Have a language expert improve your writing
Run a free plagiarism check in 10 minutes, generate accurate citations for free.
- Knowledge Base
Methodology
- Correlational Research | When & How to Use
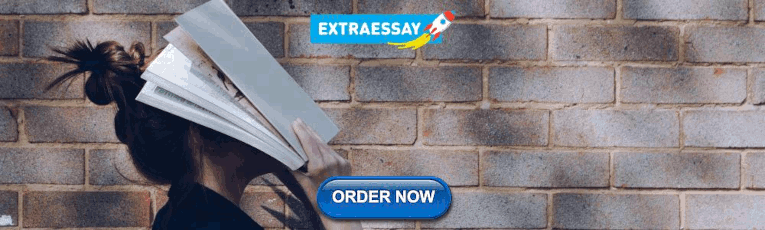
Correlational Research | When & How to Use
Published on July 7, 2021 by Pritha Bhandari . Revised on June 22, 2023.
A correlational research design investigates relationships between variables without the researcher controlling or manipulating any of them.
A correlation reflects the strength and/or direction of the relationship between two (or more) variables. The direction of a correlation can be either positive or negative.
Positive correlation | Both variables change in the same direction | As height increases, weight also increases |
---|---|---|
Negative correlation | The variables change in opposite directions | As coffee consumption increases, tiredness decreases |
Zero correlation | There is no relationship between the variables | Coffee consumption is not correlated with height |
Table of contents
Correlational vs. experimental research, when to use correlational research, how to collect correlational data, how to analyze correlational data, correlation and causation, other interesting articles, frequently asked questions about correlational research.
Correlational and experimental research both use quantitative methods to investigate relationships between variables. But there are important differences in data collection methods and the types of conclusions you can draw.
Correlational research | Experimental research | |
---|---|---|
Purpose | Used to test strength of association between variables | Used to test cause-and-effect relationships between variables |
Variables | Variables are only observed with no manipulation or intervention by researchers | An is manipulated and a dependent variable is observed |
Control | Limited is used, so other variables may play a role in the relationship | are controlled so that they can’t impact your variables of interest |
Validity | High : you can confidently generalize your conclusions to other populations or settings | High : you can confidently draw conclusions about causation |
Prevent plagiarism. Run a free check.
Correlational research is ideal for gathering data quickly from natural settings. That helps you generalize your findings to real-life situations in an externally valid way.
There are a few situations where correlational research is an appropriate choice.
To investigate non-causal relationships
You want to find out if there is an association between two variables, but you don’t expect to find a causal relationship between them.
Correlational research can provide insights into complex real-world relationships, helping researchers develop theories and make predictions.
To explore causal relationships between variables
You think there is a causal relationship between two variables, but it is impractical, unethical, or too costly to conduct experimental research that manipulates one of the variables.
Correlational research can provide initial indications or additional support for theories about causal relationships.
To test new measurement tools
You have developed a new instrument for measuring your variable, and you need to test its reliability or validity .
Correlational research can be used to assess whether a tool consistently or accurately captures the concept it aims to measure.
There are many different methods you can use in correlational research. In the social and behavioral sciences, the most common data collection methods for this type of research include surveys, observations , and secondary data.
It’s important to carefully choose and plan your methods to ensure the reliability and validity of your results. You should carefully select a representative sample so that your data reflects the population you’re interested in without research bias .
In survey research , you can use questionnaires to measure your variables of interest. You can conduct surveys online, by mail, by phone, or in person.
Surveys are a quick, flexible way to collect standardized data from many participants, but it’s important to ensure that your questions are worded in an unbiased way and capture relevant insights.
Naturalistic observation
Naturalistic observation is a type of field research where you gather data about a behavior or phenomenon in its natural environment.
This method often involves recording, counting, describing, and categorizing actions and events. Naturalistic observation can include both qualitative and quantitative elements, but to assess correlation, you collect data that can be analyzed quantitatively (e.g., frequencies, durations, scales, and amounts).
Naturalistic observation lets you easily generalize your results to real world contexts, and you can study experiences that aren’t replicable in lab settings. But data analysis can be time-consuming and unpredictable, and researcher bias may skew the interpretations.
Secondary data
Instead of collecting original data, you can also use data that has already been collected for a different purpose, such as official records, polls, or previous studies.
Using secondary data is inexpensive and fast, because data collection is complete. However, the data may be unreliable, incomplete or not entirely relevant, and you have no control over the reliability or validity of the data collection procedures.
