
An official website of the United States government
The .gov means it’s official. Federal government websites often end in .gov or .mil. Before sharing sensitive information, make sure you’re on a federal government site.
The site is secure. The https:// ensures that you are connecting to the official website and that any information you provide is encrypted and transmitted securely.
- Publications
- Account settings
Preview improvements coming to the PMC website in October 2024. Learn More or Try it out now .
- Advanced Search
- Journal List
- J Oral Maxillofac Pathol
- v.23(2); May-Aug 2019
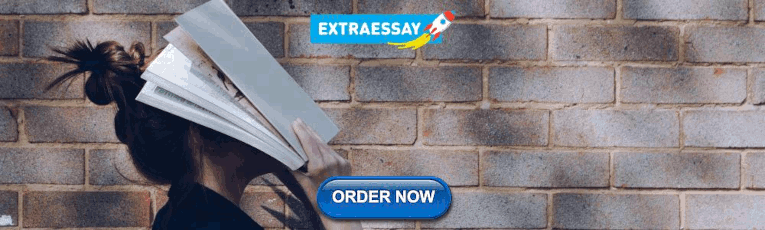
Hypothesis-driven Research
Umadevi krishnamohan rao.
1 Department of Oral and Maxillofacial Pathology, Ragas Dental College and Hospital, Chennai, Tamil Nadu, India E-mail: moc.liamg@kvuamu

As Oral Pathologists, we have the responsibility to upgrade our quality of service with an open mind attitude and gratitude for the contributions made by our professional colleagues. Teaching the students is the priority of the faculty, and with equal priority, oral pathologists have the responsibility to contribute to the literature too as a researcher.
Research is a scientific method of answering a question. This can be achieved when the work done in a representative sample of the population, i.e., the outcome of the result, can be applied to the rest of the population, from which the sample is drawn. In this context, frequently done research is a hypothesis-driven research which is based on scientific theories. Specific aims are listed in this type of research, and the objectives are stated. The scope of a well-designed methodology in a hypothesis-driven research equips the researcher to establish an opportunity to state the outcome of the study.
A provisional statement in which the relationship between two variables is described is known as hypothesis. It is very specific and offers the freedom of evaluating a prediction between the variables stated. It facilitates the researcher to envision and gauge as to what changes can occur in the listed specific outcome variables (dependent) when changes are made in a specific predictor (independent) variable. Thus, any given hypothesis should include both these variables, and the primary aim of the study should be focused on demonstrating the association between the variables, by maintaining the highest ethical standards.
The other requisites for a hypothesis-based study are we should state the level of statistical significance and should specify the power, which is defined as the probability that a statistical test will indicate a significant difference when it truly exists.[ 1 ] In a hypothesis-driven research, specifications of methodology help the grant reviewers to differentiate good science from bad science, and thus, hypothesis-driven research is the most funded research.[ 2 ]
“Hypotheses aren’t simply useful tools in some potentially outmoded vision of science; they are the whole point.” This was stated by Sean Carroll, from the California Institute of Technology, in response to Editor-In-Chief of “ Wired ” Chris Anderson, who argued that “biology is too complex for hypotheses and models, and he favored working on enormous data by correlative analysis.”[ 3 ]
Research does not stop by stating the hypotheses but must ensure that it is clear, testable and falsifiable and should serve as the fundamental basis for constructing a methodology that will allow either its acceptance (study favoring a null hypothesis) or rejection (study rejecting the null hypothesis in favor of the alternative hypothesis).
It is very worrying to observe that many research projects, which require a hypothesis, are being done without stating one. This is the fundamental backbone of the question to be asked and tested, and later, the findings need to be extrapolated in an analytical study, addressing a research question.
A good dissertation or thesis which is submitted for fulfillment of a curriculum or a submitted manuscript is comprised of a thoughtful study, addressing an interesting concept, and has to be scientifically designed. Nowadays, evolving academicians are in a competition to prove their point and be academically visible, which is very vital in their career graph. In any circumstance, unscientific research or short-cut methodology should never be conducted or encouraged to produce a research finding or publish the same as a manuscript.
The other type of research is exploratory research, which is based on a journey for discovery, which is not backed by previously established theories and is driven by hope and chance of breakthrough. The advantage of using these data is that statistics can be applied to establish predictions without the consideration of the principles of designing a study, which is the fundamental requirement of a conventional hypothesis. There is a need to set standards of statistical evidence with a much higher cutoff value for acceptance when we consider doing a study without a hypothesis.
In the past few years, there is an emergence of nonhypothesis-driven research, which does receive encouragement from funding agencies such as innovative molecular analysis technologies. The point to be taken here is that funding of nonhypothesis-driven research does not implicate decrease in support to hypothesis-driven research, but the objective is to encourage multidisciplinary research which is dependent on coordinated and cooperative execution of many branches of science and institutions. Thus, translational research is challenging and does carry a risk associated with the lack of preliminary data to establish a hypothesis.[ 4 ]
The merit of hypothesis testing is that it takes the next stride in scientific theory, having already stood the rigors of examination. Hypothesis testing is in practice for more than five decades and is considered to be a standard requirement when proposals are being submitted for evaluation. Stating a hypothesis is mandatory when we intend to make the study results applicable. Young professionals must be appraised of the merits of hypothesis-based research and must be trained to understand the scope of exploratory research.
Hypothesis Requirements
Hypotheses are a crucial part of the scientific thinking process, and most professional scientific endeavors are hypothesis-driven. That is, they seek to address a specific, measurable, and answerable question. A well-constructed hypothesis has several characteristics: it is clear, testable, falsifiable, and serves as the basis for constructing a clear set of experiments that will allow the student to discuss why it can be accepted or rejected based on the experiments. We believe that it is important for students who publish with JEI to practice rigorous scientific thinking through generating and testing hypotheses.
This means that manuscripts that merely introduce an invention, a computational method, a new machine/deep learning or AI algorithm, no matter how impressive they are, are not appropriate for JEI. Here are some common examples of unacceptable “hypotheses” relating to engineering projects:
- I hypothesize that my invention/method/machine learning model will work
- I hypothesize that I can build this invention/method/machine learning model
- I hypothesize that my machine/deep learning or AI model will be effective and yield accurate results
If your hypothesis boils down to one of the above hypotheses, your research is engineering-based. If your manuscript is related to engineering and/or computation algorithm development, please read our Guidelines for Engineering-Based Projects .
Additionally, review articles , where a review of the existing literature on a topic is presented, are not eligible for publication in JEI at this time .
This video goes over the general hypothesis requirements as they relate to research eligible for publication at JEI. It was created by one of our previous authors and current student advisory board members, Akshya Mahadevan!
When you assess whether your manuscript has a clear, well-constructed hypothesis, please ask whether it meets the following five criteria:
1. It IS NOT discovery or descriptive research
Some research is not hypothesis-driven. Terms used to describe non-hypothesis-driven research are ‘descriptive research,’ in which information is collected without a particular question in mind, and ‘discovery science,’ where large volumes of experimental data are analyzed with the goal of finding new patterns or correlations. These new observations can lead to hypothesis formation and other scientific methodologies. Some examples of discovery or descriptive research include an invention, explaining an engineered design like a program or an algorithm, mining large datasets for potential targets, or even characterizing a new species. However, if you have a pre-existing hypothesis and use large datasets to test it , this is acceptable for submission to JEI.
Another way to assess whether your research is hypothesis-driven is by analyzing the experimental setup. What variables in the experiment are independent, and which are dependent? Do the results of the dependent variable answer the scientific question? Are there positive and negative control groups?
2. It IS original
While your hypothesis does not have to be completely novel within the larger field of your research topic, it cannot be obvious to you, given the background information or experimental setup. You must have developed the hypothesis and designed experiments to test it yourself. This means that the experiments cannot be prescribed – an assigned project from an AP biology course, for example.
3. It IS NOT too general/global
Example 1: “Disease X results from the expression of virulence genes.” Instead the hypothesis should focus on the expression of a particular gene or a set of genes.
Example 2: “Quantifying X will provide significant increases in income for industry.” This is essentially untestable in an experimental setup and is really a potential outcome, not a hypothesis.
4. It IS NOT too complex
Hypothesis statements that contain words like “and” and “or” are ‘compound hypotheses’. This makes testing difficult, because while one part may be true the other may not be so. When your hypothesis has multiple parts, make sure that your experiments directly test the entire hypothesis. Possible further implications that you cannot test should be discussed in Discussion.
5. It DOES NOT misdirect to the researcher
The hypothesis should not address your capabilities. “Discovering the mechanism behind X will enable us to better detect the pathogen.” This example tests the ability of the researchers to take information and use it; this is a result of successful hypothesis-driven research, not a testable hypothesis. Instead, the hypothesis should focus on the experimental system. If it is difficult to state the hypothesis without misdirecting to the researcher, the focus of the research may be discovery science or invention-based, and should be edited to incorporate a properly formulated hypothesis.
Please contact the JEI Editorial Staff at [email protected] if you have any questions regarding the hypothesis of your research.
- Resources Home 🏠
- Try SciSpace Copilot
- Search research papers
- Add Copilot Extension
- Try AI Detector
- Try Paraphraser
- Try Citation Generator
- April Papers
- June Papers
- July Papers

The Craft of Writing a Strong Hypothesis

Table of Contents
Writing a hypothesis is one of the essential elements of a scientific research paper. It needs to be to the point, clearly communicating what your research is trying to accomplish. A blurry, drawn-out, or complexly-structured hypothesis can confuse your readers. Or worse, the editor and peer reviewers.
A captivating hypothesis is not too intricate. This blog will take you through the process so that, by the end of it, you have a better idea of how to convey your research paper's intent in just one sentence.
What is a Hypothesis?
The first step in your scientific endeavor, a hypothesis, is a strong, concise statement that forms the basis of your research. It is not the same as a thesis statement , which is a brief summary of your research paper .
The sole purpose of a hypothesis is to predict your paper's findings, data, and conclusion. It comes from a place of curiosity and intuition . When you write a hypothesis, you're essentially making an educated guess based on scientific prejudices and evidence, which is further proven or disproven through the scientific method.
The reason for undertaking research is to observe a specific phenomenon. A hypothesis, therefore, lays out what the said phenomenon is. And it does so through two variables, an independent and dependent variable.
The independent variable is the cause behind the observation, while the dependent variable is the effect of the cause. A good example of this is “mixing red and blue forms purple.” In this hypothesis, mixing red and blue is the independent variable as you're combining the two colors at your own will. The formation of purple is the dependent variable as, in this case, it is conditional to the independent variable.
Different Types of Hypotheses

Types of hypotheses
Some would stand by the notion that there are only two types of hypotheses: a Null hypothesis and an Alternative hypothesis. While that may have some truth to it, it would be better to fully distinguish the most common forms as these terms come up so often, which might leave you out of context.
Apart from Null and Alternative, there are Complex, Simple, Directional, Non-Directional, Statistical, and Associative and casual hypotheses. They don't necessarily have to be exclusive, as one hypothesis can tick many boxes, but knowing the distinctions between them will make it easier for you to construct your own.
1. Null hypothesis
A null hypothesis proposes no relationship between two variables. Denoted by H 0 , it is a negative statement like “Attending physiotherapy sessions does not affect athletes' on-field performance.” Here, the author claims physiotherapy sessions have no effect on on-field performances. Even if there is, it's only a coincidence.
2. Alternative hypothesis
Considered to be the opposite of a null hypothesis, an alternative hypothesis is donated as H1 or Ha. It explicitly states that the dependent variable affects the independent variable. A good alternative hypothesis example is “Attending physiotherapy sessions improves athletes' on-field performance.” or “Water evaporates at 100 °C. ” The alternative hypothesis further branches into directional and non-directional.
- Directional hypothesis: A hypothesis that states the result would be either positive or negative is called directional hypothesis. It accompanies H1 with either the ‘<' or ‘>' sign.
- Non-directional hypothesis: A non-directional hypothesis only claims an effect on the dependent variable. It does not clarify whether the result would be positive or negative. The sign for a non-directional hypothesis is ‘≠.'
3. Simple hypothesis
A simple hypothesis is a statement made to reflect the relation between exactly two variables. One independent and one dependent. Consider the example, “Smoking is a prominent cause of lung cancer." The dependent variable, lung cancer, is dependent on the independent variable, smoking.
4. Complex hypothesis
In contrast to a simple hypothesis, a complex hypothesis implies the relationship between multiple independent and dependent variables. For instance, “Individuals who eat more fruits tend to have higher immunity, lesser cholesterol, and high metabolism.” The independent variable is eating more fruits, while the dependent variables are higher immunity, lesser cholesterol, and high metabolism.
5. Associative and casual hypothesis
Associative and casual hypotheses don't exhibit how many variables there will be. They define the relationship between the variables. In an associative hypothesis, changing any one variable, dependent or independent, affects others. In a casual hypothesis, the independent variable directly affects the dependent.
6. Empirical hypothesis
Also referred to as the working hypothesis, an empirical hypothesis claims a theory's validation via experiments and observation. This way, the statement appears justifiable and different from a wild guess.
Say, the hypothesis is “Women who take iron tablets face a lesser risk of anemia than those who take vitamin B12.” This is an example of an empirical hypothesis where the researcher the statement after assessing a group of women who take iron tablets and charting the findings.
7. Statistical hypothesis
The point of a statistical hypothesis is to test an already existing hypothesis by studying a population sample. Hypothesis like “44% of the Indian population belong in the age group of 22-27.” leverage evidence to prove or disprove a particular statement.
Characteristics of a Good Hypothesis
Writing a hypothesis is essential as it can make or break your research for you. That includes your chances of getting published in a journal. So when you're designing one, keep an eye out for these pointers:
- A research hypothesis has to be simple yet clear to look justifiable enough.
- It has to be testable — your research would be rendered pointless if too far-fetched into reality or limited by technology.
- It has to be precise about the results —what you are trying to do and achieve through it should come out in your hypothesis.
- A research hypothesis should be self-explanatory, leaving no doubt in the reader's mind.
- If you are developing a relational hypothesis, you need to include the variables and establish an appropriate relationship among them.
- A hypothesis must keep and reflect the scope for further investigations and experiments.
Separating a Hypothesis from a Prediction
Outside of academia, hypothesis and prediction are often used interchangeably. In research writing, this is not only confusing but also incorrect. And although a hypothesis and prediction are guesses at their core, there are many differences between them.
A hypothesis is an educated guess or even a testable prediction validated through research. It aims to analyze the gathered evidence and facts to define a relationship between variables and put forth a logical explanation behind the nature of events.
Predictions are assumptions or expected outcomes made without any backing evidence. They are more fictionally inclined regardless of where they originate from.
For this reason, a hypothesis holds much more weight than a prediction. It sticks to the scientific method rather than pure guesswork. "Planets revolve around the Sun." is an example of a hypothesis as it is previous knowledge and observed trends. Additionally, we can test it through the scientific method.
Whereas "COVID-19 will be eradicated by 2030." is a prediction. Even though it results from past trends, we can't prove or disprove it. So, the only way this gets validated is to wait and watch if COVID-19 cases end by 2030.
Finally, How to Write a Hypothesis
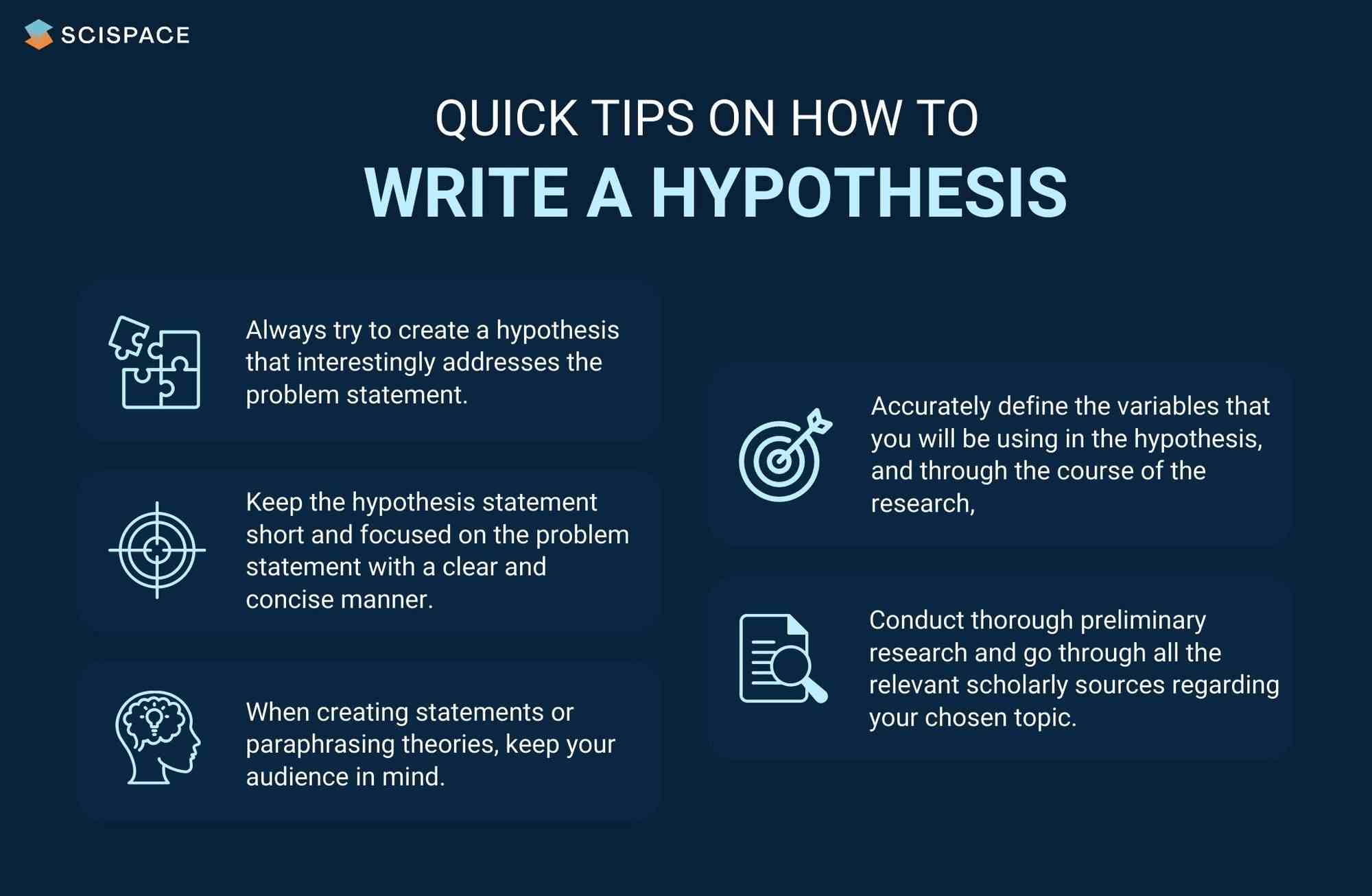
Quick tips on writing a hypothesis
1. Be clear about your research question
A hypothesis should instantly address the research question or the problem statement. To do so, you need to ask a question. Understand the constraints of your undertaken research topic and then formulate a simple and topic-centric problem. Only after that can you develop a hypothesis and further test for evidence.
2. Carry out a recce
Once you have your research's foundation laid out, it would be best to conduct preliminary research. Go through previous theories, academic papers, data, and experiments before you start curating your research hypothesis. It will give you an idea of your hypothesis's viability or originality.
Making use of references from relevant research papers helps draft a good research hypothesis. SciSpace Discover offers a repository of over 270 million research papers to browse through and gain a deeper understanding of related studies on a particular topic. Additionally, you can use SciSpace Copilot , your AI research assistant, for reading any lengthy research paper and getting a more summarized context of it. A hypothesis can be formed after evaluating many such summarized research papers. Copilot also offers explanations for theories and equations, explains paper in simplified version, allows you to highlight any text in the paper or clip math equations and tables and provides a deeper, clear understanding of what is being said. This can improve the hypothesis by helping you identify potential research gaps.
3. Create a 3-dimensional hypothesis
Variables are an essential part of any reasonable hypothesis. So, identify your independent and dependent variable(s) and form a correlation between them. The ideal way to do this is to write the hypothetical assumption in the ‘if-then' form. If you use this form, make sure that you state the predefined relationship between the variables.
In another way, you can choose to present your hypothesis as a comparison between two variables. Here, you must specify the difference you expect to observe in the results.
4. Write the first draft
Now that everything is in place, it's time to write your hypothesis. For starters, create the first draft. In this version, write what you expect to find from your research.
Clearly separate your independent and dependent variables and the link between them. Don't fixate on syntax at this stage. The goal is to ensure your hypothesis addresses the issue.
5. Proof your hypothesis
After preparing the first draft of your hypothesis, you need to inspect it thoroughly. It should tick all the boxes, like being concise, straightforward, relevant, and accurate. Your final hypothesis has to be well-structured as well.
Research projects are an exciting and crucial part of being a scholar. And once you have your research question, you need a great hypothesis to begin conducting research. Thus, knowing how to write a hypothesis is very important.
Now that you have a firmer grasp on what a good hypothesis constitutes, the different kinds there are, and what process to follow, you will find it much easier to write your hypothesis, which ultimately helps your research.
Now it's easier than ever to streamline your research workflow with SciSpace Discover . Its integrated, comprehensive end-to-end platform for research allows scholars to easily discover, write and publish their research and fosters collaboration.
It includes everything you need, including a repository of over 270 million research papers across disciplines, SEO-optimized summaries and public profiles to show your expertise and experience.
If you found these tips on writing a research hypothesis useful, head over to our blog on Statistical Hypothesis Testing to learn about the top researchers, papers, and institutions in this domain.
Frequently Asked Questions (FAQs)
1. what is the definition of hypothesis.
According to the Oxford dictionary, a hypothesis is defined as “An idea or explanation of something that is based on a few known facts, but that has not yet been proved to be true or correct”.
2. What is an example of hypothesis?
The hypothesis is a statement that proposes a relationship between two or more variables. An example: "If we increase the number of new users who join our platform by 25%, then we will see an increase in revenue."
3. What is an example of null hypothesis?
A null hypothesis is a statement that there is no relationship between two variables. The null hypothesis is written as H0. The null hypothesis states that there is no effect. For example, if you're studying whether or not a particular type of exercise increases strength, your null hypothesis will be "there is no difference in strength between people who exercise and people who don't."
4. What are the types of research?
• Fundamental research
• Applied research
• Qualitative research
• Quantitative research
• Mixed research
• Exploratory research
• Longitudinal research
• Cross-sectional research
• Field research
• Laboratory research
• Fixed research
• Flexible research
• Action research
• Policy research
• Classification research
• Comparative research
• Causal research
• Inductive research
• Deductive research
5. How to write a hypothesis?
• Your hypothesis should be able to predict the relationship and outcome.
• Avoid wordiness by keeping it simple and brief.
• Your hypothesis should contain observable and testable outcomes.
• Your hypothesis should be relevant to the research question.
6. What are the 2 types of hypothesis?
• Null hypotheses are used to test the claim that "there is no difference between two groups of data".
• Alternative hypotheses test the claim that "there is a difference between two data groups".
7. Difference between research question and research hypothesis?
A research question is a broad, open-ended question you will try to answer through your research. A hypothesis is a statement based on prior research or theory that you expect to be true due to your study. Example - Research question: What are the factors that influence the adoption of the new technology? Research hypothesis: There is a positive relationship between age, education and income level with the adoption of the new technology.
8. What is plural for hypothesis?
The plural of hypothesis is hypotheses. Here's an example of how it would be used in a statement, "Numerous well-considered hypotheses are presented in this part, and they are supported by tables and figures that are well-illustrated."
9. What is the red queen hypothesis?
The red queen hypothesis in evolutionary biology states that species must constantly evolve to avoid extinction because if they don't, they will be outcompeted by other species that are evolving. Leigh Van Valen first proposed it in 1973; since then, it has been tested and substantiated many times.
10. Who is known as the father of null hypothesis?
The father of the null hypothesis is Sir Ronald Fisher. He published a paper in 1925 that introduced the concept of null hypothesis testing, and he was also the first to use the term itself.
11. When to reject null hypothesis?
You need to find a significant difference between your two populations to reject the null hypothesis. You can determine that by running statistical tests such as an independent sample t-test or a dependent sample t-test. You should reject the null hypothesis if the p-value is less than 0.05.

