Information
- Author Services
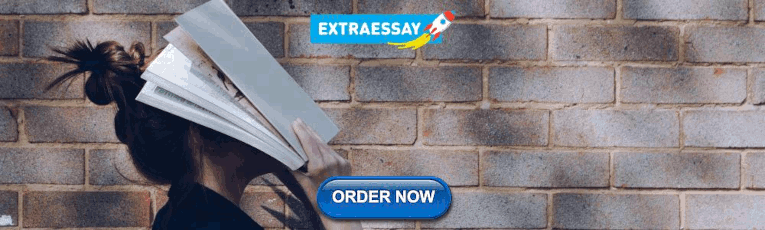
Initiatives
You are accessing a machine-readable page. In order to be human-readable, please install an RSS reader.
All articles published by MDPI are made immediately available worldwide under an open access license. No special permission is required to reuse all or part of the article published by MDPI, including figures and tables. For articles published under an open access Creative Common CC BY license, any part of the article may be reused without permission provided that the original article is clearly cited. For more information, please refer to https://www.mdpi.com/openaccess .
Feature papers represent the most advanced research with significant potential for high impact in the field. A Feature Paper should be a substantial original Article that involves several techniques or approaches, provides an outlook for future research directions and describes possible research applications.
Feature papers are submitted upon individual invitation or recommendation by the scientific editors and must receive positive feedback from the reviewers.
Editor’s Choice articles are based on recommendations by the scientific editors of MDPI journals from around the world. Editors select a small number of articles recently published in the journal that they believe will be particularly interesting to readers, or important in the respective research area. The aim is to provide a snapshot of some of the most exciting work published in the various research areas of the journal.
Original Submission Date Received: .
- Active Journals
- Find a Journal
- Proceedings Series
- For Authors
- For Reviewers
- For Editors
- For Librarians
- For Publishers
- For Societies
- For Conference Organizers
- Open Access Policy
- Institutional Open Access Program
- Special Issues Guidelines
- Editorial Process
- Research and Publication Ethics
- Article Processing Charges
- Testimonials
- Preprints.org
- SciProfiles
- Encyclopedia
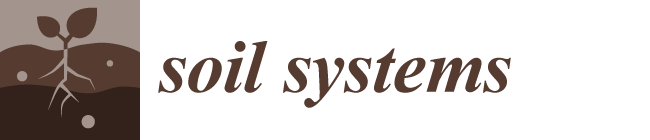
Article Menu
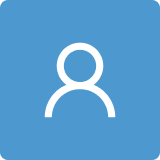
- Subscribe SciFeed
- Recommended Articles
- Google Scholar
- on Google Scholar
- Table of Contents
Find support for a specific problem in the support section of our website.
Please let us know what you think of our products and services.
Visit our dedicated information section to learn more about MDPI.
JSmol Viewer
Soil health assessment and management: recent development in science and practices.
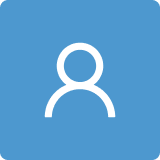
1. Introduction
2. the evolution of the scientific soil health system, 3. soil health assessment methods, 3.1. farmer perceptions of soil health, 3.2. soil health card methods, 3.3. solvita soil health tests, 3.4. haney soil health test, 3.5. comprehensive assessment of soil health (cash), 4. soil health management, 4.1. soil health principles, 4.2. best soil health management practices, 4.2.1. proper land use, 4.2.2. crop rotation, 4.2.3. cover crops, 4.2.4. conservation tillage, 4.2.5. soil organic amendment, 4.2.6. crop-range-livestock integration and rotational grazing, 5. summary and conclusions, institutional review board statement, informed consent statement, data availability statement, conflicts of interest.
- Weil, R.R.; Brady, N.C. The Nature and Properties of Soils ; Prentice Hall: Upper Saddle River, NJ, USA, 2017. [ Google Scholar ]
- USDA-NRCS. Soil Health ; Natural Resources Conservation Service, U.S. Department of Agriculture: Washington, DC, USA, 2019. Available online: https://www.nrcs.usda.gov/wps/portal/nrcs/main/soils/health/ (accessed on 11 August 2021).
- Doran, J.W.; Zeiss, M.R. Soil health and sustainability: Managing the biotic component of soil quality. Appl. Soil Ecol. 2000 , 15 , 3–11. [ Google Scholar ] [ CrossRef ] [ Green Version ]
- UNFAO. FAO Soils Portal-Degradation/Restoration ; Food and Agriculture Organization of the United Nations: Rome, Italy, 2021; Available online: http://www.fao.org/soils-portal/soil-degradation-restoration/en/ (accessed on 12 August 2021).
- Nunes, F.C.; Alves, L.J.; Carvalho, C.C.N.; Gross, E.; Majeti, P.; de Marchi Soares, T. Soil as a complex ecological system for meeting food and nutritional security. In Climate Change and Soil Interactions ; Prasad, M.N.V., Pietrzykowski, M., Eds.; Elsevier: Amsterdam, The Netherlands, 2020; pp. 229–269. [ Google Scholar ]
- Williams, H.; Colombi, T.; Keller, T. The influence of soil management on soil health: An on-farm study in southern Sweden. Geoderma 2020 , 360 , 114010. [ Google Scholar ] [ CrossRef ]
- Pereira, P.; Brevik, E.C.; Munoz-Rojas, M.; Miller, B.A. (Eds.) Soil mapping and processes modeling for sustainable land management. In Soil Mapping and Process Modeling for Sustainable Land Use Management ; Elsevier: Amsterdam, The Netherlands, 2017; pp. 29–60. [ Google Scholar ]
- Guo, M. The 3R principles for applying biochar to improve soil health. Soil Syst. 2020 , 4 , 9. [ Google Scholar ] [ CrossRef ] [ Green Version ]
- Kibblewhite, M.G.; Ritz, K.; Swift, M.J. Soil health in agricultural systems. Phil. Trans. R. Soc. B 2008 , 363 , 685–701. [ Google Scholar ] [ CrossRef ] [ Green Version ]
- Allen, D.E.; Singh, B.P.; Dalal, R.C. Soil health indicators under climate change: A review of current knowledge. In Soil Health and Climate Change ; Singh, B.P., Cowie, A.L., Chan, K.Y., Eds.; Springer: Berlin, Germany, 2011; pp. 25–45. [ Google Scholar ]
- Van Bruggena, A.H.C.; Semenov, A.M. In search of biological indicators for soil health and disease suppression. Appl. Soil Ecol. 2000 , 15 , 13–24. [ Google Scholar ] [ CrossRef ]
- Negueira Cardoso, E.J.B.; FigueiredoVasconcellos, R.L.; Bini, D.; Horta Miyauchi, M.Y.; Alcantara, C.; Alves, P.; de Paula, A.; Shigueyoshi Nakatani, A.; de Moraes, J.; Nogueira, M. Soil health: Looking for suitable indicators. What should be considered to assess the effects of use and management on soil health? Sci. Agric. 2013 , 70 , 274–289. [ Google Scholar ] [ CrossRef ] [ Green Version ]
- Moebius-Clune, B.N.; Moebiue-Clune, D.J.; Gugino, B.K.; Idowu, O.; Schindelbeck, R.; Rostow, A.; van Es, H.; Thies, J.; Shyler, H.; McBride, M.; et al. Comprehensive Assessment of Soil Health , 3rd ed.; Cornell University: Ithaca, NY, USA, 2017; pp. 19–101. [ Google Scholar ]
- USDA-NRCS. Soil Health Assessment ; Natural Resources Conservation Service, U.S. Department of Agriculture: Washington, DC, USA, 2019. Available online: https://www.nrcs.usda.gov/wps/portal/nrcs/main/soils/health/assessment (accessed on 15 August 2021).
- USDA-NRCS. Soil Health Card ; Natural Resources Conservation Service, U.S. Department of Agriculture: Washington, DC, USA, 2019. Available online: https://www.nrcs.usda.gov/wps/portal/nrcs/detail/soils/health/assessment/?cid=nrcs142p2_053871 (accessed on 15 August 2021).
- Woods End Laboratories. Solvita Soil Health Tests ; Woods End Laboratories, Inc.: Mount Vernon, ME, USA, 2021; Available online: https://solvita.com/soil/ (accessed on 15 August 2021).
- Ward Laboratories. Haney Test ; Ward Laboratories, Inc.: Kearney, NE, USA, 2021; Available online: https://www.wardlab.com/haney-test/ (accessed on 15 August 2021).
- Norris, C.E.; Congreves, K.A. Alternative management practices improve soil health indices in intensive vegetable cropping systems: A review. Front. Environ. Sci. 2018 , 6 , 50. [ Google Scholar ] [ CrossRef ]
- Idowu, J.; Ghimire, R.; Flynn, R.; Ganguli, A. Soil Health—Importance, Assessment, and Management. Circular 694B ; New Mexico State University Cooperative Extension Service: Las Cruces, NM, USA, 2019; Available online: https://ucanr.edu/sites/harecBETA/files/321673.pdf (accessed on 17 August 2021).
- PennState Extension. Managing Soil Health: Concepts and Practices ; Pennsylvania State University Cooperative Extension Service: University Park, PA, USA, 2017; Available online: https://extension.psu.edu/managing-soil-health-concepts-and-practices (accessed on 17 August 2021).
- USDA-NRCS. Soil Health Management ; Natural Resources Conservation Service, U.S. Department of Agriculture: Washington, DC, USA, 2021. Available online: https://www.nrcs.usda.gov/wps/portal/nrcs/main/soils/health/mgnt/ (accessed on 17 August 2021).
- Miner, G.L.; Delgado, J.A.; Ippolito, J.A.; Stewart, C.E. Soil health management practices and crop productivity. Agric. Environ. Lett. 2020 , 5 , e20023. [ Google Scholar ] [ CrossRef ]
- Lehmann, J.; Bossio, D.A.; Kogel-Knabner, I.; Rillig, M.C. The concept and future prospects of soil health. Nat. Rev. Earth Environ. 2020 , 1 , 544–553. [ Google Scholar ] [ CrossRef ]
- Bunemann, E.K.; Bongiorno, G.; Bai, Z.; Creamer, R.; Deyn, G.; de Goede, R.; Fleskens, L.; Geissen, V.; Kuyper, T.; Mader, P.; et al. Soil quality-A critical review. Soil Biol. Biochem. 2018 , 120 , 105–125. [ Google Scholar ] [ CrossRef ]
- Brevik, E.C. A Brief History of the Soil Health Concept ; Soil Science Society of America: Madison, WI, USA, 2018; Available online: https://profile.soils.org/files/soil-communication/documents/95_document1_d50a8524-dbf4-4856-8c62-4a03ede6c3d4.pdf (accessed on 17 August 2021).
- Wallace, H.A. Relation Between Livestock Farming and the Fertility of the Land. Retrospective Theses and Dissertations. 98. Iowa State University, Ames, IA, USA, 1910. Available online: https://lib.dr.iastate.edu/rtd/98 (accessed on 2 October 2021).
- Romig, D.E.; Garlynd, M.J.; Harris, R.F.; McSweeney, K. How farmers assess soil health and quality. J. Soil Water Cons. 1995 , 50 , 229–236. [ Google Scholar ]
- Doran, J.W.; Parkin, T.B. Defining and assessing soil quality. In Defining Soil Quality for a Sustainable Environment ; SSSA Spec. Pub. 35; Doran, J.W., Coleman, D.C., Bezdicek, D.F., Stewart, B.A., Eds.; Soil Science Society of America: Madison, WI, USA, 1994; pp. 3–21. [ Google Scholar ]
- Doran, J.W.; Sarrantonio, M.; Liebig, M.A. Soil health and sustainability. Adv. Agron. 1996 , 56 , 1–54. [ Google Scholar ]
- Sojka, R.E.; Upchurch, D.R. Reservations regarding the soil quality concept. Soil Sci. Soc. Am. J. 1999 , 63 , 1039–1054. [ Google Scholar ] [ CrossRef ] [ Green Version ]
- European Commission. Causes of the 2007–2008 Global Food Crisis Identified ; European Commission DG Environment News Alert Service: Bristol, UK, 2011; Available online: https://ec.europa.eu/environment/integration/research/newsalert/pdf/225na1_en.pdf (accessed on 19 August 2021).
- Lal, R. Soil carbon sequestration impacts on global climate change and food security. Science 2004 , 304 , 1623–1627. [ Google Scholar ] [ CrossRef ] [ Green Version ]
- McBratney, A.; Field, D.J.; Koch, A. The dimensions of soil security. Geoderma 2014 , 213 , 203–213. [ Google Scholar ] [ CrossRef ] [ Green Version ]
- Norris, C.E.; Bean, G.C.; Cappellazzi, S.B.; Cope, M.; Greub, K.; Liptzin, D.; Rieke, E.; Tracy, P.; Morgan, C.; Honeycutt, C.W. Introducing the North American project to evaluate soil health. Agron. J. 2020 , 112 , 3195–3215. [ Google Scholar ] [ CrossRef ]
- Karlen, D.L.; Venum, K.S.; Sudduth, K.A.; Obrycki, J.F.; Nunes, M.R. Soil health assessment: Past accomplishments, current activities, and future opportunities. Soil Till. Res. 2019 , 195 , 104365. [ Google Scholar ] [ CrossRef ]
- USDA-NRCS. Soil Quality Indicator Sheets ; Natural Resources Conservation Service, U.S. Department of Agriculture: Washington, DC, USA, 2015. Available online: https://www.nrcs.usda.gov/wps/portal/nrcs/detail/soils/health/assessment/?cid=stelprdb1237387 (accessed on 20 August 2021).
- SHI. North American Project to Evaluate Soil Health Measurements ; Soil Health Institute: Morrisville, NC, USA, 2021; Available online: https://soilhealthinstitute.org/north-american-project-to-evaluate-soil-health-measurements/ (accessed on 15 August 2021).
- SHI. National Soil Health Measurements to Accelerate Agricultural Transformation ; Soil Health Institute: Morrisville, NC, USA, 2021; Available online: https://soilhealthinstitute.org/national-soil-health-measurements-accelerate-agricultural-transformation/ (accessed on 21 August 2021).
- USDA-NRCS. Recommended Soil Health Indicators and Associated Laboratory Procedures ; Soil Health Technical Note No. 450-03; Natural Resources Conservation Service, U.S. Department of Agriculture: Washington, DC, USA, 2019.
- Nunes, M.R.; Veum, K.S.; Parker, P.A.; Holan, S.; Karlen, D.; Amsili, J.; van Es, H.; Wills, S.; Seybold, C.; Moorman, T. The soil health assessment protocol and evaluation applied to soil organic carbon. Soil Sci. Soc. Am. J. 2021 , 85 , 1–18. [ Google Scholar ] [ CrossRef ]
- Paz-Ferreiro, J.; Fu, S. Biological indices for soil quality evaluation: Perspectives and limitations. Land Degrad. Dev. 2016 , 27 , 14–25. [ Google Scholar ] [ CrossRef ]
- Wander, M.M.; Cihacek, L.J.; Coyne, M.; Drijber, R.; Grossman, J.; Gutknecht, J.; Horwath, W.; Jagamma, S.; Olk, D.; Ruark, M.; et al. Developments in agricultural soil quality and health: Reflections by the research committee on soil organic matter management. Front. Environ. Sci. 2019 , 7 , 109. [ Google Scholar ] [ CrossRef ] [ Green Version ]
- Andrews, S.S.; Karlen, D.L.; Cambardella, C.A. The soil management assessment framework: A quantitative soil quality evaluation method. Soil Sci. Soc. Am. J. 2004 , 68 , 1945–1962. [ Google Scholar ] [ CrossRef ]
- Veum, K.S.; Sudduth, K.A.; Kremer, R.J.; Kitchen, N.R. Sensor data fusion for soil health assessment. Geoderma 2017 , 305 , 53–61. [ Google Scholar ] [ CrossRef ]
- Jian, J.; Du, X.; Stewart, R.D. Quantifying cover crop effects on soil health and productivity. Data Brief 2020 , 29 , 105376. [ Google Scholar ] [ CrossRef ] [ PubMed ]
- SQI. Interpreting Soil Conditioning Index: A Tool for Measuring Soil Organic Matter Trends ; Soil Quality-Agronomy Technical Note No. 16; Soil Quality Institute: Auburn, AL, USA, 2003. Available online: https://www.nrcs.usda.gov/Internet/FSE_DOCUMENTS/nrcs142p2_053273.pdf (accessed on 22 August 2021).
- Wienhold, B.J.; Pikul, J.L.; Liebig, M.A.; Mikha, M.M.; Varvel, G.E.; Doran, J.W.; Andrews, S.S. Cropping system effects on soil quality in the Great Plains: Synthesis from a regional project. Renew. Agric. Food Syst. 2006 , 21 , 49–59. [ Google Scholar ] [ CrossRef ]
- USDA-NRCS. Soil Health Contacts ; Natural Resources Conservation Service, U.S. Department of Agriculture: Washington, DC, USA, 2021. Available online: https://www.nrcs.usda.gov/wps/portal/nrcs/detailfull/soils/health/?cid=stelprdb1237522 (accessed on 20 August 2021).
- SHI. About the Soil Health Institute ; Soil Health Institute: Morrisville, NC, USA, 2021; Available online: https://soilhealthinstitute.org/about-us/ (accessed on 22 August 2021).
- EC. Proposal for a Soil Health Mission: Caring for Soil is Caring for Life ; European Commission: Berlin, Germany, 2020; Available online: https://ec.europa.eu/jrc/en/science-update/proposal-soil-health-mission-caring-soil-caring-life (accessed on 22 August 2021).
- HAS. About Healthy Soil Australia ; Healthy Soil Australia: Dubbo, NSW, Australia, 2021; Available online: https://www.healthysoils.com.au/about (accessed on 22 August 2021).
- Maharjan, B.; Das, S.; Acharya, B.S. Soil health gap: A concept to establish a benchmark for soil health management. Glob. Ecol. Conserv. 2020 , 23 , e01116. [ Google Scholar ] [ CrossRef ]
- Jian, J.; Du, X.; Stewart, R.D. A database for global soil health assessment. Sci. Data 2020 , 7 , 16. [ Google Scholar ] [ CrossRef ] [ Green Version ]
- Karlen, D.L.; Goeser, N.J.; Veum, K.S.; Yost, M.A. On-farm soil health evaluations: Challenges and opportunities. J. Soil Water Conserv. 2017 , 72 , 26A–31A. [ Google Scholar ] [ CrossRef ] [ Green Version ]
- Veum, K.S.; Sudduth, K.E.; Kremer, R.J.; Kitchen, N.R. Estimating a soil quality index with VNIR reflectance spectroscopy. Soil Sci. Soc. Am. J. 2015 , 79 , 637–649. [ Google Scholar ] [ CrossRef ]
- Cho, Y.; Sheridan, A.H.; Sudduth, K.A.; Veum, K.S. Comparison of field and laboratory VNIR spectroscopy for profile soil property estimation. Trans. ASABE 2017 , 60 , 1503–1510. [ Google Scholar ] [ CrossRef ]
- Gruver, J.B.; Weil, R.R. Farmer perceptions of soil quality and their relationship to management-sensitive soil parameters. Renew. Agric. Food Syst. 2006 , 24 , 271–281. [ Google Scholar ] [ CrossRef ] [ Green Version ]
- Rekik, F.; van Es, H.; Hernandez-Aguilera, J.N.; Gomez, M.I. Understanding soil health and associated farmers’ perceptions in Columbian coffee systems. J. Soil Water Conserv. 2020 , 75 , 499–504. [ Google Scholar ] [ CrossRef ]
- USDA-NRCS. Soil Quality Card Design Guide ; Natural Resources Conservation Service, U.S. Department of Agriculture: Washington, DC, USA, 1999. Available online: https://www.nrcs.usda.gov/Internet/FSE_DOCUMENTS/nrcs142p2_051879.pdf (accessed on 24 August 2021).
- MDSHC. Maryland Soil Health Card ; Maryland Department of Agriculture: Annapolis, MD, USA, 2018. Available online: https://mda.maryland.gov/resource_conservation/counties/SoilHealthCard.pdf (accessed on 24 August 2021).
- India DACFW. Soil Health Card ; Department of Agriculture, Cooperation, and Farmers Welfare: New Delhi, India, 2021. Available online: https://www.india.gov.in/spotlight/soil-health-card#tab=tab-1 (accessed on 24 August 2021).
- Woods End Laboratories. Soil Health and Nutrient Test Quick Guide ; Woods End Laboratories, Inc.: Mount Vernon, ME, USA, 2021; Available online: https://www.woodsend.com/wp-content/uploads/2021/05/Soil-Health-Quick-Guide-Vers-4.4.pdf (accessed on 15 August 2021).
