data science Recently Published Documents
Total documents.
- Latest Documents
- Most Cited Documents
- Contributed Authors
- Related Sources
- Related Keywords
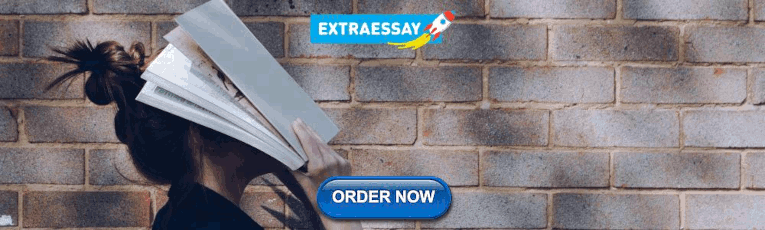
Assessing the effects of fuel energy consumption, foreign direct investment and GDP on CO2 emission: New data science evidence from Europe & Central Asia
Documentation matters: human-centered ai system to assist data science code documentation in computational notebooks.
Computational notebooks allow data scientists to express their ideas through a combination of code and documentation. However, data scientists often pay attention only to the code, and neglect creating or updating their documentation during quick iterations. Inspired by human documentation practices learned from 80 highly-voted Kaggle notebooks, we design and implement Themisto, an automated documentation generation system to explore how human-centered AI systems can support human data scientists in the machine learning code documentation scenario. Themisto facilitates the creation of documentation via three approaches: a deep-learning-based approach to generate documentation for source code, a query-based approach to retrieve online API documentation for source code, and a user prompt approach to nudge users to write documentation. We evaluated Themisto in a within-subjects experiment with 24 data science practitioners, and found that automated documentation generation techniques reduced the time for writing documentation, reminded participants to document code they would have ignored, and improved participants’ satisfaction with their computational notebook.
Data science in the business environment: Insight management for an Executive MBA
Adventures in financial data science, gecoagent: a conversational agent for empowering genomic data extraction and analysis.
With the availability of reliable and low-cost DNA sequencing, human genomics is relevant to a growing number of end-users, including biologists and clinicians. Typical interactions require applying comparative data analysis to huge repositories of genomic information for building new knowledge, taking advantage of the latest findings in applied genomics for healthcare. Powerful technology for data extraction and analysis is available, but broad use of the technology is hampered by the complexity of accessing such methods and tools. This work presents GeCoAgent, a big-data service for clinicians and biologists. GeCoAgent uses a dialogic interface, animated by a chatbot, for supporting the end-users’ interaction with computational tools accompanied by multi-modal support. While the dialogue progresses, the user is accompanied in extracting the relevant data from repositories and then performing data analysis, which often requires the use of statistical methods or machine learning. Results are returned using simple representations (spreadsheets and graphics), while at the end of a session the dialogue is summarized in textual format. The innovation presented in this article is concerned with not only the delivery of a new tool but also our novel approach to conversational technologies, potentially extensible to other healthcare domains or to general data science.
Differentially Private Medical Texts Generation Using Generative Neural Networks
Technological advancements in data science have offered us affordable storage and efficient algorithms to query a large volume of data. Our health records are a significant part of this data, which is pivotal for healthcare providers and can be utilized in our well-being. The clinical note in electronic health records is one such category that collects a patient’s complete medical information during different timesteps of patient care available in the form of free-texts. Thus, these unstructured textual notes contain events from a patient’s admission to discharge, which can prove to be significant for future medical decisions. However, since these texts also contain sensitive information about the patient and the attending medical professionals, such notes cannot be shared publicly. This privacy issue has thwarted timely discoveries on this plethora of untapped information. Therefore, in this work, we intend to generate synthetic medical texts from a private or sanitized (de-identified) clinical text corpus and analyze their utility rigorously in different metrics and levels. Experimental results promote the applicability of our generated data as it achieves more than 80\% accuracy in different pragmatic classification problems and matches (or outperforms) the original text data.
Impact on Stock Market across Covid-19 Outbreak
Abstract: This paper analysis the impact of pandemic over the global stock exchange. The stock listing values are determined by variety of factors including the seasonal changes, catastrophic calamities, pandemic, fiscal year change and many more. This paper significantly provides analysis on the variation of listing price over the world-wide outbreak of novel corona virus. The key reason to imply upon this outbreak was to provide notion on underlying regulation of stock exchanges. Daily closing prices of the stock indices from January 2017 to January 2022 has been utilized for the analysis. The predominant feature of the research is to analyse the fact that does global economy downfall impacts the financial stock exchange. Keywords: Stock Exchange, Matplotlib, Streamlit, Data Science, Web scrapping.
Information Resilience: the nexus of responsible and agile approaches to information use
AbstractThe appetite for effective use of information assets has been steadily rising in both public and private sector organisations. However, whether the information is used for social good or commercial gain, there is a growing recognition of the complex socio-technical challenges associated with balancing the diverse demands of regulatory compliance and data privacy, social expectations and ethical use, business process agility and value creation, and scarcity of data science talent. In this vision paper, we present a series of case studies that highlight these interconnected challenges, across a range of application areas. We use the insights from the case studies to introduce Information Resilience, as a scaffold within which the competing requirements of responsible and agile approaches to information use can be positioned. The aim of this paper is to develop and present a manifesto for Information Resilience that can serve as a reference for future research and development in relevant areas of responsible data management.
qEEG Analysis in the Diagnosis of Alzheimers Disease; a Comparison of Functional Connectivity and Spectral Analysis
Alzheimers disease (AD) is a brain disorder that is mainly characterized by a progressive degeneration of neurons in the brain, causing a decline in cognitive abilities and difficulties in engaging in day-to-day activities. This study compares an FFT-based spectral analysis against a functional connectivity analysis based on phase synchronization, for finding known differences between AD patients and Healthy Control (HC) subjects. Both of these quantitative analysis methods were applied on a dataset comprising bipolar EEG montages values from 20 diagnosed AD patients and 20 age-matched HC subjects. Additionally, an attempt was made to localize the identified AD-induced brain activity effects in AD patients. The obtained results showed the advantage of the functional connectivity analysis method compared to a simple spectral analysis. Specifically, while spectral analysis could not find any significant differences between the AD and HC groups, the functional connectivity analysis showed statistically higher synchronization levels in the AD group in the lower frequency bands (delta and theta), suggesting that the AD patients brains are in a phase-locked state. Further comparison of functional connectivity between the homotopic regions confirmed that the traits of AD were localized in the centro-parietal and centro-temporal areas in the theta frequency band (4-8 Hz). The contribution of this study is that it applies a neural metric for Alzheimers detection from a data science perspective rather than from a neuroscience one. The study shows that the combination of bipolar derivations with phase synchronization yields similar results to comparable studies employing alternative analysis methods.
Big Data Analytics for Long-Term Meteorological Observations at Hanford Site
A growing number of physical objects with embedded sensors with typically high volume and frequently updated data sets has accentuated the need to develop methodologies to extract useful information from big data for supporting decision making. This study applies a suite of data analytics and core principles of data science to characterize near real-time meteorological data with a focus on extreme weather events. To highlight the applicability of this work and make it more accessible from a risk management perspective, a foundation for a software platform with an intuitive Graphical User Interface (GUI) was developed to access and analyze data from a decommissioned nuclear production complex operated by the U.S. Department of Energy (DOE, Richland, USA). Exploratory data analysis (EDA), involving classical non-parametric statistics, and machine learning (ML) techniques, were used to develop statistical summaries and learn characteristic features of key weather patterns and signatures. The new approach and GUI provide key insights into using big data and ML to assist site operation related to safety management strategies for extreme weather events. Specifically, this work offers a practical guide to analyzing long-term meteorological data and highlights the integration of ML and classical statistics to applied risk and decision science.
Export Citation Format
Share document.

Thank you for visiting nature.com. You are using a browser version with limited support for CSS. To obtain the best experience, we recommend you use a more up to date browser (or turn off compatibility mode in Internet Explorer). In the meantime, to ensure continued support, we are displaying the site without styles and JavaScript.
- View all journals
- Explore content
- About the journal
- Publish with us
- Sign up for alerts
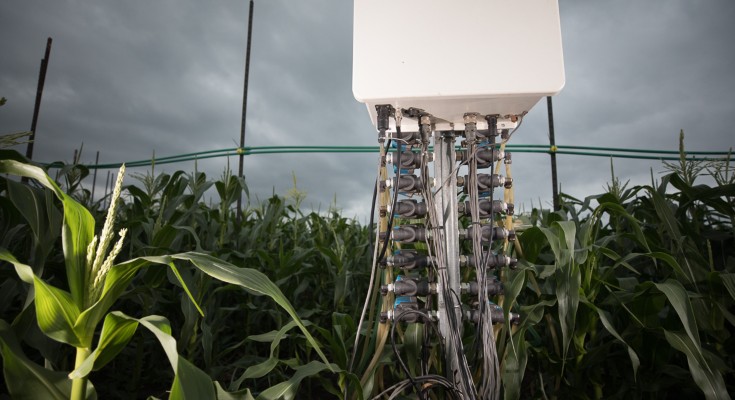
Two decades of fumigation data from the Soybean Free Air Concentration Enrichment facility
- Elise Kole Aspray
- Timothy A. Mies
- Elizabeth A. Ainsworth
Announcements
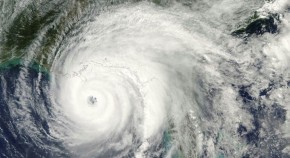
Collection open for submissions
Scientific Data is open to submissions for this special collection: Meteorology and hydroclimate observations and models
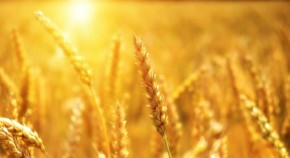
Scientific Data is open to submissions for this special collection: Genomics data for plant ecology, conservation and agriculture
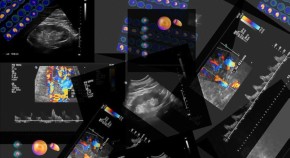
Scientific Data is open to submissions for this special collection: Medical imaging data for digital diagnostics
Find out more about Scientific Data
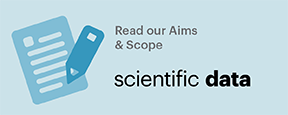
Find the right repository for your data
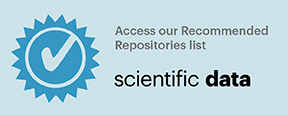
Advertisement
Latest Research articles
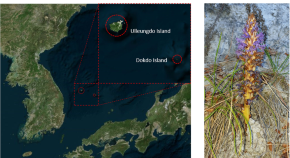
Chromosome-level genome assembly of Korean holoparasitic plants , Orobanche coerulescens
- Bongsang Kim
- So Yun Jhang
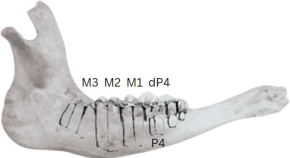
An international, open-access dataset of dental wear patterns and associated broad age classes in archaeological cattle mandibles
- Umberto Albarella
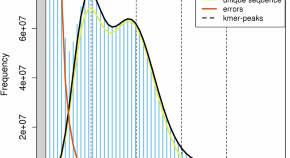
Chromosome-level genome assembly of Helwingia omeiensis : the first genome in the family Helwingiaceae
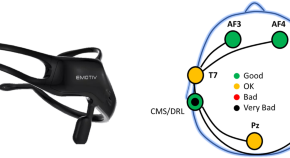
SignEEG v1.0: Multimodal Dataset with Electroencephalography and Hand-written Signature for Biometric Systems
- Ashish Ranjan Mishra
- Rakesh Kumar
- Rajkumar Saini
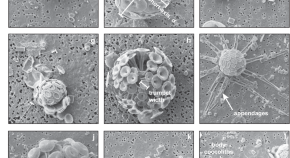
Cellular morphological trait dataset for extant coccolithophores from the Atlantic Ocean
- Rosie M. Sheward
- Alex J. Poulton
- Jens O. Herrle
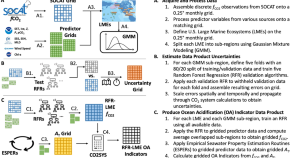
A mapped dataset of surface ocean acidification indicators in large marine ecosystems of the United States
- Jonathan D. Sharp
- Li-Qing Jiang
- Scott L. Cross
News & Comment
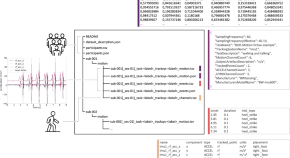
Motion-BIDS: an extension to the brain imaging data structure to organize motion data for reproducible research
We present an extension to the Brain Imaging Data Structure (BIDS) for motion data. Motion data is frequently recorded alongside human brain imaging and electrophysiological data. The goal of Motion-BIDS is to make motion data interoperable across different laboratories and with other data modalities in human brain and behavioral research. To this end, Motion-BIDS standardizes the data format and metadata structure. It describes how to document experimental details, considering the diversity of hardware and software systems for motion data. This promotes findable, accessible, interoperable, and reusable data sharing and Open Science in human motion research.
- Helena Cockx
- Julius Welzel
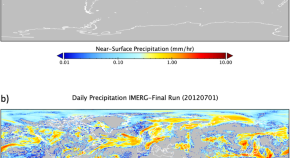
Strategizing Earth Science Data Development
Developing Earth science data products that meet the needs of diverse users is a challenging task for both data producers and service providers, as user requirements can vary significantly and evolve over time. In this comment, we discuss several strategies to improve Earth science data products that everyone can use.
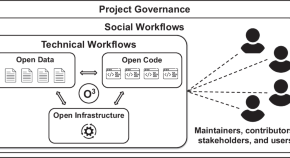
The O3 guidelines: open data, open code, and open infrastructure for sustainable curated scientific resources
Curated resources that support scientific research often go out of date or become inaccessible. This can happen for several reasons including lack of continuing funding, the departure of key personnel, or changes in institutional priorities. We introduce the Open Data, Open Code, Open Infrastructure (O3) Guidelines as an actionable road map to creating and maintaining resources that are less susceptible to such external factors and can continue to be used and maintained by the community that they serve.
- Charles Tapley Hoyt
- Benjamin M. Gyori
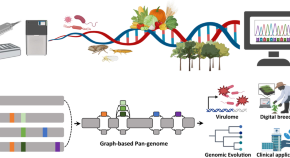
Beyond NGS data sharing for plant ecological resilience and improvement of agronomic traits
- Jayabalan Shilpha
- Seon-In Yeom
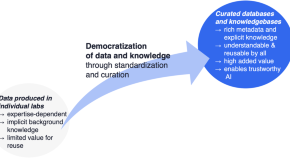
AI and the democratization of knowledge
The solution of the longstanding “protein folding problem” in 2021 showcased the transformative capabilities of AI in advancing the biomedical sciences. AI was characterized as successfully learning from protein structure data , which then spurred a more general call for AI-ready datasets to drive forward medical research. Here, we argue that it is the broad availability of knowledge , not just data, that is required to fuel further advances in AI in the scientific domain. This represents a quantum leap in a trend toward knowledge democratization that had already been developing in the biomedical sciences: knowledge is no longer primarily applied by specialists in a sub-field of biomedicine, but rather multidisciplinary teams, diverse biomedical research programs, and now machine learning. The development and application of explicit knowledge representations underpinning democratization is becoming a core scientific activity, and more investment in this activity is required if we are to achieve the promise of AI.
- Christophe Dessimoz
- Paul D. Thomas
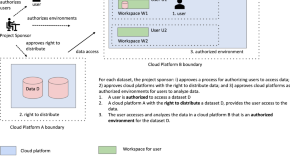
A Framework for the Interoperability of Cloud Platforms: Towards FAIR Data in SAFE Environments
As the number of cloud platforms supporting scientific research grows, there is an increasing need to support interoperability between two or more cloud platforms. A well accepted core concept is to make data in cloud platforms Findable, Accessible, Interoperable and Reusable (FAIR). We introduce a companion concept that applies to cloud-based computing environments that we call a S ecure and A uthorized F AIR E nvironment (SAFE). SAFE environments require data and platform governance structures and are designed to support the interoperability of sensitive or controlled access data, such as biomedical data. A SAFE environment is a cloud platform that has been approved through a defined data and platform governance process as authorized to hold data from another cloud platform and exposes appropriate APIs for the two platforms to interoperate.
- Robert L. Grossman
- Rebecca R. Boyles
Trending - Altmetric
A global multiproxy database for temperature reconstructions of the Common Era
Transcriptome sequencing of seven deep marine invertebrates
CODC-v1: a quality-controlled and bias-corrected ocean temperature profile database from 1940–2023
Electric vehicle charging stations in the workplace with high-resolution data from casual and habitual users
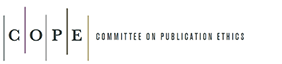
This journal is a member of and subscribes to the principles of the Committee on Publication Ethics.
Quick links
- Explore articles by subject
- Guide to authors
- Editorial policies

Journal of Big Data
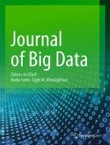
Featured Collections on Computationally Intensive Problems in General Math and Engineering
This two-part special issue covers computationally intensive problems in engineering and focuses on mathematical mechanisms of interest for emerging problems such as Partial Difference Equations, Tensor Calculus, Mathematical Logic, and Algorithmic Enhancements based on Artificial Intelligence. Applications of the research highlighted in the collection include, but are not limited to: Earthquake Engineering, Spatial Data Analysis, Geo Computation, Geophysics, Genomics and Simulations for Nature Based Construction, and Aerospace Engineering. Featured lead articles are co-authored by three esteemed Nobel laureates: Jean-Marie Lehn, Konstantin Novoselov, and Dan Shechtman.
Open Special Issues
Advancements on Automated Data Platform Management, Orchestration, and Optimization Submission Deadline: 30 September 2024
Emergent architectures and technologies for big data management and analysis Submission Deadline: 1 October 2024
View our collection of open and closed special issues
- Most accessed
New custom rating for improving recommendation system performance
Authors: Tora Fahrudin and Dedy Rahman Wijaya
Optimization-based convolutional neural model for the classification of white blood cells
Authors: Tulasi Gayatri Devi and Nagamma Patil
Advanced RIME architecture for global optimization and feature selection
Authors: Ruba Abu Khurma, Malik Braik, Abdullah Alzaqebah, Krishna Gopal Dhal, Robertas Damaševičius and Bilal Abu-Salih
Feature reduction for hepatocellular carcinoma prediction using machine learning algorithms
Authors: Ghada Mostafa, Hamdi Mahmoud, Tarek Abd El-Hafeez and Mohamed E. ElAraby
Data oversampling and imbalanced datasets: an investigation of performance for machine learning and feature engineering
Authors: Muhammad Mujahid, EROL Kına, Furqan Rustam, Monica Gracia Villar, Eduardo Silva Alvarado, Isabel De La Torre Diez and Imran Ashraf
Most recent articles RSS
View all articles
A survey on Image Data Augmentation for Deep Learning
Authors: Connor Shorten and Taghi M. Khoshgoftaar
Big data in healthcare: management, analysis and future prospects
Authors: Sabyasachi Dash, Sushil Kumar Shakyawar, Mohit Sharma and Sandeep Kaushik
Review of deep learning: concepts, CNN architectures, challenges, applications, future directions
Authors: Laith Alzubaidi, Jinglan Zhang, Amjad J. Humaidi, Ayad Al-Dujaili, Ye Duan, Omran Al-Shamma, J. Santamaría, Mohammed A. Fadhel, Muthana Al-Amidie and Laith Farhan
Deep learning applications and challenges in big data analytics
Authors: Maryam M Najafabadi, Flavio Villanustre, Taghi M Khoshgoftaar, Naeem Seliya, Randall Wald and Edin Muharemagic
Short-term stock market price trend prediction using a comprehensive deep learning system
Authors: Jingyi Shen and M. Omair Shafiq
Most accessed articles RSS
Aims and scope
Latest tweets.
Your browser needs to have JavaScript enabled to view this timeline
- Editorial Board
- Sign up for article alerts and news from this journal
- Follow us on Twitter
Annual Journal Metrics
2022 Citation Impact 8.1 - 2-year Impact Factor 5.095 - SNIP (Source Normalized Impact per Paper) 2.714 - SJR (SCImago Journal Rank)
2023 Speed 56 days submission to first editorial decision for all manuscripts (Median) 205 days submission to accept (Median)
2023 Usage 2,559,548 downloads 280 Altmetric mentions
- More about our metrics
- ISSN: 2196-1115 (electronic)
Loading metrics
Open Access
Eleven quick tips for finding research data
Contributed equally to this work with: Kathleen Gregory, Siri Jodha Khalsa, William K. Michener, Fotis E. Psomopoulos, Anita de Waard, Mingfang Wu
Affiliation Data Archiving and Networked Services, Royal Netherlands Academy of Arts and Sciences, The Hague, Netherlands
Affiliation National Snow and Ice Data Centre, Cooperative Institute for Research in Environmental Sciences, University of Colorado, Boulder, Colorado, United States of America

Affiliation College of University Libraries & Learning Sciences, The University of New Mexico, Albuquerque, New Mexico, United States of America
Affiliation Institute of Applied Biosciences, Centre for Research and Technology Hellas, Thessaloniki, Greece
Affiliation Research Data Management Solutions, Elsevier, Jericho, Vermont, United States of America
* E-mail: [email protected]
Affiliation Australia National Data Service, Melbourne, Australia
- Kathleen Gregory,
- Siri Jodha Khalsa,
- William K. Michener,
- Fotis E. Psomopoulos,
- Anita de Waard,
- Mingfang Wu
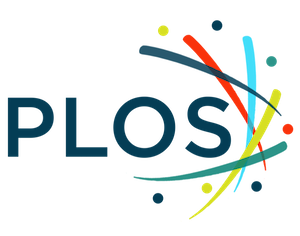
Published: April 12, 2018
- https://doi.org/10.1371/journal.pcbi.1006038
- Reader Comments
Citation: Gregory K, Khalsa SJ, Michener WK, Psomopoulos FE, de Waard A, Wu M (2018) Eleven quick tips for finding research data. PLoS Comput Biol 14(4): e1006038. https://doi.org/10.1371/journal.pcbi.1006038
Editor: Francis Ouellette, Genome Quebec, CANADA
Copyright: © 2018 Gregory et al. This is an open access article distributed under the terms of the Creative Commons Attribution License , which permits unrestricted use, distribution, and reproduction in any medium, provided the original author and source are credited.
Funding: William K. Michener was supported by NSF (#IIA-1301346 and #ACI-1430508). The funder had no role in study design, data collection and analysis, decision to publish, or preparation of the manuscript.
Competing interests: The authors have declared that no competing interests exist.
This is a PLOS Computational Biology Education paper.
Introduction
Over the past decades, science has experienced rapid growth in the volume of data available for research—from a relative paucity of data in many areas to what has been recently described as a data deluge [ 1 ]. Data volumes have increased exponentially across all fields of science and human endeavour, including data from sky, earth, and ocean observatories; social media such as Facebook and Twitter; wearable health-monitoring devices; gene sequences and protein structures; and climate simulations [ 2 ]. This brings opportunities to enable more research, especially cross-disciplinary research that could not be done before. However, it also introduces challenges in managing, describing, and making data findable, accessible, interoperable, and reusable by researchers [ 3 ].
When this vast amount and variety of data is made available, finding relevant data to meet a research need is increasingly a challenge. In the past, when data were relatively sparse, researchers discovered existing data by searching literature, attending conferences, and asking colleagues. In today’s data-rich environment, with accompanying advances in computational and networking technologies, researchers increasingly conduct web searches to find research data. The success of such searches varies greatly and depends to a large degree on the expertise of the person looking for data, the tools used, and, partially, on luck. This article offers the following 11 quick tips that researchers can follow to more effectively and precisely discover data that meet their specific needs.
- Tip 1: Think about the data you need and why you need them.
- Tip 2: Select the most appropriate resource.
- Tip 3: Construct your query strategically.
- Tip 4: Make the repository work for you.
- Tip 5: Refine your search.
- Tip 6: Assess data relevance and fitness -for -use.
- Tip 7: Save your search and data- source details.
- Tip 8: Look for data services, not just data.
- Tip 9: Monitor the latest data.
- Tip 10: Treat sensitive data responsibly.
- Tip 11: Give back (cite and share data).
Tip 1: Think about the data you need and why you need them
Before embarking on a search for data, consider how you will use the desired data in the context of your overall research question. Are you seeking data for comparison or validation, as the basis for a new study, or for another reason? List the characteristics that the data must have in order to fulfil your identified purpose(s), including requirements such as data format, spatial or temporal coverage, availability, and author or research group. In many cases, your initial data requirements and the identified constraints will change as you progress with the search. Pausing to first analyse what you need and why you need it can lead to a more analytic search, save searching time and facilitating the actions described in Tips 2–6.
Tip 2: Select the most appropriate resource
Directories of research-data repositories, such as re3data.org ( http://www.re3data.org ) and FAIRsharing ( https://fairsharing.org ), web search engines, and colleagues can be consulted to discover domain-specific portals in your discipline. Subject domain is but one criterion to consider when selecting an appropriate data repository. Various certification processes have also been implemented to help develop trustworthiness in repositories and to make their data-governing policies more transparent. For example, repositories earning the CoreTrustSeal ( https://www.coretrustseal.org/about ) Trustworthy Data Repository certification must meet 16 requirements measuring the accessibility, usability, reliability, and long-term stability of their data. Knowing what standards and criteria a repository applies to data and metadata provides more confidence in understanding and reusing the data from that repository.
Domain-specific portals provide ways to quickly narrow your search, offering interfaces and filters tailored to match the data and needs of specific disciplinary domains. Map interfaces for data collected from specific locations (see the National Water Information System, https://maps.waterdata.usgs.gov/mapper/index.html ) and specific search fields and tools (see the National Centre for Biotechnology Information’s complement of databases, ( https://www.ncbi.nlm.nih.gov/guide/all/ ) facilitate discovering disciplinary data. Other domain-focused repositories, such as the National Snow and Ice Data Centre (NSIDC, http://nsidc.org/data/search/ ), collect and apply knowledge about user requirements and incorporate domain semantics into their search engines to help data seekers quickly find appropriate data. Data aggregators, including DataONE ( https://www.dataone.org ) for environmental and earth observation data, VertNet ( http://vertnet.org ) and Global Biodiversity Information Facility (GBIF, https://www.gbif.org ) for museum specimen and biodiversity data, or DataMed ( https://datamed.org ) for biomedical datasets, enable searching multiple data repositories or collections through a single search interface. Some portals may not provide data-search functionality but instead provide a catalogue of data resources. A notable example is the AgBioData ( https://www.agbiodata.org/databases ) portal, which lists links to 12 agricultural biological databases dedicated to specific species (e.g., cotton, grain, or hardwood), where you can directly search for data.
The accessibility of data resources is another important consideration. University librarians can provide advice about particular subscription-based resources available at your institution. Research papers in your field can also point to available data repositories. In domains such as astronomy and genomics, for example, citations of datasets within journal articles are commonplace. These references usually include dataset access information that can be used to locate datasets of interest or to point toward data repositories favoured within a discipline.
Tip 3: Construct your query strategically
Describing your desired data effectively is key to communicating with the search system. Your description will determine if relevant data are retrieved and may inform the order of the hits in the results list. Help pages provide tips on how to construct basic and advanced searches within particular repositories (see for example Research Data Australia https://researchdata.ands.org.au —click on Advanced Search → Help). Note that not all repositories operate in the same manner. Some portals, such as DataONE ( https://www.dataone.org ), use semantic technologies to automatically expand the keywords entered in the search box to include synonyms. If a portal does not use automatic expansion, you may need to manually add various synonyms to your search query (e.g., in addition to ‘demography’ as a search term, one might also add ‘population density’, ‘population growth’, ‘census’, or ‘anthropology’).
- sea level (site:.edu)
Tip 4: Make the repository work for you
Repository developers invest significant time and energy organizing data in ways to make them more discoverable; use their work to your advantage. Familiarize yourself with the controlled vocabularies, subject categories, and search fields used in particular repositories. Searching for and successfully locating data is dependent on the information about the data, termed metadata, that are contained in these fields; this is particularly true for numeric or nontextual data. Browsing subject categories can also help to gauge the appropriateness of a resource, home in on an area of interest, or find related data that have been classified in the same category.
Researchers can also register or create profiles with many data repositories. By registering, you may be able to indicate your general research data interests which can be utilized in subsequent searches or receive alerts about datasets that you have previously downloaded (see also Tip 7).
Tip 5: Refine your search
In many cases, your initial search may not retrieve relevant data or all of the data that you need. Based on the retrieved results, you may need to broaden or narrow your approach. Apart from rephrasing your search query and using search operators, as discussed in Tip 3, facets or filters specific to individual repositories can be used to narrow the scope of your results. Refinements such as data format, types of analysis, and data availability allow users to quickly find usable data.
Examining results that look interesting (for example, by clicking on links for ‘more information’) can be a signal of the type of information that you find relevant. These results can then be linked to related ones (e.g., from the data provider, from different time series), and in subsequent searches, other results algorithmically determined to be related will be brought to the top of the results list.
Tip 6: Assess data relevance and fitness for use
Conduct a preliminary assessment of the retrieved data prior to investing time in subsequent data download, integration, and analytic and visualization efforts. A quick perusal of the metadata (text and/or images) can often enable you to verify that the data satisfy the initial requirements and constraints set forth in Tip 1 (e.g., spatial, temporal, and thematic coverage and data-sharing restrictions). Ideally, the metadata will also contain documentation sufficient to comprehensively assess the relevance and fitness for use of the data, including information about how the data were collected and quality assured, how the data have been previously used, etc. Some data repositories such as the National Science Foundation’s Arctic Data Centre ( https://arcticdata.io ) enable the data seeker to generate and download a metadata quality report that assesses how well the metadata adhere to community best practices for discovery and reusability. Clearly, if none of your criteria for data are met, you may not wish to download and use the associated data.
Attention should also be paid to quality parameters or flags within the data files. Make use of a visualization tool or statistics analysis tool, if provided, to examine quality or fitness of data for intended use before downloading data, especially if the data volume is large and the dataset includes many files.
Tip 7: Save your search and data-source details
Record the data source and data version if you access or download a data product. This may be accomplished by noting the persistent identifier, such as a digital object identifier (DOI) or another Global Unique Identifier (GUID) assigned to the data. Recording the URL from which you obtained the data can be a quick way of returning to it but should not be trusted in the long term for providing access to the data, as URLs can change. It is also a good practice to save a copy of any original data products that you downloaded [ 5 ]. You may, for example, need to go back to original data sources and check if there have been any changes or corrections to data. Registering with the data portal (as described in Tip 3) or registering as a user of a specific data product allows the repository to contact you when necessary. Such information may be needed when you publish a paper that builds on the data you accessed. If there are any errors found in the original data, registering with the data service allows them to contact you to see if there is an impact on any research conclusions that you have drawn from this data.
If you have registered with a portal, it may also be possible to save your searches, allowing you to resume your data search at a later time with all previously defined search criteria. Some portals use RESTful search interfaces, which means you can bookmark a results set or dataset and return to it later simply by going to the bookmark.
Tip 8: Look for data services, not just data
The data you seek may be available only via an application programming interface (API) or as linked data [ 6 ]. That is, instead of a file residing on a server, the data that best suits your purposes is provided as a service through an API. Examples of such services include the climate change projection data available through the NSW Climate Data Portal ( http://climatechange.environment.nsw.gov.au/Climate-projections-for-NSW/Download-datasets ), in which data are dynamically generated from a simulation model; Google Earth Engine ( https://earthengine.google.com ); or Amazon Web Services (AWS) public datasets ( https://aws.amazon.com/public-datasets/ ). Data made available from these services may not be searchable from general web search engines, but data services may be registered to data catalogues or federations such as Research Data Australia, DataONE, and other resources listed in re3data.org and FAIRsharing. Many repositories that host extremely large volumes of data such as sequencing, environmental observatory, and remotely sensed data provide access to tools, workflows, and computing resources that allow one to access, visualize, process, and download manageable subsets of the data. Often, the processing workflows that one might use to process and download a dataset can also be downloaded, saved, and used again in subsequent searches.
Tip 9: Monitor the latest data
One of the most effective ways to identify new data submissions is to monitor the latest literature, as many journals such as Nature , PLOS , Science , and others require that the data underlying a publication also be published in a public (e.g., Dataverse https://dataverse.org , Dryad http://datadryad.org , or Zenodo https://zenodo.org ) or discipline-based repository (e.g., EASY from Data Archiving and Networked Services [DANS] https://easy.dans.knaw.nl/ , GenBank https://www.ncbi.nlm.nih.gov/genbank/ , or PubChem https://pubchem.ncbi.nlm.nih.gov ).
In addition, many domain-based repositories, such as environmental observatories and sequencing databases, are constantly accepting similar types of data submissions. Publishers and some digital repositories also offer alerting services when new publications or data products are submitted. Depending on the resource, it may be possible to set up a recurring search API or a Rich Site Summary (RSS) feed to automatically monitor specific resources. For example, the NSIDC offers a subscription service where new data meeting a list of user-generated specifications are automatically pushed to a location specified by the user.
Tip 10: Treat sensitive data responsibly
In most cases, after you have located relevant data, you can download them straight away. However, there are cases, such as for medical and health data, endangered and threatened species, and sacred objects and archaeological finds, where you can only see a data description (the metadata) and are not able to download the data directly due to access restrictions imposed to protect the privacy of individuals represented in the data or to safeguard locations and species from harm or unwanted attention. Guidance with respect to sensitive data is available through the 2003 Fort Lauderdale Agreement ( https://www.genome.gov/pages/research/wellcomereport0303.pdf ), the 2009 Toronto Agreement ( https://www.nature.com/articles/461168a ) [ 7 ], the Australian National Data Service ( http://www.ands.org.au/working-with-data/sensitive-data ), and individual institutional and society research ethics committees.
Sensitive data are often discoverable and accessible if identity and location information are anonymized. In other cases, an established data-access agreement specifies the technical requirements as well as the ethical and scientific obligations that accessing and using the data entail. Technical requirements may include aspects such as auditing data access at the local system, defining read-only access rights, and/or ensuring constraints for nonprivileged network access. You can still contact the data owner to explain your intended use and to discuss the conditions and legal restrictions associated with using sensitive data. Such contact may even lead to collaborative research between you and the data owner. Should you be granted access to the data, it is important to use the data ethically and responsibly [ 8 ] to ensure that no harm is done to individuals, species, and culture heritages.
Tip 11: Give back (cite and share data)
There are three ways to give back to the community once you have sought, discovered, and used an existing data product. First, it is essential that you give proper attribution to the data creators (in some cases, the data owners) if you use others’ data for research, education, decision making, or other purposes [ 9 ]. Proper attribution benefits both data creators/providers and data seekers/users. Data creators/providers receive credit for their work, and their practice of sharing data is thus further encouraged. Data seekers/users make their own work more transparent and, potentially, reproducible by uniquely identifying and citing data used in their research.
Many data creators and institutions adopt standard licenses from organizations, such as Creative Commons, that govern how their data products may be shared and used. Creative Commons recommends that a proper attribution should include title, author, source, and license [ 10 ].
Second, provide feedback to the data creators or the data repository about any issues associated with data accessibility, data quality, or metadata completeness and interpretability. Data creators and repositories benefit from knowing that their data products are understandable and usable by others, as well as knowing how the data were used. Future users of the data will also benefit from your feedback.
Third, virtually all data seekers and data users also generate data. The ultimate ‘give-back’ is to also share your data with the broader community.
This paper highlights 11 quick tips that, if followed, should make it easier for a data seeker to discover data that meet a particular need. Regardless of whether you are acting as a data seeker or a data creator, remember that ‘data discovery and reuse are most easily accomplished when: (1) data are logically and clearly organized; (2) data quality is assured; (3) data are preserved and discoverable via an open data repository; (4) data are accompanied by comprehensive metadata; (5) algorithms and code used to create data products are readily available; (6) data products can be uniquely identified and associated with specific data originator(s); and (7) the data originator(s) or data repository have provided recommendations for citation of the data product(s)’ [ 11 ].
Acknowledgments
This work was developed as part of the Research Data Alliance (RDA) ‘WG/IG’ entitled ‘Data Discovery Paradigms’, and we acknowledge the support provided by the RDA community and structures. We would like to thank members of the group for their support, especially Andrea Perego, Mustapha Mokrane, Susanna-Assunta Sansone, Peter McQuilton, and Michel Dumontier who read this paper and provided constructive suggestions.
- 1. Gray J. Jim Gray on eScience: A transformed scientific method. In: Hey T, Tansley S, Tolle K, editors. The Fourth Paradigm: Data-Intensive Scientific Discovery. Richmond, WA: Microsoft Research; 2009. p.xvii–xxxi. Available from: https://www.microsoft.com/en-us/research/publication/fourth-paradigm-data-intensive-scientific-discovery/ .
- 2. Fox G, Hey T, Trefethen A. Where does all the data come from? In: Kleese van Dam K, editor. Data-Intensive Science. Chapman and Hall/CRC; Boca Raton: Taylor and Francis, May 2013. p. 15–51.
- View Article
- PubMed/NCBI
- Google Scholar
- 4. Warner, R. Google Advanced Search: A Comprehensive List of Google Search Operators [Internet]. 2015. Available from: https://bynd.com/news-ideas/google-advanced-search-comprehensive-list-google-search-operators/ . [cited 2017 Oct 26]
- 6. Heath T, Bizer C. Linked Data: Evolving the Web into a global data space. In: Hendler J, van Harmelen F, editors. Synthesis Lectures on the Semantic Web: Theory and Technology. Morgan & Claypool; 2011. p. 1–136.
- 8. Clark K, et al. Guidelines for the Ethical Use of Digital Data in Human Research. www.carltonconnect.com.au: The University of Melbourne; 2015. Available from: https://www.carltonconnect.com.au/wp-content/uploads/2015/06/Ethical-Use-of-Digital-Data.pdf . [cited 2018 Feb. 1].
- 9. Martone M, editor. Data Citation Synthesis Group: Joint Declaration of Data Citation Principles. FORCE11. San Diego, CA; 2014. [cited 2018 Feb 1]. Available from: https://www.force11.org/group/joint-declaration-data-citation-principles-final .
- 10. Creative Commons. Best practices for attribution [Internet]. 2014 [cited 2017 Sep 10]. Available from: https://wiki.creativecommons.org/wiki/Best_practices_for_attribution .
- 11. Michener W. K. Data discovery. In: Recknagel F, Michener WK, editors. Ecological informatics: Data management and knowledge discovery. Springer International Publishing, Cham, Switzerland; 2017.
- Interlibrary Loan and Scan & Deliver
- Course Reserves
- Purchase Request
- Collection Development & Maintenance
- Current Negotiations
- Ask a Librarian
- Instructor Support
- Library How-To
- Research Guides
- Research Support
- Study Rooms
- Research Rooms
- Partner Spaces
- Loanable Equipment
- Print, Scan, Copy
- 3D Printers
- Poster Printing
- OSULP Leadership
- Strategic Plan
Research Data Services
- Campus Services & Policies
- Archiving & Preservation
- Citing Datasets
- Data Papers & Journals
Data Papers & Data Journals
- Data Repositories
- ScholarsArchive@OSU data repository
- Data Storage & Backup
- Data Types & File Formats
- Defining Data
- File Organization
- IP & Licensing Data
- Laboratory Notebooks
- Research Lifecycle
- Researcher Identifiers
- Sharing Your Data
- Metadata/Documentation
- Tools & Resources
SEND US AN EMAIL
- L.K. Borland Data Management Support Coordinator Schedule an appointment with me!
- Diana Castillo College of Business/Social Sciences Data Librarian Assistant Professor 541-737-9494
- Clara Llebot Lorente Data Management Specialist Assistant Professor 541-737-1192 On sabbatical through June 2024
The rise of the "data paper"
Datasets are increasingly being recognized as scholarly products in their own right, and as such, are now being submitted for standalone publication. In many cases, the greatest value of a dataset lies in sharing it, not necessarily in providing interpretation or analysis. For example, this paper presents a global database of the abundance, biomass, and nitrogen fixation rates of marine diazotrophs. This benchmark dataset, which will continue to evolve over time, is a valuable standalone research product that has intrinsic value. Under traditional publication models, this dataset would not be considered "publishable" because it doesn't present novel research or interpretation of results. Data papers facilitate the sharing of data in a standardized framework that provides value, impact, and recognition for authors. Data papers also provide much more thorough context and description than datasets that are simply deposited to a repository (which may have very minimal metadata requirements).
What is a data paper?
Data papers thoroughly describe datasets, and do not usually include any interpretation or discussion (an exception may be discussion of different methods to collect the data, e.g.). Some data papers are published in a distinct “Data Papers” section of a well-established journal (see this article in Ecology, for example). It is becoming more common, however, to see journals that exclusively focus on the publication of datasets. The purpose of a data journal is to provide quick access to high-quality datasets that are of broad interest to the scientific community. They are intended to facilitate reuse of the dataset, which increases its original value and impact, and speeds the pace of research by avoiding unintentional duplication of effort.
Are data papers peer-reviewed?
Data papers typically go through a peer review process in the same manner as articles, but being new to scientific practice, the quality and scope of the process is variable across publishers. A good example of a peer reviewed data journal is Earth System Science Data ( ESSD ). Their review guidelines are well described and aren't all that different from manuscript review guidelines that we are all already familiar with.
You might wonder, W hat is the difference between a 'data paper' and a 'regular article + dataset published in a public repository' ? The answer to that isn’t always clear. Some data papers necessitate just as much preparation as, and are of equal quality to, ‘typical’ journal articles. Some data papers are brief, and only present enough metadata and descriptive content to make the dataset understandable and reusable. In most cases however, the datasets or databases presented in data papers include much more description than datasets deposited to a repository, even if those datasets were deposited to support a manuscript. Common practices and standards are evolving in the realm of data papers and data journals, but for now, they are the Wild West of data sharing.
Where do the data from data papers live?
Data preservation is a corollary of data papers, not their main purpose. Most data journals do not archive data in-house. Instead, they generally require that authors submit the dataset to a repository. These repositories archive the data, provide persistent access, and assign the dataset a unique identifier (DOI). Repositories do not always require that the dataset(s) be linked with a publication (data paper or ‘typical’ paper; Dryad does require one), but if you’re going to the trouble of submitting a dataset to a repository, consider exploring the option of publishing a data paper to support it.
How can I find data journals?
The article by Walters (2020) has a list of data journals in their appendix, and differentiates between "pure" data journals and journals that publish data reports but are devoted mainly to other types of contributions. They also update previous lists of data journals ( Candela et al, 2015 ).
Walters, William H.. 2020. “Data Journals: Incentivizing Data Access and Documentation Within the Scholarly Communication System”. Insights 33 (1): 18. DOI: http://doi.org/10.1629/uksg.510
Candela, L., Castelli, D., Manghi, P., & Tani, A. (2015). Data journals: A survey. Journal of the Association for Information Science and Technology , 66 (9), 1747–1762. https://doi.org/10.1002/asi.23358
This blog post by Katherine Akers , from 2014, also has a long list of existing data journals.
- << Previous: Citing Datasets
- Next: Data Repositories >>
- Last Updated: Aug 30, 2023 9:25 AM
- URL: https://guides.library.oregonstate.edu/research-data-services
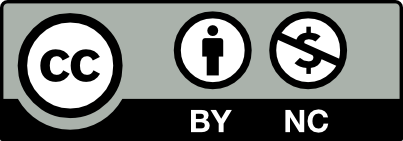
Contact Info
121 The Valley Library Corvallis OR 97331–4501
Phone: 541-737-3331
Services for Persons with Disabilities
In the Valley Library
- Oregon State University Press
- Special Collections and Archives Research Center
- Undergrad Research & Writing Studio
- Graduate Student Commons
- Tutoring Services
- Northwest Art Collection
Digital Projects
- Oregon Explorer
- Oregon Digital
- ScholarsArchive@OSU
- Digital Publishing Initiatives
- Atlas of the Pacific Northwest
- Marilyn Potts Guin Library  
- Cascades Campus Library
- McDowell Library of Vet Medicine

