were the same or different by pressing a button only if the two stimuli matched. Half of the pairs presented were “matches”, whereas the other half were “non-matches”. Number comparisons were made across notation (i.e., dot array compared with Arabic numeral), face comparisons were made across a frontal shot and an oblique view, word comparisons were made across a word in all capital letters and the other in all lowercase letters, and shape comparisons were made across two shape images. Stimuli were presented in a blocked design. Each block consisted of three 2-s trials from the same condition, separated by a 2-s intertrial interval. Three blocks of each condition were semi-randomly presented throughout a run with 8 s of fixation between blocks. These data have been previously reported 15 as the “traditional functional task”.
fMRI session
Prior to the scanning sessions, children participated in a 30-minute training session in a mock scanner to familiarize them with the scanning environment and to practice remaining motionless. Children who completed the Number Localizer paradigm practiced the task prior to the MRI session. Adults received verbal instructions prior to scanning and did not participate in a training session. During the scan, children’s heads were secured with medical tape, headphones, and foam padding, and adults’ heads were secured with headphones and foam padding.
MR parameters
Whole-brain BOLD imaging was conducted on a 3-Tesla Siemens MAGNETOM Trio scanner with a 12-channel head coil at the Rochester Center for Brain Imaging. High-resolution structural T1 contrast images were acquired using a magnetization prepared rapid gradient echo pulse sequence at the start of each session (repetition time (TR) = 2530 ms, echo time (TE) = 3.44 ms, flip angle = 7, field of view (FOV) = 256 mm, matrix = 256 × 256, 192, 176, or 160 slices depending on head size, 1 × 1 × 1 mm sagittal left-to-right slices). An echo-planar imaging pulse sequence with online motion correction was used for T2*contrast (TR = 2000 ms, TE = 30 ms, flip angle = 90 degrees, FOV = 256 mm, matrix 64 × 64, 30 axial oblique slices, parallel to the AC-PC plane, voxel size = 4 × 4 × 4 mm). The primary paradigms from Studies 1, 2, and 3 were 610 volumes, 348 volumes, and 567 volumes, respectively, and the Number Localizer paradigm was distributed over two to four functional runs of 132 volumes each.
Preprocessing
fMRI data were analyzed in BrainVoyager 33 using in-house scripts drawing on the BVQX toolbox. Data from previously published studies were analyzed as originally reported for consistency. 15 , 16 For the Number Localizer and Study 1, which were collected during the same scanning session, the first six TRs of each run were discarded prior to analysis to allow for signal equilibration. For Studies 2 and 3, the first two TRs of each run were discarded. Functional data were registered to high-resolution anatomy images for each participant in native space. Preprocessing consisted of slice scan time corrected (cubic spline interpolation), motion correction with respect to the first volume in the run, and linear trend removal in the temporal domain (cutoff: two cycles within the run). A Gaussian spatial filter with an 8 mm full-width at half-maximum was applied to each volume for Study 1, 15 and a 6 mm full-width at half-maximum was applied to each volume for Studies 2 16 and 3. The functional data from the Number Localizer were not smoothed. Adult and child echo-planar and anatomical volumes were then normalized into Talairach space 34 using piecewise affine transformation based on manual identification of anatomical landmarks. Analyses were performed on processed data in Talairach space. Average framewise displacement 35 , 36 was regressed across the brain for each child to control for sudden changes in volume-to-volume head motion.
fMRI data analyses
Neural maturity: neural data were analyzed using an intersubject correlation approach. 15 , 16 , 17 Between-group intersubject correlations were performed by using the full timecourse of each voxel for each child as a predictor for activation of the corresponding voxel in each adult brain. Functional data from each child were then correlated with that of each adult from the same study to produce paired r-maps. Paired r-maps represent the neural similarity of each child compared with each adult in every voxel of the brain. A single, average brain map was then calculated to represent the neural similarity of each child to all adults. This map is referred to as a map of “neural maturity” because it shows how “adult-like” each child’s neural timecourse appears. To ensure that these similarity maps were not confounded by any adult gender differences prior to averaging, we compared neural maturity calculated to women vs men. For each child, we conducted an Independent Samples t -test to compare neural maturity when calculated to adults of the same vs different gender as the child. This resulted in a map of between-group t values for each child. To determine whether there were any differences that were consistent at the group level, the absolute value of these individual-level between-group t value maps were then subjected to a one-sample t test vs a critical t value of 2.08. The critical value represents the t value at which a difference could be considered significant given the smallest sample of adults ( n = 20 for studies 1 and 3; t(18) = 2.08, p = 0.05). If there were a significant difference between neural maturity calculated to adults of the same vs different gender as the child, the one-sample t test should reveal a positive and significant effect, which would indicate that the absolute values of the individual-level between-group t values are significantly above 2.08. Instead, the whole-brain one-sample t test revealed that at the group level, the absolute values of the individual-level between-group t values were significantly below the critical t value of 2.08 (one-sample t test of absolute value of individual-level between-group t values vs critical t value of 2.08: t(102) ≤ −2.62, p ≤ 0.01). In other words, the majority of individual-level between-group t values were less than the critical t value of 2.08 (range of average absolute value of individual-level between-group t values across voxels: 0–1.90). This indicates that overall, the differences between neural maturity when calculated to adults of the same vs different gender were not different, so neural maturity was not biased by the gender of the adult.
Neural similarity
To compare how similar children were to each other, intersubject correlations were calculated across children following the same procedures as for calculating neural maturity. This resulted in two maps for each child: one representing the average neural similarity of a child to children of the same gender and one representing the average neural similarity of a child to children of the different gender. To compare neural similarity within and across gender, these maps were then subtracted from each other (within-gender similarity−across-gender similarity) and these difference maps were subjected to a one-sample t test vs 0.
Functional data collected during the traditional fMRI paradigm were analyzed using a general linear model (random effects analysis). Experimental events (duration = 10 s) were convolved with a standard dual gamma hemodynamic response function. There were four regressors of interest (corresponding to the four stimulus categories), one regressor for button press, and six regressors of no interest (corresponding to the motion parameters obtained during preprocessing).
Region-of-interest (ROI) analyses
Data were extracted from number network ROIs using MATLAB. Analyses were then conducted using R (version 3.3.1) and R-Studio (version 0.99.902). Independent samples t tests were conducted using the “ t test” function assuming equal variance. Tests of equivalence were conducted using the “TOSTtwo.raw” function from the “TOSTER” package (upper and lower bounds set to ±0.667 × standard deviation of the entire group; alpha = 0.05). This function returns two t values ( t 1 and t 2 ). For statistical equivalence, both t values must be statistically significant. Statistical equivalence is rejected if either t 1 or t 2 does not reach significance. Levene’s Test of Variance was carried out using the “leveneTest” function in the “car” package. Regression analyses were conducted using the built-in “lm” function.
Assessment of math ability
Math skills were evaluated by administering the TEMA-3 22 to participants aged 8 and younger. The TEMA-3 tests a variety of math concepts and is standardized for 3–8-year-old children.
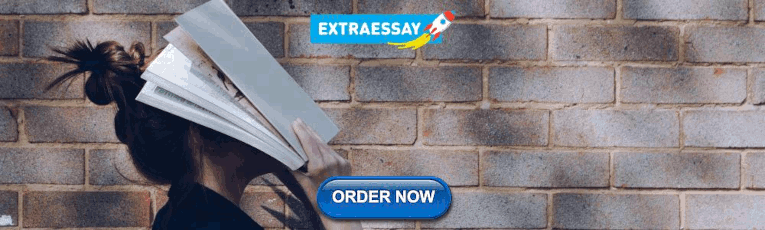
Reporting summary
Further information on research design is available in the Nature Research Reporting Summary linked to this article.
Data availability
These data sets are available from the corresponding author on reasonable request.
Code availability
All code for R and MATLAB are available from the corresponding author on reasonable request.
Levy, J. & Kimura, D. Women, men and the sciences. in The Science on Women and Science (ed. Sommers, C. H.) 202–284 (American Enterprise Institute Washington, 2009).
Sowell, E. R. et al. Sex differences in cortical thickness mapped in 176 healthy individuals between 7 and 87 years of age. Cereb. Cortex 17 , 1550–1560 (2007).