- Six, J.; Elliott, E.T.; Paustian, K. Soil structure and soil organic matter. II. A normalized stability index and the effect of mineralogy. Soil Sci. Soc. Amer. J. 2000 , 64 , 1042–1049. [ Google Scholar ] [ CrossRef ]
- Carter, M.R.; Gregorich, E.G. Soil Sampling and Methods of Analysis , 2nd ed.; CRC Press: Boca Raton: FL, USA, 2007. [ Google Scholar ]
- Chahal, I.; Hooker, D.C.; Deen, B.; Janovicek, K.; van Eerd, L.L. Long-term effects of crop rotation, tillage, and fertilizer nitrogen on soil health indicators and crop productivity in a temperate climate. Soil Till. Res. 2021 , 213 , 105121. [ Google Scholar ] [ CrossRef ]
- Sullivan, D.M.; Granatstein, D. Are “Haney Tests” Meaningful Indicators of Soil Health and Estimators of Nitrogen Fertilizer Credits? Nutr. Dig. 2015 , 7 , 3, Western Extension/Education Region Activities Nutrient Management and Water Quality Committee (WERA-103): Moscow, ID, USA. Available online: https://www.vineyardteam.org/files/resources/Are%20Haney%20Tests%20Meaningful.pdf (accessed on 25 August 2021).
- Yost, M.A.; Veum, K.S.; Kitchen, N.R.; Sawyer, J.; Camberato, J.; Carter, P.; Ferguson, R.; Fernandez, F.; Franzen, D.; Laboski, C.; et al. Evaluation of the Haney soil health tool for corn nitrogen recommendations across eight Midwest states. J. Soil. Water Conserv. 2018 , 73 , 587–592. [ Google Scholar ] [ CrossRef ] [ Green Version ]
- Chu, M.; Singh, S.; Walker, F.R.; Buschermohle, M.; Jagadamma, S.; Singh, S.; Eash, N.; Funcan, L. Soil health and soil fertility assessment by the Haney soil health test in an agricultural soil in west Tennessee. Commun. Soil Sci. Plant Anal. 2019 , 50 , 1123–1131. [ Google Scholar ] [ CrossRef ]
- Congreves, K.A.; Hayes, A.; Verhallen, E.A.; van Eerd, L.L. Long-term impact of tillage and crop rotation on soil health at four temperate agroecosystems. Soil Till. Res. 2015 , 152 , 17–28. [ Google Scholar ] [ CrossRef ]
- Ye, R.; Parajuli, B.; Szogi, A.A.; Sigua, G.C.; Ducey, T.F. Soil health assessment after 40 years of conservation and conventional tillage management in Southeastern Coastal Plain soils. Soil Sci. Soc. Am. J. 2021 , 85 , 20246. [ Google Scholar ] [ CrossRef ]
- USDA-NRCS. Soil Health: Principle 1 of 5—Soil Armor ; Natural Resources Conservation Service, U.S. Department of Agriculture: Washington, DC, USA, 2016. Available online: https://www.nrcs.usda.gov/wps/portal/nrcs/detailfull/nd/soils/health/?cid=nrcseprd1300631 (accessed on 26 August 2021).
- USDA-NRCS. Honoring 86 Years of NRCS—A Brief History ; Natural Resources Conservation Service, U.S. Department of Agriculture: Washington, DC, USA, 2020. Available online: https://www.nrcs.usda.gov/wps/portal/nrcs/detail/national/about/history/?cid=nrcs143_021392 (accessed on 27 August 2021).
- Troeh, F.R.; Donahue, R.L.; Hobbs, J.A. Soil and Water Conservation for Productivity and Environmental Production ; Person Education: Upper Saddle River, NJ, USA, 2004. [ Google Scholar ]
- Berendsen, R.L.; Pieterse, C.M.J.; Bakker, P.A.H.M. The rhizosphere microbiome and plant health. Trends Plant Sci. 2012 , 17 , 478–486. [ Google Scholar ] [ CrossRef ]
- USDA-NRCS. Rotations for Pest Management ; Natural Resources Conservation Service, U.S. Department of Agriculture: Washington, DC, USA, 2009. Available online: https://www.nrcs.usda.gov/Internet/FSE_DOCUMENTS/nrcs142p2_017827.pdf (accessed on 28 August 2021).
- Chowdhury, S.; Bolan, N.; Farrell, M.; Sarkar, B.; Sarker, J.; Kirkham, M.; Hossain, Z.; Kim, G.-H. Role of cultural and nutrient management practices in carbon sequestration in agricultural soil. Adv. Agron. 2021 , 166 , 131–169. [ Google Scholar ]
- Van Eerd, L.L.; Congreves, K.A.; Hayes, A.; Verhallen, A.; Hooker, D.C. Long-term tillage and crop rotation effects on soil quality, organic carbon, and total nitrogen. Can. J. Soil Sci. 2014 , 94 , 303–315. [ Google Scholar ] [ CrossRef ]
- Hartwig, N.L.; Ammon, H.U. Cover crops and living mulches. Weed Sci. 2002 , 50 , 688–699. [ Google Scholar ] [ CrossRef ]
- Magdoff, F.; van Es, H. Cover crops. In Building Soils for Better Crops—Ecological Management for Healthy Soils , 4th ed.; Magdoff, F., van Es, H., Eds.; Sustainable Agriculture Research and Education: College Park, MD, USA, 2021; pp. 137–158. [ Google Scholar ]
- Lawson, A.; Cogger, C.; Bary, A.; Fortuna, A.M. Influence of seeding ratio, planting date, and termination date on rye-hairy vetch cover crop mixture performance under organic management. PLoS ONE 2015 , 10 , e0129597. [ Google Scholar ] [ CrossRef ]
- Vukicevich, E.; Lowery, T.; Bowen, P.; Úrbez-Torres, J.R.; Hart, M. Cover crops to increase soil microbial diversity and mitigate decline in perennial agriculture. A review. Agron. Sustain. Dev. 2016 , 36 , 48. [ Google Scholar ] [ CrossRef ] [ Green Version ]
- Sharma, P.; Singh, A.; Kahlon, C.; Brar, A.; Grover, K.; Dia, M.; Steiner, R. The role of cover crops towards sustainable soil health and agriculture—A review paper. Am. J. Plant Sci. 2018 , 9 , 1935–1951. [ Google Scholar ] [ CrossRef ] [ Green Version ]
- Finney, D.M.; Buyer, J.S.; Kaye, J.P. Living cover crops have immediate impacts on soil microbial community structure and function. J. Soil Water. Conserv. 2017 , 72 , 361–373. [ Google Scholar ] [ CrossRef ] [ Green Version ]
- Kumar, S.; Reitsma, K. Soil tillage. In iGrow Corn: Best Management Practices ; Clay, D.E., Carlson, C.G., Clay, S.A., Byamukama, E., Eds.; South Dakota State University: Brookings, SD, USA, 2019; Available online: https://extension.sdstate.edu/sites/default/files/2019-09/S-0003-11-Corn.pdf (accessed on 28 August 2021).
- Nunes, M.; Karlen, D.L.; Veum, K.S.; Moorman, T.B. Biological soil health indicators respond to tillage intensity: A US meta analysis. Geoderma 2020 , 369 , 114335. [ Google Scholar ] [ CrossRef ]
- Fangueiro, D.; Becerra, D.; Albarrán, A.; Pena, D.; Sanchez-llerena, J.; Nunes, J.M.; Lopez Pineiro, A. Effect of tillage and wáter management on GHG emissions from Mediterranean rice growing ecosystems. Atmos. Environ. 2017 , 150 , 303–312. [ Google Scholar ] [ CrossRef ]
- Pieper, J.R.; Brown, R.N.; Amador, J.A. Effects of three conservation tillage strategies on yields and soil health in a mixed vegetable production system. HortScience 2015 , 50 , 1770–1776. [ Google Scholar ] [ CrossRef ]
- Zahid, A.; Ali, S.; Ahmed, M.; Iqbal, N. Improvement of soil health through residue management and conservation tillage in rice-wheat cropping system of Punjab, Pakistan. Agronomy 2020 , 10 , 1844. [ Google Scholar ] [ CrossRef ]
- Cooper, R.J.; Hama-Aziz, Z.Q.; Hiscock, K.M. Conservation tillage and soil health: Lessons from a 5-year UK farm trial (2013-2018). Soil Till. Res. 2020 , 202 , 104648. [ Google Scholar ] [ CrossRef ]
- Courtney, R.G.; Mullen, G.J. Soil quality and barley growth as influenced by the land application of two compost types. Bioresour. Technol. 2008 , 99 , 2913–2918. [ Google Scholar ] [ CrossRef ]
- Guo, M.; Chorover, J.; Fox, R.; Rosario, R. Leachate chemistry of field-weathered spent mushroom substrate. J. Environ. Qual. 2001 , 30 , 1699–1709. [ Google Scholar ] [ CrossRef ] [ Green Version ]
- Guo, M.; Tongtavee, N.; Labreveux, M. Nutrient dynamics of field-weathered Delmarva poultry litter: Implications for land application. Biol. Fertil. Soils 2009 , 45 , 829–838. [ Google Scholar ] [ CrossRef ]
- Guo, M.; Song, W.; Kazda, R. Fertilizer value of lime-stabilized biosolids as a soil amendment. Agron. J. 2012 , 104 , 1679–1686. [ Google Scholar ] [ CrossRef ] [ Green Version ]
- Song, W.; Guo, M. Quality variations of poultry litter biochars generated at different pyrolysis temperatures. J. Anal. Appl. Pyrolysis 2012 , 94 , 138–145. [ Google Scholar ] [ CrossRef ]
- Sekaran, U.; Kumar, S.; Gonzalez-Hernandez, J.L. Integration of crop and livestock enhanced soil biochemical properties and microbial community structure. Geoderma 2020 , 381 , 114686. [ Google Scholar ] [ CrossRef ]
- Undersander, D.; Albert, B.; Cosgrove, D.; Johnson, D.; Peterson, P. Pastures for Profit: A Guide to Rotational Grazing ; A3529; University of Wisconsin Cooperative Extension: Madison, WI, USA, 2002. Available online: https://www.nrcs.usda.gov/Internet/FSE_DOCUMENTS/stelprdb1097378.pdf (accessed on 28 August 2021).
Click here to enlarge figure
Soil Health Indicator | Soil Function | ||||
---|---|---|---|---|---|
Sustain Biological Diversity, Activity, and Productivity | Regulate and Partition Water and Solute Flow | Filter, Buffer, Degrade, Detoxify Organic and Inorganic Materials | Store and Cycle Nutrients and Carbon | Physical Stability and Support for Plants and Engineering Structures | |
SAS | ++++ | ++++ | ++++ | +++++ | |
AWC | ++++++ | +++++ | ++++ | ||
Bulk density | +++++ | ++++++ | ++ | ++++++ | |
Earthworms | ++++++ | ++++++ | ++++++ | ++++++ | |
Infiltration | ++++ | ++ | |||
POM | ++++++ | ++++++ | ++++++ | ++++++ | ++++++ |
PMN | ++++++ | ++++++ | |||
Reactive C | ++++ | ++ | ++++++ | ++++ | ++++ |
Slaking | ++ | +++++ | |||
Soil crusts | +++++ | ||||
Soil EC | ++++++ | ||||
Soil enzymes | ++++++ | ++++++ | |||
Soil nitrate | ++ | ++ | |||
Soil pH | ++++ | ++++++ | ++++++ | ++++++ | |
Soil respiration | ++++++ | ++ | ++++++ | ++++ | |
Soil structure | +++ | +++ | ++ | ++ | ++++ |
TOC | ++++++ | ++++++ | ++++++ | ++++++ | ++++++ |
Soil Health Indicators | Criteria | Examples |
---|---|---|
Tier 1 | Soil texture Soil bulk density Soil aggregate stability Available water-holding capacity Saturated hydraulic conductivity Soil pH Soil electrical conductivity Cation exchange capacity Base saturation Extractable P, Ca, Mg, K, Fe, Mn, Cu, Zn Extractable Al, As, B, Ba, Cd, Co, Cr, Mo, Ni, Pb, Si, Sr Soil total nitrogen content Nitrogen mineralization rate Soil organic carbon content Short-term carbon mineralization Crop yield | |
Tier 2 | Soil sodium adsorption ratio Macro-aggregate stability Soil stability index Soil active carbon Soil protein index Soil β-glucosidase Soil N-acetyl-β-D glucosaminidase Soil phosphomonoesterase Soil arylsulfatase Soil phospholipid fatty acid (PLFA) profile Soil fatty acid methyl ester (FAME) profile Soil microbial genomics Soil reflectance | |
Tier 3 | Soil microbial community structure Soil microbial DNA extraction and sequencing |
Tillage System | Soil Conditions Prior to Planting | Operation | Weed Control Methods |
---|---|---|---|
Ridge till | Undisturbed; ridge scalp at planting | Till land using a sweep cultivator to form 10–15 cm height ridges; seeds are planted in ridges | Combined herbicide application and soil cultivation |
Strip till | Undisturbed; strip till at planting | Narrow and shallow tillage using a rotary tiller or an in-row chisel planter; ~1/3 surface tilled at planting | Combined herbicide application and soil cultivation |
Stubble mulch till | Tilled—residues ≥ 30% | Use a chisel tiller, a blade plow, or a sweep cultivator to till stubble-covered land | Combined herbicide application and soil cultivation |
Reduced till | Tilled—residues ≥ 30% | Reduce till of cropland using a chisel tiller, a disc plow, or a blade plow to prepare seed beds | Combined herbicide application and soil cultivation |
No till | Undisturbed | Use a drill planter (no-till seeder) to sow seeds | Herbicides |
MDPI stays neutral with regard to jurisdictional claims in published maps and institutional affiliations. |
Share and Cite
Guo, M. Soil Health Assessment and Management: Recent Development in Science and Practices. Soil Syst. 2021 , 5 , 61. https://doi.org/10.3390/soilsystems5040061
Guo M. Soil Health Assessment and Management: Recent Development in Science and Practices. Soil Systems . 2021; 5(4):61. https://doi.org/10.3390/soilsystems5040061
Guo, Mingxin. 2021. "Soil Health Assessment and Management: Recent Development in Science and Practices" Soil Systems 5, no. 4: 61. https://doi.org/10.3390/soilsystems5040061
Article Metrics
Article access statistics, further information, mdpi initiatives, follow mdpi.
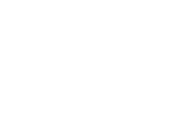
Subscribe to receive issue release notifications and newsletters from MDPI journals
Thank you for visiting nature.com. You are using a browser version with limited support for CSS. To obtain the best experience, we recommend you use a more up to date browser (or turn off compatibility mode in Internet Explorer). In the meantime, to ensure continued support, we are displaying the site without styles and JavaScript.
- View all journals
- Explore content
- About the journal
- Publish with us
- Sign up for alerts
- Perspective
- Published: 25 August 2020
The concept and future prospects of soil health
- Johannes Lehmann ORCID: orcid.org/0000-0002-4701-2936 1 , 2 , 3 ,
- Deborah A. Bossio ORCID: orcid.org/0000-0002-2296-9125 4 ,
- Ingrid Kögel-Knabner 3 , 5 &
- Matthias C. Rillig ORCID: orcid.org/0000-0003-3541-7853 6 , 7
Nature Reviews Earth & Environment volume 1 , pages 544–553 ( 2020 ) Cite this article
12k Accesses
530 Citations
158 Altmetric
Metrics details
- Biogeochemistry
- Environmental sciences
- Sustainability
Soil health is the continued capacity of soil to function as a vital living ecosystem that sustains plants, animals and humans, and connects agricultural and soil science to policy, stakeholder needs and sustainable supply-chain management. Historically, soil assessments focused on crop production, but, today, soil health also includes the role of soil in water quality, climate change and human health. However, quantifying soil health is still dominated by chemical indicators, despite growing appreciation of the importance of soil biodiversity, owing to limited functional knowledge and lack of effective methods. In this Perspective, the definition and history of soil health are described and compared with other soil concepts. We outline ecosystem services provided by soils, the indicators used to measure soil functionality and their integration into informative soil-health indices. Scientists should embrace soil health as an overarching principle that contributes to sustainability goals, rather than only a property to measure.
This is a preview of subscription content, access via your institution
Access options
Access Nature and 54 other Nature Portfolio journals
Get Nature+, our best-value online-access subscription
$29.99 / 30 days
cancel any time
Subscribe to this journal
Receive 12 digital issues and online access to articles
$99.00 per year
only $8.25 per issue
Buy this article
- Purchase on Springer Link
- Instant access to full article PDF
Prices may be subject to local taxes which are calculated during checkout
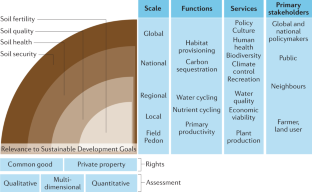
Similar content being viewed by others
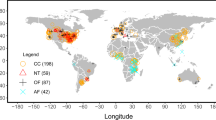
A database for global soil health assessment
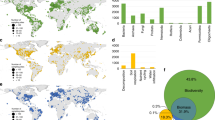
Blind spots in global soil biodiversity and ecosystem function research
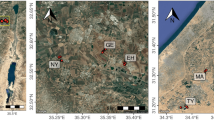
A sensitive soil biological indicator to changes in land-use in regions with Mediterranean climate
Ladyman, J., Lambert, J. & Wiesner, K. What is a complex system? Eur. J. Philos. Sci. 3 , 33–67 (2013).
Google Scholar
Brevik, E. C. et al. The interdisciplinary nature of SOIL. Soil 1 , 117–129 (2015).
Blum, W. E. Functions of soil for society and the environment. Rev. Environ. Sci. Biotechnol. 4 , 75–79 (2005).
Baveye, P. C., Baveye, J. & Gowdy, J. Soil “ecosystem” services and natural capital: critical appraisal of research on uncertain ground. Front. Environ. Sci. 4 , 41 (2016).
Keith, A. M., Schmidt, O. & McMahon, B. J. Soil stewardship as a nexus between ecosystem services and one health. Ecosyst. Serv. 17 , 40–42 (2016).
Bünemann, E. K. et al. Soil quality–a critical review. Soil Biol. Biochem. 120 , 105–125 (2018).
Patzel, N., Sticher, H. & Karlen, D. L. Soil fertility - phenomenon and concept. J. Plant Nutr. Soil Sci. 163 , 129–142 (2000).
Doran, J. W. & Parkin, T. B. in Defining Soil Quality for a Sustainable Environment Vol. 32 (eds Doran, J. W., Coleman, D. C., Bezdicek, D. F. & Stewart, B. A.) 1–21 (Soil Science Society of America, 1994).
Pankhurst, C. E., Doube, B. M. & Gupta, V. V. S. R. in Biological Indicators of Soil Health (eds Pankhurst, C., Doube, B. & Gupta, V.) 419–435 (CAB International, 1997).
McBratney, A., Field, D. J. & Koch, A. The dimensions of soil security. Geoderma 213 , 203–213 (2014).
Koch, A. et al. Soil security: solving the global soil crisis. Glob. Policy 4 , 434–441 (2013).
Stankovics, P., Tóth, G. & Tóth, Z. Identifying gaps between the legislative tools of soil protection in the EU member states for a common European soil protection legislation. Sustainability 10 , 2886 (2018).
Montanarella, L. Agricultural policy: govern our soils. Nature 528 , 32–33 (2015).
Jian, J., Du, X. & Stewart, R. D. A database for global soil health assessment. Sci. Data 7 , 16 (2020).
Karlen, D. L., Veum, K. S., Sudduth, K. A., Obrycki, J. F. & Nunes, M. R. Soil health assessment: past accomplishments, current activities, and future opportunities. Soil Tillage Res. 195 , 104365 (2019).
Norris, C. E. & Congreves, K. A. Alternative management practices improve soil health indices in intensive vegetable cropping systems: a review. Front. Environ. Sci. 6 , 50 (2018).
O’Dell, R. E. & Claassen, V. P. Vertical distribution of organic amendment influences the rooting depth of revegetation species on barren, subgrade serpentine substrate. Plant Soil 285 , 19–29 (2006).
Congreves, K. A., Hayes, A., Verhallen, E. A. & Van Eerd, L. L. Long-term impact of tillage and crop rotation on soil health at four temperate agroecosystems. Soil Tillage Res. 152 , 17–28 (2015).
Hamza, M. A. & Anderson, W. K. Soil compaction in cropping systems: a review of the nature, causes and possible solutions. Soil Tillage Res. 82 , 121–145 (2005).
Jenkinson, D. S. The Rothamsted long-term experiments: Are they still of use? Agron. J. 83 , 2–10 (1991).
Berendsen, R. L., Pieterse, C. M. & Bakker, P. A. The rhizosphere microbiome and plant health. Trends Plant Sci. 17 , 478–486 (2012).
Chaparro, J. M., Sheflin, A. M., Manter, D. K. & Vivanco, J. M. Manipulating the soil microbiome to increase soil health and plant fertility. Biol. Fertil. Soils 48 , 489–499 (2012).
Bonanomi, G., Lorito, M., Vinale, F. & Woo, S. L. Organic amendments, beneficial microbes, and soil microbiota: toward a unified framework for disease suppression. Annu. Rev. Phytopathol. 56 , 1–20 (2018).