- Sources of Data For Research: Types & Examples

Introduction
In the age of information, data has become the driving force behind decision-making and innovation. Whether in business, science, healthcare, or government, data serves as the foundation for insights and progress.
As a researcher, you need to understand the various sources of data as they are essential for conducting comprehensive and impactful studies. In this blog post, we will explore the primary data sources, their definitions, and examples to help you gather and analyze data effectively.
Primary Data Sources
Primary data sources refer to original data collected firsthand by researchers specifically for their research purposes. These sources provide fresh and relevant information tailored to the study’s objectives. Examples of primary data sources include surveys and questionnaires, direct observations, experiments, interviews, and focus groups. As a researcher, you must be familiar with primary data sources, which are original data collected firsthand specifically for your research purposes.
These sources hold significant value as they offer fresh and relevant information tailored to your study. Also, researchers use primary data to obtain accurate and specific insights into their research questions to confirm that the data is directly relevant to their study and meets their specific needs. Collecting primary data allows you as a researcher to control the data collection process, and monitor the data quality and reliability for their analyses and conclusions.
Examples of Primary Data Sources
- Surveys and questionnaires: Surveys and questionnaires are widely used data collection methods that allow you to gather information directly from respondents. Whether distributed online, through mail, or in person, surveys enable you to reach a large audience and collect quantitative data efficiently. However, it is crucial to design clear and unbiased questions to ensure the accuracy and reliability of responses.
- Observations: Direct observations involve systematically watching and recording events or behaviors as they occur. This method provides you with real-time data, offering unique insights into participants’ natural behavior and responses. It is particularly valuable in fields such as psychology, anthropology, and ecology, where understanding human or animal behavior is critical.
- Experiments: Experiments involve when you deliberately manipulate variables to study cause-and-effect relationships. When you control variables, your experiments provide rigorous and conclusive data, often used in scientific research. They are well-suited for hypothesis testing and determining causal relationships.
- Interviews and focus groups : Qualitative data collected through interviews and focus groups give you an in-depth exploration of participants’ opinions, beliefs, and experiences. These methods help you to understand complex issues and gain rich insights that quantitative data alone may not capture or provide for your study.
Read More: What is Primary Data? + [Examples & Collection Methods]
Secondary Data Sources
As a researcher, you should also be familiar with secondary data sources. Secondary data sources involve data collected by someone else for purposes other than your specific research. Therefore, secondary data complements primary data and can provide valuable context and insights to your research.
Examples of Secondary Data Sources
- Published literature: Published literature refers to academic papers, books, and reports published by researchers and scholars in various fields. These literatures serve as a rich source of secondary data. These sources contain valuable findings and analyses from previous studies, offering a foundation for new research and the ability to build upon existing knowledge. Reviewing published literature is essential for you to understand the current state of research in your area of study and identify gaps for further investigation.
- Government sources: Government agencies collect and maintain vast amounts of data on a wide range of topics. These datasets are often made available for public use and can be a valuable resource for researchers. For example, census data provides demographic information, economic indicators offer insights into the economy, and health records contribute to public health research. Government sources offer standardized and reliable data that can be used for various research purposes.
- Online databases: The internet has opened up access to a wealth of data through online databases, data repositories, and open data initiatives. These platforms host datasets on diverse subjects. This makes them easily accessible to you and other researchers worldwide. Online databases are particularly beneficial for conducting cross-disciplinary research or exploring topics beyond your immediate field of expertise.
- Market research reports: Market research companies conduct surveys and gather data to analyze market trends, consumer behavior, and industry insights. These reports provide valuable data for businesses and researchers seeking information on market dynamics and consumer preferences. Market research reports offer you a comprehensive view of industries and can inform you of how to make strategic decisions.
Read More: What is Secondary Data? + [Examples, Sources & Analysis]
Tertiary Data Sources
In addition to primary and secondary data, you should be aware of tertiary data sources, which play a critical role in aggregating and organizing existing data from various origins. Tertiary data sources focus on collecting, curating, and preserving data for easy access and analysis.
Examples of Tertiary Data Sources
- Data aggregators: Data aggregators are companies or organizations that specialize in collecting and compiling data from multiple sources into centralized databases. These sources can include government agencies, research institutions, businesses, and other data providers. These aggregators offer a convenient way for you, a researcher, to access a vast amount of data on specific topics or industries. As they consolidate data from diverse sources, they provide you and other researchers with a comprehensive view of trends, patterns, and insights.
- Data brokers: The best way to describe data brokers is that they are entities that buy and sell data, often without the direct consent or knowledge of the individuals whose data is being traded. While data brokers can offer access to large datasets, their practices raise privacy and ethical concerns. As a researcher, you should be cautious when using data obtained through data brokers to ensure compliance with ethical guidelines and data protection laws.
- Data archives: Data archives serve as repositories for historical data and research findings. These archives are essential for preserving valuable information for future reference and analysis. They often contain datasets, reports, academic papers, and other research materials. Data archives ensure that data remains accessible for replication studies, verification of previous research, and the development of longitudinal analyses.
Emerging Data Sources
As you delve into the world of data collection, it’s important to know the emerging sources that have gained prominence in recent years. These newer data sources provide valuable insights and opportunities for research across various domains. Below are some of these emerging data sources:
- Internet of Things (IoT): The Internet of Things (IoT) has changed data collection in the 21st century through the everyday connection of devices and objects to the Internet. Smart devices like sensors, wearables, and home appliances generate vast amounts of data in real-time. For example, IoT devices in healthcare can monitor patients’ health metrics, while in agriculture, they can optimize irrigation and crop management. As a researcher, you can leverage IoT data to analyze patterns, predict trends, and make data-driven decisions.
- Social media and web data: Social media platforms and websites host a wealth of information generated by users worldwide. When you analyze social media posts and online reviews, and scrap the web, they provide you with valuable insights into public opinions, consumer behavior, and trends. You can study sentiment analysis, track customer preferences, and identify emerging topics using social media data. Web scraping allows for the extraction of data from websites, enabling researchers to gather large datasets for analysis.
- Sensor data: Sensor data is becoming increasingly relevant in various fields, including environmental monitoring, urban planning, and healthcare. Sensors are capable of measuring and collecting data on environmental parameters, traffic patterns, air quality, and more. This data helps you understand environmental changes, optimize urban infrastructure, and improve public health initiatives. Sensor networks offer a continuous stream of data, that provides you with real-time and accurate information.
In conclusion, we have explored the diverse sources of data for research, such as primary data sources, secondary data sources, and tertiary data sources, which all play a crucial role in getting the accurate information needed for research. It is important that you understand the strengths and limitations of each data source.
As you embark on your research journey, explore and utilize these diverse data sources. And if you leverage a combination of primary, secondary, and tertiary data, you can make informed decisions, drive progress in your respective fields, and uncover novel insights that may not be achievable without trying out different sources.
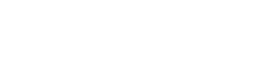
Connect to Formplus, Get Started Now - It's Free!
- data sources
- primary data sources
- research studies
- secondary data source
- tertiary data source
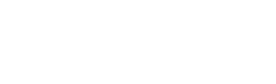
You may also like:
Projective Techniques In Surveys: Definition, Types & Pros & Cons
Introduction When you’re conducting a survey, you need to find out what people think about things. But how do you get an accurate and...

Naive vs Non Naive Participants In Research: Meaning & Implications
Introduction In research studies, naive and non-naive participant information alludes to the degree of commonality and understanding...
Subgroup Analysis: What It Is + How to Conduct It
Introduction Clinical trials are an integral part of the drug development process. They aim to assess the safety and efficacy of a new...
Desk Research: Definition, Types, Application, Pros & Cons
If you are looking for a way to conduct a research study while optimizing your resources, desk research is a great option. Desk research...
Formplus - For Seamless Data Collection
Collect data the right way with a versatile data collection tool. try formplus and transform your work productivity today..
Have a language expert improve your writing
Run a free plagiarism check in 10 minutes, generate accurate citations for free.
- Knowledge Base
Methodology
Research Methods | Definitions, Types, Examples
Research methods are specific procedures for collecting and analyzing data. Developing your research methods is an integral part of your research design . When planning your methods, there are two key decisions you will make.
First, decide how you will collect data . Your methods depend on what type of data you need to answer your research question :
- Qualitative vs. quantitative : Will your data take the form of words or numbers?
- Primary vs. secondary : Will you collect original data yourself, or will you use data that has already been collected by someone else?
- Descriptive vs. experimental : Will you take measurements of something as it is, or will you perform an experiment?
Second, decide how you will analyze the data .
- For quantitative data, you can use statistical analysis methods to test relationships between variables.
- For qualitative data, you can use methods such as thematic analysis to interpret patterns and meanings in the data.
Table of contents
Methods for collecting data, examples of data collection methods, methods for analyzing data, examples of data analysis methods, other interesting articles, frequently asked questions about research methods.
Data is the information that you collect for the purposes of answering your research question . The type of data you need depends on the aims of your research.
Qualitative vs. quantitative data
Your choice of qualitative or quantitative data collection depends on the type of knowledge you want to develop.
For questions about ideas, experiences and meanings, or to study something that can’t be described numerically, collect qualitative data .
If you want to develop a more mechanistic understanding of a topic, or your research involves hypothesis testing , collect quantitative data .
Qualitative | to broader populations. . | |
---|---|---|
Quantitative | . |
You can also take a mixed methods approach , where you use both qualitative and quantitative research methods.
Primary vs. secondary research
Primary research is any original data that you collect yourself for the purposes of answering your research question (e.g. through surveys , observations and experiments ). Secondary research is data that has already been collected by other researchers (e.g. in a government census or previous scientific studies).
If you are exploring a novel research question, you’ll probably need to collect primary data . But if you want to synthesize existing knowledge, analyze historical trends, or identify patterns on a large scale, secondary data might be a better choice.
Primary | . | methods. |
---|---|---|
Secondary |
Descriptive vs. experimental data
In descriptive research , you collect data about your study subject without intervening. The validity of your research will depend on your sampling method .
In experimental research , you systematically intervene in a process and measure the outcome. The validity of your research will depend on your experimental design .
To conduct an experiment, you need to be able to vary your independent variable , precisely measure your dependent variable, and control for confounding variables . If it’s practically and ethically possible, this method is the best choice for answering questions about cause and effect.
Descriptive | . . | |
---|---|---|
Experimental |
Receive feedback on language, structure, and formatting
Professional editors proofread and edit your paper by focusing on:
- Academic style
- Vague sentences
- Style consistency
See an example
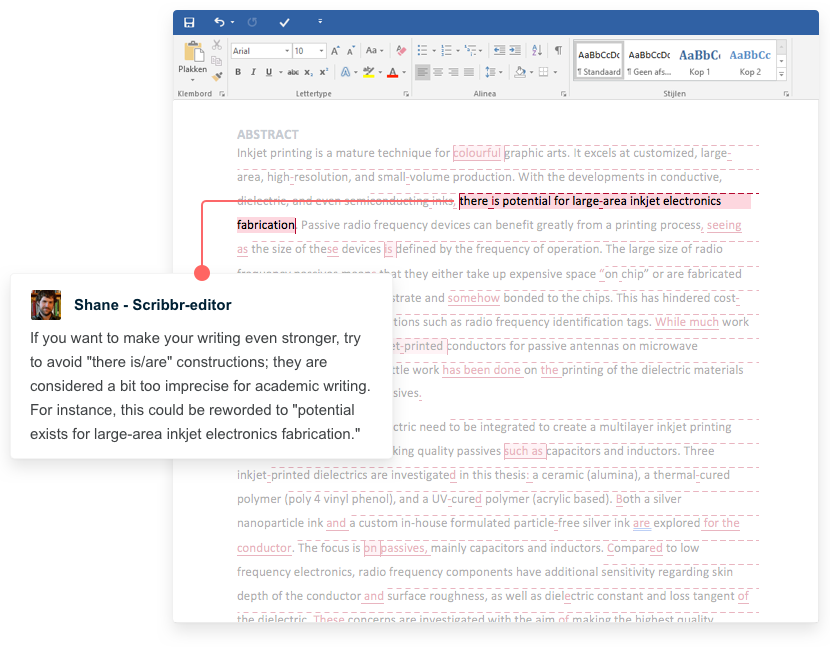
Research method | Primary or secondary? | Qualitative or quantitative? | When to use |
---|---|---|---|
Primary | Quantitative | To test cause-and-effect relationships. | |
Primary | Quantitative | To understand general characteristics of a population. | |
Interview/focus group | Primary | Qualitative | To gain more in-depth understanding of a topic. |
Observation | Primary | Either | To understand how something occurs in its natural setting. |
Secondary | Either | To situate your research in an existing body of work, or to evaluate trends within a research topic. | |
Either | Either | To gain an in-depth understanding of a specific group or context, or when you don’t have the resources for a large study. |
Your data analysis methods will depend on the type of data you collect and how you prepare it for analysis.
Data can often be analyzed both quantitatively and qualitatively. For example, survey responses could be analyzed qualitatively by studying the meanings of responses or quantitatively by studying the frequencies of responses.
Qualitative analysis methods
Qualitative analysis is used to understand words, ideas, and experiences. You can use it to interpret data that was collected:
- From open-ended surveys and interviews , literature reviews , case studies , ethnographies , and other sources that use text rather than numbers.
- Using non-probability sampling methods .
Qualitative analysis tends to be quite flexible and relies on the researcher’s judgement, so you have to reflect carefully on your choices and assumptions and be careful to avoid research bias .
Quantitative analysis methods
Quantitative analysis uses numbers and statistics to understand frequencies, averages and correlations (in descriptive studies) or cause-and-effect relationships (in experiments).
You can use quantitative analysis to interpret data that was collected either:
- During an experiment .
- Using probability sampling methods .
Because the data is collected and analyzed in a statistically valid way, the results of quantitative analysis can be easily standardized and shared among researchers.
Research method | Qualitative or quantitative? | When to use |
---|---|---|
Quantitative | To analyze data collected in a statistically valid manner (e.g. from experiments, surveys, and observations). | |
Meta-analysis | Quantitative | To statistically analyze the results of a large collection of studies. Can only be applied to studies that collected data in a statistically valid manner. |
Qualitative | To analyze data collected from interviews, , or textual sources. To understand general themes in the data and how they are communicated. | |
Either | To analyze large volumes of textual or visual data collected from surveys, literature reviews, or other sources. Can be quantitative (i.e. frequencies of words) or qualitative (i.e. meanings of words). |
If you want to know more about statistics , methodology , or research bias , make sure to check out some of our other articles with explanations and examples.
- Chi square test of independence
- Statistical power
- Descriptive statistics
- Degrees of freedom
- Pearson correlation
- Null hypothesis
- Double-blind study
- Case-control study
- Research ethics
- Data collection
- Hypothesis testing
- Structured interviews
Research bias
- Hawthorne effect
- Unconscious bias
- Recall bias
- Halo effect
- Self-serving bias
- Information bias
Quantitative research deals with numbers and statistics, while qualitative research deals with words and meanings.
Quantitative methods allow you to systematically measure variables and test hypotheses . Qualitative methods allow you to explore concepts and experiences in more detail.
In mixed methods research , you use both qualitative and quantitative data collection and analysis methods to answer your research question .
A sample is a subset of individuals from a larger population . Sampling means selecting the group that you will actually collect data from in your research. For example, if you are researching the opinions of students in your university, you could survey a sample of 100 students.
In statistics, sampling allows you to test a hypothesis about the characteristics of a population.
The research methods you use depend on the type of data you need to answer your research question .
- If you want to measure something or test a hypothesis , use quantitative methods . If you want to explore ideas, thoughts and meanings, use qualitative methods .
- If you want to analyze a large amount of readily-available data, use secondary data. If you want data specific to your purposes with control over how it is generated, collect primary data.
- If you want to establish cause-and-effect relationships between variables , use experimental methods. If you want to understand the characteristics of a research subject, use descriptive methods.
Methodology refers to the overarching strategy and rationale of your research project . It involves studying the methods used in your field and the theories or principles behind them, in order to develop an approach that matches your objectives.
Methods are the specific tools and procedures you use to collect and analyze data (for example, experiments, surveys , and statistical tests ).
In shorter scientific papers, where the aim is to report the findings of a specific study, you might simply describe what you did in a methods section .
In a longer or more complex research project, such as a thesis or dissertation , you will probably include a methodology section , where you explain your approach to answering the research questions and cite relevant sources to support your choice of methods.
Is this article helpful?
Other students also liked, writing strong research questions | criteria & examples.
- What Is a Research Design | Types, Guide & Examples
- Data Collection | Definition, Methods & Examples
More interesting articles
- Between-Subjects Design | Examples, Pros, & Cons
- Cluster Sampling | A Simple Step-by-Step Guide with Examples
- Confounding Variables | Definition, Examples & Controls
- Construct Validity | Definition, Types, & Examples
- Content Analysis | Guide, Methods & Examples
- Control Groups and Treatment Groups | Uses & Examples
- Control Variables | What Are They & Why Do They Matter?
- Correlation vs. Causation | Difference, Designs & Examples
- Correlational Research | When & How to Use
- Critical Discourse Analysis | Definition, Guide & Examples
- Cross-Sectional Study | Definition, Uses & Examples
- Descriptive Research | Definition, Types, Methods & Examples
- Ethical Considerations in Research | Types & Examples
- Explanatory and Response Variables | Definitions & Examples
- Explanatory Research | Definition, Guide, & Examples
- Exploratory Research | Definition, Guide, & Examples
- External Validity | Definition, Types, Threats & Examples
- Extraneous Variables | Examples, Types & Controls
- Guide to Experimental Design | Overview, Steps, & Examples
- How Do You Incorporate an Interview into a Dissertation? | Tips
- How to Do Thematic Analysis | Step-by-Step Guide & Examples
- How to Write a Literature Review | Guide, Examples, & Templates
- How to Write a Strong Hypothesis | Steps & Examples
- Inclusion and Exclusion Criteria | Examples & Definition
- Independent vs. Dependent Variables | Definition & Examples
- Inductive Reasoning | Types, Examples, Explanation
- Inductive vs. Deductive Research Approach | Steps & Examples
- Internal Validity in Research | Definition, Threats, & Examples
- Internal vs. External Validity | Understanding Differences & Threats
- Longitudinal Study | Definition, Approaches & Examples
- Mediator vs. Moderator Variables | Differences & Examples
- Mixed Methods Research | Definition, Guide & Examples
- Multistage Sampling | Introductory Guide & Examples
- Naturalistic Observation | Definition, Guide & Examples
- Operationalization | A Guide with Examples, Pros & Cons
- Population vs. Sample | Definitions, Differences & Examples
- Primary Research | Definition, Types, & Examples
- Qualitative vs. Quantitative Research | Differences, Examples & Methods
- Quasi-Experimental Design | Definition, Types & Examples
- Questionnaire Design | Methods, Question Types & Examples
- Random Assignment in Experiments | Introduction & Examples
- Random vs. Systematic Error | Definition & Examples
- Reliability vs. Validity in Research | Difference, Types and Examples
- Reproducibility vs Replicability | Difference & Examples
- Reproducibility vs. Replicability | Difference & Examples
- Sampling Methods | Types, Techniques & Examples
- Semi-Structured Interview | Definition, Guide & Examples
- Simple Random Sampling | Definition, Steps & Examples
- Single, Double, & Triple Blind Study | Definition & Examples
- Stratified Sampling | Definition, Guide & Examples
- Structured Interview | Definition, Guide & Examples
- Survey Research | Definition, Examples & Methods
- Systematic Review | Definition, Example, & Guide
- Systematic Sampling | A Step-by-Step Guide with Examples
- Textual Analysis | Guide, 3 Approaches & Examples
- The 4 Types of Reliability in Research | Definitions & Examples
- The 4 Types of Validity in Research | Definitions & Examples
- Transcribing an Interview | 5 Steps & Transcription Software
- Triangulation in Research | Guide, Types, Examples
- Types of Interviews in Research | Guide & Examples
- Types of Research Designs Compared | Guide & Examples
- Types of Variables in Research & Statistics | Examples
- Unstructured Interview | Definition, Guide & Examples
- What Is a Case Study? | Definition, Examples & Methods
- What Is a Case-Control Study? | Definition & Examples
- What Is a Cohort Study? | Definition & Examples
- What Is a Conceptual Framework? | Tips & Examples
- What Is a Controlled Experiment? | Definitions & Examples
- What Is a Double-Barreled Question?
- What Is a Focus Group? | Step-by-Step Guide & Examples
- What Is a Likert Scale? | Guide & Examples
- What Is a Prospective Cohort Study? | Definition & Examples
- What Is a Retrospective Cohort Study? | Definition & Examples
- What Is Action Research? | Definition & Examples
- What Is an Observational Study? | Guide & Examples
- What Is Concurrent Validity? | Definition & Examples
- What Is Content Validity? | Definition & Examples
- What Is Convenience Sampling? | Definition & Examples
- What Is Convergent Validity? | Definition & Examples
- What Is Criterion Validity? | Definition & Examples
- What Is Data Cleansing? | Definition, Guide & Examples
- What Is Deductive Reasoning? | Explanation & Examples
- What Is Discriminant Validity? | Definition & Example
- What Is Ecological Validity? | Definition & Examples
- What Is Ethnography? | Definition, Guide & Examples
- What Is Face Validity? | Guide, Definition & Examples
- What Is Non-Probability Sampling? | Types & Examples
- What Is Participant Observation? | Definition & Examples
- What Is Peer Review? | Types & Examples
- What Is Predictive Validity? | Examples & Definition
- What Is Probability Sampling? | Types & Examples
- What Is Purposive Sampling? | Definition & Examples
- What Is Qualitative Observation? | Definition & Examples
- What Is Qualitative Research? | Methods & Examples
- What Is Quantitative Observation? | Definition & Examples
- What Is Quantitative Research? | Definition, Uses & Methods
"I thought AI Proofreading was useless but.."
I've been using Scribbr for years now and I know it's a service that won't disappoint. It does a good job spotting mistakes”
- Privacy Policy

Home » Research Data – Types Methods and Examples
Research Data – Types Methods and Examples
Table of Contents

Research Data
Research data refers to any information or evidence gathered through systematic investigation or experimentation to support or refute a hypothesis or answer a research question.
It includes both primary and secondary data, and can be in various formats such as numerical, textual, audiovisual, or visual. Research data plays a critical role in scientific inquiry and is often subject to rigorous analysis, interpretation, and dissemination to advance knowledge and inform decision-making.
Types of Research Data
There are generally four types of research data:
Quantitative Data
This type of data involves the collection and analysis of numerical data. It is often gathered through surveys, experiments, or other types of structured data collection methods. Quantitative data can be analyzed using statistical techniques to identify patterns or relationships in the data.
Qualitative Data
This type of data is non-numerical and often involves the collection and analysis of words, images, or sounds. It is often gathered through methods such as interviews, focus groups, or observation. Qualitative data can be analyzed using techniques such as content analysis, thematic analysis, or discourse analysis.
Primary Data
This type of data is collected by the researcher directly from the source. It can include data gathered through surveys, experiments, interviews, or observation. Primary data is often used to answer specific research questions or to test hypotheses.
Secondary Data
This type of data is collected by someone other than the researcher. It can include data from sources such as government reports, academic journals, or industry publications. Secondary data is often used to supplement or support primary data or to provide context for a research project.
Research Data Formates
There are several formats in which research data can be collected and stored. Some common formats include:
- Text : This format includes any type of written data, such as interview transcripts, survey responses, or open-ended questionnaire answers.
- Numeric : This format includes any data that can be expressed as numerical values, such as measurements or counts.
- Audio : This format includes any recorded data in an audio form, such as interviews or focus group discussions.
- Video : This format includes any recorded data in a video form, such as observations of behavior or experimental procedures.
- Images : This format includes any visual data, such as photographs, drawings, or scans of documents.
- Mixed media: This format includes any combination of the above formats, such as a survey response that includes both text and numeric data, or an observation study that includes both video and audio recordings.
- Sensor Data: This format includes data collected from various sensors or devices, such as GPS, accelerometers, or heart rate monitors.
- Social Media Data: This format includes data collected from social media platforms, such as tweets, posts, or comments.
- Geographic Information System (GIS) Data: This format includes data with a spatial component, such as maps or satellite imagery.
- Machine-Readable Data : This format includes data that can be read and processed by machines, such as data in XML or JSON format.
- Metadata: This format includes data that describes other data, such as information about the source, format, or content of a dataset.
Data Collection Methods
Some common research data collection methods include:
- Surveys : Surveys involve asking participants to answer a series of questions about a particular topic. Surveys can be conducted online, over the phone, or in person.
- Interviews : Interviews involve asking participants a series of open-ended questions in order to gather detailed information about their experiences or perspectives. Interviews can be conducted in person, over the phone, or via video conferencing.
- Focus groups: Focus groups involve bringing together a small group of participants to discuss a particular topic or issue in depth. The group is typically led by a moderator who asks questions and encourages discussion among the participants.
- Observations : Observations involve watching and recording behaviors or events as they naturally occur. Observations can be conducted in person or through the use of video or audio recordings.
- Experiments : Experiments involve manipulating one or more variables in order to measure the effect on an outcome of interest. Experiments can be conducted in a laboratory or in the field.
- Case studies: Case studies involve conducting an in-depth analysis of a particular individual, group, or organization. Case studies typically involve gathering data from multiple sources, including interviews, observations, and document analysis.
- Secondary data analysis: Secondary data analysis involves analyzing existing data that was collected for another purpose. Examples of secondary data sources include government records, academic research studies, and market research reports.
Analysis Methods
Some common research data analysis methods include:
- Descriptive statistics: Descriptive statistics involve summarizing and describing the main features of a dataset, such as the mean, median, and standard deviation. Descriptive statistics are often used to provide an initial overview of the data.
- Inferential statistics: Inferential statistics involve using statistical techniques to draw conclusions about a population based on a sample of data. Inferential statistics are often used to test hypotheses and determine the statistical significance of relationships between variables.
- Content analysis : Content analysis involves analyzing the content of text, audio, or video data to identify patterns, themes, or other meaningful features. Content analysis is often used in qualitative research to analyze open-ended survey responses, interviews, or other types of text data.
- Discourse analysis: Discourse analysis involves analyzing the language used in text, audio, or video data to understand how meaning is constructed and communicated. Discourse analysis is often used in qualitative research to analyze interviews, focus group discussions, or other types of text data.
- Grounded theory : Grounded theory involves developing a theory or model based on an analysis of qualitative data. Grounded theory is often used in exploratory research to generate new insights and hypotheses.
- Network analysis: Network analysis involves analyzing the relationships between entities, such as individuals or organizations, in a network. Network analysis is often used in social network analysis to understand the structure and dynamics of social networks.
- Structural equation modeling: Structural equation modeling involves using statistical techniques to test complex models that include multiple variables and relationships. Structural equation modeling is often used in social science research to test theories about the relationships between variables.
Purpose of Research Data
Research data serves several important purposes, including:
- Supporting scientific discoveries : Research data provides the basis for scientific discoveries and innovations. Researchers use data to test hypotheses, develop new theories, and advance scientific knowledge in their field.
- Validating research findings: Research data provides the evidence necessary to validate research findings. By analyzing and interpreting data, researchers can determine the statistical significance of relationships between variables and draw conclusions about the research question.
- Informing policy decisions: Research data can be used to inform policy decisions by providing evidence about the effectiveness of different policies or interventions. Policymakers can use data to make informed decisions about how to allocate resources and address social or economic challenges.
- Promoting transparency and accountability: Research data promotes transparency and accountability by allowing other researchers to verify and replicate research findings. Data sharing also promotes transparency by allowing others to examine the methods used to collect and analyze data.
- Supporting education and training: Research data can be used to support education and training by providing examples of research methods, data analysis techniques, and research findings. Students and researchers can use data to learn new research skills and to develop their own research projects.
Applications of Research Data
Research data has numerous applications across various fields, including social sciences, natural sciences, engineering, and health sciences. The applications of research data can be broadly classified into the following categories:
- Academic research: Research data is widely used in academic research to test hypotheses, develop new theories, and advance scientific knowledge. Researchers use data to explore complex relationships between variables, identify patterns, and make predictions.
- Business and industry: Research data is used in business and industry to make informed decisions about product development, marketing, and customer engagement. Data analysis techniques such as market research, customer analytics, and financial analysis are widely used to gain insights and inform strategic decision-making.
- Healthcare: Research data is used in healthcare to improve patient outcomes, develop new treatments, and identify health risks. Researchers use data to analyze health trends, track disease outbreaks, and develop evidence-based treatment protocols.
- Education : Research data is used in education to improve teaching and learning outcomes. Data analysis techniques such as assessments, surveys, and evaluations are used to measure student progress, evaluate program effectiveness, and inform policy decisions.
- Government and public policy: Research data is used in government and public policy to inform decision-making and policy development. Data analysis techniques such as demographic analysis, cost-benefit analysis, and impact evaluation are widely used to evaluate policy effectiveness, identify social or economic challenges, and develop evidence-based policy solutions.
- Environmental management: Research data is used in environmental management to monitor environmental conditions, track changes, and identify emerging threats. Data analysis techniques such as spatial analysis, remote sensing, and modeling are used to map environmental features, monitor ecosystem health, and inform policy decisions.
Advantages of Research Data
Research data has numerous advantages, including:
- Empirical evidence: Research data provides empirical evidence that can be used to support or refute theories, test hypotheses, and inform decision-making. This evidence-based approach helps to ensure that decisions are based on objective, measurable data rather than subjective opinions or assumptions.
- Accuracy and reliability : Research data is typically collected using rigorous scientific methods and protocols, which helps to ensure its accuracy and reliability. Data can be validated and verified using statistical methods, which further enhances its credibility.
- Replicability: Research data can be replicated and validated by other researchers, which helps to promote transparency and accountability in research. By making data available for others to analyze and interpret, researchers can ensure that their findings are robust and reliable.
- Insights and discoveries : Research data can provide insights into complex relationships between variables, identify patterns and trends, and reveal new discoveries. These insights can lead to the development of new theories, treatments, and interventions that can improve outcomes in various fields.
- Informed decision-making: Research data can inform decision-making in a range of fields, including healthcare, business, education, and public policy. Data analysis techniques can be used to identify trends, evaluate the effectiveness of interventions, and inform policy decisions.
- Efficiency and cost-effectiveness: Research data can help to improve efficiency and cost-effectiveness by identifying areas where resources can be directed most effectively. By using data to identify the most promising approaches or interventions, researchers can optimize the use of resources and improve outcomes.
Limitations of Research Data
Research data has several limitations that researchers should be aware of, including:
- Bias and subjectivity: Research data can be influenced by biases and subjectivity, which can affect the accuracy and reliability of the data. Researchers must take steps to minimize bias and subjectivity in data collection and analysis.
- Incomplete data : Research data can be incomplete or missing, which can affect the validity of the findings. Researchers must ensure that data is complete and representative to ensure that their findings are reliable.
- Limited scope: Research data may be limited in scope, which can limit the generalizability of the findings. Researchers must carefully consider the scope of their research and ensure that their findings are applicable to the broader population.
- Data quality: Research data can be affected by issues such as measurement error, data entry errors, and missing data, which can affect the quality of the data. Researchers must ensure that data is collected and analyzed using rigorous methods to minimize these issues.
- Ethical concerns: Research data can raise ethical concerns, particularly when it involves human subjects. Researchers must ensure that their research complies with ethical standards and protects the rights and privacy of human subjects.
- Data security: Research data must be protected to prevent unauthorized access or use. Researchers must ensure that data is stored and transmitted securely to protect the confidentiality and integrity of the data.
About the author
Muhammad Hassan
Researcher, Academic Writer, Web developer
You may also like

Quantitative Data – Types, Methods and Examples

Secondary Data – Types, Methods and Examples

Information in Research – Types and Examples

Qualitative Data – Types, Methods and Examples

Primary Data – Types, Methods and Examples
Unfortunately we don't fully support your browser. If you have the option to, please upgrade to a newer version or use Mozilla Firefox , Microsoft Edge , Google Chrome , or Safari 14 or newer. If you are unable to, and need support, please send us your feedback .
We'd appreciate your feedback. Tell us what you think! opens in new tab/window
Sharing research data
As a researcher, you are increasingly encouraged, or even mandated, to make your research data available, accessible, discoverable and usable.
Sharing research data is something we are passionate about too, so we’ve created this short video and written guide to help you get started.
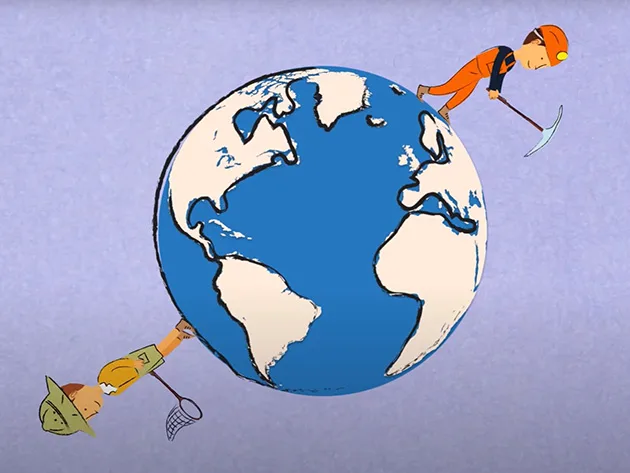
Research Data
What is research data.
While the definition often differs per field, generally, research data refers to the results of observations or experiments that validate your research findings. These span a range of useful materials associated with your research project, including:
Raw or processed data files
Research data does not include text in manuscript or final published article form, or data or other materials submitted and published as part of a journal article.
Why should I share my research data?
There are so many good reasons. We’ve listed just a few:
How you benefit
You get credit for the work you've done
Leads to more citations! 1
Can boost your number of publications
Increases your exposure and may lead to new collaborations
What it means for the research community
It's easy to reuse and reinterpret your data
Duplication of experiments can be avoided
New insights can be gained, sparking new lines of inquiry
Empowers replication
And society at large…
Greater transparency boosts public faith in research
Can play a role in guiding government policy
Improves access to research for those outside health and academia
Benefits the public purse as funding of repeat work is reduced
How do I share my research data?
The good news is it’s easy.
Yet to submit your research article? There are a number of options available. These may vary depending on the journal you have chosen, so be sure to read the Research Data section in its Guide for Authors before you begin.
Already published your research article? No problem – it’s never too late to share the research data associated with it.
Two of the most popular data sharing routes are:
Publishing a research elements article
These brief, peer-reviewed articles complement full research papers and are an easy way to receive proper credit and recognition for the work you have done. Research elements are research outputs that have come about as a result of following the research cycle – this includes things like data, methods and protocols, software, hardware and more.
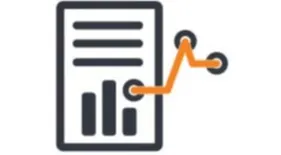
You can publish research elements articles in several different Elsevier journals, including our suite of dedicated Research Elements journals . They are easy to submit, are subject to a peer review process, receive a DOI and are fully citable. They also make your work more sharable, discoverable, comprehensible, reusable and reproducible.
The accompanying raw data can still be placed in a repository of your choice (see below).
Uploading your data to a repository like Mendeley Data
Mendeley Data is a certified, free-to-use repository that hosts open data from all disciplines, whatever its format (e.g. raw and processed data, tables, codes and software). With many Elsevier journals, it’s possible to upload and store your data to Mendeley Data during the manuscript submission process. You can also upload your data directly to the repository. In each case, your data will receive a DOI, making it independently citable and it can be linked to any associated article on ScienceDirect, making it easy for readers to find and reuse.
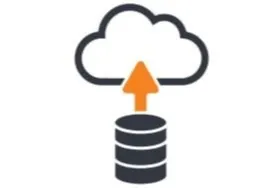
View an article featuring Mendeley data opens in new tab/window (just select the Research Data link in the left-hand bar or scroll down the page).
What if I can’t submit my research data?
Data statements offer transparency.
We understand that there are times when the data is simply not available to post or there are good reasons why it shouldn’t be shared. A number of Elsevier journals encourage authors to submit a data statement alongside their manuscript. This statement allows you to clearly explain the data you’ve used in the article and the reasons why it might not be available. The statement will appear with the article on ScienceDirect.
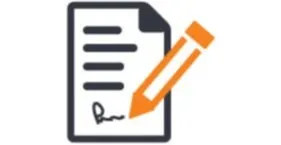
View a sample data statement opens in new tab/window (just select the Research Data link in the left-hand bar or scroll down the page).
Showcasing your research data on ScienceDirect
We have 3 top tips to help you maximize the impact of your data in your article on ScienceDirect.
Link with data repositories
You can create bidirectional links between any data repositories you’ve used to store your data and your online article. If you’ve published a data article, you can link to that too.
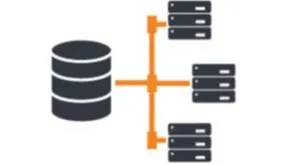
Enrich with interactive data visualizations
The days of being confined to static visuals are over. Our in-article interactive viewers let readers delve into the data with helpful functions such as zoom, configurable display options and full screen mode.
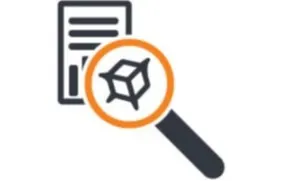
Cite your research data
Get credit for your work by citing your research data in your article and adding a data reference to the reference list. This ensures you are recognized for the data you shared and/or used in your research. Read the References section in your chosen journal’s Guide for Authors for more information.
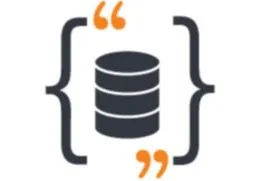
Ready to get started?
If you have yet to publish your research paper, the first step is to find the right journal for your submission and read the Guide for Authors .
Find a journal by matching paper title and abstract of your manuscript in Elsevier's JournalFinder opens in new tab/window
Find journal by title opens in new tab/window
Already published? Just view the options for sharing your research data above.
1 Several studies have now shown that making data available for an article increases article citations.