You might also like

Consensus GPT vs. SciSpace GPT: Choose the Best GPT for Research
Literature Review and Theoretical Framework: Understanding the Differences

Types of Essays in Academic Writing - Quick Guide (2024)
Have a language expert improve your writing
Run a free plagiarism check in 10 minutes, generate accurate citations for free.
- Knowledge Base
Hypothesis Testing | A Step-by-Step Guide with Easy Examples
Published on November 8, 2019 by Rebecca Bevans . Revised on June 22, 2023.
Hypothesis testing is a formal procedure for investigating our ideas about the world using statistics . It is most often used by scientists to test specific predictions, called hypotheses, that arise from theories.
There are 5 main steps in hypothesis testing:
- State your research hypothesis as a null hypothesis and alternate hypothesis (H o ) and (H a or H 1 ).
- Collect data in a way designed to test the hypothesis.
- Perform an appropriate statistical test .
- Decide whether to reject or fail to reject your null hypothesis.
- Present the findings in your results and discussion section.
Though the specific details might vary, the procedure you will use when testing a hypothesis will always follow some version of these steps.
Table of contents
Step 1: state your null and alternate hypothesis, step 2: collect data, step 3: perform a statistical test, step 4: decide whether to reject or fail to reject your null hypothesis, step 5: present your findings, other interesting articles, frequently asked questions about hypothesis testing.
After developing your initial research hypothesis (the prediction that you want to investigate), it is important to restate it as a null (H o ) and alternate (H a ) hypothesis so that you can test it mathematically.
The alternate hypothesis is usually your initial hypothesis that predicts a relationship between variables. The null hypothesis is a prediction of no relationship between the variables you are interested in.
- H 0 : Men are, on average, not taller than women. H a : Men are, on average, taller than women.
Prevent plagiarism. Run a free check.
For a statistical test to be valid , it is important to perform sampling and collect data in a way that is designed to test your hypothesis. If your data are not representative, then you cannot make statistical inferences about the population you are interested in.
There are a variety of statistical tests available, but they are all based on the comparison of within-group variance (how spread out the data is within a category) versus between-group variance (how different the categories are from one another).
If the between-group variance is large enough that there is little or no overlap between groups, then your statistical test will reflect that by showing a low p -value . This means it is unlikely that the differences between these groups came about by chance.
Alternatively, if there is high within-group variance and low between-group variance, then your statistical test will reflect that with a high p -value. This means it is likely that any difference you measure between groups is due to chance.
Your choice of statistical test will be based on the type of variables and the level of measurement of your collected data .
- an estimate of the difference in average height between the two groups.
- a p -value showing how likely you are to see this difference if the null hypothesis of no difference is true.
Based on the outcome of your statistical test, you will have to decide whether to reject or fail to reject your null hypothesis.
In most cases you will use the p -value generated by your statistical test to guide your decision. And in most cases, your predetermined level of significance for rejecting the null hypothesis will be 0.05 – that is, when there is a less than 5% chance that you would see these results if the null hypothesis were true.
In some cases, researchers choose a more conservative level of significance, such as 0.01 (1%). This minimizes the risk of incorrectly rejecting the null hypothesis ( Type I error ).
Receive feedback on language, structure, and formatting
Professional editors proofread and edit your paper by focusing on:
- Academic style
- Vague sentences
- Style consistency
See an example
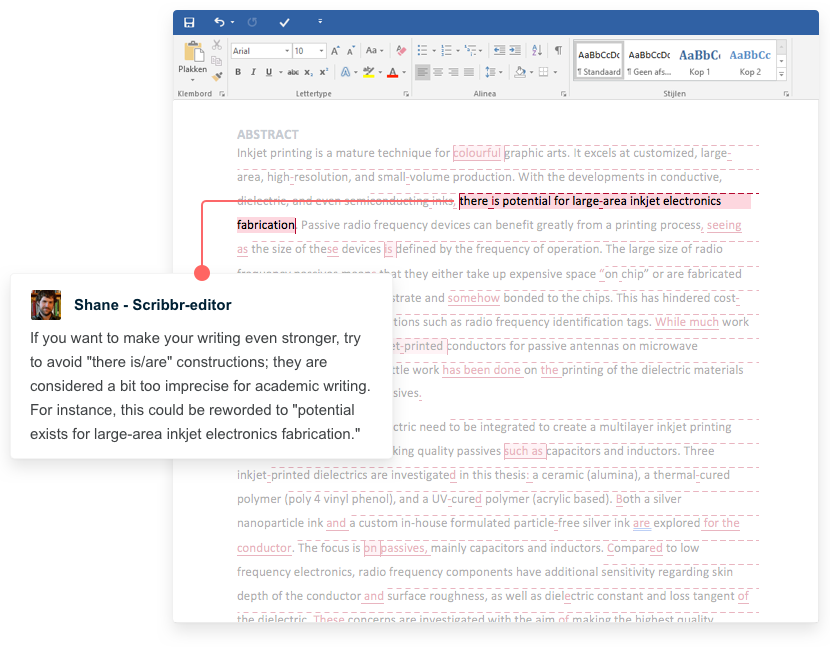
The results of hypothesis testing will be presented in the results and discussion sections of your research paper , dissertation or thesis .
In the results section you should give a brief summary of the data and a summary of the results of your statistical test (for example, the estimated difference between group means and associated p -value). In the discussion , you can discuss whether your initial hypothesis was supported by your results or not.
In the formal language of hypothesis testing, we talk about rejecting or failing to reject the null hypothesis. You will probably be asked to do this in your statistics assignments.
However, when presenting research results in academic papers we rarely talk this way. Instead, we go back to our alternate hypothesis (in this case, the hypothesis that men are on average taller than women) and state whether the result of our test did or did not support the alternate hypothesis.
If your null hypothesis was rejected, this result is interpreted as “supported the alternate hypothesis.”
These are superficial differences; you can see that they mean the same thing.
You might notice that we don’t say that we reject or fail to reject the alternate hypothesis . This is because hypothesis testing is not designed to prove or disprove anything. It is only designed to test whether a pattern we measure could have arisen spuriously, or by chance.
If we reject the null hypothesis based on our research (i.e., we find that it is unlikely that the pattern arose by chance), then we can say our test lends support to our hypothesis . But if the pattern does not pass our decision rule, meaning that it could have arisen by chance, then we say the test is inconsistent with our hypothesis .
If you want to know more about statistics , methodology , or research bias , make sure to check out some of our other articles with explanations and examples.
- Normal distribution
- Descriptive statistics
- Measures of central tendency
- Correlation coefficient
Methodology
- Cluster sampling
- Stratified sampling
- Types of interviews
- Cohort study
- Thematic analysis
Research bias
- Implicit bias
- Cognitive bias
- Survivorship bias
- Availability heuristic
- Nonresponse bias
- Regression to the mean
Hypothesis testing is a formal procedure for investigating our ideas about the world using statistics. It is used by scientists to test specific predictions, called hypotheses , by calculating how likely it is that a pattern or relationship between variables could have arisen by chance.
A hypothesis states your predictions about what your research will find. It is a tentative answer to your research question that has not yet been tested. For some research projects, you might have to write several hypotheses that address different aspects of your research question.
A hypothesis is not just a guess — it should be based on existing theories and knowledge. It also has to be testable, which means you can support or refute it through scientific research methods (such as experiments, observations and statistical analysis of data).
Null and alternative hypotheses are used in statistical hypothesis testing . The null hypothesis of a test always predicts no effect or no relationship between variables, while the alternative hypothesis states your research prediction of an effect or relationship.
Cite this Scribbr article
If you want to cite this source, you can copy and paste the citation or click the “Cite this Scribbr article” button to automatically add the citation to our free Citation Generator.
Bevans, R. (2023, June 22). Hypothesis Testing | A Step-by-Step Guide with Easy Examples. Scribbr. Retrieved July 10, 2024, from https://www.scribbr.com/statistics/hypothesis-testing/
Is this article helpful?
Rebecca Bevans
Other students also liked, choosing the right statistical test | types & examples, understanding p values | definition and examples, what is your plagiarism score.