Chen, X. D. et al. Soil biodiversity and biogeochemical function in managed ecosystems. Soil Res. 58 , 1–20 (2020).
Pittelkow, C. M. et al. Productivity limits and potentials of the principles of conservation agriculture. Nature 517 , 365–368 (2015).
Ogle, S. M., Swan, A. & Paustian, K. No-till management impacts on crop productivity, carbon input and soil carbon sequestration. Agric. Ecosyst. Environ. 149 , 37–49 (2012).
Zimnicki, T. et al. On quantifying water quality benefits of healthy soils. BioScience 70 , 343–352 (2020).
Evans, A. E., Mateo-Sagasta, J., Qadir, M., Boelee, E. & Ippolito, A. Agricultural water pollution: key knowledge gaps and research needs. Curr. Opin. Environ. Sustain. 36 , 20–27 (2019).
Carpenter, S. R. et al. Nonpoint pollution of surface waters with phosphorus and nitrogen. Ecol. Appl. 8 , 559–568 (1998).
Lamichhane, S., Krishna, K. B. & Sarukkalige, R. Polycyclic aromatic hydrocarbons (PAHs) removal by sorption: a review. Chemosphere 148 , 336–353 (2016).
Tournebize, J., Chaumont, C. & Mander, Ü. Implications for constructed wetlands to mitigate nitrate and pesticide pollution in agricultural drained watersheds. Ecol. Eng. 103 , 415–425 (2017).
Hanson, J. R., Macalady, J. L., Harris, D. & Scow, K. M. Linking toluene degradation with specific microbial populations in soil. Appl. Environ. Microbiol. 65 , 5403–5408 (1999).
Li, G., Sun, G. X., Ren, Y., Luo, X. S. & Zhu, Y. G. Urban soil and human health: a review. Eur. J. Soil Sci. 69 , 196–215 (2018).
Laurenson, G., Laurenson, S., Bolan, N., Beecham, S. & Clark, I. The role of bioretention systems in the treatment of stormwater. Adv. Agron. 120 , 223–274 (2013).
Wagg, C., Bender, S. F., Widmer, F. & van der Heijden, M. G. Soil biodiversity and soil community composition determine ecosystem multifunctionality. Proc. Natl Acad. Sci. USA 111 , 5266–5270 (2014).
Kadam, A. M., Oza, G. H., Nemade, P. D. & Shankar, H. S. Pathogen removal from municipal wastewater in constructed soil filter. Ecol. Eng. 33 , 37–44 (2008).
Welch, R. M. & Graham, R. D. Breeding for micronutrients in staple food crops from a human nutrition perspective. J. Exp. Bot. 55 , 353–364 (2004).
Barrett, C. B. & Bevis, L. E. The self-reinforcing feedback between low soil fertility and chronic poverty. Nat. Geosci. 8 , 907–912 (2015).
Wood, S. A., Tirfessa, D. & Baudron, F. Soil organic matter underlies crop nutritional quality and productivity in smallholder agriculture. Agric. Ecosyst. Environ. 266 , 100–108 (2018).
Wall, D. H., Nielsen, U. N. & Six, J. Soil biodiversity and human health. Nature 528 , 69–76 (2015).
Jacoby, R., Peukert, M., Succurro, A., Koprivova, A. & Kopriva, S. The role of soil microorganisms in plant mineral nutrition—current knowledge and future directions. Front. Plant Sci. 8 , 1617 (2017).
Schlatter, D., Kinkel, L., Thomashow, L., Weller, D. & Paulitz, T. Disease suppressive soils: new insights from the soil microbiome. Phytopathology 107 , 1284–1297 (2017).
Rillig, M. C., Lehmann, A., Lehmann, J., Camenzind, T. & Rauh, C. Soil biodiversity effects from field to fork. Trends Plant Sci. 23 , 17–24 (2018).
Oliver, M. A. & Gregory, P. J. Soil, food security and human health: a review. Eur. J. Soil Sci. 66 , 257–276 (2015).
Hussein, H. S. & Brasel, J. M. Toxicity, metabolism, and impact of mycotoxins on humans and animals. Toxicology 167 , 101–134 (2001).
Bethony, J. et al. Soil-transmitted helminth infections: ascariasis, trichuriasis, and hookworm. Lancet 367 , 1521–1532 (2006).
Schatz, A., Bugle, E. & Waksman, S. A. Streptomycin, a substance exhibiting antibiotic activity against gram-positive and gram-negative bacteria. Proc. Soc. Exp. Biol. Med. 55 , 66–69 (1944).
Ling, L. L. et al. A new antibiotic kills pathogens without detectable resistance. Nature 517 , 455–459 (2015).
Veresoglou, S. D., Halley, J. M. & Rillig, M. C. Extinction risk of soil biota. Nat. Commun. 6 , 8862 (2015).
Lal, R. Soil carbon sequestration impacts on global climate change and food security. Science 304 , 1623–1627 (2004).
Paustian, K. et al. Climate-smart soils. Nature 532 , 49–57 (2016).
Denef, K. & Six, J. Clay mineralogy determines the importance of biological versus abiotic processes for macroaggregate formation and stabilization. Eur. J. Soil Sci. 56 , 469–479 (2005).
Rinot, O., Levy, G. J., Steinberger, Y., Svoray, T. & Eshel, G. Soil health assessment: A critical review of current methodologies and a proposed new approach. Sci. Total. Environ. 648 , 1484–1491 (2019).
Van Wesemael, B. et al. An indicator for organic matter dynamics in temperate agricultural soils. Agric. Ecosyst. Environ. 274 , 62–75 (2019).
Bouma, J. et al. in Global Soil Security (eds Field, D. J., Morgan, C. L. S. & McBratney, A. B.) 27–44 (Springer, 2017).
Schoenholtz, S. H., Van Miegroet, H. & Burger, J. A. A review of chemical and physical properties as indicators of forest soil quality: challenges and opportunities. For. Ecol. Manag. 138 , 335–356 (2000).
Andrews, S. S. & Carroll, C. R. Designing a soil quality assessment tool for sustainable agroecosystem management. Ecol. Appl. 11 , 1573–1585 (2001).
Lilburne, L. R., Hewitt, A. E., Sparling, G. P. & Selvarajah, N. Soil quality in New Zealand: policy and the science response. J. Environ. Qual. 31 , 1768–1773 (2002).
Idowu, O. J. et al. Use of an integrative soil health test for evaluation of soil management impacts. Renew. Agric. Food Syst. 24 , 214–224 (2009).
Cherubin, M. R. et al. A Soil Management Assessment Framework (SMAF) evaluation of Brazilian sugarcane expansion on soil quality. Soil Sci. Soc. Am. J. 80 , 215–226 (2016).
E.U. Mission Board Soil Health and Food. Caring for Soil is Caring for Life . The Publications Office of the European Union https://op.europa.eu/en/web/eu-law-and-publications/publication-detail/-/publication/32d5d312-b689-11ea-bb7a-01aa75ed71a1 (European Commission, 2020).
Nunes, M. R., Karlen, D. L., Veum, K. S., Moorman, T. B. & Cambardella, C. A. Biological soil health indicators respond to tillage intensity: a US meta-analysis. Geoderma 369 , 114335 (2020).
Kaiser, E. A. et al. Nitrous oxide release from arable soil: importance of N-fertilization, crops and temporal variation. Soil Biol. Biochem. 30 , 1553–1563 (1998).
Baldock, J. A., Beare, M. H., Curtin, D. & Hawke, B. Stocks, composition and vulnerability to loss of soil organic carbon predicted using mid-infrared spectroscopy. Soil Res. 56 , 468–480 (2018).
Rossel, R. V. et al. Continental-scale soil carbon composition and vulnerability modulated by regional environmental controls. Nat. Geosci. 12 , 547–552 (2019).
Six, J., Bossuyt, H., Degryze, S. & Denef, K. A history of research on the link between (micro)aggregates, soil biota, and soil organic matter dynamics. Soil Tillage Res. 79 , 7–31 (2004).
Pietrelli, A., Bavasso, I., Lovecchio, N., Ferrara, V. & Allard, B. in 8th IEEE International Workshop on Advances in Sensors and Interfaces (IWASI) 302–306 (IEEE, 2019).
Shaikh, F. K. & Zeadally, S. Energy harvesting in wireless sensor networks: a comprehensive review. Renew. Sustain. Energy Rev. 55 , 1041–1054 (2016).
Tan, X., Sun, Z., Wang, P. & Sun, Y. Environment-aware localization for wireless sensor networks using magnetic induction. Ad Hoc Netw. 98 , 102030 (2020).
Lehmann, J. et al. Persistence of soil organic carbon caused by functional complexity. Nat. Geosci. 13 , 529–534 (2020).
Tedersoo, L. et al. Global diversity and geography of soil fungi. Science 346 , 1078 (2014).
Van Den Hoogen, J. et al. Soil nematode abundance and functional group composition at a global scale. Nature 572 , 194–198 (2019).
Rillig, M. C., Bonneval, K. & Lehmann, J. Sounds of soil: a new world of interactions under our feet? Soil Syst. 3 , 45 (2019).
Smolka, M. et al. A mobile lab-on-a-chip device for on-site soil nutrient analysis. Precision Agric. 18 , 152–168 (2017).
Rossel, R. A. V. & Bouma, J. Soil sensing: a new paradigm for agriculture. Agric. Syst. 148 , 71–74 (2016).
Ali, M. A., Dong, L., Dhau, J., Khosla, A. & Kaushik, A. Perspective — electrochemical sensors for soil quality assessment. J. Electrochem. Soc. 167 , 037550 (2020).
Enell, A. et al. Combining leaching and passive sampling to measure the mobility and distribution between porewater, DOC, and colloids of native oxy-PAHs, N-PACs, and PAHs in historically contaminated soil. Environ. Sci. Technol. 50 , 11797–11805 (2016).
Sismaet, H. J. & Goluch, E. D. Electrochemical probes of microbial community behaviour. Annu. Rev. Anal. Chem. 11 , 441–461 (2018).
Chabrillat, S. et al. Imaging spectroscopy for soil mapping and monitoring. Surv. Geophys. 40 , 361–399 (2019).
Mohanty, B. P., Cosh, M. H., Lakshmi, V. & Montzka, C. Soil moisture remote sensing: state-of-the-science. Vadose Zone J. 16 , 1–9 (2017).
Paustian, K. et al. Quantifying carbon for agricultural soil management: from the current status toward a global soil information system. Carbon Manage. 10 , 567–587 (2019).
Duckett, T. et al. Agricultural robotics: the future of robotic agriculture. Preprint arXiv https://arxiv.org/abs/1806.06762 (2018).
Hussain, I., Olson, K. R., Wander, M. M. & Karlen, D. L. Adaptation of soil quality indices and application to three tillage systems in southern Illinois. Soil Tillage Res. 50 , 237–249 (1999).
Fine, A. K., van Es, H. M. & Schindelbeck, R. R. Statistics, scoring functions, and regional analysis of a comprehensive soil health database. Soil Sci. Soc. Am. J. 81 , 589–601 (2017).
Svoray, T., Hassid, I., Atkinson, P. M., Moebius-Clune, B. N. & van Es, H. M. Mapping soil health over large agriculturally important areas. Soil Sci. Soc. Am. J. 79 , 1420–1434 (2015).
Moebius-Clune, B. N. et al. Comprehensive Assessment of Soil Health – The Cornell Framework, Edition 3.1 (Cornell Univ. Press, 2016).
Wiesmeier, M. et al. Soil organic carbon storage as a key function of soils-a review of drivers and indicators at various scales. Geoderma 333 , 149–162 (2019).
Lima, A. C. R., Brussaard, L., Totola, M. R., Hoogmoed, W. B. & de Goede, R. G. M. A functional evaluation of three indicator sets for assessing soil quality. Appl. Soil Ecol. 64 , 194–200 (2013).
Verheijen, F. G., Bellamy, P. H., Kibblewhite, M. G. & Gaunt, J. L. Organic carbon ranges in arable soils of England and Wales. Soil Use Manage. 21 , 2–9 (2005).
Bucka, F. B., Kölbl, A., Uteau, D., Peth, S. & Kögel-Knabner, I. Organic matter input determines structure development and aggregate formation in artificial soils. Geoderma 354 , 113881 (2019).
Kaiser, K. et al. Driving forces of soil bacterial community structure, diversity, and function in temperate grasslands and forests. Sci. Rep. 6 , 33696 (2016).
Jordan-Meille, L. et al. An overview of fertilizer-P recommendations in Europe: soil testing, calibration and fertilizer recommendations. Soil Use Manage. 28 , 419–435 (2012).
McLellan, E. L. et al. The nitrogen balancing act: tracking the environmental performance of food production. Bioscience 68 , 194–203 (2018).
Brevik, E. C. & Sauer, T. J. The past, present, and future of soils and human health studies. Soil 1 , 35–46 (2015).
Pereira, P., Bogunovic, I., Muñoz-Rojas, M. & Brevik, E. C. Soil ecosystem services, sustainability, valuation and management. Curr. Opin. Environ. Sci. Health 5 , 7–13 (2018).
Bampa, F. et al. Harvesting European knowledge on soil functions and land management using multi-criteria decision analysis. Soil Use Manage. 35 , 6–20 (2019).
Schulte, R. P. et al. Demands on land: mapping competing societal expectations for the functionality of agricultural soils in Europe. Environ. Sci. Policy 100 , 113–125 (2019).
Ward, M. O., Grinstein, G. & Keim, D. Interactive Data Visualization: Foundations, Techniques, and Applications (AK Peters/CRC Press, 2015).
Villamil, M. B., Miguez, F. E. & Bollero, G. A. Multivariate analysis and visualization of soil quality data for no-till systems. J. Environ. Qual. 37 , 2063–2069 (2008).
Börner, K., Bueckle, A. & Ginda, M. Data visualization literacy: definitions, conceptual frameworks, exercises, and assessments. Proc. Natl Acad. Sci. USA 116 , 1857–1864 (2019).
Reichstein, M. et al. Deep learning and process understanding for data-driven Earth system science. Nature 566 , 195–204 (2019).
Tian, R. & Wu, J. Groundwater quality appraisal by improved set pair analysis with game theory weightage and health risk estimation of contaminants for Xuecha drinking water source in a loess area in Northwest China. Hum. Ecol. Risk Assess. Int. J. 25 , 132–157 (2019).
Finger, R., Swinton, S. M., Benni, N. E. & Walter, A. Precision farming at the nexus of agricultural production and the environment. Annu. Rev. Resour. Econ. 11 , 313–335 (2019).
van Joolingen, W. R., de Jong, T., Lazonder, A. W., Savelsbergh, E. R. & Manlove, S. Co-Lab: research and development of an online learning environment for collaborative scientific discovery learning. Comput. Hum. Behav. 21 , 671–688 (2005).
Stott, D. E. Recommended Soil Health Indicators and Associated Laboratory Procedures. Soil Health Technical Note No. 450-03 (U.S. Department of Agriculture, Natural Resources Conservation Service, 2019).
Haberern, J. A soil health index. J. Soil Water Conserv. 47 , 6 (1992).
Pankhurst, C. E. et al. Evaluation of soil biological properties as potential bioindicators of soil health. Austr. J. Exp. Agric. 35 , 1015–1028 (1995).
Doran, J. W. & Zeiss, M. R. Soil health and sustainability: managing the biotic component of soil quality. Appl. Soil Ecol. 15 , 3–11 (2000).
Winiwarter, V. & Blum, W. E. in Footprints in the Soil. People and Ideas in Soil History (ed. Warkentin, B.) 107–122 (Elsevier, 2006).
Capra, G. F., Ganga, A. & Moore, A. F. Songs for our soils. How soil themes have been represented in popular song. Soil Sci. Plant Nutr. 63 , 517–525 (2017).
Jenny, H. in Study Week on Organic Matter and Soil Fertility. Pontificiae Academiae Scientarium Scripta, Varia 32. 947–979 (North Holland Publ. Co and Wiley Interscience Division, 1968).
Feller, C., Landa, E. R., Toland, A. & Wessolek, G. Case studies of soil in art. Soil 1 , 543–559 (2015).
Brevik, E. C. & Hartemink, A. E. Early soil knowledge and the birth and development of soil science. Catena 83 , 23–33 (2010).
Carson, R. Silent Spring (Houghton Mifflin, 1962).
Lovelock, J. E. Gaia, a New Look at Life on Earth (Oxford Univ. Press, 1979).
Keesstra, S. D. et al. The significance of soils and soil science towards realization of the United Nations Sustainable Development Goals. Soil 2 , 111–128 (2016).
Mausel, P. W. Soil quality in Illinois — an example of a soils geography resource analysis. Prof. Geogr. 23 , 127–136 (1971).
Sojka, R. E. & Upchurch, D. R. Reservations regarding the soil quality concept. Soil Sci. Soc. Am. J. 63 , 1039–1054 (1999).
Rumpel, C. et al. Put more carbon in soils to meet Paris climate pledges. Nature 564 , 32–34 (2018).
Freidberg, S. Assembled but unrehearsed: corporate food power and the ‘dance’ of supply chain sustainability. J. Peasant Stud. 47 , 383–400 (2020).
Chabbi, A. et al. Aligning agriculture and climate policy. Nat. Clim. Change 7 , 307–309 (2017).
Puig de la Bellacasa, M. Re-animating soils: transforming human–soil affections through science, culture and community. Sociol. Rev. 67 , 391–407 (2019).
Download references
Acknowledgements
J.L. acknowledges the Hans Fischer Senior Fellowship of the Institute for Advanced Study (Technical University Munich) and a TNC-ACSF project (Cornell University), D.A.B. the support by the Craig and Susan McCaw Foundation, I.K.-K. the support by the German Federal Ministry of Education and Research (BMBF) in the framework of the funding measure ‘Soil as a Sustainable Resource for the Bioeconomy’ (BonaRes project), BonaRes Centre for Soil Research (FKZ 031B0516A; BonaRes, Module A) and M.C.R. an ERC Advanced Grant (694368) and the BMBF for the project ‘Bridging in Biodiversity Science (BIBS)’ (01LC1501A). Sincere thanks to Else Bünemann-König for sharing raw data.
Author information
Authors and affiliations.
School of Integrative Plant Science, Cornell University, Ithaca, NY, USA
Johannes Lehmann
Cornell Atkinson Center for Sustainability, Cornell University, Ithaca, NY, USA
Institute for Advanced Study, Technical University of Munich, Garching, Germany
Johannes Lehmann & Ingrid Kögel-Knabner
The Nature Conservancy, Arlington, VA, USA
Deborah A. Bossio
Chair of Soil Science, Technical University of Munich, Freising, Germany
Ingrid Kögel-Knabner
Institut für Biologie, Freie Universität Berlin, Berlin, Germany
Matthias C. Rillig
Berlin-Brandenburg Institute of Advanced Biodiversity Research (BBIB), Berlin, Germany
You can also search for this author in PubMed Google Scholar
Contributions
All authors contributed substantial discussion of content, edited the manuscript and its revisions.
Corresponding author
Correspondence to Johannes Lehmann .
Ethics declarations
Competing interests.
The authors declare no competing interests.
Additional information
Peer review information.
Nature Reviews Earth & Environment thanks Hailin Zhang, Nanthi Bolan and the other, anonymous, reviewer(s) for their contribution to the peer review of this work.
Publisher’s note
Springer Nature remains neutral with regard to jurisdictional claims in published maps and institutional affiliations.
Rights and permissions
Reprints and permissions
About this article
Cite this article.
Lehmann, J., Bossio, D.A., Kögel-Knabner, I. et al. The concept and future prospects of soil health. Nat Rev Earth Environ 1 , 544–553 (2020). https://doi.org/10.1038/s43017-020-0080-8
Download citation
Accepted : 06 July 2020
Published : 25 August 2020
Issue Date : October 2020
DOI : https://doi.org/10.1038/s43017-020-0080-8
Share this article
Anyone you share the following link with will be able to read this content:
Sorry, a shareable link is not currently available for this article.
Provided by the Springer Nature SharedIt content-sharing initiative
This article is cited by
Artificially regulated humification in creating humic-like biostimulators.
npj Clean Water (2024)
Patterns and determinants of microbial- and plant-derived carbon contributions to soil organic carbon in tea plantation chronosequence
- Xiaozhi Wang
Plant and Soil (2024)
An Assessment of Subjective Well-Being of Inter-Provincial Migrant Workers in Balochistan, Pakistan
International Journal of Community Well-Being (2024)
No farm is an island: constrained choice, landscape thinking, and ecological insect management among Wisconsin farmers
- Benjamin Iuliano
Agriculture and Human Values (2024)
Hydrochars as slow-release phosphorus fertilizers for enhancing corn and soybean growth in an agricultural soil
- Anahita Khosravi
- Yanfei Yuan
- Baoshan Xing
Carbon Research (2024)
Quick links
- Explore articles by subject
- Guide to authors
- Editorial policies
Sign up for the Nature Briefing: Microbiology newsletter — what matters in microbiology research, free to your inbox weekly.