After collecting data, you can statistically analyze the relationship between variables using correlation or regression analyses, or both. You can also visualize the relationships between variables with a scatterplot.
Different types of correlation coefficients and regression analyses are appropriate for your data based on their levels of measurement and distributions .
Correlation analysis
Using a correlation analysis, you can summarize the relationship between variables into a correlation coefficient : a single number that describes the strength and direction of the relationship between variables. With this number, you’ll quantify the degree of the relationship between variables.
The Pearson product-moment correlation coefficient , also known as Pearson’s r , is commonly used for assessing a linear relationship between two quantitative variables.
Correlation coefficients are usually found for two variables at a time, but you can use a multiple correlation coefficient for three or more variables.
Regression analysis
With a regression analysis , you can predict how much a change in one variable will be associated with a change in the other variable. The result is a regression equation that describes the line on a graph of your variables.
You can use this equation to predict the value of one variable based on the given value(s) of the other variable(s). It’s best to perform a regression analysis after testing for a correlation between your variables.
Here's why students love Scribbr's proofreading services
Discover proofreading & editing
It’s important to remember that correlation does not imply causation . Just because you find a correlation between two things doesn’t mean you can conclude one of them causes the other for a few reasons.
Directionality problem
If two variables are correlated, it could be because one of them is a cause and the other is an effect. But the correlational research design doesn’t allow you to infer which is which. To err on the side of caution, researchers don’t conclude causality from correlational studies.
Third variable problem
A confounding variable is a third variable that influences other variables to make them seem causally related even though they are not. Instead, there are separate causal links between the confounder and each variable.
In correlational research, there’s limited or no researcher control over extraneous variables . Even if you statistically control for some potential confounders, there may still be other hidden variables that disguise the relationship between your study variables.
Although a correlational study can’t demonstrate causation on its own, it can help you develop a causal hypothesis that’s tested in controlled experiments.
If you want to know more about statistics , methodology , or research bias , make sure to check out some of our other articles with explanations and examples.
- Normal distribution
- Degrees of freedom
- Null hypothesis
- Discourse analysis
- Control groups
- Mixed methods research
- Non-probability sampling
- Quantitative research
- Ecological validity
Research bias
- Rosenthal effect
- Implicit bias
- Cognitive bias
- Selection bias
- Negativity bias
- Status quo bias
A correlation reflects the strength and/or direction of the association between two or more variables.
- A positive correlation means that both variables change in the same direction.
- A negative correlation means that the variables change in opposite directions.
- A zero correlation means there’s no relationship between the variables.
A correlational research design investigates relationships between two variables (or more) without the researcher controlling or manipulating any of them. It’s a non-experimental type of quantitative research .
Controlled experiments establish causality, whereas correlational studies only show associations between variables.
- In an experimental design , you manipulate an independent variable and measure its effect on a dependent variable. Other variables are controlled so they can’t impact the results.
- In a correlational design , you measure variables without manipulating any of them. You can test whether your variables change together, but you can’t be sure that one variable caused a change in another.
In general, correlational research is high in external validity while experimental research is high in internal validity .
A correlation is usually tested for two variables at a time, but you can test correlations between three or more variables.
A correlation coefficient is a single number that describes the strength and direction of the relationship between your variables.
Different types of correlation coefficients might be appropriate for your data based on their levels of measurement and distributions . The Pearson product-moment correlation coefficient (Pearson’s r ) is commonly used to assess a linear relationship between two quantitative variables.
Cite this Scribbr article
If you want to cite this source, you can copy and paste the citation or click the “Cite this Scribbr article” button to automatically add the citation to our free Citation Generator.
Bhandari, P. (2023, June 22). Correlational Research | When & How to Use. Scribbr. Retrieved June 18, 2024, from https://www.scribbr.com/methodology/correlational-research/
Is this article helpful?
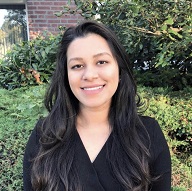
Pritha Bhandari
Other students also liked, what is quantitative research | definition, uses & methods, correlation vs. causation | difference, designs & examples, correlation coefficient | types, formulas & examples, what is your plagiarism score.
Have a language expert improve your writing
Run a free plagiarism check in 10 minutes, automatically generate references for free.
- Knowledge Base
- Methodology
- Correlational Research | Guide, Design & Examples
Correlational Research | Guide, Design & Examples
Published on 5 May 2022 by Pritha Bhandari . Revised on 5 December 2022.