What Is A Research (Scientific) Hypothesis? A plain-language explainer + examples
By: Derek Jansen (MBA) | Reviewed By: Dr Eunice Rautenbach | June 2020
If you’re new to the world of research, or it’s your first time writing a dissertation or thesis, you’re probably noticing that the words “research hypothesis” and “scientific hypothesis” are used quite a bit, and you’re wondering what they mean in a research context .
“Hypothesis” is one of those words that people use loosely, thinking they understand what it means. However, it has a very specific meaning within academic research. So, it’s important to understand the exact meaning before you start hypothesizing.
Research Hypothesis 101
- What is a hypothesis ?
- What is a research hypothesis (scientific hypothesis)?
- Requirements for a research hypothesis
- Definition of a research hypothesis
- The null hypothesis
What is a hypothesis?
Let’s start with the general definition of a hypothesis (not a research hypothesis or scientific hypothesis), according to the Cambridge Dictionary:
Hypothesis: an idea or explanation for something that is based on known facts but has not yet been proved.
In other words, it’s a statement that provides an explanation for why or how something works, based on facts (or some reasonable assumptions), but that has not yet been specifically tested . For example, a hypothesis might look something like this:
Hypothesis: sleep impacts academic performance.
This statement predicts that academic performance will be influenced by the amount and/or quality of sleep a student engages in – sounds reasonable, right? It’s based on reasonable assumptions , underpinned by what we currently know about sleep and health (from the existing literature). So, loosely speaking, we could call it a hypothesis, at least by the dictionary definition.
But that’s not good enough…
Unfortunately, that’s not quite sophisticated enough to describe a research hypothesis (also sometimes called a scientific hypothesis), and it wouldn’t be acceptable in a dissertation, thesis or research paper . In the world of academic research, a statement needs a few more criteria to constitute a true research hypothesis .
What is a research hypothesis?
A research hypothesis (also called a scientific hypothesis) is a statement about the expected outcome of a study (for example, a dissertation or thesis). To constitute a quality hypothesis, the statement needs to have three attributes – specificity , clarity and testability .
Let’s take a look at these more closely.
Need a helping hand?
Hypothesis Essential #1: Specificity & Clarity
A good research hypothesis needs to be extremely clear and articulate about both what’ s being assessed (who or what variables are involved ) and the expected outcome (for example, a difference between groups, a relationship between variables, etc.).
Let’s stick with our sleepy students example and look at how this statement could be more specific and clear.
Hypothesis: Students who sleep at least 8 hours per night will, on average, achieve higher grades in standardised tests than students who sleep less than 8 hours a night.
As you can see, the statement is very specific as it identifies the variables involved (sleep hours and test grades), the parties involved (two groups of students), as well as the predicted relationship type (a positive relationship). There’s no ambiguity or uncertainty about who or what is involved in the statement, and the expected outcome is clear.
Contrast that to the original hypothesis we looked at – “Sleep impacts academic performance” – and you can see the difference. “Sleep” and “academic performance” are both comparatively vague , and there’s no indication of what the expected relationship direction is (more sleep or less sleep). As you can see, specificity and clarity are key.
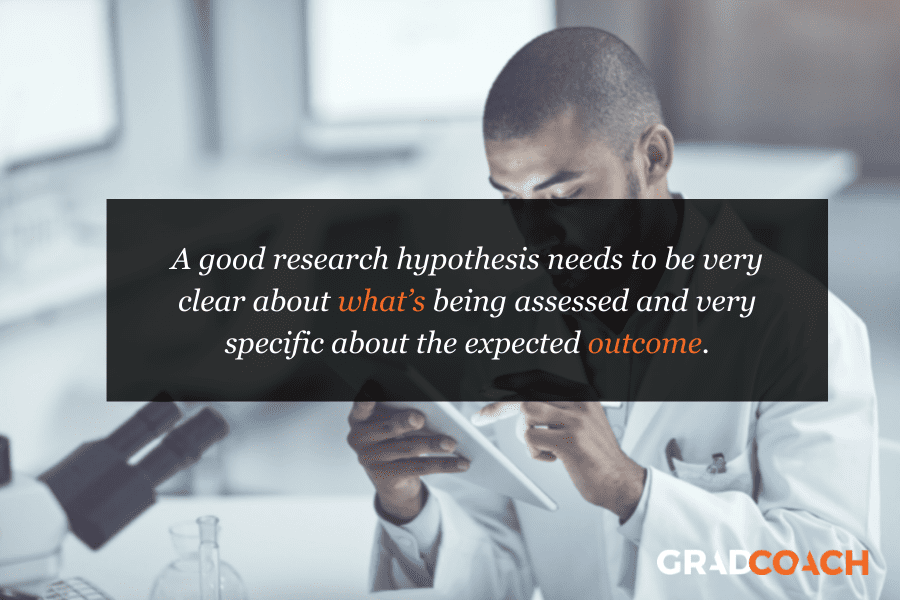
Hypothesis Essential #2: Testability (Provability)
A statement must be testable to qualify as a research hypothesis. In other words, there needs to be a way to prove (or disprove) the statement. If it’s not testable, it’s not a hypothesis – simple as that.
For example, consider the hypothesis we mentioned earlier:
Hypothesis: Students who sleep at least 8 hours per night will, on average, achieve higher grades in standardised tests than students who sleep less than 8 hours a night.
We could test this statement by undertaking a quantitative study involving two groups of students, one that gets 8 or more hours of sleep per night for a fixed period, and one that gets less. We could then compare the standardised test results for both groups to see if there’s a statistically significant difference.
Again, if you compare this to the original hypothesis we looked at – “Sleep impacts academic performance” – you can see that it would be quite difficult to test that statement, primarily because it isn’t specific enough. How much sleep? By who? What type of academic performance?
So, remember the mantra – if you can’t test it, it’s not a hypothesis 🙂
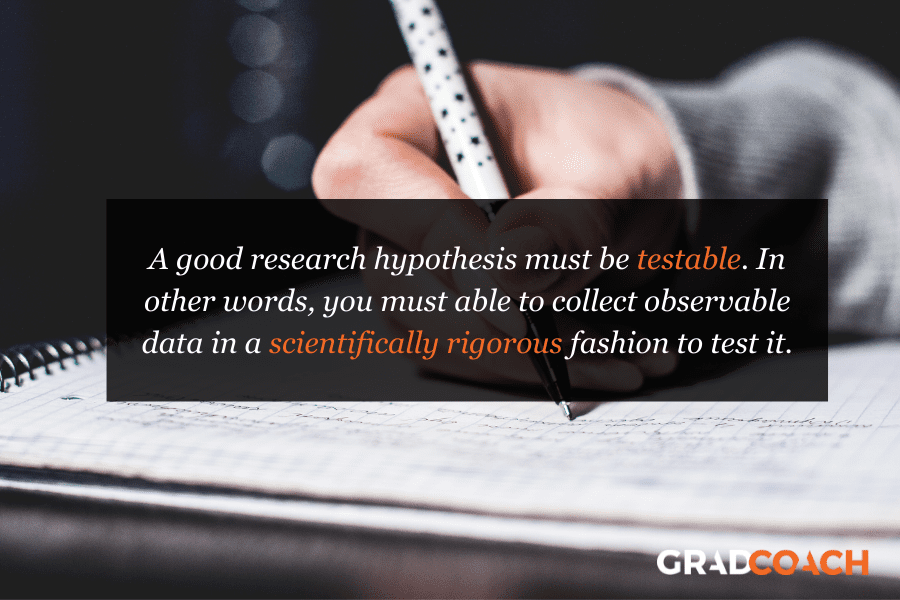
Defining A Research Hypothesis
You’re still with us? Great! Let’s recap and pin down a clear definition of a hypothesis.
A research hypothesis (or scientific hypothesis) is a statement about an expected relationship between variables, or explanation of an occurrence, that is clear, specific and testable.
So, when you write up hypotheses for your dissertation or thesis, make sure that they meet all these criteria. If you do, you’ll not only have rock-solid hypotheses but you’ll also ensure a clear focus for your entire research project.
What about the null hypothesis?
You may have also heard the terms null hypothesis , alternative hypothesis, or H-zero thrown around. At a simple level, the null hypothesis is the counter-proposal to the original hypothesis.
For example, if the hypothesis predicts that there is a relationship between two variables (for example, sleep and academic performance), the null hypothesis would predict that there is no relationship between those variables.
At a more technical level, the null hypothesis proposes that no statistical significance exists in a set of given observations and that any differences are due to chance alone.
And there you have it – hypotheses in a nutshell.
If you have any questions, be sure to leave a comment below and we’ll do our best to help you. If you need hands-on help developing and testing your hypotheses, consider our private coaching service , where we hold your hand through the research journey.
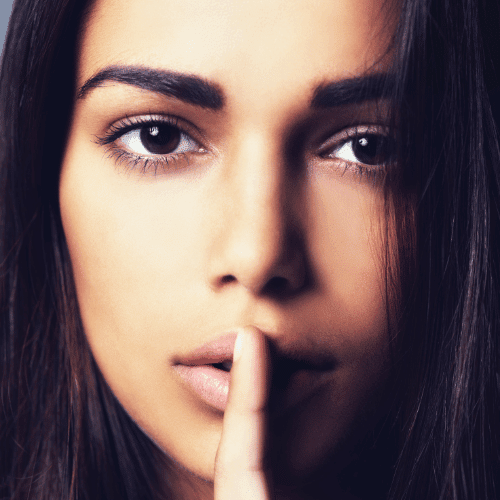
Psst... there’s more!
This post was based on one of our popular Research Bootcamps . If you're working on a research project, you'll definitely want to check this out ...
16 Comments
Very useful information. I benefit more from getting more information in this regard.
Very great insight,educative and informative. Please give meet deep critics on many research data of public international Law like human rights, environment, natural resources, law of the sea etc
In a book I read a distinction is made between null, research, and alternative hypothesis. As far as I understand, alternative and research hypotheses are the same. Can you please elaborate? Best Afshin
This is a self explanatory, easy going site. I will recommend this to my friends and colleagues.
Very good definition. How can I cite your definition in my thesis? Thank you. Is nul hypothesis compulsory in a research?
It’s a counter-proposal to be proven as a rejection
Please what is the difference between alternate hypothesis and research hypothesis?
It is a very good explanation. However, it limits hypotheses to statistically tasteable ideas. What about for qualitative researches or other researches that involve quantitative data that don’t need statistical tests?
In qualitative research, one typically uses propositions, not hypotheses.
could you please elaborate it more
I’ve benefited greatly from these notes, thank you.
This is very helpful
well articulated ideas are presented here, thank you for being reliable sources of information
Excellent. Thanks for being clear and sound about the research methodology and hypothesis (quantitative research)
I have only a simple question regarding the null hypothesis. – Is the null hypothesis (Ho) known as the reversible hypothesis of the alternative hypothesis (H1? – How to test it in academic research?
this is very important note help me much more
Trackbacks/Pingbacks
- What Is Research Methodology? Simple Definition (With Examples) - Grad Coach - […] Contrasted to this, a quantitative methodology is typically used when the research aims and objectives are confirmatory in nature. For example,…
Submit a Comment Cancel reply
Your email address will not be published. Required fields are marked *
Save my name, email, and website in this browser for the next time I comment.
- Print Friendly
- Bipolar Disorder
- Therapy Center
- When To See a Therapist
- Types of Therapy
- Best Online Therapy
- Best Couples Therapy
- Best Family Therapy
- Managing Stress
- Sleep and Dreaming
- Understanding Emotions
- Self-Improvement
- Healthy Relationships
- Student Resources
- Personality Types
- Guided Meditations
- Verywell Mind Insights
- 2024 Verywell Mind 25
- Mental Health in the Classroom
- Editorial Process
- Meet Our Review Board
- Crisis Support
How to Write a Great Hypothesis
Hypothesis Definition, Format, Examples, and Tips
Kendra Cherry, MS, is a psychosocial rehabilitation specialist, psychology educator, and author of the "Everything Psychology Book."
:max_bytes(150000):strip_icc():format(webp)/IMG_9791-89504ab694d54b66bbd72cb84ffb860e.jpg)
Amy Morin, LCSW, is a psychotherapist and international bestselling author. Her books, including "13 Things Mentally Strong People Don't Do," have been translated into more than 40 languages. Her TEDx talk, "The Secret of Becoming Mentally Strong," is one of the most viewed talks of all time.
:max_bytes(150000):strip_icc():format(webp)/VW-MIND-Amy-2b338105f1ee493f94d7e333e410fa76.jpg)
Verywell / Alex Dos Diaz
- The Scientific Method
Hypothesis Format
Falsifiability of a hypothesis.
- Operationalization
Hypothesis Types
Hypotheses examples.
- Collecting Data
A hypothesis is a tentative statement about the relationship between two or more variables. It is a specific, testable prediction about what you expect to happen in a study. It is a preliminary answer to your question that helps guide the research process.
Consider a study designed to examine the relationship between sleep deprivation and test performance. The hypothesis might be: "This study is designed to assess the hypothesis that sleep-deprived people will perform worse on a test than individuals who are not sleep-deprived."
At a Glance
A hypothesis is crucial to scientific research because it offers a clear direction for what the researchers are looking to find. This allows them to design experiments to test their predictions and add to our scientific knowledge about the world. This article explores how a hypothesis is used in psychology research, how to write a good hypothesis, and the different types of hypotheses you might use.
The Hypothesis in the Scientific Method
In the scientific method , whether it involves research in psychology, biology, or some other area, a hypothesis represents what the researchers think will happen in an experiment. The scientific method involves the following steps:
- Forming a question
- Performing background research
- Creating a hypothesis
- Designing an experiment
- Collecting data
- Analyzing the results
- Drawing conclusions
- Communicating the results
The hypothesis is a prediction, but it involves more than a guess. Most of the time, the hypothesis begins with a question which is then explored through background research. At this point, researchers then begin to develop a testable hypothesis.
Unless you are creating an exploratory study, your hypothesis should always explain what you expect to happen.
In a study exploring the effects of a particular drug, the hypothesis might be that researchers expect the drug to have some type of effect on the symptoms of a specific illness. In psychology, the hypothesis might focus on how a certain aspect of the environment might influence a particular behavior.
Remember, a hypothesis does not have to be correct. While the hypothesis predicts what the researchers expect to see, the goal of the research is to determine whether this guess is right or wrong. When conducting an experiment, researchers might explore numerous factors to determine which ones might contribute to the ultimate outcome.
In many cases, researchers may find that the results of an experiment do not support the original hypothesis. When writing up these results, the researchers might suggest other options that should be explored in future studies.
In many cases, researchers might draw a hypothesis from a specific theory or build on previous research. For example, prior research has shown that stress can impact the immune system. So a researcher might hypothesize: "People with high-stress levels will be more likely to contract a common cold after being exposed to the virus than people who have low-stress levels."
In other instances, researchers might look at commonly held beliefs or folk wisdom. "Birds of a feather flock together" is one example of folk adage that a psychologist might try to investigate. The researcher might pose a specific hypothesis that "People tend to select romantic partners who are similar to them in interests and educational level."
Elements of a Good Hypothesis
So how do you write a good hypothesis? When trying to come up with a hypothesis for your research or experiments, ask yourself the following questions:
- Is your hypothesis based on your research on a topic?
- Can your hypothesis be tested?
- Does your hypothesis include independent and dependent variables?
Before you come up with a specific hypothesis, spend some time doing background research. Once you have completed a literature review, start thinking about potential questions you still have. Pay attention to the discussion section in the journal articles you read . Many authors will suggest questions that still need to be explored.
How to Formulate a Good Hypothesis
To form a hypothesis, you should take these steps:
- Collect as many observations about a topic or problem as you can.
- Evaluate these observations and look for possible causes of the problem.
- Create a list of possible explanations that you might want to explore.
- After you have developed some possible hypotheses, think of ways that you could confirm or disprove each hypothesis through experimentation. This is known as falsifiability.
In the scientific method , falsifiability is an important part of any valid hypothesis. In order to test a claim scientifically, it must be possible that the claim could be proven false.
Students sometimes confuse the idea of falsifiability with the idea that it means that something is false, which is not the case. What falsifiability means is that if something was false, then it is possible to demonstrate that it is false.
One of the hallmarks of pseudoscience is that it makes claims that cannot be refuted or proven false.
The Importance of Operational Definitions
A variable is a factor or element that can be changed and manipulated in ways that are observable and measurable. However, the researcher must also define how the variable will be manipulated and measured in the study.
Operational definitions are specific definitions for all relevant factors in a study. This process helps make vague or ambiguous concepts detailed and measurable.
For example, a researcher might operationally define the variable " test anxiety " as the results of a self-report measure of anxiety experienced during an exam. A "study habits" variable might be defined by the amount of studying that actually occurs as measured by time.
These precise descriptions are important because many things can be measured in various ways. Clearly defining these variables and how they are measured helps ensure that other researchers can replicate your results.
Replicability
One of the basic principles of any type of scientific research is that the results must be replicable.
Replication means repeating an experiment in the same way to produce the same results. By clearly detailing the specifics of how the variables were measured and manipulated, other researchers can better understand the results and repeat the study if needed.
Some variables are more difficult than others to define. For example, how would you operationally define a variable such as aggression ? For obvious ethical reasons, researchers cannot create a situation in which a person behaves aggressively toward others.
To measure this variable, the researcher must devise a measurement that assesses aggressive behavior without harming others. The researcher might utilize a simulated task to measure aggressiveness in this situation.
Hypothesis Checklist
- Does your hypothesis focus on something that you can actually test?
- Does your hypothesis include both an independent and dependent variable?
- Can you manipulate the variables?
- Can your hypothesis be tested without violating ethical standards?
The hypothesis you use will depend on what you are investigating and hoping to find. Some of the main types of hypotheses that you might use include:
- Simple hypothesis : This type of hypothesis suggests there is a relationship between one independent variable and one dependent variable.
- Complex hypothesis : This type suggests a relationship between three or more variables, such as two independent and dependent variables.
- Null hypothesis : This hypothesis suggests no relationship exists between two or more variables.
- Alternative hypothesis : This hypothesis states the opposite of the null hypothesis.
- Statistical hypothesis : This hypothesis uses statistical analysis to evaluate a representative population sample and then generalizes the findings to the larger group.
- Logical hypothesis : This hypothesis assumes a relationship between variables without collecting data or evidence.
A hypothesis often follows a basic format of "If {this happens} then {this will happen}." One way to structure your hypothesis is to describe what will happen to the dependent variable if you change the independent variable .
The basic format might be: "If {these changes are made to a certain independent variable}, then we will observe {a change in a specific dependent variable}."
A few examples of simple hypotheses:
- "Students who eat breakfast will perform better on a math exam than students who do not eat breakfast."
- "Students who experience test anxiety before an English exam will get lower scores than students who do not experience test anxiety."
- "Motorists who talk on the phone while driving will be more likely to make errors on a driving course than those who do not talk on the phone."
- "Children who receive a new reading intervention will have higher reading scores than students who do not receive the intervention."
Examples of a complex hypothesis include:
- "People with high-sugar diets and sedentary activity levels are more likely to develop depression."
- "Younger people who are regularly exposed to green, outdoor areas have better subjective well-being than older adults who have limited exposure to green spaces."
Examples of a null hypothesis include:
- "There is no difference in anxiety levels between people who take St. John's wort supplements and those who do not."
- "There is no difference in scores on a memory recall task between children and adults."
- "There is no difference in aggression levels between children who play first-person shooter games and those who do not."
Examples of an alternative hypothesis:
- "People who take St. John's wort supplements will have less anxiety than those who do not."
- "Adults will perform better on a memory task than children."
- "Children who play first-person shooter games will show higher levels of aggression than children who do not."
Collecting Data on Your Hypothesis
Once a researcher has formed a testable hypothesis, the next step is to select a research design and start collecting data. The research method depends largely on exactly what they are studying. There are two basic types of research methods: descriptive research and experimental research.
Descriptive Research Methods
Descriptive research such as case studies , naturalistic observations , and surveys are often used when conducting an experiment is difficult or impossible. These methods are best used to describe different aspects of a behavior or psychological phenomenon.
Once a researcher has collected data using descriptive methods, a correlational study can examine how the variables are related. This research method might be used to investigate a hypothesis that is difficult to test experimentally.
Experimental Research Methods
Experimental methods are used to demonstrate causal relationships between variables. In an experiment, the researcher systematically manipulates a variable of interest (known as the independent variable) and measures the effect on another variable (known as the dependent variable).
Unlike correlational studies, which can only be used to determine if there is a relationship between two variables, experimental methods can be used to determine the actual nature of the relationship—whether changes in one variable actually cause another to change.
The hypothesis is a critical part of any scientific exploration. It represents what researchers expect to find in a study or experiment. In situations where the hypothesis is unsupported by the research, the research still has value. Such research helps us better understand how different aspects of the natural world relate to one another. It also helps us develop new hypotheses that can then be tested in the future.
Thompson WH, Skau S. On the scope of scientific hypotheses . R Soc Open Sci . 2023;10(8):230607. doi:10.1098/rsos.230607
Taran S, Adhikari NKJ, Fan E. Falsifiability in medicine: what clinicians can learn from Karl Popper [published correction appears in Intensive Care Med. 2021 Jun 17;:]. Intensive Care Med . 2021;47(9):1054-1056. doi:10.1007/s00134-021-06432-z
Eyler AA. Research Methods for Public Health . 1st ed. Springer Publishing Company; 2020. doi:10.1891/9780826182067.0004
Nosek BA, Errington TM. What is replication ? PLoS Biol . 2020;18(3):e3000691. doi:10.1371/journal.pbio.3000691
Aggarwal R, Ranganathan P. Study designs: Part 2 - Descriptive studies . Perspect Clin Res . 2019;10(1):34-36. doi:10.4103/picr.PICR_154_18
Nevid J. Psychology: Concepts and Applications. Wadworth, 2013.
By Kendra Cherry, MSEd Kendra Cherry, MS, is a psychosocial rehabilitation specialist, psychology educator, and author of the "Everything Psychology Book."
Society Homepage About Public Health Policy Contact
Data-driven hypothesis generation in clinical research: what we learned from a human subject study, article sidebar.
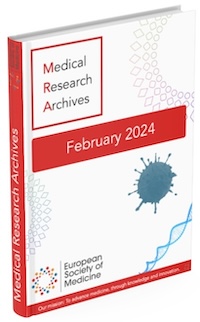
Submit your own article
Register as an author to reserve your spot in the next issue of the Medical Research Archives.
Join the Society
The European Society of Medicine is more than a professional association. We are a community. Our members work in countries across the globe, yet are united by a common goal: to promote health and health equity, around the world.
Join Europe’s leading medical society and discover the many advantages of membership, including free article publication.
Main Article Content
Hypothesis generation is an early and critical step in any hypothesis-driven clinical research project. Because it is not yet a well-understood cognitive process, the need to improve the process goes unrecognized. Without an impactful hypothesis, the significance of any research project can be questionable, regardless of the rigor or diligence applied in other steps of the study, e.g., study design, data collection, and result analysis. In this perspective article, the authors provide a literature review on the following topics first: scientific thinking, reasoning, medical reasoning, literature-based discovery, and a field study to explore scientific thinking and discovery. Over the years, scientific thinking has shown excellent progress in cognitive science and its applied areas: education, medicine, and biomedical research. However, a review of the literature reveals the lack of original studies on hypothesis generation in clinical research. The authors then summarize their first human participant study exploring data-driven hypothesis generation by clinical researchers in a simulated setting. The results indicate that a secondary data analytical tool, VIADS—a visual interactive analytic tool for filtering, summarizing, and visualizing large health data sets coded with hierarchical terminologies, can shorten the time participants need, on average, to generate a hypothesis and also requires fewer cognitive events to generate each hypothesis. As a counterpoint, this exploration also indicates that the quality ratings of the hypotheses thus generated carry significantly lower ratings for feasibility when applying VIADS. Despite its small scale, the study confirmed the feasibility of conducting a human participant study directly to explore the hypothesis generation process in clinical research. This study provides supporting evidence to conduct a larger-scale study with a specifically designed tool to facilitate the hypothesis-generation process among inexperienced clinical researchers. A larger study could provide generalizable evidence, which in turn can potentially improve clinical research productivity and overall clinical research enterprise.
Article Details
The Medical Research Archives grants authors the right to publish and reproduce the unrevised contribution in whole or in part at any time and in any form for any scholarly non-commercial purpose with the condition that all publications of the contribution include a full citation to the journal as published by the Medical Research Archives .
Hypothesis-driven science in large-scale studies: the case of GWAS
- Open access
- Published: 19 September 2021
- Volume 36 , article number 46 , ( 2021 )
Cite this article
You have full access to this open access article
- James Read ORCID: orcid.org/0000-0003-2226-0340 1 &
- Sumana Sharma ORCID: orcid.org/0000-0003-0598-2181 2
6804 Accesses
1 Altmetric
Explore all metrics
It is now well-appreciated by philosophers that contemporary large-scale ‘-omics’ studies in biology stand in non-trivial relationships to more orthodox hypothesis-driven approaches. These relationships have been clarified by Ratti ( 2015 ); however, there remains much more to be said regarding how an important field of genomics cited in that work—‘genome-wide association studies’ (GWAS)—fits into this framework. In the present article, we propose a revision to Ratti’s framework more suited to studies such as GWAS. In the process of doing so, we introduce to the philosophical literature novel exploratory experiments in (phospho)proteomics, and demonstrate how these experiments interplay with the above considerations.
Similar content being viewed by others
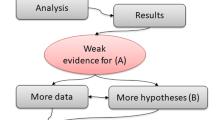
Raiders of the lost HARK: a reproducible inference framework for big data science
Experimental Discovery, Data Models, and Mechanisms in Biology: An Example from Mendel’s Work
The Importance of Being Dynamic: Systems Biology Beyond the Hairball
Avoid common mistakes on your manuscript.
Introduction
The fields of molecular biology and genetics were transformed upon completion in 2001 of the Human Genome Project (Lander et al. 2001 ). This provided for the first time near-complete information on the genetic makeup of human beings, and marked the advent of what has become known as the ‘post-genomics’ era, defined by the availability of large-scale data sets derived from ‘genome-scale’ approaches. In turn, this has led to a shift in biological methodology, from carefully constructed hypothesis-driven research, to unbiased data-driven approaches, sometimes called ‘-omics’ studies. These studies have attracted philosophical interest in recent years: see e.g. Burian ( 2007 ); O’Malley et al. ( 2010 ); Ratti ( 2015 ); for more general philosophical discussions of large-scale data-driven approaches in contemporary post-genomics biology, see e.g. Leonelli ( 2016 ); Richardson and Stevens ( 2015 ).
Recall that -omics studies fall into three main categories: ‘genomics’, ‘transcriptomics’, and ‘proteomics’. The salient features of these three categories as as follows (we make no claim that these features exhaust any of the three categories; they are, however, the features which are relevant to the present article). Genomics is the study of the complete set of genes (composed of DNA) inside a cell. Cellular processes lead to genetic information being transcribed (copied) into molecules known as RNA. ‘Messenger RNA’ (mRNA) carries information corresponding to the genetic sequence of a gene. Transcriptomics is the study of the complete set of RNA transcripts that are produced by the genome. Finally, the information encoded in mRNA is used by cellular machinery called ribosomes to construct proteins; proteomics is the systematic study of these proteins within a cell. Proteins are the ultimate workhorses of the cell; proteomics studies aim to characterise cellular functions mediated by protein networks, in which nodes represent proteins and edges represent physical/functional interactions between them. For further background on genomics, transcriptomics, and proteomics, see Hasin et al. ( 2017 ).
Large-scale -omics studies are often described as being ‘hypothesis-free’. To take one example from genomics: advances in genome-editing techniques mean that it is now possible to generate ‘loss-of-function’ mutants in the laboratory. Such mutations are inactivating in the sense that they lead to the loss in the function of a gene within a cell. In the last few years, CRISPR-Cas9 technology has emerged, which makes it possible to create targetted loss-of-function mutants for any of the nearly 20,000 genes in the human genome (Doudna and Charpentier 2014 ). This allows researchers to ‘screen’ for a gene the loss of which leads to the phenotype of interest, thereby identifying the function of that gene. The methodological idea behind such screening approaches is that one does not require any background hypothesis as to which gene could be involved in a particular biological process, or associated with a particular phenotype: hence the widespread declaration that such approaches are ‘hypothesis-free’ (Shalem et al. 2015 ). As Burian writes, “Genomics, proteomics, and related “omics” disciplines represent a break with the ideal of hypothesis-driven science” (Burian 2007 , p. 289).
With Ratti ( 2015 ); Franklin ( 2005 ), and others, we find the terminology of ‘hypothesis-free’ to be misleading—for, in fact, such large-scale studies exhibit a Janus-faced dependence on mechanistic hypotheses of a quite standard sort. Ratti characterises such studies, and their connections with more orthodox mechanistic hypothesis-driven science, as involving three steps:
1. The generation of a preliminary set of hypotheses from an established set of premises; 2. The prioritization of some hypotheses and discarding of others by means of other premises and new evidence; 3. The search for more stringent evidence for prioritized hypotheses. (Ratti 2015 , p. 201)
In step (1), scientific hypothesising plays a role, insofar as it is used to delimit the domain of inquiry of the study. For example, a loss-of-function screen to identify the receptor for a pathogen would hypothesise that there exists a non-redundant mechanism for the interaction of the pathogen with the cells, and that the loss of this cellular factor/mechanism would lead to diminution of interaction of the pathogen with the cell surface. For the purpose of the test, such hypotheses are regarded as indubitable: they delimit the range of acceptable empirical enquiry. But there is also a forward-looking dependence of these approaches on scientific hypothesising: the results of such studies can be used to generate more specific mechanistic hypotheses, certain of which are prioritised in step (2) (based on certain additional assumptions—e.g., that there is a single cellular factor/mechanism responsible for pathogen-cell interaction in the above example), and which can then be validated in downstream analysis in step (3). For example, identification of candidate viral receptors using genome-wide loss-of-function screens can be used to generate specific hypotheses regarding the identity of the associated receptor, which can then be subject to empirical test.
Although broadly speaking we concur with Ratti on these matters (in addition to concurring with other philosophers who have written on this topic, e.g. Franklin ( 2005 ); Burian ( 2007 )), and find his work to deliver significant advances in our conceptual understanding of such large-scale studies, his citing of ‘genome-wide association studies’ (GWAS) as a means of illustrating the above points (see Ratti 2015 , p. 201) invites further consideration. GWAS aims to identify causal associations between genetic variations and diseases/traits; however, it encounters serious difficulties in identifying concrete hypotheses to prioritise, as per Ratti’s (2). Different solutions to this issue (and the related issue of GWAS ‘missing heritability’) manifest in different approaches to this prioritisation: something which deserves to be made explicit in the context of Ratti’s framework. Specifically, while Ratti focuses implicitly on a ‘core gene’ approach to GWAS (cf. Boyle et al. ( 2017 )), according to which a small number of ‘single nucleotide polymorphisms’ (this terminology will be explained in the body of this paper) are primarily responsible for the trait in question (note that this does not imply that only a small number of genes are associated with the relevant phenotype—rather, it assumes that there are some genes which are more central for the manifestation of the phenotype than the majority), there are other approaches to GWAS which do not presuppose this core gene model; as explained in Wray et al. ( 2018 ) (albeit without direct reference to Ratti’s work), such approaches would lead to the prioritisation of different hypotheses in Ratti’s (2). Footnote 1
The first goal of the present paper is to expand on these matters in full detail, and to revise Ratti’s framework in order to incorporate the above points: in so doing, we gain a clearer understanding of how GWAS approaches relate to more traditional, mechanistic, hypothesis-driven science. But there is also a second goal of this paper: to explore for the first time (to our knowledge) in the philosophical literature what it would take for the above-mentioned alternative approaches (often relying on network models)—particularly those which appeal to the field of (phospho)proteomics—to succeed. Although we make no claim that such (phospho)proteomics approaches are per se superior to other strategies for hypothesis prioritisation, they are nevertheless in our view worthy of philosophical attention unto themselves, for they constitute (we contend) a novel form of exploratory experimentation (cf. Burian ( 2007 ); Franklin ( 2005 ); Steinle ( 1997 )) featuring both iterativity (cf. Elliott ( 2012 ); O’Malley et al. ( 2010 )) and appeal to deep learning (cf. Bechtel ( 2019 ); Ratti ( 2020 )).
Bringing all this together, the plan for the paper is as follows. In Sect. " GWAS studies and prioritisation ", we recall the details of GWAS, and witness how different approaches to the so-called missing heritability and coherence problems lead to the prioritisation of different hypotheses in Ratti’s (2). In Sect. " Proteomics and iterative methodology ", we turn our attention to network approaches—specifically to those informed by (phospho)proteomics—and study these through the lens of the literature on exploratory experimentation, before returning to our considerations of GWAS and addressing the question of how such network-based approaches inform the question of hypothesis prioritisation in that context. We close with some discussion of future work to be done in the philosophy both of GWAS, and of big-data biology at large.
GWAS studies and prioritisation
Background on gwas.
Many applications of the framework presented in the introduction—perform genome-wide screens based on a general hypothesis (for example, ‘a gene/process is responsible for a disease’), and on the basis of the results obtained construct a more refined hypothesis for further testing—have been highly successful in biomedical research. However, there are cases in which the application of the approach has not been so straightforward. This can best be illustrated using the example of a field of genomics that studies common diseases such as inflammatory bowel disease (IBD), coronary artery disease, insomnia, and depression. These are often diseases complex in nature, and are thought to be controlled not by a single mutation, but rather to be influenced by multiple loci in the genome and even through the effect of the environment.
In the past decades, researchers have developed a method to characterise the genotype-phenotype associations in these diseases: the method is called ‘genome-wide association studies’ (GWAS). To understand this method, it is important to understand single nucleotide polymorphisms (SNPs). SNPs are variations in a single DNA building block, called a ‘nucleotide’, and they constitute the most common type of genetic variation among individuals. There are around 4-5 million SNPs in a person’s genome. Most SNPs have no effect on human health, but there are some cases in which these variations lead to increased chances of disease. GWAS was based originally upon a ‘common disease, common variant’ hypothesis, which states that common diseases can be attributed to common genetic variants (present in more than 1–5% of the population). By scanning the genomes of many different people, GWAS sought to identify the relationships between common genetic variations and common traits. GWAS studies remain very popular in the field of human genetics, and have been successful in identifying a number of novel variant-trait associations (for example, in diseases such as those mentioned above). For a clear introduction to GWAS from the biology literature, see Tam et al. ( 2019 ); for existing philosophical works on GWAS, with further details on such studies complimentary to those presented in this paper, see e.g. Bourrat ( 2020 ); Bourrat and Lu ( 2017 ).
GWAS’ discontents
GWAS is, however, not without its critics. A clear conclusion from multiple GWAS studies is that even statistically highly significant hits identified from such studies are able to account only for a small fraction of the heritability of the trait/disease in question. (Recall that ‘heritability’ is the measure of proportion of the phenotypic variance in a population that can be attributed to genetic differences—see Downes and Matthews ( 2020 ) and references therein for further details.) Moreover, GWAS studies often implicate large numbers of genes. To put this into perspective, three GWAS studies performed for height in 2008 identified 27, 12 and 20 associated genomic regions, which accounted merely for 3.7, 2.0, and 2.9% of the population variation in height, respectively ( Lettre et al. ( 2008 ); Weedon et al. ( 2008 ); Gudbjartsson et al. ( 2008 )). This was in sharp contrast with estimates from previous genetic epidemiology studies, based upon twin studies, Footnote 2 that estimated the heritability of height to be around 80% (Yang et al. ( 2010 )). In the early days of GWAS, this apparent discrepancy from GWAS came to be known as the missing heritability problem . For recent philosophical discussion of this problem, see Bourrat ( 2020 ); Bourrat and Lu ( 2017 ); Bourrat et al. ( 2017 ); Bourrat ( 2019 ); Downes and Matthews ( 2020 ); Matthews and Turkheimer ( 2019 ).
Geneticists have since proposed a number of solutions to the missing heritibility problem. The three most commonly-discussed such solutions are classified by Gibson ( 2012 ) as follows:
Complex dieseases are polygenic and many loci with small effects account for the phenotype variance.
Common diseases are caused by rare genetic variants each of which have large effect sizes.
Most common diseases are a result of interactions between many factors such as gene-gene interaction effects and effects from environmental factors.
(We take the proposals for solving the missing heritability problem presented in Bourrat ( 2020 ); Bourrat and Lu ( 2017 ); Bourrat et al. ( 2017 ); Bourrat ( 2019 ), which invoke factors from the epigenome, to fall into category (3); we discuss further these proposals in Sect. GWAS reprise .) From multiple GWAS studies on common diseases there is now overwhelming evidence that common diseases are polygenic, as large numbers of genes are often implicated for a given disease. However, using this framework, it is estimated that it would take 90,000–100,000 SNPs to explain 80% of the population variation in height. In light of this, Goldstein ( 2009 ) raised the concern with GWAS studies that “[i]n pointing at ‘everything’, the danger is that GWAS could point at ‘nothing”’.
It is understandable that one would find unpalatable its not being the case that a single gene or process can be associated with a particular disease. But the situation here is not as straightforward as the above remarks might suggest. Indeed, Boyle et al. ( 2017 ) propose the following refinement of this idea:
Intuitively, one might expect disease-causing variants to cluster into key pathways that drive disease etiology. But for complex traits, association signals tend to be spread across most of the genome—including near many genes without an obvious connection to disease. We propose that gene regulatory networks are sufficiently interconnected such that all genes expressed in disease-relevant cells are liable to affect the functions of core disease-related genes and that most heritability can be explained by effects on genes outside core pathways. We refer to this hypothesis as an ‘omnigenic’ model.
Boyle et al. ( 2017 ) propose that within the large number of implicated genes in GWAS, there are a few ‘core’ genes that play a direct role in disease biology; the large number of other genes identified are ‘peripheral’ and have no direct relevance to the specific disease but play a role in general regulatory cellular networks. By introducing their ‘omnigenic’ model, Boyle et al. ( 2017 ) acknowledge the empirical evidence that GWAS on complex diseases does in fact implicate large number of genes; they thereby seem to draw a distinction between complex diseases and classical Mendelian disorders, in which small number of highly deleterious variants drive the disease. However, their suggestion of the existence of a small number of ‘core’ genes backtracks on this and paints complex diseases in the same brushstrokes as classical Mendelian disorders. A number of authors have welcomed the suggestion that genes implicated for complex diseases play a role in regulatory networks but have found the dicotomy between core and peripheral genes to be an ill-motivated attempt to fit complex disease into what we intuitively think should be the framework of a disease (‘a small number of genes should be responsible for a given disease’). For example, Wray et al. ( 2018 ) write:
It seems to us to be a strong assumption that only a few genes have a core role in a common disease. Given the extent of biological robustness, we cannot exclude an etiology of many core genes, which in turn may become indistinguishable from a model of no core genes.
We concur with this verdict. One possible reconstruction of the reasons underlying the endorsement by Boyle et al. ( 2017 ) of ‘core’ versus ’peripheral’ genes could be in order to solve the missing heritability problem. These authors advocate for using experimental methods that are able to identify rare variants that have high effect sizes (solution (2) of the missing heritability problem as presented above), as this is where they suspect the ‘core’ genes can be identified. However, there is at present no evidence that the ‘core gene’ hypothesis need invariably be true for complex diseases (cf. Wray et al. ( 2018 )), so one might be inclined to reject the original hypothesis that all diseases must fit the mould of ‘small number of genes cause complex diseases’. In so doing, one would thereby need to embrace the claim that at least some complex diseases are polygenic and that putative ‘core’ genes are, in fact, no more important than putative ‘peripheral’ genes in this context.
This, however, still leaves us with the original issue that Boyle et al. ( 2017 ) were trying to address: how is it that genes which look disconnected are, in fact, together implicated in a given disease? In addressing this question, we again concur with Wray et al. ( 2018 ), who write:
To assume that a limited number of core genes are key to our understanding of common disease may underestimate the true biological complexity, which is better represented by systems genetics and network approaches.
That is to say, understanding gene functions and the interplay between the different genes is key to answering why many genes are involved in complex diseases. This is not a straightforward task and a full characterisation of the roles that genes play in biological systems remains a distant prospect.
One approach to addressing this issue is to identify relationships between genes in a cell by way of a systems biology approach, underlying premises of which are that cells are complex systems and that genetic units in cells rarely operate in isolation. Hence, on this view, understanding how genes relate to one another in a given context is key to establishing the true role of variants identified from GWAS hits. There are a number of approaches described in the field of systems biology to identify gene-gene relationships. One widely-implemented approach is to construct ‘regulatory networks’ relating these genes. A regulatory network is a set of genes, or parts of genes, that interact with each other to control a specific cell function. With recent advances in high-throughput transcriptomics, it is now possible to generate complex regulatory networks of how genes interact with each other in biological processes and define the roles of genes in a context-dependent manner based on mRNA expression in a cell. As the majority of GWAS hits often lie in non-coding regions of the genome, which are often involved in regulating gene expressions, networks based on mRNA expression are powerful means to interpret of the functional role of variants identified by GWAS.
Another approach to the functional validation of GWAS hits—currently substantially less common—proceeds by constructing networks generated from expression of proteins/phosphoproteins in a cell (more details of these approaches will be provided in the following section). Such approaches would in principle depict completely the underlying state of the cell. Combined with gene expression data, protein expression networks and signalling networks from proteomics would make transparent the functional role of the variants identified in GWAS studies in a given context—that is, they would provide a mechanistic account of disease pathogenesis without recourse to a neo-Mendelian ‘core gene’ model. Genes which prima facie appear disconnected and irrelevant to disease biology may be revealed by these approaches to be relevant after all. To illustrate, consider a complex disease such as IBD: it is thought that both (i) a disturbed interaction between the gut and the intestinal microbiota, and (ii) an over-reaction of the immune system, are required for this disease phenotype to manifest. Thus, it is likely that a number of genetic pathways will be important—pathways which need not prima facie be connected, but which may ultimately be discovered to be related in some deeper way. These proteomics-informed network approaches would thereby afford one resolution to what has been dubbed by Reimers et al. ( 2019 ) and Craver et al. ( 2020 ) the ‘coherence problem’ of GWAS: to explain how it is that all genes implicated in these studies are related to one another mechanistically. Footnote 3 Clearly, these approaches could be brought to bear in order to vindicate responses (1) or (3) to the missing heritability problem, presented above. Footnote 4
To close this subsection, it is worth reflecting on how the ‘core gene’ hypothesis might intersect with network-based approaches. If a core gene exists, then a network analysis should (at least in principle) be able to identify it; in this sense, a ‘core gene’ hypothesis can be compatible with a network approach. As already mentioned above, however, there is no evidence that such core genes invariably exist: a network analysis could (in principle) identify many ‘central hubs’, rather than just one—an outcome not obviously compatible with the ‘core gene’ hypothesis. (For more on this latter possibility, cf. the very recent work of Barrio-Hernandez et al. ( 2021 ), discussed further below.)
Ratti’s framework for large-scale studies
Suppose that one follows (our reconstruction of) Boyle et al. ( 2017 ), in embracing option (2) presented above as a solution to the GWAS missing heritability problem. One will thereby, in Ratti’s second step in his three-step programme characterising these data-driven approaches to biology, prioritise hypotheses according to which a few rare genes are responsible for the disease in question. This, indeed, is what Ratti ( 2015 ) suggests in §2.2 of his article. However, one might question whether this prioritisation is warranted, in light of the lack of direct empirical evidence for this neo-Mendelian hypothesis (as already discussed). Wray et al. ( 2018 ), for example, write that
... [t]o bias experimental design towards a hypothesis based upon a critical assumption that only a few genes play key roles in complex disease would be putting all eggs in one basket.
If one concurs with Wray et al. ( 2018 ) on this matter (as, indeed, we do), then one may prioritise different hypotheses in the second step of Ratti’s programme—in particular, one may prioritise specific hypotheses associated with ‘polygenic’ models which would constitute approach (1) and/or approach (3) to the missing heritability problem.
This latter point should be expanded. Even if one does embrace a ‘polygenic’ approach to the missing heritability problem (i.e., approach (1) and/or approach (3)), and applies e.g. networks (whether transcriptomics-based, or (phospho)proteomics-informed, or otherwise—nothing hinges on this for our purposes here) in order to model the genetic factors responsible for disease pathogenesis, ultimately one must prioritise specific hypotheses for laboratory test. For example, Schwartzentruber et al. ( 2021 ) implement in parallel a range of network models within the framework of a polygenic approach in order to prioritise genes such as TSPAN14 and ADAM10 in studies on Alzheimer’s disease (we discuss further the methodology of Schwartzentruber et al. ( 2021 ) in §3.3 ). Note, however, that these specific hypotheses might be selected for a range of reasons—e.g., our prior knowledge of the entities involved, or ease of testability, or even financial considerations—and that making such prioritisations emphatically does not imply that one is making implicit appeal to a ‘core gene’ model. This point is corroborated further by the fact that the above two genes are not the most statistically significant hits in the studies undertaken by Schwartzentruber et al. ( 2021 ), as one might expect from those working within the ‘core gene’ framework.
Returning to Ratti’s framework: we take our noting this plurality of options vis-à-vis hypothesis prioritisation to constitute a friendly modification to this framework appropriate to contexts such as that of GWAS. But of course, if one were to leave things here, questions would remain—for it would remain unclear which polygenic model of disease pathogenesis is to be preferred, and how such models are generated. Given this, it is now incumbent upon us to consider in more detail how such approaches set about achieving these tasks in practice: due both to their potential to offer underlying mechanistic models of the cell, as well as due to the novel iterative methodology for hypothesis generation involved, we focus largely in the remainder upon (phospho)proteomics-based approaches.
Proteomics and iterative methodology
Proteomics promises to afford the ultimate fundamental mechanistic account of cellular processes; data from proteomics would, therefore, illuminate the underlying relationships between the variants identified in GWAS studies. In this section, we explore in greater detail how such proteomics approaches proceed; they constitute a novel form of ‘exploratory experimentation’ (in the terminology of Burian ( 2007 ); Steinle ( 1997 )) worthy unto themselves of exposure in the philosophical literature. Footnote 5 In proteomics, further complications for hypothesis generation and testing arise, for data is sparse, and experiments often prohibitively expensive to perform. Given these constraints, how is progress to be made? It is to this question which we now turn; the structure of the section is as follows. In Sect. Proteomics: a data-deprived field , we present relevant background regarding proteomics. Then, in Sect. Methodological iteration , we argue that the development of this field can be understood on a model of a novel form of iterative methodology (cf. Chang 2004 ; O’Malley et al. 2010 ). We return to the relevance of these approaches for GWAS in Sect. GWAS reprise .
Proteomics: a data-deprived field