- Open access
- Published: 07 December 2023
AI and machine learning for soil analysis: an assessment of sustainable agricultural practices
- Muhammad Awais 1 , 2 ,
- Syed Muhammad Zaigham Abbas Naqvi 1 , 2 ,
- Hao Zhang 1 , 2 ,
- Linze Li 1 , 2 ,
- Wei Zhang 1 , 2 ,
- Fuad A. Awwad 3 ,
- Emad A. A. Ismail 3 ,
- M. Ijaz Khan 4 , 5 ,
- Vijaya Raghavan 6 &
- Jiandong Hu 1 , 2
Bioresources and Bioprocessing volume 10 , Article number: 90 ( 2023 ) Cite this article
5785 Accesses
3 Citations
Metrics details
Sustainable agricultural practices help to manage and use natural resources efficiently. Due to global climate and geospatial land design, soil texture, soil–water content (SWC), and other parameters vary greatly; thus, real time, robust, and accurate soil analytical measurements are difficult to be developed. Conventional statistical analysis tools take longer to analyze and interpret data, which may have delayed a crucial decision. Therefore, this review paper is presented to develop the researcher’s insight toward robust, accurate, and quick soil analysis using artificial intelligence (AI), deep learning (DL), and machine learning (ML) platforms to attain robustness in SWC and soil texture analysis. Machine learning algorithms, such as random forests, support vector machines, and neural networks, can be employed to develop predictive models based on available soil data and auxiliary environmental variables. Geostatistical techniques, including kriging and co-kriging, help interpolate and extrapolate soil property values to unsampled locations, improving the spatial representation of the data set. The false positivity in SWC results and bugs in advanced detection techniques are also evaluated, which may lead to wrong agricultural practices. Moreover, the advantages of AI data processing over general statistical analysis for robust and noise-free results have also been discussed in light of smart irrigation technologies. Conclusively, the conventional statistical tools for SWCs and soil texture analysis are not enough to practice and manage ergonomic land management. The broader geospatial non-numeric data are more suitable for AI processing that may soon help soil scientists develop a global SWC database.
Graphical Abstract
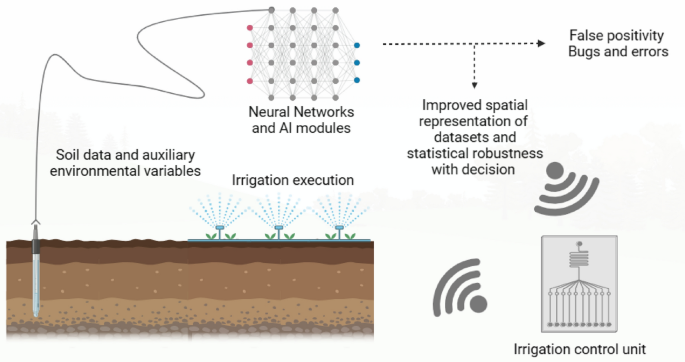
Introduction
Organisms need water as a compulsory part of routine metabolic activities, and especially plants require a continual supply of soil–water to maintain their turgor and transport mechanisms (Huang et al. 2020 ). The predominant allocation of water within plants is dedicated to the hydrolysis process, which serves as a means of generating energy required for the sustenance of a diverse array of chemical reactions and physiological processes (Bhunia et al. 2023 ). Soil–water contents (SWCs) are often confused with soil–moisture contents (SMCs), which in general are different. SWCs account for a ratio between the volume of water present in a unit of soil volume (Hueso et al. 2012 ). It has been discovered that the rate of mineralization significantly affects the microbial contents and activity, which helps to regulate plant growth (Ma et al. 2022 ). Moreover, the soil porosity and SWC saturation in designated soil pores may hinder O 2 diffusion, because the rate of O 2 diffusion is about one hundred times less when compared with air.
Hence, the ideal soil–water contents SWCs are limited in their ability to sustain crop growth as a result of the impeded diffusion caused by saturated soil pores (Zhang et al. 2021 ). Similarly, when the saturation of soil pores decreases from an optimal level, it results in significant damage to the microbial flora residing within these pores. Consequently, this leads to a decline in nitrogen and carbon mineralization (Schlüter et al. 2098 ). Furthermore, when drought is induced in soil pores, the water films surrounding soil particles become thinner, and dryness prevails in the soil, which primes water channels in the soil pores to become disconnected (Dwevedi et al. 2017 ).
The optimal SWCs are closely associated with carbon allocation, plant growth, nutrient recycling, photosynthetic rate, and microbial activity. The regulation of these parameters has also invariably been linked with the physicochemical properties of water that are held in soil (Wang et al. 2023 ). There is a fuzzy concept that all of the water present in soil can be taken up by plants. However, this is not a true concept; holding the water against the gravitational pull is a soil art that most often depends on the type of soil. Another indicator of the soil's overall ability to store water is its porosity. Measuring soil–water content and potential is the initial step in doing soil research, since they are important to state quantities of soil (Heiskanen 1997 ; Vereecken et al. 2015 ). Therefore, this water-holding capacity against the gravitational pull feeds the crop during water scarcity posed by low precipitation.
Though, due to complicated laboratory protocols and high cost, it remains a mere challenge to analyze available water contents for plant growth (Zhao et al. 2015 ). Soil–water holding capacity (SWHC) is mainly affected by soil texture, which is further dependent upon pH, temperature, microbial community, precipitation, type of soil, and other relatable factors. For example, an elevated environmental temperature will lead to the thawing of permafrost, which leads to many fluctuations in soil properties finally nutrient availability to plants is compromised (Månsson et al. 2014 ). Changes in crop rotation and land use can make the soil better in ways, such as C/N ratio, bulk density, tillage (Beheshti et al. 2012 ), and soil–organic carbon distribution is influenced by general topography (Sun et al. 2015 ). Moreover, carbon stocks are influenced by precipitation. There are very few studies available on the subject that encapsulate multifactorial factors influencing soil’s porosity and texture (Jamil et al. 2016 ). For instance, nutrient movement, pore size, and soil structure are influenced by the soil physical properties. Soil fertility is improved by particle surface absorption of ions in clay (Wang et al. 2022 ). Hence, the comprehensive examination of environmental factors pertaining to soil texture, porosity, and water content availability necessitates a laborious endeavor (Dragone et al. 2020 ).
The electrical conductivity (EC) and SWC measurements with minimal damage to soil have been anticipated by scientists for many years (Masha et al. 2021 ). While from the previous literature, it has been found that these factors are greatly influenced by soil porosity, which in turn is compromised by fluctuating environmental influencers (Bittelli 2011 ). Besides, the effects of environmental influencing variables are a time-demanding and complex task (Ruszczak and Boguszewska-Mańkowska 2022 ) that has not been reviewed and has also not been experimentally evaluated in a single manuscript (Heiskanen 1997 ).
Due to the diversified data available, it is very difficult to establish and study the effects of all influencing factors on soil parameters (Zhao et al. 2020 ). This creates a huge hurdle to getting a complete insight into the influencing parameter and making further decisions for intelligent agricultural practices manually (Pastén-Zapata et al. 2014 ). The accurate outcomes and decisions may be facilitated by considering the processing time and prerequisites of both conventional and advanced statistical techniques (Clauser et al. 2022 ). However, on the other hand, the critical time to perform a management response may have surpassed. For instance, the SWCs have been recorded on the landmark, and it is time to irrigate. While conventional statistics play a significant role in this irrigation decision-making process, the relative analysis is consistently and flawlessly present (Blanco and Lal 2023 ).
Artificial intelligence (AI) has been found to process non-numerical data, such as images, videos, text, and voice data with greater perfection. Therefore, there is a need to align the geophysical influence data on soil quality with artificially intelligent systems to process the decision-making more robustly (Liu et al. 2014 ). The AI does not even require the data to be large enough to process and suggest a crop management practice. The AI tools have previously been found to be smart enough to remove the noise from SWC, EC, and DC data for soil parameters. The noise removal was found to give an accurate SWC measurement that is actually available for the crop to be taken (Ratshiedana et al. 2023 ). The current study explains a review of soil–water content data and its possible processing using AI tools. The false positivity in SWC results and bugs in advanced detection techniques are also evaluated, which may lead to wrong agricultural practices. This review first covers the soil–water relationships and advancements in measurement techniques for SWC and soil texture. Second, the initial efforts for the development of global SWC and soil texture databases using AI networks have been discussed. Furthermore, the conventional statistical and AI analysis platforms have been compared, and conclusions are drawn for future recommendations.
The diverse SWCs inside the soil
The soil has the property of water anchorage, which may change with physicochemical texture and climate (Singh and Nair 2023 ). The water-holding capacity of soil can vary among different types of soil. However, the comprehensive depiction of soil–water is insufficient to elucidate the scientific principles governing water absorption by field crops (Adhikari et al. 2022 ). The soil–water can be attributed to different types, i.e., hygroscopic soil–water (HSW), gravitational soil–water (GSW), and capillary soil–water (CSW). These various varieties of soil–water are subject to distinct and variable forces that degrade the soil (Rayne and Aula 2020 ). The soil architecture is quite variable and is affected by various environmental factors that regulate the soil pore distribution; likewise, the soil–water distribution is affected (Jian et al. 2015 ). The HSW contents are held by soil–particle physical interactions in vapor form, which is more often hydrogen bonding. These contents are very unlikely to be strained by the crops for their growth due to strong soil binding (Wuddivira et al. 2012 ). After the precipitation, the GSW is rapidly increasing but is drained with more speed than any other type of soil–water due to its humongous gravitational pull. The gravitational forces drag GSW contents sharply to the larger pores deep down in the soil and often add to the water table (Fu et al. 2021 ). Due to shorter root lengths, the crops are also much less likely to use this GSW. As a general concept, GSW contents are temporarily available to the crops only before they are drained. Ideally, plants can easily access the soil–water when water contents are -33 bar; this only happens after all of the GSW drainage is completed and is termed field capacity (Leucci 2012 ). Water is usually considered the most important factor for crop growth, but actually, the soil–water content causes plants to wilt if all of the soil pores are filled with water (saturation). Total saturation hinders oxygen diffusion and halts the respiration of roots, which destroy the whole crop lot (Bhattarai et al. 2005 ). Therefore, the actual and readily available water contents utilized by crops are the CSW contents that make up the maximum field capacity. Moreover, when field capacity is not accessible by the plants due to the strong bonding of remaining water with soil, the permanent wilting point is achieved which is actually the point of no water uptake by the plants (Ben-Noah et al. 2021 ). At this point, the crop water uptake forces cannot overcome the available soil–water, which is often calculated at -15 bar. Hence, the water draw point for crops lies between field capacity and the permanent wilting point.
Soil and water interactions affect nutrient uptake
The water storage in soil has a direct relationship with the movement of water in soil pores, making water potential and SWCs relatable (Richards 2004 ). The soil texture and layering profiles also influence the water flow (Khaled and Fawy 2011 ). The manner in which soil–water interacts with soil particles has varying effects on the absorption of water and nutrients by crops. The disparity between soil and crop root water potential serves as a determining factor in facilitating the process of crop water uptake (Vico et al. 2023 ).
The instantaneous water concentration and force generated by water inside the crop roots are called crop root water potential. This is the key determinant for the direction of water movement in or out of the plant, because water movement is always explained as spontaneous from higher to lower potentials (Agegnehu et al. 2016 ). The pressure potential, turgor pressure, and solute potentials are also the denominators for crop root water potential (Boyer 2015 ). However, the real factor that governs water flow is the interaction of water molecules with soil (adhesion) and with each other (cohesion). Gravitational pull is another key factor that restricts uphill water flow (Miranda-Apodaca et al. 2018 ).
In general, water molecules are associated with one another, and plants are only able to uptake soil–water when they overcome the adhesion and gravitational pull. Therefore, the water uptake by the plants, even at more feasible bar pressure is difficult with varying soil textures and porosity. The water movement from soil to plant roots is justified by the suction phenomena elucidated by TACT theory (transpiration, adhesion, cohesion, and tension). The present theory elucidates the mechanism underlying water transport within the xylem and the generation of negative water potential within plant roots, resulting in the development of suction forces that facilitate the uptake of water into the plant roots (Lambers et al. 2019 ). The supporters of this theory are of the view that water suction is only possible when the TACT forces overcome the gravitational pull and other physical interactions established by water. The schematic of TACT and water–soil interaction is explained in Fig. 1 .
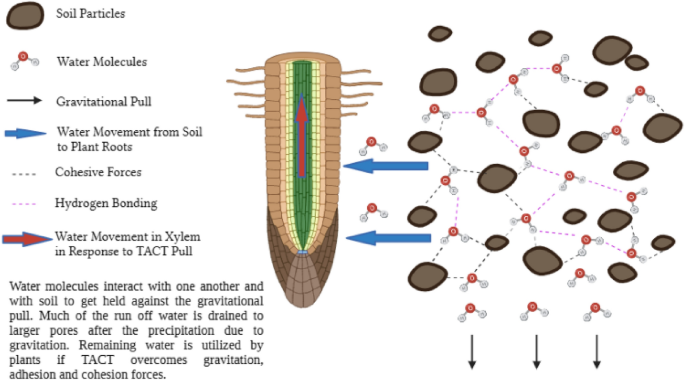
Schematics of water–water and water–soil interaction affecting the movement and availability of SWCs to plant roots (Drawn using Biorender.com)
The disparities in nutrient composition between soil and crop roots facilitate the process by which crops absorb nutrients. The plant nutrient concentration refers to the quantity of nutrients found in the sap of a plant, which is measured as a ratio of mass or molarity per unit volume (Chen et al. 2022 ). Plant nutrient concentration varies with plant species, growth stage, and environmental conditions. Crop uptake of water and nutrients also depends on the characteristics of the root system, such as root branching pattern, root surface area, root diameter, root length, root hair density, root depth distribution, etc. (Dotaniya and Meena 2015 ). The characteristics of the root system affect the contact area between roots and soil particles as well as the transport capacity of roots (Pregitzer and King 2005 ).
Crops need adequate amounts of both water and nutrients for optimal growth and yield. The interaction of soil–water with soil particles depends on various factors, such as soil texture, structure, organic matter, pH, cation exchange capacity, fertilizer application, climate, crop species, growth stage, environmental conditions, root system characteristics, etc. Therefore, understanding these factors and their effects on soil–water–plant relationships is important for managing soil fertility and irrigation practices effectively (Fu et al. 2019 ).
The relative proportions of clay, silt, and sand are expressed as soil texture (Barman and Choudhury 2020 ). The texture of soils is considered to influence nutrient availability either by changing the water holding capacity or by manipulating the cation exchange capacity (Sharma et al. 2015 ). The water retained by soil for plant usage is subjected to water holding capacity, as this is the most crucial feature that supports nutrient uptake and transport mechanisms in plants. In general, higher water-holding capacity is a feature of finer-textured soils (clayey soils) than coarser textured soils (sandy soils), because they have 8–10 times more total pore space and smaller pores that hold water more tightly (Li et al. 2014 ). This means that clayey soils can provide more water and nutrients to plants than sandy soils, especially during drought periods. However, clayey soils can also become waterlogged or anaerobic if drainage is poor, which can limit nutrient availability and plant growth (Wang et al. 2021a ).
The concentration of soil nutrients exhibits variability based on factors, such as soil texture, structure, organic matter content, pH levels, cation exchange capacity, and the application of fertilizers (Bouajila et al. 2023 ). In general, finer-textured soils (finer than 1 mm) have higher soil nutrient concentrations than coarser-textured soils, because they have a larger surface area and a more negative charge on their surfaces that can adsorb cations (positively charged nutrients). However, finer-textured soils can also bind some nutrients too strongly or make them unavailable by forming insoluble compounds with other elements.
Cation exchange capacity (CEC) reflects the availability of cationic nutrients present in soil–water, such as ammonium, calcium, potassium, magnesium, iron, zinc, etc. Cover crops in seed maize or soybean treatment (SCCC) had a significant effect on soil exchangeable K in the topsoil (0–5 cm soil layer) (Emamgolizadeh et al. 2015 ). These positively charged ions are found in close interaction with negatively charged organic soil constituents. The positively charged ions are absorbed by the plant root using an anti-port cation exchange mechanism (Ulusoy et al. 2016 ). In general, finer-textured soils have a higher CEC than coarser-textured soils, because they have a larger surface area and more negative charge on their surfaces. This means that clayey soils can store more cations and prevent their leaching than sandy soils. However, clayey soils can also bind some cations too strongly or make them unavailable by forming insoluble compounds with other elements, such as phosphorus.
Soil texture also affects the mobility and retention of negatively charged nutrients (anions), such as nitrate, phosphate, sulfate, etc. Previously, 10% clay soil addition was nearly as effective in reducing N and P leaching as 20% clay soil. Adding only 10% clay soil to a sandy soil is likely to be less expensive than 20%. (Yan et al. 2022 ). Anions are not held by the soil particles but move freely with the soil–water. In general, coarser-textured soils have higher anion leaching potential than finer-textured soils, because they have larger pores that allow more water flow. This means that sandy soils can lose more anions by leaching than clayey soils, especially under high rainfall or irrigation conditions (Ali et al. 2020 ). However, sandy soils can also allow more anion uptake by plant roots than clayey soils, because they have lower anion adsorption potential. Convincingly, soil texture affects nutrient availability by influencing the water-holding capacity and the cation exchange capacity of the soil. Finer-textured soils tend to have higher nutrient retention and lower nutrient leaching than coarser-textured soils, but they may also have lower nutrient availability and aeration under certain conditions (Liu et al. 2021 ). Therefore, soil texture needs to be considered when managing soil fertility and applying fertilizers.
SWCs in different soil types
The predominant land textures in arable areas primarily depend on precipitation to sustain SWCs, which is crucial for supporting arid vegetation and facilitating the ecohydrological cycle (Xu et al. 2023 ). The SWCs in arid and semi-arid regions exhibit increased heterogeneity in response to variations in precipitation patterns and vegetation types (Obade and Gaya 2021 ). Due to the exhaustive evaporation factor, the smaller precipitation index has insignificant effects on SWC (Wilson et al. 2004 ). SWC is reported to decrease with increasing soil depth due to lessening influence of precipitation factors on deep soils. Such as, the coefficient of variation was found to be high for SWC in the horizontal direction (48%), but was relatively small for SWC in the vertical direction (9%) (Zhao et al. 2017 ). Therefore, it can be established that precipitation and SWCs are strongly associated with local climate (Mei et al. 2019 ). Diverse microbial communities coexist in various soil types to maintain their textural heterogeneity at microscale (Huang et al. 2023 ). Soil textures mainly hinge on soil heterogeneity, which has a direct linkage to soil pores distribution (Rooney et al. 2022 ). The differential pore distribution affects the SWC and pore saturation at large; therefore, soil–microbial flora can also influence the SWC measurement techniques that involve EM waves. The development of more microbial communities in soil pores often uplifts the water density (Amarasekare 2003 ) and also adds to the relative water volume, which later on results in false positivity for volumetric SWC observations (Vos et al. 2013 ). Scientists believe that this microbial flora is involved in the natural biogeochemical cycles and offers colonization resistance to the soil (Stein et al. 2014 ).
The water matrix suction by the crops has been a recent topic of research in environmental science and agriculture engineering (Xu and Yang 2018 ; Rahardjo et al. 2019 ; Tian et al. 2020 ). Measurement of SWCs in specific soil types is limited to detection techniques whether recent or advanced (Wang et al. 2021 ; Karakan 2022 ; Ojeda Olivares et al. 2020 ). These studies have presented the idea of hydraulic retention and SWC strength using quantitative analytical techniques. Soil texture (Liu et al. 2012 ) and pore conformations (Chen et al. 2017 ) have been found to pose a significant influence on total SWCs. Moreover, the initial soil wetting has also been investigated to impact the water content at large (Zhang et al. 2021 ). However, the rapid draining of water due to gravitational pull generates a sufficient number of atrocities in data collection and further analytical processing.
In another study, it was found that out of 120 samples, the water contents of more fine clayey soils were significantly higher compared with those of more sandy soils (Li et al. 2016 ). Nonetheless, the finer and clayey soil holds water sufficiently well and halts water mobility for crops even at ideal water bar of -30 units. Furthermore, when the soils are tuned to be finer, the porosity may increase, but the crop efficacy to drag water from these pores is significantly reduced (Fig. 2 ). Moreover, the suction matrix fractal analysis model for VSWC in various soil types confirms the significantly differential VSWCs (Fig. 3 ).