A correlational research design investigates relationships between variables without the researcher controlling or manipulating any of them.
A correlation reflects the strength and/or direction of the relationship between two (or more) variables. The direction of a correlation can be either positive or negative.
Positive correlation | Both variables change in the same direction | As height increases, weight also increases |
---|---|---|
Negative correlation | The variables change in opposite directions | As coffee consumption increases, tiredness decreases |
Zero correlation | There is no relationship between the variables | Coffee consumption is not correlated with height |
Table of contents
Correlational vs experimental research, when to use correlational research, how to collect correlational data, how to analyse correlational data, correlation and causation, frequently asked questions about correlational research.
Correlational and experimental research both use quantitative methods to investigate relationships between variables. But there are important differences in how data is collected and the types of conclusions you can draw.
Correlational research | Experimental research | |
---|---|---|
Purpose | Used to test strength of association between variables | Used to test cause-and-effect relationships between variables |
Variables | Variables are only observed with no manipulation or intervention by researchers | An is manipulated and a dependent variable is observed |
Control | Limited is used, so other variables may play a role in the relationship | are controlled so that they can’t impact your variables of interest |
Validity | High : you can confidently generalise your conclusions to other populations or settings | High : you can confidently draw conclusions about causation |
Prevent plagiarism, run a free check.
Correlational research is ideal for gathering data quickly from natural settings. That helps you generalise your findings to real-life situations in an externally valid way.
There are a few situations where correlational research is an appropriate choice.
To investigate non-causal relationships
You want to find out if there is an association between two variables, but you don’t expect to find a causal relationship between them.
Correlational research can provide insights into complex real-world relationships, helping researchers develop theories and make predictions.
To explore causal relationships between variables
You think there is a causal relationship between two variables, but it is impractical, unethical, or too costly to conduct experimental research that manipulates one of the variables.
Correlational research can provide initial indications or additional support for theories about causal relationships.
To test new measurement tools
You have developed a new instrument for measuring your variable, and you need to test its reliability or validity .
Correlational research can be used to assess whether a tool consistently or accurately captures the concept it aims to measure.
There are many different methods you can use in correlational research. In the social and behavioural sciences, the most common data collection methods for this type of research include surveys, observations, and secondary data.
It’s important to carefully choose and plan your methods to ensure the reliability and validity of your results. You should carefully select a representative sample so that your data reflects the population you’re interested in without bias .
In survey research , you can use questionnaires to measure your variables of interest. You can conduct surveys online, by post, by phone, or in person.
Surveys are a quick, flexible way to collect standardised data from many participants, but it’s important to ensure that your questions are worded in an unbiased way and capture relevant insights.
Naturalistic observation
Naturalistic observation is a type of field research where you gather data about a behaviour or phenomenon in its natural environment.
This method often involves recording, counting, describing, and categorising actions and events. Naturalistic observation can include both qualitative and quantitative elements, but to assess correlation, you collect data that can be analysed quantitatively (e.g., frequencies, durations, scales, and amounts).
Naturalistic observation lets you easily generalise your results to real-world contexts, and you can study experiences that aren’t replicable in lab settings. But data analysis can be time-consuming and unpredictable, and researcher bias may skew the interpretations.
Secondary data
Instead of collecting original data, you can also use data that has already been collected for a different purpose, such as official records, polls, or previous studies.
Using secondary data is inexpensive and fast, because data collection is complete. However, the data may be unreliable, incomplete, or not entirely relevant, and you have no control over the reliability or validity of the data collection procedures.
After collecting data, you can statistically analyse the relationship between variables using correlation or regression analyses, or both. You can also visualise the relationships between variables with a scatterplot.
Different types of correlation coefficients and regression analyses are appropriate for your data based on their levels of measurement and distributions .
Correlation analysis
Using a correlation analysis, you can summarise the relationship between variables into a correlation coefficient : a single number that describes the strength and direction of the relationship between variables. With this number, you’ll quantify the degree of the relationship between variables.
The Pearson product-moment correlation coefficient, also known as Pearson’s r , is commonly used for assessing a linear relationship between two quantitative variables.
Correlation coefficients are usually found for two variables at a time, but you can use a multiple correlation coefficient for three or more variables.
Regression analysis
With a regression analysis , you can predict how much a change in one variable will be associated with a change in the other variable. The result is a regression equation that describes the line on a graph of your variables.