Article Google Scholar
Giedd, J. N., Raznahan, A., Mills, K. L. & Lenroot, R. K. Review: magnetic resonance imaging of male/female differences in human adolescent brain anatomy. Biol. Sex Differ. 3 , 19 (2012).
Joel, D. & Fausto-Sterling, A. Beyond sex differences: New approaches for thinking about variation in brain structure and function. Philos. Trans. R. Soc. B Biol. Sci. 371 , 20150451 (2016).
Spelke, E. S. & Ellison, K. Gender, math and science. in The Science on Women and Science (ed. Sommers, C. H.) 24–53 (American Enterprise Institute Washington, 2009).
Halpern, D. F. et al. The science of sex differences in science and mathematics. Psychol. Sci. Public Interes. 8 , 1–51 (2007).
Hyde, J. S., Fennema, E. & Lamon, S. J. Gender differences in mathematics performance - a metaanalysis. Psychol. Bull. 107 , 139–155 (1990).
Article CAS Google Scholar
Keller, K. & Menon, V. Gender differences in the functional and structural neuroanatomy of mathematical cognition. Neuroimage 47 , 342–352 (2009).
Pletzer, B. Sex differences in number processing: Differential systems for subtraction and multiplication were confirmed in men, but not in women. Sci. Rep. 6 , 36904 (2016).
Hyde, J. S., Lindberg, S. M., Linn, M. C., Ellis, A. B. & Williams, C. C. Gender similarities characterize math performance. Science 321 , 494–495 (2008).
Lindberg, S. M., Hyde, J. S., Petersen, J. L. & Linn, M. C. New trends in gender and mathematics performance: a meta-analysis. Psychol. Bull. 136 , 1123–1135 (2010).
Kersey, A. J., Braham, E. J., Csumitta, K. D., Libertus, M. E. & Cantlon, J. F. No intrinsic gender differences in children’s earliest numerical abilities. npj Sci. Learn. 3 , 12 (2018).
Hutchison, J. E., Lyons, I. M. & Ansari, D. More similar than different: gender differences in children’s basic numerical skills are the exception not the rule. Child Dev. 00 , 1–14 (2018).
Google Scholar
Jirout, J. J. & Newcombe, N. S. Building blocks for developing spatial skills: evidence from a large, representative U.S. sample. Psychol. Sci. 26 , 302–310 (2015).
Cantlon, J. F. & Li, R. Neural activity during natural viewing of Sesame Street statistically predicts test scores in early childhood. PLoS Biol. 11 , e1001462 (2013).
Kersey, A. J., Wakim, K.-M., Li, R. & Cantlon, J. F. Developing, mature, and unique functions of the child’s brain in reading and mathematics. Dev. Cogn. Neurosci. 39 , 100684 (2019).
Hasson, U., Nir, Y., Levy, I., Fuhrmann, G. & Malach, R. Intersubject synchronization of cortical activity during natural vision. Science 303 , 1634–1640 (2004).
Schuirmann, D. J. A comparison of the two one-sided tests procedure and the powers approach for assessing the equivalence of average bioavailability. J. Pharmacokinet. Biopharm. 15 , 657–680 (1987).
Ball, L. C., Cribbie, R. A. & Steele, J. R. Beyond gender differences: using tests of equivalence to evaluate gender similarities. Psychol. Women Q. 37 , 147–154 (2013).
Hedges, L. & Nowell, A. Sex differences in mental test scores, variability, and numbers of high-scoring individuals. Science 269 , 41–45 (1995).
Benbow, C. P. & Stanley, J. C. Sex differences in mathematical reasoning ability: more facts. Science 22 , 1029–1031 (1983).
Ginsburg, H. & Baroody, A. J. Test of Early Mathematics Ability , 3rd Edn (TEMA-3) . (Pro-Ed, 2003).
Hyde, J. S. The gender similarities hypothesis. Am. Psychol. 60 , 581–592 (2005).
American Association of University Women Educational Foundation. How Schools Shortchange Girls. AAUW Rep . 1–13 (1992).
Jones, M. G. & Wheatley, J. Gender differences in teacher-student interactions in science classrooms. J. Res. Sci. Teach. 27 , 861–874 (1990).
Kelly, S. N. Teachers’ useful skills. J. Res. Music Educ. 46 , 374–383 (1998).
Becker, J. R. Differential treatment of females and males in mathematics classes. J. Res. Math. Educ. 12 , 40–53 (1981).
Jussim, L. & Eccles, J. S. Teacher expectations II: constructions and reflection of student achievement. J. Pers. Soc. Psychol. 63 , 947–961 (1992).
Bleeker, M. M. & Jacobs, J. E. Achievement in math and science: do mothers’ beliefs matter 12 years later? J. Educ. Psychol. 96 , 97–109 (2004).
Parsons, J. E., Adler, T. F. & Kaczala, C. M. Socialization of achievement attitudes and beliefs: parental influences. Child Dev. 53 , 310–321 (1982).
Geary, D. C. A model for representing gender differences in the pattern of cognitive abilities. Am. Psychol. 44 , 1155–1156 (1989).
Arnold, A. P. et al. Minireview: sex chromosomes and brain sexual differentiation. Endocrinology 145 , 1057–1062 (2004).
Goebel, R., Esposito, F. & Formisano, E. Analysis of functional image analysis contest (FIAC) data with brainvoyager QX: From single-subject to cortically aligned group general linear model analysis and self-organizing group independent component analysis. Hum. Brain Mapp. 27 , 392–401 (2006).
Talairach, J. & Tournoux, P. Co-Planar Stereotaxic Atlas of the Human Brain . (Thieme, 1988).
Grill-Spector, K., Golarai, G. & Gabrieli, J. Developmental neuroimaging of the human ventral visual cortex. Trends Cogn. Sci. 12 , 152–162 (2008).
Power, J. D., Barnes, K. A., Snyder, A. Z., Schlaggar, B. L. & Petersen, S. E. Spurious but systematic correlations in functional connectivity MRI networks arise from subject motion. Neuroimage 59 , 2142–2154 (2012).
Download references
Acknowledgements
We thank Rosa Li, Courtney Lussier, and Pat Weber for help with data collection and Rosa Li and Kamy Wakim for help with data processing. This work was supported by the National Science Foundation (DRL-1459625 to J.F.C. and DGE- 1419118 to A.J.K.) and the National Institutes of Health (R01 HD064636 to J.F.C).
Author information
Authors and affiliations.
Department of Brain and Cognitive Sciences, University of Rochester, Rochester, NY, 14627, USA
Alyssa J. Kersey, Kelsey D. Csumitta & Jessica F. Cantlon
Department of Psychology, University of Chicago, Chicago, IL, 60637, USA
Alyssa J. Kersey
Department of Psychology, Carnegie Mellon University, Pittsburgh, PA, 15213, USA
Jessica F. Cantlon
You can also search for this author in PubMed Google Scholar
Contributions
All authors designed the research, analyzed the data, and revised and approved the manuscript.
Corresponding author
Correspondence to Alyssa J. Kersey .
Ethics declarations
Competing interests.
The authors declare no competing interests.
Additional information
Publisher’s note Springer Nature remains neutral with regard to jurisdictional claims in published maps and institutional affiliations.
Supplementary information
Kerseycsumittacantlon_supplementalmaterial, reporting summary, rights and permissions.
Open Access This article is licensed under a Creative Commons Attribution 4.0 International License, which permits use, sharing, adaptation, distribution and reproduction in any medium or format, as long as you give appropriate credit to the original author(s) and the source, provide a link to the Creative Commons license, and indicate if changes were made. The images or other third party material in this article are included in the article’s Creative Commons license, unless indicated otherwise in a credit line to the material. If material is not included in the article’s Creative Commons license and your intended use is not permitted by statutory regulation or exceeds the permitted use, you will need to obtain permission directly from the copyright holder. To view a copy of this license, visit http://creativecommons.org/licenses/by/4.0/ .
Reprints and permissions
About this article
Cite this article.
Kersey, A.J., Csumitta, K.D. & Cantlon, J.F. Gender similarities in the brain during mathematics development. npj Sci. Learn. 4 , 19 (2019). https://doi.org/10.1038/s41539-019-0057-x
Download citation
Received : 27 March 2019
Accepted : 11 September 2019
Published : 08 November 2019
DOI : https://doi.org/10.1038/s41539-019-0057-x
Share this article
Anyone you share the following link with will be able to read this content:
Sorry, a shareable link is not currently available for this article.