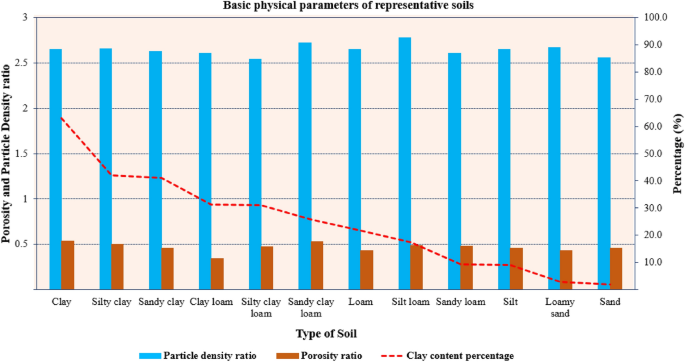
Physical parameters of differently textured soils in relation to water contents (Redrawn from data source) open access license (Obade and Gaya 2021 )
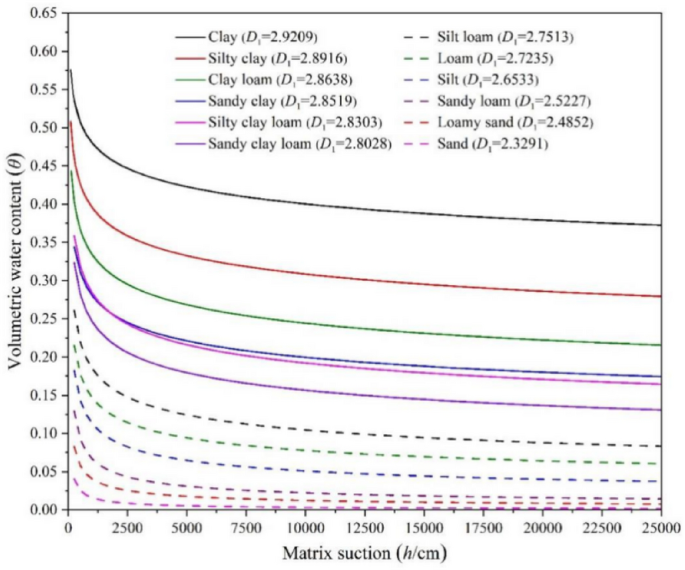
Results of fractal model showing the association between VSWC and matrix suction from diverse soil textures (Reprint from open access license) (Obade and Gaya 2021 )
In general, sandy soils have higher hydraulic conductivity than clayey soils, because they have larger pores that offer less resistance to water flow (Hao et al. 2019 ). This means that sandy soils can drain faster than clayey soils after rainfall or irrigation. However, this also means that sandy soils can lose more water by evaporation or transpiration than clayey soils, because they have a lower matric potential and cannot retain water against atmospheric demand. Because clayey soils have smaller pores that give more barrier to water movement, they have poorer hydraulic conductivity than sandy soils. This means that clayey soils can hold more water after rainfall or irrigation than sandy soils. However, because clayey soils have a larger matric potential, which prevents gravitational drainage, they can become waterlogged or anaerobic if drainage is poor (Mulla et al. 2023 ). SWCs vary with soil texture due to differences in pore space and pore size distribution. Clayey soils have a higher SWC and lower hydraulic conductivity than sandy soils under all moisture conditions. This affects the water holding capacity and water movement in the soil, which in turn affect various processes, such as plant growth, nutrient cycling, water balance, and soil erosion. Therefore, understanding the relationship between SWC and soil texture is important for managing soil and water resources effectively.
Advancements in SWCs measurements
Soil–water content (SWC) is a crucial parameter that affects various biophysical processes, such as plant growth, nutrient cycling, and water balance (Pereira et al. 2020 ). Measuring SWC accurately and efficiently is important for many applications in ecology, agriculture, hydrology, and engineering. The SWC is often termed wetness of soil, which in general has no specified but relative value (Lal and Shukla 2004 ). This relative value is often a ratio, often calculated as water over soil volume (V w /V S ) and volumetric SWC (VSWC), is usually represented as θ (García-Gamero et al. 2022 ). Moreover, as a general convention, this VSWC is often referred to as relative soil volume filled with water. Being a basic quantity for soil research initiation, SWC measurement is a routine analysis for soil examination. There are diverse methods that have been reported to measure SWC and have their own pros and cons. One of the basic, or so-called absolute, methods of measuring SWC is the gravimetric method (GM), which is noncalibrated and the most basic method.
The GM is a destructive approach that often fails to provide real-time knowledge and cannot measure the same sample area again (Villalobos et al. 2008 ). This method may also leave a space from where the sample has been taken, which will abruptly change the SWC, bulk density, and relative volume of nearby areas. These factors might leave the GM method unreliable and nonrepresentative if we are more involved in the real-time measurement of SWC. The neutron method (NM) was then developed for more likely real-time and non-destructive SWC measurements. This method accounts for only elastic collisions between neutrons and water molecules. Consequently, the presence of tightly bound hygroscopic water molecules and neutron dissipation can lead to the acquisition of false-positive outcomes.
The determination of the actual water content accessible for crops may continue to lack definitive findings (Drizo et al. 2022 ). Due to high radiation levels, this method also remains banned in most countries. This method is non-destructive, continuous, and capable of measuring SWC at different depths and large volumes of soil. However, it is expensive, hazardous, and requires a license to operate. It also requires calibration with other methods and correction for soil bulk density and temperature. With the research and development of SWC measurement technology, more accurate and noninvasive methods were also introduced, which require little calibration and are more sensitive. These methods either rely on electrical or electromagnetic (EM) signal travel in the soil and water, and then the resistance, capacitance, frequency, or time of travel can be compared as a measure of SWC. These methods are more robust and require prior installation and calibration (Karimi, et al. 2020 ). However, once installed, real-time and noise-free results can be obtained.
It remains a key factor that electromagnetic wave propagation is inhibited by air gaps, so the techniques utilizing EM waves are limited to certain soil types. For instance, the ground penetration radar (GPR) method involves EM wave propagation in soil and then a reflection of these waves by soil entities. It uses a transmitter and a receiver that are moved along the soil surface or placed in boreholes and measure the travel time, amplitude, frequency, or envelope of the reflected waves (Pandya 2021 ). This method is non-destructive, high-resolution, and capable of measuring SWC at different depths and large areas of soil. However, it is complex, expensive, and affected by soil texture, structure, salinity, and surface roughness. It also requires inversion models and calibration with other methods (Sharma and Sen 2022 ). The comparative analysis of the techniques discussed is also summarized in Table 1 .
Conclusively, there are various techniques available for measuring SWC at different scales and for different purposes. Each technique has its own strengths and weaknesses that need to be considered when choosing the most suitable one for a given situation. There is no single best technique that can measure SWC universally and accurately. Therefore, it is often necessary to combine or compare different techniques to obtain reliable and representative estimates of SWC. Future development of SWC measurement techniques may focus on improving their accuracy, precision, resolution, cost-effectiveness, ease of use, and integration with other sensors, models, and programs developed by AI.
Soil texture prediction and analysis using artificial intelligence
Soil texture has been found to play a crucial role in ecosystem health, agricultural production, and sustainable farmland management (Zhai et al. 2006 ). Among the diverse soil properties, the texture plays a pivotal role in decision-making for the planning and management of agricultural land. The conventional approaches with agriculture sensors and statistical analysis were found to be non-robust, time-consuming, non-instantaneous, and expensive (Bormann 2010 ). However, with advanced AI processing tools and ML applications, new avenues for texture prediction and revolutionized soil management practices have been opened.
Conventional soil texture analysis is performed by sieving, sedimentation, and other hydrometric laboratory methods. Later, the results from these experiments are statistically analyzed, and conclusions are drawn manually. The complexity of this analysis can be presumed from the variable soil textures and environmental attributes that affect it. This creates heaps of data that cannot be translated into a single conclusion for correct decision-making (Riese and Keller 2019 ). Therefore, all of these manual dealings require skilled professionals, a significant amount of time, and specialized instruments. However, AI tools are a promising set of alternatives for these limitations that otherwise confine soil management.
AI techniques that include machine learning (ML) and deep learning (DL) are potentially remarkable for accurate and efficient soil texture predictions. The inputs utilized by these algorithms are compositional, spectral, and geographical data sets that can be in non-numerical form (Johnson et al. 2020 ). AI processing of these data sets mainly reduces the cost, time, and labor involved compared with conventional laboratory protocols. The complexity of relationships among the data sets is quickly learned and applied using the ML and DL algorithms (Wang et al. 2021b ). This is not the only scale available with this technology; cloud systems and mobile applications are another step forward. The wider scalability of AI enables farmers and land managers to access and process land management operations with ease.
The subjective and error-prone data analysis from traditionally practiced laboratory protocols is then dazzled by the objective, more accurate, and real-time data processing using AI tools (Hassan-Esfahani et al. 2015 ). By leveraging AI techniques, we can overcome the limitations of traditional laboratory-based methods and enable real-time decision-making in soil management practices. However, addressing challenges related to data quality, interpretability, and system integration will be crucial for the successful implementation of AI-based soil texture analysis (Liu et al. 2022 ). With continued research, collaboration, and innovation, AI-driven soil analysis can contribute significantly to sustainable land management, agricultural productivity, and environmental conservation. Integrating AI models seamlessly into existing soil management practices and decision support systems requires collaboration among scientists, engineers, and policymakers. This integration would ensure the practical implementation of AI-based soil texture analysis on a broader scale.
Artificial intelligence and SWC measurement
The highest cadre of the information technology revolution is AI, which has influenced and reshaped every field of life. AI has a robust approach, where the computers learn from already existing data sets and get themselves trained enough to solve complex problems. This AI resides on very complex modules that are cladistical in their linkage and are very complex to understand (Barros et al. 2022 ). AI is generally compared with human neurons for its signal-processing complexity. The inputs, processing, decision-making, and outputs are similar to those of human neurological systems (Fig. 4 ).
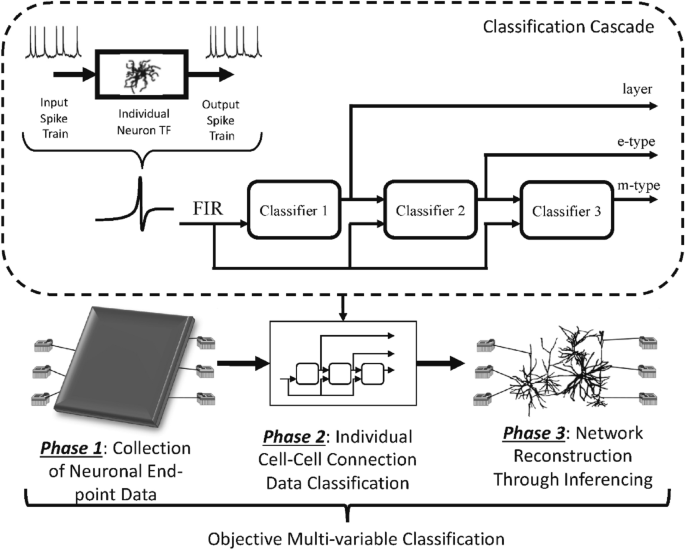
Comparison of artificial and biological neuron (Barros et al. 2022 ) (Open Access)
A variety of fields have benefited from AI, for instance, robotics, medical imaging, disease detection, and flight control systems. AI has also been found to be capable of solving key issues in agronomy, meteorology, and hydrology. The science of SWC measurement has also benefited from AI source codes recently. It has presented itself as a water- and soil-state manager with high performance, correlation, and statistical correctness (Gao et al. 2022 ). This is a very smart add-on to robust agricultural decision-making under the influence of various environmental factors. This section of the manuscript will review AI strategies for agriculture biosensing, with a special focus on SWC management.
Climate variability can readily impact the outcomes of the FDR and TDR techniques for measuring SWC. This has inspired scientists to report a novel method in 2020 (Mallet et al. 2020 ), which included measuring the temperature response and heating the surrounding soil in short bursts. Due to its high degree of automation and being less influenced by climate, this actively heated optical fiber (AHOF) method can be applied, where conventional TDR and FDR might not be enough (Ciocca et al. 2012 ). However, the analysis performed by AHOF still requires correction due to unpredicted errors, types of soil, and other climatic variables. Therefore, the artificial neural network (ANN) utilized for removing the errors generated by the AHOF method to improve the effectiveness of acquired results (Liu et al. 2023 ). The prescribed model was recommended for usage, which contained the use of a climate layer or cover layer that was found to be highly correlated with SWC contents.
The field of agriculture sensing has now been closely linked with ANN models to rectify and filter the most accurate results and forecast the future for irrigation and agricultural management. Precision agriculture has now been supplemented with pH, humidity, SWC, mechanical, and airflow sensors that provide enough results for robust decision-making using ANN tools. Considerable efforts have been made to forecast the future of wheat crops using ANN model training based on previous sensor readings (Roshan et al. 2022 ). The scientists then concluded multilayered perceptron model was the most effective, with lower MSE and RMSE when compared with other ANN models. Accurate assessment of SWCs has grabbed the attention of researchers over the past few years.
Many efforts have been made to contribute to in-situ data collection and the development of remote databases for SWCs (Owe et al. 2008 ). However, these efforts are still in progress, and to date, we do not have such a database on a global scale. SWC measurement using pseudo-transfer functions (PTFs) is a solution to maximize the right soil data acquisition from lands, where there are no data available. Regression model analysis usually generates PTF data that can be fed to an adaptive neuro-fuzzy interference system (ANFIS) that gives a non-explicit insight into SWC variables (Liu et al. 2020 ). The research found the ANFIS model to be more efficient, robust, and quick compared to conventional statistical models that have higher standard errors (Hosseini et al. 2021 ). Understanding soil texture and water content is key to proper crop management. Precipitation and droughts considerably account for more than anything else that can affect soil textures (Keller and Håkansson 2010 ).
The main components of a Bragg grating AH–FBG moisture sensor are a resistance wire, an optical fiber with quasi-distributed FBGs for temperature monitoring, and an enclosed tube. The capacity of an FBG to reflect light waves with a specific center wavelength is affected by temperature and strain. The AH–FBG sensor's center wavelength shift is unaffected by strain, because a corundum tube encircles it, taking the strain out of the equation. If the sensor is placed in the ground, the soil temperature at the relevant measurement point may be calculated by examining the FBG reflection spectrum (Fig. 5 ). This superior technology, however, is strictly confined to certain situations. For example, when heated, the AH–FBG sensor may be seen as an endless linear heat source. Furthermore, the soil being tested is expected to be homogenous and isotropic (Liu et al. 2023 ).
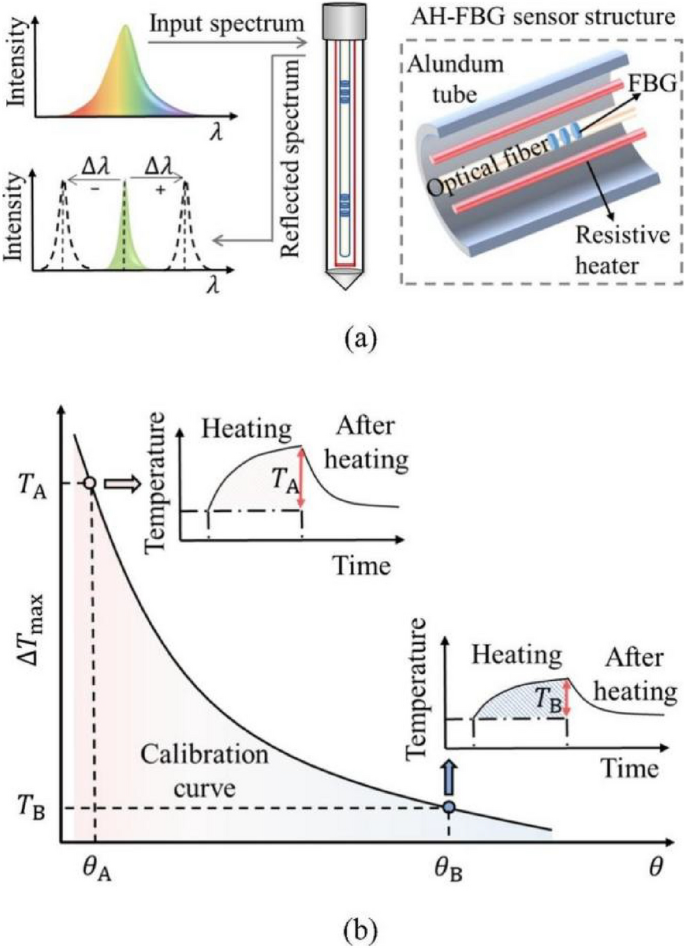
Artificial intelligence-based fiber optic sensing for soil moisture measurement (Liu et al. 2023 ) ( a ) an optical fiber with quasi-distributed FBG for temperature measurement ( b ) example of result plot from the fiber with quasi-distributed FBG
Role of AI in smart agricultural irrigation systems
AI has enabled computer science to create artificial systems that work similar to the human brain. These artificial systems or machines can help humans perform more precise learning, logical reasoning, problem-solving, and decision-making. Due to its enormous potential, AI has been integrated into many research fields, such as agriculture, where it can help farmers optimize their use of water, land, and other resources, increase their productivity and profitability, and reduce their environmental impact (Kose et al. 2022 ). Agriculture faces the key challenge of water scarcity in regions with low precipitation and where droughts are prevalent.
The Food and Agriculture Organization (FAO) states that agriculture accounts for about 70% of global freshwater withdrawals, and it can go up to 15% by 2050 to meet the mounting demand for food by a growing population (Pernet and Ribi Forclaz 2019 ). However, water availability is unevenly distributed across the world, and climate change is expected to exacerbate the variability and impulsiveness of rainfall patterns, distressing crop yields and quality. To address this challenge, many researchers and practitioners have proposed and implemented smart irrigation systems that use sensors, controllers, actuators, communication networks, and data analysis tools to monitor and control the delivery of water to crops according to their needs and environmental conditions (Jong et al. 2021 ).
These systems aim to improve water use efficiency, reduce water waste and runoff, enhance crop growth and quality, and save energy and labor costs (Penghui et al. 2020 ). However, smart irrigation systems face some limitations, such as high maintenance cost, a lack of interoperability and standardization among different devices and platforms, the complexity of data processing and interpretation, and the uncertainty and variability of crop responses to irrigation. Moreover, traditional irrigation scheduling methods based on fixed rules or thresholds may not be intelligent enough to detach the dynamic and nonlinear interactions between soil, crops, water, management practices, and weather (Kouadio et al. 2018 ). Therefore, AI is considered to play a significant role in enhancing of functionality and performance of smart irrigation systems. Agriculture, when factionalized with AI systems, can help farmers identify instantaneous and accurate measurements as well as decisions for fertilization, irrigation, SWC, pH, and related factors (Raja and Shukla 2021 ). AI can also help farmers optimize their irrigation schedules and distribution using machine learning algorithms that can learn from historical and current data, forecast future scenarios, and adapt to changing conditions.
There are a variety of insights available, where AI can help smart irrigation systems obtaining optimal results. Fuzzy logic is one of the examples that deals with imprecise and uncertain information using linguistic variables and rules instead of numerical values. Fuzzy logic can be used to model complex systems, such as SWC, soil–total moisture, nutrient dynamics, or crop water requirements by incorporating expert knowledge and human intuition (Mahmoudi et al. 2022 ). Second, the Internet of Things (IoT) is a network of interconnected devices that can collect, transmit, process, and act on data without human intervention. IoT can be used to implement smart irrigation systems by integrating sensors, controllers, actuators, communication modules, cloud computing services, and mobile applications (Kodali and Sahu 2016 ).
Finally, ML is another smart advancement that is empowered by ANNs, DL, and reinforcement learning (RL). These attributes of ML have the advantage of processing soil imagery to extract information about soil textures, SWCs, nutrition, and other parameters (Pham et al. 2018 ). Therefore, utilizing these resources efficiently over conventional statistical approaches and vigorous data analysis can be the new era approach for smarter agriculture practices, especially for soil management. In the last couple of years, numerous researchers and engineers worked on different types of materials subject to biological sciences, agricultural sciences and environments.
Statistical and AI tools comparison
The statistical approaches have served the science from its beginning and have presented enormous ways of data analysis for further decision-making and drawing conclusions (Phoon et al. 2010a ). Most mathematical or statistical approaches involve numerical data processing, stigmatization, and drawing data correlations. Rather, the AI tools rely on computational modules to carry out such challenging tasks that otherwise require human intelligence. Drawing the line between statistical analysis and AI processing is difficult due to the reliability of both in various conditions (Henderson et al. 1992 ). However, AI processing for the same subject of experiments can be more advantageous, robust, and decisive compared to statistics. Statistical analysis usually requires structured and large numerical data inputs, such as SWCs, soil properties, weather data, etc. (Phoon et al. 2010b ). However, on the other hand, AI tools can efficiently operate with non-structured and qualitative data, such as images, videos, texts, voice data, etc. (Yu and Kumbier 2018 ). In addition, the AI tools are smart enough to handle data augmentation, regularization, and ensemble learning of small and noisy data sets (Friedrich et al. 2022 ). More specifically, ML tools are data-driven models and novel algorithms for training, processing, and making predictions for given data sets that are not addressed while using predefined statistical models. The interpretation and visualization of data have been simplified for human interface in AI tools, but the statistical approach is not robust and time-consuming for this purpose. Statistical tools can be used for descriptive and inferential purposes, such as describing the distribution of soil–water content, identifying the factors that affect soil–water content, testing the differences or relationships among soil–water content variables (Wadoux et al. 2020 ). On the other side, AI tools can be used for predictive and prescriptive purposes, such as predicting SWCs based on various inputs, optimizing the irrigation schedule based on SWC goals, recommending the best management practices, etc. Statistical and AI tools are known to have different strengths and limitations for SWC analysis. Depending on the research question and objective, one may choose to use either or both of them to obtain comprehensive and accurate insights from the data. The link between statistical and AI tools is an advanced key to opening the doors for robust management and agricultural biosensing (Table 2 ).