The ultimate aim of -omics studies is to understand the cell qua biological system. Transcriptomics is now sufficiently well-advanced to accommodate large-scale systematic studies to the point of being used to validate variants identified from GWAS. Footnote 6 By contrast, proteomics—the study of proteins in a cell—remains significantly under-studied. Technologies allowing for the systematic study of proteins are not as advanced as those for studying genes and transcripts; this is mainly because no method currently exists for directly amplifying proteins (i.e., increasing the amount of a desired protein in a controlled laboratory context): a methodology which has been key for genomics and transcriptomics. Proteins are very diverse in the cell: a single gene/transcript gives rise to multiple proteins. Proteins themselves can be modified in the cell after being created, thus further increasing the complexity of proteomics studies. Unlike genomics and transcriptomics, in which it is now common to perform systematic genome-wide or transcriptome-wide approaches, studies of proteins are therefore usually taken piecemeal.
Proteomics research tends to focus on families of proteins that are involved in a particular known biological process. Among the important families of proteins are kinases and phosphatases, which are molecules that are responsible for signal transmission in the cell. These proteins are able to modify other proteins by adding or removing a phosphate group (respectively). This modification changes the shape (‘conformation’) of the protein, rendering it active or inactive, Footnote 7 depending on the context. By examining the phopsphorylation state of the proteins inside a cell, it is possible to infer the signalling state of that cell. The field of phosphoproteomics aims to characterise all phospho-modified proteins within a cell. This is thought to be one of the most powerful and fundamental ways of inferring the signalling process within a cell; the approach could add a substantial new layer to our understanding of both basic and disease biology. That said, a recent estimate suggests that current approaches have identified kinases for less than 5% of the phosphoproteome. What is even more staggering is that almost 90% of the phosphorylation modifications that have been identified have been attributed to only 20% of kinases. The other 80% of the kinases are completely dark: their functions remain unknown. For many such kinases, we do not even know where in the cell they are located. (See Needham et al. ( 2019 ) for a review of the current state of play in phosphoproteomics.)
In such a field, systematic studies to quantify the entire phosphoproteome in a cell and an ability to assign a kinase to every phosphorylated component would be the ultimate aim. But phosphoproteomics studies themselves are currently extremely expensive, and there are technological limitations in mapping the global phosphoproteome—not least sparsity of data, which often comes as a result of limitations in the technical setup of laboratory measurements and experiments. For example: the same sample measured in the same machine at two different instances will give readings for different phosphoproteins. Some statistical methods can be used to overcome these limitations, but these require making assumptions regarding the underlying biology, which defeats the point of an unbiased study.
In spite of these difficulties, it has been shown that if one combines multiple large-scale phosphoprotemics data sets (each admittedly incomplete), it is possible to predict kinase-kinase regulatory relationships in a cell using data-driven phosphoprotein signalling networks obtained via supervised machine learning approaches (a recent study from Invergo et al. 2020 showcases one such approach; we will use this as a running example in the ensuing). Footnote 8 First, a training set of data is used to teach a machine a classification algorithm. Once the classification algorithm is learnt, the machine is set to the task of applying it to unlabelled data: in our case, the goal is to identify further, as-yet unknown, regulatory protein relationships or non-relationships. (On machine learning and network analysis of biological systems, see also Bechtel ( 2019 ) and Ratti ( 2020 ).)
Before assessing such phosphoproteomics machine learning algorithms as that of Invergo et al. ( 2020 ), there are two further complications with the current state of play in proteomics which need to be mentioned. First: it is much easier to curate positive lists of interactions than negative lists. (This is essentially a case of its being easier to confirm existentially quantified statements than universally quantifies statements: for how can we ever truly ascertain that any two given proteins never interact?) Thus, at present, negative lists obtained from laboratory experiments are underpopulated. Invergo et al. ( 2020 ) attempt to circumvent this issue in the following way: they assume that regulatory relationships are rare, so that if one were to randomly sample protein associations, one could create reliably large artificial negative sets; indeed, they do generate artificial negative sets in exactly this way. (Clearly, this means that these approaches again cannot be understood as being ‘hypothesis-free’: cf. Sect. Introduction .)
The second problem with the current state of play in proteomics is this: when a given interaction occurs is a function of multifarious factors, most notably cell context. This context-dependence means that an entry in a negative set in one context might, in fact, be an entry in a positive set in another. To illustrate: in the case of regulatory relationships between two kinases, it is known that such relationships can be prone to dysregulation in diseases such as cancer. Hence, a well-annotated positive set relationship can very well be dysregulated in a cancer context, so that this relationship no longer exists, effectively putting it into a negative set. The problem is that many data-driven approaches rely on data that are generated in simple reductionist systems such as cancer cell lines—so that the results obtained might not carry across to the target physiological context. (Cancer cell lines can grow infinitely, and thus are ideal for experiments.) The approach taken by Invergo et al. ( 2020 ) utilises data from breast cancer cell lines; hence, the relationships they predict could be specific to a dysregulated system. In response to this second problem, we suggest replying on behalf of Invergo et al. ( 2020 ) that most regulatory relationships fundamental to the functioning of the cell should hold true in most contexts. At present, however, given the data-deprived nature of proteomics, there is little direct evidence for this hypothesis. (Again, the appeal to any such hypothesis would mean that such proteomics approaches cannot be ‘hypothesis-free’.)
Thus, the fact that Invergo et al. ( 2020 ) utilise data from breast cancer cell lines raises the possibility that their machine learning algorithms might be trained on data unsuited to other contexts, leading to concerns regarding error propagation. This general concern regarding the context-specificity (or lack thereof) of input data sets is, however, recognised by authors in the field—for example, Barrio-Hernandez et al. ( 2021 ) note that “improvements in mapping coverage and computational or experimental approaches to derive tissue or cell type specific networks could have a large impact on future effectiveness of network expansion” (Barrio-Hernandez et al. 2021 , p. 14).
Methodological iteration
In spite of these problems, Invergo et al. ( 2020 ) argue that the results obtained from their approach afford a useful means of bootstrapping further progress in phosphoproteomics. As they put it:
Although we do not suggest that these predictions can replace established methods for confirming regulatory relationships, they can nevertheless be used to reduce the vast space of possible relationships under consideration in order to form credible hypotheses and to prioritize experiments, particularly for understudied kinases. (Invergo et al. 2020 , p. 393)
One way to take this point is the following. Ideally, in order to construct positive and negative sets, one would test in the laboratory each individual protein association. Practically, however, this would be an unrealistic undertaking, as we have already seen. What can be done instead is this:
Generate a global phosphoproteomics data set, albeit one that is incomplete and sparse (e.g., that presented in Wilkes et al. ( 2015 )), based upon laboratory experiments.
Train, using this data set and input background hypotheses of the kind discussed above, a machine learning algorithm (such as that presented in Invergo et al. ( 2020 )) to identify candidate interactions in the unknown space of protein-protein interactions. Footnote 9
Use these results to guide further laboratory experimentation, leading to the development of more complete data sets.
Train one’s machine learning algorithms on these new data sets, to improve performance; in turn, repeat further the above process.
Clearly, a process of reflective equilibrium is at play here (cf. Daniels ( 2016 )). As is well-known, Chang ( 2004 ) has proposed an iterative conception of scientific methodology, according to which the accrual of scientific hypotheses is not a linear matter; rather, initial data may lead to the construction of a theoretical edifice which leads one to develop new experiments to revise one’s data; at which point, the process iterates. This fits well with the above-described procedures deployed in phosphoproteomics; it also accords with previous registration of the role of iterative procedures in large-scale biological studies—see e.g. O’Malley et al. ( 2010 ) and Elliott ( 2012 ).
Let us delve into this a little deeper. As Chang notes,
There are two modes of progress enabled by iteration: enrichment , in which the initially affirmed system is not negated but refined, resulting in the enhancement of some of its epistemic virtues; and self-correction , in which the initially affirmed system is actually altered in its content as a result of inquiry based on itself. (Chang 2004 , p. 228)
Certainly and uncontroversially, enrichment occurs in the above four-step process in phosophoproteomics: the new data yield a refinement of our previous hypotheses in the field. In addition, however, it is plausible to understand the above iterative methodology as involving self-correction: for example, in might be that the machine learning algorithm of Invergo et al. ( 2020 ) identifies a false positive, yet nevertheless makes sufficiently focused novel predictions with respect to other candidate interactions in order to drive new experimentation, leading to a new data set on which the algorithm can be trained, such that, ultimately, the refined algorithm does not make a false positive prediction for that particular interaction. This is entirely possible in the above iterative programme; thus, we maintain that both modes of Changian iterative methodology are at play in this approach.
There is another distinction which is also relevant here: that drawn by Elliott ( 2012 ) between ‘epistemic iteration’—“a process by which scientific knowledge claims are progressively altered and refined via self-correction or enrichment”—and ‘methodological iteration’—“a process by which scientists move repetitively back and forth between different modes of research practice” (Elliott 2012 , p. 378). It should be transparent from our above discussion that epistemic iteration is involved in these proteomics approaches. Equally, though, it should be clear that methodological iteration is involved, for the approach alternates between machine learning and more traditional laboratory experimentation. That machine learning can play a role in an iterative methodology does not seem to have been noted previously in the philosophical literature—for example, it is not identified by Elliott ( 2012 ) as a potential element of a methodologically iterative approach; on the other hand, although the role of machine learning in network modelling and large-scale studies is acknowledged by Bechtel ( 2019 ) and Ratti ( 2020 ) (the latter of whom also discusses—albeit without explicitly using this terminology—the role of machine learning in epistemic iteration: see (Ratti 2020 , p. 89)), there is no mention of its role in an iterative methodology such as that described above.
GWAS reprise
Given the foregoing, we hope it is reasonable to state that the approaches to proteomics of e.g. Invergo et al. ( 2020 ) constitute novels forms of exploratory experimentation, worthy of study in their own right. Let us, however, return now to the matter of polygenic approaches to GWAS hits. In principle, the results of the methodologies of e.g. Invergo et al. ( 2020 ) could further vindicate these approaches, by providing mechanistic models of which genes interact in a disease context, and when and why they do so. In turn, they have the capacity to allow biologists to prioritise specific hypotheses in Ratti’s step (2), without falling back upon assumptions that only few genes are directly involved in complex disease biology.
Note that that there is a complex interplay between this iterative methodology and the ‘eliminative induction’ of stages (1) and (2) Ratti’s analysis (see Sect. Introduction ; for earlier sources on eliminative induction, see Earman ( 1992 ); Kitcher ( 1993 ); Norton ( 1995 )). We take this to consist in the following. First, a methodology such as that of Invergo et al. ( 2020 ) is used to generate a particular network-based model for the factors which are taken to underlie a particular phenotype. This model is used to prioritise ( à la eliminative induction) particular hypotheses, as per stage (2) of Ratti’s framework; these are then subject to specific test, as per stage (3) of Ratti’s framework. The data obtained from such more traditional experimentation is then used to construct more sophisticated network models within the framework of Invergo et al. ( 2020 ); these in turn lead to the (eliminative inductive) prioritisation of further specific hypotheses amenable to specific test. As already discussed above, this is a clear example of the ‘methodological iteration’ of Elliott ( 2012 ).
It bears stressing that (phospho)proteomics network-based approaches may, ultimately, constitute only one piece of the solution to the broader puzzle that is GWAS hypothesis prioritisation. In very recent work, Schwartzentruber et al. ( 2021 ) have brought to bear upon this problem consideration of, inter alia , epigenomic factors alongside network-based analyses. There are two salient points to be made on this work. First: although Bourrat et al. ( 2017 ) are correct that epigenomic studies and background may have a role to play in addressing the missing heritability problem (cf. Bourrat ( 2019 , 2020 ); Bourrat and Lu ( 2017 )), a view in contemporary large-scale biological studies—evident in papers such as Schwartzentruber et al. ( 2021 )—is that these considerations can be supplemented with yet other resources, such as network-based studies; we concur with this verdict. Second: in order to construct these networks, Schwartzentruber et al. ( 2021 ) rely on established protein-protein interaction databases such as STRING, IntAct and BioGRID (Schwartzentruber et al. 2021 , p. 397). While effective in their own right, networks developed from such databases have the disadvantage that they represent signalling in an ‘average’ cell, and are therefore unsuitable for studying dynamic context- and cell-type-specific signalling responses (cf. Sharma and Petsalaki ( 2019 )). In this regard, it would (at least in principle) be preferable to utilise regulatory and context-specific networks developed using methods described in work such as that of Invergo et al. ( 2020 ) in future approaches to GWAS hypothesis prioritisation. That being said, in practice this may not yet be fruitful, as at present contemporary large-scale biology is only at the early stages of the iterative processes discussed above; moreover, the training data sets used by such methods remain at this stage not completely context-specific (recall that Invergo et al. ( 2020 ) utilise a breast cancer training set)—meaning that the potential of such work to yield detailed, context-specific network-based models is yet to be realised in full.
With all of the above in hand, we close this subsection by considering more precisely the question of how the machine learning algorithms of Invergo et al. ( 2020 ) bear upon the missing heritability problem. Having developed regulatory protein-protein interaction networks on the basis of such algorithms, one can take (following here for the sake of concreteness the lead of Barrio-Hernandez et al. ( 2021 )) the connection with hypothesis prioritisation in GWAS (and, in turn, the missing heritability problem) to proceed via the following steps (also summarised visually in Fig. 1 ):
Select a protein-protein interaction network. Usually, this is a pre-existing curated network, such as those defined in the STRING database (discussed above). However, instead of such curated networks, use in their place networks developed on the machine learning models of e.g. Invergo et al. ( 2020 ).
Within those networks, identify the nodes (i.e., proteins) which correspond to hits from a particular GWAS (i.e., the proteins associated with the genes identified in the GWAS). Footnote 10
Use network propagation methods (see e.g. Cowen et al. ( 2017 ) for a review of such methods), potentially alongside other factors (as discussed in e.g. Schwartzentruber et al. ( 2021 )) in order to identify known modules (i.e., separated substructures within a network) associated with the disease in question.
Target elements of those modules, regardless of whether or not they were hits in the original GWAS. (This latter approach—of targeting beyond the original GWAS hits—is novel to the very recent work of Barrio-Hernandez et al. ( 2021 ).)
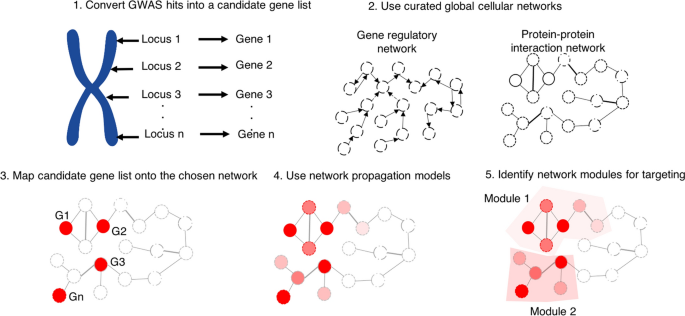
The application of networks to GWAS hit prioritisation. In (1), GWAS hits are converted to candidate gene lists. In (2), one selects a cellular network: this could be a gene regulatory network, or a protein-protein interaction network (e.g. from STRING), or a protein-protein regulatory network (possibly constructed via the machine learning methodologies of Invergo et al. ( 2020 )). In (3), genes associated with the GWAS loci are mapped to the chosen network. In (4), network propagation methods (e.g. diffusion techniques) are applied in order identify potential disease-related genes not picked up by the GWAS. In (5), the results of these network analyses are used to identify significant genetic modules to be targeted experimentally in investigations into disease pathogenesis. Note, following Wray et al. ( 2018 ) and Barrio-Hernandez et al. ( 2021 ), that this particular means of bridging the gap between cellular networks and investigations into the results of GWAS hits does not presuppose a ‘core gene’ hypothesis
On (2) and (3): Boyle et al. ( 2017 ) may or may not be correct that many genes are implicated (either in the original screen, or after the network analysis has been undertaken)—recall from Sect. GWAS’ discontents their ‘omnigenic’ model. However, on the basis of the work of Barrio-Hernandez et al. ( 2021 ) one might argue that this is not the most important question—rather, the important question is this: which gene modules provide insights into the disease mechanism? One can ask this question without subscribing to a ‘core gene’ model; thus, we take the work of Barrio-Hernandez et al. ( 2021 ) to be consistent with the above-discussed points raised by Wray et al. ( 2018 ).
This paper has had two goals. The first has been to propose revisions to the framework of Ratti ( 2015 ) for the study of the role of hypothesis-driven research in large-scale contemporary biological studies, in light of studies such as GWAS and its associated missing heritability problem. In this regard, we have seen that different hypotheses may be prioritised, depending upon whether one adopts a ‘core’ gene model (as Ratti ( 2015 ) assumes, and as is also advocated in Boyle et al. ( 2017 )), or whether one adopts a polygenic model (as endorsed by Wray et al. ( 2018 ); cf. Barrio-Hernandez et al. ( 2021 )). The second goal of this paper has been to consider how these hypotheses would be developed on polygenic approaches via (phospho)proteomics—which itself constitutes a novel form of exploratory experiment, featuring as it does both iterativity and deep learning—and to consider what it would take for these network-based proteomics approaches to succeed. A broader upshot of this paper has been the exposure for the first time to the philosophical literature of proteomics: given its potential to provide mechanistic models associated with disease phenotypes, the significance of this field cannot be overstated.
The issues discussed in this paper raise important questions regarding how researchers prioritise not just first-order hypotheses as per Ratti’s (2), but also the background assumptions which allow one to make such adjudications to begin with. To be concrete: in the case of GWAS, should one prioritise the assumption that rare variants of large effect in a small number of genes drive complex diseases, or rather invest in developing systems-based approaches and in improving under-studied fields, such as (phospho)proteomics, which may or may not ultimately shed light on the question of why complex diseases have thus far manifested empirically as polygenic? These choices lead to different first-order prioritisations in Ratti’s second step, and thereby have great potential to steer the course of large-scale studies in future years. Given limited resources in the field, it is, in our view, worth pausing to reflect on whether said resources are appropriately allocated between these options, and to strive to avoid any status quo bias in favour of currently-popular assumptions. Footnote 11
In fairness to Ratti, in other articles, e.g. López-Rubio and Ratti ( 2021 ), he does not make assumptions tantamount to a ‘core gene’ hypothesis; in this sense, our criticism falls most squarely on assumptions made in Ratti ( 2015 ).
Twin studies are powerful approaches to studying the genetics of complex traits. In simple terms, twin studies compare the phenotypic similarity of identical (monozygotic) twins to non-identical (dizygotic) twins. As monozygotic twins are genetically identical and non-identical twins are on average ‘half identical’, observing greater similarity of identical over non-identical twins can be used as an evidence to estimate the contribution of genetic variation to trait manifestation. For further discussion of twin studies in the philosophical literature, see e.g. Matthews and Turkheimer ( 2019 ); Downes and Matthews ( 2020 ).
There are many further questions to be addressed here in connection with the literature of mechanisms and mechanistic explanations. For example, are these network approaches best understood as revealing specific mechanisms, or rather as revealing mechanism schema (to use the terminology of (Craver and Darden 2013 , ch.3))? Although interesting and worthy of pursuit, for simplicity we set such questions aside in this paper, and simply speak of certain contemporary biology approaches as revealing ‘underlying mechanisms’. In this regard, we follow the lead of Ratti ( 2015 ).
To be completely clear: we do not claim that these (phospho)proteomics-based network approaches are superior to regulatory network approaches, given the current state of technology in the field. On the contrary—as we explain in Sect. Proteomics and iterative methodology —the former of these fields is very much nascent, and has yet to yield significant predictive or explanatory fruit. Nevertheless—again as we explain in Srct. Proteomics and iterative methodology —in our view these approaches are worthy of exposure in the philosophical literature in their own right, for (a) they offer one of the most promising means (in principle, if not yet in practice) of providing a mechanistic account of disease pathogenesis, and (b) the particular way in which hypotheses are developed and prioritised on these approaches is conceptually rich.
Recall: “Experiments count as exploratory when the concepts or categories in terms of which results should be understood are not obvious, the experimental methods and instruments for answering the questions are uncertain, or it is necessary first to establish relevant factual correlations in order to characterize the phenomena of a domain and the regularities that require (perhaps causal) explanation” (Burian 2013 ). Cf. e.g. Franklin ( 2005 ); Steinle ( 1997 ). All of the -omics approaches discussed in this paper were identified in Burian ( 2007 ) as cases of exploratory experimentation; the details of contemporary proteomics approaches have, however, not been presented in the philosophical literature up to this point (at least to our knowledge).
In this paper, we do not go into the details of specific transcriptomics studies. One interesting approach worthy of mention, however, is ‘single-cell RNA sequencing’ (SC-RNA), which allows biologists to assay the full transcriptome of hundreds of cells in an unbiased manner (see e.g. Hwang et al. ( 2018 ) for a recent review). The advantage of SC-RNA over older methods lies in its ability to identify the transcriptomes from heterocellular and poorly-classified tissue populations and disease-associated cell states.
As the addition or removal of phosphate groups regulates the activity of a protein, such relationships between a kinase and its target (also called a ‘substrate’) are referred to as ‘regulatory relationships’. Kinases themselves can also be phosphorylated by other kinases, so there exist also kinase-kinase regulatory relationships in a cell.
Supervised machine learning involves training a machine on a given data set (for example, a collection of cat photos versus dog photos), before assigning the machine the task of classifying entries in some new data set. By contrast, in unsupervised learning, the machine is instructed to find its own patterns in a given data set. For some recent philosophical considerations regarding machine learning, see Sullivan ( 2019 ).
One can also test the results of the machine binary classification algorithm on other data sets: this Invergo et al. ( 2020 ) did with reference to the data presented in Hijazi et al. ( 2020 ). The design of the algorithmic system and algorithm used by Invergo et al. ( 2020 ) is described with admirable clarity at (Invergo et al. 2020 , pp. e5ff.), to which the reader is referred for further details.
Note that identification of candidate genes from the loci which constitute GWAS hits is non-trivial. The recently-described ‘locus-to-gene’ (L2G) approach is a machine learning tool which can be used to prioritise likely causal genes at each locus given genetic and functional genomics features (see Mountjoy et al. ( 2020 )).
Cf. Samuelson and Zeckhauser ( 1988 ). For related discussion of funding decisions in the context of -omics studies, see Burian ( 2007 ).
Barrio-Hernandez I, Schwartzentruber J, Shrivastava A, del Toro N, Zhang Q, Bradley G, Hermjakob H, Orchard S, Dunham I, Anderson CA, Porras P, Beltrao P (2021) ‘Network expansion of genetic associations defines a pleiotropy map of human cell biology’, bioRxiv . https://www.biorxiv.org/content/early/2021/07/19/2021.07.19.452924
Bechtel W (2019) Hierarchy and levels: analysing networks to study mechanisms in molecular biology. Philos Transact R Soc B 375(20190320):20190320
Google Scholar
Bourrat P (2019) Evolutionary transitions in heritability and individuality. Theory Biosci 138:305–323
Article Google Scholar
Bourrat P (2020) Causation and single nucleotide polymorphism heritability. Philos Sci 87:1073–1083
Bourrat P, Lu Q (2017) Dissolving the Missing Heritability Problem. Philosophy of Science 84:1055–1067
Bourrat P, Lu Q, Jablonka E (2017) Why the missing heritability might not be in the DNA. BioEssays 39:1700067
Boyle E, Li Y, Pritchard J (2017) An expanded view of complex traits: from polygenic to omnigenic. Cell 169:1177–1186
Burian R (2013) Exploratory experimentation. In: Dubitzky W, Wolkenhauer O, Cho K-H, Yokota H (eds) Encyclopedia of systems biology. Springer, Berlin
Burian RM (2007) On MicroRNA and the need for exploratory experimentation in post-genomic molecular biology. Hist Philos Life Sci. 29(3):285–311. http://www.jstor.org/stable/23334263
Chang H (2004) Inventing temperature: measurement and scientific progress. Oxford University Press, Oxford
Book Google Scholar
Cowen L, Ideker T, Raphael BJ, Sharan R (2017) Network propagation: a universal amplifier of genetic associations. Nat Rev Genet 18(9):551–562. https://doi.org/10.1038/nrg.2017.38
Craver CF, Darden L (2013) In search of mechanisms. University of Chicago Press, Chicago
Craver CF, Dozmorov M, Reimers M, Kendler KS (2020) Gloomy prospects and roller coasters: finding coherence in genome-wide association studies. Philos Sci 87(5):1084–1095
Daniels N (2016) Reflective equilibrium. The Stanford Encyclopedia of Philosophy
Doudna JA, Charpentier E (2014) The new frontier of genome engineering with CRISPR-Cas9. Science 346(6213):1258096
Downes SM, Matthews L (2020) Heritability. In: Zalta EN (ed) The Stanford encyclopedia of philosophy. Stanford University, Metaphysics Research Lab
Earman J (1992) Bayes or bust? a critical examination of BayesianConfirmation Theory,. MIT Press, Cambridge
Elliott KC (2012) Epistemic and methodological iteration in scientific research. Stud Hist Philos Sci Part A 43(2):376–382
Franklin L (2005) Exploratory experiments. Philos Sci. 72(5):888–899. https://www.jstor.org/stable/10.1086/508117
Gibson G (2012) Rare and common variants: twenty arguments. Nat Rev Genet 13(2):135–145
Goldstein D (2009) Common genetic variation and human traits. N Engl J Med 360:1696–1698
Gudbjartsson DF, Walters GB, Thorleifsson G, Stefansson H, Halldorsson BV, Zusmanovich P, Sulem P, Thorlacius S, Gylfason A, Steinberg S, Helgadottir A, Ingason A, Steinthorsdottir V, Olafsdottir EJ, Olafsdottir GH, Jonsson T, Borch-Johnsen K, Hansen T, Andersen G, Jorgensen T, Pedersen O, Aben KK, Witjes JA, Swinkels DW, Heijer Md, Franke B, Verbeek ALM, Becker DM, Yanek LR, Becker LC, Tryggvadottir L, Rafnar T, Gulcher J, Kiemeney LA, Kong A, Thorsteinsdottir U, Stefansson K (2008) Many sequence variants affecting diversity of adult human height. Nat Genet 40(5):609–615. https://doi.org/10.1038/ng.122
Hasin Y, Seldin M, Lusis A (2017) Multi-omics approaches to disease. Genome Biol 18(1):83
Hijazi M, Smith R, Rajeeve V, Bessant C, Cutillas PR (2020) Reconstructing kinase network topologies from phosphoproteomics data reveals cancer-associated rewiring. Nat Biotechnol 38(4):493–502
Hwang B, Lee JH, Bang D (2018) Single-cell RNA sequencing technologies and bioinformatics pipelines. Exp Mol Med 50(8):96. https://doi.org/10.1038/s12276-018-0071-8
Invergo BM, Petursson B, Akhtar N, Bradley D, Giudice G, Hijazi M, Cutillas P, Petsalaki E, Beltrao P (2020) Prediction of signed protein kinase regulatory circuits. Cell Syst 10(5):384-396.e9
Kitcher PS (1993) The advancement of science. Oxford University Press, Oxford
Lander ES, Linton LM, Birren B, Nusbaum C, Zody MC, Baldwin J, Devon K, Dewar K, Doyle M, FitzHugh W, Funke R, Gage D, Harris K, Heaford A, Howland J, Kann L, Lehoczky J, LeVine R, McEwan P, McKernan K, Meldrim J, Mesirov JP, Miranda C, Morris W, Naylor J, Raymond C, Rosetti M, Santos R, Sheridan A, Sougnez C, Stange-Thomann Y, Stojanovic N, Subramanian A, Wyman D, Rogers J, Sulston J, Ainscough R, Beck S, Bentley D, Burton J, Clee C, Carter N, Coulson A, Deadman R, Deloukas P, Dunham A, Dunham I, Durbin R, French L, Grafham D, Gregory S, Hubbard T, Humphray S, Hunt A, Jones M, Lloyd C, McMurray A, Matthews L, Mercer S, Milne S, Mullikin JC, Mungall A, Plumb R, Ross M, Shownkeen R, Sims S, Waterston RH, Wilson RK, Hillier LW, McPherson JD, Marra MA, Mardis ER, Fulton LA, Chinwalla AT, Pepin KH, Gish WR, Chissoe SL, Wendl MC, Delehaunty KD, Miner TL, Delehaunty A, Kramer JB, Cook LL, Fulton RS, Johnson DL, Minx PJ, Clifton SW, Hawkins T, Branscomb E, Predki P, Richardson P, Wenning S, Slezak T, Doggett N, Cheng JF, Olsen A, Lucas S, Elkin C, Uberbacher E, Frazier M, Gibbs RA, Muzny DM, Scherer SE, Bouck JB, Sodergren EJ, Worley KC, Rives CM, Gorrell JH, Metzker ML, Naylor SL, Kucherlapati RS, Nelson DL, Weinstock GM, Sakaki Y, Fujiyama A, Hattori M, Yada T, Toyoda A, Itoh T, Kawagoe C, Watanabe H, Totoki Y, Taylor T, Weissenbach J, Heilig R, Saurin W, Artiguenave F, Brottier P, Bruls T, Pelletier E, Robert C, Wincker P, Smith DR, Doucette-Stamm L, Rubenfield M, Weinstock K, Lee HM, Dubois J, Rosenthal A, Platzer M, Nyakatura G, Taudien S, Rump A, Yang H, Yu J, Wang J, Huang G, Gu J, Hood L, Rowen L, Madan A, Qin S, Davis RW, Federspiel NA, Abola AP, Proctor MJ, Myers RM, Schmutz J, Dickson M, Grimwood J, Cox DR, Olson MV, Kaul R, Raymond C, Shimizu N, Kawasaki K, Minoshima S, Evans GA, Athanasiou M, Schultz R, Roe BA, Chen F, Pan H, Ramser J, Lehrach H, Reinhardt R, McCombie WR, de la Bastide M, Dedhia N, Blöcker H, Hornischer K, Nordsiek G, Agarwala R, Aravind L, Bailey JA, Bateman A, Batzoglou S, Birney E, Bork P, Brown DG, Burge CB, Cerutti L, Chen HC, Church D, Clamp M, Copley RR, Doerks T, Eddy SR, Eichler EE, Furey TS, Galagan J, Gilbert JG, Harmon C, Hayashizaki Y, Haussler D, Hermjakob H, Hokamp K, Jang W, Johnson LS, Jones TA, Kasif S, Kaspryzk A, Kennedy S, Kent WJ, Kitts, P, Koonin, E V, Korf I, Kulp, D, Lancet D, Lowe T M, McLysaght A, Mikkelsen T, Moran J V, Mulder N, Pollara V J, Ponting C P, Schuler G, Schultz J, Slater G, Smit A F, Stupka E, Szustakowki J, Thierry-Mieg D, Thierry-Mieg J, Wagner L, Wallis J, Wheeler R, Williams A, Wolf Y I, Wolfe, K H, Yang S P, Yeh R F, Collins F, Guyer M S, Peterson J, Felsenfeld A, Wetterstrand K A, Patrinos A, Morgan M J, de Jong P, Catanese J J, Osoegawa K, Shizuya H, Choi S, Chen Y J, Szustakowki J, and International Human Genome Sequencing Consortium (2001) Initial sequencing and analysis of the human genome. Nature 409(6822):860–921
Leonelli S (2016) Data-centric biology: a philosophical study. University of Chicago Press, Chicago
Lettre G, Jackson A. U., Gieger C., Schumacher F. R., Berndt S. I., Sanna S., Eyheramendy S., Voight B. F., Butler J. L., Guiducci C., Illig T., Hackett R., Heid I. M., Jacobs K. B., Lyssenko V., Uda M., Boehnke M., Chanock S. J., Groop L. C., Hu F. B., Isomaa B., Kraft P., Peltonen L., Salomaa V., Schlessinger D., Hunter D. J., Hayes R. B., Abecasis G. R., Wichmann H.-E., Mohlke K. L., Hirschhorn J. N., Initiative T. D. G., FUSION, KORA, The Prostate, LC, Trial OCS, Study TNH, SardiNIA (2008) Identification of ten loci associated with height highlights new biological pathways in human growth. Nat Genet 40(5):584–591. https://doi.org/10.1038/ng.125
López-Rubio E, Ratti E (2021) Data science and molecular biology: prediction and mechanistic explanation. Synthese 198(4):3131–3156. https://doi.org/10.1007/s11229-019-02271-0
Matthews LJ, Turkheimer E (2019) Across the great divide: pluralism and the hunt for missing heritability. Synthese. https://doi.org/10.1007/s11229-019-02205-w
Mountjoy E, Schmidt EM, Carmona M, Peat G, Miranda A, Fumis L, Hayhurst J, Buniello A, Schwartzentruber J, Karim MA, Wright D, Hercules A, Papa E, Fauman E, Barrett JC, Todd JA, Ochoa D, Dunham I, Ghoussaini M (2020) Open targets genetics: an open approach to systematically prioritize causal variants and genes at all published human GWAS trait-associated loci, bioRxiv . https://www.biorxiv.org/content/early/2020/09/21/2020.09.16.299271
Needham E, Parker B, Burykin T, James D, Humphreys S (2019) Illuminating the dark phosphoproteome, Sci Signal. Vol. 12
Norton J (1995) Eliminative induction as a method of discovery: how Einstein discovered general relativity. In: Leplin J (ed) The creation of ideas in physics. Kluwer, Alphen aan den Rijn, pp 29–69
Chapter Google Scholar
O‘Malley M, Elliott K, Burian R (2010) From genetic to genomic regulation: iterativity in microRNA research. Stud Hist Philos Sci Part C Stud Hist Philos Biol Biomed Sci 41(4):407–417
Ratti E (2015) Big Data biology: between eliminative inferences and exploratory experiments. Philos Sci 82:198–218
Ratti E (2020) What kind of novelties can machine learning possibly generate? The case of genomics. Stud Hist Philos Sci Part A. 83:86–96. https://www.sciencedirect.com/science/article/pii/S0039368119302924
Reimers M, Craver C, Dozmorov M, Bacanu S-A, Kendler K (2019) The coherence problem: finding meaning in GWAS complexity. Behav Genet 49:187–195
Richardson S, Stevens H (2015) Postgenomics: perspectives on biology after the genome. Duke University Press, Durham
Samuelson W, Zeckhauser R (1988) Status quo bias in decision making. J Risk Uncertain 1(1):7–59. https://doi.org/10.1007/BF00055564
Schwartzentruber J, Cooper S, Liu JZ, Barrio-Hernandez I, Bello E, Kumasaka N, Young AMH, Franklin RJM, Johnson T, Estrada K, Gaffney DJ, Beltrao P, Bassett A (2021) Genome-wide meta-analysis, fine-mapping and integrative prioritization implicate new Alzheimer’s disease risk genes. Nat Genet 53(3):392–402. https://doi.org/10.1038/s41588-020-00776-w
Shalem O, Sanjana NE, Zhang F (2015) High-throughput functional genomics using CRISPR-Cas9. Nat Rev Genet 16(5):299–311
Sharma S, Petsalaki E (2019) Large-scale datasets uncovering cell signalling networks in cancer: context matters. Curr Opin Genet Dev. 54:118–124 Cancer Genomics. https://www.sciencedirect.com/science/article/pii/S0959437X18301278
Steinle F (1997) Entering new fields: exploratory uses of experimentation. Philos Sci. 64:S65–S74. http://www.jstor.org/stable/188390
Sullivan E (2019) Understanding from machine learning models. British J Philos Sci
Tam V, Patel N, Turcotte M, Bossé Y, Paré G, Meyre D (2019) Benefits and limitations of genome-wide association studies. Nat Rev Genet 20(8):467–484. https://doi.org/10.1038/s41576-019-0127-1
Weedon MN, Lango H, Lindgren CM, Wallace C, Evans, DM, Mangino M, Freathy RM, Perry J. RB, Stevens S, Hall AS, Samani NJ, Shields B, Prokopenko I, Farrall M, Dominiczak A, Johnson T, Bergmann S, Beckmann, JS, Vollenweider, P, Waterworth DM, Mooser V, Palmer CNA Morris AD Ouwehand WH, Zhao JH, Li S, Loos R JF, Barroso I, Deloukas P, Sandhu MS, Wheeler E, Soranzo N, Inouye M, Wareham NJ, Caulfield M, Munroe PB, Hattersley AT, McCarthy MI, Frayling TM, Initiative, DG, Consortium TWTCC, Consortium, CG (2008) Genome-wide association analysis identifies 20 loci that influence adult height. Nat Genet 40(5):575–583. https://doi.org/10.1038/ng.121
Wilkes EH, Terfve C, Gribben JG, Saez-Rodriguez J, Cutillas PR (2015) Empirical inference of circuitry and plasticity in a kinase signaling network. Proc Natl Acad Sci U S A 112(25):7719–7724
Wray N, Wijmenga C, Sullivan P, Yang J, Visscher P (2018) Common disease is more complex than implied by the core gene omnigenic model. Cell 173:1573–1580
Yang J, Benyamin B, McEvoy BP, Gordon S, Henders AK, Nyholt DR, Madden PA, Heath AC, Martin NG, Montgomery GW, Goddard ME, Visscher PM (2010) Common SNPs explain a large proportion of the heritability for human height. Nat Genet 42(7):565–569. https://doi.org/10.1038/ng.608
Download references
Acknowledgements
We are grateful to Simon Davis, Katie de Lange, and the anonymous reviewers (one of whom turned out to be Pierrick Bourrat) for helpful discussions and feedback. S.S. is supported by a Sir Henry Wellcome Postdoctoral Fellowship at the University of Oxford.
Author information
Authors and affiliations.
Faculty of Philosophy, University of Oxford, Oxford, UK
Weatherall Institute for Molecular Medicine, University of Oxford, Oxford, UK
Sumana Sharma
You can also search for this author in PubMed Google Scholar
Corresponding author
Correspondence to James Read .
Ethics declarations
Conflict of interest, additional information, publisher's note.
Springer Nature remains neutral with regard to jurisdictional claims in published maps and institutional affiliations.
Rights and permissions
Open Access This article is licensed under a Creative Commons Attribution 4.0 International License, which permits use, sharing, adaptation, distribution and reproduction in any medium or format, as long as you give appropriate credit to the original author(s) and the source, provide a link to the Creative Commons licence, and indicate if changes were made. The images or other third party material in this article are included in the article's Creative Commons licence, unless indicated otherwise in a credit line to the material. If material is not included in the article's Creative Commons licence and your intended use is not permitted by statutory regulation or exceeds the permitted use, you will need to obtain permission directly from the copyright holder. To view a copy of this licence, visit http://creativecommons.org/licenses/by/4.0/ .
Reprints and permissions
About this article
Read, J., Sharma, S. Hypothesis-driven science in large-scale studies: the case of GWAS. Biol Philos 36 , 46 (2021). https://doi.org/10.1007/s10539-021-09823-0
Download citation
Received : 24 May 2021
Accepted : 08 September 2021
Published : 19 September 2021
DOI : https://doi.org/10.1007/s10539-021-09823-0
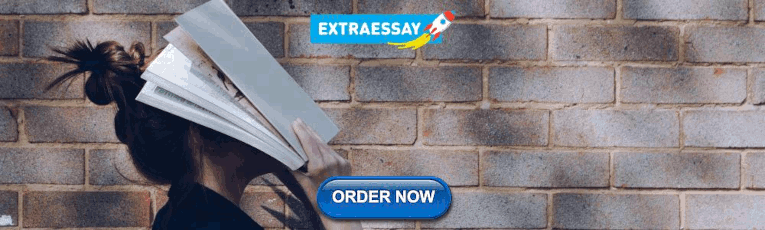
Share this article
Anyone you share the following link with will be able to read this content:
Sorry, a shareable link is not currently available for this article.
Provided by the Springer Nature SharedIt content-sharing initiative
- Systems biology
- Hypothesis-driven science
- Machine learning
- Find a journal
- Publish with us
- Track your research
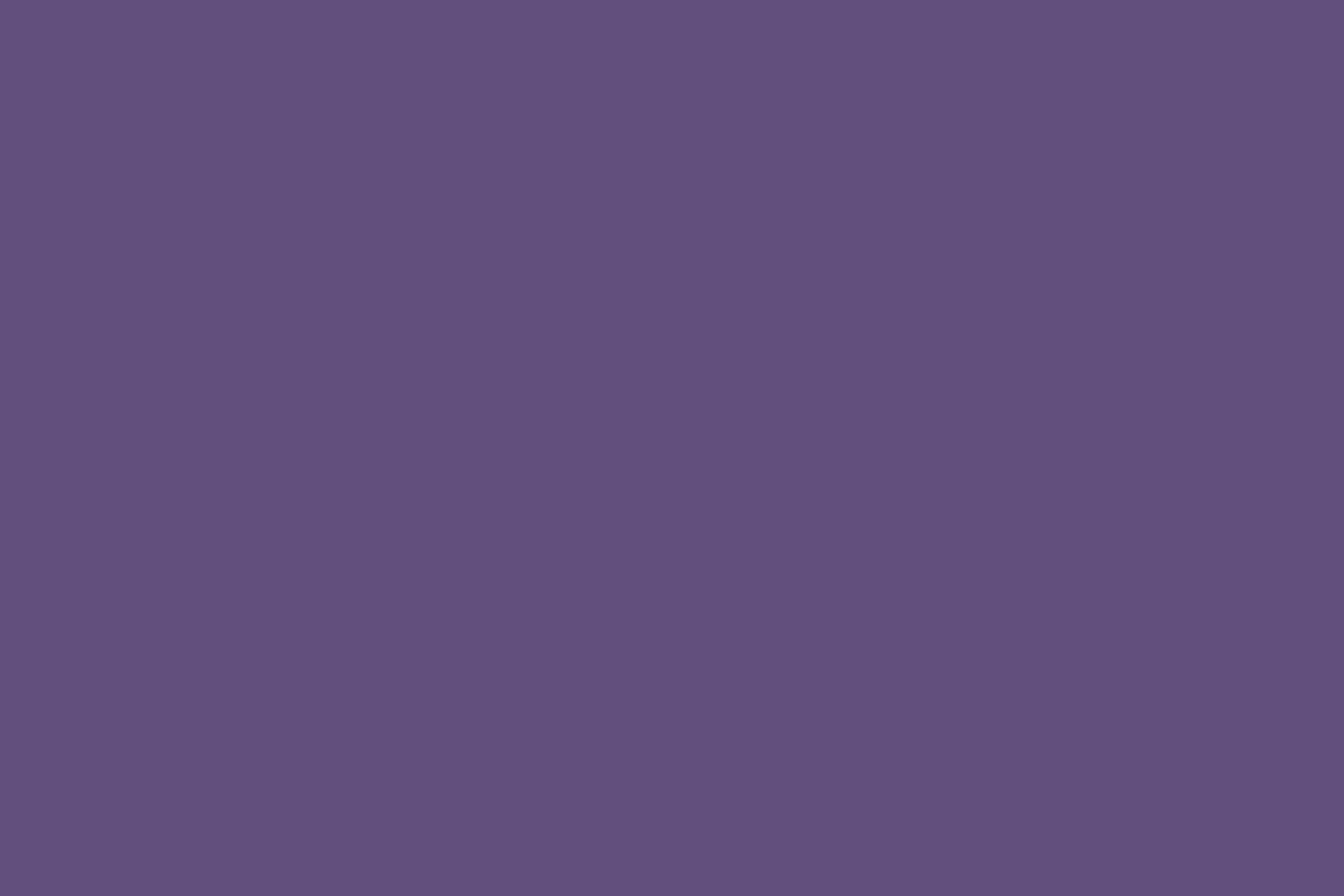
How to Implement Hypothesis-Driven Development
Remember back to the time when we were in high school science class. Our teachers had a framework for helping us learn – an experimental approach based on the best available evidence at hand. We were asked to make observations about the world around us, then attempt to form an explanation or hypothesis to explain what we had observed. We then tested this hypothesis by predicting an outcome based on our theory that would be achieved in a controlled experiment – if the outcome was achieved, we had proven our theory to be correct.
We could then apply this learning to inform and test other hypotheses by constructing more sophisticated experiments, and tuning, evolving or abandoning any hypothesis as we made further observations from the results we achieved.
Experimentation is the foundation of the scientific method, which is a systematic means of exploring the world around us. Although some experiments take place in laboratories, it is possible to perform an experiment anywhere, at any time, even in software development.
Practicing Hypothesis-Driven Development is thinking about the development of new ideas, products and services – even organizational change – as a series of experiments to determine whether an expected outcome will be achieved. The process is iterated upon until a desirable outcome is obtained or the idea is determined to be not viable.
We need to change our mindset to view our proposed solution to a problem statement as a hypothesis, especially in new product or service development – the market we are targeting, how a business model will work, how code will execute and even how the customer will use it.
We do not do projects anymore, only experiments. Customer discovery and Lean Startup strategies are designed to test assumptions about customers. Quality Assurance is testing system behavior against defined specifications. The experimental principle also applies in Test-Driven Development – we write the test first, then use the test to validate that our code is correct, and succeed if the code passes the test. Ultimately, product or service development is a process to test a hypothesis about system behaviour in the environment or market it is developed for.
The key outcome of an experimental approach is measurable evidence and learning.
Learning is the information we have gained from conducting the experiment. Did what we expect to occur actually happen? If not, what did and how does that inform what we should do next?
In order to learn we need use the scientific method for investigating phenomena, acquiring new knowledge, and correcting and integrating previous knowledge back into our thinking.
As the software development industry continues to mature, we now have an opportunity to leverage improved capabilities such as Continuous Design and Delivery to maximize our potential to learn quickly what works and what does not. By taking an experimental approach to information discovery, we can more rapidly test our solutions against the problems we have identified in the products or services we are attempting to build. With the goal to optimize our effectiveness of solving the right problems, over simply becoming a feature factory by continually building solutions.
The steps of the scientific method are to:
- Make observations
- Formulate a hypothesis
- Design an experiment to test the hypothesis
- State the indicators to evaluate if the experiment has succeeded
- Conduct the experiment
- Evaluate the results of the experiment
- Accept or reject the hypothesis
- If necessary, make and test a new hypothesis
Using an experimentation approach to software development
We need to challenge the concept of having fixed requirements for a product or service. Requirements are valuable when teams execute a well known or understood phase of an initiative, and can leverage well understood practices to achieve the outcome. However, when you are in an exploratory, complex and uncertain phase you need hypotheses.
Handing teams a set of business requirements reinforces an order-taking approach and mindset that is flawed.
Business does the thinking and ‘knows’ what is right. The purpose of the development team is to implement what they are told. But when operating in an area of uncertainty and complexity, all the members of the development team should be encouraged to think and share insights on the problem and potential solutions. A team simply taking orders from a business owner is not utilizing the full potential, experience and competency that a cross-functional multi-disciplined team offers.
Framing hypotheses
The traditional user story framework is focused on capturing requirements for what we want to build and for whom, to enable the user to receive a specific benefit from the system.
As A…. <role>
I Want… <goal/desire>
So That… <receive benefit>
Behaviour Driven Development (BDD) and Feature Injection aims to improve the original framework by supporting communication and collaboration between developers, tester and non-technical participants in a software project.
In Order To… <receive benefit>
As A… <role>
When viewing work as an experiment, the traditional story framework is insufficient. As in our high school science experiment, we need to define the steps we will take to achieve the desired outcome. We then need to state the specific indicators (or signals) we expect to observe that provide evidence that our hypothesis is valid. These need to be stated before conducting the test to reduce biased interpretations of the results.
If we observe signals that indicate our hypothesis is correct, we can be more confident that we are on the right path and can alter the user story framework to reflect this.
Therefore, a user story structure to support Hypothesis-Driven Development would be;
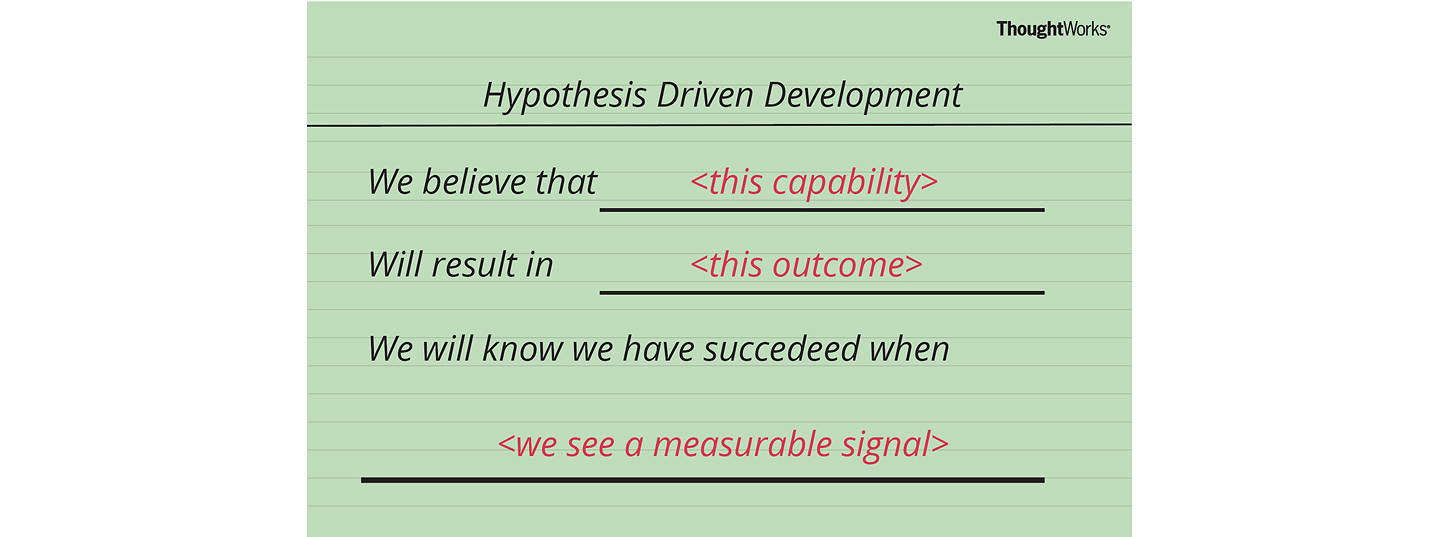
We believe < this capability >
What functionality we will develop to test our hypothesis? By defining a ‘test’ capability of the product or service that we are attempting to build, we identify the functionality and hypothesis we want to test.
Will result in < this outcome >
What is the expected outcome of our experiment? What is the specific result we expect to achieve by building the ‘test’ capability?
We will know we have succeeded when < we see a measurable signal >
What signals will indicate that the capability we have built is effective? What key metrics (qualitative or quantitative) we will measure to provide evidence that our experiment has succeeded and give us enough confidence to move to the next stage.
The threshold you use for statistically significance will depend on your understanding of the business and context you are operating within. Not every company has the user sample size of Amazon or Google to run statistically significant experiments in a short period of time. Limits and controls need to be defined by your organization to determine acceptable evidence thresholds that will allow the team to advance to the next step.
For example if you are building a rocket ship you may want your experiments to have a high threshold for statistical significance. If you are deciding between two different flows intended to help increase user sign up you may be happy to tolerate a lower significance threshold.
The final step is to clearly and visibly state any assumptions made about our hypothesis, to create a feedback loop for the team to provide further input, debate and understanding of the circumstance under which we are performing the test. Are they valid and make sense from a technical and business perspective?
Hypotheses when aligned to your MVP can provide a testing mechanism for your product or service vision. They can test the most uncertain areas of your product or service, in order to gain information and improve confidence.
Examples of Hypothesis-Driven Development user stories are;
Business story
We Believe That increasing the size of hotel images on the booking page
Will Result In improved customer engagement and conversion
We Will Know We Have Succeeded When we see a 5% increase in customers who review hotel images who then proceed to book in 48 hours.
It is imperative to have effective monitoring and evaluation tools in place when using an experimental approach to software development in order to measure the impact of our efforts and provide a feedback loop to the team. Otherwise we are essentially blind to the outcomes of our efforts.
In agile software development we define working software as the primary measure of progress.
By combining Continuous Delivery and Hypothesis-Driven Development we can now define working software and validated learning as the primary measures of progress.
Ideally we should not say we are done until we have measured the value of what is being delivered – in other words, gathered data to validate our hypothesis.
Examples of how to gather data is performing A/B Testing to test a hypothesis and measure to change in customer behaviour. Alternative testings options can be customer surveys, paper prototypes, user and/or guerrilla testing.
One example of a company we have worked with that uses Hypothesis-Driven Development is lastminute.com . The team formulated a hypothesis that customers are only willing to pay a max price for a hotel based on the time of day they book. Tom Klein, CEO and President of Sabre Holdings shared the story of how they improved conversion by 400% within a week.
Combining practices such as Hypothesis-Driven Development and Continuous Delivery accelerates experimentation and amplifies validated learning. This gives us the opportunity to accelerate the rate at which we innovate while relentlessly reducing cost, leaving our competitors in the dust. Ideally we can achieve the ideal of one piece flow: atomic changes that enable us to identify causal relationships between the changes we make to our products and services, and their impact on key metrics.
As Kent Beck said, “Test-Driven Development is a great excuse to think about the problem before you think about the solution”. Hypothesis-Driven Development is a great opportunity to test what you think the problem is, before you work on the solution.
How can you achieve faster growth?
Educational resources and simple solutions for your research journey
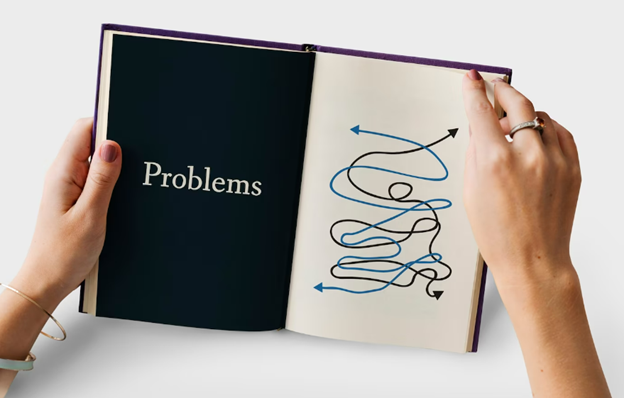
What is a Research Hypothesis: How to Write it, Types, and Examples
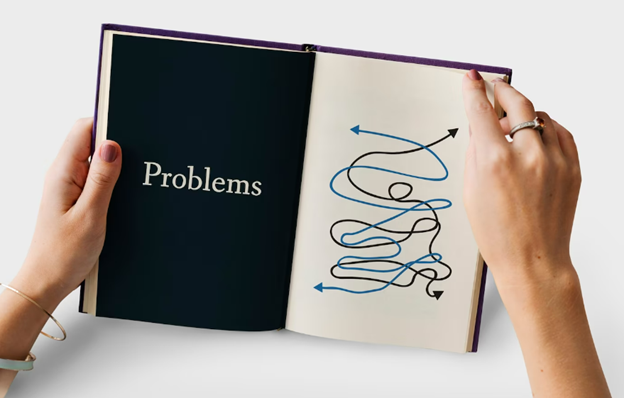
Any research begins with a research question and a research hypothesis . A research question alone may not suffice to design the experiment(s) needed to answer it. A hypothesis is central to the scientific method. But what is a hypothesis ? A hypothesis is a testable statement that proposes a possible explanation to a phenomenon, and it may include a prediction. Next, you may ask what is a research hypothesis ? Simply put, a research hypothesis is a prediction or educated guess about the relationship between the variables that you want to investigate.
It is important to be thorough when developing your research hypothesis. Shortcomings in the framing of a hypothesis can affect the study design and the results. A better understanding of the research hypothesis definition and characteristics of a good hypothesis will make it easier for you to develop your own hypothesis for your research. Let’s dive in to know more about the types of research hypothesis , how to write a research hypothesis , and some research hypothesis examples .
Table of Contents
What is a hypothesis ?
A hypothesis is based on the existing body of knowledge in a study area. Framed before the data are collected, a hypothesis states the tentative relationship between independent and dependent variables, along with a prediction of the outcome.
What is a research hypothesis ?
Young researchers starting out their journey are usually brimming with questions like “ What is a hypothesis ?” “ What is a research hypothesis ?” “How can I write a good research hypothesis ?”
A research hypothesis is a statement that proposes a possible explanation for an observable phenomenon or pattern. It guides the direction of a study and predicts the outcome of the investigation. A research hypothesis is testable, i.e., it can be supported or disproven through experimentation or observation.