Provided by the Springer Nature SharedIt content-sharing initiative
This article is cited by
The conundrum of gender-science stereotypes: a review and discussion of measurements.
- Elena De Gioannis
Quality & Quantity (2023)
The Representation of Gender Stereotypes in Spanish Mathematics Textbooks for Elementary Education
- Virginia Guichot-Reina
- Ana María De la Torre-Sierra
Sexuality & Culture (2023)
On the association between gender-science stereotypes’ endorsement and gender bias attribution
Implicit gender-science stereotypes and college-major intentions of italian adolescents.
Social Psychology of Education (2022)
The effect of language on performance: do gendered languages fail women in maths?
- Tamar Kricheli-Katz
npj Science of Learning (2021)
Quick links
- Explore articles by subject
- Guide to authors
- Editorial policies
Sign up for the Nature Briefing newsletter — what matters in science, free to your inbox daily.


The gender similarities hypothesis.
Chat with Paper: Save time, read 10X faster with AI
Content maybe subject to copyright Report
Visible Learning: A Synthesis of Over 800 Meta-Analyses Relating to Achievement
Bayesian t tests for accepting and rejecting the null hypothesis, intersectionality and research in psychology, default bayes factors for anova designs, cross-national patterns of gender differences in mathematics:, statistical power analysis for the behavioral sciences, statistical power analysis for the behavioral sciences (2nd ed.), in a different voice: psychological theory and women's development, statistical methods for meta-analysis, stereotype threat and the intellectual test performance of african americans, related papers (5), the psychology of sex differences, magnitude of sex differences in spatial abilities: a meta-analysis and consideration of critical variables., the measurement of psychological androgyny., gender differences in personality: a meta-analysis..
USU Home A-Z Index

- Author Gallery
- < Previous
Home > Research Centers > Gender Equity and Diversity > ADVANCE > 142
ADVANCE Library Collection
The gender similarities hypothesis.
Janet Shibley Hyde
Document Type
Journal/book title/conference.
American Psychologist
American Psychological Association
Publication Date
The differences model, which argues that males and females are vastly different psychologically, dominates the popular media. Here, the author advances a very different view, the gender similarities hypothesis, which holds that males and females are similar on most, but not all, psychological variables. Results from a review of 46 metaanalyses support the gender similarities hypothesis. Gender differences can vary substantially in magnitude at different ages and depend on the context in which measurement occurs. Overinflated claims of gender differences carry substantial costs in areas such as the workplace and relationships.
Originally published by the American Psychological Association . Publisher's PDF available through remote link.
Recommended Citation
Hyde, Janet Shibley, "The Gender Similarities Hypothesis" (2005). ADVANCE Library Collection. Paper 142. https://digitalcommons.usu.edu/advance/142
Since March 05, 2010
https://doi.org/10.1037/0003-066X.60.6.581
Advanced Search
- Notify me via email or RSS
- Departments
- Research Centers
- Conferences and Events
- Disciplines
- Student Work
- Open Educational Resources
For Authors
- Author Dashboard
- Expert Gallery
- Open Access
Scholarly Communication
- (435) 797-0816
Research Data
- 435-797-2632
- Research Data Management Services @ USU
Home | About | FAQ | My Account | Accessibility Statement | Privacy Policy | Copyright
USU Library - 3000 Old Main Hill - Logan UT 84322 - 435.797.0816
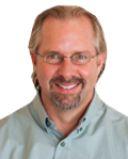
Emotions and the Gender Similarities Hypothesis
Men and women are far more alike than different in their emotions..
Posted April 8, 2015
- Coping With Guilt
- Find a therapist near me
I began to write this blog with the intent of exploring gender differences in the feeling of guilt . Specifically, the topic of women and guilt came up in a recent discussion with a student, and I recalled a similar conversation with my mother in which she was querying me about why it was it seemed that women experienced guilt so much more regularly than men. Indeed, this is a topic several folks have written on (see, e.g., here ).
There is research evidence that women do experience more guilt than men , and the short answer that I planned on elaborating upon was that women tend to be more relationally and other-oriented in their thinking and feeling styles than men and the reason that women tend to feel more guilt is that guilt orients you to how you might have injured the interests of others and then motivates one to make amends. Thus, it follows that because women are more relationally oriented, they are more likely to experience guilt. I planned on deconstructing why from the unified appraoch that combines evolutionary, learning and social role perspectives, on average women are more prone to experience relational guilt than men.
My theme for the blog shifted when I began to engage in more research analyzing the issue. When I examined a study in published in The Spanish Journal of Psychology, the authors noted that although there is a tradition of research supporting the notion that, at least in Western cultures, women tend to experience more guilt, the results were mixed. Indeed, in an earlier study the authors conducted, men and women experienced almost identical levels of guilt.
I then recalled that several years ago my research group collected data on the frequency with which college students felt different kinds of emotions. We collected data using the Positive and Negative Affect Scale , which asked participants to rate the frequency of various emotions they felt over the past week. It was a decent sample of over 500 students and we never looked at gender differneces in emotion . So I decided to run the numbers, and based on my preconceived notions, theory about gender and emotions, and prior research, I fully expected to see significant differences in the levels of guilty feelings reported by men (n = 195) compared with the women (n = 313).
The mean score for men in the extent to which the felt guilty during the previous week was a 2.14 (SD = 1.1), which corresponded to “a little” on the scale. On the other hand, the mean score for women feeling guilty was—wait for it—a mean score of 1.97 (SD = 1.1)! Thus, women scored (nonsignificantly) lower than men on guilt!
What does this mean, given prior research seems to point to their being important differences between the genders on this variable? First, it is a good reminder that humans are complicated, and research often yields inconsistent findings. (Indeed, consistent with my findings here, the PANAS manual reports tendencies for males to score higher on the guilt item). Second, I certainly acknowledge that the measure we used was not a sophisticated measure of guilt per se and there are significant questions about what those results really mean (e.g., Are men and women interpreting the word the same way, does the score mean the same thing to the two genders, do they different in guilt-proneness or the kinds of events that trigger guilt, etc.).
Despite these caveats, to my mind the results have some basic validity in the sense that if indeed this sample of college student women were indeed truly and chronically wracked with guilty feelings, as is suggested by some who consider guilt a pervasive " woman's disease ", it should have popped on the measure.
The fact that men and women scored so similarly on the measure reminded me of the importance of keeping in mind what Janet Hyde called “The Gender Similarities Hypothesis”, which is the idea that “men and women, as well as boys and girls, are more alike than they are different” (2005, p. 581). Essentially Hyde argues that, although there are some important group differences between men and women, people have role expectations and engage in confirmatory biases such that they tend to greatly exaggerate the magnitude of the differences and come to experience men and women more as a function of a priori beliefs and perceptions as opposed to “real” differences.
I agree with Hyde that we need to look through the lens of gender similarity and be cautious of expectancies and confirmation biases. I also think it is important to point that the degree of similarity or of difference depends on one’s reference point and focus. As I articulate in this previous blog , I find it enormously helpful at times to identify gender differences in tendencies in communication and cognitive and relational styles (i.e., the idea that men tend to be more agentic-instrumental and women more relational-communal in their way of being).

Given that I found a rather unexpected lack of a difference in guilt, I decided to compare the genders on the other 25 emotions that were ranked. Consistent with the gender similarities hypothesis , men and women scored similarly (i.e., not significantly different at the .05 level) on 17 of the emotions ranked, including:
Guilty, Interested, Distressed, Excited, Enthusiastic, Proud, Alert, Ashamed, Inspired, Determined, Attentive, Jittery, Active, Happy, Angry, Resentful, Embarrassed
In short, at least via this manner of evaluation, men and women are experiencing emotions quite similarly.
The nine emotions that were found to be statistically significant are listed in the following table.