Development of a global dataset for soil texture and SWC data
Many ecological processes, water availability, and agricultural productivity depend on the type and strength of agricultural land. Soil texture and SWC are the more crucial parameters to determine effective land usage, sustainable agriculture, and water administration (Maino et al. 2022 ). However, due to the vast earth’s surface and spatial soil heterogeneity, it is very difficult to obtain comprehensive and reliable soil information (Martinelli and Gasser 2022 ). The global data set of SWC and soil texture information has the potential to enable farmers, policymakers, and land managers to timely engage in smart agricultural practices. Such data sets can also help in crucial hydrological analysis and climate modeling.
Soil–water dynamics, climate change, flood prediction, and water movement modeling can be reshaped after the development of these global data sets. Environmental conservation is another crucial concern that can be smartly managed after developing global data sets for soil parameters (Zhang and Shi 2019 ). This will help in the assessment of soil erosion, fertility, and ecological health. Moreover, areas with vulnerable soils can be identified more accurately, and targeted conservation and land restoration efforts can be practiced more efficiently. Considerable efforts have been made in past toward the development of a global soil parameter database. These efforts involve everything from general laboratory analysis of soil from various localities to the installation of advanced sensors globally or geospatial technologies (Mallah et al. 2022 ).
Remote sensing technologies, such as satellite imagery and airborne sensors, can provide valuable information on soil properties indirectly. Spectral signatures obtained from these sensors can be correlated with soil texture and soil–water content data collected from ground-based measurements. Proximal sensing techniques, such as electromagnetic induction and ground-penetrating radar, also contribute to the acquisition of soil data on a larger scale. Machine learning algorithms, such as random forests, support vector machines, and neural networks, can be employed to develop predictive models based on available soil data and auxiliary environmental variables. Geostatistical techniques, including kriging and co-kriging, help interpolate and extrapolate soil property values to unsampled locations, improving the spatial representation of the dataset (Naimi et al. 2022 ).
Ensuring an adequate distribution of soil samples across different regions, soil types, and land cover categories is essential for capturing the spatial heterogeneity of soils globally. Sampling biases and limited access to certain regions can pose challenges in achieving a representative data set. Soil data collected using different protocols, laboratory methods, and instruments needs to be standardized and harmonized to ensure consistency and compatibility. Developing robust quality control procedures and data harmonization protocols is necessary to integrate diverse data sets into a coherent global database. Encouraging data sharing among researchers, institutions, and national soil agencies is crucial for developing a comprehensive global data set. Collaboration at regional and international levels can help overcome data gaps and promote data exchange, leading to a completer and more reliable dataset.
The need for robust, quick, and accurate soil analysis using AI technology holds a great and promising future for sustainable agricultural practices and efficient natural resource management (Pandey et al. 2023 ). The nonuniform geospatial distribution of SWCs and soil textures is a big impediment to the development of a global soil database. Even advanced statistical data processing is time-consuming and has delayed decision-making to practice intelligent agriculture. However, by leveraging AI, DL, and ML techniques, researchers can overcome these challenges and obtain more efficient and reliable soil analysis results. Artificial neural networks (ANNs) and other related AI modules have shown promising results in achieving robustness in SWC and soil texture analysis. These techniques allow for the processing of non-numeric geospatial data, providing valuable insights for soil scientists and aiding in the development of a global SWC database.
Machine learning algorithms, such as random forests, support vector machines, and neural networks, can be employed to develop predictive models based on available soil data and auxiliary environmental variables. In addition, geostatistical techniques such as kriging and co-kriging play a significant role in interpolating and extrapolating soil property values, improving the spatial representation of the data set. However, challenges such as false positivity in SWC results and bugs in advanced detection techniques need to be addressed to ensure accurate and reliable soil analysis. Further research and development are required to refine AI models and improve their performance in soil analysis applications. It is evident that conventional statistical tools alone are insufficient for robust SWC and soil texture analysis. The integration of AI and related technologies provides a promising pathway to enhance soil analysis efficiency, enable intelligent decision-making, and facilitate sustainable land management practices. By harnessing the power of AI, researchers can make significant strides in understanding soil–water relationships, improving agricultural productivity, and developing a comprehensive global SWC database to support sustainable agriculture and resource management.
Availability of data and materials
Data sharing not applicable to this article as no data sets were generated or analyzed during the current study.
Adhikari S, Timms W, Mahmud MAP (2022) Optimising water holding capacity and hydrophobicity of biochar for soil amendment—a review. Sci Total Environ 851:158043
Article CAS PubMed Google Scholar
Agegnehu G, Nelson PN, Bird MI (2016) Crop yield, plant nutrient uptake and soil physicochemical properties under organic soil amendments and nitrogen fertilization on Nitisols. Soil Tillage Res 160:1–13
Article Google Scholar
Ali N, Bilal M, Khan A, Ali F, Iqbal HMN (2020) Effective exploitation of anionic, nonionic, and nanoparticle-stabilized surfactant foams for petroleum hydrocarbon contaminated soil remediation. Sci Total Environ 704:135391
Amarasekare P (2003) Competitive coexistence in spatially structured environments: a synthesis. Ecol Lett 6(12):1109–1122. https://doi.org/10.1046/j.1461-0248.2003.00530.x
Barman U, Choudhury RD (2020) Soil texture classification using multi class support vector machine. Inform Process Agric 7(2):318–332
Google Scholar
Barros MT, Siljak H, Mullen P, Papadias C, Hyttinen J, Marchetti N (2022) Objective supervised machine learning-based classification and inference of biological neuronal networks. Molecules. https://doi.org/10.3390/molecules27196256
Article PubMed PubMed Central Google Scholar
Beheshti A, Raiesi F, Golchin A (2012) Soil properties, C fractions and their dynamics in land use conversion from native forests to croplands in northern Iran. Agric Ecosyst Environ 148:121–133
Ben-Noah I, Nitsan I, Cohen B, Kaplan G, Friedman SP (2021) Soil aeration using air injection in a citrus orchard with shallow groundwater. Agric Water Manag 245:106664
Bhattarai SP, Su N, Midmore DJ (2005) Oxygation unlocks yield potentials of crops in oxygen-limited soil environments in advances in agronomy. Academic Press, Cambridge, pp 313–377
Bhunia RK, Sinha K, Kaur R, Kaur S, Chawla K (2023) A holistic view of the genetic factors involved in triggering hydrolytic and oxidative rancidity of rice bran lipids. Food Rev Int 39(1):441–466
Article CAS Google Scholar
Bittelli M (2011) Measuring soil water content: a review. HortTechnology 21(3):293–300
Blanco H, Lal R (2023) Soil fertility management. In: Blanco H, Lal R (eds) Soil conservation and management. Cham, Springer Nature Switzerland, pp 363–390
Chapter Google Scholar
Bormann H (2010) Towards a hydrologically motivated soil texture classification. Geoderma 157(3):142–153
Bouajila K, Hechmi S, Mechri M, Jeddi FB, Jedidi N (2023) Short-term effects of Sulla residues and farmyard manure amendments on soil properties: cation exchange capacity (CEC), base cations (BC), and percentage base saturation (PBS). Arab J Geosci 16(7):410
Boyer JS (2015) Turgor and the transport of CO2 and water across the cuticle (epidermis) of leaves. J Exp Bot 66(9):2625–2633
Article CAS PubMed PubMed Central Google Scholar
Bünemann EK et al (2018) Soil quality—a critical review. Soil Biol Biochem 120:105–125
Chen P, Liu J, Wei C, Xue W, Tian H (2017) Approach to rapidly determining the water retention curves for fine-grained soils in capillary regime based on the NMR technique. J Eng Mech 143(7):04017032
Chen X et al (2022) Root physiological adaptations that enhance the grain yield and nutrient use efficiency of maize (Zea mays L) and their dependency on phosphorus placement depth. Field Crops Res 276:108378
Ciocca F, Lunati I, Van de Giesen N, Parlange MB (2012) Heated optical fiber for distributed soil-moisture measurements: a lysimeter experiment. Vadose Zone J. https://doi.org/10.2136/vzj2011.0199
Clauser NM, Felissia FE, Area MC, Vallejos ME (2022) Integrating the new age of bioeconomy and industry 4.0 into biorefinery process design. BioResources 17(3):5510
de Obade VP, Gaya C (2021) Digital technology dilemma: on unlocking the soil quality index conundrum. Bioresour Bioprocess 8(1):6
Dotaniya ML, Meena VD (2015) Rhizosphere effect on nutrient availability in soil and its uptake by plants: a review. Proc Natl Acad Sci India Sect B Biol Sci 85(1):1–12
Dragone G, Kerssemakers AAJ, Driessen JLSP, Yamakawa CK, Brumano LP, Mussatto SI (2020) Innovation and strategic orientations for the development of advanced biorefineries. Bioresour Technol 302:122847
Drizo A, Johnston C, Guðmundsson J (2022) An inventory of good management practices for nutrient reduction, recycling and recovery from agricultural runoff in Europe’s Northern Periphery and Arctic Region. Water. https://doi.org/10.3390/w14132132
Dwevedi A, Kumar P, Kumar P, Kumar Y, Sharma YK, Kayastha AM (2017) 15—Soil sensors: detailed insight into research updates, significance, and future prospects. In: Grumezescu AM (ed) New pesticides and soil sensors. Academic Press, Cambridge, pp 561–594
Emamgolizadeh S, Bateni SM, Shahsavani D, Ashrafi T, Ghorbani H (2015) Estimation of soil cation exchange capacity using Genetic Expression Programming (GEP) and Multivariate Adaptive Regression Splines (MARS). J Hydrol 529:1590–1600
Friedrich S et al (2022) Is there a role for statistics in artificial intelligence. Adv Data Anal Classif 16(4):823–846
Fu Z-D et al (2019) Effects of maize-soybean relay intercropping on crop nutrient uptake and soil bacterial community. J Integr Agric 18(9):2006–2018
Fu Y, Horton R, Heitman J (2021) Estimation of soil water retention curves from soil bulk electrical conductivity and water content measurements. Soil Tillage Res 209:104948
Gao L et al (2022) A deep neural network based SMAP soil moisture product. Remote Sens Environ 277:113059
García-Gamero V, Vanwalleghem T, Peña A, Román-Sánchez A, Finke PA (2022) Modelling the effect of catena position and hydrology on soil chemical weathering. SOIL 8(1):319–335
Hao M et al (2019) Impacts of changes in vegetation on saturated hydraulic conductivity of soil in subtropical forests. Sci Rep 9(1):8372
Hassan-Esfahani L, Torres-Rua A, Jensen A, McKee M (2015) Assessment of surface soil moisture using high-resolution multi-spectral imagery and artificial neural networks. Remote Sens 7(3):2627–2646. https://doi.org/10.3390/rs70302627
Heiskanen J (1997) Air-filled porosity of eight growing media based on sphagnum peat during drying from container capacity. International Society for Horticultural Science (ISHS), Leuven, Belgium, pp. 277–286
Henderson TL, Baumgardner MF, Franzmeier DP, Stott DE, Coster DC (1992) High dimensional reflectance analysis of soil organic matter. Soil Sci Soc Am J 56(3):865–872. https://doi.org/10.2136/sssaj1992.03615995005600030031x
Hosseini M, Bahrami H, Khormali F, Khavazi K, Mokhtassi-Bidgoli A (2021) Artificial intelligence statistical analysis of soil respiration improves predictions compared to regression methods. J Soil Sci Plant Nutr 21(3):2242–2251
Huang H et al (2020) Water content quantitatively affects metabolic rates over the course of plant ontogeny. New Phytol 228(5):1524–1534. https://doi.org/10.1111/nph.16808
Huang Q, Zhang H, Zhang L, Xu B (2023) Bacterial microbiota in different types of processed meat products: diversity, adaptation, and co-occurrence. Crit Rev Food Sci Nutr. https://doi.org/10.1080/10408398.2023.2272770
Article PubMed Google Scholar
Hueso S, García C, Hernández T (2012) Severe drought conditions modify the microbial community structure, size and activity in amended and unamended soils. Soil Biol Biochem 50:167–173
Jamil N et al (2016) Physical and chemical properties of soil quality indicating forests productivity: a review. American-Eurasian J Toxicol Sci 8(2):60–68
Jian S, Zhao C, Fang S, Yu K (2015) Effects of different vegetation restoration on soil water storage and water balance in the Chinese Loess Plateau. Agric for Meteorol 206:85–96
Johnson NS et al (2020) Invited review: machine learning for materials developments in metals additive manufacturing. Addit Manuf 36:101641
CAS Google Scholar
Jong SC, Ong DEL, Oh E (2021) State-of-the-art review of geotechnical-driven artificial intelligence techniques in underground soil-structure interaction. Tunn Undergr Space Technol 113:103946
Karakan E (2022) Comparative analysis of atterberg limits, liquidity index, flow index and undrained shear strength behavior in binary clay mixtures. Appl Sci 12(17):8616
Karimi B et al (2020) A meta-analysis of the ecotoxicological impact of viticultural practices on soil biodiversity. Environ Chem Lett 18(6):1947–1966
Keller T, Håkansson I (2010) Estimation of reference bulk density from soil particle size distribution and soil organic matter content. Geoderma 154(3):398–406
Khaled H, Fawy AH (2011) Effect of different levels of humic acids on the nutrient content, plant growth, and soil properties under conditions of salinity. Soil Water Res 6(1):21–29
Kodali RK Sahu A (2016) An IoT based soil moisture monitoring on Losant platform. In 2016 2nd International Conference on Contemporary Computing and Informatics (IC3I), 2016, pp. 764–768.
Kose U, Prasath VS, Mondal MRH, Podder P, Bharati S (2022) Artificial intelligence and smart agriculture technology. CRC Press, Boca Raton
Book Google Scholar
Kouadio L, Deo RC, Byrareddy V, Adamowski JF, Mushtaq S, Phuong Nguyen V (2018) Artificial intelligence approach for the prediction of Robusta coffee yield using soil fertility properties. Comput Electron Agric 155:324–338
Lal R, Shukla MK (2004) Principles of soil physics. CRC Press, Boca Raton
Lambers H, Oliveira RS (2019) Plant water relations. In: Lambers H, Oliveira RS (eds) Plant physiological ecology. Springer International Publishing, Cham, pp 187–263
Leucci G (2012) Ground penetrating radar: an application to estimate volumetric water content and reinforced bar diameter in concrete structures. J Adv Concr Technol 10(12):411–422
Li X, Chang SX, Salifu KF (2014) Soil texture and layering effects on water and salt dynamics in the presence of a water table: a review. Environ Rev 22(1):41–50
Li D, Gao G, Shao M, Fu B (2016) Predicting available water of soil from particle-size distribution and bulk density in an oasis–desert transect in northwestern China. J Hydrol 538:539–550
Liu Q, Yasufuku N, Omine K, Hazarika H (2012) Automatic soil water retention test system with volume change measurement for sandy and silty soils. Soils Found 52(2):368–380
Liu Z, Zhou W, Shen J, Li S, Ai C (2014) Soil quality assessment of yellow clayey paddy soils with different productivity. Biol Fertil Soils 50(3):537–548
Liu X, Liang J, Gu L (2020) Photosynthetic and environmental regulations of the dynamics of soil respiration in a forest ecosystem revealed by analyses of decadal time series. Agric for Meteorol 282–283:107863
Liu J-W et al (2021) Surfactant-enhanced remediation of oil-contaminated soil and groundwater: a review. Sci Total Environ 756:144142
Liu X et al (2022) PiLSL: pairwise interaction learning-based graph neural network for synthetic lethality prediction in human cancers. Bioinformatics 38:ii106–ii112
Liu X-F, Zhu H-H, Wu B, Li J, Liu T-X, Shi B (2023) Artificial intelligence-based fiber optic sensing for soil moisture measurement with different cover conditions. Measurement 206:112312
Ma C et al (2022) The role and mechanism of commercial macroalgae for soil conditioner and nutrient uptake catalyzer. Plant Growth Regul 97(3):455–476
Mahmoudi N et al (2022) Mutating fuzzy logic model with various rigorous meta-heuristic algorithms for soil moisture content estimation. Agric Water Manag 261:107342
Maino A et al (2022) Airborne radiometric surveys and machine learning algorithms for revealing soil texture. Remote Sens. https://doi.org/10.3390/rs14153814
Mallah S et al (2022) Predicting soil textural classes using random forest models: learning from imbalanced dataset. Agronomy. https://doi.org/10.3390/agronomy12112613
Mallet F, Marc V, Douvinet J, Rossello P, Joly D, Ruy S (2020) Assessing soil water content variation in a small mountainous catchment over different time scales and land covers using geographical variables. J Hydrol 591:125593
Månsson KF, Olsson MO, Falkengren-Grerup U, Bengtsson G (2014) Soil moisture variations affect short-term plant-microbial competition for ammonium, glycine, and glutamate. Ecol Evol 4(7):1061–1072. https://doi.org/10.1002/ece3.1004
Martinelli G, Gasser M-O (2022) Machine learning models for predicting soil particle size fractions from routine soil analyses in Quebec. Soil Sci Soc Am J 86(6):1509–1522. https://doi.org/10.1002/saj2.20469
Masha M, Yirgu T, Debele M, Belayneh M (2021) Effectiveness of community-based soil and water conservation in improving soil property in Damota Area, Southern Ethiopia. Appl Environ Soil Sci 2021:5510587
Mei X et al (2019) The variability in soil water storage on the loess hillslopes in China and its estimation. CATENA 172:807–818
Miranda-Apodaca J, Pérez-López U, Lacuesta M, Mena-Petite A, Muñoz-Rueda A (2018) The interaction between drought and elevated CO2 in water relations in two grassland species is species-specific. J Plant Physiol 220:193–202
Mulla AR, Rodd SM, Sankpal SV, Yedave PM, Ghorpade YB, Nejkar MV (2023) Assessment of water logging and its remedial measures. Int J New Media Stud Int Peer Rev Sch Index J 10(1):143–151
Naimi S, Ayoubi S, Demattê JAM, Zeraatpisheh M, Amorim MTA, Mello FA (2022) Spatial prediction of soil surface properties in an arid region using synthetic soil image and machine learning. Geocarto Int 37(25):8230–8253
Ojeda Olivares EA, Belmonte Jiménez SI, Sandoval Torres S, Campos Enríquez JO, Tiefenbacher JP, Takaro TK (2020) A simple method to evaluate groundwater vulnerability in urbanizing agricultural regions. J Environ Manag 261:110164
Owe M, de Jeu R, Holmes T (2008) Multisensor historical climatology of satellite-derived global land surface moisture. J Geophys Res Earth Surf. https://doi.org/10.1029/2007JF000769
Pandey DK, Hunjra AI, Bhaskar R, Al-Faryan MA (2023) Artificial intelligence, machine learning and big data in natural resources management: a comprehensive bibliometric review of literature spanning 1975–2022. Resour Policy 86:104250
Pandya AB (2021) Data usage for development, management of water resources. In: Chadha G, Pandya AB (eds) Water governance and management in India: issues and perspectives. Springer Singapore, Singapore, pp 131–163
Pastén-Zapata E, Ledesma-Ruiz R, Harter T, Ramírez AI, Mahlknecht J (2014) Assessment of sources and fate of nitrate in shallow groundwater of an agricultural area by using a multi-tracer approach. Sci Total Environ 470:855–864