Characteristics of a good hypothesis
Here are the characteristics of a good hypothesis :
- Clearly formulated and free of language errors and ambiguity
- Concise and not unnecessarily verbose
- Has clearly defined variables
- Testable and stated in a way that allows for it to be disproven
- Can be tested using a research design that is feasible, ethical, and practical
- Specific and relevant to the research problem
- Rooted in a thorough literature search
- Can generate new knowledge or understanding.
How to create an effective research hypothesis
A study begins with the formulation of a research question. A researcher then performs background research. This background information forms the basis for building a good research hypothesis . The researcher then performs experiments, collects, and analyzes the data, interprets the findings, and ultimately, determines if the findings support or negate the original hypothesis.
Let’s look at each step for creating an effective, testable, and good research hypothesis :
- Identify a research problem or question: Start by identifying a specific research problem.
- Review the literature: Conduct an in-depth review of the existing literature related to the research problem to grasp the current knowledge and gaps in the field.
- Formulate a clear and testable hypothesis : Based on the research question, use existing knowledge to form a clear and testable hypothesis . The hypothesis should state a predicted relationship between two or more variables that can be measured and manipulated. Improve the original draft till it is clear and meaningful.
- State the null hypothesis: The null hypothesis is a statement that there is no relationship between the variables you are studying.
- Define the population and sample: Clearly define the population you are studying and the sample you will be using for your research.
- Select appropriate methods for testing the hypothesis: Select appropriate research methods, such as experiments, surveys, or observational studies, which will allow you to test your research hypothesis .
Remember that creating a research hypothesis is an iterative process, i.e., you might have to revise it based on the data you collect. You may need to test and reject several hypotheses before answering the research problem.
How to write a research hypothesis
When you start writing a research hypothesis , you use an “if–then” statement format, which states the predicted relationship between two or more variables. Clearly identify the independent variables (the variables being changed) and the dependent variables (the variables being measured), as well as the population you are studying. Review and revise your hypothesis as needed.
An example of a research hypothesis in this format is as follows:
“ If [athletes] follow [cold water showers daily], then their [endurance] increases.”
Population: athletes
Independent variable: daily cold water showers
Dependent variable: endurance
You may have understood the characteristics of a good hypothesis . But note that a research hypothesis is not always confirmed; a researcher should be prepared to accept or reject the hypothesis based on the study findings.
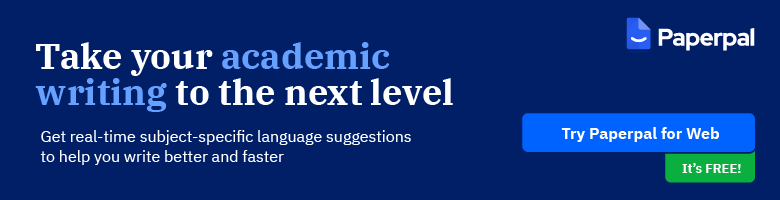
Research hypothesis checklist
Following from above, here is a 10-point checklist for a good research hypothesis :
- Testable: A research hypothesis should be able to be tested via experimentation or observation.
- Specific: A research hypothesis should clearly state the relationship between the variables being studied.
- Based on prior research: A research hypothesis should be based on existing knowledge and previous research in the field.
- Falsifiable: A research hypothesis should be able to be disproven through testing.
- Clear and concise: A research hypothesis should be stated in a clear and concise manner.
- Logical: A research hypothesis should be logical and consistent with current understanding of the subject.
- Relevant: A research hypothesis should be relevant to the research question and objectives.
- Feasible: A research hypothesis should be feasible to test within the scope of the study.
- Reflects the population: A research hypothesis should consider the population or sample being studied.
- Uncomplicated: A good research hypothesis is written in a way that is easy for the target audience to understand.
By following this research hypothesis checklist , you will be able to create a research hypothesis that is strong, well-constructed, and more likely to yield meaningful results.
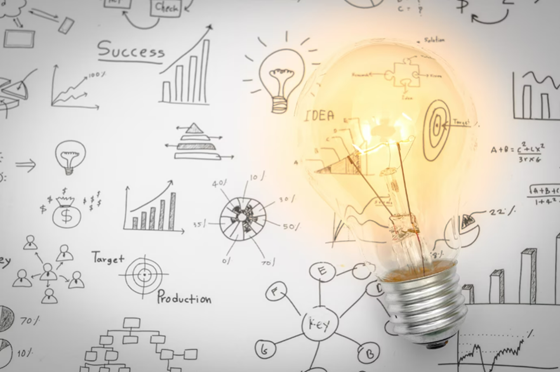
Types of research hypothesis
Different types of research hypothesis are used in scientific research:
1. Null hypothesis:
A null hypothesis states that there is no change in the dependent variable due to changes to the independent variable. This means that the results are due to chance and are not significant. A null hypothesis is denoted as H0 and is stated as the opposite of what the alternative hypothesis states.
Example: “ The newly identified virus is not zoonotic .”
2. Alternative hypothesis:
This states that there is a significant difference or relationship between the variables being studied. It is denoted as H1 or Ha and is usually accepted or rejected in favor of the null hypothesis.
Example: “ The newly identified virus is zoonotic .”
3. Directional hypothesis :
This specifies the direction of the relationship or difference between variables; therefore, it tends to use terms like increase, decrease, positive, negative, more, or less.
Example: “ The inclusion of intervention X decreases infant mortality compared to the original treatment .”
4. Non-directional hypothesis:
While it does not predict the exact direction or nature of the relationship between the two variables, a non-directional hypothesis states the existence of a relationship or difference between variables but not the direction, nature, or magnitude of the relationship. A non-directional hypothesis may be used when there is no underlying theory or when findings contradict previous research.
Example, “ Cats and dogs differ in the amount of affection they express .”
5. Simple hypothesis :
A simple hypothesis only predicts the relationship between one independent and another independent variable.
Example: “ Applying sunscreen every day slows skin aging .”
6 . Complex hypothesis :
A complex hypothesis states the relationship or difference between two or more independent and dependent variables.
Example: “ Applying sunscreen every day slows skin aging, reduces sun burn, and reduces the chances of skin cancer .” (Here, the three dependent variables are slowing skin aging, reducing sun burn, and reducing the chances of skin cancer.)
7. Associative hypothesis:
An associative hypothesis states that a change in one variable results in the change of the other variable. The associative hypothesis defines interdependency between variables.
Example: “ There is a positive association between physical activity levels and overall health .”
8 . Causal hypothesis:
A causal hypothesis proposes a cause-and-effect interaction between variables.
Example: “ Long-term alcohol use causes liver damage .”
Note that some of the types of research hypothesis mentioned above might overlap. The types of hypothesis chosen will depend on the research question and the objective of the study.
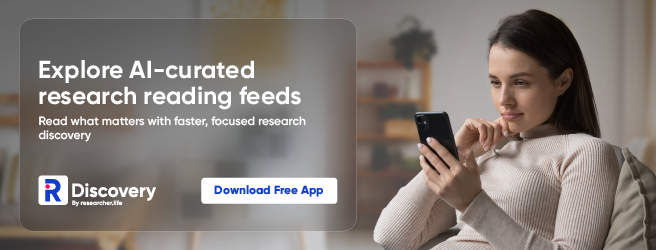
Research hypothesis examples
Here are some good research hypothesis examples :
“The use of a specific type of therapy will lead to a reduction in symptoms of depression in individuals with a history of major depressive disorder.”
“Providing educational interventions on healthy eating habits will result in weight loss in overweight individuals.”
“Plants that are exposed to certain types of music will grow taller than those that are not exposed to music.”
“The use of the plant growth regulator X will lead to an increase in the number of flowers produced by plants.”
Characteristics that make a research hypothesis weak are unclear variables, unoriginality, being too general or too vague, and being untestable. A weak hypothesis leads to weak research and improper methods.
Some bad research hypothesis examples (and the reasons why they are “bad”) are as follows:
“This study will show that treatment X is better than any other treatment . ” (This statement is not testable, too broad, and does not consider other treatments that may be effective.)
“This study will prove that this type of therapy is effective for all mental disorders . ” (This statement is too broad and not testable as mental disorders are complex and different disorders may respond differently to different types of therapy.)
“Plants can communicate with each other through telepathy . ” (This statement is not testable and lacks a scientific basis.)
Importance of testable hypothesis
If a research hypothesis is not testable, the results will not prove or disprove anything meaningful. The conclusions will be vague at best. A testable hypothesis helps a researcher focus on the study outcome and understand the implication of the question and the different variables involved. A testable hypothesis helps a researcher make precise predictions based on prior research.
To be considered testable, there must be a way to prove that the hypothesis is true or false; further, the results of the hypothesis must be reproducible.
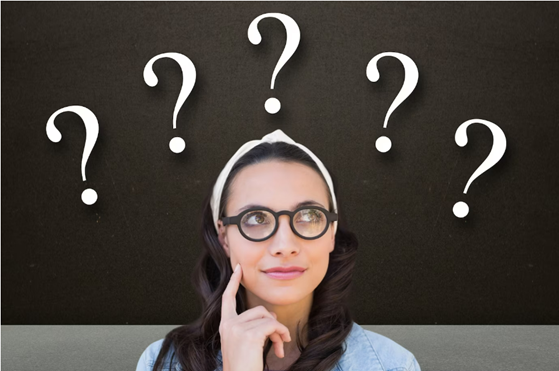
Frequently Asked Questions (FAQs) on research hypothesis
1. What is the difference between research question and research hypothesis ?
A research question defines the problem and helps outline the study objective(s). It is an open-ended statement that is exploratory or probing in nature. Therefore, it does not make predictions or assumptions. It helps a researcher identify what information to collect. A research hypothesis , however, is a specific, testable prediction about the relationship between variables. Accordingly, it guides the study design and data analysis approach.
2. When to reject null hypothesis ?
A null hypothesis should be rejected when the evidence from a statistical test shows that it is unlikely to be true. This happens when the test statistic (e.g., p -value) is less than the defined significance level (e.g., 0.05). Rejecting the null hypothesis does not necessarily mean that the alternative hypothesis is true; it simply means that the evidence found is not compatible with the null hypothesis.
3. How can I be sure my hypothesis is testable?
A testable hypothesis should be specific and measurable, and it should state a clear relationship between variables that can be tested with data. To ensure that your hypothesis is testable, consider the following:
- Clearly define the key variables in your hypothesis. You should be able to measure and manipulate these variables in a way that allows you to test the hypothesis.
- The hypothesis should predict a specific outcome or relationship between variables that can be measured or quantified.
- You should be able to collect the necessary data within the constraints of your study.
- It should be possible for other researchers to replicate your study, using the same methods and variables.
- Your hypothesis should be testable by using appropriate statistical analysis techniques, so you can draw conclusions, and make inferences about the population from the sample data.
- The hypothesis should be able to be disproven or rejected through the collection of data.
4. How do I revise my research hypothesis if my data does not support it?
If your data does not support your research hypothesis , you will need to revise it or develop a new one. You should examine your data carefully and identify any patterns or anomalies, re-examine your research question, and/or revisit your theory to look for any alternative explanations for your results. Based on your review of the data, literature, and theories, modify your research hypothesis to better align it with the results you obtained. Use your revised hypothesis to guide your research design and data collection. It is important to remain objective throughout the process.
5. I am performing exploratory research. Do I need to formulate a research hypothesis?
As opposed to “confirmatory” research, where a researcher has some idea about the relationship between the variables under investigation, exploratory research (or hypothesis-generating research) looks into a completely new topic about which limited information is available. Therefore, the researcher will not have any prior hypotheses. In such cases, a researcher will need to develop a post-hoc hypothesis. A post-hoc research hypothesis is generated after these results are known.
6. How is a research hypothesis different from a research question?
A research question is an inquiry about a specific topic or phenomenon, typically expressed as a question. It seeks to explore and understand a particular aspect of the research subject. In contrast, a research hypothesis is a specific statement or prediction that suggests an expected relationship between variables. It is formulated based on existing knowledge or theories and guides the research design and data analysis.
7. Can a research hypothesis change during the research process?
Yes, research hypotheses can change during the research process. As researchers collect and analyze data, new insights and information may emerge that require modification or refinement of the initial hypotheses. This can be due to unexpected findings, limitations in the original hypotheses, or the need to explore additional dimensions of the research topic. Flexibility is crucial in research, allowing for adaptation and adjustment of hypotheses to align with the evolving understanding of the subject matter.
8. How many hypotheses should be included in a research study?
The number of research hypotheses in a research study varies depending on the nature and scope of the research. It is not necessary to have multiple hypotheses in every study. Some studies may have only one primary hypothesis, while others may have several related hypotheses. The number of hypotheses should be determined based on the research objectives, research questions, and the complexity of the research topic. It is important to ensure that the hypotheses are focused, testable, and directly related to the research aims.
9. Can research hypotheses be used in qualitative research?
Yes, research hypotheses can be used in qualitative research, although they are more commonly associated with quantitative research. In qualitative research, hypotheses may be formulated as tentative or exploratory statements that guide the investigation. Instead of testing hypotheses through statistical analysis, qualitative researchers may use the hypotheses to guide data collection and analysis, seeking to uncover patterns, themes, or relationships within the qualitative data. The emphasis in qualitative research is often on generating insights and understanding rather than confirming or rejecting specific research hypotheses through statistical testing.
Editage All Access is a subscription-based platform that unifies the best AI tools and services designed to speed up, simplify, and streamline every step of a researcher’s journey. The Editage All Access Pack is a one-of-a-kind subscription that unlocks full access to an AI writing assistant, literature recommender, journal finder, scientific illustration tool, and exclusive discounts on professional publication services from Editage.
Based on 22+ years of experience in academia, Editage All Access empowers researchers to put their best research forward and move closer to success. Explore our top AI Tools pack, AI Tools + Publication Services pack, or Build Your Own Plan. Find everything a researcher needs to succeed, all in one place – Get All Access now starting at just $14 a month !
Related Posts