An official website of the United States government
The .gov means it’s official. Federal government websites often end in .gov or .mil. Before sharing sensitive information, make sure you’re on a federal government site.
The site is secure. The https:// ensures that you are connecting to the official website and that any information you provide is encrypted and transmitted securely.
- Publications
- Account settings
Preview improvements coming to the PMC website in October 2024. Learn More or Try it out now .
- Advanced Search
- Journal List
- Can J Hosp Pharm
- v.68(3); May-Jun 2015

Qualitative Research: Data Collection, Analysis, and Management
Introduction.
In an earlier paper, 1 we presented an introduction to using qualitative research methods in pharmacy practice. In this article, we review some principles of the collection, analysis, and management of qualitative data to help pharmacists interested in doing research in their practice to continue their learning in this area. Qualitative research can help researchers to access the thoughts and feelings of research participants, which can enable development of an understanding of the meaning that people ascribe to their experiences. Whereas quantitative research methods can be used to determine how many people undertake particular behaviours, qualitative methods can help researchers to understand how and why such behaviours take place. Within the context of pharmacy practice research, qualitative approaches have been used to examine a diverse array of topics, including the perceptions of key stakeholders regarding prescribing by pharmacists and the postgraduation employment experiences of young pharmacists (see “Further Reading” section at the end of this article).
In the previous paper, 1 we outlined 3 commonly used methodologies: ethnography 2 , grounded theory 3 , and phenomenology. 4 Briefly, ethnography involves researchers using direct observation to study participants in their “real life” environment, sometimes over extended periods. Grounded theory and its later modified versions (e.g., Strauss and Corbin 5 ) use face-to-face interviews and interactions such as focus groups to explore a particular research phenomenon and may help in clarifying a less-well-understood problem, situation, or context. Phenomenology shares some features with grounded theory (such as an exploration of participants’ behaviour) and uses similar techniques to collect data, but it focuses on understanding how human beings experience their world. It gives researchers the opportunity to put themselves in another person’s shoes and to understand the subjective experiences of participants. 6 Some researchers use qualitative methodologies but adopt a different standpoint, and an example of this appears in the work of Thurston and others, 7 discussed later in this paper.
Qualitative work requires reflection on the part of researchers, both before and during the research process, as a way of providing context and understanding for readers. When being reflexive, researchers should not try to simply ignore or avoid their own biases (as this would likely be impossible); instead, reflexivity requires researchers to reflect upon and clearly articulate their position and subjectivities (world view, perspectives, biases), so that readers can better understand the filters through which questions were asked, data were gathered and analyzed, and findings were reported. From this perspective, bias and subjectivity are not inherently negative but they are unavoidable; as a result, it is best that they be articulated up-front in a manner that is clear and coherent for readers.
THE PARTICIPANT’S VIEWPOINT
What qualitative study seeks to convey is why people have thoughts and feelings that might affect the way they behave. Such study may occur in any number of contexts, but here, we focus on pharmacy practice and the way people behave with regard to medicines use (e.g., to understand patients’ reasons for nonadherence with medication therapy or to explore physicians’ resistance to pharmacists’ clinical suggestions). As we suggested in our earlier article, 1 an important point about qualitative research is that there is no attempt to generalize the findings to a wider population. Qualitative research is used to gain insights into people’s feelings and thoughts, which may provide the basis for a future stand-alone qualitative study or may help researchers to map out survey instruments for use in a quantitative study. It is also possible to use different types of research in the same study, an approach known as “mixed methods” research, and further reading on this topic may be found at the end of this paper.
The role of the researcher in qualitative research is to attempt to access the thoughts and feelings of study participants. This is not an easy task, as it involves asking people to talk about things that may be very personal to them. Sometimes the experiences being explored are fresh in the participant’s mind, whereas on other occasions reliving past experiences may be difficult. However the data are being collected, a primary responsibility of the researcher is to safeguard participants and their data. Mechanisms for such safeguarding must be clearly articulated to participants and must be approved by a relevant research ethics review board before the research begins. Researchers and practitioners new to qualitative research should seek advice from an experienced qualitative researcher before embarking on their project.
DATA COLLECTION
Whatever philosophical standpoint the researcher is taking and whatever the data collection method (e.g., focus group, one-to-one interviews), the process will involve the generation of large amounts of data. In addition to the variety of study methodologies available, there are also different ways of making a record of what is said and done during an interview or focus group, such as taking handwritten notes or video-recording. If the researcher is audio- or video-recording data collection, then the recordings must be transcribed verbatim before data analysis can begin. As a rough guide, it can take an experienced researcher/transcriber 8 hours to transcribe one 45-minute audio-recorded interview, a process than will generate 20–30 pages of written dialogue.
Many researchers will also maintain a folder of “field notes” to complement audio-taped interviews. Field notes allow the researcher to maintain and comment upon impressions, environmental contexts, behaviours, and nonverbal cues that may not be adequately captured through the audio-recording; they are typically handwritten in a small notebook at the same time the interview takes place. Field notes can provide important context to the interpretation of audio-taped data and can help remind the researcher of situational factors that may be important during data analysis. Such notes need not be formal, but they should be maintained and secured in a similar manner to audio tapes and transcripts, as they contain sensitive information and are relevant to the research. For more information about collecting qualitative data, please see the “Further Reading” section at the end of this paper.
DATA ANALYSIS AND MANAGEMENT
If, as suggested earlier, doing qualitative research is about putting oneself in another person’s shoes and seeing the world from that person’s perspective, the most important part of data analysis and management is to be true to the participants. It is their voices that the researcher is trying to hear, so that they can be interpreted and reported on for others to read and learn from. To illustrate this point, consider the anonymized transcript excerpt presented in Appendix 1 , which is taken from a research interview conducted by one of the authors (J.S.). We refer to this excerpt throughout the remainder of this paper to illustrate how data can be managed, analyzed, and presented.
Interpretation of Data
Interpretation of the data will depend on the theoretical standpoint taken by researchers. For example, the title of the research report by Thurston and others, 7 “Discordant indigenous and provider frames explain challenges in improving access to arthritis care: a qualitative study using constructivist grounded theory,” indicates at least 2 theoretical standpoints. The first is the culture of the indigenous population of Canada and the place of this population in society, and the second is the social constructivist theory used in the constructivist grounded theory method. With regard to the first standpoint, it can be surmised that, to have decided to conduct the research, the researchers must have felt that there was anecdotal evidence of differences in access to arthritis care for patients from indigenous and non-indigenous backgrounds. With regard to the second standpoint, it can be surmised that the researchers used social constructivist theory because it assumes that behaviour is socially constructed; in other words, people do things because of the expectations of those in their personal world or in the wider society in which they live. (Please see the “Further Reading” section for resources providing more information about social constructivist theory and reflexivity.) Thus, these 2 standpoints (and there may have been others relevant to the research of Thurston and others 7 ) will have affected the way in which these researchers interpreted the experiences of the indigenous population participants and those providing their care. Another standpoint is feminist standpoint theory which, among other things, focuses on marginalized groups in society. Such theories are helpful to researchers, as they enable us to think about things from a different perspective. Being aware of the standpoints you are taking in your own research is one of the foundations of qualitative work. Without such awareness, it is easy to slip into interpreting other people’s narratives from your own viewpoint, rather than that of the participants.
To analyze the example in Appendix 1 , we will adopt a phenomenological approach because we want to understand how the participant experienced the illness and we want to try to see the experience from that person’s perspective. It is important for the researcher to reflect upon and articulate his or her starting point for such analysis; for example, in the example, the coder could reflect upon her own experience as a female of a majority ethnocultural group who has lived within middle class and upper middle class settings. This personal history therefore forms the filter through which the data will be examined. This filter does not diminish the quality or significance of the analysis, since every researcher has his or her own filters; however, by explicitly stating and acknowledging what these filters are, the researcher makes it easer for readers to contextualize the work.
Transcribing and Checking
For the purposes of this paper it is assumed that interviews or focus groups have been audio-recorded. As mentioned above, transcribing is an arduous process, even for the most experienced transcribers, but it must be done to convert the spoken word to the written word to facilitate analysis. For anyone new to conducting qualitative research, it is beneficial to transcribe at least one interview and one focus group. It is only by doing this that researchers realize how difficult the task is, and this realization affects their expectations when asking others to transcribe. If the research project has sufficient funding, then a professional transcriber can be hired to do the work. If this is the case, then it is a good idea to sit down with the transcriber, if possible, and talk through the research and what the participants were talking about. This background knowledge for the transcriber is especially important in research in which people are using jargon or medical terms (as in pharmacy practice). Involving your transcriber in this way makes the work both easier and more rewarding, as he or she will feel part of the team. Transcription editing software is also available, but it is expensive. For example, ELAN (more formally known as EUDICO Linguistic Annotator, developed at the Technical University of Berlin) 8 is a tool that can help keep data organized by linking media and data files (particularly valuable if, for example, video-taping of interviews is complemented by transcriptions). It can also be helpful in searching complex data sets. Products such as ELAN do not actually automatically transcribe interviews or complete analyses, and they do require some time and effort to learn; nonetheless, for some research applications, it may be a valuable to consider such software tools.
All audio recordings should be transcribed verbatim, regardless of how intelligible the transcript may be when it is read back. Lines of text should be numbered. Once the transcription is complete, the researcher should read it while listening to the recording and do the following: correct any spelling or other errors; anonymize the transcript so that the participant cannot be identified from anything that is said (e.g., names, places, significant events); insert notations for pauses, laughter, looks of discomfort; insert any punctuation, such as commas and full stops (periods) (see Appendix 1 for examples of inserted punctuation), and include any other contextual information that might have affected the participant (e.g., temperature or comfort of the room).
Dealing with the transcription of a focus group is slightly more difficult, as multiple voices are involved. One way of transcribing such data is to “tag” each voice (e.g., Voice A, Voice B). In addition, the focus group will usually have 2 facilitators, whose respective roles will help in making sense of the data. While one facilitator guides participants through the topic, the other can make notes about context and group dynamics. More information about group dynamics and focus groups can be found in resources listed in the “Further Reading” section.
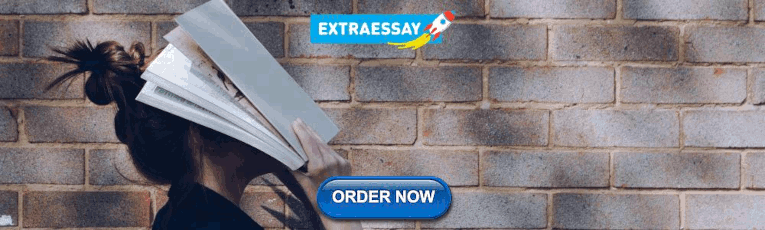
Reading between the Lines
During the process outlined above, the researcher can begin to get a feel for the participant’s experience of the phenomenon in question and can start to think about things that could be pursued in subsequent interviews or focus groups (if appropriate). In this way, one participant’s narrative informs the next, and the researcher can continue to interview until nothing new is being heard or, as it says in the text books, “saturation is reached”. While continuing with the processes of coding and theming (described in the next 2 sections), it is important to consider not just what the person is saying but also what they are not saying. For example, is a lengthy pause an indication that the participant is finding the subject difficult, or is the person simply deciding what to say? The aim of the whole process from data collection to presentation is to tell the participants’ stories using exemplars from their own narratives, thus grounding the research findings in the participants’ lived experiences.
Smith 9 suggested a qualitative research method known as interpretative phenomenological analysis, which has 2 basic tenets: first, that it is rooted in phenomenology, attempting to understand the meaning that individuals ascribe to their lived experiences, and second, that the researcher must attempt to interpret this meaning in the context of the research. That the researcher has some knowledge and expertise in the subject of the research means that he or she can have considerable scope in interpreting the participant’s experiences. Larkin and others 10 discussed the importance of not just providing a description of what participants say. Rather, interpretative phenomenological analysis is about getting underneath what a person is saying to try to truly understand the world from his or her perspective.
Once all of the research interviews have been transcribed and checked, it is time to begin coding. Field notes compiled during an interview can be a useful complementary source of information to facilitate this process, as the gap in time between an interview, transcribing, and coding can result in memory bias regarding nonverbal or environmental context issues that may affect interpretation of data.
Coding refers to the identification of topics, issues, similarities, and differences that are revealed through the participants’ narratives and interpreted by the researcher. This process enables the researcher to begin to understand the world from each participant’s perspective. Coding can be done by hand on a hard copy of the transcript, by making notes in the margin or by highlighting and naming sections of text. More commonly, researchers use qualitative research software (e.g., NVivo, QSR International Pty Ltd; www.qsrinternational.com/products_nvivo.aspx ) to help manage their transcriptions. It is advised that researchers undertake a formal course in the use of such software or seek supervision from a researcher experienced in these tools.
Returning to Appendix 1 and reading from lines 8–11, a code for this section might be “diagnosis of mental health condition”, but this would just be a description of what the participant is talking about at that point. If we read a little more deeply, we can ask ourselves how the participant might have come to feel that the doctor assumed he or she was aware of the diagnosis or indeed that they had only just been told the diagnosis. There are a number of pauses in the narrative that might suggest the participant is finding it difficult to recall that experience. Later in the text, the participant says “nobody asked me any questions about my life” (line 19). This could be coded simply as “health care professionals’ consultation skills”, but that would not reflect how the participant must have felt never to be asked anything about his or her personal life, about the participant as a human being. At the end of this excerpt, the participant just trails off, recalling that no-one showed any interest, which makes for very moving reading. For practitioners in pharmacy, it might also be pertinent to explore the participant’s experience of akathisia and why this was left untreated for 20 years.
One of the questions that arises about qualitative research relates to the reliability of the interpretation and representation of the participants’ narratives. There are no statistical tests that can be used to check reliability and validity as there are in quantitative research. However, work by Lincoln and Guba 11 suggests that there are other ways to “establish confidence in the ‘truth’ of the findings” (p. 218). They call this confidence “trustworthiness” and suggest that there are 4 criteria of trustworthiness: credibility (confidence in the “truth” of the findings), transferability (showing that the findings have applicability in other contexts), dependability (showing that the findings are consistent and could be repeated), and confirmability (the extent to which the findings of a study are shaped by the respondents and not researcher bias, motivation, or interest).
One way of establishing the “credibility” of the coding is to ask another researcher to code the same transcript and then to discuss any similarities and differences in the 2 resulting sets of codes. This simple act can result in revisions to the codes and can help to clarify and confirm the research findings.
Theming refers to the drawing together of codes from one or more transcripts to present the findings of qualitative research in a coherent and meaningful way. For example, there may be examples across participants’ narratives of the way in which they were treated in hospital, such as “not being listened to” or “lack of interest in personal experiences” (see Appendix 1 ). These may be drawn together as a theme running through the narratives that could be named “the patient’s experience of hospital care”. The importance of going through this process is that at its conclusion, it will be possible to present the data from the interviews using quotations from the individual transcripts to illustrate the source of the researchers’ interpretations. Thus, when the findings are organized for presentation, each theme can become the heading of a section in the report or presentation. Underneath each theme will be the codes, examples from the transcripts, and the researcher’s own interpretation of what the themes mean. Implications for real life (e.g., the treatment of people with chronic mental health problems) should also be given.
DATA SYNTHESIS
In this final section of this paper, we describe some ways of drawing together or “synthesizing” research findings to represent, as faithfully as possible, the meaning that participants ascribe to their life experiences. This synthesis is the aim of the final stage of qualitative research. For most readers, the synthesis of data presented by the researcher is of crucial significance—this is usually where “the story” of the participants can be distilled, summarized, and told in a manner that is both respectful to those participants and meaningful to readers. There are a number of ways in which researchers can synthesize and present their findings, but any conclusions drawn by the researchers must be supported by direct quotations from the participants. In this way, it is made clear to the reader that the themes under discussion have emerged from the participants’ interviews and not the mind of the researcher. The work of Latif and others 12 gives an example of how qualitative research findings might be presented.
Planning and Writing the Report
As has been suggested above, if researchers code and theme their material appropriately, they will naturally find the headings for sections of their report. Qualitative researchers tend to report “findings” rather than “results”, as the latter term typically implies that the data have come from a quantitative source. The final presentation of the research will usually be in the form of a report or a paper and so should follow accepted academic guidelines. In particular, the article should begin with an introduction, including a literature review and rationale for the research. There should be a section on the chosen methodology and a brief discussion about why qualitative methodology was most appropriate for the study question and why one particular methodology (e.g., interpretative phenomenological analysis rather than grounded theory) was selected to guide the research. The method itself should then be described, including ethics approval, choice of participants, mode of recruitment, and method of data collection (e.g., semistructured interviews or focus groups), followed by the research findings, which will be the main body of the report or paper. The findings should be written as if a story is being told; as such, it is not necessary to have a lengthy discussion section at the end. This is because much of the discussion will take place around the participants’ quotes, such that all that is needed to close the report or paper is a summary, limitations of the research, and the implications that the research has for practice. As stated earlier, it is not the intention of qualitative research to allow the findings to be generalized, and therefore this is not, in itself, a limitation.
Planning out the way that findings are to be presented is helpful. It is useful to insert the headings of the sections (the themes) and then make a note of the codes that exemplify the thoughts and feelings of your participants. It is generally advisable to put in the quotations that you want to use for each theme, using each quotation only once. After all this is done, the telling of the story can begin as you give your voice to the experiences of the participants, writing around their quotations. Do not be afraid to draw assumptions from the participants’ narratives, as this is necessary to give an in-depth account of the phenomena in question. Discuss these assumptions, drawing on your participants’ words to support you as you move from one code to another and from one theme to the next. Finally, as appropriate, it is possible to include examples from literature or policy documents that add support for your findings. As an exercise, you may wish to code and theme the sample excerpt in Appendix 1 and tell the participant’s story in your own way. Further reading about “doing” qualitative research can be found at the end of this paper.
CONCLUSIONS
Qualitative research can help researchers to access the thoughts and feelings of research participants, which can enable development of an understanding of the meaning that people ascribe to their experiences. It can be used in pharmacy practice research to explore how patients feel about their health and their treatment. Qualitative research has been used by pharmacists to explore a variety of questions and problems (see the “Further Reading” section for examples). An understanding of these issues can help pharmacists and other health care professionals to tailor health care to match the individual needs of patients and to develop a concordant relationship. Doing qualitative research is not easy and may require a complete rethink of how research is conducted, particularly for researchers who are more familiar with quantitative approaches. There are many ways of conducting qualitative research, and this paper has covered some of the practical issues regarding data collection, analysis, and management. Further reading around the subject will be essential to truly understand this method of accessing peoples’ thoughts and feelings to enable researchers to tell participants’ stories.
Appendix 1. Excerpt from a sample transcript
The participant (age late 50s) had suffered from a chronic mental health illness for 30 years. The participant had become a “revolving door patient,” someone who is frequently in and out of hospital. As the participant talked about past experiences, the researcher asked:
- What was treatment like 30 years ago?
- Umm—well it was pretty much they could do what they wanted with you because I was put into the er, the er kind of system er, I was just on
- endless section threes.
- Really…
- But what I didn’t realize until later was that if you haven’t actually posed a threat to someone or yourself they can’t really do that but I didn’t know
- that. So wh-when I first went into hospital they put me on the forensic ward ’cause they said, “We don’t think you’ll stay here we think you’ll just
- run-run away.” So they put me then onto the acute admissions ward and – er – I can remember one of the first things I recall when I got onto that
- ward was sitting down with a er a Dr XXX. He had a book this thick [gestures] and on each page it was like three questions and he went through
- all these questions and I answered all these questions. So we’re there for I don’t maybe two hours doing all that and he asked me he said “well
- when did somebody tell you then that you have schizophrenia” I said “well nobody’s told me that” so he seemed very surprised but nobody had
- actually [pause] whe-when I first went up there under police escort erm the senior kind of consultants people I’d been to where I was staying and
- ermm so er [pause] I . . . the, I can remember the very first night that I was there and given this injection in this muscle here [gestures] and just
- having dreadful side effects the next day I woke up [pause]
- . . . and I suffered that akathesia I swear to you, every minute of every day for about 20 years.
- Oh how awful.
- And that side of it just makes life impossible so the care on the wards [pause] umm I don’t know it’s kind of, it’s kind of hard to put into words
- [pause]. Because I’m not saying they were sort of like not friendly or interested but then nobody ever seemed to want to talk about your life [pause]
- nobody asked me any questions about my life. The only questions that came into was they asked me if I’d be a volunteer for these student exams
- and things and I said “yeah” so all the questions were like “oh what jobs have you done,” er about your relationships and things and er but
- nobody actually sat down and had a talk and showed some interest in you as a person you were just there basically [pause] um labelled and you
- know there was there was [pause] but umm [pause] yeah . . .
This article is the 10th in the CJHP Research Primer Series, an initiative of the CJHP Editorial Board and the CSHP Research Committee. The planned 2-year series is intended to appeal to relatively inexperienced researchers, with the goal of building research capacity among practising pharmacists. The articles, presenting simple but rigorous guidance to encourage and support novice researchers, are being solicited from authors with appropriate expertise.
Previous articles in this series:
Bond CM. The research jigsaw: how to get started. Can J Hosp Pharm . 2014;67(1):28–30.
Tully MP. Research: articulating questions, generating hypotheses, and choosing study designs. Can J Hosp Pharm . 2014;67(1):31–4.
Loewen P. Ethical issues in pharmacy practice research: an introductory guide. Can J Hosp Pharm. 2014;67(2):133–7.
Tsuyuki RT. Designing pharmacy practice research trials. Can J Hosp Pharm . 2014;67(3):226–9.
Bresee LC. An introduction to developing surveys for pharmacy practice research. Can J Hosp Pharm . 2014;67(4):286–91.
Gamble JM. An introduction to the fundamentals of cohort and case–control studies. Can J Hosp Pharm . 2014;67(5):366–72.
Austin Z, Sutton J. Qualitative research: getting started. C an J Hosp Pharm . 2014;67(6):436–40.
Houle S. An introduction to the fundamentals of randomized controlled trials in pharmacy research. Can J Hosp Pharm . 2014; 68(1):28–32.
Charrois TL. Systematic reviews: What do you need to know to get started? Can J Hosp Pharm . 2014;68(2):144–8.
Competing interests: None declared.
Further Reading
Examples of qualitative research in pharmacy practice.
- Farrell B, Pottie K, Woodend K, Yao V, Dolovich L, Kennie N, et al. Shifts in expectations: evaluating physicians’ perceptions as pharmacists integrated into family practice. J Interprof Care. 2010; 24 (1):80–9. [ PubMed ] [ Google Scholar ]
- Gregory P, Austin Z. Postgraduation employment experiences of new pharmacists in Ontario in 2012–2013. Can Pharm J. 2014; 147 (5):290–9. [ PMC free article ] [ PubMed ] [ Google Scholar ]
- Marks PZ, Jennnings B, Farrell B, Kennie-Kaulbach N, Jorgenson D, Pearson-Sharpe J, et al. “I gained a skill and a change in attitude”: a case study describing how an online continuing professional education course for pharmacists supported achievement of its transfer to practice outcomes. Can J Univ Contin Educ. 2014; 40 (2):1–18. [ Google Scholar ]
- Nair KM, Dolovich L, Brazil K, Raina P. It’s all about relationships: a qualitative study of health researchers’ perspectives on interdisciplinary research. BMC Health Serv Res. 2008; 8 :110. [ PMC free article ] [ PubMed ] [ Google Scholar ]
- Pojskic N, MacKeigan L, Boon H, Austin Z. Initial perceptions of key stakeholders in Ontario regarding independent prescriptive authority for pharmacists. Res Soc Adm Pharm. 2014; 10 (2):341–54. [ PubMed ] [ Google Scholar ]
Qualitative Research in General
- Breakwell GM, Hammond S, Fife-Schaw C. Research methods in psychology. Thousand Oaks (CA): Sage Publications; 1995. [ Google Scholar ]
- Given LM. 100 questions (and answers) about qualitative research. Thousand Oaks (CA): Sage Publications; 2015. [ Google Scholar ]
- Miles B, Huberman AM. Qualitative data analysis. Thousand Oaks (CA): Sage Publications; 2009. [ Google Scholar ]
- Patton M. Qualitative research and evaluation methods. Thousand Oaks (CA): Sage Publications; 2002. [ Google Scholar ]
- Willig C. Introducing qualitative research in psychology. Buckingham (UK): Open University Press; 2001. [ Google Scholar ]
Group Dynamics in Focus Groups
- Farnsworth J, Boon B. Analysing group dynamics within the focus group. Qual Res. 2010; 10 (5):605–24. [ Google Scholar ]
Social Constructivism
- Social constructivism. Berkeley (CA): University of California, Berkeley, Berkeley Graduate Division, Graduate Student Instruction Teaching & Resource Center; [cited 2015 June 4]. Available from: http://gsi.berkeley.edu/gsi-guide-contents/learning-theory-research/social-constructivism/ [ Google Scholar ]
Mixed Methods
- Creswell J. Research design: qualitative, quantitative, and mixed methods approaches. Thousand Oaks (CA): Sage Publications; 2009. [ Google Scholar ]
Collecting Qualitative Data
- Arksey H, Knight P. Interviewing for social scientists: an introductory resource with examples. Thousand Oaks (CA): Sage Publications; 1999. [ Google Scholar ]
- Guest G, Namey EE, Mitchel ML. Collecting qualitative data: a field manual for applied research. Thousand Oaks (CA): Sage Publications; 2013. [ Google Scholar ]
Constructivist Grounded Theory
- Charmaz K. Grounded theory: objectivist and constructivist methods. In: Denzin N, Lincoln Y, editors. Handbook of qualitative research. 2nd ed. Thousand Oaks (CA): Sage Publications; 2000. pp. 509–35. [ Google Scholar ]
Leveraging AI and Big Data for Advancements in Biomedical Research
11 Pages Posted: 29 Jun 2024
Dimitrios Sargiotis
National Technical University of Athens - Department of Transportation Planning and Engineering
Date Written: June 28, 2024
The convergence of Artificial Intelligence (AI) and Big Data has significantly transformed biomedical research, enhancing the precision and efficiency of disease diagnosis, personalized medicine, and drug discovery. AI, particularly machine learning (ML) and deep learning (DL), excels at analyzing vast datasets to identify patterns and make accurate predictions. When integrated with the extensive datasets of Big Data, these technologies facilitate more accurate diagnoses, individualized treatments, and expedited drug discovery processes. This review explores the substantial contributions of AI and Big Data to biomedical research, highlighting key advancements and applications such as the use of AI-driven models in disease diagnosis, the development of personalized medical treatments based on genetic profiles, and the acceleration of drug discovery through AI analysis. The synergy of these technologies is demonstrated through case studies in cancer diagnosis, predictive analytics in myelofibrosis, and other areas, underscoring their potential to revolutionize healthcare and improve patient outcomes. The future of biomedical research lies in the continued integration of AI and Big Data, promising further advancements and improved quality of care.
Keywords: Artificial Intelligence, Big Data, Biomedical Research, Machine Learning, Deep Learning, Disease Diagnosis, Personalized Medicine, Drug Discovery, Genomic Technologies, Predictive Analytics
Suggested Citation: Suggested Citation
Dimitrios Sargiotis (Contact Author)
National technical university of athens - department of transportation planning and engineering ( email ), do you have a job opening that you would like to promote on ssrn, paper statistics, related ejournals, applied computing ejournal.
Subscribe to this fee journal for more curated articles on this topic
Information Systems eJournal
Computation theory ejournal, biotechnology ejournal, computing methodology ejournal, computational biology ejournal.
Stack Exchange Network
Stack Exchange network consists of 183 Q&A communities including Stack Overflow , the largest, most trusted online community for developers to learn, share their knowledge, and build their careers.
Q&A for work
Connect and share knowledge within a single location that is structured and easy to search.
How to find papers having datasets?
It is becoming common for authors to upload raw data of their research when publishing their papers. However, it is still a small fraction of papers include the dataset.
Is there a way to search for papers whose data are available in repositories?
My field is computational chemistry, and I hope to find papers which have posted the raw data for the DFT analysis.
- literature-search
- You should probabably cross-post this question at opendata.stackexchange.com . You might get some helpful responses from there. – Tripartio Commented Apr 27, 2018 at 9:09
- 1 What is "DFT analysis"? Please spell it out so that more people might be able to help you. – Tripartio Commented Apr 27, 2018 at 9:14
- There are a number of such databases ( PubChemQC comes to mind), but you need to be more specific about what data you're interested in, then you can worry about searching for papers that report databases/repositories. – pentavalentcarbon Commented May 13, 2018 at 15:16
3 Answers 3
Do you know if there exists a database for the type of data you are interested in? That would be the easiest way: browse the database until you find an interesting data set, and the paper will likely be referenced by the database entry (if it is a serious database).
Here is an analogy with my own field (structural biology): most (if not all) journals require that structural models of biological molecules be deposited in the PDB in order to publish the article describing the structure. Only the model used to be deposited, but nowadays researchers are also encouraged to deposit the data that led to the model. Therefore, if I am looking for the article describing a particular structure, it is often easier to look up the molecule in the PDB, possibly choose between different entries (one of they may have associated data, not only the model) and find the paper from there.
What you are looking for is probably DataCite search: https://search.datacite.org/works . If your dataset of interest has a DOI, you should be able to find it that way. You can search by keyword and DFT should bring up about 72,000 datasets. Those datasets may or may not be linked to publications.
Though it will not be useful for your work, for those that might be interested to find biological datasets I have an additional tip. I work for a life science literature database (Europe PMC), and we link publications and data. We identify data citations (in the form of DOI or citation numbers) in the text and this information is searchable. You can either add (HAS_DATA:y) to your search to identify all papers that reference data, or specify the data type (e.g. PDB accession number, or clinical trial reference) using the advanced search. Here is one example for papers on cancer that reference protein structural data: https://europepmc.org/search?query=Cancer%20AND%20(ACCESSION_TYPE%3Apdb)
I suggest you directly looking at theses/dissertations databases. They may not put the data in the article but their theses most probably include them in the appendix.
Not a direct way to filter only data including reports, yet it will really increase the likelihood of finding data for you. Proquest is an example of such theses/dissertations search engine.
You must log in to answer this question.
Not the answer you're looking for browse other questions tagged data literature-search chemistry ..
- Featured on Meta
- We spent a sprint addressing your requests — here’s how it went
- Upcoming initiatives on Stack Overflow and across the Stack Exchange network...
Hot Network Questions
- Can you arrange 25 whole numbers (not necessarily all different) so that the sum of any three successive terms is even but the sum of all 25 is odd?
- Who originated the idea that the purpose of government is to protect its citizens?
- Book that I read around 1975, where the main character is a retired space pilot hired to steal an object from a lab called Menlo Park
- Hölder continuity in time of heat semigroup for regular initial distribution
- Line from Song KÄMPFERHERZ
- Why does Paul's fight with Feyd-Rautha take so long?
- Book in 90's (?) about rewriting your own genetic code
- Test whether a string is in a list
- What does '\($*\)' mean in sed regular expression in a makefile?
- Does the Ogre-Faced Spider regenerate part of its eyes daily?
- Unsorted Intersection
- Imagining Graham's number in your head collapses your head to a black hole
- Is arXiv strictly for new stuff?
- How far back in time have historians estimated the rate of economic growth and the economic power of various empires?
- In equation (3) from lecture 7 in Leonard Susskind’s ‘Classical Mechanics’, should the derivatives be partial?
- Wait a minute, this *is* 1 across!
- Are there examples of triple entendres in English?
- In the UK, how do scientists address each other?
- Should "as a ..." and "unlike ..." clauses refer to the subject?
- How to maintain dependencies shared among microservices?
- Geometry question about a six-pack of beer
- What does a letter "R" means in a helipad?
- What does it mean if Deutsche Bahn say that a train is cancelled between two instances of the same stop?
- Why does the Trump immunity decision further delay the trial?