Penghui L et al (2020) Metaheuristic optimization algorithms hybridized with artificial intelligence model for soil temperature prediction: novel model. IEEE Access 8:51884–51904
Pereira LS, Paredes P, Jovanovic N (2020) Soil water balance models for determining crop water and irrigation requirements and irrigation scheduling focusing on the FAO56 method and the dual Kc approach. Agric Water Manag 241:106357
Pernet CA, Ribi Forclaz A (2019) Revisiting the Food and Agriculture Organization (FAO): international histories of agriculture, nutrition, and development. Int Hist Rev 41(2):345–350
Pham BT, Son LH, Hoang T-A, Nguyen D-M, Tien Bui D (2018) Prediction of shear strength of soft soil using machine learning methods. CATENA 166:181–191
Phoon K-K, Santoso A, Quek S-T (2010a) Probabilistic analysis of soil-water characteristic curves. J Geotech Geoenviron Eng 136(3):445–455
Pregitzer KS, King JS (2005) Effects of soil temperature on nutrient uptake. In: BassiriRad H (ed) Nutrient acquisition by plants an ecological perspective. Springer, Berlin, Heidelberg, pp 277–310
Rahardjo H, Kim Y, Satyanaga A (2019) Role of unsaturated soil mechanics in geotechnical engineering. Int J Geo-Eng 10(1):8
Raja MNA, Shukla SK (2021) Predicting the settlement of geosynthetic-reinforced soil foundations using evolutionary artificial intelligence technique. Geotext Geomembr 49(5):1280–1293
Ratshiedana PE, Abd Elbasit MAM, Adam E, Chirima JG, Liu G, Economon EB (2023) Determination of soil electrical conductivity and moisture on different soil layers using electromagnetic techniques in irrigated arid environments in South Africa. Water. https://doi.org/10.3390/w15101911
Rayne N, Aula L (2020) Livestock manure and the impacts on soil health: a review. Soil Syst. https://doi.org/10.3390/soilsystems4040064
Richards LA (2004) Capillary conduction of liquids through porous mediums. Physics 1(5):318–333
Riese FM, Keller S (2019) Soil texture classification with 1D convolutional neural networks based on hyperspectral data, ISPRS Annals of the Photogrammetry, Remote Sensing and Spatial Information Sciences, Volume IV-2/W5, 2019 ISPRS Geospatial Week 2019, 10–14 June 2019, Enschede, The Netherlands
Rooney EC et al (2022) Soil pore network response to freeze-thaw cycles in permafrost aggregates. Geoderma 411:115674
Roshan SH, Kazemitabar J, Kheradmandian G (2022) Artificial intelligence aided agricultural sensors for plant frostbite protection. Appl Artif Intell 36(1):2031814
Ruszczak B, Boguszewska-Mańkowska DJS (2022) Soil moisture a posteriori measurements enhancement using ensemble learning. Sensors 22(12):4591
Schlüter S et al (2022) Microscale carbon distribution around pores and particulate organic matter varies with soil moisture regime. Nat Commun 13(1):2098
Sharma A, Sen S (2022) Droughts risk management strategies and determinants of preparedness: insights from Madhya Pradesh, India. Nat Hazards 114(2):2243–2281
Sharma A, Weindorf DC, Wang D, Chakraborty S (2015) Characterizing soils via portable X-ray fluorescence spectrometer: 4. Cation exchange capacity (CEC). Geoderma 239–240:130–134
Singh IR, Nair PN (2023) Evaluation of physicochemical properties with the availability of plant nutrients in forests and crop farms in different Fijian provinces. Plant Sci Today 10(3):211–219
Stein A, Gerstner K, Kreft H (2014) Environmental heterogeneity as a universal driver of species richness across taxa, biomes and spatial scales. Ecol Lett 17(7):866–880. https://doi.org/10.1111/ele.12277
Sun W, Zhu H, Guo S (2015) Soil organic carbon as a function of land use and topography on the Loess Plateau of China. Ecol Eng 83:249–257
Tian K, Yang A, Nie K, Zhang H, Xu J, Wang X (2020) Experimental study of steady seepage in unsaturated loess soil. Acta Geotech 15(9):2681–2689
Ulusoy Y, Tekin Y, Tümsavaş Z, Mouazen AM (2016) Prediction of soil cation exchange capacity using visible and near infrared spectroscopy. Biosyst Eng 152:79–93
Vereecken H et al (2015) Soil hydrology: recent methodological advances, challenges, and perspectives. Water Resour Res 51(4):2616–2633
Vico G, Tang FHM, Brunsell NA, Crews TE, Katul GG (2023) Photosynthetic capacity, canopy size and rooting depth mediate response to heat and water stress of annual and perennial grain crops. Agric for Meteorol 341:109666
Villalobos M, Avila-Forcada AP, Gutierrez-Ruiz ME (2008) An improved gravimetric method to determine total petroleum hydrocarbons in contaminated soils. Water Air Soil Pollut 194(1):151–161
Vos M, Wolf AB, Jennings SJ, Kowalchuk GA (2013) Micro-scale determinants of bacterial diversity in soil. FEMS Microbiol Rev 37(6):936–954
Wadoux AM-C, Minasny B, McBratney AB (2020) Machine learning for digital soil mapping: applications, challenges and suggested solutions. Earth Sci Rev 210:103359
Wang C, Li S-Y, He X-J, Chen Q, Zhang H, Liu X-Y (2021) Improved prediction of water retention characteristic based on soil gradation and clay fraction. Geoderma 404:115293
Wang Q et al (2021a) Coastal soil texture controls soil organic carbon distribution and storage of mangroves in China. CATENA 207:105709
Wang S et al (2021b) Advances in data preprocessing for biomedical data fusion: an overview of the methods, challenges, and prospects. Inform Fus 76:376–421
Wang F, Wang Q, Adams CA, Sun Y, Zhang S (2022) Effects of microplastics on soil properties: current knowledge and future perspectives. J Hazard Mater 424:127531
Wang H, Garg A, Ping Y, Sreedeep S, Chen R (2023) Effects of biochar derived from coconut shell on soil hydraulic properties under salt stress in roadside bioretention. Waste Biomass Valorization 14(3):1005–1022
Wilson DJ, Western AW, Grayson RB (2004) Identifying and quantifying sources of variability in temporal and spatial soil moisture observations. Water Resour Res. https://doi.org/10.1029/2003WR002306
Wuddivira MN et al (2012) Estimation of soil clay content from hygroscopic water content measurements. Soil Sci Soc Am J 76(5):1529–1535. https://doi.org/10.2136/sssaj2012.0034
Xu J-S, Yang X-L (2018) Three-dimensional stability analysis of slope in unsaturated soils considering strength nonlinearity under water drawdown. Eng Geol 237:102–115
Xu Y, Gao Y, Li W, Chen S, Li Y, Shi Y (2023) Effects of compound water retention agent on soil nutrients and soil microbial diversity of winter wheat in saline-alkali land. Chem Biol Technol Agric 10(1):2
Yan Y et al (2022) Sulfur mass balance and speciation in the water cap during early-stage development in the first pilot pit lake in the Alberta Oil Sands. Environ Chem 19(4):236–253
Yu B, Kumbier K (2018) Artificial intelligence and statistics. Front Inform Technol Electron Eng 19(1):6–9
Zhai Y, Thomasson JA, Boggess JE, Sui R (2006) Soil texture classification with artificial neural networks operating on remote sensing data. Comput Electron Agric 54(2):53–68
Zhang M, Shi W (2019) Systematic comparison of five machine-learning methods in classification and interpolation of soil particle size fractions using different transformed data. Hydrol Earth Syst Sci Discuss 2019:1–39
Zhang F, Zhao C, Lourenço SDN, Dong S, Jiang Y (2021) Factors affecting the soil–water retention curve of Chinese loess. Bull Eng Geol Environ 80(1):717–729
Zhang Y et al (2021) Evaluating soil salt dynamics in a field drip-irrigated with brackish water and leached with freshwater during different crop growth stages. Agric Water Manag 244:106601
Zhao J, Lin L, Yang K, Liu Q, Qian G (2015) Influences of land use on water quality in a reticular river network area: a case study in Shanghai, China. Landsc Urban Plan 137:20–29
Zhao C, Jia X, Zhu Y, Shao M (2017) Long-term temporal variations of soil water content under different vegetation types in the Loess Plateau, China. CATENA 158:55–62
Zhao H, Xiao Q, Miao Y, Wang Z, Wang Q (2020) Sources and transformations of nitrate constrained by nitrate isotopes and Bayesian model in karst surface water, Guilin, Southwest China. Environ Sci Pollut Res 27(17):21299–21310
Download references
Acknowledgements
Researchers Supporting Project number (RSPD2024R1060), King Saud University, Riyadh, Saudi Arabia. The authors are thankful to Henan agriculture university for providing the research facilities.
Researchers Supporting Project number (RSPD2024R1060), King Saud University, Riyadh, Saudi Arabia. Also, this work was supported by the National Natural Science Foundation of China, Grant Number 32071890, 31671581 and supported by the Henan Center for Outstanding Overseas Scientists, Grant Number GZS2021007.
Author information
Authors and affiliations.
College of Mechanical and Electrical Engineering, Henan Agricultural University, Zhengzhou, 450002, China
Muhammad Awais, Syed Muhammad Zaigham Abbas Naqvi, Hao Zhang, Linze Li, Wei Zhang & Jiandong Hu
Henan International Joint Laboratory of Laser Technology in Agriculture Sciences, Zhengzhou, 450002, China
Department of Quantitative Analysis, College of Business Administration, King Saud University, P.O. Box 71115, Riyadh 11587, Saudi Arabia
Fuad A. Awwad & Emad A. A. Ismail
Department of Mathematics and Statistics, Riphah International University, I-14, Islamabad, 44000, Pakistan
M. Ijaz Khan
Department of Mechanical Engineering, Lebanese American University, Kraytem, Beirut, 1102-2801, Lebanon
Department of Bioresource Engineering, Faculty of Agriculture and Environmental Studies, McGill University, Sainte-Anne-de-Bellevue, QC, H9X 3V9, Canada
Vijaya Raghavan
You can also search for this author in PubMed Google Scholar
Corresponding author
Correspondence to Jiandong Hu .
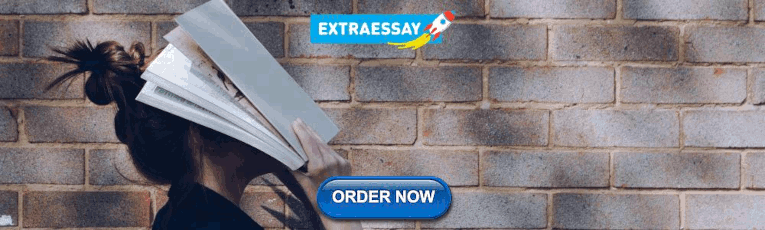
Ethics declarations
Ethics approval and consent to participate.
Not applicable.
Competing interests
The authors declares that they have no competing interests.
Additional information
Publisher's note.
Springer Nature remains neutral with regard to jurisdictional claims in published maps and institutional affiliations.
All authors are equally contributed in the research work.
Rights and permissions
Open Access This article is licensed under a Creative Commons Attribution 4.0 International License, which permits use, sharing, adaptation, distribution and reproduction in any medium or format, as long as you give appropriate credit to the original author(s) and the source, provide a link to the Creative Commons licence, and indicate if changes were made. The images or other third party material in this article are included in the article's Creative Commons licence, unless indicated otherwise in a credit line to the material. If material is not included in the article's Creative Commons licence and your intended use is not permitted by statutory regulation or exceeds the permitted use, you will need to obtain permission directly from the copyright holder. To view a copy of this licence, visit http://creativecommons.org/licenses/by/4.0/ .
Reprints and permissions
About this article
Cite this article.
Awais, M., Naqvi, S.M.Z.A., Zhang, H. et al. AI and machine learning for soil analysis: an assessment of sustainable agricultural practices. Bioresour. Bioprocess. 10 , 90 (2023). https://doi.org/10.1186/s40643-023-00710-y
Download citation
Received : 08 August 2023
Accepted : 25 November 2023
Published : 07 December 2023
DOI : https://doi.org/10.1186/s40643-023-00710-y
Share this article
Anyone you share the following link with will be able to read this content:
Sorry, a shareable link is not currently available for this article.
Provided by the Springer Nature SharedIt content-sharing initiative
- Intelligent agriculture
- Agronomic forecasting
- Soil texture
- Water content analysis
- Smarter agriculture 4.0
Soil Remediation Science and Technology
- © 2024
- Jose Julio Ortega-Calvo 0 ,
- Frederic Coulon 1
Instituto de Recursos Naturales y Agrobiologia de Sevilla, Consejo Superior de Investigaciones Cientificas Sevilla, Sevilla, Spain
You can also search for this editor in PubMed Google Scholar
School of Water, Energy, and Environment, Cranfield University, Cranfield, UK
- Reviews soil pollution and remediation strategies
- Discusses the latest innovative sustainable solutions in the field
- Covers environmental chemistry, microbiology, ecotoxicology and regulation
Part of the book series: The Handbook of Environmental Chemistry (HEC, volume 130)
148 Accesses
1 Citations
This is a preview of subscription content, log in via an institution to check access.
Access this book
- Available as EPUB and PDF
- Read on any device
- Instant download
- Own it forever
- Durable hardcover edition
- Dispatched in 3 to 5 business days
- Free shipping worldwide - see info
Tax calculation will be finalised at checkout
Other ways to access
Licence this eBook for your library
Institutional subscriptions
About this book
This book reviews the latest advances in soil remediation and is an authoritative account of the environmental chemistry, microbiology, ecotoxicology, and regulation policies of soil pollution. The book also discusses possible pathways for innovation, by incorporating state-of-the-art knowledge on sustainability, nature-based solutions, and socio-economical aspects. Divided into four parts, the book opens with an overview of the legal context and policy economy of soil pollution and remediation. The management of contaminated soils has a high cost and, although much of this cost is borne by companies, there is also high public spending. The strategic value of soil, the extensive costs associated with the remediation of many polluted sites throughout the World, and the current crisis demand for new solutions to soil remediation that are addressed in the following parts of the book. In this book, readers will find a comprehensive description of several remediation strategies by different pollution sources, nature-based solutions, and physicochemical methods for the remediation of contaminated soils. Particular attention is given to contaminated soils from industrial activities, urban settings, mining, and military activities. In the final chapter of the book, the editors present a perspective of the field, research trends and needs. Given its breadth, this book appeals to regulators, industrial scientists, and scholars alike.
- Soil Pollution
- Environmental Policy
- Remediation
- Nature-based solutions
- Sustainability
- Contaminated Soils
- Ecotoxicology
- low-carbon bioremediation
- Phytoremediation
- Electro-nano remediation
- Soil Pollution by industry
- soil pollution by urban settings
- soil pollution by mining activities
- Bioaugmentation
- soil pollution by military activities
Table of contents (16 chapters)
Front matter, introduction setting of the scene, definitions and guide to volume.
- Frederic Coulon, Jose Julio Ortega-Calvo
Legal and Policy Context
Soil pollution framework in europe.
- Bożena Smreczak, Agnieszka Klimkowicz-Pawlas, Aleksandra Ukalska-Jaruga
Industrial Soil Remediation Policy and Regulation in the European Union
- Anna Espinoza-Tofalos, Carlos de Miguel Perales
Remediation of Contaminated Soils
Managing per- and polyfluoroalkyl substance (pfas) contamination in agricultural soils: investigating remediation approaches in non-conventional agriculture.
- Juan C. Sanchez-Hernandez, Rodrigo Pardo Fernández, Natividad I. Navarro Pacheco, Ximena Andrade Cares, Jorge Domínguez
Remediation of Soils Polluted by Urban Settings
- Magdalena Sut-Lohmann, Artur Pędziwiatr, Jerzy Jonczak, Bogusława Kruczkowska
Review of Digital Solutions for Soil Contamination Management by Mining Activities
- F. J. Blanco-Velázquez, M. Anaya-Romero
Remediation of Soils Polluted by Military Activities
- Carmen Fernández-López, Tracey Temple, Federica Persico, Frederic Coulon, Jose Julio Ortega-Calvo
Remediation of Soils Polluted by Oil Industries
- G. K. Vasilyeva, E. R. Strijakova, J. J. Ortega-Calvo
Nature-based Solutions for Soil Remediation
Soil bioamendment as a low-carbon approach for microbial remediation of organic and inorganic pollutants.
- Emmanuel Atai, Ikeabiama Azuazu, Raphael Butler Jumbo, Vinod Kumar, Kabari Sam, Carmen Fernández López et al.
Drivers for Efficient Bioaugmentation and Clean-Up of Contaminated Soil
- María Balseiro-Romero, Lukas Y. Wick, Joaquim Vila, Magdalena Grifoll, José Julio Ortega-Calvo
Sustainable Low Carbon and Bioaugmentation Strategies for Bioremediation of Oil-Contaminated Acidic Wetlands
- Raphael Butler Jumbo, Emmanuel Atai, Ike Azuazu, Imma Bortone, Frederic Coulon, Ying Jiang
Phytoremediation of Co-contaminated Soils by Heavy Metals and Persistent Organic Pollutants
- Longfei Jiang, Yuchu Xie, Jurate Kumpiene, Chunling Luo
Bioremediation of Soils Contaminated with PFAS: An Update on Available Techniques, Pilot Studies, Challenges, and Future Directions
- Vladimir Beškoski, Marija Lješević, Begoña Jiménez, Juan Muñoz-Arnanz, Pere Colomer-Vidal, Hideyuki Inui et al.
Physicochemical Processes Employed in Soil Remediation
Physicochemical remediation of soil contamination: from laboratory to field.
- Hanwen Wang, Jingqi Zhang, Hai Huang, Xingrun Wang, Xiaojin Yang, Frederic Coulon
Coupling Physical and Chemical-Biological Techniques for the Remediation of Contaminated Soils and Groundwater
- Paolo Ciampi, Marco Zeppilli, Laura Lorini, Marianna Villano, Carlo Esposito, Christian Nielsen et al.
Editors and Affiliations
Jose Julio Ortega-Calvo
Frederic Coulon
About the editors
José-J. Ortega-Calvo is a Researcher at the Institute of Natural Resources and Agrobiology of Seville, Spanish National Research Council (IRNAS-CSIC). He has worked on bioavailability and biodegradation of organic pollutants for the last 25 years. After obtaining his PhD in 1991 at the University of Seville, he worked on different microbial ecology aspects during postdoctoral stays at the University of Amsterdam (UvA, The Netherlands) and Cornell University (USA). Dr Ortega was in 2016/2017 the European President of the Society for Environmental Toxicology and Chemistry (SETAC Europe) and, from June 2017 to June 2023, a member of the Stakeholder Bureau of the European Food Safety Authority (EFSA). He is a member of the External Science Advisory Panel (ESAP) of the Long-Range Research Initiative at the European Chemical Industry Council (CEFIC-LRI), and Associate Editor of Science of the Total Environment and Critical Reviews in Environmental Science and Technology journals.
Frederic Coulon is a Professor of Environmental Chemistry and Microbiology at the Centre for Water, Environment and Development at Cranfield University, UK. He has a 24-year international research and consultancy career in water, energy and soil science, diagnostic strategy and risk analysis and management. Trained in chemistry, microbiology, and environmental engineering (University of Perpignan, France) and risk management (Cranfield, UK), he led several projects on soil and water protection and recovery, industrial and hazardous waste management, wastewater treatment and risk management. His work enabled to construct innovative decision-support tools for contaminated sites that improved the confidence in remediation (restoring to use) technology, reduce remediation costs and minimize waste disposal to landfill, with subsequent savings in CO2 emissions. His work further influences policy development and provides pragmatic, risk-based solutions to remediate contaminated sites. He has published numerous articles in leading environmental engineering and science journals, book chapters and articles for the popular press with substantive contributions to oil remediation, process emission and control at industrial sites and environmental risk management. He is the chair of the NICOLE Academic subgroup, the leading European network on sustainable land management of industrially contaminated sites, co-Editor in Chief of Environment International, Section Editor of Heliyon Environment and Associate Editors of Science of the Total Environment and Critical Reviews in Environmental Science and Technology journals.