How to Make a Graphical Abstract for Your Research Paper (with Examples)

Leveraging AI in Research: Kick-Start Your Academic Year with Editage All Access
Type of Research projects Part 2: Hypothesis-driven versus hypothesis-generating research (1 August 2018)
Thank you for visiting nature.com. You are using a browser version with limited support for CSS. To obtain the best experience, we recommend you use a more up to date browser (or turn off compatibility mode in Internet Explorer). In the meantime, to ensure continued support, we are displaying the site without styles and JavaScript.
- View all journals
- Explore content
- About the journal
- Publish with us
- Sign up for alerts
- Review Article
- Published: 04 July 2024
Harnessing EHR data for health research
- Alice S. Tang ORCID: orcid.org/0000-0003-4745-0714 1 ,
- Sarah R. Woldemariam 1 ,
- Silvia Miramontes 1 ,
- Beau Norgeot ORCID: orcid.org/0000-0003-2629-701X 2 ,
- Tomiko T. Oskotsky ORCID: orcid.org/0000-0001-7393-5120 1 &
- Marina Sirota ORCID: orcid.org/0000-0002-7246-6083 1 , 3
Nature Medicine ( 2024 ) Cite this article
432 Accesses
13 Altmetric
Metrics details
- Computational biology and bioinformatics
- Machine learning
With the increasing availability of rich, longitudinal, real-world clinical data recorded in electronic health records (EHRs) for millions of patients, there is a growing interest in leveraging these records to improve the understanding of human health and disease and translate these insights into clinical applications. However, there is also a need to consider the limitations of these data due to various biases and to understand the impact of missing information. Recognizing and addressing these limitations can inform the design and interpretation of EHR-based informatics studies that avoid confusing or incorrect conclusions, particularly when applied to population or precision medicine. Here we discuss key considerations in the design, implementation and interpretation of EHR-based informatics studies, drawing from examples in the literature across hypothesis generation, hypothesis testing and machine learning applications. We outline the growing opportunities for EHR-based informatics studies, including association studies and predictive modeling, enabled by evolving AI capabilities—while addressing limitations and potential pitfalls to avoid.
This is a preview of subscription content, access via your institution
Access options
Access Nature and 54 other Nature Portfolio journals
Get Nature+, our best-value online-access subscription
24,99 € / 30 days
cancel any time
Subscribe to this journal
Receive 12 print issues and online access
195,33 € per year
only 16,28 € per issue
Buy this article
- Purchase on Springer Link
- Instant access to full article PDF
Prices may be subject to local taxes which are calculated during checkout
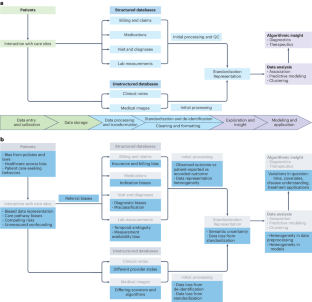
Similar content being viewed by others
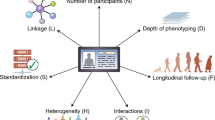
Axes of a revolution: challenges and promises of big data in healthcare
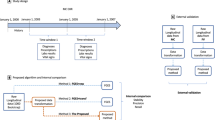
A novel method for causal structure discovery from EHR data and its application to type-2 diabetes mellitus
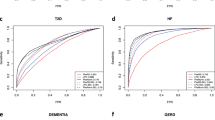
Quantitative disease risk scores from EHR with applications to clinical risk stratification and genetic studies
Gillum, R. F. From papyrus to the electronic tablet: a brief history of the clinical medical record with lessons for the digital age. Am. J. Med. 126 , 853–857 (2013).
Article PubMed Google Scholar
US Food and Drug Administration. Real-World Evidence. FDA https://www.fda.gov/science-research/science-and-research-special-topics/real-world-evidence/ (5 February 2023).
Office of the National Coordinator for Health Information Technology. National Trends in Hospital and Physician Adoption of Electronic Health Records. HealthIT.gov https://www.healthit.gov/data/quickstats/national-trends-hospital-and-physician-adoption-electronic-health-records/ (2021).
Liu, F. & Panagiotakos, D. Real-world data: a brief review of the methods, applications, challenges and opportunities. BMC Med. Res. Methodol. 22 , 287 (2022).
Article PubMed PubMed Central Google Scholar
Cowie, M. R. et al. Electronic health records to facilitate clinical research. Clin. Res. Cardiol. 106 , 1–9 (2017).
Kierkegaard, P. Electronic health record: wiring Europe’s healthcare. Comput. Law Secur. Rev. 27 , 503–515 (2011).
Article Google Scholar
Wen, H. -C., Chang, W. -P., Hsu, M. -H., Ho, C. -H. & Chu, C. -M. An assessment of the interoperability of electronic health record exchanges among hospitals and clinics in Taiwan. JMIR Med. Inform. 7 , e12630 (2019).
Johnson, A. E. W. et al. MIMIC-IV, a freely accessible electronic health record dataset. Sci. Data 10 , 1 (2023).
Article CAS PubMed PubMed Central Google Scholar
All of Us Research Program Investigators. The ‘All of Us’ Research Program. N. Engl. J. Med . 381 , 668–676 (2019).
Sudlow, C. et al. UK Biobank: an open access resource for identifying the causes of a wide range of complex diseases of middle and old age. PLoS Med. 12 , e1001779 (2015).
Sinha, P., Sunder, G., Bendale, P., Mantri, M. & Dande, A. Electronic Health Record: Standards, Coding Systems, Frameworks, and Infrastructures (Wiley, 2012); https://doi.org/10.1002/9781118479612
Overhage, J. M., Ryan, P. B., Reich, C. G., Hartzema, A. G. & Stang, P. E. Validation of a common data model for active safety surveillance research. J. Am. Med. Inform. Assoc. 19 , 54–60 (2012).
Murugadoss, K. et al. Building a best-in-class automated de-identification tool for electronic health records through ensemble learning. Patterns 2 , 100255 (2021).
Yogarajan, V., Pfahringer, B. & Mayo, M. A review of automatic end-to-end de-identification: is high accuracy the only metric? Appl. Artif. Intell. 34 , 251–269 (2020).
Mandl, K. D. & Perakslis, E. D. HIPAA and the leak of ‘deidentified’ EHR data. N. Engl. J. Med. 384 , 2171–2173 (2021).
Norgeot, B. et al. Protected Health Information filter (Philter): accurately and securely de-identifying free-text clinical notes. NPJ Digit. Med. 3 , 57 (2020).
Steurer, M. A. et al. Cohort study of respiratory hospital admissions, air quality and sociodemographic factors in preterm infants born in California. Paediatr. Perinat. Epidemiol. 34 , 130–138 (2020).
Costello, J. M., Steurer, M. A., Baer, R. J., Witte, J. S. & Jelliffe‐Pawlowski, L. L. Residential particulate matter, proximity to major roads, traffic density and traffic volume as risk factors for preterm birth in California. Paediatr. Perinat. Epidemiol. 36 , 70–79 (2022).
Yan, C. et al. Differences in health professionals’ engagement with electronic health records based on inpatient race and ethnicity. JAMA Netw. Open 6 , e2336383 (2023).
Lotfata, A., Moosazadeh, M., Helbich, M. & Hoseini, B. Socioeconomic and environmental determinants of asthma prevalence: a cross-sectional study at the U.S. county level using geographically weighted random forests. Int. J. Health Geogr. 22 , 18 (2023).
Li, L. et al. Identification of type 2 diabetes subgroups through topological analysis of patient similarity. Sci. Transl. Med. 7 , 311ra174 (2015).
De Freitas, J. K. et al. Phe2vec: automated disease phenotyping based on unsupervised embeddings from electronic health records. Patterns 2 , 100337 (2021).
Tang, A. S. et al. Deep phenotyping of Alzheimer’s disease leveraging electronic medical records identifies sex-specific clinical associations. Nat. Commun. 13 , 675 (2022).
Su, C. et al. Clinical subphenotypes in COVID-19: derivation, validation, prediction, temporal patterns, and interaction with social determinants of health. NPJ Digit. Med. 4 , 110 (2021).
Glicksberg, B. S. et al. PatientExploreR: an extensible application for dynamic visualization of patient clinical history from electronic health records in the OMOP common data model. Bioinformatics 35 , 4515–4518 (2019).
Huang, Z., Dong, W., Bath, P., Ji, L. & Duan, H. On mining latent treatment patterns from electronic medical records. Data Min. Knowl. Discov. 29 , 914–949 (2015).
Zaballa, O., Pérez, A., Gómez Inhiesto, E., Acaiturri Ayesta, T. & Lozano, J. A. Identifying common treatments from electronic health records with missing information. An application to breast cancer. PLoS ONE 15 , e0244004 (2020).
Lou, S. S., Liu, H., Harford, D., Lu, C. & Kannampallil, T. Characterizing the macrostructure of electronic health record work using raw audit logs: an unsupervised action embeddings approach. J. Am. Med. Inform. Assoc. 30 , 539–544 (2023).
Glicksberg, B. S. et al. Comparative analyses of population-scale phenomic data in electronic medical records reveal race-specific disease networks. Bioinformatics 32 , i101–i110 (2016).
Obermeyer, Z., Powers, B., Vogeli, C. & Mullainathan, S. Dissecting racial bias in an algorithm used to manage the health of populations. Science 366 , 447–453 (2019).
Article CAS PubMed Google Scholar
Smith, M. A. et al. Insights into measuring health disparities using electronic health records from a statewide network of health systems: a case study. J. Clin. Transl. Sci. 7 , e54 (2023).
Swerdel, J. N., Hripcsak, G. & Ryan, P. B. PheValuator: development and evaluation of a phenotype algorithm evaluator. J. Biomed. Inform. 97 , 103258 (2019).
Denny, J. C. et al. PheWAS: demonstrating the feasibility of a phenome-wide scan to discover gene-disease associations. Bioinformatics 26 , 1205–1210 (2010).
Chen, C., Ding, S. & Wang, J. Digital health for aging populations. Nat. Med. 29 , 1623–1630 (2023).
Woldemariam, S. R., Tang, A. S., Oskotsky, T. T., Yaffe, K. & Sirota, M. Similarities and differences in Alzheimer’s dementia comorbidities in racialized populations identified from electronic medical records. Commun. Med. 3 , 50 (2023).
Austin, P. C. An introduction to propensity score methods for reducing the effects of confounding in observational studies. Multivariate Behav. Res. 46 , 399–424 (2011).
Karlin, L. et al. Use of the propensity score matching method to reduce recruitment bias in observational studies: application to the estimation of survival benefit of non-myeloablative allogeneic transplantation in patients with multiple myeloma relapsing after a first autologous transplantation. Blood 112 , 1133 (2008).
Ho, D., Imai, K., King, G. & Stuart, E. A. MatchIt: nonparametric preprocessing for parametric causal inference. J. Stat. Softw. 42 , 8 (2011).
Zhang, Z., Kim, H. J., Lonjon, G. & Zhu, Y. Balance diagnostics after propensity score matching. Ann. Transl. Med. 7 , 16 (2019).
Landi, I. et al. Deep representation learning of electronic health records to unlock patient stratification at scale. NPJ Digit. Med. 3 , 96 (2020).
Bai, W. et al. A population-based phenome-wide association study of cardiac and aortic structure and function. Nat. Med . https://doi.org/10.1038/s41591-020-1009-y (2020).
Engels, E. A. et al. Comprehensive evaluation of medical conditions associated with risk of non-Hodgkin lymphoma using medicare claims (‘MedWAS’). Cancer Epidemiol. Biomark. Prev. 25 , 1105–1113 (2016).
Article CAS Google Scholar
Bastarache, L., Denny, J. C. & Roden, D. M. Phenome-wide association studies. J. Am. Med. Assoc. 327 , 75–76 (2022).
Yazdany, J. et al. Rheumatology informatics system for effectiveness: a national informatics‐enabled registry for quality improvement. Arthritis Care Res. 68 , 1866–1873 (2016).
Nelson, C. A., Bove, R., Butte, A. J. & Baranzini, S. E. Embedding electronic health records onto a knowledge network recognizes prodromal features of multiple sclerosis and predicts diagnosis. J. Am. Med. Inform. Assoc. 29 , 424–434 (2022).
Tang, A. S. et al. Leveraging electronic health records and knowledge networks for Alzheimer’s disease prediction and sex-specific biological insights. Nat. Aging 4 , 379–395 (2024).
Mullainathan, S. & Obermeyer, Z. Diagnosing physician error: a machine learning approach to low-value health care. Q. J. Econ. 137 , 679–727 (2022).
Makin, T. R. & Orban De Xivry, J. -J. Ten common statistical mistakes to watch out for when writing or reviewing a manuscript. eLife 8 , e48175 (2019).
Carrigan, G. et al. External comparator groups derived from real-world data used in support of regulatory decision making: use cases and challenges. Curr. Epidemiol. Rep. 9 , 326–337 (2022).
Hersh, W. R. et al. Caveats for the use of operational electronic health record data in comparative effectiveness research. Med. Care 51 , S30–S37 (2013).
Rudrapatna, V. A. & Butte, A. J. Opportunities and challenges in using real-world data for health care. J. Clin. Invest. 130 , 565–574 (2020).
Belthangady, C. et al. Causal deep learning reveals the comparative effectiveness of antihyperglycemic treatments in poorly controlled diabetes. Nat. Commun. 13 , 6921 (2022).
Roger, J. et al. Leveraging electronic health records to identify risk factors for recurrent pregnancy loss across two medical centers: a case–control study. Preprint at Res. Sq. https://doi.org/10.21203/rs.3.rs-2631220/v2 (2023).
Gervasi, S. S. et al. The potential for bias in machine learning and opportunities for health insurers to address it: article examines the potential for bias in machine learning and opportunities for health insurers to address it. Health Aff. 41 , 212–218 (2022).
Sai, S. et al. Generative AI for transformative healthcare: a comprehensive study of emerging models, applications, case studies, and limitations. IEEE Access 12 , 31078–31106 (2024).
Wang, M. et al. A systematic review of automatic text summarization for biomedical literature and EHRs. J. Am. Med. Inform. Assoc. 28 , 2287–2297 (2021).
Katsoulakis, E. et al. Digital twins for health: a scoping review. NPJ Digit. Med. 7 , 77 (2024).
Thirunavukarasu, A. J. et al. Large language models in medicine. Nat. Med. 29 , 1930–1940 (2023).
Meskó, B. & Topol, E. J. The imperative for regulatory oversight of large language models (or generative AI) in healthcare. NPJ Digit. Med. 6 , 120 (2023).
Hastings, J. Preventing harm from non-conscious bias in medical generative AI. Lancet Digit. Health 6 , e2–e3 (2024).
Lett, E., Asabor, E., Beltrán, S., Cannon, A. M. & Arah, O. A. Conceptualizing, contextualizing, and operationalizing race in quantitative health sciences research. Ann. Fam. Med. 20 , 157–163 (2022).
Belonwu, S. A. et al. Sex-stratified single-cell RNA-seq analysis identifies sex-specific and cell type-specific transcriptional responses in Alzheimer’s disease across two brain regions. Mol. Neurobiol. https://doi.org/10.1007/s12035-021-02591-8 (2021).
Krumholz, A. Driving and epilepsy: a review and reappraisal. J. Am. Med. Assoc. 265 , 622–626 (1991).
Xu, J. et al. Data-driven discovery of probable Alzheimer’s disease and related dementia subphenotypes using electronic health records. Learn. Health Syst. 4 , e10246 (2020).
Vyas, D. A., Eisenstein, L. G. & Jones, D. S. Hidden in plain sight—reconsidering the use of race correction in clinical algorithms. N. Engl. J. Med. 383 , 874–882 (2020).
Dagdelen, J. et al. Structured information extraction from scientific text with large language models. Nat. Commun. 15 , 1418 (2024).
Hu, Y. et al. Improving large language models for clinical named entity recognition via prompt engineering. J. Am. Med. Inform. Assoc. 27 , ocad259 (2024).
Microsoft. microsoft/FHIR-Converter (2024).
Torfi, A., Fox, E. A. & Reddy, C. K. Differentially private synthetic medical data generation using convolutional GANs. Inf. Sci. 586 , 485–500 (2022).
Yoon, J., Jordon, J. & van der Schaar, M. GAIN: missing data imputation using generative adversarial nets. Preprint at https://arxiv.org/abs/1806.02920v1 (2018).
Shi, J., Wang, D., Tesei, G. & Norgeot, B. Generating high-fidelity privacy-conscious synthetic patient data for causal effect estimation with multiple treatments. Front. Artif. Intell. 5 , 918813 (2022).
Stuart, E. A. Matching methods for causal inference: a review and a look forward. Stat. Sci. 25 , 1–21 (2010).
Murali, L., Gopakumar, G., Viswanathan, D. M. & Nedungadi, P. Towards electronic health record-based medical knowledge graph construction, completion, and applications: a literature study. J. Biomed. Inform. 143 , 104403 (2023).
Li, Y. et al. BEHRT: transformer for electronic health records. Sci. Rep. 10 , 7155 (2020).
Guo, L. L. et al. EHR foundation models improve robustness in the presence of temporal distribution shift. Sci. Rep. 13 , 3767 (2023).
Zhu, R. et al. Clinical pharmacology applications of real‐world data and real‐world evidence in drug development and approval—an industry perspective. Clin. Pharmacol. Ther. 114 , 751–767 (2023).
Voss, E. A. et al. Accuracy of an automated knowledge base for identifying drug adverse reactions. J. Biomed. Inform. 66 , 72–81 (2017).
Taubes, A. et al. Experimental and real-world evidence supporting the computational repurposing of bumetanide for APOE4-related Alzheimer’s disease. Nat. Aging 1 , 932–947 (2021).
Gold, R. et al. Using electronic health record-based clinical decision support to provide social risk-informed care in community health centers: protocol for the design and assessment of a clinical decision support tool. JMIR Res. Protoc. 10 , e31733 (2021).
Varga, A. N. et al. Dealing with confounding in observational studies: a scoping review of methods evaluated in simulation studies with single‐point exposure. Stat. Med. 42 , 487–516 (2023).
Carrigan, G. et al. Using electronic health records to derive control arms for early phase single‐arm lung cancer trials: proof‐of‐concept in randomized controlled trials. Clin. Pharmacol. Ther. 107 , 369–377 (2020).
Infante-Rivard, C. & Cusson, A. Reflection on modern methods: selection bias—a review of recent developments. Int. J. Epidemiol. 47 , 1714–1722 (2018).
Degtiar, I. & Rose, S. A review of generalizability and transportability. Annu. Rev. Stat. Appl. 10 , 501–524 (2023).
Badhwar, A. et al. A multiomics approach to heterogeneity in Alzheimer’s disease: focused review and roadmap. Brain 143 , 1315–1331 (2020).
Stuart, E. A. & Rubin, D. B. Matching with multiple control groups with adjustment for group differences. J. Educ. Behav. Stat. 33 , 279–306 (2008).
Hernan, M. A. & Robins, J. M. Causal Inference: What If (Taylor and Francis, 2024).
Hernan, M. A. Causal knowledge as a prerequisite for confounding evaluation: an application to birth defects epidemiology. Am. J. Epidemiol. 155 , 176–184 (2002).
Dang, L. E. et al. A causal roadmap for generating high-quality real-world evidence. J. Clin. Transl. Sci. 7 , e212 (2023).
Hernán, M. A. & Robins, J. M. Using big data to emulate a target trial when a randomized trial is not available. Am. J. Epidemiol. 183 , 758–764 (2016).
Oskotsky, T. et al. Mortality risk among patients with COVID-19 prescribed selective serotonin reuptake inhibitor antidepressants. JAMA Netw. Open 4 , e2133090 (2021).
Sperry, M. M. et al. Target-agnostic drug prediction integrated with medical record analysis uncovers differential associations of statins with increased survival in COVID-19 patients. PLoS Comput. Biol. 19 , e1011050 (2023).
Amit, G. et al. Antidepressant use during pregnancy and the risk of preterm birth – a cohort study. NPJ Womens Health 2 , 5 (2024); https://doi.org/10.1038/s44294-024-00008-0
Download references
Author information
Authors and affiliations.
Bakar Computational Health Sciences Institute, University of California, San Francisco, San Francisco, CA, USA
Alice S. Tang, Sarah R. Woldemariam, Silvia Miramontes, Tomiko T. Oskotsky & Marina Sirota
Qualified Health, Palo Alto, CA, USA
Beau Norgeot
Department of Pediatrics, University of California, San Francisco, San Francisco, CA, USA
Marina Sirota
You can also search for this author in PubMed Google Scholar
Corresponding author
Correspondence to Marina Sirota .
Ethics declarations
Competing interests.
B.N. is an employee at Qualified Health. The other authors declare no competing interests.
Peer review
Peer review information.
Nature Medicine thanks Wenbo Wu and the other, anonymous, reviewer(s) for their contribution to the peer review of this work. Primary Handling Editor: Karen O’Leary, in collaboration with the Nature Medicine team.
Additional information
Publisher’s note Springer Nature remains neutral with regard to jurisdictional claims in published maps and institutional affiliations.
Rights and permissions
Springer Nature or its licensor (e.g. a society or other partner) holds exclusive rights to this article under a publishing agreement with the author(s) or other rightsholder(s); author self-archiving of the accepted manuscript version of this article is solely governed by the terms of such publishing agreement and applicable law.
Reprints and permissions
About this article
Cite this article.
Tang, A.S., Woldemariam, S.R., Miramontes, S. et al. Harnessing EHR data for health research. Nat Med (2024). https://doi.org/10.1038/s41591-024-03074-8
Download citation
Received : 03 January 2024
Accepted : 17 May 2024
Published : 04 July 2024
DOI : https://doi.org/10.1038/s41591-024-03074-8
Share this article
Anyone you share the following link with will be able to read this content:
Sorry, a shareable link is not currently available for this article.
Provided by the Springer Nature SharedIt content-sharing initiative
Quick links
- Explore articles by subject
- Guide to authors
- Editorial policies
Sign up for the Nature Briefing newsletter — what matters in science, free to your inbox daily.