You can use this equation to predict the value of one variable based on the given value(s) of the other variable(s). It’s best to perform a regression analysis after testing for a correlation between your variables.
It’s important to remember that correlation does not imply causation . Just because you find a correlation between two things doesn’t mean you can conclude one of them causes the other, for a few reasons.
Directionality problem
If two variables are correlated, it could be because one of them is a cause and the other is an effect. But the correlational research design doesn’t allow you to infer which is which. To err on the side of caution, researchers don’t conclude causality from correlational studies.
Third variable problem
A confounding variable is a third variable that influences other variables to make them seem causally related even though they are not. Instead, there are separate causal links between the confounder and each variable.
In correlational research, there’s limited or no researcher control over extraneous variables . Even if you statistically control for some potential confounders, there may still be other hidden variables that disguise the relationship between your study variables.
Although a correlational study can’t demonstrate causation on its own, it can help you develop a causal hypothesis that’s tested in controlled experiments.
A correlation reflects the strength and/or direction of the association between two or more variables.
- A positive correlation means that both variables change in the same direction.
- A negative correlation means that the variables change in opposite directions.
- A zero correlation means there’s no relationship between the variables.
A correlational research design investigates relationships between two variables (or more) without the researcher controlling or manipulating any of them. It’s a non-experimental type of quantitative research .
Controlled experiments establish causality, whereas correlational studies only show associations between variables.
- In an experimental design , you manipulate an independent variable and measure its effect on a dependent variable. Other variables are controlled so they can’t impact the results.
- In a correlational design , you measure variables without manipulating any of them. You can test whether your variables change together, but you can’t be sure that one variable caused a change in another.
In general, correlational research is high in external validity while experimental research is high in internal validity .
A correlation is usually tested for two variables at a time, but you can test correlations between three or more variables.
A correlation coefficient is a single number that describes the strength and direction of the relationship between your variables.
Different types of correlation coefficients might be appropriate for your data based on their levels of measurement and distributions . The Pearson product-moment correlation coefficient (Pearson’s r ) is commonly used to assess a linear relationship between two quantitative variables.
Cite this Scribbr article
If you want to cite this source, you can copy and paste the citation or click the ‘Cite this Scribbr article’ button to automatically add the citation to our free Reference Generator.
Bhandari, P. (2022, December 05). Correlational Research | Guide, Design & Examples. Scribbr. Retrieved 18 June 2024, from https://www.scribbr.co.uk/research-methods/correlational-research-design/
Is this article helpful?
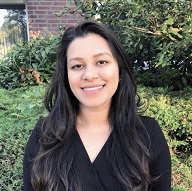
Pritha Bhandari
Other students also liked, a quick guide to experimental design | 5 steps & examples, quasi-experimental design | definition, types & examples, qualitative vs quantitative research | examples & methods.
- Privacy Policy

Home » Correlational Research – Methods, Types and Examples
Correlational Research – Methods, Types and Examples
Table of Contents

Correlational Research
Correlational Research is a type of research that examines the statistical relationship between two or more variables without manipulating them. It is a non-experimental research design that seeks to establish the degree of association or correlation between two or more variables.
Types of Correlational Research
There are three types of correlational research:
Positive Correlation
A positive correlation occurs when two variables increase or decrease together. This means that as one variable increases, the other variable also tends to increase. Similarly, as one variable decreases, the other variable also tends to decrease. For example, there is a positive correlation between the amount of time spent studying and academic performance. The more time a student spends studying, the higher their academic performance is likely to be. Similarly, there is a positive correlation between a person’s age and their income level. As a person gets older, they tend to earn more money.
Negative Correlation
A negative correlation occurs when one variable increases while the other decreases. This means that as one variable increases, the other variable tends to decrease. Similarly, as one variable decreases, the other variable tends to increase. For example, there is a negative correlation between the number of hours spent watching TV and physical activity level. The more time a person spends watching TV, the less physically active they are likely to be. Similarly, there is a negative correlation between the amount of stress a person experiences and their overall happiness. As stress levels increase, happiness levels tend to decrease.
Zero Correlation
A zero correlation occurs when there is no relationship between two variables. This means that the variables are unrelated and do not affect each other. For example, there is zero correlation between a person’s shoe size and their IQ score. The size of a person’s feet has no relationship to their level of intelligence. Similarly, there is zero correlation between a person’s height and their favorite color. The two variables are unrelated to each other.