Bibliographic Information
Book Title : Soil Remediation Science and Technology
Editors : Jose Julio Ortega-Calvo, Frederic Coulon
Series Title : The Handbook of Environmental Chemistry
DOI : https://doi.org/10.1007/978-3-031-60192-7
Publisher : Springer Cham
eBook Packages : Chemistry and Materials Science , Chemistry and Material Science (R0)
Copyright Information : The Editor(s) (if applicable) and The Author(s), under exclusive license to Springer Nature Switzerland AG 2024
Hardcover ISBN : 978-3-031-60191-0 Published: 22 June 2024
Softcover ISBN : 978-3-031-60194-1 Due: 23 July 2024
eBook ISBN : 978-3-031-60192-7 Published: 21 June 2024
Series ISSN : 1867-979X
Series E-ISSN : 1616-864X
Edition Number : 1
Number of Pages : X, 437
Number of Illustrations : 26 b/w illustrations, 26 illustrations in colour
Topics : Environmental Chemistry , Agriculture , Pollution, general , Microbial Ecology , Physical Chemistry , Environmental Engineering/Biotechnology
- Publish with us
Policies and ethics
- Find a journal
- Track your research
Understanding Your Soil Test Report
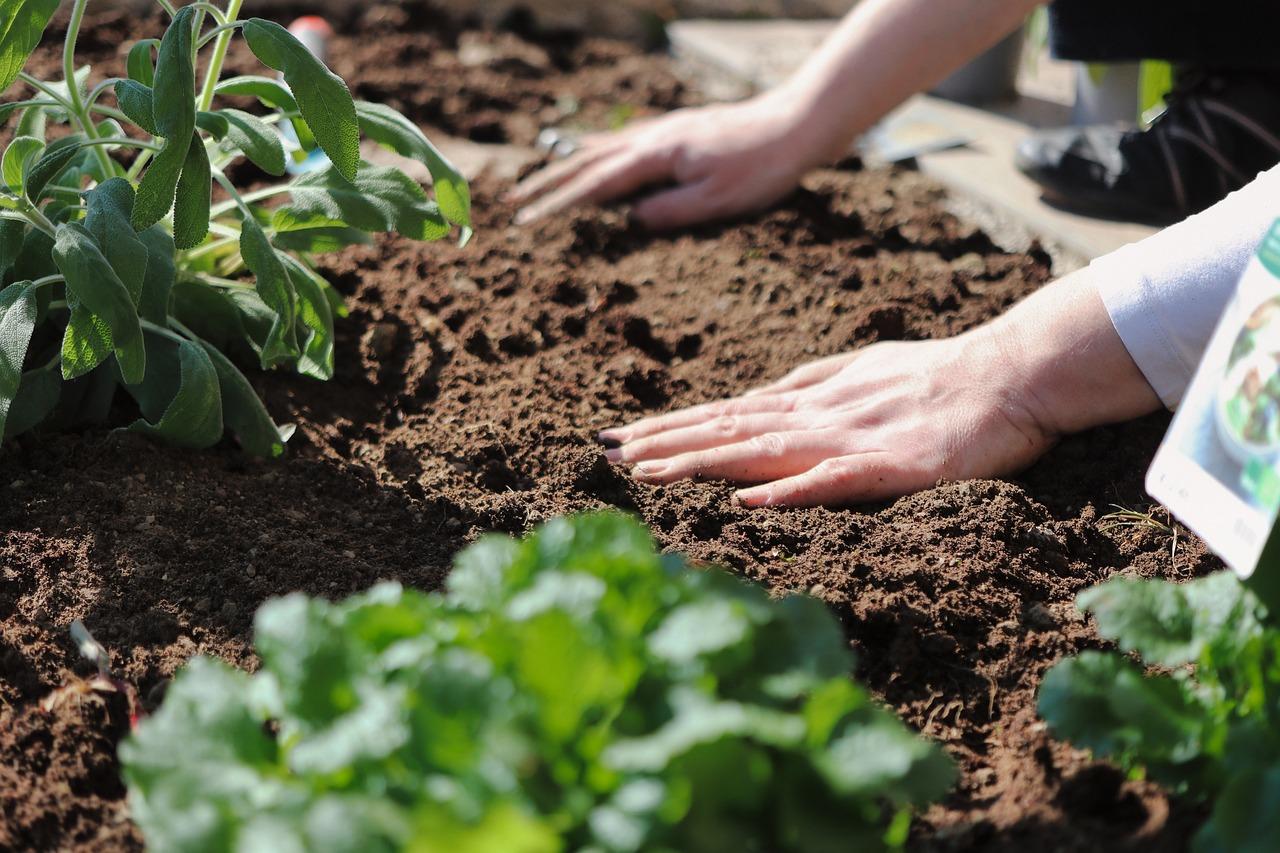
Information you will find on a soil test report
A soil test report provided by a laboratory will indicate the levels of nutrients in your soil as well as other components. Soil pH, Phosphorus (P), Potassium (K), Magnesium (Mg), Calcium (Ca), organic matter (OM), and fertilizer and lime recommendations are especially important for maintaining a garden or lawn.
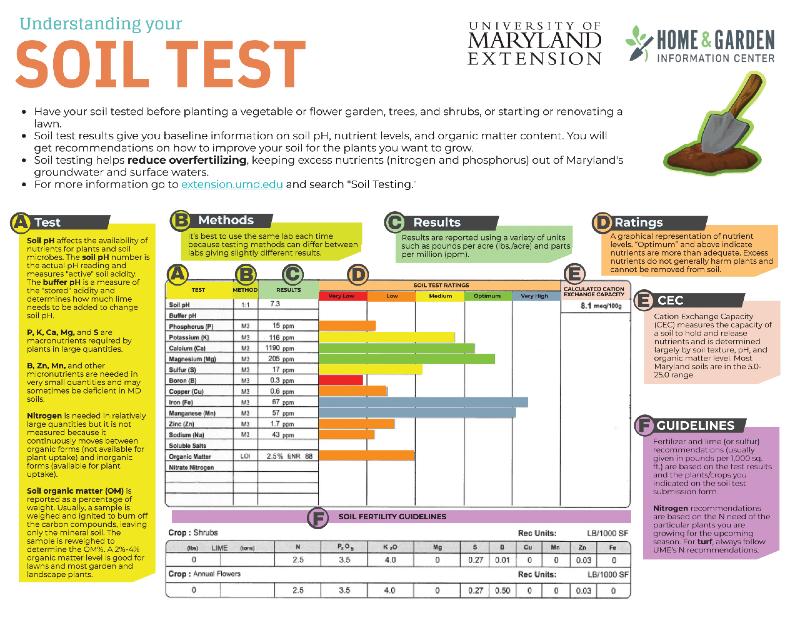
Download sample soil report
- An "optimal" or "excessive" or “very high” level means that a nutrient in the soil is more than adequate. Adding more of that nutrient will not improve plant growth.
- Excessive amounts of nutrients do not harm plants and cannot be physically removed from the soil. There are exceptions, such as when soils are very low in pH and high in aluminum or manganese, making those nutrients available to plants at toxic levels.
- Phosphorus (P) , in particular, should not be added if levels are in the "medium" to "optimum" to “high” range. It can get into waterways when soil is moved off-site by water and gravity. Along with nitrogen, phosphorus feeds the growth of algae in bodies of water like the Chesapeake Bay. When the algae die, it causes a condition called eutrophication - excess nutrients in the water - which leads to low oxygen levels that kill aquatic plants and wildlife
Cation Exchange Capacity
Cation Exchange Capacity (CEC) measures the capacity of soil to hold and release nutrient ions. Negative charges on clay and organic matter particles attract positively charged ions (cations) like potassium and calcium. Soils with higher percentages of clay and/or organic matter generally have higher CEC. This measurement will vary across Maryland soils. Add organic matter if your soil's CEC is less than 10.
Organic Matter
- Soil organic matter (OM) is made up of living, dead, and decomposing plants, small animals, and microorganisms. Soils high in OM retain more moisture, have a crumbly structure that resists soil compaction, and contain a reservoir of nutrients that are slowly released over time.
- OM is reported on a percentage-by-weight basis in the lab report. Usually, a sample is weighed in the lab and then ignited to burn off the carbon compounds, leaving only the mineral soil. The sample is reweighed to determine the OM%. Gardeners who add lots of organic matter to their soils may be surprised that the OM content is less than 5%. This is because OM is less dense than mineral soil and the measurement is based on weight, not volume.
- Healthy soils have a 2% or greater OM content. Regular additions of organic matter will gradually increase the OM level. Where the topsoil is thin and OM is low you may need to add 2-4 inches of compost for a few years. Yearly additions of 1 inch of compost can help maintain high productivity in vegetable and flower gardens. It is not uncommon for well-established vegetable gardens to have an OM content >12%.
- Soil pH is a measure of how acidic (sour) or basic (sweet) your soil is. Soil pH directly affects nutrient availability. For example, phosphorous (P) combines with iron and aluminum when the soil pH is low (below 6.0), and with calcium when the soil pH is high (above 7.3). In both cases, less P is available for plant uptake. The pH scale ranges from 0-14 with 7 as neutral. Numbers less than 7 indicate acidity, while numbers greater than 7 are basic.
- Vegetables, grasses, and most native and ornamentals grow best in the 5.5-7.0 pH range.
- Conifers and ericaceous plants (azaleas, rhododendrons, blueberries, and their relatives) prefer acidic soils (pH 4.5-5.5)
- Soil pH values significantly above or below these ranges may result in less vigorous growth or symptoms of nutrient deficiency or toxicity.
- Soils high in clay and organic matter have a lot of "stored" acidity because these particles have many negatively charged sites that hold cations (positive charges). Hydrogen ions (H+) and aluminum ions (Al+++) are the principal sources of stored acidity. It takes more calcium (lime) to raise the pH of clayey soil than it would sandy soil. Such soil is more highly buffered, meaning it resists pH changes.
- Buffer pH is measured by adding a weak 8.0 pH base to low pH soil samples.
- The lower the buffer pH (the closer it is to the actual soil pH), the more lime is required to raise soil pH.
How to Change Soil pH
Raising soil ph.
- Lime is added to soil to raise soil pH.
- “Garden lime” is made from ground-up rock (calcitic limestone) and provides calcium.
- “Dolomitic lime” contains calcium and magnesium and is recommended by soil testing labs when soil pH and magnesium levels both need to be increased..
- Gardeners can choose between powdered, coarsely ground (granular), or pelletized lime. The finer the particle or mesh size the quicker it will act to raise soil pH.
- Coarsely ground and pelletized lime is less likely to clog when applied with a fertilizer spreader to turf.
- Hydrated lime (calcium hydroxide) is quick-acting and is not recommended because it can burn garden plants and lawns if used incorrectly.
- Liming recommendations from soil testing labs are usually given as pounds of ground limestone per 1,000 sq. ft. Don't spread more than 50 lbs./1,000 sq. ft. of lime on your lawn in a single application. For example: if the recommended amount is 85 lbs., apply the first 50 lbs. now and the remaining 35 lbs. roughly six months from now. This gives the lime the time to move down into the soil profile without spiking the pH in the top few inches of soil.
- Up to 70 lbs of limestone per thousand square feet can be applied in a single application if it is worked into the soil.
Lowering Soil pH
- Sulfur is a highly mobile and soluble nutrient found in the soil as sulfate anions (negative charge). It is difficult to measure accurately and may be omitted from basic soil tests.
- Soil sulfur deficiencies may be increasing in Maryland as less sulfur is emitted by coal-burning power plants.
- Elemental sulfur (90% S), sold as flowers of sulfur, micro-fine sulfur, and granular sulfur, and iron sulfate (12% S) are used to lower soil pH. These and other soil-acidifying products are widely available at garden centers and hardware stores.
- Avoid using aluminum sulfate because aluminum becomes more available with declining pH values and may lead to plant toxicity problems.
- Use the chart for reducing soil pH if the lab does not provide a specific recommendation.
Fertilizer recommendations in a soil test report
- Always follow the University of Maryland lawn fertilization guidelines for nitrogen regardless of the recommendations given in the soil test report.
- Apply phosphorus (P) to turf only if the levels are low.
- lbs. per acre/ 43.56 = lbs. / 1,000 sq. ft.
- lbs. per acre/ 435.6 = lbs. / 100 sq. ft.
For other plants
- Read garden fertilizer basics .
Fertilizing Trees & Shrubs Fertilizing Vegetables Fertilizing Fruits
Common questions
How do i select a fertilizer based on the lab’s nutrient recommendations.
Example: Your soil test report recommends applying 2 lbs. of nitrogen (N), 3.5 lbs. of phosphate (P 2 O 5 ), and 4.0 lbs. of potash (K 2 O) per 1,000 sq. ft. to your flower garden soil.
Solutions :
- Look for fertilizers with an N-P-K ratio that is close to the amounts of recommended N, P, and K. In this case, 2-3.5-4.0; or,
- Purchase and apply three separate single-nutrient fertilizers. Examples: nitrate of soda (16-0-0), superphosphate (0-22-0), and muriate of potash (0-0-60)
How do I convert a synthetic (chemical) fertilizer recommendation for an organic fertilizer?
Example: the recommendation is to apply 2 lbs. of N per 1,000 sq. ft. by applying 4.35 lbs. of urea fertilizer (46-0-0). You want to substitute cottonseed meal (6-2-1).
Solution #1: Divide the percentage of N in the synthetic fertilizer by the percentage of N in the organic fertilizer and multiply by 4.35 (amount of urea): 0.46 ÷ by 0.06 X 4.35 = 33.3 lbs. of cottonseed meal
Solution #2: Calculate the amount of the fertilizer product needed by dividing the pounds of N needed by the percentage of N in the product. 2.0 ÷ by 0.06 = 33.3 lbs. of cottonseed meal fertilizer per 1,000 sq. ft. If your garden is 500 sq. ft. you would apply ½ the amount.
Read more: How to Convert an Inorganic Fertilizer Recommendation to an Organic One
Related information
Soil pH Affects Nutrient Availability
The Importance of pH and Liming Material
Additional resources
(PDF) Fertilizer Conversions | University of Connecticut
(PDF) Converting Fertilizer Rates from Tons to Teaspoons | Louisiana State University
The USDA National Resources Conservation Service Web Soil Survey
Author: Jon Traunfeld, Director HGIC, Extension Specialist, Fruits, and Vegetables. Revised 2023. Still have a question? Send it to Ask Extension . Please upload a copy of your soil test results with your question.
Dynamic evaluation of tiered geogrid mechanically stabilized earth (MSE) walls using shake table test
- Yazdandoust, Majid
- Jamnani, Amirhossein Rasouli
- Sabermahani, Mohsen
Shaking table tests were performed on reduced-scale models of integrated and two-tiered mechanically stabilized earth walls (TMSEWs) to evaluate the effect of a tiered configuration on the dynamic behavior of geogrid-reinforced soil walls. The results of particle image velocimetry and instrumentation indicate that preventing the development of a slip surface in the lower half of the wall, improving the seismic stability by increasing the failure threshold acceleration, mitigating acceleration amplification and decreasing the reinforcement load were the main advantages of a tiered configuration. It was found that the use of an insufficient offset distance in TMSEWs not only eliminated the advantage of the tiered configuration for reducing wall deformations, but also increased the lateral displacement at the wall crest. In this regard, 0.22H was identified as the minimum offset distance required when constructing MSE walls in a tiered configuration. Moreover, comparison of integrated and tiered MSE walls showed that the effect of a tiered configuration on reducing the force of the reinforcements in the lower tier was approximately 2.2 times that for the upper tier reinforcements. It was also found that Mononobe-Okabe method can be used to find the upper bound for estimating the load of reinforcements in TMSEWs.
- Dynamic performance;
- Failure mechanism;
- Particle image velocimetry;
- Reinforcement load;
- Two-tiered MSE wall
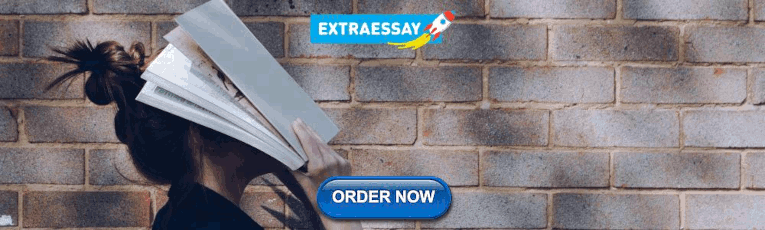
IMAGES
VIDEO
COMMENTS
Soil is a critical element that carries food nutrients for plant growth (Van Walt, 2015). Farmers. ntmanageme. t and provides critical information to help soybean, corn, and other agricultural cro. production. Soil testing can also help farmers prevent unnecessary nutrient applicati. s if their.
Soil testing is a cornerstone of sustainable agriculture, offering a range of benefits that align with the principles of economic viability, environmental stewardship, and social responsibility ...
1. Introduction. Soil is the thin layer covering Earth's surface upon which living things depend for food, feed and fiber production; environmental buffering; and ecosystem services (Barrios, 2007).Depending on how it's managed, soil will be considered to have either positive or negative environmental effects (Steffan et al., 2018).If well-managed, the long-term organic farming system can ...
Ultimately soil and crop nutrient balance is negative. Almost, farmers are giving straight fertilizer and other nutrients will be completely mined. On the basis of soil test values can be achieved ...
soil nutrients. Introduction. Soil contains 16 essential elements like carbon, hydrogen, oxygen, nitrogen, calcium, etc. for the. proper completion of the plants life cycle. Out of these, three ...
Testing the relations between soil attributes and soil functions may increase the importance of such attributes (Andrews and Carroll, 2001). Fine et al. (2017) used PCA to examine the relationships between SH indicators measured in distinctively different regions across the US. They found a relatively high number (6) of significant principal ...
Conventional soil tests are commonly used to assess single soil characteristics. Thus, many different tests are needed for a full soil fertility/soil quality assessment, which is laborious and expensive. New broad-spectrum soil tests offer the potential to assess many soil characteristics quickly, but often face challenges with calibration, validation, and acceptance in practice. Here, we ...
The concept of soil quality by Doran and Parkin (1994) was heavily criticized in a series of papers (Letey et al., 2003, Sojka and Upchurch, 1999, ... For example, in the outputs the Cornell soil health test, in Sindi, and in the Australian soil quality monitoring framework a traffic light system of 3-5 colours indicates low, adequate or ...
Soil health plays a crucial role in crop production, both in terms of quality and quantity, highlighting the importance of effective methods for preserving soil quality to ensure global food security. Soil quality indices (SQIs) have been widely utilized as comprehensive measures of soil function by integrating multiple physical, chemical, and biological soil properties. Traditional SQI ...
In the past decade soil health has been intensively studied as a science and practiced as a means to help improve the global social, environmental, and economic sustainability. This paper reviews the recent advances of the scientific soil health system. The current understanding and interpretation of soil health from the perspectives of soil functions, processes, and properties is summarized.
The concepts also differ in the view of soil rights and assessments. Soil health encompasses a broad range of ecosystem functions, services and actors, impacting a wide array of sustainability ...
The disparities in nutrient composition between soil and crop roots facilitate the process by which crops absorb nutrients. The plant nutrient concentration refers to the quantity of nutrients found in the sap of a plant, which is measured as a ratio of mass or molarity per unit volume (Chen et al. 2022).Plant nutrient concentration varies with plant species, growth stage, and environmental ...
Testing soil pH as a first experiment in quantitative analysis; involves sampling and subsampling, massing techniques, moisture determination, data recording, and the variability common to real samples. KEYWORDS (Audience): Second-Year Undergraduate. KEYWORDS (Domain): Analytical Chemistry. KEYWORDS (Pedagogy): Hands-On Learning / Manipulatives.
Here we review the microbial indices used to assess or monitor soil health and discuss some of the broader issues associated with their use. We provide recommendations to more effectively guide and improve how microbial information could be used to yield relevant and actionable assessments of soil health. Soil health.
The soil chemical and physical properties included in soil-test correlation papers vary widely and are seldom consistent among published research (Breker et al., 2019; Heckman et al., 2006; Mallarino & Blackmer, 1992, 1994; Slaton et al., 2010; Williams et al., 2018). The lack of a consistent suite of soil-test information requirements limits ...
Department of Agriculture & Natural Resources, Delaware State U niversity, Dover, DE. 19901, USA; [email protected]. Abstract: In the past decade soil health has been intensively studied as a. science ...
Procedure: 1. Remove 1-2 cm of surface soil from the spot where sample will be taken, and level the spot. 2. Drive a 5 cm diameter thin sheet metal tube of known weight (W1) and volume (V) 5 cm into the soil. 3. Excavate the soil from around the tube and cut the soil beneath the tube bottom.
Soil analysis can provide important information about physical conditions, fertility (nutrient) status, and chemical properties that affect a soil's suitability for growing plants. Four steps associated with soil testing include: 1) soil sample collection, 2) laboratory analysis, 3) interpretation of results, and 4) fertilizer or other ...
Typically, a composite sample should be comprised of between 10 and 20 subsamples. The more subsamples you add into a composite, the more reliable a sample becomes. Using the trowel, thoroughly mix the soil in the bucket until you have a homogeneous mixture. Place 1-2 cups of the mixture into a sample bag.
He has a 24-year international research and consultancy career in water, energy and soil science, diagnostic strategy and risk analysis and management. Trained in chemistry, microbiology, and environmental engineering (University of Perpignan, France) and risk management (Cranfield, UK), he led several projects on soil and water protection and ...
quality is not limited to the degree of soil pollution, but is commonly. de fined much more broadly as the capacity of a soil to function within. ecosystem and land-use boundaries to sustain ...
particles will not be balanced, and the soil will need to be altered by adding organic amendments. To evaluate soil texture, use a simple jar test to determine the percentages of sand silt, and clay. Once the percentages are calculated, the soil textural triangle can be used to determine the soil type. Soil Texture Analysis "The Jar Test ...
2.3 Soil tests for P. Soil testing is the rapid extraction and analysis of P to assess the plant-available P status of a soil. It involves sampling soil from fields, laboratory extraction and analysis, interpretation of results, and making a fertilizer recommendation (Beegle, 2005 ).
Information you will find on a soil test report. A soil test report provided by a laboratory will indicate the levels of nutrients in your soil as well as other components. Soil pH, Phosphorus (P), Potassium (K), Magnesium (Mg), Calcium (Ca), organic matter (OM), and fertilizer and lime recommendations are especially important for maintaining a garden or lawn.
The values of soil pH (Table 1) in this area range from 7.4 to 7.9 indicating an alkaline nature of. soil while EC values r ange from 0.3 to 0.6 mS/cm (normal EC ranges from 0.02 to 2.0 mS/cm) and ...
Shaking table tests were performed on reduced-scale models of integrated and two-tiered mechanically stabilized earth walls (TMSEWs) to evaluate the effect of a tiered configuration on the dynamic behavior of geogrid-reinforced soil walls. The results of particle image velocimetry and instrumentation indicate that preventing the development of a slip surface in the lower half of the wall ...