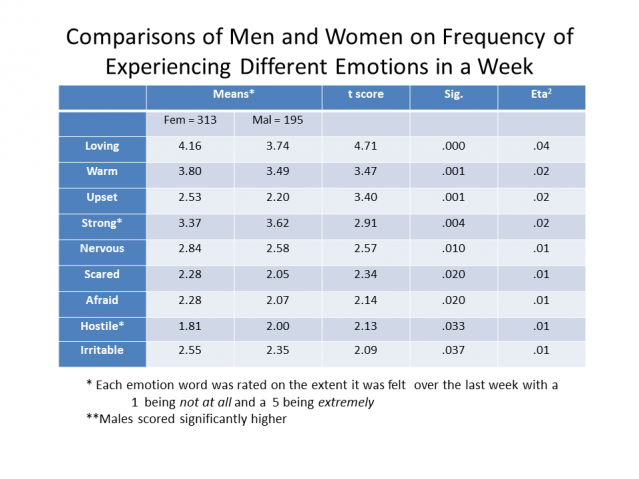
These emotion words do show some of the expected differences associated with gender, namely that women tend to endorse experiencing more affiliative (i.e., warm, loving) and more negative/ neurotic (e.g., anxiety , nervousness) feelings. But even here the gender similarities angle is important because, as the eta squared values show, these differences are not large in magnitude, meaning that the group differences really account for very little and there are far more differences within the groups than between them.
In sum, like so many people, I find gender differences fascinating and at times very illuminating. However, we need to be reminded that one tends to see based on the lens one uses and although gender differences can be a useful lens, it is also important to see men and women through the lens of gender similarities and realize that, on the whole, we are far more alike than different.
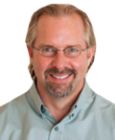
Gregg Henriques, Ph.D. , is a professor of psychology at James Madison University.
- Find a Therapist
- Find a Treatment Center
- Find a Psychiatrist
- Find a Support Group
- Find Online Therapy
- United States
- Brooklyn, NY
- Chicago, IL
- Houston, TX
- Los Angeles, CA
- New York, NY
- Portland, OR
- San Diego, CA
- San Francisco, CA
- Seattle, WA
- Washington, DC
- Asperger's
- Bipolar Disorder
- Chronic Pain
- Eating Disorders
- Passive Aggression
- Personality
- Goal Setting
- Positive Psychology
- Stopping Smoking
- Low Sexual Desire
- Relationships
- Child Development
- Self Tests NEW
- Therapy Center
- Diagnosis Dictionary
- Types of Therapy
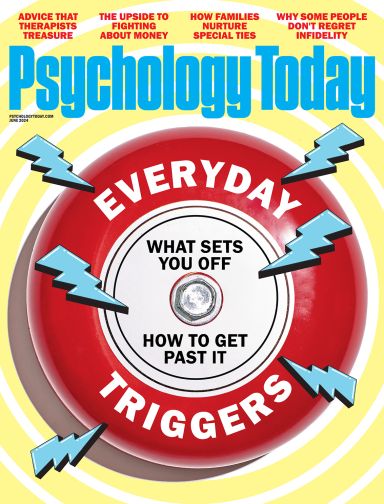
At any moment, someone’s aggravating behavior or our own bad luck can set us off on an emotional spiral that could derail our entire day. Here’s how we can face triggers with less reactivity and get on with our lives.
- Emotional Intelligence
- Gaslighting
- Affective Forecasting
- Neuroscience

Janet Shibley Hyde Sinks Stereotypes With Data
- Gender Roles
- Mathematical Ability
- Stereotypes
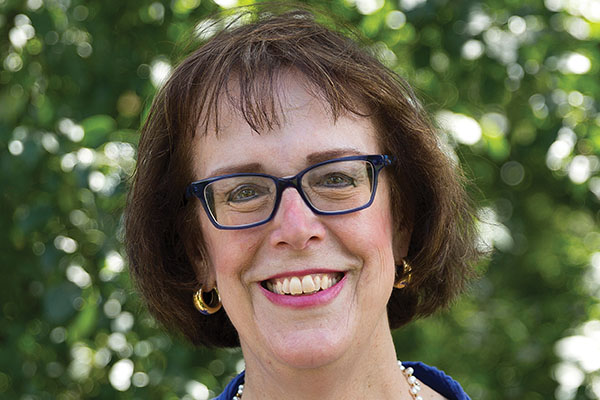
Growing up, one of APS James McKeen Cattell Fellow Janet Shibley Hyde’s favorite activities in school was Around the World, a math game in which students compete to “travel” around their classmates by answering as many problems as possible without making a mistake.
“Nobody told me I was a girl and I wasn’t supposed to be competitive,” Hyde, a professor of psychology and women’s studies at the University of Wisconsin-Madison, said during her Award Address at the 2018 APS Annual Convention in San Francisco. “I just loved the competition, what can I say?”
Even today, the kind of benevolent, and sometimes not so well-meaning, sexism epitomized by John Gray’s 1992 book “Men Are from Mars, Women Are from Venus” remains popular in mass media portrayals of gender. People think they’re being kind when they say women are hardwired by hormones or other “immutable” biological factors to be nurturing and caring, or possess a “nearly psychic” ability to perceive emotions. But none of that corresponds to the data, Hyde explained.
“Unfortunately, it’s all wrong,” she said. “As I’m fond of saying, you’ll never hear a good neuroscientist use the term ‘hardwired’ because the brain is anything but hardwired — it is plastic, so anyone who is trying to sell you on [that notion], you know you have to be suspicious of.”
Hyde’s meta-analyses of the current body of research on gender difference cast aside both the difference and deficit models — the idea that women just aren’t quite as capable as men overall — in support of what she has titled the gender similarities hypothesis: the theory that “men and women are very similar on most (not all) psychological variables.”
Or, to put it even more simply: “women are from Earth and men are from Earth.”
Order and Method
Hyde’s approach to psychological science revolves around quantitative literature reviews, a method of combining the results of numerous studies on a given topic to identify not only whether an effect exists, but how large it is.
Meta-analysis is the “gold standard” for conclusions in medicine, education, and psychological science, Hyde said, and, in the case of gender differences, comes down to computing a weighted average, d, that estimates the standardized difference between male and female scores across a set of studies.
Typically, researchers examining gender differences calculate this number so that it has a positive score if males score higher on a particular measure and a negative score if females score higher. According to psychological scientist Jacob Cohen’s guidelines for interpreting effect sizes, the number can be small (0.2), medium (0.5), or large (0.8) — or, Hyde added, completely trivial (less than 0.1), indicating an almost complete overlap in scores. For example, data indicate that the effect size of the height difference between men and women is about 2.0. This suggests a noticeable and meaningful difference between males and females, but even then, taller-than-average women can still stand head and shoulders above shorter-than-average men. A d of 0.15, on the other hand, would suggest an almost complete overlap in the distributions of scores for males and for females.
One limitation of reviewing research on gender difference, Hyde noted, is that the bulk of studies available for review are based primarily on the gender binary — a social construct facing increasing academic and cultural criticism. As such, the focus tends to be on cisgender men and women to the exclusion of both transgender and non-binary individuals. Hyde’s recent paper, “The future of sex and gender in psychology: Five challenges to the gender binary,” further elaborates on the empirical evidence for a more diverse range of gender experiences.
Running the Numbers on Women in Mathematics
In 2005, former President of Harvard University Lawrence Summers made a lengthy statement to the United States’ National Bureau of Economic Research outlining his belief that women simply don’t have the math ability to compete with men for jobs in science and engineering.
“His speech annoyed me, but I fight not with my fists, but with data, so I went to NSF and got a grant to test whether Larry Summers was right,” Hyde said.
To start, a quick glance at the National Science Foundation’s website nipped the myth that women simply aren’t interested in math in the bud: 47% of undergraduate degrees in mathematics were awarded to women in 2002.
“If women can’t do math, I don’t see how they’re getting half the undergraduate degrees in math, despite what Larry Summers says, so this is our first clue that things are not quite what the stereotypes say,” Hyde quipped.
Next, she held the question of women and girl’s performance in mathematics up to meta-analytic scrutiny using the annual state assessment scores mandated by No Child Left Behind policies in the United States. Just 10 states responded to Hyde’s request for data sorted by gender, grade level, and ethnicity, but even that was enough to provide information on more than 7 million kids.
The result? The difference between boys’ and girls’ scores in grades 2–11 had an effect size that ranged from about 0.01 to 0.06 d, with an average d value for all grades of just 0.0065.
“I don’t know what could be more ‘no gender difference’ than that,” Hyde said.