Data Science Journal
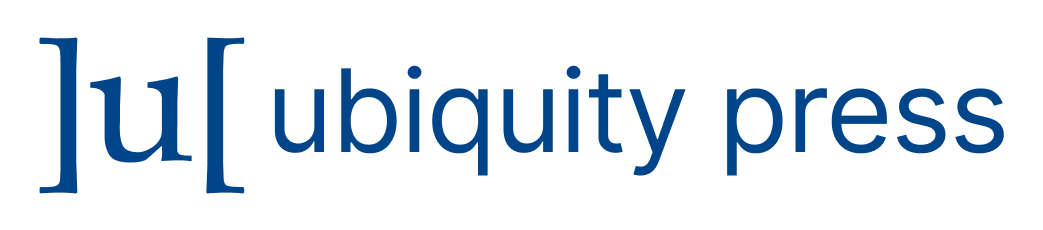
- Download PDF (English) XML (English)
- Alt. Display
Research Papers
Are researchers citing their data a case study from the u.s. geological survey.
- Grace C. Donovan
- Madison L. Langseth
Data citation promotes accessibility and discoverability of data through measures carried out by researchers, publishers, repositories, and the scientific community. This paper examines how a data citation workflow has been implemented by the U.S. Geological Survey (USGS) by evaluating publication and data linkages. Two different methods were used to identify data citations: examining publication structural metadata and examining the full text of the publication. A growing number of USGS researchers are complying with publisher data sharing policies aimed to capture data citation information in a standardized way within associated publications. However, inconsistencies in how data citation information is documented in publications has limited the accessibility and discoverability of the data. This paper demonstrates how organizational evaluations of publication and data linkages can be used to identify obstacles in advancing data citation efforts and improve data citation workflows.
- Data citation
- structural metadata
- data linkages
- data sharing
Introduction
Data citations promote increased transparency and credit attribution for published data ( ESIP Data Preservation and Stewardship Committee 2019 ; Parks et al. 2018 ; Zhao et al. 2017 , Huang et al. 2015 ). These citations incorporate several components: author name, publication year, data release title, version number (if applicable), publisher name, and a digital object identifier (DOI) ( USGS Data Management 2022 ). Similar to citations for published manuscripts, data citations ensure that contributors receive credit for their work ( Mooney 2011 ) and allow contributors to track the impact of their data. Additionally, data citations enable the use and reuse of data by providing users with information to identify and access data ( Lafia et al. 2023 ). Digital Object Identifiers (DOIs) assigned to data products are a primary means of tracking publication and data linkages ( Zhao et al. 2017 ; Belter 2014 ). DOIs for data products also act as a ‘standard mechanism for retrieval of metadata about the object’ ( Wilkinson et al. 2016 ).
Groups are working to promote data citation in research through community engagement. For example, Make Data Count is a global, community-led initiative, focused on incentivizing data sharing by developing ‘open research data assessment metrics’ ( Make Data Count 2022 ). Two contributing organizations to Make Data Count are DataCite and Crossref. DataCite is a DOI and metadata registration organization focusing primarily on research data ( DataCite 2022 ). Similarly, Crossref is a DOI and metadata registration organization focusing primarily on manuscripts and reports ( Wilkinson 2022 ). Together, these organizations ensure the accessibility and discoverability of data and associated research artifacts through their partnership in linking publications registered with Crossref to data DOIs ( Lin 2016 ).
Make Data Count ( 2022 ) outlines the ideal data citation workflow as follows:
- Researchers include data citation in their publications according to journal data policies.
- Publishers send data citation to Crossref as part of the publications’ DOI metadata.
- Repositories send publication references to DataCite as part of the datasets’ DOI metadata.
- Crossref and DataCite share DOI metadata with the research community through Application Programming Interfaces (APIs), such as Event Data ( Rittman 2020 ).
- Research community can access metrics related to links between datasets and publications using the Crossref and DataCite APIs.
DOI metadata is the foundation of the Make Data Count Initiative and data citation workflows. Crossref and DataCite document information about their DOIs in structural metadata. Structural metadata is machine-readable information that outlines the ‘structure, type, and relationships of data’ ( Melton & Buxton 2006 ). While the infrastructure to support data citation is in place, variations in data citation practices have introduced complexities into data citation tracking ( Gregory et al. 2023 ). Organizations like Crossref and DataCite, as well as some publishers, encourage researchers to include data citations within reference lists through data citation policies ( Gregory et al. 2023 ; Farley 2022 ). However, several studies demonstrate that researchers continue to cite data in ‘informal’ ways (i.e., the data is mentioned within the full text of publications) that may not be included in publication structural metadata ( Parks et al. 2018 ; Zhao et al. 2017 ; Belter 2014 ). Parks et al. ( 2018 ), Zhao et al. ( 2017 ), and Lafia et al. ( 2023 ) found that several inconsistencies in how researchers cite data were due to a lack of understanding regarding how to cite data and the importance and implications of citing data. However, researchers are not solely responsible for creating consistent data citations. Publishers also have a large role to play in data citation. For example, even though publishers are responsible for submitting reference lists to Crossref, some publishers may not have developed workflows necessary to include reference lists in the Crossref structural metadata. Deviations from the ideal data citation workflow ultimately impede our ‘ability to consistently analyze, detect, and quantify data citations’ ( Irrera et al. 2023 ) through structural data analysis methods.
While it may be impossible to assess whether data citations are missing from a corpus of works using these methods alone, it may be possible to gauge uptake of data citations within a smaller research community using additional methods like text and data mining. Previous studies have demonstrated the efficacy of text and data mining techniques in identifying data citations within the full text of publications ( Kafkas et al. 2013 ; Parks et al. 2018 ; Parsons et al. 2019 ). In this analysis, we leverage two text and data mining tools, Publink and xDD, to identify data citations that may not be present in structural metadata records. Publink is a Python package that allows users to find relationships between publications and data ( Wieferich et al. 2020 ). In cases where references are not included in the publication’s DOI structural metadata, Publink can be used to see if researchers are referencing their data by searching for mentions of data DOIs in the full text of publications included in the eXtract Dark Data (xDD) digital library. xDD, formerly known as GeoDeepDive, is a cyberinfrastructure that compiles data on published literature and provides users with the ability to perform full text searches of published literature using the xDD API ( Peters et al. 2021a ). As of 2021, xDD contained over 14 million commercial and open access publications of scientific works. While xDD initially compiled Earth science publications, it currently aims to be discipline agnostic.
In this analysis, publications authored by U.S. Geological Survey (USGS) researchers were evaluated to determine the presence of data citations. The USGS is a research agency that provides science about natural hazards, natural resources, ecosystems and environmental health, and the effects of climate and land-use change ( USGS 2022 ). USGS research is disseminated through various types of publications, including USGS-authored journal articles through external publishers and series reports published by the USGS ( USGS OSQI 2021c ). An agreement between USGS and xDD has enabled xDD to index USGS series reports ( Peters et al. 2021b ). Publink and xDD are ideal tools for examining data mentioned within the full text of USGS series reports as well as USGS-authored publications indexed in xDD. Additionally, USGS researchers, through an instructional memorandum, were encouraged to publicly release data associated with their scholarly publications as of 2015 ( USGS OSQI 2017 ). This instructional memorandum became policy and went into full effect in 2016 ( USGS OSQI 2016 ). USGS policy requires that these data be assigned a DOI, be accompanied by a citation, and be referenced from the associated publication ( USGS OSQI 2017 ). When USGS researchers acquire a DOI for their data through the USGS DOI Tool, they are asked to provide the DOI for the associated publication. The data DOI structural metadata offers access to a corpus of publications that should include data citations in some form (i.e., within the structural metadata or the full text). Considering these factors, the USGS presents a unique case study to evaluate the current state of data citations within a subset of the scientific research community. Our analysis shows how combined data citation tracking methods can be used to evaluate the extent to which researchers, publishers, and repositories have adhered to the ideal data citation workflow. This evaluation can help identify areas for improvement in data discoverability and accessibility.
Metrics on data citations in publications produced by USGS authors were collected and analyzed using the USGS data DOI database (USGS DOI Tool), xDD, and the Crossref Application Programming Interface (API) in Jupyter Notebooks. These data were used to create a baseline analysis of how often researchers have cited the associated data in publications. Publications released from 2016 through 2022 were included in the collection. Publications released prior to 2016 were not included in the collection on account of the USGS instructional memorandum ( USGS OSQI 2016 ) that became policy and went into full effect in 2016. Using the USGS DOI Tool API, we created an initial dataset by extracting data DOIs whose metadata included a related primary publication DOI. Additional related primary publication DOIs were identified through quality checks that captured incorrectly formatted DOIs (e.g., related primary publication DOIs not being stored in the DOI URL format) or placeholder DOIs (e.g., https://doi.org/10.xxxxxxx.xxxxxx) ( Donovan & Langseth 2024 ). In total, there were 2,772 publications included in the analysis dataset. Links from a data DOI to a related primary publication are manually supplied by data authors in the USGS DOI Tool and are not required. Additionally, not all USGS publications use newly generated data to support their conclusions, which means that their authors are not minting USGS DOIs for data referenced in the publication. Therefore, the related primary publications included in the analysis dataset represent only a subset (around 16%) of all USGS publications (17,841) between 2016 and 2022. 1
First, we checked if a formal data citation was present in the publication’s Crossref structural metadata. We obtained the article title, publication year, and publisher, using the habanero Python library ( Chamberlain et al. 2022 ), based on the primary publication DOI. We also documented whether the Crossref structural metadata contained references. If references were included, the ‘reference-count’ value in the Crossref structural metadata was greater than zero and the publication was recorded as having references ( Figure 1 ). For cases where the ‘reference count’ value was greater than zero, the publication was recorded as citing the data DOI if the associated data DOI was included in the ‘doi’ element of a reference in the Crossref structural metadata ( Figure 2 ) ( Donovan & Langseth 2024 ). Only publications with references in the Crossref structural metadata could be definitively recorded as citing the data DOI. For example, a publication could have a human-readable references section that included a data citation with a data DOI; however, for the purposes of this study, if the data DOI was not included in the ‘doi’ element of a reference in the Crossref structural metadata, then the data DOI would not be found using this method and would not count as a cited data DOI.
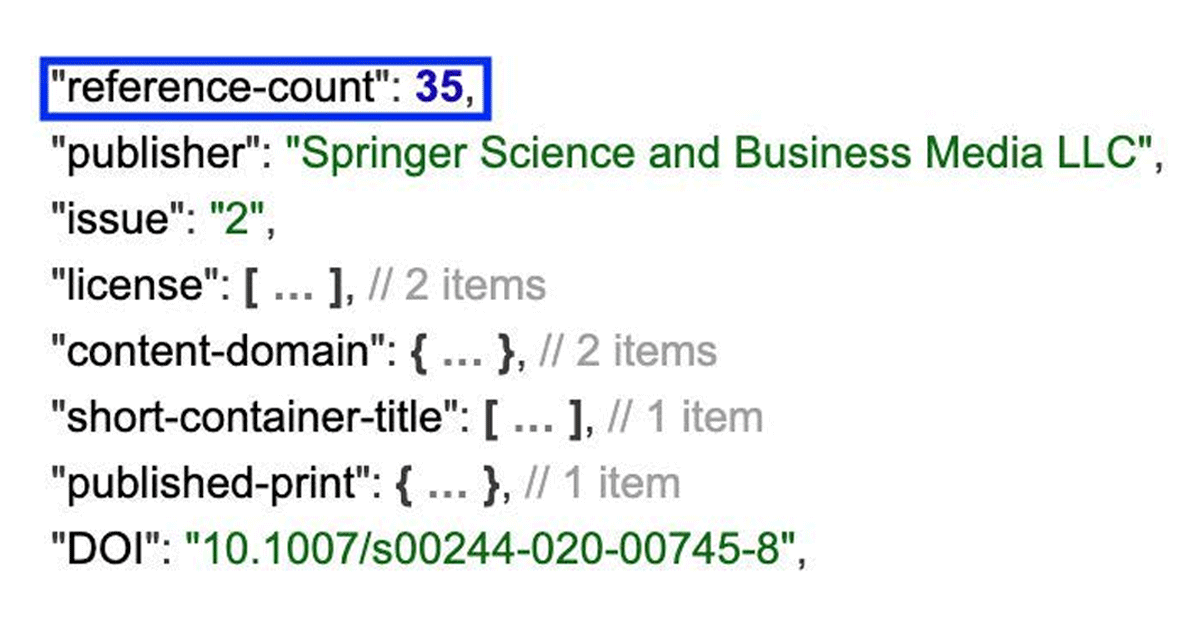
Crossref API call ( https://api.Crossref.org/works/10.1007/s00244-020-00745-8 ) indicating Crossref structural metadata contains references.
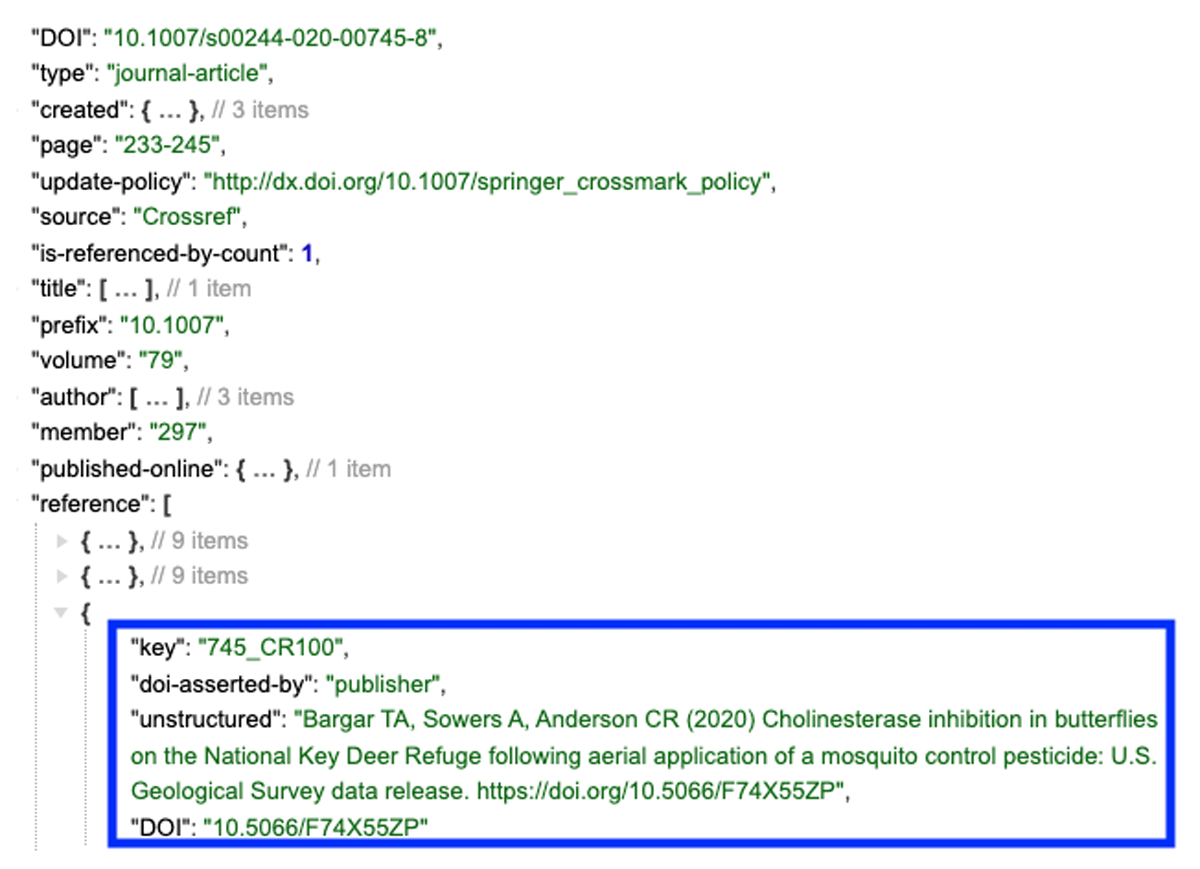
Crossref API call ( https://api.Crossref.org/works/10.1007/s00244-020-00745-8 ) indicating the data DOI is listed in the ‘doi’ element in the reference of the Crossref structural metadata.
Second, we checked if there was a data citation in the full text of the publication, rather than in the publication’s structural metadata. For publications with full text available in xDD (49% of the full publication list), the presence of a data DOI mentioned anywhere in the full text was identified using the Publink python package, built on top of the xDD API ( Wieferich et al. 2020 ; Donovan & Langseth 2024 ).
Information on Crossref references and data DOIs captured within the Crossref references was used to create three subsets to analyze the data between 2016 and 2022 ( Figure 3 ):
- Publications with Crossref references that contained data DOIs
- Publications with Crossref references that did not contain data DOIs
- Publications without Crossref references
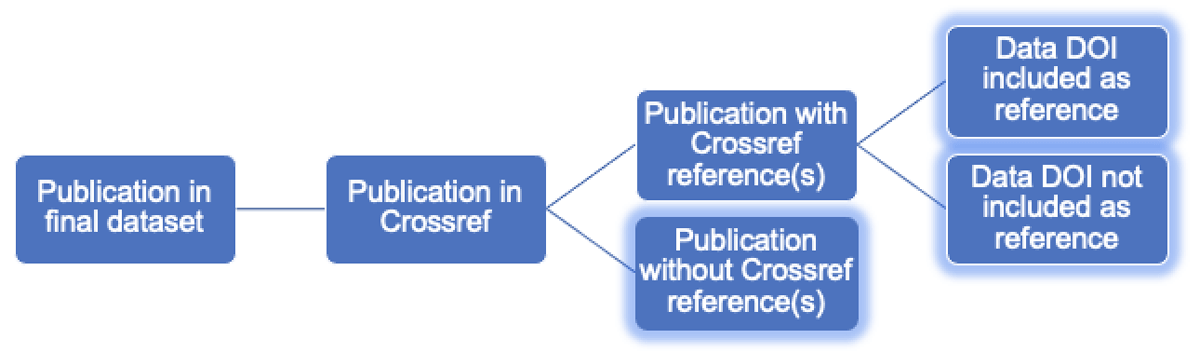
Overview of Crossref analysis method demonstrating how publications were subset and data DOIs were identified in Crossref structural metadata.
Binomial Generalized Linear Models (GLMs) were used to examine trends in the proportion of publications with data DOIs captured in the Crossref reference(s) of their associated publications between 2016 and 2022.
Similarly, information on publications in xDD and data DOIs mentioned within the full text of the publications was used to subset the data into three categories for analysis between 2016 and 2022 ( Figure 4 ):
- Publications in xDD that mentioned the data DOI
- Publications in xDD that did not mention the data DOI
- Publications that were not in xDD
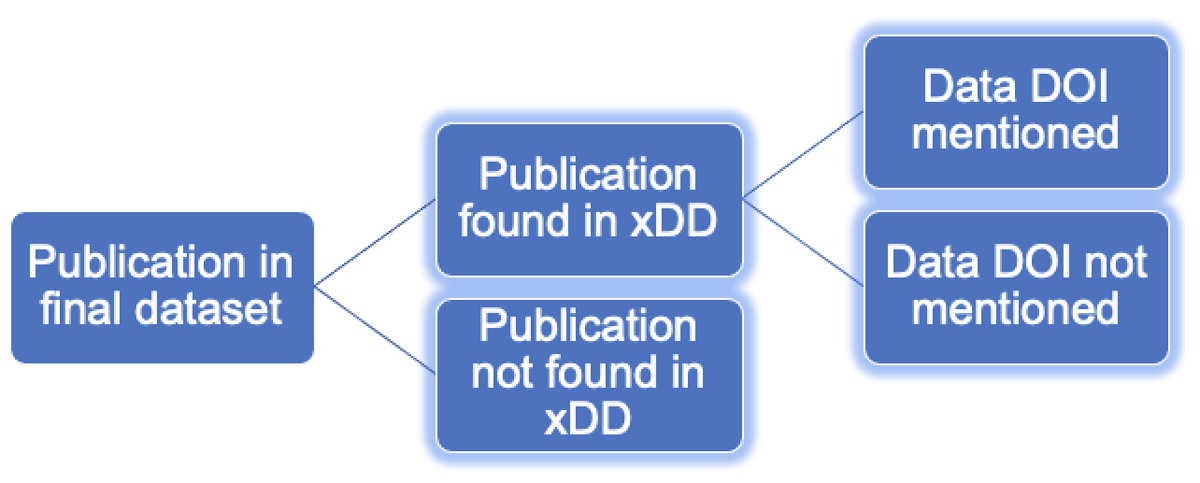
Overview of the xDD analysis method demonstrating how publications were subset and data DOIs mentions were identified in the full text of publications indexed in xDD.
Binomial GLMs were used to examine trends in the number of publications with data DOIs mentioned in publications found in xDD between 2016 and 2022.
We examined differences in data citations for different publishers to understand how different publisher data policies may have contributed to data access and data citation efforts. Web searches were also performed to assess publishers’ publicly documented data policies.
Crossref References
Fifty-three percent of the publications in the analysis dataset included references in their Crossref structural metadata, whereas 47% of the publications did not include references. The lack of references in the publication structural metadata does not necessarily imply that a given publication is devoid of references in its full text. However, missing references from structural metadata may point to an obstacle with the implementation of the ideal data citation workflow. The percentage of publications with indexed Crossref reference(s) fluctuated between 2016 and 2022 ( Figure 5 ). However, this did not represent a statistically significant trend (p = 0.41).
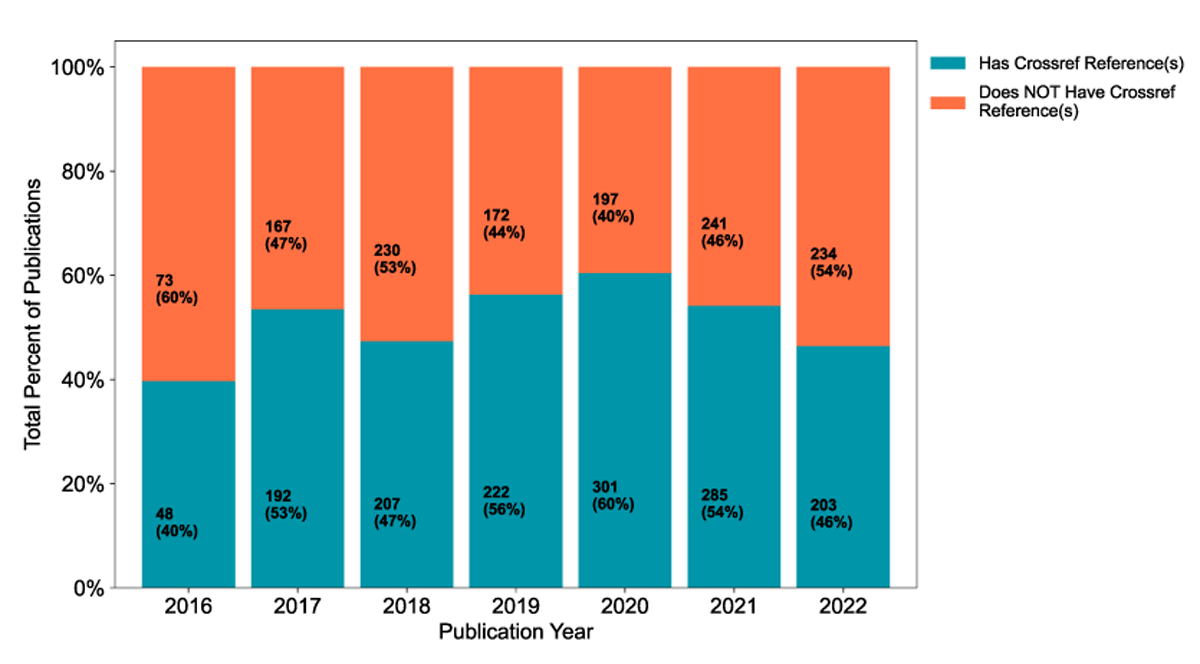
Percentage of publications with indexed Crossref reference(s) in their Crossref structural metadata by publication year.
Two hundred and thirty-nine publications included data DOIs within the Crossref references, which accounted for 9% of publications in the analysis dataset and 16% of publications with references included in the Crossref structural metadata ( Figure 6 ). The percentage of publications with data DOIs included in the Crossref structural metadata’s references grew between 2016 and 2022 from 4% to 30%, representing a statistically significant trend (p < 0.001) ( Figure 6 ).
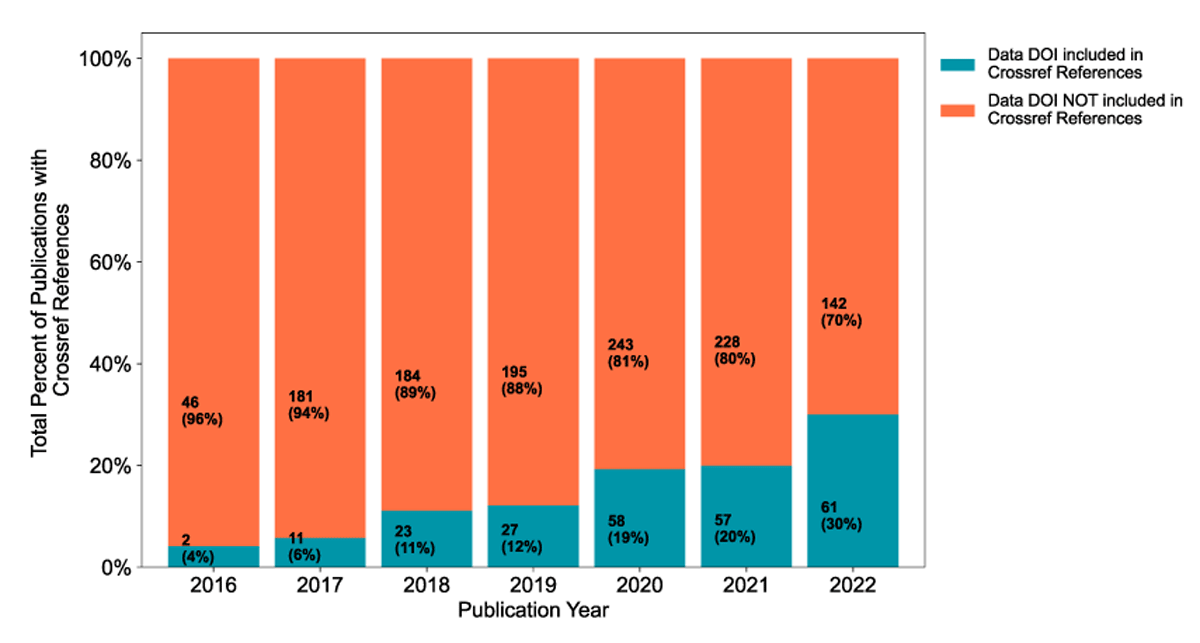
Percentage of publications with indexed Crossref references that cite or do not cite their associated data DOI in their Crossref structural metadata by publication year.
xDD Mentions
Forty-nine percent of the publications included in the analysis dataset had their full text indexed in xDD ( Figure 7 ). Over three quarters of the publications with full text indexed in xDD (77%) mentioned their data DOI ( Figure 7 ).
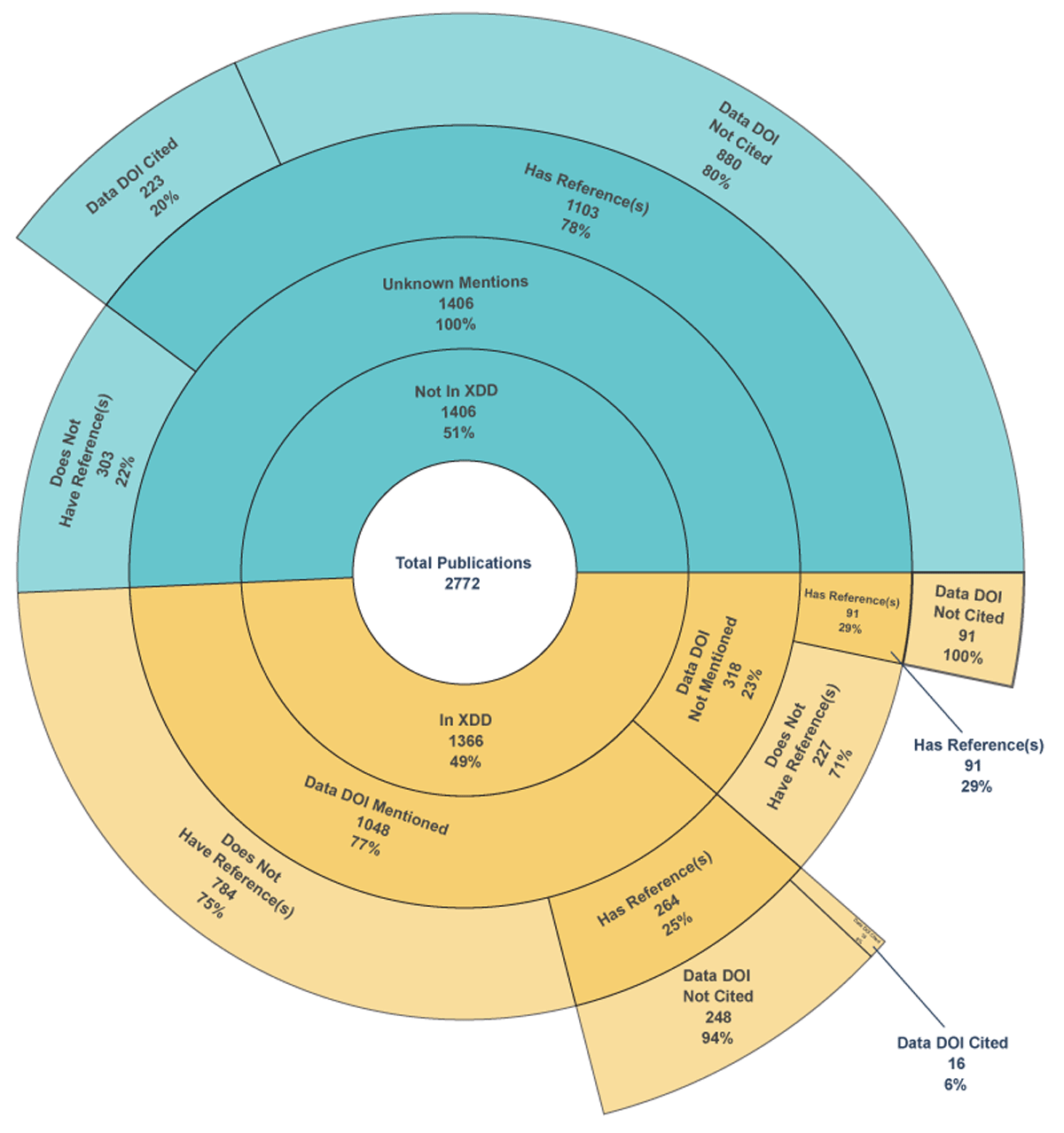
Publications subset by Crossref and xDD analysis method results, demonstrating the percentage of publications that mention a data DOI in their full text and/or cite a data DOI in their Crossref structural metadata references.
Between 2016 and 2022, there was an overall increase in the number of publications mentioning their data DOIs (from 63% to 82%); however, there was no statistically significant trend in the increase in number of publications per year within this period (p = 0.53) ( Figure 8 ).
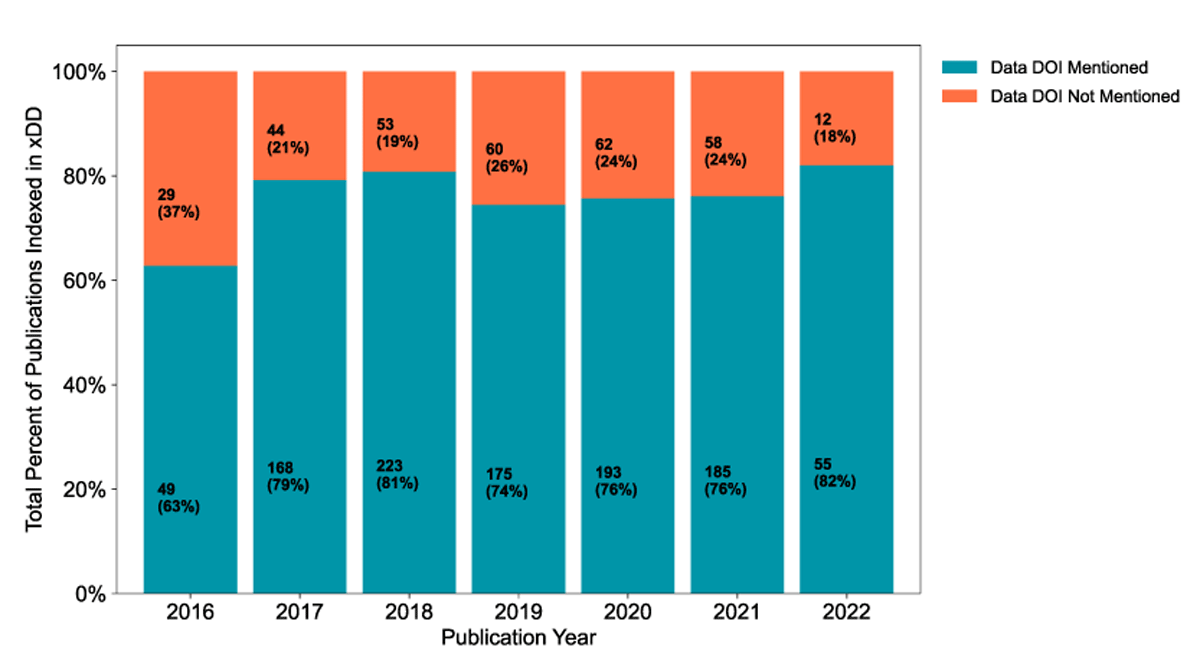
Percentage of publications with full text indexed in xDD with and without data DOI mentioned by publication year.
Effect of Publisher Data Policy
Fifty-eight different publishers released the 2,772 publications included in the analysis dataset. Eight out of the 58 publishers have the full text of their publications indexed in xDD. The proportion of publications found in xDD that mentioned a data DOI were analyzed by these publishers ( Figure 9 ).
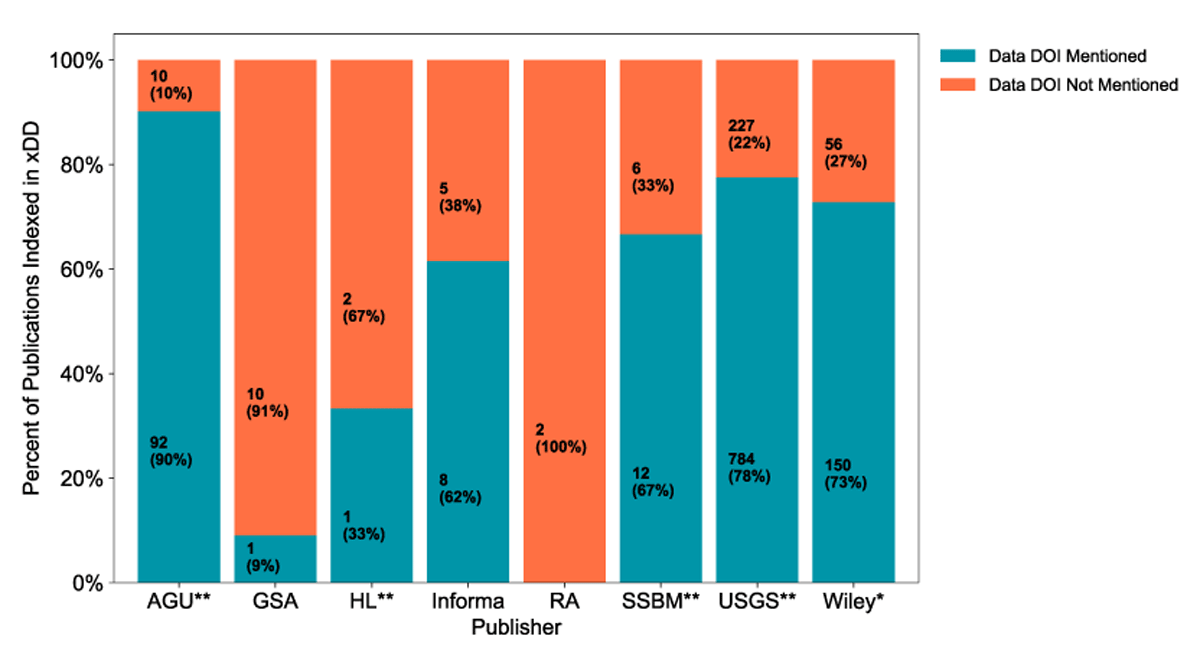
Percentages of publications with full text indexed in xDD that mention or do not mention their associated data DOI (see publisher abbreviations table above for publisher names). **Indicates publishers with data policies encouraging either a data availability statement or data citations in their reference lists.
The top 10 publishers in this analysis published over 90% of the publications in the analysis dataset. The data availability policy for each of the top 10 publishers and all publishers with their full text indexed in xDD was analyzed ( Table 1 ).
Information on data policies for top 10 publishers of publications in analysis dataset and publishers with full text indexed in xDD. *For publishers with different data availability policy levels, the most lenient policy level is documented.
PUBLISHER | NUMBER OF PUBLICATIONS IN ANALYSIS DATASET | DATA AVAILABILITY STATEMENTS | DATA CITATIONS IN REFERENCES LIST | LINK TO POLICY |
---|---|---|---|---|
Regional Euro-Asian Biological Invasions Centre Oy (REABIC) | 29 | Not Mentioned | Not Mentioned | None Found |
Oxford University Press (OUP) | 34 | Not Mentioned* | Not Mentioned | |
Frontiers Media SA | 49 | Required | Required | |
American Chemical Society (ACS) | 58 | Encouraged* | Encouraged* | |
Public Library of Science (PLoS) | 69 | Required | Encouraged | |
MDPI | 132 | Required | Not Mentioned | |
American Geophysical Union (AGU) | 135 | Required | Required | |
Springer Science and Business Media LLC (SSBM) | 234 | Required | Not Mentioned | |
Wiley | 521 | Encouraged* | Encouraged* | |
U.S. Geological Survey (USGS) | 1237 | Not Mentioned | Required | |
The sample size by publishers varied greatly, with some having an extremely small number of publications in xDD. It may be possible to discern the significance of publisher data policies requiring or encouraging data availability statements or data citation and their impact on whether data DOIs are mentioned within the full text of publications for the publishers with smaller numbers of publications in xDD within the analysis dataset by contacting individual publishers directly. Yet, based on the criteria selected and the methodology used, it was not possible to link the data policies to the results in this analysis for the publishers with small sample sizes of publications in xDD. However, publishers with larger sample sizes (i.e., AGU, USGS, Wiley) in the analysis dataset, all had some version of data policy ( Table 1 ), and more than 70% of their publications mentioned data DOIs.
Eight of the top ten publishers included references in their Crossref structural metadata ( Table 2 ). The analysis showed that the USGS and Regional Euro-Asian Biological Invasions Centre did not send references to Crossref between 2016 and 2022. Out of all the publishers, 18 (31%) have not sent any references to Crossref, seven (12%) have sent some references, and 33 (57%) have sent references for all of their publications.
The number of publications with and without indexed references for each of the top 10 publishers.
PUBLISHER | PUBLICATIONS WITH INDEXED REFERENCES | PUBLICATIONS WITHOUT INDEXED REFERENCES |
---|---|---|
American Chemical Society (ACS) | 58 | 0 |
American Geophysical Union (AGU) | 135 | 0 |
Frontiers Media SA | 49 | 0 |
MDPI | 131 | 1 |
Oxford University Press (OUP) | 34 | 0 |
Public Library of Science (PLoS) | 69 | 0 |
Regional Euro-Asian Biological Invasions Centre Oy (REABIC) | 0 | 29 |
Springer Science and Business Media LLC (SSBM) | 234 | 0 |
U.S. Geological Survey (USGS) | 0 | 1,236 |
Wiley | 517 | 4 |
Numerous publications released by the top 10 publishers that contained references within the Crossref structural metadata did not include data DOIs within the ‘doi’ element ( Figure 10 ). Publishers that require or encourage data citations in the reference section of their publications through data policies had a lower proportion of publications with data DOIs in their Crossref structural metadata (e.g., American Geophysical Union (AGU) and Wiley) compared to publishers that do not require or encourage data citations in the reference section of their publications (e.g., MDPI and Springer Science and Business Media LLC (SSBM)). The results also indicate that SSBM (45%) and MDPI AG (41%) released the largest percentage of publications with data DOIs included as references within the Crossref structural metadata. Missing data DOIs from the ‘doi’ element in Crossref structural metadata did not necessarily mean that a reference to the data was not made in the references section of the paper or as unstructured text in the Crossref structural metadata. Publishers with publications within the analysis dataset included data references in the Crossref structural metadata in various ways:
- Data DOI listed along with all citation fields (e.g., title, authors) in ‘unstructured’ element in Crossref references
- Data reference included in Crossref references without the DOI
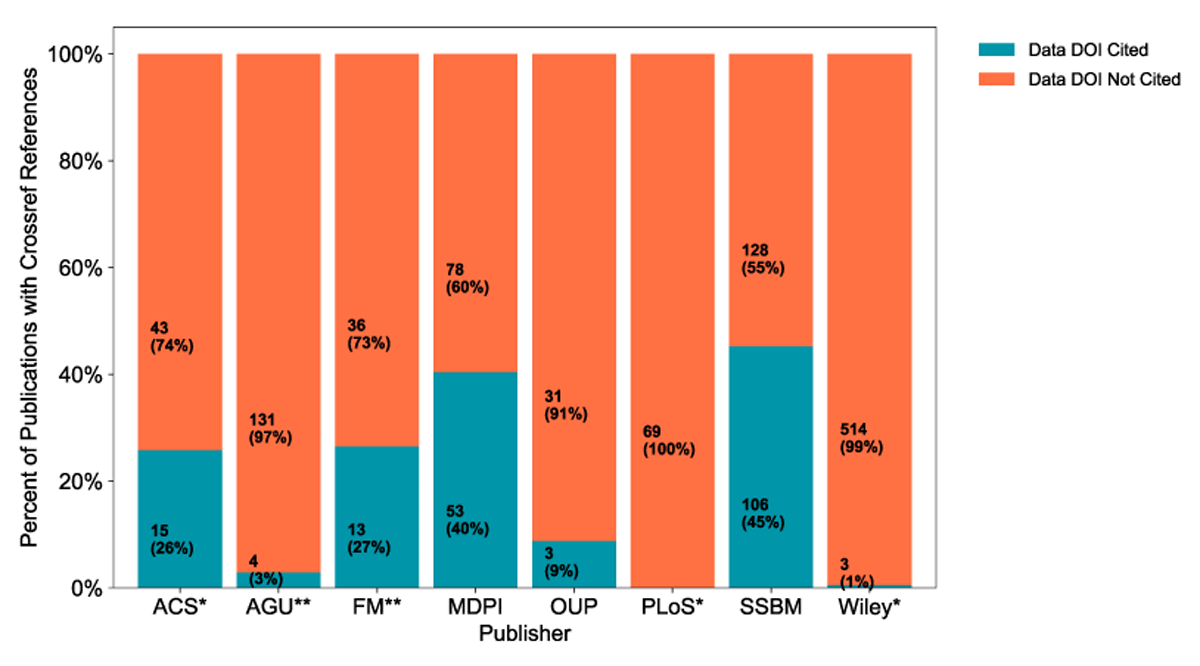
Percentages of publications with data DOIs cited and not cited in the publication’s Crossref structural metadata for the eight out of the top ten publishers with Crossref references (see publisher abbreviations table above for publisher names). **Indicates publishers with data policies requiring data citations in their reference lists. *Indicates publishers with data policies encouraging data citations in their reference lists.
This assessment of data DOI mentions and citations within scholarly works and associated Crossref structural metadata provides insight into the implementation of the ideal data citation workflow for USGS authored publications. With over 2,000 publications analyzed, the analysis dataset provided a sample of USGS scholarly works between 2016 and 2022 expected to have data citations for known USGS data DOIs. This analysis revealed that not all USGS researchers have included a DOI for data within the references of their publications. However, a considerable portion of USGS researchers (77%) have included data DOIs in their publications, at least for the publications that were indexed in xDD ( Figure 7 ). These data DOI mentions could be found anywhere within the publication, not only in the reference list. Given current methods using Crossref and DataCite structural metadata to track citations, it was difficult to assess how the data DOIs were being referenced within publications (within the reference list, a data availability statement, or within the body of the publication). Despite a high percentage (77% of publications in xDD) of data DOI mentions ( Figure 8 ), there is still work, such as policy updates, outreach campaigns, and adoption of consistent reference sharing methods, that could be done to ensure that USGS researchers are meeting USGS policy requiring that publications reference their data ( USGS OSQI 2017 ; USGS OSQI 2021a ; USGS OSQI 2021b ).
Many research institutions such as government agencies and universities have embraced the movement toward scientific reproducibility and transparency ( Kretser et al. 2019 ), prompting publishers to ‘adapt their workflows to enable data citation practices and provide tools and guidelines that improve the implementation process for authors and editors, and relieve stress points around compliance’ ( Cousijn et al. 2018 ). The addition of USGS Survey Manual Chapter 1100.2 ( USGS OSQI 2021b ; USGS OSQI 2021a ) aims to support researchers through the implementation of procedures to verify data are cited in USGS series publications during the editorial review process. Hardwicke et al. (2018), suggest that this type of implementation of dedicated staff and resources geared towards assessing data citations, has the potential to improve policy compliance and ensure that data are cited properly. Given that the USGS Survey Manual Chapter 1100.2 was released in 2021, future analysis could determine if the Survey Manual is helping to increase the number USGS data citations. Regardless of this undertaking by the USGS or similar efforts among research organizations, other publishers of scientific content may not incorporate this step in their editorial process. Without this level of assistance, researchers are solely responsible for ensuring that any associated data are cited properly. As Belter ( 2014 ) suggests, publishers that are not already working with researchers to ensure proper citation of data in their publications may consider becoming involved in this process to support data sharing.
Data citation outreach campaigns within organizations, such as the USGS, could be used to inform researchers about the importance and benefits of including data citations in their works, as well as how to include references to their data to maximize citation tracking efforts. Many publishers are making strides to promote the ideal data citation workflow by informing researchers about their responsibilities related to providing access to and citing their data ( Table 1 ). Although our results do not definitively link publisher data citation policies to an increase in the occurrence of data citations in their publications, other studies ( Colavizza et al. 2020 ) suggest this type of impact from such policies. Publishers also play a large role in ensuring that any data that researchers cite in their publications get included in the structural metadata sent to Crossref. As part of the ideal data citation workflow, publishers are strongly encouraged to send data citations to Crossref as part of their publications’ structural metadata references. Publishers are responsible for maintaining structural metadata, which supply key information about publication and data relationships ( Wilkinson 2022 ; Mooney 2011 ) and offer a means of programmatically tracking these relationships. Most publishers in this analysis (69%) are sending references to Crossref for all or some of their publications. Yet, there is a notable percentage of publications that did not include reference(s) in their Crossref structural metadata between 2016 and 2022 ( Figure 5 ). These missing references suggest a breakdown in step two of the ideal data citation workflow, where publishers may not be including references in the publication DOI metadata that they send to Crossref. USGS, which is the publisher that makes up 45% of publications included in the analysis dataset, does not send any references to Crossref. The authors of this paper are working with the USGS Library and USGS SPN to develop a workflow for sending references to Crossref.
Despite these data policies and the fact that some of these publishers are sending references to Crossref, this does not necessarily translate to data DOIs appearing in the Crossref references in a consistent manner (within the ‘doi’ element). Crossref encourages publishers to use the ‘doi’ element whenever possible for more precise linking ( Farley 2022 ). However, Crossref also states that data and software references can be included in the ‘unstructured_citation’ element. This approach is likely much easier for publishers to achieve, instead of parsing data and software citations in individual elements, which may be different than the process for parsing their citations for publications. However, using the ‘unstructured_citation’ element is less useful for data citation tracking efforts such as this analysis because the content within the element is not structured and may not always contain the data DOI. Cases where certain elements from the data citation were included (e.g., ‘title’) but the data DOI was excluded, were also identified. This approach is less useful for data citation tracking efforts because there is no way to find the data DOI using the Crossref metadata. AGU staff recently uncovered some issues in data citation workflows that may be partially responsible for many Crossref references not listing the data DOI in the ‘doi’ element (S. Stall, personal communication, July 19, 2023). They have published a preprint describing the steps publishers need to take to improve their workflows ( Stall et al. 2022 ). Until publisher workflows are aligned with this new guidance, and for cases where the data DOI is either not captured or not easily parsed, data citation tracking efforts can be supplemented by using workflows involving literature databases such as xDD and associated tools like Publink.
xDD allows users to discover relationships between publications and data that may not be captured in the Crossref and DataCite structural metadata ( Wieferich et al. 2020 ). Although only half of the publications in the total dataset were in xDD ( Figure 7 ), more mentions of data DOIs were found through the xDD method than through the Crossref method. Using xDD, 38% of all publications in the dataset were identified as having mentioned the data DOI. Whereas, using the Crossref methods, only 9% of publications were identified with links to the data DOIs. By combining the Crossref and xDD methods, links to the data DOIs in 1,271 publications (46% of the analysis dataset) were identified. While the most ideal approach to finding connections between data and publications would be through DataCite and Crossref structural metadata, it may take time for smaller publishers, such as USGS, to develop workflows to document and maintain this information. xDD can be used to discover data citation information in publications where these connections are missing in the DataCite and Crossref structural metadata. xDD also provides the means to retroactively add information about data and publication linkages to DataCite structural metadata through tools like Publink ( Wieferich et al. 2020 ). Although xDD may not contain an all-inclusive library of all publications, it can be used in tandem with structural metadata infrastructures to inform users about relationships between publications and associated data. Advancements in these tools and infrastructures could promote more in-depth analysis of data citation practices and be used to identify gaps more clearly in resources or opportunities for data citation training.
Data accessibility is fundamental to the transparency and integrity of published research. Without clear linkages between publications and their associated data, data may be inaccessible, stifling data sharing and the reproducibility of scientific findings. Incorporation of data citations in publications allow users access to data while ensuring that researchers can track the impact of their data and receive credit for their work. The roles defined in the Make Data Count Initiative’s ideal data citation workflow describe how researchers, publishers, repositories, and the scientific community can take steps to ensure data and publications are linked through data citations. Although the results of this analysis indicate that portions of the ideal citation workflow are being implemented within this subset of the scientific community, improvements can be made to fully satisfy the objective of the ideal data citation workflow. For instance, it would be beneficial to continue to encourage USGS researchers to follow publisher data-sharing policies and for publishers to consider adopting consistent reference-sharing methods with repositories. As the scientific community continues to improve data and publication linkages, coupled data citation tracking methods can offer information to further refine implementations of the ideal data citation workflow.
Data Accessibility Statement
Data used to support conclusions in this study about data DOI mentions and citations within USGS authored publications are available at: Donovan, G.C., & Langseth, M.L., 2024, U.S. Geological Survey Data Citation Analysis, 2016–2022: U.S. Geological Survey data release, https://doi.org/10.5066/P9CPC9M2 .
Total USGS publication count retrieved from the USGS Publications Warehouse, which catalogs all USGS series publications and articles published through external journals. https://pubs.er.usgs.gov/search?q=&startYear=2016&endYear=2022&subtypeName=Journal+Article&subtypeName=USGS+Numbered+Series&subtypeName=USGS+Unnumbered+Series .
Abbreviations
TERM | ABBREVIATION |
---|---|
White House Office of Science and Technology Policy | OSTP |
Office of Management and Budget | OMB |
Office of Science Quality and Integrity | OSQI |
U.S. Geological Survey | USGS |
Trusted Digital Repository | TDR |
Digital Object Identifier | DOI |
USGS Fundamental Science Practices | FSP |
Joint Declaration of Data Citation Principles | JDDCP |
eXtract Dark Data | xDD |
Application Programming Interface | API |
Publisher Abbreviations
PUBLISHER NAME | ABBREVIATION |
---|---|
American Chemical Society | ACS |
American Geophysical Union | AGU |
Frontiers Media SA | FM |
Geological Society of America | GSA |
Hindawi Limited | HL |
Informa UK Limited | Informa |
MDPI AG | MDPI |
Oxford University Press (OUP) | OUP |
Public Library of Science | PLoS |
Regional Euro-Asian Biological Invasions Centre Oy | REABIC |
Springer Science and Business Media LLC | SSBM |
U.S. Geological Survey | USGS |
Wiley | Wiley |
Acknowledgements
We would like to thank Max Joseph and Taylor Hunt from the University of Colorado Boulder’s Earth Lab for their help developing the initial Python code used to gather data from the Crossref API. We would also like to thank Dalton Hance and Karen Ryberg for assistance in determining appropriate statistical analyses for our data and Shelley Stall for helping us understand challenges associated with getting data citations to Crossref from the publishers’ perspective. Finally, we would like to thank our reviewers, Leslie Hsu, Katharine Dahm, and Daniel Wieferich, who provided invaluable feedback on this manuscript.
Competing Interests
The authors have no competing interests to declare.
Belter, C W 2014 Measuring the value of research data: A citation analysis of oceanographic data sets. PLoS ONE , 9(3): e92590. DOI: https://doi.org/10.1371/journal.pone.0092590
Chamberlain, S, Maupetit, J, Peak, S, et al. 2022 Habanero version 1.2.2 . Available at https://github.com/sckott/habanero [Last accessed 29 January 2022].
Colavizza, G, Hrynaszkiewicz, I, Staden, I, et al. 2020 The citation advantage of linking publications to research data. PLoS ONE , 15(4): e0230416. DOI: https://doi.org/10.1371/journal.pone.0230416
Cousijn, H, Kenall, A, Ganley, E, et al. 2018 A data citation roadmap for scientific publishers. Scientific Data , 5(1): 180259. DOI: https://doi.org/10.1038/sdata.2018.259 .
DataCite 2022 Welcome to DataCite . Available at https://datacite.org/index.html [Last accessed 14 September 2022].
Donovan, G C and Langseth, M L. 2024 U.S. Geological Survey Data Citation Analysis, 2016–2022: U.S. Geological Survey data release. DOI: https://doi.org/10.5066/P9CPC9M2
ESIP Data Preservation and Stewardship Committee 2019 Data Citation Guidelines for Earth Science Data, Version 2 . ESIP. DOI: https://doi.org/10.6084/m9.figshare.8441816.v1
Farley, I 2022 References . Available at https://www.crossref.org/documentation/schema-library/markup-guide-metadata-segments/references/ [Last accessed 15 June 2023].
Gregory, K, Ninkov, A, Ripp, C, et al. 2023 Tracing data: A survey investigating disciplinary differences in data citation. Quantitative Science Studies , 4(3): 622–649. DOI: https://doi.org/10.1162/qss_a_00264
Huang, Y H, Rose, P W and Hsu C N 2015 Citing a data repository: A case study of the protein data bank. PLoS ONE , 10(8): e0136631. DOI: https://doi.org/10.1371/journal.pone.0136631
Irrera, O, Mannocci, A, Manghi, P, et al. 2023 tracing data footprints: Formal and informal data citations in the scientific literature. Springer . DOI: https://doi.org/10.1007/978-3-031-43849-3_7
Kafkas, Ş, Kim, J H and McEntyre, J R 2013 Database citation in full text biomedical articles. PLoS One , 8: e63184. DOI: https://doi.org/10.1371/journal.pone.0063184
Kretser, A, Murphy, D, Bertuzzi, S, et al. 2019 Scientific integrity principles and best practices: Recommendations from a scientific integrity consortium. Science and Engineering Ethics , 25(2): 327–355. DOI: https://doi.org/10.1007/s11948-019-00094-3
Lafia, S, Thomer, A, Moss, E, et al. 2023 How and why do researchers reference data? A study of rhetorical features and functions of data references in academic articles. Data Science Journal , 22(1): 10. DOI: https://doi.org/10.5334/dsj-2023-010
Lin, J 2016 Linking Publications to Data and Software. Crossref Blog . Available at https://www.Crossref.org/blog/linking-publications-to-data-and-software/#:~:text=Crossref%20and%20DataCite%20have%20partnered%20to%20provide%20automatic,research%20information%20network%20with%20full%20and%20accurate%20metadata [Last accessed 14 September 2022].
Make Data Count 2022 Make Data Count . Available at https://makedatacount.org/ [Last accessed 18 November 2022].
Melton, J and Buxton, S 2006 Querying XML: XQuery, Xpath, and SQL/XML in context . Elsevier Science. pp. 67–84. DOI: https://doi.org/10.1016/B978-155860711-8/50005-8
Mooney, H 2011 Citing data sources in the social sciences: Do authors do it? Learned Publishing , 24(2): 99–108. DOI: https://doi.org/10.1087/20110204
Parks, H, You, S and Wolfram, D 2018 Informal data citation for data sharing and reuse is more common than formal data citation in biomedical fields. Journal of the Association for Information Science and Technology , 69(11): 1346–1354. DOI: https://doi.org/10.1002/asi.24049
Parsons, M A, Duerr, R E, Jones, M B 2019 The history and future of data citation in practice. Data Science Journal , 18(1): 52. DOI: https://doi.org/10.5334/dsj-2019-052
Peters, S E, Ross, I A, Rekatsinas, T, et al. 2021a xDD: A Digital Library and Cyberinfrastructure Facilitating the Discovery and Utilization of Data & Knowledge in Published Documents . Available at https://geodeepdive.org [Last accessed on 28 December 2021].
Peters, S E, Ross, I A, Rekatsinas, T, et al. 2021b xDD: About . Available at https://geodeepdive.org/about.html [Last accessed on 28 December 2021].
Rittman, M 2020 Event Data . Available at https://www.crossref.org/services/event-data/ [Last accessed 15 June 2023].
Stall, S, Bilder, G, Cannon, M, et al. 2022 Journal production guidance for software and data citations. ESS Open Archive . DOI: https://doi.org/10.22541/essoar.167252601.17695321/v1
U.S. Geological Survey (USGS) 2022 Who We Are . Available at https://www.usgs.gov/about/about-us/who-we-are [Last accessed on 18 November 2022].
U.S. Geological Survey Data Management (USGS Data Management) 2022 Data Citation | U.S. Geological Survey . Available at https://www.usgs.gov/data-management/data-citation [Last accessed on 14 September 2022].
U.S. Geological Survey Office of Science Quality and Integrity (USGS OSQI) 2016 Public Access to Results of Federally Funded Research at the U.S. Geological Survey: Scholarly Publications and Digital Data . Available at http://sparcopen.org/wp-content/uploads/2016/04/USGS-PublicAccessPlan-APPROVED.pdf [Last accessed on 14 September 2022].
U.S. Geological Survey Office of Science Quality and Integrity (USGS OSQI) 2017 502.8 – Fundamental Science Practices: Review and Approval of Scientific Data for Release . Available at https://www.usgs.gov/survey-manual/5028-fundamental-science-practices-review-and-approval-scientific-data-release [Last accessed on 14 September 2022].
U.S. Geological Survey Office of Science Quality and Integrity (USGS OSQI) 2021a Fundamental Science Practices (FSP) Guide to Data Releases with or Without a Companion Publication . Available at https://www.usgs.gov/office-of-science-quality-and-integrity/fundamental-science-practices-fspguide-data-releases-or [Last accessed on 14 September 2022].
U.S. Geological Survey Office of Science Quality and Integrity (USGS OSQI) 2021b 1100.2 – Editorial Review of U.S. Geological Survey Publication Series Information Products . Available at https://www.usgs.gov/survey-manual/11002-editorial-review-us-geological-survey-publication-series-information-products [Last accessed on 14 September 2022].
U.S. Geological Survey Office of Science Quality and Integrity (USGS OSQI) 2021c 1100.3 – U.S. Geological Survey Publication Series . Available at https://www.usgs.gov/survey-manual/11003-us-geological-survey-publication-series [Last accessed on 15 June 2023].
Wieferich, D, Serna, B, Langseth, M, et al. 2020 Publink . U.S. Geological Survey Software Release. DOI: https://doi.org/10.5066/P92MX1NF
Wilkinson, L 2022 About us . Available at https://www.crossref.org/about/ [Last accessed 14 September 2022].
Wilkinson, M, Dumontier, M, Aalbersberg, I, et al. 2016 The FAIR Guiding Principles for scientific data management and stewardship. Scientific Data , 3(1): 160018. DOI: https://doi.org/10.1038/sdata.2016.18
Zhao, M, Yan, E and Li, K 2017 Data set mentions and citations: A content analysis of full-text publications. Journal of the Association for Information Science and Technology , 69(1): 32–46. DOI: https://doi.org/10.1002/asi.23919
A systematic review and meta-data analysis of clinical data repositories in Africa and beyond: recent development, challenges, and future directions
- Open access
- Published: 26 June 2024
- Volume 2 , article number 8 , ( 2024 )
Cite this article
You have full access to this open access article
- Kayode S. Adewole 1 ,
- Emmanuel Alozie 2 ,
- Hawau Olagunju 2 ,
- Nasir Faruk 2 ,
- Ruqayyah Yusuf Aliyu 3 ,
- Agbotiname Lucky Imoize 4 ,
- Abubakar Abdulkarim 5 , 13 ,
- Yusuf Olayinka Imam-Fulani 6 ,
- Salisu Garba 7 ,
- Bashir Abdullahi Baba 8 ,
- Mustapha Hussaini 9 ,
- Abdulkarim A. Oloyede 6 ,
- Aminu Abdullahi 10 ,
- Rislan Abdulazeez Kanya 11 &
- Dahiru Jafaru Usman 12
122 Accesses
Explore all metrics
A Clinical Data Repository (CDR) is a dynamic database capable of real-time updates with patients' data, organized to facilitate rapid and easy retrieval. CDRs offer numerous benefits, ranging from preserving patients' medical records for follow-up care and prescriptions to enabling the development of intelligent models that can predict, and potentially mitigate serious health conditions. While several research works have attempted to provide state-of-the-art reviews on CDR design and implementation, reviews from 2013 to 2023 cover CDR regulations, guidelines, standards, and challenges in CDR implementation without providing a holistic overview of CDRs. Additionally, these reviews need to adequately address critical aspects of CDR; development and utilization, CDR architecture and metadata, CDR management tools, CDR security, use cases, and artificial intelligence (AI) in CDR design and implementation. The collective knowledge gaps in these works underscore the imperative for a comprehensive overview of the diverse spectrum of CDR as presented in the current study. Existing reviews conducted over the past decade, from 2013 to 2023 have yet to comprehensively cover the critical aspects of CDR development, which are essential for uncovering trends and potential future research directions in Africa and beyond. These aspects include architecture and metadata, security and privacy concerns, tools employed, and more. To bridge this gap, in particular, this study conducts a comprehensive systematic review of CDR, considering critical facets such as architecture and metadata, security and privacy issues, regulations guiding development, practical use cases, tools employed, the role of AI and machine learning (ML) in CDR development, existing CDRs, and challenges faced during CDR development and deployment in Africa and beyond. Specifically, the study extracts valuable discussions and analyses of the different aspects of CDR. Key findings revealed that most architectural models for CDR are still in the theoretical phase, with low awareness and adoption of CDR in healthcare environments, susceptibility to several security threats, and the need to integrate federated learning in CDR systems. Overall, this paper would serve as a valuable reference for designing and implementing cutting-edge clinical data repositories in Africa and beyond.
Similar content being viewed by others
Data Management Strategy for AI Deployment in Ethiopian Healthcare System
Public Electronic Health Record Platform Compliant with the ISO EN13606 Standard as Support to Research Groups
Integrating Electronic Health Records in Clinical Decision Support Systems
Avoid common mistakes on your manuscript.
1 Introduction
A clinical record is a document that entails a patient's medical history, clinical findings, diagnostic test results, pre- and post-operative treatment, patient progress, and medication [ 1 ]. Clinical data have recently been used for further objectives outside clinical ones, such as research, therapy improvement, and critical decision-making through AI and ML modeling. The desire to provide patients with the best care possible and discover the best platform for decision-making necessitates advancing medical systems [ 2 ]. Before the advent of digital and electronic devices in healthcare centers or hospitals, paper-based methods of collecting and storing clinical data were adopted. However, the large volume of data being collected as well as the growing security and privacy concerns, among others, exposed the inefficiency of the paper-based method. As a result, there is a need to develop a better way to collect and particularly store this large volume of data for easy accessibility and management while enabling the security and privacy requirements of the data. Toward this end, the development of clinical data repositories was proposed.
A CDR is a comprehensive patient-centered database that is updated in real-time and arranged to support quick and easy retrieval of clinical data. The data is frequently clinically focused, giving care providers the requisite to decide how to treat patients. CDRs are essential because they provide quicker and easier access to medical records [ 3 , 4 ]. It has several benefits including providing a longitudinal medical history about patients and information on previous procedures and test results, to avoid duplication in testing and redundancies in care. Furthermore, it can facilitate the prediction and risk modeling using intelligent algorithms since clinical data can be easily harvested from the repository [ 5 ]. Typical examples of the types of information that may be found in a CDR include patient demographics, patient’s primary care provider, medication list, lab results, allergies, procedures, diagnosis, scheduling information, medical record data, images such as x-rays, etc. [ 4 ]. However, their adoption in the medical system has been gradual.
Currently, there are only a few developed and functional CDRs in the existing literature. This can be due to a lack of adequate clinical data, security and privacy concerns of clinical data, or the inability of the clinical personnel to effectively utilize the repository. Existing research works [ 2 , 3 , 6 , 7 , 8 , 9 , 10 ] have attempted to provide a state-of-the-art review on the design and implementation of CDR, considering other aspects of CDR such as standards, the role of AI and ML in CDR, as well as the associated challenges.
In particular, while the work [ 2 ] focuses on the clinical data warehouse, highlighting the reviews of 42 papers 42 extracted from 784. The study reiterated the need for a clinical data warehouse to facilitate clinical trials and practice. The study of Dainton and Chu [ 3 ] reviews electronic medical record-keeping on mobile medical service trips in Austere settings. The study affirms the importance of electronic medical record keeping and it can be simplified for user-friendliness. In another related work by Gamal et al. [ 9 ] on a comparative review of standardized electronic health record data modeling and persistence, the authors shed light on the significance of medical record keeping and modeling using advanced technologies. In the work of [ 7 ], a survey of OpenEHR storage implementations is conducted. Key findings revealed the place of innovative design of modern EHR storage, enhancing efficient medical data storage and smooth retrieval systems cannot be overemphasized. The work [ 8 ] dwells on clinical data acquisition standards, highlighting harmonization, importance, and benefits in clinical data management. Similarly, the study by [ 10 ] presents a systematic review of administrative and clinical databases of infants admitted to neonatal units. Last, the work of de Mello et al. [ 6 ] presents a systematic literature review of semantic interoperability in health records standards.
Some examples of the use of AI and ML in healthcare systems are outlined as follows. In [ 11 ] AI-based services for healthcare are highlighted. In particular, the study remarks that the usefulness of AI in healthcare is measured in direct proportion to the capabilities of AI in improving healthcare outcomes, facilitating support for caregivers, and a drastic reduction in healthcare costs. In another related work, Zafeiropoulos et al. [ 12 ] study the results from diverse ML models and demonstrate whether the results are true and realistic or not, using diverse metric functions. These metrics were used to test the validity of the ML models in extracting efficient and reliable outcomes. In particular, the authors developed several ML models to predict the likelihood of stroke in humans. Generally, the findings attest to the potential of ML revolutionizing healthcare management.
Currently, artificial intelligence is changing the dynamics of modern healthcare, leveraging cutting-edge technologies capable of predicting, grasping, learning, and acting, depending on the application. In line with the study reported by Shaheen [ 13 ] can detect minor patterns that are difficult for humans to process. Specifically, the study focuses on AI-powered healthcare, covering AI-led drug discovery, clinical trials, and patient care. The findings revealed that pharmaceutical industries are the leading beneficiaries of AI in healthcare. Specifically, AI helps speed their drug discovery process and automate target identification. From the preceding, medical AI companies can develop systems that assist patients at every stage of the treatment lifecycle.
However, existing reviews still need to adequately address critical aspects of CDR; development and utilization, CDR architecture and metadata, CDR management tools, CDR security, use cases, and AI in CDR design and implementation. The collective knowledge gaps in these works underscore the imperative for a comprehensive overview of the diverse spectrum of CDR as presented in the current study.
Thus, this paper aims to provide a comprehensive and systematic review, covering 2013 to 2023, of the various aspects of a CDR, including its architecture and metadata, security and privacy issues, rules and regulations guiding the development and utilization of a CDR, tools utilized, as well as the role of AI and ML in the development of CDR, the existing CDRs in literature with a specific focus on African countries and finally, the challenges encountered in the development of a functional CDR. Specifically, the key contributions of this paper are listed as follows;
An exhaustive review of previous reviews on CDR including the limitations of these reviews.
A comprehensive review of the different CDR architectures and metadata proposed in literature.
A review of the different tools adopted for the development of CDR as well as the regulatory standards that have been utilized.
An extensive review of the risk, security, and privacy associated with the development of CDR.
A detailed review of the role of AI and ML in the development of CDR.
An exhaustive review of the current challenges encountered in the development of CDR.
Critical open research issues and future directions are identified for CDR.
The remaining parts of the paper are organized as follows: Sect. 2 presents the methodology adopted in conducting the systematic review. Section 3 provides a review of existing review work on CDR as well as their limitations. The existing CDR architecture and metadata are provided in Sect. 4 . Section 5 presents a review of the existing regulations, guidelines, and standards for clinical data management. Section 6 provides the different tools for clinical data management. The security and privacy issues in CDR development are provided in Sect. 7 . The role of AI in CDR is presented in Sect. 8 . Section 9 presents the existing CDR projects in the literature with a specific focus on some selected African countries including their respective use cases. The current challenges faced in the development of CDR are reviewed in Sect. 10 . General analysis and discussion of the different aspects of CDR reviewed are provided in Sect. 11 . Section 12 presents the further research directions, and finally, Sect. 13 concludes the paper.
2 Methodology
The methodology adopted for the systematic review in this paper comprises the research questions, the search strategy including the inclusion and exclusion criteria, and the analysis of publications obtained [ 14 , 15 ].
2.1 Planning the review
In this paper, the planning of the systematic review commences with the establishment of a procedure that provides adequate guidelines for carrying out the review work. The guidelines adopted in this paper include [ 14 , 15 , 16 , 17 , 18 , 19 ] (a) Definition of the research questions; (b) Outlining the search strategy (c) listing the inclusion and exclusion criteria which are essentially the study selection criteria) and (d) data extraction, analysis, and synthesis of results.
2.2 Research questions (RQ)
The review is guided by specific Research Questions (RQs). Table 1 presents the research questions to guide the systematic review and to extract information of interest from the papers selected for the review.
In this review of clinical data repositories, we have posed a series of critical research questions to advance our understanding and contribute to the existing literature. We start by mapping the available review papers to establish the landscape of prior research (RQ1) and proceed to investigate the state-of-the-art clinical data repository, including its architecture, data sources, and metadata (RQ2). We delve into regulatory aspects (RQ3) and practical tools (RQ4) while addressing the pressing issues of security and privacy (RQ5) and the role of artificial intelligence (RQ6) in data management. Additionally, we explore the presence of clinical data repository projects in Nigeria or Africa (RQ7a) and assess their analytical capabilities (RQ7b) to offer regional insights. Finally, we identify the current challenges in developing clinical data repositories (RQ8), contributing valuable knowledge to inform research and practical applications in healthcare data management.
2.3 Search Strategy (SS)
The search strategy outlines the specific protocol adopted in searching for relevant materials for the review, such as the channels used for the literature search, relevant keywords used during the search, the extent of the sampling strategy, the stopping rule, and other possible restrictions that may be imposed. In this review work, seven (7) academic databases were utilized. The databases are Science Direct Footnote 1 , PubMed, Footnote 2 IEEE Xplore Digital Library, Footnote 3 Springer Nature, Footnote 4 Google Scholar, Footnote 5 MDPI Footnote 6 , and ACM Digital Library. Footnote 7 These databases were carefully selected due to their popularity, ease of use, and dominance in the academic community, as they consist of reliable and quality peer-reviewed publications such as research, review, and conference articles. In this review work, we considered peer-reviewed publications that were written in English Language only and were published in the last 10 years (i.e. between 2013 and 2023). The following keywords were used; “clinical data repository”, “health data repository”, “health information database”, “clinical data warehouse”, and “clinical data management” among others. We utilized the Boolean operators to link the keywords as must of the database supports it. The “OR” operator was used for linking identical keywords, while the “AND” operator was for combining the main terms in the search string in Table 2 [ 19 ]. Details of the search terms utilized per RQs are provided in Table 2 .
2.4 Study selection criteria
Considering the research questions provided in Table 1 and the objectives of the review paper, inclusion and exclusion criteria were defined, as follows:
Inclusion criteria.
Research papers that are included in this paper contain the following:
Research paper that is written in English Language
Research paper that is written between 2013 and 2023
Research paper that is reported in peer-reviewed journals, Conference proceedings, workshops, or journals.
Study that investigates the state-of-the-art CDR, including its architecture, data sources, and metadata
Study that presents results related to regulatory aspects of CDR including practical tools, security and privacy, and artificial intelligence
Exclusion criteria.
Research papers that are excluded in this paper contain the following:
Study on CDR, without including its architecture, data sources, and metadata
Paper not accessible electronically
Paper that is not complete (content and incomplete results) OR.
Encyclopedia, posters, books, book chapters, keynotes, and editorials
Research paper that is a duplicate (if two versions of a paper are found, the less complete version is excluded).
2.5 Analysis of publications obtained
Numerous research publications were retrieved from the different databases considered based on the search terms related to each research question. A total of 9,488 research documents were retrieved across all the databases. Among these, 3,617 were duplicates. To streamline these search results to the subject of interest, we conducted refinements based on the titles, abstracts, related topics, language, and peer review as outlined in the inclusion and exclusion criteria. A total of 3,947 documents were excluded. Furthermore, the documents were screened based on the year of publication, full texts availability, and accessibility of the publications. As a result of these refinements, it was observed that only 53 research papers met the selection criteria and were subsequently reviewed. Figure 1 provides the sequence and flow of the selection process. A total of 9 papers met the criteria for RQ1 and were reviewed, while, for RQ2, 16 papers were reviewed. A summary of these analyses is presented in Fig. 2 . In the same vein, Figs. 2 and 3 illustrate the distribution of articles based on the year of publication and article type, respectively.
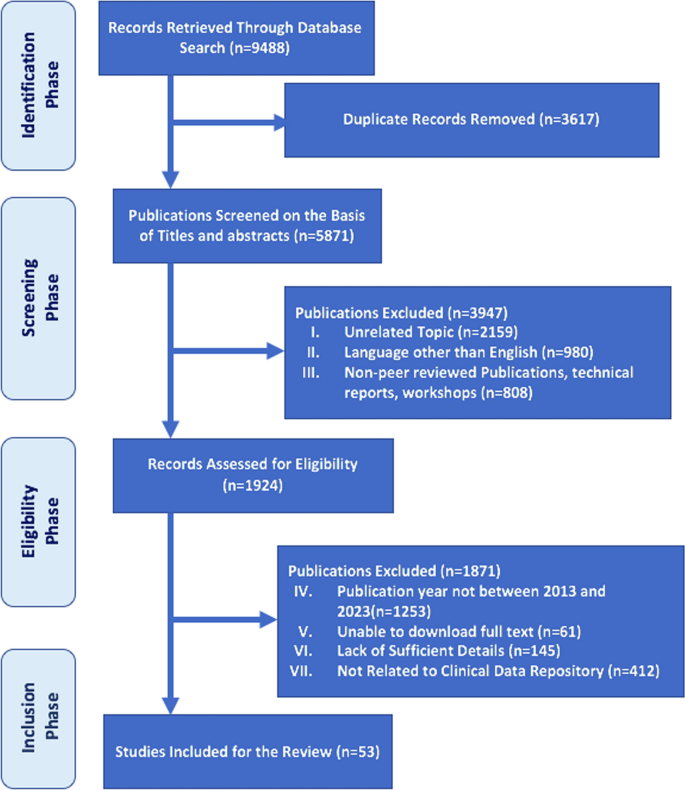
Study selection flow and sequence
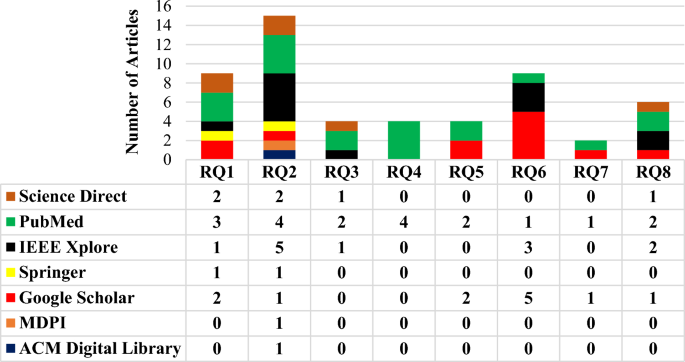
Articles distribution across databases Per RQs
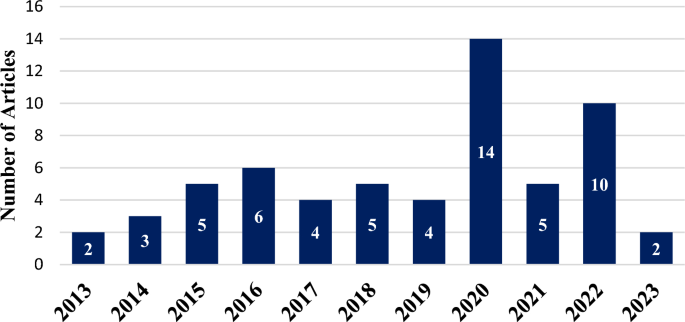
Article distribution across years (2013–2023)
Figure 2 illustrates the distribution of articles across various RQs and databases examined. Notably, a predominant portion of the articles included in this review originates from the PubMed database. This prevalence can be attributed to the exclusive dedication of PubMed to biomedical and healthcare research. As a resource meticulously maintained by the National Library of Medicine (NLM), PubMed excels in curating and indexing a vast spectrum of medical and life sciences literature, rendering it the primary and preferred resource for healthcare professionals and researchers. While other databases cover a broader range of topics, PubMed's specialization and credibility in the healthcare sector make it a central hub for CDR-related research, fostering a wealth of knowledge and insights in this niche area.
In Fig. 3 , it is observed that a significant upswing in published work on CDRs in the year 2020. This notable increase can be attributed to a convergence of various factors. Firstly, the COVID-19 pandemic heightened the demand for health-related research and advanced data management solutions, in which CDRs played a pivotal role in facilitating pandemic response efforts. Secondly, the ongoing transition towards electronic health records (EHRs) and the digitization of healthcare systems contributed to the surge in studies related to CDRs. Lastly, the integration of AI and ML into healthcare practices fueled interest in CDRs as a means to leverage AI's potential for enhanced data analysis and decision support in the medical field.
The comparable percentages of review papers to technical papers in the domain of CDRs, as shown in Fig. 4 , may be influenced by a variety of factors. One possible factor could be the inherent challenges associated with data collection and experimentation in healthcare settings. Clinical data, often sensitive and regulated, can be difficult to access and utilize for experimental purposes, leading researchers to focus on comprehensive literature reviews that analyze existing knowledge. Additionally, the complex and interdisciplinary nature of CDRs necessitates a thorough understanding of existing research and technical advancements, prompting a substantial number of review papers. These reviews not only synthesize knowledge but also highlight gaps and suggest future research directions, contributing significantly to the academic discourse.
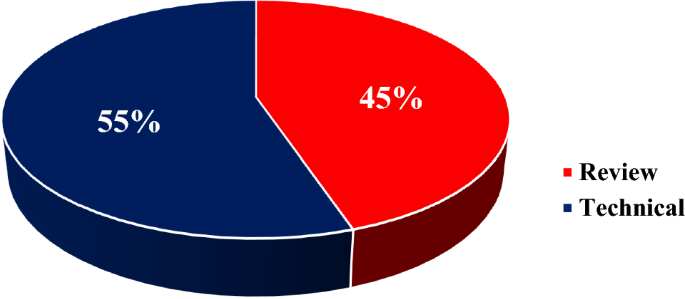
Article type distribution
3 Previous reviews on clinical data repository