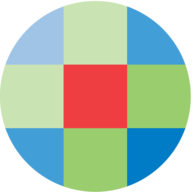
- Get new issue alerts Get alerts
- Submit a Manuscript
Secondary Logo
Journal logo.
Colleague's E-mail is Invalid
Your message has been successfully sent to your colleague.
Save my selection
Hypothesis-driven Research
Rao, Umadevi Krishnamohan
1 Department of Oral and Maxillofacial Pathology, Ragas Dental College and Hospital, Chennai, Tamil Nadu, India E-mail: [email protected]
This is an open access journal, and articles are distributed under the terms of the Creative Commons Attribution-NonCommercial-ShareAlike 4.0 License, which allows others to remix, tweak, and build upon the work non-commercially, as long as appropriate credit is given and the new creations are licensed under the identical terms.

As Oral Pathologists, we have the responsibility to upgrade our quality of service with an open mind attitude and gratitude for the contributions made by our professional colleagues. Teaching the students is the priority of the faculty, and with equal priority, oral pathologists have the responsibility to contribute to the literature too as a researcher.
Research is a scientific method of answering a question. This can be achieved when the work done in a representative sample of the population, i.e., the outcome of the result, can be applied to the rest of the population, from which the sample is drawn. In this context, frequently done research is a hypothesis-driven research which is based on scientific theories. Specific aims are listed in this type of research, and the objectives are stated. The scope of a well-designed methodology in a hypothesis-driven research equips the researcher to establish an opportunity to state the outcome of the study.
A provisional statement in which the relationship between two variables is described is known as hypothesis. It is very specific and offers the freedom of evaluating a prediction between the variables stated. It facilitates the researcher to envision and gauge as to what changes can occur in the listed specific outcome variables (dependent) when changes are made in a specific predictor (independent) variable. Thus, any given hypothesis should include both these variables, and the primary aim of the study should be focused on demonstrating the association between the variables, by maintaining the highest ethical standards.
The other requisites for a hypothesis-based study are we should state the level of statistical significance and should specify the power, which is defined as the probability that a statistical test will indicate a significant difference when it truly exists.[ 1 ] In a hypothesis-driven research, specifications of methodology help the grant reviewers to differentiate good science from bad science, and thus, hypothesis-driven research is the most funded research.[ 2 ]
“Hypotheses aren’t simply useful tools in some potentially outmoded vision of science; they are the whole point.” This was stated by Sean Carroll, from the California Institute of Technology, in response to Editor-In-Chief of “ Wired ” Chris Anderson, who argued that “biology is too complex for hypotheses and models, and he favored working on enormous data by correlative analysis.”[ 3 ]
Research does not stop by stating the hypotheses but must ensure that it is clear, testable and falsifiable and should serve as the fundamental basis for constructing a methodology that will allow either its acceptance (study favoring a null hypothesis) or rejection (study rejecting the null hypothesis in favor of the alternative hypothesis).
It is very worrying to observe that many research projects, which require a hypothesis, are being done without stating one. This is the fundamental backbone of the question to be asked and tested, and later, the findings need to be extrapolated in an analytical study, addressing a research question.
A good dissertation or thesis which is submitted for fulfillment of a curriculum or a submitted manuscript is comprised of a thoughtful study, addressing an interesting concept, and has to be scientifically designed. Nowadays, evolving academicians are in a competition to prove their point and be academically visible, which is very vital in their career graph. In any circumstance, unscientific research or short-cut methodology should never be conducted or encouraged to produce a research finding or publish the same as a manuscript.
The other type of research is exploratory research, which is based on a journey for discovery, which is not backed by previously established theories and is driven by hope and chance of breakthrough. The advantage of using these data is that statistics can be applied to establish predictions without the consideration of the principles of designing a study, which is the fundamental requirement of a conventional hypothesis. There is a need to set standards of statistical evidence with a much higher cutoff value for acceptance when we consider doing a study without a hypothesis.
In the past few years, there is an emergence of nonhypothesis-driven research, which does receive encouragement from funding agencies such as innovative molecular analysis technologies. The point to be taken here is that funding of nonhypothesis-driven research does not implicate decrease in support to hypothesis-driven research, but the objective is to encourage multidisciplinary research which is dependent on coordinated and cooperative execution of many branches of science and institutions. Thus, translational research is challenging and does carry a risk associated with the lack of preliminary data to establish a hypothesis.[ 4 ]
The merit of hypothesis testing is that it takes the next stride in scientific theory, having already stood the rigors of examination. Hypothesis testing is in practice for more than five decades and is considered to be a standard requirement when proposals are being submitted for evaluation. Stating a hypothesis is mandatory when we intend to make the study results applicable. Young professionals must be appraised of the merits of hypothesis-based research and must be trained to understand the scope of exploratory research.
- + Favorites
- View in Gallery
- Privacy Policy

Home » What is a Hypothesis – Types, Examples and Writing Guide
What is a Hypothesis – Types, Examples and Writing Guide
Table of Contents