A previous meta-analysis Hyde had conducted in the 1990s found evidence of a gender performance gap in math, but these new data showed that female students had clearly reached parity with their male peers. Another 1990 study by Max Lummis and Harold W. Stevenson found that boys performed better on word problems than girls in all cultures — but that wasn’t the real takeaway. Lummis and Stevenson also found that American students were outperformed substantially by their peers of both genders in other countries.
“If girls can’t do math, why is it that girls in Taiwan do so much better than American boys?” she asked. “The big effect here is culture and how cultures treat math and how they teach math and so on. The big effect here is not gender differences.”
In fact, a 2008 study by APS Fellow Edward C. Melhuish et al. found that the best predictor of a child’s mathematical ability wasn’t gender, but their mother’s education level, a possible proxy for social class, Hyde said. Other strong predictors included their home learning environment, elementary school quality, and their family’s socioeconomic status, all factors that influence children’s educational outcomes regardless of gender.
Assertions that boys and girls learn differently due to girls’ inherent ability to cooperate and sit still for extended periods of time or higher rates of Attention Deficit/Hyperactivity Disorder (ADHD) in boys simply aren’t supported by the data, Hyde continued, but that hasn’t stopped so-called education “gurus” from promoting single-sex classrooms. In a 2014 meta-analysis of well-controlled studies with random assignment, Erin Pahlke of Whitman College, Hyde, and colleagues found no performance differences in math and other domains between single-sex and co-ed classrooms.
“What you want is a mixing,” Hyde said. “Getting them to work together and minimizing, rather than emphasizing, differences between boys and girls.”
Encouraging existing mathematical talent is important not only on the individual level, where it grants access to prestigious, high-paying jobs, Hyde explained, but on the national level.
“We can’t afford to waste 50% of our talent in the US,” she said. “We’re no longer a manufacturing society. The thing that’s going to save us in the global economic competition is our scientific and technological innovation.”
The Data on Depression
Gender stereotypes aren’t harmful only to women, of course — the depiction of depression as a “female problem” can lead clinicians to overlook boys who are struggling with the condition as well.
Rates of depression in boys and girls were thought to diverge around ages 13 to 15 years old, when rates in girls jump dramatically. But studies on the topic of gender and depression had never been subject to meta-analysis until Hyde and her team took on the challenge in 2017 — possibly because there were over 56,000 studies to wade through on the topic.
Hyde and colleagues narrowed down their search by excluding studies based on convenient samples of college students, focusing instead on representative samples drawing on populations from over 90 countries worldwide. They then split the analysis into two parts: one drawing on diagnostic measure datasets from 66 articles with 1.7 million participants, and the other drawing from 97 articles featuring over 1.9 million participants’ self-reported symptoms of depression.
The diagnosis-based meta-analysis confirmed much of the current thinking on gender and depression. Rather than computing a weighted average, Hyde used a female-to-male odds ratio (OR) to find that, averaged over all ages and nationalities included in the study, roughly 1.95 females per male met the diagnostic criteria for depression — just slightly under the commonly cited 2.0 OR.
Beyond that, there were some surprising findings, Hyde said. To begin with, the OR at age 12 was found to be 2.37, suggesting that gender differences in depression diagnoses may arise even earlier than previously believed.
Furthermore, the OR was highest — at nearly 3.0 — among 13- to 15-year-olds. From there, the OR declined across age groups, reaching the overall average of 2.0 for participants in their 20s, and remaining stable thereafter, a previously unidentified trend in adulthood, Hyde continued.
These findings have significant clinical, methodological, and theoretical implications, Hyde said. An OR of 2.0 suggests that the majority of people with depression are female, but that still means 1/3 of depressed adults are men. Yet the perception of depression as a somehow “feminine” condition can make men reluctant to seek help. The prevalence of studies on depression with single-sex designs that exclude male participants exacerbates these stereotypes, preventing progress on the question of how depression manifests in boys and men, she added.
Theoretically, Hyde continued, conceptualizations of how depression may emerge as a reaction to factors such as pubertal hormones, stress, and sexual harassment victimization in adolescence need to account for the narrowing of the gender gap in the 20s.
When Sexuality Met Gender Empowerment
Educational outcomes and measures of mental health can tell us a lot about the similarities and differences between men and women, but there are few domains of life where traditional stereotypes remain more resilient than when it comes to sexuality. In a 2010 meta-analysis of datasets from 840 articles involving more than 1.4 million participants from 82 countries, Jennifer L. Peterson and Hyde found that although differences between men’s and women’s sexual attitudes (such as supporting gay rights or premarital sex) were generally small or trivial, men were more likely to view pornography, engage in masturbation, and have casual sexual encounters.
The researchers also identified an important moderating factor: gender empowerment. In countries that the United Nations Development Programme rated as having higher gender empowerment — a measure that factors in the ratio of women to men holding parliamentary seats, average estimated income, and the percentage of women working in high-level positions such as senior management, public office, and academic jobs — the behavioral differences between men and women were smaller.
Hyde notes that they did not observe this trend for every behavior they examined, but the overall effect aligns with the social role theory, which posits that men and women behave differently in large part due to societal expectations.
In 2005, when Hyde first put forward the gender similarities hypothesis, the data created a clear pattern in support of her claim: of 124 effect sizes from 46 existing meta-analyses, 78% reported d values that were small or close to zero. The “meta-meta-analysis,” independently replicated in 2015, found evidence of some gender differences, but not nearly enough to suggest that men and women come from different psychological worlds — and studies of very young children suggest that the differences that do exist may take years of socialization to develop.
“What we need to do is think more systematically about where the messages are coming from that create those early differences,” Hyde said. “I don’t for a minute believe they’re hardwired into the brain.”
Hyde, J. S. (2005). The gender similarities hypothesis. American Psychologist , 60(6), 581-592. doi:10.1037/0003-066x.60.6.581
Hyde, J. S., Lindberg, S. M., Linn, M. C., Ellis, A. B., & Williams, C. C. (2008). Gender similarities characterize math performance. Science , 321(5888), 494-495. doi:10.1126/science.1160364
Lummis, M., & Stevenson, H. W. (1990). Gender differences in beliefs and achievement: A cross-cultural study. Developmental Psychology , 26(2), 254-263. doi:10.1037/0012-1649.26.2.254
Melhuish, E. C., Sylva, K., Sammons, P., Siraj-Blatchford, I., Taggart, B., Phan, M. B., & Malin, A. (2008). The early years: Preschool influences on mathematics achievement. Science , 321(5893), 1161-1162. doi:10.1126/science.1158808
Pahlke, E., Hyde, J., & Allison, C. M. (2014). Supplemental material for the effects of single-sex compared with coeducational schooling on students’ performance and attitudes: A meta-analysis. Psychological Bulletin . doi:10.1037/a0035740.supp
Petersen, J. L., & Hyde, J. S. (2010). A meta-analytic review of research on gender differences in sexuality, 1993–2007. Psychological Bulletin , 136(1), 21-38. doi:10.1037/a0017504
I thought that Summers’ point was that although male and female average math scores were much the same, the males showed greater variance. Hence more men at the top and bottom of the scale and thus more men in high-end math discipline. Does Prof. Shibley’s work address this point?
APS regularly opens certain online articles for discussion on our website. Effective February 2021, you must be a logged-in APS member to post comments. By posting a comment, you agree to our Community Guidelines and the display of your profile information, including your name and affiliation. Any opinions, findings, conclusions, or recommendations present in article comments are those of the writers and do not necessarily reflect the views of APS or the article’s author. For more information, please see our Community Guidelines .
Please login with your APS account to comment.

Back Page: Singular Sensation
Clinical psychologist Dominika Ochnik discusses her research into singlehood and well-being, mental health risks among young people, and her plans to study associations between urbanization and mental health.

Up-and-Coming Voices: Artificial Intelligence in Psychological Science
Previews of relevant research by students and early-career scientists.

Encouraging Girls to Roleplay as Successful Female Scientists Could Help Close the Gender Gap in STEM
Girls may persist longer in science activities when they pretend to be successful female scientists. This kind of play-based intervention could help close the gender gap in science, technology, engineering, and math (STEM) fields.