This section presents the previous reviews on CDR as summarized in Table 3 based on the objectives, findings, application areas, and year published. In addition, Table 4 presents the limitations of these works, which are highlighted in Table 3 . These tables collectively highlight the diverse landscape of clinical data repository research, spanning standards, mobile medical service trips, neonatal databases, Clinical Data Warehouses (CDWs), EHR storage, semantic interoperability, big data research, and AI applications. The findings emphasized the importance of data standards, efficient storage solutions, and the potential for AI to transform clinical data repositories while identifying research gaps for further research and development.
From Table 4 , it can be seen that most of these existing reviews focused on aspects related to regulations, guidelines, and standards, such as the CDASH standards. Some reviews also delve into specific challenges associated with CDR implementation. However, a holistic overview of CDRs still needs to be addressed in the current body of literature. These reviews tend to lack a comprehensive perspective that encompasses key facets of CDR development and utilization. These overlooked areas include the integration of CDR architecture and metadata, an exploration of tools for CDR management, an examination of the role of AI and ML in CDR development, a thorough analysis of CDR use cases, as well as discussions on security, privacy preservation, risk indicators, and the challenges faced in CDR development. While each existing review contributes valuable insights within its respective domain, the collective knowledge gaps underscore the pressing need for comprehensive research that extensively covers the diverse spectrum of CDR-related topics.
4 CDR architectures and metadata
CDR has been implemented based on several approaches such as a template relational mapping (TRM) archetype-drive approach used in [ 22 ] where results indicated that this implementation is feasible against clinical practices and supports the characteristics of adaptability and user-involved. A DSpace approach was utilized in [ 23 ] to develop a demonstrator repository where results obtained showed that it performed well in terms of handling sensitive personal data from clinical trials, with 14 requirements met (74%), including support for metadata and identifiers. Oracle 10 g was utilized in [ 24 ] for the implementation of a proposed database model for a medical information system that would support decision-making. Results from the study showed that the proposed model managed clinical data as well as helped data analysts and clinical managers to conduct data mining and analysis over data stored in the warehouse. A Hadoop-based architecture was proposed by Lyu et al. [ 25 ] and Khan et al. [ 26 ] for the design and implementation of a clinical data integration and management system where the system can connect with multiple heterogeneous data sources. Results showed that the system achieved the goal of sharing and managing clinical data and also was able to handle queries about patients' medical records as well as data visualization. It is also suitable for solving a variety of previously unsolvable medical problems. Interestingly, a novel architecture that consists of four layers; infrastructure, storage, computation, and service, was proposed by Rouzbeh et al. [ 27 ] for a software-hardware-date ecosystem using open-source technologies such as Apache Hadoop, Kubernetes, and JupyterHub in a distributed environment to support (hard) reproducibility of analytic workflow with a massive volume of heterogeneous data where results obtained showed that the response time increases with a corresponding increase in the data size for both types of tables. Figure 5 presents the typical CDR architecture, including various data sources which allows data to be collected from various sources, cleansed, transformed, and loaded into a central repository. This data can then be analyzed using a variety of tools and technologies to support data-driven decision-making. The figure illustrates four major phases or layers of a typical CDR architecture. These phases or layers are explained below:
Data sources: This layer includes various sources from which data is collected. Examples shown in the figure include patient records, pharmaceutical data, health surveys, clinical trials, and claims data.
Data staging: In this layer, data is extracted from the source systems, transformed into a consistent format, and then loaded into the CDR. This process is typically performed by an ETL (Extract, Transform, Load) tool.
Data repository: This is the core of the CDR where the data is stored. Here, data is structured and organized for analysis. Data marts are subsets of the CDR that are designed to support the specific needs of a particular department or business function. For instance, a sales data mart could be created to support the sales team.
Data analysis and technologies: This layer includes the tools and technologies used to analyze the data in the CDR. Examples include Online Analytical Processing (OLAP) tools, data mining tools, and reporting tools.
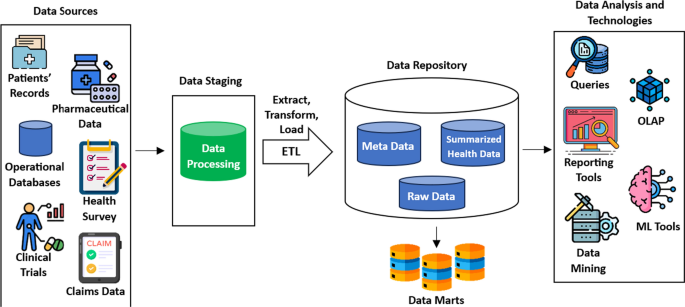
A Typical CDR architecture
Architectures such as Oracle NoSQL-based architecture, Natural Language Processing (NLP) architecture, and cloud-based architecture have been studied throughout the literature. For example, in [ 28 ], an Oracle NoSQL-based CDR architecture with database functionalities was proposed to store and manage data in different structures, combining relational and non-relational databases. The study concluded that Oracle’s NoSQL tool has adequate functionality for the required implementation, particularly in resource allocation and easier troubleshooting. A high throughput NLP architecture was developed in [ 29 ] using the clinical text analysis and knowledge extraction system where results obtained showed that the architecture processed 83,867,802 clinical documents in 13.33 days and produced 37,721,886,606 concept unique identifiers (CUIs) across 8 standardized medical vocabularies. A cloud-based architecture was proposed by Augustyn et al. [ 30 ] and Sarwar et al. [ 31 ] for Poland and Pakistan, respectively. Here, results showed that the proposed architectures met the set requirements and the criteria of the sustainable development paradigms and could result in the generation of a huge data repository that would help improve patient care, diagnostics and patient outcomes, disease prevention, and disease surveillance of possible outbreaks. Other proposed architectures for the data warehouse are also included such as a data warehouse architecture proposed in [ 32 ] which was based on the Italian EHR technological infrastructure. Conclusions made were that the deployment of EHR systems could improve interoperability because these systems share standardized clinical data among diverse stakeholders participating in various healthcare settings.
In addition to the CDR architectures, different frameworks have also been proposed in literature. For instance, a breast imaging data warehouse framework was proposed and designed by Amara et al. [ 33 ] based on two proposed evident medical applications which include clinical testing studies on multimodality breast imaging cases and studies on patient data processing and decision limit on lateralization of inflammatory breast cancer. Similarly, in the work of Dagliati et al. [ 34 ], a data-gathering framework was presented to collect Type-2 Diabetes (T2D) patient data within the EU project MOSAIC, coming from three European hospitals and a local health care agency. The conclusion made was that the MOSAIC framework was capable of seamlessly managing the data of more than 5,000 T2D patients in three different countries across Europe. An architecture for improving data quality in clinical and translational data warehouse infrastructures was described and implemented in [ 35 ], using ETL processes. The approach enabled the monitoring of the data quality evolution over time using configurable dashboards and alerting mechanisms for important medical research platforms such as i2b2, tranSMART, and warehouses based on the OMOP CDM. Results obtained from the implementation showed that the most frequent issues were missing values and invalid categories. Furthermore, four common architectural models of integrated data repositories (IDRs) that used different approaches for data processing and integration were identified in [ 36 ] which include general architecture with optimal clinical decision support systems, biobank-based architecture, user-controlled application layer, and federated architecture.
The review shows that several architectures and frameworks have been proposed, reviewed, assessed, and implemented. However, one of the common limitations that have been witnessed in these research works is the lack of validation in real-world medical settings, as a result, most of these proposed architectures and frameworks are still in their theoretical phases.
5 Regulations, guidelines, and standards in clinical data management
This section presents a brief description of what regulations, guidelines, and standards mean with regard to clinical data management as well as describes widely known standards and regulations. A systematic review of existing works that considered regulations and standards in clinical data management is provided. In Clinical Data Management (CDM), regulation, guidelines, and standards collectively constitute the cornerstone of responsible and secure healthcare data management.
5.1 Regulations
Regulations, emanating from government authorities, impart legally binding requirements, such as the Health Insurance Portability and Accountability Act (HIPAA), General Data Protection Regulation (GDPR), and California Consumer Privacy Act (CCPA), among others, governing the protection and privacy of patient data. Guidelines, though not legally enforceable, offer invaluable best practices and insights into effective CDR design, data quality assurance, and interoperability. Figure 6 highlights five major standards. A further description of these regulations is presented below;
Health Insurance Portability and Accountability Act (HIPAA): The HIPAA is a pivotal U.S. federal law enacted in 1996. Its primary purpose is to safeguard the privacy and security of protected health information (PHI) and improve the portability of health insurance for individuals [ 37 ]. HIPAA has a profound impact on CDM by imposing strict regulations and standards for the handling, storage, and transmission of healthcare data [ 38 ]. It compels healthcare organizations to adopt stringent data protection practices, invest in secure EHR systems, and conduct risk assessments to identify vulnerabilities. It also influences the design of CDRs to ensure compliance with its privacy and security requirements.
General Data Protection Regulation (GDPR): The GDPR is a comprehensive data protection regulation adopted by the European Union (EU) and enforced on May 25, 2018 [ 39 , 40 ]. Its primary objective is to enhance the protection of individuals' data and provide them with greater control over how their data is collected, processed, and stored. It also imposes strict requirements on the handling of patient data, including EHRs and CDRs.
California Consumer Privacy Act (CCPA): The CCPA is a comprehensive data privacy law enacted in California, USA, aimed at safeguarding the personal information of California residents [ 41 , 42 ]. It grants consumers certain rights over their data, including the right to know what information is being collected, the right to access it, and the right to request its deletion. Healthcare providers and organizations handling clinical data must ensure compliance with the CCPA's stringent privacy requirements. This includes providing clear and transparent notices to patients regarding data collection and usage, obtaining explicit consent where necessary, implementing robust data security measures, and responding promptly to consumer requests for access to or deletion of their data.
Personal Information Protection Law (PIPL): The PIPL is one of China's existing regulations concerning privacy in medical AI, alongside the Civil Code and applicable national standards. These regulations outline specific guidelines regarding individual consent and authorization for data processing. Individuals must be informed about the purpose, method, and extent of information processing [ 37 ].
The Nigeria Data Protection Act (NDPA): NDPA came into effect following its signing into law on June 14, 2023. This legislation introduces significant measures for safeguarding personal data and marks the first instance of a legislative body in Nigeria addressing such protection explicitly. Although stakeholders view the NDPA as a significant advancement in personal data regulation within Nigeria, some uncertainties linger regarding its legitimacy and scope of application [ 38 ].
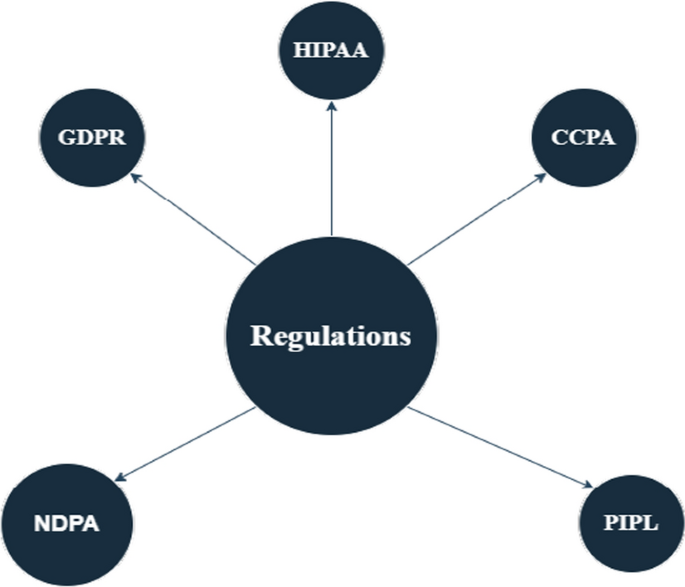
Regulations in clinical data repository
5.2 Standards and guidelines
Standards, established by industry bodies like Health Level Seven (HL7) and Digital Imaging and Communications in Medicine (DICOM), facilitate seamless data exchange and compatibility across diverse healthcare systems. Together, this trio forms a robust framework that ensures the ethical, secure, and standardized management of clinical data within CDRs, safeguarding patient information and promoting interoperability in the healthcare ecosystem. Figure 7 presents well-known global standards and regulations applicable when clinical data are involved. The figure highlights eight major standards. A further description of these standards is presented below;
International Council for Harmonization (ICH) Guidelines: The ICH Guidelines are a set of international standards and guidelines developed collaboratively by regulatory authorities and pharmaceutical industry experts to ensure the quality, safety, efficacy, and integrity of pharmaceutical products, including those used in clinical trials [ 39 ]. The ICH guidelines cover various aspects of drug development, including clinical data management. They promote standardized data collection and documentation practices, ensuring that clinical trial data is accurate, reliable, and compliant with regulatory requirements.
Digital Imaging and Communications in Medicine (DICOM): DICOM is a widely adopted international standard used for the management, storage, transmission, and exchange of medical images and related information [ 40 , 41 ]. It ensures interoperability and consistency among various medical imaging devices, picture archiving and communication systems (PACS), and healthcare information systems, allowing for the seamless sharing and integration of medical images and associated data across different healthcare environments. DICOM facilitates the efficient storage and retrieval of medical images, such as X-rays, Magnetic Resonance Images (MRIs), and Computerized Tomography (CT) scans, within EHRs and clinical databases. This standardization enables healthcare providers to access patient images promptly, aiding in accurate diagnosis and treatment planning.
Health Level Seven (HL7): HL7 is a set of international standards for the exchange, integration, sharing, and retrieval of electronic health information [ 42 ]. They facilitate interoperability and data exchange among healthcare systems, ensuring that clinical data can be effectively shared and used across different healthcare settings and applications [ 43 ]. They enable healthcare organizations to securely transmit clinical data, such as patient records, lab results, and medical images, among various systems and applications, including EHRs, laboratory information systems (LIS), and radiology systems. This standardized approach enhances data accuracy, reduces errors, and improves the overall quality of patient care.
Clinical Data Interchange Standards Consortium (CDISC): The CDISC is a global nonprofit organization that develops and promotes data standards for clinical research and healthcare [ 44 , 45 ]. CDISC standards are designed to streamline the collection, exchange, and analysis of clinical data, making it more consistent, efficient, and interoperable across the healthcare industry. They improve data quality, simplify data integration, and enhance the ability to compare and analyze data across different studies and systems. This standardization reduces errors, accelerates drug development, and ensures that clinical data are readily available for regulatory submissions and research collaborations.
Fast Healthcare Interoperability Resources (FHIR): FHIR is a standard for electronic health data exchange and interoperability in the healthcare domain [ 44 , 46 ]. Developed by HL7, FHIR is designed to make it easier for different healthcare systems and applications to share and exchange healthcare data in a standardized, structured format [ 47 , 48 ]. It streamlines the exchange of patient information, medical records, and other healthcare data among different providers, hospitals, and applications. This enables healthcare organizations to improve care coordination, enhance patient outcomes, and facilitate clinical research by efficiently accessing and sharing clinical data in a standardized format.
Clinical Data Acquisition Standards Harmonization (CDASH): CDASH is a set of standards developed by the CDISC to facilitate the collection of clinical trial data in a consistent and standardized format [ 8 ]. It provides guidelines for structuring case report forms (CRFs) and electronic data capture (EDC) systems, ensuring that data collected during clinical trials are clear, complete, and compliant with regulatory requirements. By adhering to CDASH standards, clinical data managers can streamline data entry processes, reduce errors, and enhance data quality. This, in turn, accelerates the data review and analysis process, ultimately improving the accuracy and reliability of clinical trial results.
Systematized Medical Nomenclature for Medicine–Clinical Terminology (SNOMED CT): SNOMED CT is a robust clinical terminology managed by SNOMED International, formerly known as the International Health Terminology Standards Development Organization (IHTSDO). It serves as both a coding scheme and a multi-hierarchical ontology for term identification and interrelated concepts in clinical settings. SNOMED International offers a standardized approach to representing clinical data recorded by healthcare professionals [ 49 ].
Logical Observation Identifier Names and Codes (LOINC): The LOINC database offers a universal coding system designed for reporting clinical and laboratory observations. Its primary goal is to facilitate the identification of observations within electronic messages like HL7 observation messages. This enables healthcare institutions, pharmaceutical companies, researchers, and public health agencies to efficiently organize incoming data from various sources into the appropriate sections of their medical records, research endeavors, and public health systems [ 50 ].
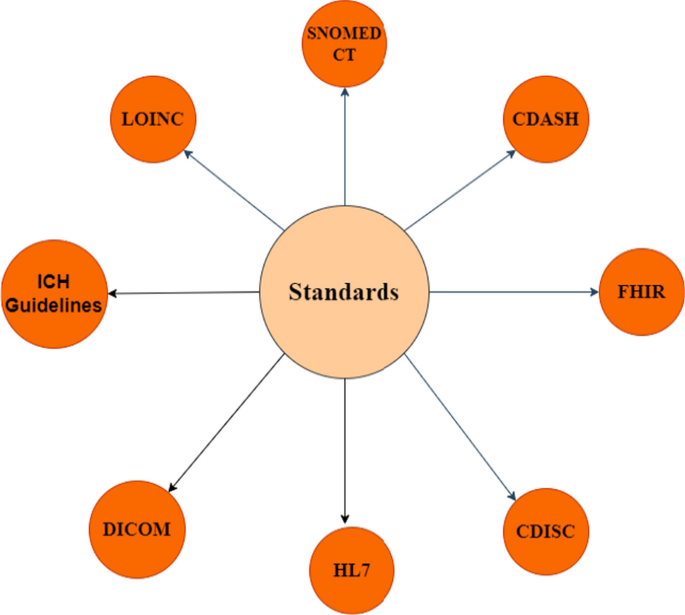
Standards in clinical data repository
5.3 Related works on regulations, guidelines, and standards in clinical data management
Different studies have been conducted on the regulations, standards, and guidelines for clinical data management such as in [ 51 ] where clinical data standards were utilized to measure quality. Specifically, the study presented details and addressed problems associated with the usage of interoperability standards to meet the aim of quality measurement through a Quality Reporting Document Architecture (QRDA) using data from 11 ambulatory care facilities and 5 distinct EHRs. Results revealed that iterative solutions for 14 measures enhanced patient inclusion and measurement accuracy. Additionally, the data verified this technique to enhance measure accuracy while preserving measure certification. Similarly, in the work of Lin et al. [ 52 ], a standard-based method for electronic submission to pharmaceutical regulatory authorities was proposed to increase the efficiency and quality of standard-compliant documents where results showed that the proposed approach accelerated CRF and guaranteed data tabulation integrity. A systematic review of the semantic interoperability in health records standards was provided in the work of de Mello et al. [ 6 ] where one of the review’s findings was that ontologies have been widely employed, and good results have been observed in the adoption of semantic web technologies, mostly employing ontologies mixed with patterns, to boost data representation in formats with a semantic focus. To provide authorization interoperability, the work of Rashid et al. [ 53 ], proposed an access control model for CDR-based shared care environments based on the HL7 Role-based Access Control (RBAC) standard. The system provided the separation of policy development and system implementation to avoid changes in access control rules for any role or permission affecting the system itself. To evaluate the utilization of advanced DICOM standard features beyond imaging data storage in research practices, the study of Aiello et al. [ 54 ] scrutinized publicly accessible medical imaging databases. The aim was to gauge the extent to which common medical imaging software tools fully support DICOM in its entirety. Through a systematic methodology, 100 public databases and ten medical imaging software tools were analyzed. Findings revealed that fewer than one-third of the examined databases employ the DICOM format to capture meaningful information for image management. Moreover, the majority of software tools lack support for managing, reading, and writing certain or all DICOM fields. The study underscores DICOM's potential in facilitating comprehensive big data management, yet it emphasizes the need for additional efforts from the scientific and technological communities to promote widespread adoption of this standard. Encouraging data sharing and interoperability is pivotal for fostering concrete advancements in big data analytics.
This review highlights various studies that explore the utilization of clinical data standards and interoperability standards to enhance the quality of healthcare data management and reporting. It specifically discusses how these standards have been employed to improve patient inclusion, measurement accuracy, data tabulation integrity, semantic interoperability, and access control within clinical data repositories. However, the limitations of the review include the absence of a comprehensive evaluation of the methodologies used in the discussed studies and the need for further research to assess the scalability and real-world implementation of these standards in diverse healthcare settings.
6 Tools for clinical data management
This section presents a systematic review of the different tools proposed and developed in literature for clinical data management and also presents a summary of these tools based on their functions and limitations.
The clinical data management could be classified into Electronic Data Capture (EDC) systems, Clinical Data Management Systems (CDMS), Electronic Patients Reported Outcomes (ePRO), Randomization and Trial Supply Management (RTSM), and Clinical Trial Management Systems (CTMS). Footnote 8 The EDC systems are web-based applications that allow for the collection, management, and storage of clinical trial data. These tools include the Medidata Rave, Oracle Clinical, OpenClinica, REDCap and many more. CDMS are specialized software solutions that help manage the entire clinical data lifecycle, from data collection to database lock. E.g. Veeva Vault CDMS, Oracle Health Sciences InForm, Medidata Rave. CTMS are used to manage the operational and administrative aspects of clinical trials, including patient enrollment, site management, and study monitoring. E.g. Oracle Health Sciences Clinical One, Veeva Vault CTMS, Medidata Rave CTMS. The ePRO on the other hand, is an electronic method of collecting a patient-reported outcomes which include the symptoms such as diarrhea, pains, fatigue, headache etc. [ 55 ]. There are quite a lot of ePRO systems and have been deployed for many applications such as in Oncology practice [ 55 ] and Cancer Care [ 56 ].
RTSM also known as Interactive Response Technology (IRT) is a software solution designed to efficiently and accurately controls patient randomization, drug and dose administration and supplies, site supply management and accountability and cohort enrollment and management., Footnote 9 Footnote 10
Several tools have been proposed and developed in literature that can be utilized for clinical data management, particularly in the area of improved data querying. For instance, a patient-screening tool was developed by Li et al. [ 57 ] based on EHRs for clinical research using OpenEHR to solve the issue of concept mismatch and improve the overall query performance. Results obtained showed that 589 medical concepts were found in 500 random sentences of which only 513 concepts could be represented. The BMI Investigator (BMII) tool was proposed in [ 58 ] to query structured, unstructured, genomic, and image data contained in a data warehouse. The conclusion made was that the proposed tool can provide an efficient, effective, and useful method for querying data warehouses and the necessary clinical informatics knowledge. Similarly, in [ 59 ], a search tool known as Doc’EDS was proposed to obtain a multilevel search engine combining structured data, clinical narratives, and segmentation. Formal evaluations of the proposed tool semantics features showed excellent results for negation and average results for hypothesis/future. A prediction tool was integrated with CDR by Li et al. [ 5 ] based on pattern discovery considering a case study on contrast related to acute kidney injury. It was concluded that the tool showed user-friendliness by physicians and demonstrated competitive performance compared with other state-of-the-art models. From the review conducted, some of the tools that have been developed and considered are as follows:
Patient-Screening Tool: this tool was developed in [ 57 ] using OpenEHR. It utilized a loosely coupled architecture and was divided into three major parts. The first part involves concept editing and management, where screening concepts can be maintained and managed through definition and generation. The second part is the screening conditions’ construction or execution, where a user-friendly interface is provided for users to edit screening conditions, and restful APIs are utilized to execute queries in Elasticsearch. Finally, the third part involves the results of the screening configuration, aimed at different data requirements. Researchers can predefine specific data views in forms, making it more convenient to access screening results through customized views.
Biomedical Informatics Investigator (BMII) Tool: this tool, proposed in [ 58 ], is an interactive web-based tool with a learning knowledge base. It offers researchers a means to query structured, unstructured, genomic, and image data contained in a data warehouse.
Doc’EDS Search Tool: Doc’EDS (EDS = Entrepôt de Données de Santé in French, Health Data Warehouse in English), presented in [ 59 ] stands as a search tool developed on the clinical data warehouse platform at Rouen University Hospital. This tool serves as a multilevel search engine that effectively combines both structured and unstructured data. Implemented as a web application, Doc’EDS is coded in Java EE and operates on a Tomcat web container. Its functionality relies on a Lucene index and encompasses various additional tools for visualizing, analyzing, and exporting results. Moreover, it offers essential analytics features and semantic utilities. The foundation of Doc’EDS lies in a document-oriented database, where each document corresponds to unstructured data documents from the CDW.
Prediction Tool Integration: Unlike the other tools, a predictive tool was built and integrated with CDR based on pattern discovery for AKI as a case study. The tool was trained on 70% of consecutive patient records with three knowledge incorporation modes. Initially, the pre-mode, or pre-data-driven mode, adopted a purely data-driven approach without integrating any prior knowledge. Subsequently, the in-mode, or clinician interactive mode, provided clinicians with the ability to interactively view and edit existing patterns. This involved adding or removing variables and choosing variable values based on their domain knowledge. Finally, the post-mode, or clinician-refined mode, allowed clinicians to further refine patterns solely based on their knowledge. This refinement process could include manual adjustments to numeric values in the pattern or optimizing the matching ratio, without referencing the training data.
Table 5 presents the summary of the different tools reviewed for clinical data management including their respective functions and limitations.
The tools discussed in this review primarily focus on data querying, mapping, and prediction within clinical data management. From Table 5 , it can be seen that they do not cover more complex data analytics, machine learning, advanced statistical reporting, or data visualization, which are essential functions for in-depth clinical data analysis and research. Therefore, there is a need for further tools or integrations to address these advanced analytical needs in clinical data management.
7 Security and privacy issues in CDR development
CDRs play a crucial role in modern healthcare by centralizing and managing a vast amount of sensitive patient information. However, their implementation raises several security and privacy concerns that must be addressed to ensure the confidentiality, integrity, and availability of the stored data. Security is a paramount concern in the development of CDRs as medical systems become more interconnected and integrated across different hospitals. Safeguarding clinical data from potential threats such as hackers, malware, and fraud is crucial to maintaining the integrity and confidentiality of patient information [ 65 ]. This section, thus, discusses the security and privacy issues in the development of the CDR. The several attacks and mitigations that have been proposed in literature as well as previous reviews that have been done were also presented.
7.1 Security issues in CDR
Several key security concerns in CDR development include the vulnerability to data breaches and unauthorized access, making CDRs attractive targets for cybercriminals due to the wealth of personal and medical information they contain [ 66 ]. To mitigate this risk, robust measures like implementing access controls, encryption protocols, and continuous monitoring are essential components of a comprehensive security strategy. Interoperability challenges pose another security concern as CDRs interface with various healthcare systems and applications, potentially introducing vulnerabilities during data transfers. Securing data exchange through standardized protocols and implementing strong encryption during transmission is vital to prevent interception and tampering. Additionally, maintaining data integrity and quality assurance is critical for informed decision-making in healthcare. Robust data validation mechanisms, regular audits, and the implementation of checksums or hashing techniques are essential to detect and prevent intentional or unintentional alterations to patient records. Moreover, regulatory compliance, particularly with privacy regulations such as HIPAA, is imperative, requiring stringent access controls, encryption, and audit trails to align with legal requirements. Addressing these security issues is imperative to ensure the ethical and successful utilization of CDRs in healthcare while upholding patient privacy and data security.
7.2 Privacy issues in CDR
Key privacy issues in CDR development include the risk of patient identifiability, as CDRs often house Personally Identifiable Information (PII) like names and addresses as well as several non-PII data items, which can be used to identify a patient. The potential for patient re-identification, even after de-identification attempts, poses a privacy risk, emphasizing the importance of robust de-identification techniques, such as anonymization and pseudonymization, to protect patient identities while preserving data utility for research and analysis [ 67 ]. Furthermore, non-PII can become PII whenever additional information is made publicly available, in any medium and from any source, that, when combined with other available information, could be used to identify an individual. E.g. quasi-identifiers (e.g., race) that can be combined with other quasi-identifiers (e.g., date of birth) to successfully recognize an individual [ 68 ]. The United States National Institutes of Health (NIH), Department of Health and Human Services has provided the HIPAA privacy rule guidelines for exempting Eighteen (18) identifiers that could have potential of impact in identifying protected health information [ 69 ]. Mitigation approaches to minimize risk for identification of individual health information is provided in [ 70 ].
Access controls and prevention of unauthorized use are critical privacy considerations, as unauthorized access to CDRs could lead to privacy breaches and malicious data use. Mitigation measures involve implementing stringent access controls, role-based permissions, and continuous monitoring of user activities. Additionally, privacy concerns extend to data sharing and consent practices, particularly as CDRs facilitate data sharing among healthcare entities. Ensuring patient consent, transparency in data-sharing practices, and clear privacy policies are paramount to building trust among patients regarding the use of their health information. Furthermore, compliance with privacy regulations such as HIPAA or GDPR is imperative for CDRs, involving the implementation of privacy-by-design principles, regular privacy assessments, and alignment of data handling practices with legal requirements. Proactively addressing these privacy issues enables healthcare organizations to build a foundation of trust, fostering responsible and ethical use of Clinical Data Repositories while maximizing the potential benefits of centralized health data management.
7.3 Threats and attacks
CDRs face a myriad of security threats, necessitating a comprehensive understanding and mitigation strategy to preserve the confidentiality and integrity of healthcare data. A primary concern is the risk of data breaches, with CDRs being attractive targets for hackers seeking unauthorized access to valuable healthcare information. To counteract this, robust authentication mechanisms, encryption protocols, and continuous monitoring are crucial measures to prevent and detect unauthorized access, reducing vulnerability to breaches. Ransomware attacks pose another significant threat, potentially leading to system paralysis and disrupting patient care. Mitigation strategies involve regular data backups, network segmentation, and employee training to recognize and thwart phishing attempts [ 71 ].
Insider threats, whether intentional or unintentional, present additional security risks, emphasizing the need for strict access controls, regular security awareness training, and user activity monitoring within organizations. Interoperability risks also emerge as CDRs interact with diverse healthcare systems, creating potential vulnerabilities for attackers during data exchange. Ensuring secure interoperability through standardized protocols, robust encryption, and regular security assessments is essential to thwart attacks related to data interchange [ 72 ]. Effectively managing these security challenges requires a proactive approach, encompassing regular security audits, adherence to industry best practices, staying informed about emerging threats, and fostering a culture of security awareness among healthcare staff and stakeholders.
7.4 Mitigation techniques
Mitigating security threats and attacks in CDR management is paramount for the protection of sensitive healthcare information. A multifaceted approach, combining technical measures, well-defined policies, and user awareness initiatives, can significantly bolster the security posture of CDRs [ 73 ]. Robust access controls are pivotal, restricting access to authorized personnel and enforcing role-based permissions to ensure minimal necessary privileges. The incorporation of strong authentication mechanisms, including multi-factor authentication (MFA), adds an extra layer of security by requiring multiple forms of verification before accessing the CDR.
Encryption emerges as a fundamental mitigation technique, encompassing data at rest, in transit, and during processing, rendering unauthorized access futile without the proper decryption keys. Supplementing this, data loss prevention (DLP) measures help thwart the unauthorized transfer of sensitive information from the CDR environment. Regular security audits and continuous monitoring of CDR activities play a crucial role in early threat detection. Intrusion detection systems, log analysis, and anomaly detection mechanisms identify unusual patterns, enabling timely responses to potential security incidents. Furthermore, user education and awareness initiatives are imperative, with regular training programs helping users recognize phishing attempts, emphasizing strong password management, and enhancing awareness of social engineering tactics [ 72 , 74 ]. By fostering a culture of security awareness, organizations can mitigate the risk of insider threats and empower users to contribute to the overall security of the CDR. In summary, a comprehensive security approach in CDR management encompasses access controls, encryption, continuous monitoring, and user education, collectively fortifying the defense against security threats and attacks on patient data.
7.5 Review of related works
The study by Jayanthilladevi et al. [ 75 ], analyzed healthcare data breaches and their key causes, as well as the function of PHI and HIPAA in discouraging data breaches, and then presented a biometric security-based healthcare system for identification and authentication. Conclusions were obtained that biometrics protects patient data and privacy in the healthcare industry. Furthermore, HIPAA security, privacy, and breach notification requirements protect health information security and confidentiality and may grant humans some personal health information rights.
To secure patient personal information, the study of Kong and Xiao [ 76 ] advocated utilizing a combination of symmetric block ciphers, asymmetric ciphers, and cryptographic hashing methods. The suggested privacy protection approach's effectiveness and innovation are based on message-level data encryption, a key caching system, and a cryptographic key management system that is scalable to clinical data warehouse buildings with any size of medical data. Afterward extensive testing and evaluation, it was determined that the proposed method could successfully secure confidential data in a clinical warehouse.
To address the problem of security and privacy breaches in electronic healthcare systems. The work of Ajayi et al. [ 77 ] suggested and implemented a security and privacy architecture in the Methodist Environment for Translational and Outcomes Research (METEOR). METEOR, which was created at Houston Methodist Hospital, is made up of two parts: an enterprise data warehouse (EDW) and a software intelligence and analytics (SIA) layer. According to this approach, the best way to preserve patient privacy is to deploy a systematic combination of technologies and best practices such as technical de-identification of data, restricted data access, and security measures in the underlying technical platforms. The findings indicated that the proposed security approach has little potential to breach data security or allow unauthorized access to protected patient health information. However, it is just for an enterprise-based clinical data warehouse. Table 6 presents a summary of the related works in terms of the pros and cons of the proposed approach to ensure security and privacy.
8 Role of artificial intelligence and federated learning in clinical data repository
The role of artificial intelligence in our day-to-day activities in today’s world cannot be underestimated, this section thus presents the role of artificial intelligence in a clinical data repository and previous works that have been done on it.
8.1 Artificial intelligence in CDR
Artificial Intelligence significantly enhances healthcare data management, analysis, and decision-making within CDRs. AI plays a crucial role in various aspects, including data analysis and interpretation, where algorithms analyze vast clinical datasets, extracting patterns and insights that might be challenging for human analysis. Machine learning models within AI contribute to diagnostic and treatment planning by identifying correlations and making predictions based on historical data [ 78 ].
In addition, AI provides essential clinical decision support in CDRs, offering relevant information, treatment suggestions, and potential diagnoses to healthcare professionals. This assists in making more informed decisions, ultimately improving the accuracy and effectiveness of medical interventions. AI's capabilities extend to predictive analytics, personalized medicine, and image and signal processing within CDRs, enabling forecasting of patient outcomes, tailoring treatment plans, and aiding in the detection of abnormalities in medical images [ 79 ]. Furthermore, AI contributes to maintaining data integrity through quality assurance processes and enhances security by detecting unusual patterns, ensuring efficient, accurate, and patient-centric healthcare delivery [ 80 ].
8.2 Federated machine learning in CDR
Federated Machine Learning (FML) technology plays a pivotal role in enhancing CDRs by enabling collaborative learning across various healthcare centers while ensuring privacy and security. In this approach, diverse local machine-learning models are developed using distributed training data from different health facilities, as illustrated in Fig. 8 . Each health center uses its unique dataset to train the machine learning algorithm, generating local models. Importantly, these datasets remain within the respective centers, preserving data privacy. Through secure exchanges of model training parameters, a shared global model is created without centralizing the training data, bolstering security. During this process, local models are trained using heterogeneous or homogeneous clinical datasets without compromising data privacy. The encrypted parameters ensure security during parameter exchanges, upholding data protection. The resulting global model, derived from collaborative learning, integrates back into local machine models, empowering healthcare professionals like doctors, physiotherapists, radiologists, and laboratory scientists to make informed decisions based on updated local models and predictions. The FML approach significantly contributes to data security and overall advancement in leveraging distributed clinical data within CDRs.
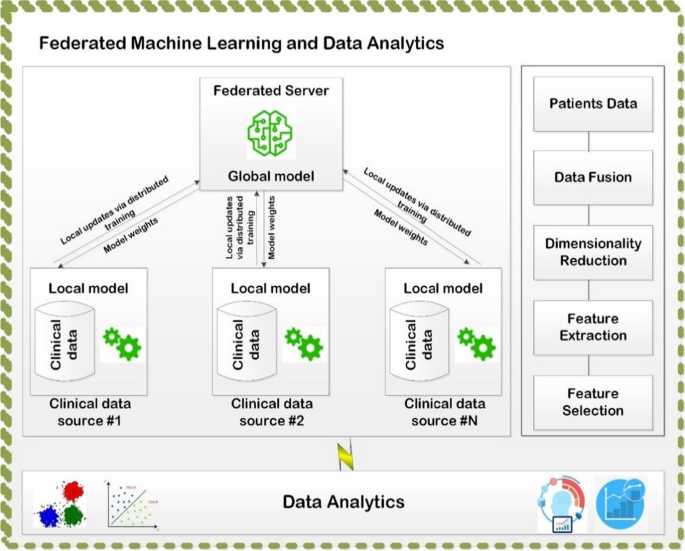
Architecture of a federated machine learning system
Formally, during the distributed training phase of the federated learning system, the goal is to minimize the cost function given as Eq. ( 1 ),
where m is the total number of local devices where the clinical data resides, \({p}_{k}\ge 0\) and \(\sum k{p}_{k}=1\) , and \({F}_{k}\) is the local objective function for the kth device. In most cases, the local objective function is often defined in terms of empirical risk over the local clinical data. This gives a function as Eq. ( 2 ),
where \({n}_{k}\) denotes the number of samples available locally, \({p}_{k}\) represents the user-defined term, which specifies the relative impact of each device, considering two natural settings being \({p}_{k}=(1/n)\) or \({p}_{k}=({n}_{k}/n)\) where \(n=\sum {kn}_{k}\) is the total number of samples.
8.3 Review of related works
AI has been introduced and utilized in several aspects of the clinical data repository for activities such as collection, analysis, processing, and classification of data, as used in [ 81 ] where the development of a standardized interoperable repository and intelligent applications, that can be produced in large quantities to help patients receive prompt treatment by analyzing radiological pictures and reports was proposed, coupled with clinical records under FHIR to allow gathering data from different sources in a distributed fashion. The development of data mining services through a WebApp that uses this repository that focuses on a specific pathology for achieving high impact both on the public and private healthcare systems was also proposed, considering the technical and institutional feasibility. However, only a prototype was developed.
A visual analytics tool integrated with CDR based on pattern discovery was developed by Li et al. [ 5 ], to solve the issue of missing data and concept drift in the existing CDR and EHR. A case study on contrast-related acute kidney injury (AKI) was demonstrated and the proposed system can be calculated in real-time to identify high-risk patients because it was developed from the data available on the conventional CDR and EHR systems. Although the system demonstrated a competitive performance to the conventional models and is user-friendly to physicians, the system has some limitations. The dataset is gotten from a single source which can thus introduce bias and lack of generalization. Hence, it needs to be enhanced and evaluated with more case studies as well as investigated into extra variables to improve AKI prediction.
In the work of Johnson et al. [ 82 ], an overview of the issues associated with collection and preprocessing mainly; compartmentalization, corruption, and complexity of critical care data was presented, and the machine learning strategies that have been applied to solve them were outlined. However, most of the developed systems have loopholes. Hence, the study recommends and discusses the enhancement of the subject of data analytics in critical care, that is, careful consideration of the compartmentalization, corruption, and complexity during the collection and processing of data. To demonstrate the potential of using machine learning algorithms for heterogeneous data such as clinical routine data sets. The effectiveness of machine learning algorithms for computer-aided identification of dementia based on T1 Weighted Imaging Magnetic Resonance Imaging (T1w MRI) was examined by Bottani et al. [ 83 ], by leveraging a real-world clinical routine cohort from a clinical data repository. However, the study utilized datasets from only one clinic, which can thus introduce bias and lack of generalization. Additionally, it focused only on MRI-based methods which might not encompass the full spectrum of diagnostic tools for dementia. A domain-independent, ontology-based clinical data warehouse for medical research was presented in [ 84 ]. It was created using a generalized metadata paradigm and can accommodate both the current domain ontology and the relevant research data. On this basis, a system may be created that can handle data with any structure while also acting to the user as though it were specifically designed for the given application. However further research is needed on how to extract ontology-guided information from unstructured or semi-structured data. To address the gaps in the existing CDRs, A novel ML technique was proposed in [ 85 ]. Data of different formats, such as, structured, unstructured, semi-structured, image, and pathological data was extracted from a data warehouse in the healthcare domain. After ETL stages, the data was systematically selected using machine learning techniques for further decision-making processes. However, more work needs to be done in the research area.
To meet patient needs and make storage decisions quickly, even with data streaming from wearable sensors, in real-time. The study of Uddin et al. [ 86 ] suggested a predictive model for the storage of health data. A machine learning classifier that understands the mapping between the properties of health data and features of storage repositories was used to develop the model and a training set that was created artificially using correlations found in tiny samples of expert data was employed. Experimental results showed that the machine learning method applied is effective. However, the model still needs to be improved. To help provide a set of tools that may effectively address core medical needs and improve the quality of patients’ care, the relevance of the Internet of Things (IoT) in CDRs in terms of how data are being collected, how they are analyzed and stored, and its impact in terms of security and privacy-related issues was analyzed in [ 87 ]. Conclusions were drawn that the biggest challenge from the regulatory perspective would be the standardization of the data management process across institutes, and the development of regulations to define the procedures to be followed and the data standards. And for the security aspect, the biggest hurdle would be the planning and implementation of highly secured infrastructure that will protect the clinical data from hackers.
Several works of relevant literature in the health domain were provided and the implications for cancer care were described by Cheng et al. [ 88 ]. An example of how to apply these two technologies, ML and Blockchain Technology (BCT) in cancer survivorship care was given. The study then indicated that both technologies can be integrated feasibly and effectively. However, wider exploration and deeper integration of these most notable technologies in cancer care are recommended. Table 7 presents the taxonomy of artificial intelligence in clinical data repository.
As indicated by the table provided above, machine learning offers various advantages in the healthcare domain. However, it's essential to acknowledge that the application of machine learning in certain healthcare areas comes with inherent limitations. These limitations can include challenges related to data privacy, model interpretability, and the need for large and diverse datasets to train accurate models. Despite these constraints, the potential benefits of machine learning in healthcare, such as improved diagnostics and personalized treatment, continue to drive innovation in the field.
9 Existing clinical data repository projects in Africa
Adoption, research work, and development of CDR in Nigeria and Africa have been gradual. However, some of these works are reviewed in this section.
9.1 A. Nigeria National Data Repository (NDR)
The design and development of a Nigeria National Data Repository (NDR) for Human-deficiency Immune Virus (HIV) was conducted in [ 90 ]. The study described how the NDR allows the utilization of data for real-time program monitoring, service delivery, and implementation of strategies to promote program improvements for the Nigeria HIV program. The use of the NDR for HIV surveillance, including HIV recency surveillance, HIV case-based surveillance, and mortality surveillance was also discussed. However, more work still needs to be done to enhance patient identifiers, record matching, and the use of open standards for secure data exchange between other non-HIV-specific EMRs and the NDR.
9.2 B. OpenMRS-Ebola
An Electronic Medical Record system named OpenMRS-Ebola was designed and developed in [ 91 ] for the Kerry Town Ebola Treatment Center (ETC) in Sierra Leone. The system is very appropriate for patient records in an ETC because it allows for instant communication and access to full clinical histories in both zones as well as an easy UI interface. However, the proposed system must be integrated with a well-designed and tested set of interoperable electronic systems ready for deployment with appropriate hardware and training materials to know and understand its real impact.
This review was able to highlight a few developed in Nigeria and Africa at large which are Nigeria NDR and Open MRS-Ebola for HIV/AIDS and Ebola respectively. Thus, there is a need to develop more CDRs that consider different ailments and can be updated in real-time, particularly in Nigeria. Table 8 presents a summary of the different developed CDRs in Africa, that were not captured in the systematic review, based on the name, country, use case, year developed, and web link.
10 Current challenges in the development of clinical data repository
Awareness of the potential benefits and challenges of sharing individual clinical data, how to overcome the challenges, and future direction recommendations in the field for the clinical pharmacology community was presented in [ 92 ]. The study highlighted ethical challenges such as privacy of individuals, data ownership, and control as one of the major challenges affecting clinical data sharing. However, the study only focused on the benefits and challenges of clinical data sharing. To advance knowledge, enhance care, and move research forward, the study of Bocquet et al. [ 93 ], presented the challenges of implementing a comprehensive clinical data warehouse. Conclusions were drawn that although large-scale use of these data can lead to significant technological and medical progress, it also raises many issues such as heterogeneity, structuration, interoperability temporality, purpose of use, quality, and storage as well as the issue of the legal and ethical framework for reusing these data. A brief overview of informatics approaches for clinical research, particularly research and quality improvement initiatives germane to Colon and Rectal Surgery was presented in [ 94 ].
Current challenges and key areas for improvement such as unstructured data and data heterogeneity were identified. The issues and challenges to developing a successful CDW were discussed in [ 95 ]. The study stated data integration as an important issue that performed the intergradations of large volumes of data from several sources and built a robust technique to solve all data quality problems at each phase of the ETL process. Furthermore, the ETL process must meet special requirements to ensure the quality of the data in the CDW. However, poorly characterized mathematically, the difficult data type for mining, difficulty in determining hidden relationships, as well as security and privacy are also issues to be considered when developing a CDW.
A brief overview of the secondary uses of an Electronic Health Record system as well as a systematic review was presented in [ 96 ] to analyze their effect on patients’ privacy. Also, the GDPR as well as HIPAA regulations were critically analyzed and their possible areas of improvement were highlighted, taking the wide adoption of technology and different secondary uses of EHR into consideration. However, the study focused only on the secondary uses of an EHR. Strategies for addressing the challenges presented by online healthcare platforms, in particular, the data processing requirements presented by the associated clinical medical records (CMR) repositories were explored in [ 97 ]. Personalized patient analytics strategy was argued to have the capacity to address the clinical big-data challenge by presenting and utilizing data in a patient-specific manner such as would be required in actual clinical practice. Conclusions were drawn that the strategy is appropriate for effectively leveraging clinical medical record databases for decision support. However, the study focused only on the data processing requirements. Several challenges such as security and privacy, data quality, data heterogeneity, unstructured data, etc. have been identified as challenges that can hinder the development of a CDR.
10.1 Security and privacy challenge
Security and privacy challenges in CDRs are critical due to the sensitive nature of the patient health information they contain. Safeguarding data confidentiality, integrity, and availability is vital for maintaining trust and compliance with regulations like HIPAA and GDPR [ 98 ]. Data breaches pose a significant threat, with CDRs being lucrative targets for cyberattacks. Insider threats, whether intentional or unintentional, can lead to unauthorized access or data disclosure. Data encryption, both at rest and in transit, is essential but can introduce performance and key management challenges [ 99 ]. Access control must be carefully managed to prevent data exposure due to misconfigurations or weak policies. Data redundancy, necessary for recovery, must be maintained securely. Data integrity must be ensured to prevent tampering or corruption. Integrations with third-party systems can introduce vulnerabilities. On the privacy front, challenges such as obtaining informed patient consent for data collection and sharing, adhering to data minimization principles, and addressing risks of re-identification even after de-identification efforts. Monitoring data access through logs while protecting patient identities is crucial, as is ensuring secure data-sharing mechanisms. Patient portals need protection from unauthorized access, and cross-border data flow must navigate varying privacy regulations. Determining data retention policies and securely disposing of unnecessary data is another privacy challenge [ 76 ].
To tackle these issues, a comprehensive approach is essential, including robust access controls, encryption, regular security audits, staff training, and compliance with regulations. Privacy-enhancing technologies like differential privacy and federated learning can balance data utility with patient privacy in CDRs. Continuous monitoring and adaptation to evolving threats and regulations are crucial for maintaining the security and privacy of clinical data.
10.2 Data quality challenge
Data quality challenges in CDRs pose significant concerns with direct implications for patient care, research outcomes, and healthcare decision-making. These multifaceted challenges encompass issues like ensuring data completeness to avoid inaccuracies in diagnoses and treatments due to missing information [ 100 ]. Maintaining data accuracy is vital, as errors during entry, transcription, or integration can lead to misdiagnoses and compromised research findings. Data consistency is crucial to prevent confusion arising from non-uniform data formats or coding systems [ 101 ]. Timeliness is essential for informed healthcare decisions, and outdated data can lead to inappropriate treatments. Relevance must be considered to avoid cluttering the system with irrelevant data. Data integrity is vital to prevent unauthorized alterations, while interoperability challenges arise from aggregating data from various sources [ 102 ]. Effective data governance, regular cleaning processes, and robust security measures are essential components of overcoming these challenges, ultimately ensuring the reliability of CDRs for improved healthcare outcomes and research reliability.
10.3 Data heterogeneity
Data heterogeneity in CDRs poses significant barriers to the effective integration and utilization of healthcare data. The complexities arise from the diversity of data sources, formats, and standards within the healthcare ecosystem. CDRs must grapple with diverse data sources, ranging from structured data like EHRs to unstructured data like physician's notes and radiology images [ 103 ]. Moreover, variations in medical coding standards, data encoding, units of measurement, and even semantic differences across institutions compound the challenge [ 104 ]. Ensuring data quality and compliance with privacy regulations amidst this heterogeneity is imperative. Strategies such as data standardization, normalization, interoperability frameworks, semantic mapping, advanced data integration platforms, and robust data governance practices are deployed to tackle these challenges. Addressing data heterogeneity is pivotal for CDRs to unleash their full potential in enhancing patient care and advancing healthcare research [ 105 , 106 ].
10.4 Unstructured data challenges
Unstructured data poses significant challenges in CDRs due to its diverse formats, volume, semantics, and privacy concerns [ 107 ]. Unstructured data encompasses a wide range of information types, including clinical narratives, medical images, handwritten documents, and more, making integration and analysis complex. Data quality, privacy, and retrieval can be particularly challenging in unstructured data [ 108 ]. To address these issues, healthcare organizations leverage NLP, data governance practices, advanced analytics, and semantic standards. NLP helps extract structured information from clinical narratives, while data governance ensures quality and compliance. Advanced analytics and machine learning enable insights from unstructured data, and semantic standards promote standardization. Overcoming these challenges is vital for CDRs to unlock the potential of unstructured data, enhancing patient care and advancing healthcare research [ 109 ].
11 General analysis and discussions
This section presents a discussion and analysis of the different aspects of CDR as reviewed in the previous sections. We reviewed and analyzed primary studies based on the domain of CDR architecture and metadata, regulations, standards, and guidelines in CDR, tools for CDR, security and privacy issues in CDR development, roles in AI and ML in CDR, existing CDR projects in Africa, particularly in Nigeria, and finally, challenges encountered in CDR development.
Understanding the acceptance of research findings is crucial for their effective implementation and impact. In this discussion we delve into the perspectives of the beneficiaries of our research, exploring their tendencies in embracing the insights presented by answering the questions who are the beneficiaries of the research? How to communicate the research findings to them, and what if the research will not be acceptable or credible to them?
Question : Who are the beneficiaries of this research?
Answer : The beneficiaries of this research are mainly the community members and other stake holders such as Clinical Professionals, Developers and IT Professionals, Healthcare Institutions, Policy Makers and Regulators, and Research Community.
Question : How to communicate the research findings to them?
The research findings can be communicated to the community members through the following:
Patient Education Materials: Develop patient-friendly materials explaining the benefits of CDRs and how they can contribute to improved healthcare outcomes. Distribute these materials through healthcare facilities, community centers, and online platforms.
Community Workshops and Seminars: Organize workshops and seminars in community centers or local healthcare facilities to educate community members about CDRs, data privacy, and the importance of informed consent.
Community Engagement Events: Host community engagement events where researchers and healthcare professionals can interact directly with community members, answer questions, and address concerns about CDRs.