Correlational Research Methods
Correlational research can be conducted using different methods, including:
Surveys are a common method used in correlational research. Researchers collect data by asking participants to complete questionnaires or surveys that measure different variables of interest. Surveys are useful for exploring the relationships between variables such as personality traits, attitudes, and behaviors.
Observational Studies
Observational studies involve observing and recording the behavior of participants in natural settings. Researchers can use observational studies to examine the relationships between variables such as social interactions, group dynamics, and communication patterns.
Archival Data
Archival data involves using existing data sources such as historical records, census data, or medical records to explore the relationships between variables. Archival data is useful for investigating the relationships between variables that cannot be manipulated or controlled.
Experimental Design
While correlational research does not involve manipulating variables, researchers can use experimental design to establish cause-and-effect relationships between variables. Experimental design involves manipulating one variable while holding other variables constant to determine the effect on the dependent variable.
Meta-Analysis
Meta-analysis involves combining and analyzing the results of multiple studies to explore the relationships between variables across different contexts and populations. Meta-analysis is useful for identifying patterns and inconsistencies in the literature and can provide insights into the strength and direction of relationships between variables.
Data Analysis Methods
Correlational research data analysis methods depend on the type of data collected and the research questions being investigated. Here are some common data analysis methods used in correlational research:
Correlation Coefficient
A correlation coefficient is a statistical measure that quantifies the strength and direction of the relationship between two variables. The correlation coefficient ranges from -1 to +1, with -1 indicating a perfect negative correlation, +1 indicating a perfect positive correlation, and 0 indicating no correlation. Researchers use correlation coefficients to determine the degree to which two variables are related.
Scatterplots
A scatterplot is a graphical representation of the relationship between two variables. Each data point on the plot represents a single observation. The x-axis represents one variable, and the y-axis represents the other variable. The pattern of data points on the plot can provide insights into the strength and direction of the relationship between the two variables.
Regression Analysis
Regression analysis is a statistical method used to model the relationship between two or more variables. Researchers use regression analysis to predict the value of one variable based on the value of another variable. Regression analysis can help identify the strength and direction of the relationship between variables, as well as the degree to which one variable can be used to predict the other.
Factor Analysis
Factor analysis is a statistical method used to identify patterns among variables. Researchers use factor analysis to group variables into factors that are related to each other. Factor analysis can help identify underlying factors that influence the relationship between two variables.
Path Analysis
Path analysis is a statistical method used to model the relationship between multiple variables. Researchers use path analysis to test causal models and identify direct and indirect effects between variables.
Applications of Correlational Research
Correlational research has many practical applications in various fields, including:
- Psychology : Correlational research is commonly used in psychology to explore the relationships between variables such as personality traits, behaviors, and mental health outcomes. For example, researchers may use correlational research to examine the relationship between anxiety and depression, or the relationship between self-esteem and academic achievement.
- Education : Correlational research is useful in educational research to explore the relationships between variables such as teaching methods, student motivation, and academic performance. For example, researchers may use correlational research to examine the relationship between student engagement and academic success, or the relationship between teacher feedback and student learning outcomes.
- Business : Correlational research can be used in business to explore the relationships between variables such as consumer behavior, marketing strategies, and sales outcomes. For example, marketers may use correlational research to examine the relationship between advertising spending and sales revenue, or the relationship between customer satisfaction and brand loyalty.
- Medicine : Correlational research is useful in medical research to explore the relationships between variables such as risk factors, disease outcomes, and treatment effectiveness. For example, researchers may use correlational research to examine the relationship between smoking and lung cancer, or the relationship between exercise and heart health.
- Social Science : Correlational research is commonly used in social science research to explore the relationships between variables such as socioeconomic status, cultural factors, and social behavior. For example, researchers may use correlational research to examine the relationship between income and voting behavior, or the relationship between cultural values and attitudes towards immigration.
Examples of Correlational Research
- Psychology : Researchers might be interested in exploring the relationship between two variables, such as parental attachment and anxiety levels in young adults. The study could involve measuring levels of attachment and anxiety using established scales or questionnaires, and then analyzing the data to determine if there is a correlation between the two variables. This information could be useful in identifying potential risk factors for anxiety in young adults, and in developing interventions that could help improve attachment and reduce anxiety.
- Education : In a correlational study in education, researchers might investigate the relationship between two variables, such as teacher engagement and student motivation in a classroom setting. The study could involve measuring levels of teacher engagement and student motivation using established scales or questionnaires, and then analyzing the data to determine if there is a correlation between the two variables. This information could be useful in identifying strategies that teachers could use to improve student motivation and engagement in the classroom.