Privacy Overview
Cookie | Duration | Description |
---|---|---|
__cf_bm | 30 minutes | This cookie, set by Cloudflare, is used to support Cloudflare Bot Management. |
Cookie | Duration | Description |
---|---|---|
AWSELBCORS | 5 minutes | This cookie is used by Elastic Load Balancing from Amazon Web Services to effectively balance load on the servers. |
Cookie | Duration | Description |
---|---|---|
at-rand | never | AddThis sets this cookie to track page visits, sources of traffic and share counts. |
CONSENT | 2 years | YouTube sets this cookie via embedded youtube-videos and registers anonymous statistical data. |
uvc | 1 year 27 days | Set by addthis.com to determine the usage of addthis.com service. |
_ga | 2 years | The _ga cookie, installed by Google Analytics, calculates visitor, session and campaign data and also keeps track of site usage for the site's analytics report. The cookie stores information anonymously and assigns a randomly generated number to recognize unique visitors. |
_gat_gtag_UA_3507334_1 | 1 minute | Set by Google to distinguish users. |
_gid | 1 day | Installed by Google Analytics, _gid cookie stores information on how visitors use a website, while also creating an analytics report of the website's performance. Some of the data that are collected include the number of visitors, their source, and the pages they visit anonymously. |
Cookie | Duration | Description |
---|---|---|
loc | 1 year 27 days | AddThis sets this geolocation cookie to help understand the location of users who share the information. |
VISITOR_INFO1_LIVE | 5 months 27 days | A cookie set by YouTube to measure bandwidth that determines whether the user gets the new or old player interface. |
YSC | session | YSC cookie is set by Youtube and is used to track the views of embedded videos on Youtube pages. |
yt-remote-connected-devices | never | YouTube sets this cookie to store the video preferences of the user using embedded YouTube video. |
yt-remote-device-id | never | YouTube sets this cookie to store the video preferences of the user using embedded YouTube video. |
yt.innertube::nextId | never | This cookie, set by YouTube, registers a unique ID to store data on what videos from YouTube the user has seen. |
yt.innertube::requests | never | This cookie, set by YouTube, registers a unique ID to store data on what videos from YouTube the user has seen. |
Ischemic Heart Disease: The Copper Deficiency Theory

Related content:

Ischemic heart disease, the leading cause of death in the United States, became increasingly common during the 20 th century. Its etiology remains obscure. Increased serum cholesterol predicts cardiovascular risk, and thousands of medical articles have described the effects of dietary fat on cholesterol metabolism. However, more than 50 studies of various populations have failed to associate fat intake with risk. Other characteristics predictive of cardiovascular risk include electrocardiographic abnormalities, glucose intolerance, and high blood pressure. Uric acid is so closely associated with risk that the Framingham multivariate analyses corrected for uric acid levels.
However, if the incidence of a disease, in this case heart disease, changes over time, one might expect similar changes in the suspected causal agents. Dietary fat has not changed in the US for a century. In contrast, dietary copper has decreased since the 1930s.
Deficiency diseases, such as beriberi, pellagra, and scurvy, have nearly disappeared in the US. Inspired by the now well-known protective effect of hard drinking water on heart disease, I began to study trace elements and discovered fifty years ago that rats deficient in copper have very high cholesterol levels. This discovery prompted a search for characteristics of animals deficient in copper and relationships between copper metabolism and heart disease. Feeding cholesterol to animals also has produced similarities to heart disease because cholesterol feeding induces copper deficiency.
Recently, more than 30 men and women have been depleted of copper with care. Some people had abnormal blood pressure responses, abnormal heartbeats, high cholesterol, and glucose intolerance. One of the experiments was modified because some men experienced severe tachycardias, heart block, and a heart attack.
At least 80 anatomical, chemical, and physiological similarities exist between people with ischemic heart disease and animals deficient in copper. Coronary artery thrombosis, decreased cardiac copper, hyperuricemia, heart blocks, glucose intolerance, and myocardial infarction are among the more important. Recently, paraoxonase was found decreased in copper deficiency. This enzyme destroys homocysteine thiolactone, which inhibits an enzyme required for the synthesis and repair of arterial connective tissue.
Sugar-sweetened beverages and soft drinks have also been associated with increased heart disease risk. Two-thirds of sugary beverages likely contain “soft” water [1] without copper. While sugars, especially fructose, interfere with copper metabolism in animals, the use of “hard” water in beer and wine may contribute to the protective effect of alcoholic beverages on heart disease risk.
Identifying individuals with low levels of copper can be difficult; serum copper is increased by inflammation, and recent research suggests that serum copper should be corrected for the degree of inflammation present. Newer tests, such as enzyme activity of superoxide dismutase in red blood cells, should be used more widely. This enzyme defends against oxidative stress.
Our daily copper requirements must be determined by depletion studies. For copper, this amount is 0.7mg/day; the recommended dietary allowance (RDA) is 0.9mg/day. Diets with less copper than the RDA for copper are typical. It is unknown if men have a different copper requirement than women.
The copper deficiency theory on the etiology and pathophysiology of ischemic heart disease is the simplest and most general theory on the etiology and pathophysiology of ischemic heart disease that has been proposed because it encompasses the observations of Barker concerning small babies, McCully concerning homocysteine, and Sullivan concerning iron overload.
- Small babies often are born before maternal metabolism supplements them with copper, and small birth weight is associated with heart disease in middle age.
- High levels of homocysteine are associated with injury to the coronary arteries; Copper supplementation lowered plasma homocysteine in men.
- Loss of iron through menstruation may protect women from heart disease. Excess iron can interfere with copper utilization. Iron supplements may well require additional copper.
Tests of this theory need not involve hundreds of patients, as is the case with many current therapeutic studies on heart disease. A small study of septuagenarian patients with chronic heart failure and ischemic heart disease showed that a supplement containing copper improved left ventricular function and quality of life over ten months. More research on copper may lead to the disappearance of ischemic heart disease.
[1] Hard water contains high concentrations of calcium and magnesium. Water softeners replace these elements with sodium.
- ischemic heart disease
- Copper deficiency theory
- Dietary copper intake
View the discussion thread.

Leslie Klevay
Recent articles by this author:, acsh relies on donors like you. if you enjoy our work, please contribute. make your tax-deductible gift today.

Popular articles

ACSH Podcasts

Latest from Henry
Latest from josh, latest from chuck.
Mobile Menu Overlay
The White House 1600 Pennsylvania Ave NW Washington, DC 20500
Fact Sheet: Biden- Harris Administration’s Actions to Advance American Biotechnology and Biomanufacturing
Through his Investing in America Agenda, President Biden is making a once-in-a-generation investment in our clean energy future. His leadership has spurred a revolution in clean electricity, transportation, and efficiency that is cutting costs for American families and creating good-paying jobs across the country. To meet the challenges of decarbonizing the U.S. economy, President Biden is also driving progress to advance domestic biomanufacturing and biotechnology which will provide an alternative to petroleum-based production for chemicals, medicines, fuels, materials, and more. These investments support President Biden’s climate and clean energy goals, build stronger supply chains, and advance American leadership around the globe.
To advance these efforts, President Biden signed an Executive Order on Advancing Biotechnology and Biomanufacturing Innovation for a Sustainable, Safe, and Secure American Bioeconomy that has catalyzed action across the government to set bold goals, develop the biomanufacturing workforce, promote American leadership in biotechnology research and development, and ensure that these technologies are developed and utilized responsibly. The President’s commitment to a clean energy economy has spurred $29 billion in public and private sector biomanufacturing investments for projects across the country since the start of the Biden-Harris Administration.
Today, the White House, members of the National Bioeconomy Board, and the Departments of Energy (DOE), Defense (DOD), and Agriculture convened major biotechnology and biomanufacturing companies, nonprofits, and other stakeholders met to highlight progress to date and discuss critical upcoming milestones to support the long-term success of the nation’s bioeconomy.
This month, the Biden-Harris Administration announced a set of actions to accelerate U.S. domestic biomanufacturing capacity and highlighting progress in the two years since the President signed the Executive Order, including:
- DOE’s Loan Programs Office announced a $213.6 million conditional loan guarantee to Solugen Inc’s Bioforge Marshall facility in Marshall, Minnesota. This commitment is the largest single federal investment in bioindustial manufacturing since President Biden signed Executive Order 14081.The new facility, Bioforge Marshall, will house three modular trains manufacturing various organic acids for use in the concrete, cleaning, agricultural, and energy industries—cutting harmful emissions from hard-to-decarbonize sectors while helping deliver healthier communities across the nation. As part of President Biden’s Investing in America agenda to create good-paying, high-quality job opportunities for American workers, this project will create up to 100 jobs during construction and 56 highly skilled full-time manufacturing jobs once fully operational.