Participatory Research: Involve community members in the research process through participatory approaches such as community-based participatory research (CBPR), ensuring that their perspectives and needs are taken into account.
Digital Outreach: Utilize digital platforms such as social media, websites, and online forums to share information about CDRs and research findings with a wider audience, including community members who may not have access to traditional healthcare settings.
Communicating the research findings to stakeholders requires tailored approaches:
Peer-Reviewed Publications: Publishing research findings in reputable academic journals ensures visibility and credibility within the research community.
Conferences and Workshops: Presenting research findings at conferences and workshops allows for direct engagement with stakeholders, fostering discussion and collaboration.
Policy Briefs and Reports: Developing concise policy briefs and reports summarizing key findings and implications facilitates communication with policy makers and regulators.
Training Programs and Workshops: Organizing training programs and workshops enables knowledge dissemination and capacity building among healthcare professionals and IT personnel.
Media and Public Outreach: Engaging with media outlets and conducting public outreach activities helps raise awareness about the importance of CDRs and the potential benefits for healthcare delivery.
Question : What if the research will not be acceptable or credible to them?
Answer : If the research findings are not acceptable or credible to stakeholders, it's essential to address their concerns through transparent communication and further evidence-based research:
Engage in Dialogue: Listen to stakeholders' feedback and concerns, and engage in open dialogue to understand their perspectives.
Provide Additional Evidence: Conduct further research to address any gaps or limitations identified by stakeholders, providing additional evidence to support the research findings.
Seek Collaboration: Collaborate with stakeholders to co-design research studies or initiatives that address their specific concerns and priorities.
Highlight Benefits and Implications: Clearly communicate the potential benefits and implications of the research findings, emphasizing their relevance and importance for improving healthcare outcomes.
Address Ethical Considerations: Address any ethical considerations raised by stakeholders and ensure that research methodologies and practices adhere to ethical standards and guidelines.
RQ2: What is the state-of-the-art clinical data repository including its architectures, types, data sources, and metadata information?
The review of state-of-the-art CDRs in response to RQ2 reveals a diverse range of architectural approaches, data sources, and metadata information in the literature. Various innovative architectures have been proposed, such as Oracle NoSQL-based, NLP, cloud-based, and Hadoop-based architectures, each offering unique advantages. These architectures show promise in improving interoperability, meeting requirements, and enhancing data management. However, a common limitation is the lack of real-world validation, with many of these approaches remaining in the theoretical phase. In terms of data sources, the review identifies a wide array of inputs, including patient records, clinical trial data, pharmaceutical information, health surveys, and claims data. This diversity underscores the potential richness of data available for CDRs, highlighting their significance in healthcare research. Overall, while innovative CDR architectures and data sources are abundant, practical implementation and validation in clinical settings remain essential challenges.
RQ3: Are there existing regulations, guidelines, and standards for clinical data repository development?
Yes, there are existing regulations, guidelines, and standards for CDR development, and they play a critical role in ensuring the responsible management of patient data in a medical setting. These regulations and standards are essential to achieving interoperability, data security, privacy protection, and maintaining electronic data capture standards. In the context of CDR, the review indicates that several standards have been proposed or recognized. Notably, the CDASH stands out as a fundamental standard that defines the basic norms for data collection, ensuring a standardized approach in this aspect. Additionally, HL7 RBAC standards are highlighted for efficiently defining access control rules concerning roles and permissions in CDM. Furthermore, the review highlights that the studies reviewed prominently utilize different standards, particularly in the realms of access control, interoperability, and quality measurements. This highlights the importance of not only having standards but also effectively applying them in CDR development to enhance security, data quality, and data accessibility. The integration and application of these standards play a significant role in shaping efficient and compliant clinical data management systems.
RQ4: What are the existing tools for clinical data management?
In clinical data management, tools play a pivotal role in ensuring robust data management practices. These tools serve various purposes, including maintaining audit trails, managing discrepancies, creating user access restrictions, facilitating data entry, supporting medical coding, aiding database design, and conducting quality checks. They contribute to the systematic and controlled management of clinical data, ensuring data integrity and security. However, the review reveals that the existing tools for CDM, such as the BMII tool, patient-screening tool, and Doc’EDS search tool, are primarily focused on enhancing the efficiency and effectiveness of data querying within data warehouses or repositories. While these tools excel in their querying capabilities and contribute to improved data retrieval and analysis, they are primarily oriented toward specific functionalities related to data access and retrieval. Therefore, it is important to recognize that while these tools offer valuable support for specific aspects of CDM, the landscape of CDM encompasses a broader spectrum of functionalities and requirements. These include not only data querying but also complex data analytics, ML, advanced statistical reporting, data visualization, data entry and validation, audit trail maintenance, and more. The existing tools, while addressing critical needs, may need to be complemented with additional tools and approaches to comprehensively cover the diverse and evolving requirements of CDM.
RQ5: What are the security and privacy issues in CDR development?
RQ5 explored the intricate landscape of security and privacy concerns within the realm of CDR development, highlighting the paramount importance of addressing these issues in a heterogeneous and data-rich healthcare environment. The significance of pre-emptively assessing security and privacy stems from the vulnerability of CDRs to an array of potential threats, including data breaches and hacking attempts, especially when accessed by diverse personnel from various healthcare institutions. Building patients' trust in CDRs necessitates robust security measures to ensure data confidentiality and secure storage. This review presents several proposed solutions designed to fortify the protection of patients' data within CDRs. Notably, cryptography emerges as a pivotal tool in safeguarding patient information, with approaches encompassing a combination of symmetric block ciphers, asymmetric ciphers, and cryptographic hashing methods. Additionally, the implementation of key caching systems and cryptographic key management systems contributes to enhancing data security. Beyond cryptography, the adoption of biometric security measures for authentication and identification adds an extra layer of protection, further fortifying patient data and privacy. This review underscores the efficacy of biometrics in safeguarding sensitive healthcare data and reiterates the critical role of regulations like HIPAA in preserving health information security and confidentiality. By comprehensively addressing these security and privacy challenges, CDR development can continue to evolve while respecting patient rights and instilling trust in the healthcare ecosystem.
RQ6: What is the role of artificial intelligence in CDR development?
RQ6 looked into the critical role of AI in CDR development, highlighting its multifaceted influence in revolutionizing healthcare data management. AI has emerged as an indispensable tool across various aspects of CDRs, contributing significantly to the collection, analysis, processing, and classification of healthcare data. In particular, AI has made substantial strides in cancer care, where it aids in early detection, precise diagnosis, and personalized treatment planning. By leveraging advanced algorithms and machine learning models, AI can discern intricate patterns and identify potential indicators, enabling healthcare practitioners to intervene at earlier stages and enhance patient outcomes. Furthermore, AI's transformative impact extends to medical imaging, notably benefiting imaging modalities such as T1-weighted imaging. AI-driven image processing techniques improve the quality and accuracy of medical images, empowering clinicians with enhanced visualization of anatomical structures and pathological conditions. However, despite AI's remarkable potential, this review underscores the need for continued research and development in the field. Addressing concerns related to data privacy, security, ethics, and regulatory compliance remains paramount. Additionally, ongoing refinement of AI algorithms ensures their reliability and adaptability to meet the evolving challenges of CDRs. AI stands as a pivotal force in shaping the future of CDRs, promising to optimize patient care and advance medical research.
RQ7: What are the existing clinical data repository projects in Nigeria or Africa?
In RQ7, the existing CDR projects in Africa were highlighted and reviewed, shedding light on the progress, challenges, and opportunities in the adoption of these critical healthcare data management systems. The region's journey towards embracing CDRs has been characterized by a gradual transition, influenced by a myriad of challenges. These challenges include the lack of robust healthcare infrastructure, particularly in remote rural areas where access to advanced technology remains limited. Additionally, the scarcity of funding, inadequate clinical datasets, and a shortage of skilled manpower have posed substantial hurdles in establishing comprehensive CDR systems.
Despite these challenges, this review identifies notable CDR projects that have emerged in Africa, exemplifying the region's commitment to advancing healthcare data management. Among these projects are the Nigeria NDR in Nigeria, the NDMC in Ethiopia, KeHMIS in Kenya, and Open MRS-Ebola in Sierra Leone, which have demonstrated the potential of CDRs in addressing specific healthcare needs, particularly in managing HIV/AIDS, COVID-19, and Ebola data. Nevertheless, the review emphasizes the need for more research efforts and continued CDR development across Africa. This is not only essential for overcoming the existing challenges but also for harnessing the transformative power of CDRs in improving healthcare delivery, promoting medical research, and ultimately enhancing the well-being of the region's population.
RQ8: What are the current challenges in the development of clinical data repository?
RQ8 provides a comprehensive examination of the contemporary challenges confronting the development of CDRs. Despite the substantial progress in CDR research and development, several formidable obstacles continue to impede their full realization. A predominant challenge highlighted in this review is the paramount concern for security and privacy. In the age of digitized healthcare, safeguarding sensitive patient information from potential breaches, hackers, and unauthorized access remains a critical concern. The integration of robust security measures and privacy-preserving protocols is pivotal in ensuring the confidentiality and integrity of clinical data within CDRs.
Another significant hurdle in CDR development is the multifaceted issue of data quality. The inherent variability and potential inaccuracies in healthcare data can compromise the reliability and utility of CDRs. Achieving high data quality standards necessitates meticulous data cleansing, validation, and normalization processes, demanding substantial resources and expertise. Data heterogeneity, characterized by the diverse formats, structures, and sources of clinical data, poses a significant challenge in CDR development. Effectively harmonizing and integrating these disparate data types require innovative strategies and standardized approaches. Furthermore, the incorporation of unstructured data, such as clinical narratives and free-text entries, into CDRs introduces complexities in data extraction, structuring, and semantic interoperability. Overcoming these hurdles demands sophisticated NLP and ML techniques for meaningful data extraction and integration.
Moreover, the challenges extend to issues of data temporality, interoperability, and purpose of use. Clinical data are often time-sensitive, and capturing temporal changes accurately is crucial for comprehensive patient care. Ensuring data interoperability across different healthcare systems and platforms remains a persistent challenge, hindering seamless data exchange and integration. Additionally, aligning the purpose of CDRs with the needs of diverse stakeholders, including healthcare providers, researchers, and policymakers, requires careful planning and governance. Finally, the review underscores the legal and ethical dimensions of CDR development, emphasizing the need for a robust framework that navigates issues of consent, data ownership, and responsible data sharing. Addressing these multifaceted challenges is essential for unlocking the full potential of CDRs in improving healthcare delivery, research, and patient outcomes.
12 Further research directions
This section presents further research directions for clinical data repositories in terms of development, deployment, and overall improvement.
12.1 Development of a Robust CDR for Nigeria
The healthcare data landscape in Nigeria has made considerable strides with the development of the Nigerian NDR. This repository has played a vital role in aggregating anonymous patient-level data from healthcare facilities across the country. The user-friendly dashboards of the NDR have proven invaluable for fulfilling program management data requirements. These dashboards provide automated evaluations that allow for data segmentation based on various factors, including sex, age groups, and different programmatic levels, spanning healthcare facilities, states, implementing partners, and at the national level. However, it is crucial to acknowledge that the NDR primarily focuses on HIV-related data, which aligns with its design and funding objectives. To comprehensively strengthen healthcare data management in Nigeria, there is an urgent need for the development of a versatile and robust CDR. This CDR should transcend specific diseases or conditions and be designed to accommodate diverse ailments while enabling real-time data updates. This would not only enhance the capacity to monitor and address a broader spectrum of health concerns but also foster a more comprehensive understanding of healthcare trends, patient demographics, and epidemiological patterns.
The application of Federated Learning (FL) in CDRs represents a ground-breaking paradigm shift in healthcare data management. FL's unique approach to machine learning enables collaborative research while upholding the utmost privacy and security of patient data. By allowing machine learning models to be trained on decentralized data sources without sharing sensitive raw data, FL aligns seamlessly with stringent healthcare data regulations, including the HIPAA and the GDPR. This privacy-preserving feature empowers healthcare institutions to collaborate on research initiatives without breaching data privacy boundaries, fostering an environment of trust and compliance. Despite its immense potential, FL's adoption in CDRs remains limited, presenting a significant untapped opportunity. Future research directions should aim to harness FL's capabilities to their fullest extent. This entails exploring how FL can revolutionize clinical data management by enabling secure, privacy-compliant data analysis and prediction. Additionally, the integration of FL for early detection and prediction of malignant diseases using data within CDRs could represent a pivotal breakthrough in the realm of healthcare.
12.2 Security and privacy models of CDR
The research emphasizes that security and privacy problems pose significant challenges in the context of CDRs. Data breaches orchestrated by hackers, malware, and fraudulent activities represent significant threats to the integrity and confidentiality of clinical data. Within the healthcare sector, where the sensitivity of patient information is paramount, ensuring data confidentiality is non-negotiable. Consequently, the pursuit of innovative and stringent security solutions remains an enduring challenge and top priority for the industry. Although notable progress has been made in the field of CDR security, the review highlights a continuous and pressing need for the development of a robust and tailored security and privacy model. This model should encompass a comprehensive approach, extending beyond the fortification of defenses against external threats. It should also proactively address internal vulnerabilities, mitigating the risks associated with potential data breaches from within. The development of such a model not only serves as a means to protect patient data but also upholds the trust and integrity of healthcare systems.
12.3 CDR awareness campaign
One of the critical findings obtained from this review is that there is very low adoption of CDR in the modern healthcare system. This can be seen in the Existing Projects in Africa section where only a few countries have developed a functional clinical data repository, particularly in Africa. This can be due to the lack of awareness about the benefits CDR can offer, security and privacy issues, tech phobia, and lack of adequate personnel with adequate knowledge to operate and sustain a CDR, among others. This, however, leaves a huge gap to bridge. Thus, there is a critical need for researchers, healthcare providers, policymakers, and other stakeholders to take several steps to create adequate CDR awareness campaigns. Such initiatives may involve implementing digital literacy training programs, discussion on CDR and its many benefits, advocating for increased usage of CDR in healthcare facilities, and enhancing the overall usability and user-friendliness of CDR interfaces, among other strategies. In addressing these challenges, a proactive approach is essential to promote the understanding and acceptance of CDR, paving the way for its seamless integration into the fabric of modern healthcare practices.
12.4 CDR research and development
CDR research and development involve a comprehensive investigation of the existing challenges and limitations encountered in current CDR implementations. These challenges, as identified and reviewed in this work, encompass issues such as security and privacy concerns, interoperability with diverse healthcare systems, data heterogeneity, and the optimization of the scalability and efficiency of CDR infrastructure. Consequently, there is a continuous need for research endeavors aimed at offering solutions to these persistent challenges. The goal is to streamline the development and implementation of diverse CDRs capable of housing clinical datasets for various ailments. Additionally, research efforts should be directed toward leveraging stored clinical datasets, and adhering to appropriate data privacy regulations, to develop intelligent predictive models. These models should be able to effectively detect and potentially mitigate terminal ailments at an early stage.
Limitations and assumptions:
Theoretical Architectural Models: Many proposed architectural models for CDRs lack real-world validation.
Limited Coverage in Nigeria and Africa: There is a gap in comprehensive CDRs in Nigeria and Africa, particularly beyond HIV/AIDS and Ebola data.
Limited Adoption of AI and ML: While there is potential for AI and ML in CDRs, adoption remains limited.
Roadmap for Future Research:
Validation of Architectural Models:
Limitation: Theoretical architectural models need real-world validation.
Assumption: Real-world validation ensures the effectiveness and reliability of architectural frameworks.
Roadmap: Conduct empirical studies and pilot implementations to validate theoretical models. Collaborate with healthcare institutions for testing and validation. Publish findings to bridge the theory–practice gap and inform future architectural developments.
Comprehensive CDR Development in Nigeria and Africa:
Limitation: Limited coverage of comprehensive CDRs beyond HIV/AIDS and Ebola data in Nigeria and Africa.
Assumption: Developing comprehensive CDRs can address broader health concerns and enhance data management capabilities.
Roadmap: Collaborate with healthcare stakeholders to design and implement versatile CDRs that accommodate diverse ailments. Conduct pilot studies to evaluate effectiveness and scalability across different healthcare settings.
Enhanced Adoption of AI and ML:
Limitation: Limited adoption of AI and ML in CDRs despite their potential benefits.
Assumption: AI and ML have the potential to revolutionize clinical data management and analysis.
Roadmap: Conduct research to explore the application of AI and ML in CDRs, focusing on data analysis, predictive modeling, and decision support systems. Develop frameworks and protocols for integrating AI and ML into existing CDR infrastructures. Collaborate with healthcare institutions to pilot AI and ML-enabled CDR systems and evaluate their efficacy.
Sustainable Roadmap:
Collaboration: Foster collaboration between researchers, healthcare institutions, and policymakers to ensure the relevance and applicability of research findings.
Long-Term Planning: Develop long-term research plans that address current limitations while anticipating future challenges and opportunities in healthcare data management.
Education and Training: Invest in education and training programs to build capacity among healthcare professionals and researchers in utilizing advanced technologies and methodologies for CDR development and management.
Ethical Considerations: Prioritize ethical considerations in research and development, particularly regarding data privacy, security, and patient consent.
Knowledge Sharing: Facilitate knowledge sharing and dissemination through conferences, workshops, and publications to foster a culture of collaboration and innovation in healthcare data management.
13 Lessons learned
This section presents the salient points and insights obtained from the review of the clinical data repository.
13.1 Lesson 1: most architectural models for CDR are still in the theoretical phase
From the systematic review presented, it can be seen that several architectural models have been proposed on different platforms, such as NLP, Oracle NoSQL-based, cloud-based, and Hadoop-based, among others. While these architectural models exhibit the potential to address critical system requirements, such as ensuring high performance, reliability, portability, easy maintenance, and interoperability, it was observed from the review that most of these models are yet to be validated or evaluated in real-world scenarios. While theoretical models provide a solid foundation for conceptualization, their true value is realized when subjected to real-world testing and validation. Bridging the theory–practice gap is imperative for advancing the field of architecture, as it ensures that proposed models can withstand the complexities and challenges inherent in practical, dynamic environments. As the technological landscape evolves, emphasizing the translation of theoretical models into practical solutions becomes paramount. A comprehensive approach that combines theoretical soundness with empirical validation will contribute not only to the credibility of architectural frameworks but also to their practical utility, fostering a more robust and adaptable technological ecosystem.
13.2 Lesson 2: limited awareness and adoption of CDR in healthcare environments
Despite the numerous advantages of CDR, which include providing a comprehensive medical history for patients, information on past procedures, and test results to prevent duplicate testing and care redundancies, as well as facilitating the development of intelligent prediction and risk algorithms for early ailment predictions and possible mitigations, the review highlighted a low level of awareness and adoption of CDR in healthcare settings. This can be seen with the low number of existing CDR projects in Africa. A significant number of clinics and healthcare centers still rely on manual data entry into software such as Microsoft Excel, which lacks inter-departmental collaboration capabilities. Consequently, the data tends to be disorganized and unsuitable for further processing. Addressing this issue requires comprehensive CDR campaigns and awareness programs aimed at promoting widespread adoption in various clinics and healthcare centers. This approach will not only foster improved collaboration among healthcare facilities but will also ensure the secure preservation of clinical data, making it easily accessible for further utilization.
13.3 Lesson 3: limited application of federated learning in existing CDR systems
AI plays a pivotal role in CDR, especially in functions such as data collection, analysis, processing, and classification, contributing to the development of intelligent models for early ailment detection and potential mitigation. Federated Learning (FL) holds promise in enhancing data analysis and processing by leveraging data from various healthcare centers securely. However, despite its numerous advantages, FL has yet to find widespread application in CDR. Existing CDR systems have not integrated federated learning into their framework, indicating a gap in the utilization of this decentralized learning approach for collaborative and secure data analysis. Expanding the integration of FL in CDR systems could offer substantial benefits, such as improved model robustness through diverse data sources, enhanced privacy preservation, and increased collaborative potential among healthcare institutions. This necessitates further exploration and advocacy for the incorporation of federated learning techniques into the existing CDR systems.
13.4 Lesson 4: CDR is susceptible to several security and privacy threats and attacks
Security and privacy are the two most pressing concerns particularly when it comes to clinical datasets because they are highly confidential and a breach could cause damages which can invariably lead to death. It is observed from this review that CDR is susceptible to several threats and attacks, these include, but are not limited to, unauthorized access, malware, ransomware, data manipulation, phishing attacks, etc. These vulnerabilities pose substantial risks and may hinder the widespread adoption of CDR. Furthermore, addressing these security challenges is not only crucial for the successful integration of CDR into healthcare systems but also imperative for maintaining patient trust and ensuring the integrity of medical data. Implementing robust security measures, such as encryption protocols, access controls, and regular security audits, will be essential to fortify CDR systems against potential threats, thereby fostering greater confidence in the utilization of clinical data repositories in healthcare settings.
14 Conclusions and future scope
The comprehensive systematic review of CDRs conducted in this paper sheds light on several pivotal aspects. CDRs offer numerous advantages, including the provision of longitudinal patient medical histories, reduction of redundant tests and care redundancies, and their potential to fuel the development of AI and ML models. The review encompassed seven different databases and addressed eight research questions. Key findings reveal that while various architectural models have been proposed, many remain in a theoretical phase without robust real-world validation. Research in the realm of CDR standards and regulations predominantly focuses on access control, interoperability, and quality measurements. Existing tools for CDM primarily center around data querying, highlighting the need for diversification in tool functionalities. Security and privacy concerns, particularly data breaches, emerged as significant challenges in CDRs, underlining the critical importance of robust protective measures. Artificial intelligence presents opportunities in CDRs, particularly in data analysis and model development. Notably, the review exposed a gap in comprehensive CDRs in Nigeria and Africa, which currently lack coverage for various ailments beyond HIV/AIDS and Ebola. Moreover, various challenges in CDR development were identified, emphasizing the need for further research works which were identified and discussed. This research work provides valuable insights for clinical professionals, developers, and the research community. It serves as a foundational resource for the development, enhancement, and evaluation of clinical data repositories, facilitating the advancement of healthcare data management and analysis.
Data availability
No datasets were generated or analysed during the current study.
https://www.sciencedirect.com .
https://pubmed.ncbi.nlm.nih.gov .
https://ieeexplore.ieee.org/Xplore/home.jsp .
https://www.springer.com/gp .
https://scholar.google.com .
https://www.mdpi.com .
https://dl.acm.org .
https://elementtechnologies.net/clinical-data-management-roles-steps-and-software-tools .
https://www.medidata.com/en/life-science-resources/medidata-blog/what-is-rtsm/ .
https://www.medidata.com/en/clinical-trial-products/clinical-data-management/rtsm/ .
Abbreviations
Artificial intelligence
California Consumer Privacy Act
Clinical Data Interchange Standards Consortium
Clinical data repository
Clinical data management
Case report form
Computerized tomography
Concept unique identifiers
Electronic data capture
- Electronic health records
Electronic medical record
Extraction, transformation, and load
European Union
Fast healthcare interoperability resources
Federated machine learning
General data protection regulation
Health Insurance Portability and Accountability Act
Health level seven
International Council for Harmonization
Integrated data repository
Internet of things
Picture archiving and communication systems
Protected health information
Laboratory information systems
Machine learning
Magnetic resonance image
National data repository
National health information database
Natural language processing
Role-based Access control
Research question
Qualified clinical data registry
Quality reporting document architecture
Software intelligence and analytics
Template relational mapping
Type-2 diabetes
Bali A, Bali D, Iyer N, Iyer M. Management of medical records: facts and figures for surgeons. J Maxillofac Oral Surg. 2011;10:199–202. https://doi.org/10.1007/s12663-011-0219-8 .
Article Google Scholar
Hamoud A, Hashim A, Awadh W. Clinical data warehouse: a review. Iraqi J Comput Inform. 2018;44:16–26. https://doi.org/10.25195/ijci.v44i2.53 .
Dainton C, Chu CH. A review of electronic medical record keeping on mobile medical service trips in austere settings. Int J Med Inform. 2017;98:33–40. https://doi.org/10.1016/j.ijmedinf.2016.11.008 .
Smith A, Nelson M. Data warehouses and clinical data repositories. In: Ball MJ, Douglas JV, Garets DE, editors. Strategies and technologies for healthcare information: theory into practice. New York: Springer; 1999. p. 17–31.
Chapter Google Scholar
Li Y, Chan TM, Feng J, Tao L, Jiang J, Zheng B, Huo Y, Li J. A pattern-discovery-based outcome predictive tool integrated with clinical data repository: design and a case study on contrast related acute kidney injury. BMC Med Inform Decis Mak. 2022;22:1–7. https://doi.org/10.1186/s12911-022-01841-6 .
de Mello BH, Rigo SJ, da Costa CA, da Rosa Righi R, Donida B, Bez MR, Schunke LC. Semantic interoperability in health records standards: a systematic literature review. Health Technol. 2022;12:255–72. https://doi.org/10.1007/s12553-022-00639-w .
Frade S, Freire SM, Sundvall E, Patriarca-Almeida JH, Cruz-Correia R. Survey of OpenEHR Storage Implementations. In Proceedings of the Proceedings of the 26th IEEE International Symposium on Computer-Based Medical Systems; IEEE, June 2013; pp. 303–307.
Gaddale J. Clinical data acquisition standards harmonization importance and benefits in clinical data management. Perspect Clin Res. 2015;6:179. https://doi.org/10.4103/2229-3485.167101 .
Gamal A, Barakat S, Rezk A. Standardized electronic health record data modeling and persistence: a comparative review. J Biomed Inform. 2021;114:103670. https://doi.org/10.1016/j.jbi.2020.103670 .
Statnikov Y, Ibrahim B, Modi N. A systematic review of administrative and clinical databases of infants admitted to neonatal units. Arch Dis Child Fetal Neonatal Ed. 2017;102:F270–6. https://doi.org/10.1136/archdischild-2016-312010 .
Väänänen A, Haataja K, Vehviläinen-Julkunen K, Toivanen P. AI in healthcare: a narrative review. F1000research. 2021;10:6. https://doi.org/10.12688/f1000research.26997.2 .
Zafeiropoulos N, Mavrogiorgou A, Kleftakis S, Mavrogiorgos K, Kiourtis A, Kyriazis D. Interpretable stroke risk prediction using machine learning algorithms. In: Nagar AK, Singh Jat D, Mishra DK, Joshi A, editors. Lecture notes in networks and systems. Singapore: Springer; 2023. p. 647–56.
Google Scholar
Shaheen MY. Applications of Artificial Intelligence (AI) in healthcare: a review. Sci Prepr. 2021. https://doi.org/10.14293/S2199-1006.1.SOR-.PPVRY8K.v1 .
Abdulrahaman MD, Faruk N, Oloyede AA, Surajudeen-Bakinde NT, Olawoyin LA, Mejabi OV, Imam-Fulani YO, Fahm AO, Azeez AL. Multimedia tools in the teaching and learning processes: a systematic review. Heliyon. 2020;6:e05312. https://doi.org/10.1016/j.heliyon.2020.e05312 .
Imam-Fulani YO, Faruk N, Sowande OA, Abdulkarim A, Alozie E, Usman AD, Adewole KS, Oloyede AA, Chiroma H, Garba S, et al. 5G frequency standardization, technologies, channel models, and network deployment: advances, challenges, and future directions. Sustainability. 2023;15:5173. https://doi.org/10.3390/su15065173 .
Adebowale QR, Faruk N, Adewole KS, Abdulkarim A, Olawoyin LA, Oloyede AA, Chiroma H, Usman AD, Calafate CT. Application of computational intelligence algorithms in radio propagation: a systematic review and metadata analysis. Mob Inf Syst. 2021;2021:1–20. https://doi.org/10.1155/2021/6619364 .
Adewole KS, Mojeed HA, Ogunmodede JA, Gabralla LA, Faruk N, Abdulkarim A, Ifada E, Folawiyo YY, Oloyede AA, Olawoyin LA, et al. Expert system and decision support system for electrocardiogram interpretation and diagnosis: review, challenges and research directions. Appl Sci. 2022;12:12342. https://doi.org/10.3390/app122312342 .
Faruk N, Abdulkarim A, Emmanuel I, Folawiyo YY, Adewole KS, Mojeed HA, Oloyede AA, Olawoyin LA, Sikiru IA, Nehemiah M, et al. A comprehensive survey on low-cost ecg acquisition systems: advances on design specifications, challenges and future direction. Biocybern Biomed Eng. 2021;41:474–502. https://doi.org/10.1016/j.bbe.2021.02.007 .
Musa N, Gital AY, Aljojo N, Chiroma H, Adewole KS, Mojeed HA, Faruk N, Abdulkarim A, Emmanuel I, Folawiyo YY, et al. A systematic review and meta-data analysis on the applications of deep learning in electrocardiogram. J Ambient Intell Humaniz Comput. 2023;14:9677–750. https://doi.org/10.1007/s12652-022-03868-z .
Kim MKMK, Han K, Lee S-HS-H. Current trends of big data research using the korean national health information database. Diabetes Metab J. 2022;46:552–63. https://doi.org/10.4093/dmj.2022.0193 .
Nizami NS, Anjum S, Manikanta AS, Vanamula S. Artificial intelligence in clinical data management: a review of current application and future directions. World J Pharm Res. 2023;12:953–9. https://doi.org/10.20959/wjpr20235-27678 .
Min L, Liu J, Lu X Duan H, Qiao Q. An implementation of clinical data repository with openehr approach: from data modeling to architecture. In Proceedings Of The Studies In Health Technology And Informatics. 2016; Vol. 227, pp. 100–105
Ohmann C, Tilki B, Schulenberg T, Canham S, Banzi R, Kuchinke W. Assessment of a demonstrator repository for individual clinical trial data built upon DSpace. F1000Research. 2020. https://doi.org/10.12688/f1000research.23468.1 .
Farooqui NA, Mehra R. Design of a data warehouse for medical information system using data mining techniques. In Proceedings of the PDGC 2018 - 2018 5th International Conference on Parallel, Distributed and Grid Computing. 2018; pp. 199–203.
Lyu DM, Tian Y, Wang Y, Tong DY, Yin WW, Li JS. Design and implementation of clinical data integration and management system based on Hadoop platform. In Proceedings of the Proceedings - 2015 7th International Conference on Information Technology in Medicine and Education, ITME 2015; 2016; pp. 76–79.
Khan MZ, Kidwai MS, Ahamad F, Khan MU. Hadoop based EMH framework: a big data approach. In Proceedings of the 2021 International Conference on Advance Computing and Innovative Technologies in Engineering, ICACITE 2021. 2021; pp. 1068–1070.
Rouzbeh F, Grama A, Griffin P, Adibuzzaman M. Collaborative cloud computing framework for health data with open source technologies. In Proceedings of the Proceedings of the 11th ACM International Conference on Bioinformatics, Computational Biology and Health Informatics, BCB 2020. 2020; pp. 1–10.
Hak F, Guimarães T, Abelha A, Santos M. An exploratory study of a NoSQL database for a clinical data repository. 2020; Vol. 1161 AISC; ISBN 9783030456962.
Afshar M, Dligach D, Sharma B, Cai X, Boyda J, Birch S, Valdez D, Zelisko S, Joyce C, Modave F, et al. Development and application of a high throughput natural language processing architecture to convert all clinical documents in a clinical data warehouse into standardized medical vocabularies. J Am Med Informatics Assoc. 2019;26:1364–9. https://doi.org/10.1093/jamia/ocz068 .
Augustyn DR, Wyciślik Ł, Sojka M. The cloud-enabled architecture of the clinical data repository in Poland. Sustainability. 2021;13:14050. https://doi.org/10.3390/su132414050 .
Sarwar MA, Bashir T, Shahzad O, Abbas A. Cloud-based architecture to implement Electronic Health Record (EHR) system in Pakistan. IT Prof. 2019;21:49–54. https://doi.org/10.1109/MITP.2018.2882437 .
Pecoraro F, Luzi D, Ricci FL. A clinical data warehouse architecture based on the electronic healthcare record infrastructure. In Proceedings of the HEALTHINF 2014 - 7th International Conference on Health Informatics, Proceedings; Part of 7th International Joint Conference on Biomedical Engineering Systems and Technologies, BIOSTEC 2014. 2014; pp. 287–294.
Amara N, Lamouchi O, Gattoufi S. Design of a breast image data warehouse framework. In Proceedings of the 2020 International Multi-Conference on: “Organization of Knowledge and Advanced Technologies” (OCTA); IEEE. February 2020; pp. 1–13.
Dagliati A, Sacchi L, Bucalo M, Segagni D, Zarkogianni K, Millana AM, Cancela J, Sambo F, Fico G, Barreira MTM et al. A data gathering framework to collect type 2 diabetes patients data. In Proceedings of the 2014 IEEE-EMBS International Conference on Biomedical and Health Informatics, BHI 2014. 2014; pp. 244–247.
Spengler H, Gatz I, Kohlmayer F, Kuhn KA, Prasser F. Improving data quality in medical research: a monitoring architecture for clinical and translational data warehouses. In Proceedings of the Proceedings - IEEE Symposium on Computer-Based Medical Systems. 2020; Vol. 2020-July, pp. 415–420.
Gagalova KK, Elizalde MAL, Portales-Casamar E, Görges M. What you need to know before implementing a clinical research data warehouse: comparative review of integrated data repositories in health care institutions. JMIR Form Res. 2020;4:e17687. https://doi.org/10.2196/17687 .
Wang C, Zhang J, Lassi N, Zhang X. Privacy protection in using artificial intelligence for healthcare: Chinese regulation in comparative perspective. Healthcare. 1878;2022:10. https://doi.org/10.3390/healthcare10101878 .
Bhadmus D, Nkwor L. Interrogating the data protection act 2023. SSRN Electron J. 2023. https://doi.org/10.2139/ssrn.4504935 .
Rahi S, Rana A. Role of ICH guidelines in registration of pharmaceutical products. Int J Drug Regul Aff. 2019;7:14–27. https://doi.org/10.22270/ijdra.v7i4.365 .
Pianykh OS. What is DICOM. In: Pianykh OS, editor. Digital Imaging and Communications in Medicine (DICOM). Berlin: Springer; 2012. p. 3–5.
Pianykh OS. Brief history of DICOM. In: Pianykh OS, editor. Digital Imaging and Communications in Medicine (DICOM). Berlin: Springer; 2012. p. 19–25.
Joyia GJ, Akram MU, Akbar CN, Maqsood MF. Evolution of Health Level-7. In Proceedings of the Proceedings of the 2018 International Conference on Software Engineering and Information Management; ACM: New York, NY, USA. January 4 2018; pp. 118–123.
Ait Abdelouahid R, Debauche O, Mahmoudi S, Marzak A. Literature review: clinical data interoperability models. Inf. 2023. https://doi.org/10.3390/info14070364 .
Facile R, Muhlbradt EE, Gong M, Li Q, Popat V, Pétavy F, Cornet R, Ruan Y, Koide D, Saito TI, et al. Use of clinical data interchange standards consortium (cdisc) standards for real-world data: expert perspectives from a qualitative Delphi survey. JMIR Med Informatics. 2022;10:e30363. https://doi.org/10.2196/30363 .
Hume S, Aerts J, Sarnikar S, Huser V. Current applications and future directions for the CDISC operational data model standard: a methodological review. J Biomed Inform. 2016;60:352–62. https://doi.org/10.1016/j.jbi.2016.02.016 .
Chatterjee A, Pahari N, Prinz A. HL7 FHIR with SNOMED-CT to achieve semantic and structural interoperability in personal health data: a proof-of-concept study. Sensors. 2022;22:3756. https://doi.org/10.3390/s22103756 .
Mukhiya SK, Lamo Y. An HL7 FHIR and GraphQL approach for interoperability between heterogeneous electronic health record systems. Health Inform J. 2021;27:146045822110439. https://doi.org/10.1177/14604582211043920 .
Saripalle R, Runyan C, Russell M. Using HL7 FHIR to achieve interoperability in patient health record. J Biomed Inform. 2019;94:103188. https://doi.org/10.1016/j.jbi.2019.103188 .
Chang E, Mostafa J. The use of SNOMED CT, 2013–2020: a literature review. J Am Med Informatics Assoc. 2021;28:2017–26. https://doi.org/10.1093/jamia/ocab084 .
McDonald CJ, Huff SM, Suico JG, Hill G, Leavelle D, Aller R, Forrey A, Mercer K, DeMoor G, Hook J, et al. LOINC, a universal standard for identifying laboratory observations: A 5-Year update. Clin Chem. 2003;49:624–33. https://doi.org/10.1373/49.4.624 .
D’Amore JD, Li C, McCrary L, Niloff JM, Sittig DF, McCoy AB, Wright A. Using clinical data standards to measure quality: a new approach. Appl Clin Inform. 2018;9:422–31. https://doi.org/10.1055/s-0038-1656548 .
Lin CH, Chou HI, Yang UC. A standard-driven approach for electronic submission to pharmaceutical regulatory authorities. J Biomed Inform. 2018;79:60–70. https://doi.org/10.1016/j.jbi.2018.01.006 .
Rashid A, Kim IK, Khan OA. Providing authorization interoperability using rule based HL7 RBAC for CDR (Clinical Data Repository) Framework. In Proceedings of the Proceedings of 2015 12th International Bhurban Conference on Applied Sciences and Technology, IBCAST 2015; IEEE, 2015; pp. 343–348.
Aiello M, Esposito G, Pagliari G, Borrelli P, Brancato V, Salvatore M. How does DICOM support big data management? Investigating its use in medical imaging community. Insights Imaging. 2021;12:164. https://doi.org/10.1186/s13244-021-01081-8 .
Bennett AV, Jensen RE, Basch E. Electronic patient-reported outcome systems in oncology clinical practice. CA Cancer J Clin. 2012;62:336–47. https://doi.org/10.3322/caac.21150 .
Jensen RE, Snyder CF, Abernethy AP, Basch E, Potosky AL, Roberts AC, Loeffler DR, Reeve BB. Review of electronic patient-reported outcomes systems used in cancer clinical care. J Oncol Pract. 2014;10:e215–22. https://doi.org/10.1200/JOP.2013.001067 .
Li M, Cai H, Nan S, Li J, Lu X, Duan H. A patient-screening tool for clinical research based on electronic health records using OpenEHR: development study. JMIR Med Inform. 2021. https://doi.org/10.2196/33192 .
Mullin S, Zhao J, Sinha S, Lee R, Song B, Elkin PL. Clinical data warehouse query and learning tool using a human-centered participatory design process. Stud Health Technol Inform. 2018;251:59–62. https://doi.org/10.3233/978-1-61499-880-8-59 .
Pressat-Laffouilhère T, Balayé P, Dahamna B, Lelong R, Billey K, Darmoni SJ, Grosjean J. Evaluation of Doc’EDS: a french semantic search tool to query health documents from a clinical data warehouse. BMC Med Inform Decis Mak. 2022;22:34. https://doi.org/10.1186/s12911-022-01762-4 .
Bertagnolli MM, Anderson B, Quina A, Piantadosi S. The electronic health record as a clinical trials tool: opportunities and challenges. Clin Trials. 2020;17:237–42. https://doi.org/10.1177/1740774520913819 .
Cavelaars M, Rousseau J, Parlayan C, de Ridder S, Verburg A, Ross R, Visser GR, Rotte A, Azevedo R, Boiten J-W. OpenClinica. J Clin Bioinforma. 2015;5:1–2.
Patridge EF, Bardyn TP. Research Electronic Data Capture (REDCap). J Med Libr Assoc. 2018;106:142–4. https://doi.org/10.5195/jmla.2018.319 .
Henderson L. Does clinical operations need a makeover? industry experts weigh in on how much traditional approaches in clinical operations need to change to meet new expectations for clinical delivery. Appl Clin Trials. 2020;29:8.
Oracle oracle health sciences inform: comprehensive clinical data capture and management cloud 2015. https://clinical.dk/wpcontent/uploads/2017/01/health-sciences-inform-ds-397109.pdf . Accessed 17 May 2024.
Khan SI, Hoque ASL. Privacy and security problems of national health data warehouse: a convenient solution for developing countries. In Proceedings of the 2016 International Conference on Networking Systems and Security (NSysS). 2016; pp. 1–6.
Thantilage RD, Le-Khac NA, Kechadi MT. Towards a privacy, secured and distributed clinical data warehouse architecture. In Proceedings of the Communications in Computer and Information Science; Springer. 2022; Vol. 1688 CCIS, pp. 73–87.
Senarathne GNS. Cyber security threats and mitigations in the healthcare sector with emphasis on internet of medical things. 2020. https://www.researchgate.net/profile/Nuwan-Sayuru/publication/370504002_Cyber_Security_threats_and_mitigations_in_the_Healthcare_Sector_with_emphasis_on_Internet_of_Medical_Things/links/6453a6fc809a53502149a244/Cyber-Security-threats-and-mitigations-in-the-Healthcare-Sector-with-emphasis-on-Internet-of-Medical-Things.pdf .
U.S. Department of health and human services deidentifying protected health information under the privacy rule. 2007.
NIH HIPAA privacy rule and its impacts on research. https://privacyruleandresearch.nih.gov . Accessed 14 May 2024.
U.S. Department of Health and Human Services Guidance Regarding Methods for De-Identification of Protected Health Information in Accordance with the Health Insurance Portability and Accountability Act (HIPAA) Privacy Rule 2022.
Alabdulatif A, Khalil I, Saidur Rahman M. Security of blockchain and ai-empowered smart healthcare: application-based analysis. Appl Sci. 2022;12:11039. https://doi.org/10.3390/app122111039 .
Wijayarathne SN. Cyber security threats & mitigations in the healthcare sector.
Thomasian, Nicole M, Eli YA. Cybersecurity in the internet of medical things. Health Policy and Technology. 2021;10(3):100549.
Meisami S, Meisami S, Yousefi M, Aref MR. Combining blockchain and iot for decentralized healthcare data management. Int J Cryptogr Inf Secur. 2023;13:35–50. https://doi.org/10.5121/ijcis.2023.13102 .
Jayanthilladevi A, Sangeetha K, Balamurugan E. Healthcare biometrics security and regulations: biometrics data security and regulations governing PHI and HIPAA Act for patient privacy. In Proceedings of the 2020 International Conference on Emerging Smart Computing and Informatics (ESCI); IEEE, March 2020; pp. 244–247.
Kong G, Xiao Z. Protecting privacy in a clinical data warehouse. Health Inform J. 2015;21:93–106. https://doi.org/10.1177/1460458213504204 .
Ajayi OJ, Smith EJ, Viangteeravat T, Huang EY, Nagisetty NSVR, Urraca N, Lusk L, Finucane B, Arkilo D, Young J, et al. Multisite semiautomated clinical data repository for duplication 15q syndrome: study protocol and early uses. JMIR Res Protoc. 2017;6:e194. https://doi.org/10.2196/resprot.7989 .
Gill SK, Karwath A, Uh H-W, Cardoso VR, Gu Z, Barsky A, Slater L, Acharjee A, Duan J, DallOlio L, et al. Artificial intelligence to enhance clinical value across the spectrum of cardiovascular healthcare. Eur Heart J. 2023;44:713–25. https://doi.org/10.1093/eurheartj/ehac758 .
Davenport T, Kalakota R. The potential for artificial intelligence in healthcare. Futur Healthc J. 2019;6:94.
Khalid N, Qayyum A, Bilal M, Al-Fuqaha A, Qadir J. Privacy-preserving artificial intelligence in healthcare: techniques and applications. Comput Biol Med. 2023;158:106848.
Solar M, Araya-Lopez M, Cockbaine J, Castaneda V, Mendoza M. An interoperable repository of clinical data. In Proceedings of the 2020 7th International Conference on eDemocracy and eGovernment, ICEDEG 2020. 2020; pp. 287–290.
Johnson AEWAEW, Ghassemi MMMM, Nemati S, Niehaus KEKE, Clifton DA, Clifford GDGD. Machine learning and decision support in critical care. Proc IEEE Inst Electr Electron Eng. 2016;104:444–66. https://doi.org/10.1109/JPROC.2015.2501978 .
Bottani S, Burgos N, Maire A, Wild A, Ströer S, Dormont D, Colliot O. Automatic quality control of brain T1-weighted magnetic resonance images for a clinical data warehouse. Med Image Anal. 2022;75:102219. https://doi.org/10.1016/j.media.2021.102219 .
Girardi D, Dirnberger J, Giretzlehner M. An ontology-based clinical data warehouse for scientific research. Saf Heal. 2015;1:1–9. https://doi.org/10.1186/2056-5917-1-6 .
Sakib N, Jamil SJ, Mukta SH. A novel approach on machine learning based data warehousing for intelligent healthcare services. In Proceedings of the 2022 IEEE Region 10 Symposium (TENSYMP). 2022; pp. 1–5.
Uddin MA, Stranieri A, Gondal I, Balasubramanian V. Rapid health data repository allocation using predictive machine learning. Health Inform J. 2020;26:3009–36. https://doi.org/10.1177/1460458220957486 .
Philosophy L, Mesagan FO. DigitalCommons @ University of Nebraska - lincoln relevance of internet of things to health institutions in clinical data management : implication for librarians. Libr Philos Pract. 2022; 1–16.
Cheng A, Guan Q, Su Y, Zhou P, Zeng Y. Integration of machine learning and blockchain technology in the healthcare field: a literature review and implications for cancer care. Asia-Pacific J Oncol Nurs. 2021;8:720–4. https://doi.org/10.4103/apjon.apjon-2140 .
Bottani S, Burgos N, Maire A, Saracino D, Ströer S, Dormont D, Colliot O. Evaluation of MRI-based machine learning approaches for computer-aided diagnosis of dementia in a clinical data warehouse. Medical Image Analysis 2023;(89):102903.
Dalhatu I, Aniekwe ÃC, Bashorun ÃA, Abdulkadir A, Dirlikov E, Ohakanu S, Adedokun O, Oladipo A, Jahun I, Murie L et al. From paper files to web-based application for data-driven monitoring of HIV Programs : Nigeria ’ s Journey to a National Data Repository for Decision-Making and Patient Care. Methods of Information in Medicine 2023;62(03/04):130–139.
Oza S, Jazayeri D, Teich JM, Ball E, Nankubuge PA, Rwebembera J, Wing K, Sesay AA, Kanter AS, Ramos GD, et al. Development and deployment of the OpenMRS-Ebola electronic health record system for an ebola treatment center in sierra leone. J Med Internet Res. 2017;19:e294. https://doi.org/10.2196/jmir.7881 .
Shahin MH, Bhattacharya S, Silva D, Kim S, Burton J, Podichetty J, Romero K, Conrado DJ. Open data revolution in clinical research: opportunities and challenges. Clin Transl Sci. 2020;13:665–74. https://doi.org/10.1111/cts.12756 .
Bocquet F, Campone M, Cuggia M. The challenges of implementing comprehensive clinical data warehouses in hospitals. Int J Environ Res Public Health. 2022. https://doi.org/10.3390/ijerph19127379 .
Arsoniadis EG, Melton GB. Leveraging the electronic health record for research and quality improvement: current strengths and future challenges. Semin Colon Rectal Surg. 2016;27:102–10. https://doi.org/10.1053/j.scrs.2016.01.009 .
Mohammed RO, Talab SA. Clinical data warehouse issues and challenges. Int J u and e Serv Sci Technol. 2014;7:251–62.
Shah SM, Khan RA. Secondary use of electronic health record: opportunities and challenges. IEEE access. 2020;8:136947–65.
Poh N, Tirunagari S, Windridge D. Challenges in designing an online healthcare platform for personalised patient analytics. In Proceedings of the IEEE SSCI 2014 - 2014 IEEE Symposium Series on Computational Intelligence - CIBD 2014: 2014 IEEE Symposium on Computational Intelligence in Big Data, Proceedings; IEEE. 2015; pp. 1–6.
Dwivedi AD, Srivastava G, Dhar S, Singh R. A decentralized privacy-preserving healthcare blockchain for IoT. Sensors. 2019;19:326.
Chernyshev M, Zeadally S, Baig Z. Healthcare data breaches: implications for digital forensic readiness. J Med Syst. 2019;43:1–12.
AbuHalimeh A. Improving data quality in clinical research informatics tools. Front Big Data. 2022;5:871897.
Devine EB, Van Eaton E, Zadworny ME, Symons R, Devlin A, Yanez D, Yetisgen M, Keyloun KR, Capurro D, Alfonso-Cristancho R. Automating electronic clinical data capture for quality improvement and research: the CERTAIN validation project of real world evidence. eGEMs. 2018;6:8.
Tian Q, Han Z, Yu P, An J, Lu X, Duan H. Application of OpenEHR archetypes to automate data quality rules for electronic health records: a case study. BMC Med Inform Decis Mak. 2021. https://doi.org/10.1186/s12911-021-01481-2 .
Le Sueur H, Bruce IN, Geifman N, Consortium M. The challenges in data integration-heterogeneity and complexity in clinical trials and patient registries of systemic lupus erythematosus. BMC Med Res Methodol. 2020;20:1–5.
Deshpande P, Rasin A, Tchoua R, Furst J, Raicu D, Schinkel M, Trivedi H, Antani S. Biomedical heterogeneous data categorization and schema mapping toward data integration. Front big Data. 2023;6:1173038.
Kaur H, Alam MA, Jameel R, Mourya AK, Chang V. A proposed solution and future direction for blockchain-based heterogeneous medicare data in cloud environment. J Med Syst. 2018;42:1–11.
Ranchal R, Bastide P, Wang X, Gkoulalas-Divanis A, Mehra M, Bakthavachalam S, Lei H, Mohindra A. Disrupting Healthcare Silos: Addressing Data Volume, Velocity and Variety with a Cloud-Native Healthcare Data Ingestion Service. IEEE J Biomed Heal Informatics. 2020;24:3182–8.
Polnaszek B, Gilmore-Bykovskyi A, Hovanes M, Roiland R, Ferguson P, Brown R, Kind A. Overcoming the challenges of unstructured data in multisite, electronic medical record-based abstraction. Med Care. 2014. https://doi.org/10.1097/MLR.0000000000000108 .
Hong L, Luo M, Wang R, Lu P, Lu W, Lu L. Big data in health care: applications and challenges. Data Inf Manag. 2019. https://doi.org/10.2478/dim-2018-0014 .
Li I, Pan J, Goldwasser J, Verma N, Wong WP, Nuzumlalı MY, Rosand B, Li Y, Zhang M, Chang D. Neural natural language processing for unstructured data in electronic health records: a review. Comput Sci Rev. 2022;46:100511.
Download references
Acknowledgements
This work is funded by the Federal Republic of Nigeria under the National Research Fund (NRF) of the Tertiary Education Trust Fund (TETFund) Grant No. TETF/ES/DR&D-CE/NRF-2021/SETI/ICT/00112/VOL.1.
Author information
Authors and affiliations.
Department of Computer Science, University of Ilorin, Ilorin, Nigeria
Kayode S. Adewole
Department of Information Technology, Sule Lamido University, Kafin Hausa, Nigeria
Emmanuel Alozie, Hawau Olagunju & Nasir Faruk
Department Information and Media Studies, Faculty of Communication, Bayero University, Kano, Nigeria
Ruqayyah Yusuf Aliyu
Department of Electrical and Electronics Engineering, Faculty of Engineering, University of Lagos, Lagos, Nigeria
Agbotiname Lucky Imoize
Department of Electrical Engineering, Ahmadu Bello University, Zaria, Nigeria
Abubakar Abdulkarim
Department of Telecommunication Science, University of Ilorin, Ilorin, Nigeria
Yusuf Olayinka Imam-Fulani & Abdulkarim A. Oloyede
Department of Software Engineering, Sule Lamido University Kafin Hausa, Kafin Hausa, Nigeria
Salisu Garba
Department of Cyber Security, Sule Lamido University Kafin Hausa, Kafin Hausa, Nigeria
Bashir Abdullahi Baba
Department of Economics, Faculty of Social and Management Sciences, Kafin Hausa, Nigeria
Mustapha Hussaini
Department: University Medical Services, Sule Lamido University Kafin Hausa, Kafin Hausa, Jigawa State, Nigeria
Aminu Abdullahi
Department of Computer Science and Information Technology, Baze University, Abuja, Nigeria
Rislan Abdulazeez Kanya
Department: International Law and Jurisprudence, Faculty of Law, Bayero University, Kano, Nigeria
Dahiru Jafaru Usman
Department of Electrical and Telecommunications Engineering, Kampala International University, Kan-Sanga, P.O. Box 20000, Kampala, Uganda
You can also search for this author in PubMed Google Scholar
Contributions
Conceptualization: K.S.A., N.F., E.A., H.O., and R.Y.A. Methodology, A.L.I., Y.O.I., K.S.A., and A.A.O. Figures: E.A., H.O., S.G., A.A., and A.L.I. Validation: R.Y.A., B.A.B., A.A., R.A.K, and D.J.U. Formal analysis: E.A., H.O., A.A., K.S.A, and A.A.O. Resources: N.F., S.G., A.A., R.A.K, and D.J.U. Writing—original draft preparation: K.S.A., E.A., H.O., N.F., and R.Y.A. Writing—review and editing: A.L.I., S.G., A.A.O, M.H., and Y.O.I Visualization: E.A, B.A.B., H.O., S.G., R.A.K, D.J.U., and A.A Supervision: N.F. Project administration: N.F. Funding acquisition: N.F. All authors reviewed the manuscript.
Corresponding author
Correspondence to Nasir Faruk .
Ethics declarations
Competing interests.
The authors declare no competing interests.
Additional information
Publisher's note.
Springer Nature remains neutral with regard to jurisdictional claims in published maps and institutional affiliations.
Rights and permissions
Open Access This article is licensed under a Creative Commons Attribution 4.0 International License, which permits use, sharing, adaptation, distribution and reproduction in any medium or format, as long as you give appropriate credit to the original author(s) and the source, provide a link to the Creative Commons licence, and indicate if changes were made. The images or other third party material in this article are included in the article's Creative Commons licence, unless indicated otherwise in a credit line to the material. If material is not included in the article's Creative Commons licence and your intended use is not permitted by statutory regulation or exceeds the permitted use, you will need to obtain permission directly from the copyright holder. To view a copy of this licence, visit http://creativecommons.org/licenses/by/4.0/ .
Reprints and permissions
About this article
Adewole, K.S., Alozie, E., Olagunju, H. et al. A systematic review and meta-data analysis of clinical data repositories in Africa and beyond: recent development, challenges, and future directions. Discov Data 2 , 8 (2024). https://doi.org/10.1007/s44248-024-00012-4
Download citation
Received : 29 March 2024
Accepted : 29 May 2024
Published : 26 June 2024
DOI : https://doi.org/10.1007/s44248-024-00012-4
Share this article
Anyone you share the following link with will be able to read this content:
Sorry, a shareable link is not currently available for this article.
Provided by the Springer Nature SharedIt content-sharing initiative
- Clinical Data Repository (CDR)
- Systematic review
- Clinical data warehouse
- Find a journal
- Publish with us
- Track your research
Science, technology and innovation
International co-operation on science, technology and innovation pushes the knowledge frontier and accelerates progress towards tackling shared global challenges like climate change and biodiversity loss. The OECD provides data and evidence-based analysis on supporting research and innovation and fostering policies that promote responsible innovation and technology governance for resilient and inclusive societies.
Select a language
Policy issues.
- Chemical safety and biosafety The chemical industry is one of the largest industrial sectors in the world and is expected to quadruple by 2060. Governments and industry share the responsibility for ensuring safe chemical production and use. The OECD helps countries develop and implement policies for safeguarding human health and the environment, and in making their systems for managing chemicals as efficient as possible. Learn more
- Science policy Science policy focuses on actions to improve the efficiency and effectiveness of public investment in research. Publicly funded research in universities and research institutes plays an essential role in generating the knowledge that supports evidence-based decision making and underpins technological development. There is increasing policy emphasis on “open science” and the mobilisation of public research to address urgent and complex societal challenges. Learn more
- Space economy The space economy encompasses all activities and resources that contribute to human progress through the exploration, research, understanding, management, and utilisation of space. The sector provides critical infrastructure on Earth, contributes fundamental scientific data for decision-making, and supports societal well-being. Learn more
- Technology policy Technological innovation is an engine of human well-being and economic activity, but also raises concerns for individuals and society. Governments use a mix of policies targeting specific technologies to steer their responsible development and use. This includes national plans that provide strategic orientation and support measures for research, innovation and diffusion activities. Policies also promote ethical practice through regulations and guidelines. Learn more
Programmes of work
- OECD Eurasia Competitiveness Programme Enhancing regional dialogue, competitiveness and improving the business climate. Learn more
- AI in Work, Innovation, Productivity and Skills The OECD is working with governments around the world to measure and analyse the impact of AI on training needs and labour markets. We aim to help governments to create AI-related policies that are both responsible and human-centred, and that improve the wellbeing of individuals and society as a whole. Learn more
Related publications