Definition:
Hypothesis is an educated guess or proposed explanation for a phenomenon, based on some initial observations or data. It is a tentative statement that can be tested and potentially proven or disproven through further investigation and experimentation.
Hypothesis is often used in scientific research to guide the design of experiments and the collection and analysis of data. It is an essential element of the scientific method, as it allows researchers to make predictions about the outcome of their experiments and to test those predictions to determine their accuracy.
Types of Hypothesis
Types of Hypothesis are as follows:
Research Hypothesis
A research hypothesis is a statement that predicts a relationship between variables. It is usually formulated as a specific statement that can be tested through research, and it is often used in scientific research to guide the design of experiments.
Null Hypothesis
The null hypothesis is a statement that assumes there is no significant difference or relationship between variables. It is often used as a starting point for testing the research hypothesis, and if the results of the study reject the null hypothesis, it suggests that there is a significant difference or relationship between variables.
Alternative Hypothesis
An alternative hypothesis is a statement that assumes there is a significant difference or relationship between variables. It is often used as an alternative to the null hypothesis and is tested against the null hypothesis to determine which statement is more accurate.
Directional Hypothesis
A directional hypothesis is a statement that predicts the direction of the relationship between variables. For example, a researcher might predict that increasing the amount of exercise will result in a decrease in body weight.
Non-directional Hypothesis
A non-directional hypothesis is a statement that predicts the relationship between variables but does not specify the direction. For example, a researcher might predict that there is a relationship between the amount of exercise and body weight, but they do not specify whether increasing or decreasing exercise will affect body weight.
Statistical Hypothesis
A statistical hypothesis is a statement that assumes a particular statistical model or distribution for the data. It is often used in statistical analysis to test the significance of a particular result.
Composite Hypothesis
A composite hypothesis is a statement that assumes more than one condition or outcome. It can be divided into several sub-hypotheses, each of which represents a different possible outcome.
Empirical Hypothesis
An empirical hypothesis is a statement that is based on observed phenomena or data. It is often used in scientific research to develop theories or models that explain the observed phenomena.
Simple Hypothesis
A simple hypothesis is a statement that assumes only one outcome or condition. It is often used in scientific research to test a single variable or factor.
Complex Hypothesis
A complex hypothesis is a statement that assumes multiple outcomes or conditions. It is often used in scientific research to test the effects of multiple variables or factors on a particular outcome.
Applications of Hypothesis
Hypotheses are used in various fields to guide research and make predictions about the outcomes of experiments or observations. Here are some examples of how hypotheses are applied in different fields:
- Science : In scientific research, hypotheses are used to test the validity of theories and models that explain natural phenomena. For example, a hypothesis might be formulated to test the effects of a particular variable on a natural system, such as the effects of climate change on an ecosystem.
- Medicine : In medical research, hypotheses are used to test the effectiveness of treatments and therapies for specific conditions. For example, a hypothesis might be formulated to test the effects of a new drug on a particular disease.
- Psychology : In psychology, hypotheses are used to test theories and models of human behavior and cognition. For example, a hypothesis might be formulated to test the effects of a particular stimulus on the brain or behavior.
- Sociology : In sociology, hypotheses are used to test theories and models of social phenomena, such as the effects of social structures or institutions on human behavior. For example, a hypothesis might be formulated to test the effects of income inequality on crime rates.
- Business : In business research, hypotheses are used to test the validity of theories and models that explain business phenomena, such as consumer behavior or market trends. For example, a hypothesis might be formulated to test the effects of a new marketing campaign on consumer buying behavior.
- Engineering : In engineering, hypotheses are used to test the effectiveness of new technologies or designs. For example, a hypothesis might be formulated to test the efficiency of a new solar panel design.
How to write a Hypothesis
Here are the steps to follow when writing a hypothesis:
Identify the Research Question
The first step is to identify the research question that you want to answer through your study. This question should be clear, specific, and focused. It should be something that can be investigated empirically and that has some relevance or significance in the field.
Conduct a Literature Review
Before writing your hypothesis, it’s essential to conduct a thorough literature review to understand what is already known about the topic. This will help you to identify the research gap and formulate a hypothesis that builds on existing knowledge.
Determine the Variables
The next step is to identify the variables involved in the research question. A variable is any characteristic or factor that can vary or change. There are two types of variables: independent and dependent. The independent variable is the one that is manipulated or changed by the researcher, while the dependent variable is the one that is measured or observed as a result of the independent variable.
Formulate the Hypothesis
Based on the research question and the variables involved, you can now formulate your hypothesis. A hypothesis should be a clear and concise statement that predicts the relationship between the variables. It should be testable through empirical research and based on existing theory or evidence.
Write the Null Hypothesis
The null hypothesis is the opposite of the alternative hypothesis, which is the hypothesis that you are testing. The null hypothesis states that there is no significant difference or relationship between the variables. It is important to write the null hypothesis because it allows you to compare your results with what would be expected by chance.
Refine the Hypothesis
After formulating the hypothesis, it’s important to refine it and make it more precise. This may involve clarifying the variables, specifying the direction of the relationship, or making the hypothesis more testable.
Examples of Hypothesis
Here are a few examples of hypotheses in different fields:
- Psychology : “Increased exposure to violent video games leads to increased aggressive behavior in adolescents.”
- Biology : “Higher levels of carbon dioxide in the atmosphere will lead to increased plant growth.”
- Sociology : “Individuals who grow up in households with higher socioeconomic status will have higher levels of education and income as adults.”
- Education : “Implementing a new teaching method will result in higher student achievement scores.”
- Marketing : “Customers who receive a personalized email will be more likely to make a purchase than those who receive a generic email.”
- Physics : “An increase in temperature will cause an increase in the volume of a gas, assuming all other variables remain constant.”
- Medicine : “Consuming a diet high in saturated fats will increase the risk of developing heart disease.”
Purpose of Hypothesis
The purpose of a hypothesis is to provide a testable explanation for an observed phenomenon or a prediction of a future outcome based on existing knowledge or theories. A hypothesis is an essential part of the scientific method and helps to guide the research process by providing a clear focus for investigation. It enables scientists to design experiments or studies to gather evidence and data that can support or refute the proposed explanation or prediction.
The formulation of a hypothesis is based on existing knowledge, observations, and theories, and it should be specific, testable, and falsifiable. A specific hypothesis helps to define the research question, which is important in the research process as it guides the selection of an appropriate research design and methodology. Testability of the hypothesis means that it can be proven or disproven through empirical data collection and analysis. Falsifiability means that the hypothesis should be formulated in such a way that it can be proven wrong if it is incorrect.
In addition to guiding the research process, the testing of hypotheses can lead to new discoveries and advancements in scientific knowledge. When a hypothesis is supported by the data, it can be used to develop new theories or models to explain the observed phenomenon. When a hypothesis is not supported by the data, it can help to refine existing theories or prompt the development of new hypotheses to explain the phenomenon.
When to use Hypothesis
Here are some common situations in which hypotheses are used:
- In scientific research , hypotheses are used to guide the design of experiments and to help researchers make predictions about the outcomes of those experiments.
- In social science research , hypotheses are used to test theories about human behavior, social relationships, and other phenomena.
- I n business , hypotheses can be used to guide decisions about marketing, product development, and other areas. For example, a hypothesis might be that a new product will sell well in a particular market, and this hypothesis can be tested through market research.
Characteristics of Hypothesis
Here are some common characteristics of a hypothesis:
- Testable : A hypothesis must be able to be tested through observation or experimentation. This means that it must be possible to collect data that will either support or refute the hypothesis.
- Falsifiable : A hypothesis must be able to be proven false if it is not supported by the data. If a hypothesis cannot be falsified, then it is not a scientific hypothesis.
- Clear and concise : A hypothesis should be stated in a clear and concise manner so that it can be easily understood and tested.
- Based on existing knowledge : A hypothesis should be based on existing knowledge and research in the field. It should not be based on personal beliefs or opinions.
- Specific : A hypothesis should be specific in terms of the variables being tested and the predicted outcome. This will help to ensure that the research is focused and well-designed.
- Tentative: A hypothesis is a tentative statement or assumption that requires further testing and evidence to be confirmed or refuted. It is not a final conclusion or assertion.
- Relevant : A hypothesis should be relevant to the research question or problem being studied. It should address a gap in knowledge or provide a new perspective on the issue.
Advantages of Hypothesis
Hypotheses have several advantages in scientific research and experimentation:
- Guides research: A hypothesis provides a clear and specific direction for research. It helps to focus the research question, select appropriate methods and variables, and interpret the results.
- Predictive powe r: A hypothesis makes predictions about the outcome of research, which can be tested through experimentation. This allows researchers to evaluate the validity of the hypothesis and make new discoveries.
- Facilitates communication: A hypothesis provides a common language and framework for scientists to communicate with one another about their research. This helps to facilitate the exchange of ideas and promotes collaboration.
- Efficient use of resources: A hypothesis helps researchers to use their time, resources, and funding efficiently by directing them towards specific research questions and methods that are most likely to yield results.
- Provides a basis for further research: A hypothesis that is supported by data provides a basis for further research and exploration. It can lead to new hypotheses, theories, and discoveries.
- Increases objectivity: A hypothesis can help to increase objectivity in research by providing a clear and specific framework for testing and interpreting results. This can reduce bias and increase the reliability of research findings.
Limitations of Hypothesis
Some Limitations of the Hypothesis are as follows:
- Limited to observable phenomena: Hypotheses are limited to observable phenomena and cannot account for unobservable or intangible factors. This means that some research questions may not be amenable to hypothesis testing.
- May be inaccurate or incomplete: Hypotheses are based on existing knowledge and research, which may be incomplete or inaccurate. This can lead to flawed hypotheses and erroneous conclusions.
- May be biased: Hypotheses may be biased by the researcher’s own beliefs, values, or assumptions. This can lead to selective interpretation of data and a lack of objectivity in research.
- Cannot prove causation: A hypothesis can only show a correlation between variables, but it cannot prove causation. This requires further experimentation and analysis.
- Limited to specific contexts: Hypotheses are limited to specific contexts and may not be generalizable to other situations or populations. This means that results may not be applicable in other contexts or may require further testing.
- May be affected by chance : Hypotheses may be affected by chance or random variation, which can obscure or distort the true relationship between variables.
About the author
Muhammad Hassan
Researcher, Academic Writer, Web developer
You may also like

Thesis Statement – Examples, Writing Guide

Thesis – Structure, Example and Writing Guide

Assignment – Types, Examples and Writing Guide

Data Verification – Process, Types and Examples

Background of The Study – Examples and Writing...

Research Recommendations – Examples and Writing...
- Faculty/Staff
- MyMichiganTech
- Safety Data Sheets
- Website Settings
- Engineering
- Materials Science and Engineering
- Preparation of the PhD Qualifying Exam Proposal
Hypothesis-Based Research
Mse phd research proposal, two types of research proposals, exploratory.
"We think we can make something better or find out what is going on in this interesting area if we try a bunch of things and apply several sophisticated techniques to study this."
These proposals are pretty easy to write, but the undisciplined nature of the research may result in significant waste.
Hypothesis-based
"This area has a particular point with a lack of understanding. Based on the previous studies, we think this explanation applies here. We propose these experiments to test this explanation."
These proposals are very hard to write, but the inherent design forces a conclusion with efficient use of resources.
Michigan Tech MSE has decided to strongly emphasize hypothesis-based research in the PhD qualifier.
Wiki Definition
A hypothesis is a proposed explanation for a phenomenon. For a hypothesis to be put forward in science or engineering, the scientific method requires that one can test it. Scientists/engineers generally base hypotheses on previous observations that cannot satisfactorily be explained with the available scientific theories.
Previous Observations
Your hypothesis must be based on previous observations from the literature or your laboratory. You should be very familiar with the previous work in the subject area of your hypothesis.
- Your hypothesis needs to be based on some observations or ideas, while at the same time it must be original.
- You need to have a good familiarity with the literature related to your work. Your panel members may look at a literature search related to your proposal for a couple of hours before your presentation. You need to be aware of anything they may find. Do not let a cursory review of the literature by your panel "show you up."
- You can use the literature to justify your hypothesis by showing there is an open question regarding a particular phenomenon, process, design, approach, etc.
Your hypothesis must be testable in that there is some proposed analysis or experimentation that will produce data that can be quantitatively compared to the prediction of your hypothesis.
- The research that you propose should be focused on testing your hypothesis. The approach should be explained in a step by step, detailed manner. A superficial description that expects the panel to assume details of the experimental method, statistics of error and method of comparison with predictions of hypothesis may be deem unsatisfactory.
- You may want to create an experimental design matrix which shows which independent variables will be varied and over what range, and what dependent variables you intend to measure. Be realistic about how many experiments are planned. Note that parameter space can be explored in numerical models as well as in the laboratory.
- If possible, a realistic assessment of error, sensitivity or statistical significance of experimental or numerical data is helpful.
This example is not the only way to test a hypothesis.
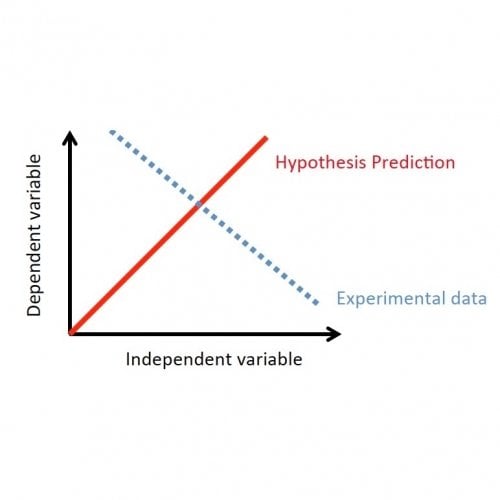
Non-trivial
Your hypothesis must be non-trivial in that it cannot be explained by simple application of well known laws.
Trivial Hypotheses
The observed chemical transformation from A to B occurs because there is a negative free energy change.
The solidification occurs because the liquid is cooled below the melting temperature.
The yield stress of Al will increase when it is alloyed to make a solid solution.

An official website of the United States government
The .gov means it’s official. Federal government websites often end in .gov or .mil. Before sharing sensitive information, make sure you’re on a federal government site.
The site is secure. The https:// ensures that you are connecting to the official website and that any information you provide is encrypted and transmitted securely.
- Publications
- Account settings
- My Bibliography
- Collections
- Citation manager
Save citation to file
Email citation, add to collections.
- Create a new collection
- Add to an existing collection
Add to My Bibliography
Your saved search, create a file for external citation management software, your rss feed.
- Search in PubMed
- Search in NLM Catalog
- Add to Search
A Comparison of Hypothesis-Driven and Data-Driven Research: A Case Study in Multimodal Data Science in Gut-Brain Axis Research
Affiliation.
- 1 Author Affiliations: Data Science Institute, Columbia University, New York, NY (Dr Dreisbach); and Translational Biobehavioral and Health Disparities Branch, National Institutes of Health Clinical Center (Dr Maki), Bethesda, MD.
- PMID: 36730994
- PMCID: PMC10102251
- DOI: 10.1097/CIN.0000000000000954
Data science, bioinformatics, and machine learning are the advent and progression of the fourth paradigm of exploratory science. The need for human-supported algorithms to capture patterns in big data is at the center of personalized healthcare and directly related to translational research. This paper argues that hypothesis-driven and data-driven research work together to inform the research process. At the core of these approaches are theoretical underpinnings that drive progress in the field. Here, we present several exemplars of research on the gut-brain axis that outline the innate values and challenges of these approaches. As nurses are trained to integrate multiple body systems to inform holistic human health promotion and disease prevention, nurses and nurse scientists serve an important role as mediators between this advancing technology and the patients. At the center of person-knowing, nurses need to be aware of the data revolution and use their unique skills to supplement the data science cycle from data to knowledge to insight.
Copyright © 2022 Wolters Kluwer Health, Inc. All rights reserved.
PubMed Disclaimer
Conflict of interest statement
Conflict of Interest : The author(s) declared no potential conflicts of interest with respect to the research, authorship, and/or publication of this article.
Figure 1.. Examples of Biomarker and Patient…
Figure 1.. Examples of Biomarker and Patient Phenotype Features in Gut-Brain Axis Research
MRI: Magnetic…
Comparison of data-driven and hypothesis-driven…
Comparison of data-driven and hypothesis-driven approaches.
Similar articles
- ASAS-NANP symposium: mathematical modeling in animal nutrition-Making sense of big data and machine learning: how open-source code can advance training of animal scientists. Brennan JR, Menendez HM 3rd, Ehlert K, Tedeschi LO. Brennan JR, et al. J Anim Sci. 2023 Jan 3;101:skad317. doi: 10.1093/jas/skad317. J Anim Sci. 2023. PMID: 37997926
- The role of data science in healthcare advancements: applications, benefits, and future prospects. Subrahmanya SVG, Shetty DK, Patil V, Hameed BMZ, Paul R, Smriti K, Naik N, Somani BK. Subrahmanya SVG, et al. Ir J Med Sci. 2022 Aug;191(4):1473-1483. doi: 10.1007/s11845-021-02730-z. Epub 2021 Aug 16. Ir J Med Sci. 2022. PMID: 34398394 Free PMC article. Review.
- How Can Big Data Science Transform the Psychological Sciences? Albritton BH, Tonidandel S. Albritton BH, et al. Span J Psychol. 2020 Nov 5;23:e44. doi: 10.1017/SJP.2020.45. Span J Psychol. 2020. PMID: 33148362
- Machine learning and big data analytics in bipolar disorder: A position paper from the International Society for Bipolar Disorders Big Data Task Force. Passos IC, Ballester PL, Barros RC, Librenza-Garcia D, Mwangi B, Birmaher B, Brietzke E, Hajek T, Lopez Jaramillo C, Mansur RB, Alda M, Haarman BCM, Isometsa E, Lam RW, McIntyre RS, Minuzzi L, Kessing LV, Yatham LN, Duffy A, Kapczinski F. Passos IC, et al. Bipolar Disord. 2019 Nov;21(7):582-594. doi: 10.1111/bdi.12828. Epub 2019 Sep 18. Bipolar Disord. 2019. PMID: 31465619 Review.
- Big Data and Data Science in Critical Care. Sanchez-Pinto LN, Luo Y, Churpek MM. Sanchez-Pinto LN, et al. Chest. 2018 Nov;154(5):1239-1248. doi: 10.1016/j.chest.2018.04.037. Epub 2018 May 9. Chest. 2018. PMID: 29752973 Free PMC article. Review.
- Dhar V Data science and prediction. Commun ACM. 2013;56(12):64–73. doi:10.1145/2500499 - DOI
- Diniz WJS, Canduri F. REVIEW-ARTICLE Bioinformatics: an overview and its applications. Genet Mol Res. 2017;16(1). doi:10.4238/gmr16019645 - DOI - PubMed
- Kitchin R, McArdle G. What makes Big Data, Big Data? Exploring the ontological characteristics of 26 datasets. Big Data Soc. 2016;3(1). Accessed October 13, 2021. https://journals.sagepub.com/doi/10.1177/2053951716631130 - DOI
- Maki KA, Joseph, Ames NJ, Wallen GR. Leveraging Microbiome Science From the Bedside to Bench and Back: A Nursing Perspective. Nurs Res. 2021;70(1):3–5. doi:10.1097/NNR.0000000000000475 - DOI - PMC - PubMed
- Koza JR, Bennett FH, Andre D, Keane MA. Automated Design of Both the Topology and Sizing of Analog Electrical Circuits Using Genetic Programming. In: Gero JS, Sudweeks F, eds. Artificial Intelligence in Design ’96. Springer; Netherlands; 1996:151–170. doi:10.1007/978-94-009-0279-4_9 - DOI
- Search in MeSH
Grants and funding
- Z99 CL999999/ImNIH/Intramural NIH HHS/United States
LinkOut - more resources
Full text sources.
- Europe PubMed Central
- Ovid Technologies, Inc.
- PubMed Central
- Wolters Kluwer
Research Materials
- NCI CPTC Antibody Characterization Program
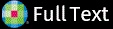
- Citation Manager
NCBI Literature Resources
MeSH PMC Bookshelf Disclaimer
The PubMed wordmark and PubMed logo are registered trademarks of the U.S. Department of Health and Human Services (HHS). Unauthorized use of these marks is strictly prohibited.
Your browser is not fully supported!
You are running an outdated browser version, which is not fully supported by OpenWHO. You might not be able to use crucial functionality such as the submission of quizzes . Please update your browser to the latest version before you continue (we recommend Mozilla Firefox or Google Chrome ).
Click here to hide this warning permanently.
- Discussions
- Certificates
- Collab Space
- Course Details
- Announcements
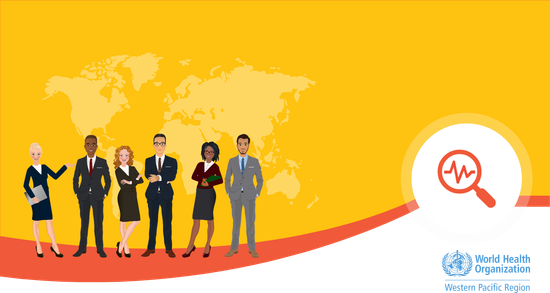
In this course, you will learn about the hypothesis-driven approach to problem-solving. This approach originated from academic research and later adopted in management consulting. The course consists of 4 modules that take you through a step-by-step process of solving problems in an effective and timely manner.
Course information
This course is also available in the following languages:
The hypothesis-driven approach is a problem-solving method that is necessary at WHO because the environment around us is changing rapidly. WHO needs a new way of problem-solving to process large amounts of information from different fields and deliver quick, tailored recommendations to meet the needs of Member States. The hypothesis-driven approach produces solutions quickly with continuous refinement throughout the research process.
What you'll learn
- Define the most important questions to address.
- Break down the question into components and develop an issue tree.
- Develop and validate the hypothesis.
- Synthesize findings and support recommendations by presenting evidence in a structured manner.
Who this course is for
- This course is for everyone. Whether your position is in administrative, operations, or technical area of work, you’re sure to run into problems to solve. Problem-solving is a key skill to continue developing and refining—the hypothesis-driven approach will surely be a great addition in your toolbox!
Course contents
Introduction: hypothesis-driven approach to problem solving:, module 1: identify the question:, module 2: develop & validate hypothesis:, module 3: synthesize findings & make recommendations:, enroll me for this course, certificate requirements.
- Gain a Record of Achievement by earning at least 80% of the maximum number of points from all graded assignments.
- Gain a Confirmation of Participation by completing at least 80% of the course material.
Peak Velocity
Warehouse native year in review, craig sexauer, a/b testing performance wins on nestjs api servers, stephen royal, an overview of making early decisions on experiments, mike london, statsig's eurotrip: a/b talks roadshow highlights, morgan scalzo, announcing the new suite of statsig javascript sdks, daniel loomb, how to export experimentation results, sophie saouma, statsig's autotune adds contextual bandits for personalization, how to add feature flags to next.js, brock lumbard, go from 0 to 1 with statsig's free suite of data tools for startups, the marketers go-to tech stack for website optimization, elizabeth george, experiment scorecards: essentials and best practices, ryan musser, what's the difference between statsig and posthog, margaret-ann seger, announcing statsig web analytics with autocapture, how to improve funnel conversion, how we use dynamic configs for distributed development at statsig, akin olugbade, try statsig today.
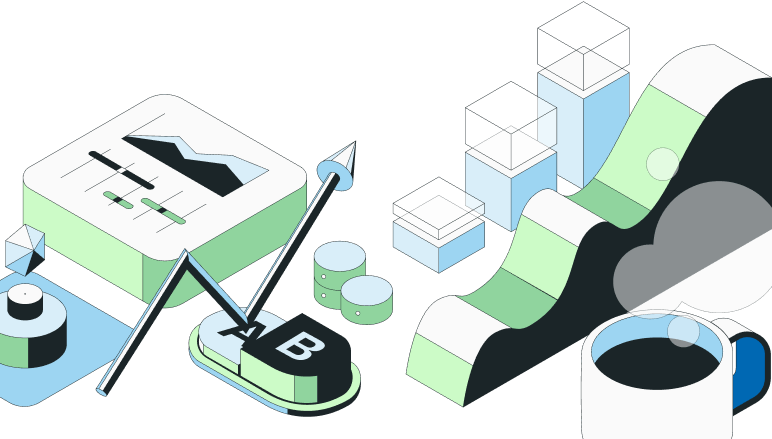
What builders love about us
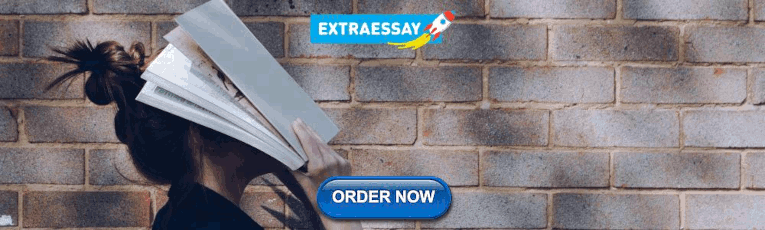
IMAGES
VIDEO
COMMENTS
What is hypothesis-driven science? The accumulation of knowledge about the world through the testing of causal theories (explanations). Science attempts to infer causal relationships ("If A, then B") by application of the scientific method. Unique to modern science.
The scope of a well-designed methodology in a hypothesis-driven research equips the researcher to establish an opportunity to state the outcome of the study. A provisional statement in which the relationship between two variables is described is known as hypothesis. It is very specific and offers the freedom of evaluating a prediction between ...
Some research is not hypothesis-driven. Terms used to describe non-hypothesis-driven research are 'descriptive research,' in which information is collected without a particular question in mind, and 'discovery science,' where large volumes of experimental data are analyzed with the goal of finding new patterns or correlations.
To explore these questions, we examine several fields and describe an historical progression in knowledge production. We believe that in these contexts large scale data collection and analysis represent the next step - going beyond the capabilities of todays simulation models with an empirical data-collection driven approach.
Hypothesis-driven research evaluates a proposed process or explanation (a hypothesis), finding the likelihood of the proposal being true.
A hypothesis is a statement that can be tested by scientific research. If you want to test a relationship between two or more variables, you need to write hypotheses before you start your experiment or data collection.
A research hypothesis is an assumption or a tentative explanation for a specific process observed during research. Unlike a guess, research hypothesis is a calculated, educated guess proven or disproven through research methods.
There are 5 main steps in hypothesis testing: State your research hypothesis as a null hypothesis and alternate hypothesis (H o) and (H a or H 1 ). Collect data in a way designed to test the hypothesis. Perform an appropriate statistical test. Decide whether to reject or fail to reject your null hypothesis. Present the findings in your results ...
A research hypothesis (also called a scientific hypothesis) is a statement about the expected outcome of a study (for example, a dissertation or thesis). To constitute a quality hypothesis, the statement needs to have three attributes - specificity, clarity and testability. Let's take a look at these more closely.
A hypothesis is a tentative statement about the relationship between two or more variables. Explore examples and learn how to format your research hypothesis.
Hypothesis generation is an early and critical step in any hypothesis-driven clinical research project. Because it is not yet a well-understood cognitive process, the need to improve the process goes unrecognized.
This paper has had two goals. The first has been to propose revisions to the framework of Ratti ( 2015) for the study of the role of hypothesis-driven research in large-scale contemporary biological studies, in light of studies such as GWAS and its associated missing heritability problem.
Practicing Hypothesis-Driven Development is thinking about the development of new ideas, products and services - even organizational change - as a series of experiments to determine whether an expected outcome will be achieved. The process is iterated upon until a desirable outcome is obtained or the idea is determined to be not viable.
Research begins with a research question and a research hypothesis. But what are the characteristics of a good hypothesis? In this article, we dive into the types of research hypothesis, explain how to write a research hypothesis, offer research hypothesis examples and answer top FAQs on research hypothesis. Read more!
(a) Hypothesis-driven approach to build multiple lines of evidence based on previous observations. Testing a hypothesis with multiple complementary experiments should yield interrelated results, allowing researchers to test more robustly their hypothesis considering the complexity of their study system.
This paper argues that hypothesis-driven and data-driven research work together to inform the research process. At the core of these approaches are theoretical underpinnings that drive progress in the field.
In hypothesis-driven research, we basically come up with a hypothesis that might explain a certain phenomenon. The hypothesis is usually based on doing prior research (published research or work in your own laboratory) and requires that you read, analyze and come up with a new idea.
Here we discuss key considerations in the design, implementation and interpretation of EHR-based informatics studies, drawing from examples in the literature across hypothesis generation ...
The other requisites for a hypothesis-based study are we should state the level of statistical significance and should specify the power, which is defined as the probability that a statistical test will indicate a significant difference when it truly exists. [ 1] In a hypothesis-driven research, specifications of methodology help the grant reviewers to differentiate good science from bad ...
Definition: Hypothesis is an educated guess or proposed explanation for a phenomenon, based on some initial observations or data. It is a tentative statement that can be tested and potentially proven or disproven through further investigation and experimentation. Hypothesis is often used in scientific research to guide the design of experiments ...
A hypothesis is a proposed explanation for a phenomenon. For a hypothesis to be put forward in science or engineering, the scientific method requires that one can test it. Scientists/engineers generally base hypotheses on previous observations that cannot satisfactorily be explained with the available scientific theories.
The need for human-supported algorithms to capture patterns in big data is at the center of personalized healthcare and directly related to translational research. This paper argues that hypothesis-driven and data-driven research work together to inform the research process.
In this course, you will learn about the hypothesis-driven approach to problem-solving. This approach originated from academic research and later adopted in management consulting. The course consists of 4 modules that take you through a step-by-step process of solving problems in an effective and timely manner.
Hypothesis and Non Hypothesis Research" \f C \l "1" Most scientific research is hypothesis-driven. That is, it seeks to address a specific, measurable, and answerable question, which may be intermediate to its ultimate objective, but essential to attaining the same.
Understanding statistical significance empowers researchers and businesses to make data-driven decisions based on reliable evidence.