- The Department of Agriculture is making available recommendations for revisions to the North American Industry Classification System and North American Product Classification System codes for the bioeconomy . These classifications provide a systematic organization of both industries (NAICS) and products (NAPCS) and are currently lacking for the bioeconomy. NAICS industries are defined by similarities in production processes. NAPCS products are defined based on use. The recommendations for revisions to the NAICS and NAPCS are guided by the National Institutes of Standards and Technology’s Bioeconomy Lexicon , and a study of the feasibility of measuring the bioeconomy by the Bureau of Economic. These revisions will lay the foundation to organize, sustain, and accelerate the growth of the U.S. bioeconomy through data-driven decision-making.
- The Department of Agriculture is also releasing a request for information from the public as it explores options for reducing the regulatory burden for modified microbes. Engineered organisms have the potential to improve agricultural productivity and address sustainability and climate goals. The information USDA hopes to receive will inform how to ensure proportionate oversight that will allow for both safety and commercialization of beneficial products.
- The National Security Council is announcing the newly formed Biopharma Coalition (Bio-5) to support secure biopharmaceutical supply chains, in partnership with the Office of Pandemic Preparedness and Response. The United States, EU, India, Japan, and the Republic of Korea will work together to strengthen biopharmaceutical supply chain resilience. The Bio-5, which initially met in a track 1.5 format alongside industry representatives from each entity, will focus on building resilient supply chains for active pharmaceutical ingredients (APIs) currently sourced primarily from the People’s Republic of China. The five countries will seek opportunities for their governments and the private sector to deepen coordination on policy, regulations, R&D capabilities, and other tools to enhance the resilience of this vital sector.
These announcements build upon more than $3.5 billion in investments and a wide range of actions the Biden-Harris Administration has already taken to increase U.S. biomanufacturing capacity:
- The Administration established the National Bioeconomy Board to lead actions to achieve a sustainable, safe, and secure American bioeconomy.
- The Department of Defense, which released its Biomanufacturing Strategy in March 2023, announced an over $1 billion investment in domestic biomanufacturing to strengthen defense supply chains. These efforts will accelerate the bio-materials development pipeline with the Air Force, Army, and Navy laboratories; establish a commercial investment program that seeks to industrialize maturing biomaterials; and build supply chain resilience for the Department. This investment prioritizes applications where technologies have both military and commercial applications covering the primary focus areas of: Food, Fuel, Fitness, Fabrication, and Firepower.
- DOE’s Office of Science supported $264 million for 29 projects to address the scientific challenges underlying DOE’s Energy Earthshots™ Initiative to advance clean energy technologies within the decade and drive broader innovation for a sustainable bioeconomy. The funding will support 11 new Energy Earthshot Research Centers led by DOE National Laboratories and 18 university research teams addressing the Energy Earthshots™ that are focused on six different areas, including biologically-driven solutions in carbon-neutral energy, industrial decarbonization, and carbon storage.
- DOE’s Bioenergy Technologies Office (BETO ) is supporting $151 million to scale promising technologies that convert biomass to biofuels and bioproducts, accelerating the growing Bioeconomy. The selected projects include demonstration-scale biomanufacturing facilities that will ramp up to produce millions of gallons of low-carbon fuel annually. By investing in these technologies, the projects will create good-paying jobs in rural and underserved communities in nine states and enhance education and training opportunities regionally to build the bioenergy workforce.
- DOE’s Fossil Energy and Carbon Management Office (FECM) and BETO have also provided $80M to support research, and field trials for biomass and waste feedstocks including CO2 gases, micro- and macro algae and energy crops to fuel the bioeconomy and over $40M to support research in the development of industrially relevant biocatalysts/microorganisms to make more affordable biofuels and bioproducts.
- DOE’s Office of Clean Energy Demonstration (OCED) announced up to $200 million in Federal Cost Share for the Sustainable Ethylene from CO2 Utilization with Renewable Energy (SECURE) project, led by T.EN Stone & Webster Process Technology, Inc. in partnership with LanzaTech, with plans to demonstrate an integrated process to utilize captured carbon dioxide from ethylene production—an important building block for many products—by applying a biotech-based processand green hydrogen to create clean ethanol and ethylene.
- The National Science Foundation (NSF) announced a $30 million investment in the NSF Science and Technology Center for Quantitative Cell Biology. Led by researchers at the University of Illinois Urbana-Champaign, Harvard Medical School, and the J. Craig Venter Institute, this center brings together an interdisciplinary group of experts to develop whole cell models—a full quantitative description of the physical and chemical processes that define the state of a cell. Whole cell models can be used to predict and design new bioengineered systems or compare the function of healthy cells to diseased cells, leading to a better understanding of what goes wrong in diseased cells.
- NSF, in partnership with the Simons Foundation, announced a $50 million investment in an institute tasked with bringing together experts in mathematics and biology to uncover the fundamental principles governing life through theories, data-informed mathematical models, and computational, statistical, and AI tools, critical fundamental research that will fuel innovations across all sectors of the bioeconomy. The National Institute for Theory and Mathematics in Biology (NITMB), led by Northwestern University and the University of Chicago, will create a nationwide collaborative research community that will target promising and challenging areas of exploration that help address some of societies greatest challenges, including those with impacts on the bioeconomy like sustainable agriculture, preventing pandemics, and mitigating the effects of climate change. The NITMB will also train the next generation of researchers to use to power of mathematics and biology to advances in areas as diverse as the environment, biomedicine, and technology development.
- NSF awarded a first in its kind National Synthesis Center for Emergence in Molecular and Cellular Sciences, a center focused on data synthesis, use and reuse in the molecular and cellular biotechnology space. The $20 million center, led by Pennsylvania State University, with partners including the University of Arizona, Claflin University, Alcorn State University, and Fayetteville State University, will tackle many of the data infrastructure and data workforce issues that are critical to enabling a robust U.S. bioeconomy.
- NSF has committed to increasing U.S. investment in bioeconomy-related infrastructure, including biofoundries and centers, and is completing its first competition for its new bioFoundries to Enable Access to Infrastructure and Resources for Advancing Modern Biology and Biotechnology program. With up to $75 million in funds committed towards the first BioFoundries, NSF is investing in and democratizing access to critical infrastructure that will advance all sectors of the bioeconomy, spur tool development, enable user-initiated research provide user facilities, and train and grow the biotechnology workforce across the U.S. In addition, with the latest Global Centers competition underway, on Use Inspired Research Addressing Global Challenges through the Bioeconomy, with topics that include biofoundries, and partners from Canada, Finland, Japan, Korea, and the United Kingdom, NSF is poised to commit an additional $25 million, a commitment that will be amplified by the international partners.
- USDA’s Rural Development program has invested more than $500 million in several different loan and grant programs to impact the domestic bioeconomy. These programs invested in areas such as advanced biofuels, biofuel infrastructure, renewable fertilizer production, and biogas and biomass projects. In addition, the RD Biopreferred catalog of biobased products added 1,517 net new products and 559 net new companies offering those products.
- USDA has invested over $500 million in new research and development to advance the nation’s bioeconomy since the President’s Executive Order. Pioneering work by USDA scientists created the foundation for the first commercial harvests of new oilseed crops—domesticated pennycress and carinata—which provide new sources of fuel and feed without requiring additional cropland. Advancements in biomanufacturing by USDA have resulted in adhesives for grocery PLU stickers that can be composted, bio-asphalts that generate fewer emissions, and biobased antioxidants for stabilization of natural rubber in tires. USDA’s research strives to harness the power of biotechnology and biomanufacturing to expand opportunities for U.S. agricultural producers while also tackling some of our greatest challenges like climate change, nutrition insecurity, environmental justice, emerging diseases and pests, and growing competition overseas.
- The USDA Forest Service has made catalytic investments in the wood-based bioeconomy to support land management, wildfire risk reduction, climate adaptation, and local economies. These projects support the critical connection between healthy and resilient federal, state, tribal and private forests and the wood products economy. Through USDA’s Forest Service, the Biden-Harris Administration has funded 482 projects totaling nearly $190 million in investments to support the wood-based bioeconomy, including supporting innovation, market development and new and expanded manufacturing capacity.