Subscribe to our science, technology and innovation newsletter
StarTribune
Landmark university of minnesota papers on alzheimer's disease and stem cells retracted.
Years after questions were raised about their integrity, two of the University of Minnesota's highest-profile scientific discoveries have been retracted in one week — one that offered hope over the therapeutic potential of stem cells and another that offered a promising path toward treating Alzheimer's disease.
The studies are more than a decade old and superseded by other discoveries in their fields. But the retractions of the Alzheimer's paper on Monday and the stem cell paper on June 17 are setbacks for an institution that is fighting to move up the U.S. rankings in academic reputation and federal research dollars.
Both studies were published in the prestigious journal Nature and collectively have been cited nearly 7,000 times. Researchers worldwide were using these papers to support their work years after they had been disputed.
That shows the harm in the drawn-out university investigation and the journal's retractions, said Dr. Matthew Schrag, a neurologist who scrutinized the Alzheimer's paper in 2022 outside of his role at Vanderbilt University. "We are squandering not only resources but the credibility and reputation of our profession by failing to address obvious misconduct."
The university in a statement on Tuesday said that it has many ethics requirements that weren't in place when these papers were published that should prevent future disputes and retractions.
The discoveries were notable in their days because they offered unexpected solutions to vexing scientific and political problems.
Dr. Catherine Verfaillie and colleagues in 2002 reported that they coaxed mesenchymal stem cells from adult bone marrow into growing numerous other cell types and tissues in the body. Only stem cells from early-stage human embryos had shown such regenerative potential at that time, and they were controversial because they were derived from aborted fetuses or leftover embryos from infertility treatments. President George W. Bush had banned federal funding for embryonic research, fueling a search for alternative stem cell sources.
Dr. Karen Ashe and colleagues similarly gained global attention in 2006 when they found a molecular target that appeared influential in the onset of Alzheimer's disease, which remains incurable and a leading source of dementia and death in America's aging population. Mice mimicking that molecule, amyloid beta star 56, showed worse memory loss based on their ability to navigate a maze. Ashe theorized that a drug targeting that molecule could help people overcome or slow Alzheimer's debilitating effects.
The problems leading to the retractions were remarkably similar. Colleagues at other institutions struggled to replicate their findings, which prompted others to look closer at the images of cellular or molecular activity in mice on which their findings were based.
Peter Aldhous first raised concerns in 2006 over the stem cell discovery as a science journalist and San Francisco bureau chief for New Scientist magazine.
"The big claim that these were essentially the same as embryonic stem cells and can differentiate into anything, nobody was able to replicate that," he said.
Verfaillie and colleagues corrected the Nature paper in 2007, which contained an image of cellular activity in mice that appeared identical to an image in a different paper that supposedly came from different mice. The U then launched an investigation over complaints of image duplications or manipulations in more of Verfaillie's papers. It eventually cleared her of misconduct , but blamed her for inadequate training and oversight and claimed that a junior researcher had falsified data in a similar study published in the journal Blood. That article was retracted in 2009.
Concerns resurfaced in 2019 over the Nature stem cell paper when Elisabeth Bik, a microbiologist-turned-research detective, found more examples of image duplication.
Bik also turned out to be a key critic of Ashe's Alzheimer's discoveries, raising concerns about images in her Nature paper and related studies. Much of the blame has fallen on coauthor Sylvain Lesne, a U neuroscientist who was responsible for the published images. Lesne did not reply to a request for comment, but authorized the university to disclose that it completed its internal investigation into the Nature paper without finding evidence of misconduct. Reviews of other publications from Lesne's lab are ongoing.
Changes over the past decade at the university have sought to reduce academic scandals, including a system added in 2008 for anonymous reporting and for managing accusations. All researchers leading studies at the U are now trained in avoiding conflicts of interest, plagiarism and misconduct.
The retractions are "painful" but the university accepts the journal's decisions and remains committed to ethical research, said Shashank Priya, vice president for research and innovation. "What I know is that the vast majority of researchers ... go to their labs, their fields or their classrooms every day with a strong sense of purpose and integrity."
Even as the papers continue to be cited, researchers have turned to other targets. Ashe has pivoted to the search for a medication that can prevent dysfunctional tau proteins from disrupting the brain's thinking cells, or neurons.
Ashe said she agreed to the Nature retraction reluctantly because she had published follow-up research that offered fresh proof of her findings and recommended a correction to the Nature paper that would have further upheld those findings.
"When the editors decided not to publish the correction, however, I opted to retract the article," she said in an email, adding that "we are encouraged by results of ongoing experiments about Abeta*56, and continue to believe that it could improve our understanding of Alzheimer's disease and the development of better treatments."
Lesne was the only coauthor to disagree with the retraction, even though Nature stated that the paper contained "excessive manipulation, including splicing, duplication and the use of an eraser tool" to edit the images.
Verfaillie directed the university's stem cell institute and remained involved in its research even after returning to Belgium in 2006. The recent retiree did not reply to an email for comment, but said in a translation of a Belgium newspaper article that the retraction is "a stain on our reputation." Nature called for the correction because Verfaillie and other authors couldn't locate authentic images to prove the validity of their research.
"There is indeed a problem with a photo," she said. "We have not found the correct photo twenty years after the research was conducted. But even without that photo, the conclusion still stands."
The dispute over the utility of mesenchymal stem cells became less important in 2007, when Shinya Yamanaka revealed a process for reprogramming mouse skin cells so that they could mimic the versatility of embryonic stem cells. Others were able to repeat the process, which earned the Japanese researcher a share of the Nobel Prize for Medicine in 2012.
Aldhous said it is disappointing that it took years to resolve questions over the Alzheimer's paper, and much longer to do the same over the stem cell paper. He said he doesn't believe the university has adequately solved whether the researchers made repeated mistakes or committed intentional misconduct. The junior researcher blamed for errors in one stem cell paper was not involved in other disputed papers, he noted.
However, he said it is arguably more important to quickly correct the scientific record so that faulty or unsubstantiated research doesn't influence other scientists and send them in wrong directions.
"Why have we had to wait for so long to consign this to the trash can, essentially?" he asked. "This should have happened years ago."
Jeremy Olson is a Pulitzer Prize-winning reporter covering health care for the Star Tribune. Trained in investigative and computer-assisted reporting, Olson has covered politics, social services, and family issues.
- Replacing Joe Biden is a fantasy Democrats must abandon
- Minnesota health insurers seek premium hikes for individuals up to 12.75%
- Trouble getting a Minnesota driver's license? Here's why.
- Northern Minnesota's Moondance Jam rock fest cancels all headliners without offering refunds
- Minnesota e-bike rebate program fills up in minutes after shaky initial rollout
- 'Good Morning America' spotlights 'St. Anthony Main neighborhood' in Minneapolis
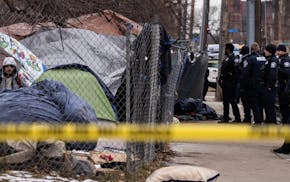
Experts working to end homelessness in Minnesota say high court ruling will make jobs harder
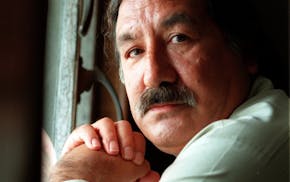
Leonard Peltier's parole denied in 1975 murder of 2 FBI agents
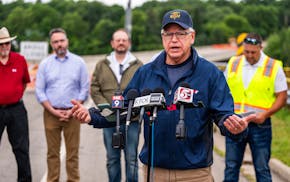
Walz and other Democratic governors meeting at White House following Biden's debate performance
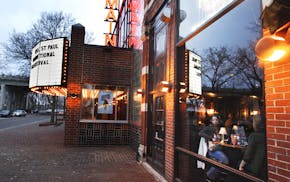
'Good Morning America' spotlights 'St. Anthony Main neighborhood' in Minneapolis
Cat survives toss from 12th-floor balcony of Minneapolis apartment
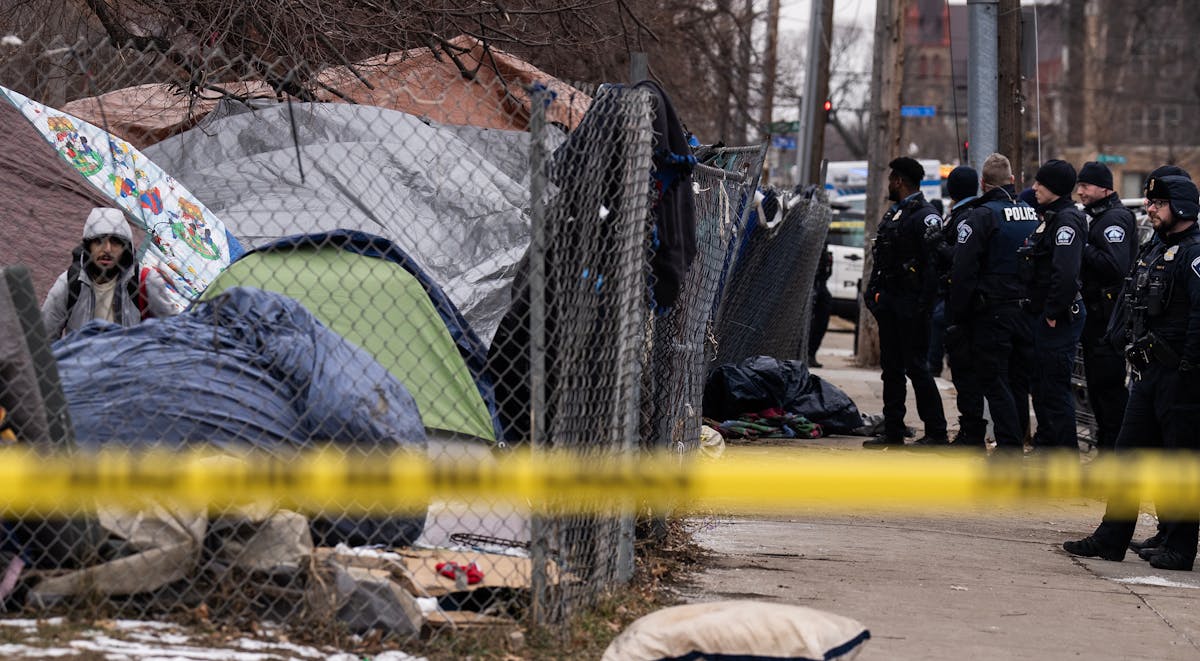
- Minnesota health insurers seek premium hikes for individuals up to 12.75% 12:23pm
- Cat survives toss from 12th-floor balcony of Minneapolis apartment 55 minutes ago
- Cat survives toss from 12th-floor balcony of Minneapolis apartment • Minneapolis
- Trouble getting a Minnesota driver's license? Here's why. • Local
- Former head of Hennepin County public defender's office admits to drunken driving in Wayzata • West Metro
- Leonard Peltier's parole denied in 1975 murder of 2 FBI agents • Local
- Minnesota e-bike rebate program fills up in minutes after shaky initial rollout • Local
© 2024 StarTribune. All rights reserved.
share this!
June 24, 2024 report
This article has been reviewed according to Science X's editorial process and policies . Editors have highlighted the following attributes while ensuring the content's credibility:
fact-checked
peer-reviewed publication
trusted source
Analysis of data suggests homosexual behavior in other animals is far more common than previously thought
by Bob Yirka , Phys.org
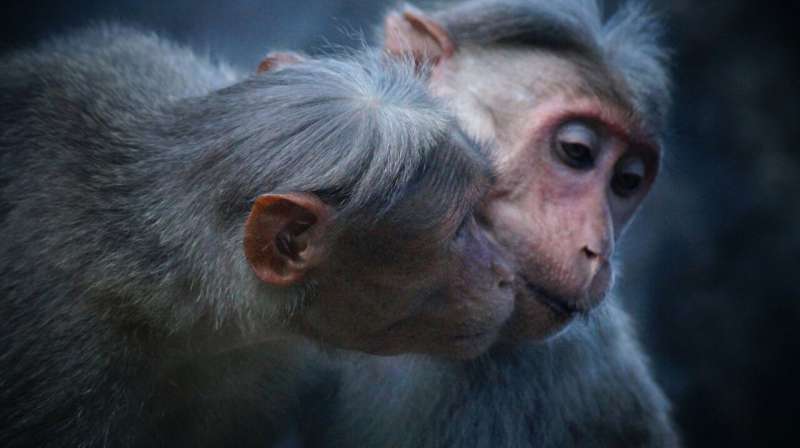
A team of anthropologists and biologists from Canada, Poland, and the U.S., working with researchers at the American Museum of Natural History, in New York, has found via meta-analysis of data from prior research efforts that homosexual behavior is far more common in other animals than previously thought. The paper is published in PLOS ONE .
For many years, the biology community has accepted the notion that homosexuality is less common in animals than in humans, despite a lack of research on the topic. In this new effort, the researchers sought to find out if such assumptions are true.
The work involved study of 65 studies into the behavior of multiple species of animals, mostly mammals, such as elephants, squirrels, monkeys, rats and racoons.
The researchers found that 76% of the studies mentioned observations of homosexual behavior, though they also noted that only 46% had collected data surrounding such behavior—and only 18.5% of those who had mentioned such behavior in their papers had focused their efforts on it to the extent of publishing work with homosexuality as it core topic.
They noted that homosexual behavior observed in other species included mounting, intromission and oral contact—and that researchers who identified as LGBTQ+ were no more or less likely to study the topic than other researchers.
The researchers point to a hesitancy in the biological community to study homosexuality in other species , and thus, little research has been conducted. They further suggest that some of the reluctance has been due to the belief that such behavior is too rare to warrant further study.
The research team suggests that homosexuality is far more common in the animal kingdom than has been reported—they further suggest more work is required regarding homosexual behaviors in other animals to dispel the myth of rarity.
Journal information: PLoS ONE
© 2024 Science X Network
Explore further
Feedback to editors