- The State Department has initiated additional extensive international engagement to assess opportunities for global cooperation on the bioeconomy, coordinated by the Office of the Special Envoy for Critical and Emerging Technology. This work complements ongoing bilateral and multilateral efforts led by the Bureaus of Oceans and International Environmental and Scientific Affairs, Economic and Business Affairs, and others.
- NSF and the Indian Department of Biotechnology signed an implementation arrangement to enable collaborative biotechnology and biomanufacturing research partnerships between engineers and scientists from the United States and India.
- President Biden launched the Global Biofuels Alliance at the 2023 G20 Leader’s Summit to make progress in our shared commitment to deploy cleaner, greener fuels around the world that help meet our decarbonization goals. This alliance between the United States, Argentina, Brazil, India, Italy, Mauritius, and the United Arab Emirates will focus on raising awareness on the benefits of biofuels, policy lessons learned, existing standards and certification; and an emphasis on sustainability, security of supply and affordability principles while avoiding overlap with existing biofuels initiatives.
These critical achievements highlight the Biden-Harris Administration’s government-wide approach to advancing the biotechnology and biomanufacturing towards innovative solutions in health, climate change, energy, food security, agriculture, supply chain resilience, and national and economic security.
Stay Connected
We'll be in touch with the latest information on how President Biden and his administration are working for the American people, as well as ways you can get involved and help our country build back better.
Opt in to send and receive text messages from President Biden.
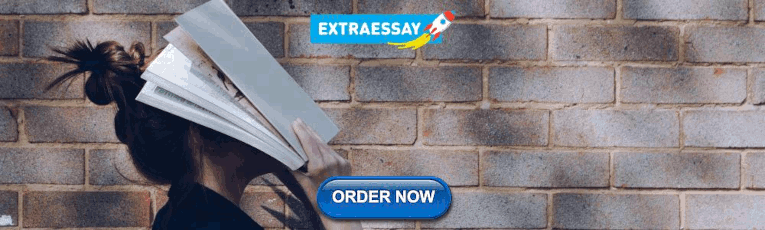
IMAGES
VIDEO
COMMENTS
The gender similarities hypothesis holds that males and females are similar on most, but not all, psychological variables. That is, men and women, as well as boys and girls, are more alike than they are different. In terms of effect sizes, the gender similarities hypothesis states that most psychological gender differences are in the close-to-
The gender similarities hypothesis, proposed by Hyde (2005), states that males and females are similar on most, but not all, psychological variables. Based on a meta-analysis of 46 meta-analyses of psychological gender differences, 30% of effect sizes were trivial in magnitude (d between 0 and 0.10) and an additional 48% were small (0.10-0.35).
The differences model, which argues that males and females are vastly different psychologically, dominates the popular media. Here, the author advances a very different view, the gender similarities hypothesis, which holds that males and females are similar on most, but not all, psychological variables. Results from a review of 46 meta-analyses ...
The gender similarities hypothesis proposed by Hyde ( 2005) argues that women and men are similar on most, but not all, psychological variables. Evidence in favor of this hypothesis as opposed to the gender differences hypothesis is accumulating while acknowledging exceptions and the role of contextual variations.
Numerous psychological theories lay the foundation for gender similarities in psychological constructs and attempt to explain why gender differences exist. This chapter focuses on three specific theories that are often applied to the theoretical foundation of psychological gender differences. It provides a brief description of evolutionary theory, social structural theory, and cognitive social ...
Results from a review of 46 meta-analyses support the gender similarities hypothesis, which holds that males and females are similar on most, but not all, psychological variables. The differences model, which argues that males and females are vastly different psychologically, dominates the popular media. Here, the author advances a very different view, the gender similarities hypothesis, which ...
The differences model, which argues that males and females are vastly different psychologically, dominates the popular media. Here, the author advances a very different view, the gender similarities hypothesis, which holds that males and females are similar on most, but not all, psychological variables. Results from a review of 46 meta-analyses support the gender similarities hypothesis ...
Gender similarities hypothesis: the hypothesis that males and females are similar on most, but not all, psychological variables Meta-analysis: a statistical technique that allows a researcher to combine the results of many research studies on a given topic Effect size: in the study of gender
Here, the author advances a very different view, the gender similarities hypothesis, which holds that males and females are similar on most, but not all, psychological variables. Results from a review of 46 meta-analyses support the gender similarities hypothesis. Gender differences can vary substantially in magnitude at different ages and ...
Whether men and women are fundamentally different or similar has been debated for more than a century. This review summarizes major theories designed to explain gender differences: evolutionary theories, cognitive social learning theory, sociocultural theory, and expectancy-value theory. The gender similarities hypothesis raises the possibility ...
Whether men and women are fundamentally different or similar has been debated for more than a century. This review summarizes major theories designed to explain gender differences: evolutionary theories, cognitive social learning theory, sociocultural theory, and expectancy-value theory. The gender similarities hypothesis raises the possibility of theorizing gender similarities. Statistical ...
Abstract. I review new trends in research on the psychology of gender. The gender similarities hypothesis holds that males and females are similar on most, but not all, psychological variables. Gender is not only an individual-difference or person variable but also a stimulus variable. Emerging approaches to cross-national measurement of ...
The Hypothesis. The gender similarities hypothesis holds that males. and females are similar on most, but not all, psychological. variables. That is, men and women, as well as boys and. girls, are ...
The analysis reported upon in this paper is used to test the gender similarities hypothesis (Hyde, 2005, 2014, 2016) in relation to student achievement in high-stakes mathematics examinations in Northern Ireland.Based on a review of 46 meta-analyses, Hyde concluded that, "males and females are similar on most, but not all, psychological variables" (p. 581).
The gender similarities hypothesis raises the possibility of theorizing gender similarities. Statistical methods for the analysis of gender differences and similarities are reviewed, including effect sizes, meta-analysis, taxometric analysis, and equivalence testing. Then, relying mainly on evidence from meta-analyses, gender differences are ...
The gender similarities hypothesis. Am. Psychol. 60, 581-592 (2005). Article Google Scholar American Association of University Women Educational Foundation. How Schools Shortchange Girls.
PDF. TL;DR: Results from a review of 46 meta-analyses support the gender similarities hypothesis, which holds that males and females are similar on most, but not all, psychological variables. Abstract: The differences model, which argues that males and females are vastly different psychologically, dominates the popular media. Here, the author ...
Whether men and women are fundamentally different or similar has been debated for more than a century. This review summarizes major theories designed to explain gender differences: evolutionary theories, cognitive social learning theory, sociocultural theory, and expectancy-value theory. The gender similarities hypothesis raises the possibility of theorizing gender similarities. Statistical ...
The differences model, which argues that males and females are vastly different psychologically, dominates the popular media. Here, the author advances a very different view, the gender similarities hypothesis, which holds that males and females are similar on most, but not all, psychological variables. Results from a review of 46 metaanalyses support the gender similarities hypothesis. Gender ...
The fact that men and women scored so similarly on the measure reminded me of the importance of keeping in mind what Janet Hyde called "The Gender Similarities Hypothesis", which is the idea ...
In 2005, when Hyde first put forward the gender similarities hypothesis, the data created a clear pattern in support of her claim: of 124 effect sizes from 46 existing meta-analyses, 78% reported d values that were small or close to zero. The "meta-meta-analysis," independently replicated in 2015, found evidence of some gender differences ...
The gender similarities hypothesis holds that males and females are similar on most, but not all, psychological variables. That is, men and women, as well as boys and girls, are more alike than they are different. In terms of effect sizes, the gender similarities hypothesis states that most psychological gender differences are in the close-to-
Gender Similarities Hypothesis. April 2023. DOI: 10.1007/978-3-031-08956-5_163-1. In book: Encyclopedia of Sexual Psychology and Behavior (pp.1-5) Authors: Janine Bosak. Dublin City University ...
Ischemic heart disease, the leading cause of death in the United States, has long puzzled researchers. While high serum cholesterol levels are linked to cardiovascular risk, the role of dietary fat remains unclear. The copper deficiency theory, suggesting a strong link between decreased copper intake since the 1930s and the rise of ischemic heart disease, can provide a new perspective on ...
The National Institute for Theory and Mathematics in Biology (NITMB), led by Northwestern University and the University of Chicago, will create a nationwide collaborative research community that ...