UV radiation damage leads to ribosome roadblocks, causing early skin cell death
4 minutes ago

Dual-laser approach could lower cost of high-resolution 3D printing
8 minutes ago

Novel method enhances size-controlled production of luminescent quantum dots
19 minutes ago

Cosmic simulation reveals how black holes grow and evolve

How climate change is affecting where species live

Human presence shifts balance between leopards and hyenas in East Africa

Physicists' laser experiment excites atom's nucleus, may enable new type of atomic clock

Treatment with a mixture of antimicrobial peptides found to impede antibiotic resistance

Study reveals fireworks' impact on air quality
2 hours ago

Research shows how RNA 'junk' controls our genes
Relevant physicsforums posts, who chooses official designations for individual dolphins, such as fb15, f153, f286.
Jun 26, 2024
Color Recognition: What we see vs animals with a larger color range
Jun 25, 2024
Innovative ideas and technologies to help folks with disabilities
Jun 24, 2024
Is meat broth really nutritious?
Covid virus lives longer with higher co2 in the air.
Jun 22, 2024
Periodical Cicada Life Cycle
Jun 21, 2024
More from Biology and Medical
Related Stories

How, and why, did homosexual behavior evolve in humans and other animals?
Oct 12, 2023

Male rhesus macaques often have sex with each other, a trait they have inherited in part from their parents
Jul 15, 2023

Same-gender sexual behavior found to be widespread across mammal species and to have multiple origins
Oct 4, 2023

Stop calling it a choice: Biological factors drive homosexuality
Sep 4, 2019
Clinicians' personal religious beliefs may impact treatment provided to patients who are homosexual
Oct 23, 2017

Study shows same-sex sexual behavior is widespread and heritable in macaque monkeys
Jul 10, 2023
Recommended for you

New understanding of a common plant enzyme could lead to better crop management
3 hours ago

Study illuminates cues algae use to 'listen' to their environment

Invasive brown widow spiders found to host novel bacteria related to chlamydia

Ants perform amputations to save injured nestmates
4 hours ago

Searching for the missing link between growth and longevity
5 hours ago
Let us know if there is a problem with our content
Use this form if you have come across a typo, inaccuracy or would like to send an edit request for the content on this page. For general inquiries, please use our contact form . For general feedback, use the public comments section below (please adhere to guidelines ).
Please select the most appropriate category to facilitate processing of your request
Thank you for taking time to provide your feedback to the editors.
Your feedback is important to us. However, we do not guarantee individual replies due to the high volume of messages.
E-mail the story
Your email address is used only to let the recipient know who sent the email. Neither your address nor the recipient's address will be used for any other purpose. The information you enter will appear in your e-mail message and is not retained by Phys.org in any form.
Newsletter sign up
Get weekly and/or daily updates delivered to your inbox. You can unsubscribe at any time and we'll never share your details to third parties.
More information Privacy policy
Donate and enjoy an ad-free experience
We keep our content available to everyone. Consider supporting Science X's mission by getting a premium account.
E-mail newsletter
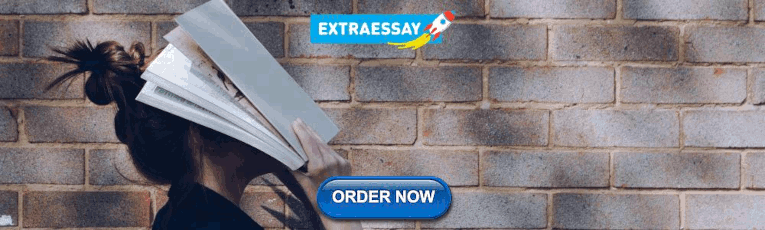
COMMENTS
About the journal. The journal aims to promote and communicate advances in big data research by providing a fast and high quality forum for researchers, practitioners and policy makers from the very many different communities working on, and with, this topic. The journal will accept papers on foundational aspects in …. View full aims & scope.
As an open access platform of the Harvard Data Science Initiative, Harvard Data Science Review (HDSR) features foundational thinking, research milestones, educational innovations, and major applications, with a primary emphasis on reproducibility, replicability, and readability.We aim to publish content that helps define and shape data science as a scientifically rigorous and globally ...
Data Science . Information Use . Regulatory Compliance . Future Research . Public And Private . Social Good . Public And Private Sector . Effective Use. AbstractThe appetite for effective use of information assets has been steadily rising in both public and private sector organisations.
Scientific Data is an open access journal dedicated to data, publishing descriptions of research datasets and articles on research data sharing from all areas ...
PDF | Learn how to choose the best data collection methods and tools for your research project, with examples and tips from ResearchGate experts. | Download and read the full-text PDF.
The Journal of Big Data publishes open-access original research on data science and data analytics. Deep learning algorithms and all applications of big data are welcomed. Survey papers and case studies are also considered. The journal examines the challenges facing big data today and going forward including, but not limited to: data capture and storage; search, sharing, and analytics; big ...
This paper shows data science's potential for disruptive innovation in science, industry, policy, and people's lives. We present how data science impacts science and society at large in the coming years, including ethical problems in managing human behavior data and considering the quantitative expectations of data science economic impact. We introduce concepts such as open science and e ...
This article is a practical guide to conducting data analysis in general literature reviews. The general literature review is a synthesis and analysis of published research on a relevant clinical issue, and is a common format for academic theses at the bachelor's and master's levels in nursing, physiotherapy, occupational therapy, public health and other related fields.
Ten Research Challenge Areas in Data Science. To drive progress in the field of data science, we propose 10 challenge areas for the research community to pursue. Since data science is broad, with methods drawing from computer science, statistics, and other disciplines, and with applications appearing in all sectors, these challenge areas speak ...
This paper focuses on privacy and security concerns in Big Data. This paper also covers the encryption techniques by taking existing methods such as differential privacy, k-anonymity, T-closeness, and L-diversity.Several privacy-preserving techniques have been created to safeguard privacy at various phases of a large data life cycle.
This research contributes to the creation of a research vector on the role of data science in central banking. In , the authors provide an overview and tutorial on the data-driven design of intelligent wireless networks. The authors in provide a thorough understanding of computational optimal transport with application to data science.
Data Collection | Definition, Methods & Examples. Published on June 5, 2020 by Pritha Bhandari.Revised on June 21, 2023. Data collection is a systematic process of gathering observations or measurements. Whether you are performing research for business, governmental or academic purposes, data collection allows you to gain first-hand knowledge and original insights into your research problem.
Tip 1: Think about the data you need and why you need them. Tip 2: Select the most appropriate resource. Tip 3: Construct your query strategically. Tip 4: Make the repository work for you. Tip 5: Refine your search. Tip 6: Assess data relevance and fitness -for -use. Tip 7: Save your search and data- source details.
For many researchers unfamiliar with qualitative research, determining how to conduct qualitative analyses is often quite challenging. Part of this challenge is due to the seemingly limitless approaches that a qualitative researcher might leverage, as well as simply learning to think like a qualitative researcher when analyzing data. From framework analysis (Ritchie & Spencer, 1994) to content ...
This study provides an in-depth examination of space launch data over the long-time frame from 1957 to 2023. By combining data from a Kaggle dataset with web-scraped data for 2023, the research ...
Data preservation is a corollary of data papers, not their main purpose. Most data journals do not archive data in-house. Instead, they generally require that authors submit the dataset to a repository. These repositories archive the data, provide persistent access, and assign the dataset a unique identifier (DOI).
Primary data sources refer to original data collected firsthand by researchers specifically for their research purposes. These sources provide fresh and relevant information tailored to the study's objectives. Examples of primary data sources include surveys and questionnaires, direct observations, experiments, interviews, and focus groups.
A research question is what a study aims to answer after data analysis and interpretation. The answer is written in length in the discussion section of the paper. Thus, the research question gives a preview of the different parts and variables of the study meant to address the problem posed in the research question.1 An excellent research ...
Research methods are specific procedures for collecting and analyzing data. Developing your research methods is an integral part of your research design. When planning your methods, there are two key decisions you will make. First, decide how you will collect data. Your methods depend on what type of data you need to answer your research question:
Analysis Methods. Some common research data analysis methods include: Descriptive statistics: Descriptive statistics involve summarizing and describing the main features of a dataset, such as the mean, median, and standard deviation. Descriptive statistics are often used to provide an initial overview of the data.
Definition of research data and overview of the different options to share data: store, link, enrich, publish, declare ... These brief, peer-reviewed articles complement full research papers and are an easy way to receive proper credit and recognition for the work you have done. Research elements are research outputs that have come about as a ...
Doing qualitative research is not easy and may require a complete rethink of how research is conducted, particularly for researchers who are more familiar with quantitative approaches. There are many ways of conducting qualitative research, and this paper has covered some of the practical issues regarding data collection, analysis, and management.
This review explores the substantial contributions of AI and Big Data to biomedical research, highlighting key advancements and applications such as the use of AI-driven models in disease diagnosis, the development of personalized medical treatments based on genetic profiles, and the acceleration of drug discovery through AI analysis.
We identify data citations (in the form of DOI or citation numbers) in the text and this information is searchable. You can either add (HAS_DATA:y) to your search to identify all papers that reference data, or specify the data type (e.g. PDB accession number, or clinical trial reference) using the advanced search.
The CODATA Data Science Journal is a peer-reviewed, open access, electronic journal, publishing papers on the management, dissemination, use and reuse of research data and databases across all research domains, including science, technology, the humanities and the arts. The scope of the journal includes descriptions of data systems, their implementations and their publication, applications ...
The methodology adopted for the systematic review in this paper comprises the research questions, the search strategy including the inclusion and exclusion criteria, and the analysis of publications obtained [14, 15].2.1 Planning the review. In this paper, the planning of the systematic review commences with the establishment of a procedure that provides adequate guidelines for carrying out ...
International co-operation on science, technology and innovation pushes the knowledge frontier and accelerates progress towards tackling shared global challenges like climate change and biodiversity loss. The OECD provides data and evidence-based analysis on supporting research and innovation and fostering policies that promote responsible innovation and technology governance for resilient and ...
This paper aims to understand the wide range of harm caused by ransomware attacks to individuals, ... 'Your Data is Stolen and Encrypted': The Ransomware Victim Experience. Dr Pia Hüsch, Dr Gareth Mott, ... we produce evidence-based research, publications and events on defence, security and international affairs to help build a safer UK ...
Concerns resurfaced in 2019 over the Nature stem cell paper when Elisabeth Bik, a microbiologist-turned-research detective, found more examples of image duplication.
The paper is published in PLOS ONE. ... has found via meta-analysis of data from prior research efforts that homosexual behavior is far more common in other animals than previously thought.