- Business : Researchers might explore the relationship between two variables, such as employee satisfaction and productivity levels in a company. The study could involve measuring levels of employee satisfaction and productivity using established scales or questionnaires, and then analyzing the data to determine if there is a correlation between the two variables. This information could be useful in identifying factors that could help increase productivity and improve job satisfaction among employees.
- Medicine : Researchers might examine the relationship between two variables, such as smoking and the risk of developing lung cancer. The study could involve collecting data on smoking habits and lung cancer diagnoses, and then analyzing the data to determine if there is a correlation between the two variables. This information could be useful in identifying risk factors for lung cancer and in developing interventions that could help reduce smoking rates.
- Sociology : Researchers might investigate the relationship between two variables, such as income levels and political attitudes. The study could involve measuring income levels and political attitudes using established scales or questionnaires, and then analyzing the data to determine if there is a correlation between the two variables. This information could be useful in understanding how socioeconomic factors can influence political beliefs and attitudes.
How to Conduct Correlational Research
Here are the general steps to conduct correlational research:
- Identify the Research Question : Start by identifying the research question that you want to explore. It should involve two or more variables that you want to investigate for a correlation.
- Choose the research method: Decide on the research method that will be most appropriate for your research question. The most common methods for correlational research are surveys, archival research, and naturalistic observation.
- Choose the Sample: Select the participants or data sources that you will use in your study. Your sample should be representative of the population you want to generalize the results to.
- Measure the variables: Choose the measures that will be used to assess the variables of interest. Ensure that the measures are reliable and valid.
- Collect the Data: Collect the data from your sample using the chosen research method. Be sure to maintain ethical standards and obtain informed consent from your participants.
- Analyze the data: Use statistical software to analyze the data and compute the correlation coefficient. This will help you determine the strength and direction of the correlation between the variables.
- Interpret the results: Interpret the results and draw conclusions based on the findings. Consider any limitations or alternative explanations for the results.
- Report the findings: Report the findings of your study in a research report or manuscript. Be sure to include the research question, methods, results, and conclusions.
Purpose of Correlational Research
The purpose of correlational research is to examine the relationship between two or more variables. Correlational research allows researchers to identify whether there is a relationship between variables, and if so, the strength and direction of that relationship. This information can be useful for predicting and explaining behavior, and for identifying potential risk factors or areas for intervention.
Correlational research can be used in a variety of fields, including psychology, education, medicine, business, and sociology. For example, in psychology, correlational research can be used to explore the relationship between personality traits and behavior, or between early life experiences and later mental health outcomes. In education, correlational research can be used to examine the relationship between teaching practices and student achievement. In medicine, correlational research can be used to investigate the relationship between lifestyle factors and disease outcomes.
Overall, the purpose of correlational research is to provide insight into the relationship between variables, which can be used to inform further research, interventions, or policy decisions.
When to use Correlational Research
Here are some situations when correlational research can be particularly useful:
- When experimental research is not possible or ethical: In some situations, it may not be possible or ethical to manipulate variables in an experimental design. In these cases, correlational research can be used to explore the relationship between variables without manipulating them.
- When exploring new areas of research: Correlational research can be useful when exploring new areas of research or when researchers are unsure of the direction of the relationship between variables. Correlational research can help identify potential areas for further investigation.
- When testing theories: Correlational research can be useful for testing theories about the relationship between variables. Researchers can use correlational research to examine the relationship between variables predicted by a theory, and to determine whether the theory is supported by the data.
- When making predictions: Correlational research can be used to make predictions about future behavior or outcomes. For example, if there is a strong positive correlation between education level and income, one could predict that individuals with higher levels of education will have higher incomes.
- When identifying risk factors: Correlational research can be useful for identifying potential risk factors for negative outcomes. For example, a study might find a positive correlation between drug use and depression, indicating that drug use could be a risk factor for depression.
Characteristics of Correlational Research
Here are some common characteristics of correlational research:
- Examines the relationship between two or more variables: Correlational research is designed to examine the relationship between two or more variables. It seeks to determine if there is a relationship between the variables, and if so, the strength and direction of that relationship.
- Non-experimental design: Correlational research is typically non-experimental in design, meaning that the researcher does not manipulate any variables. Instead, the researcher observes and measures the variables as they naturally occur.
- Cannot establish causation : Correlational research cannot establish causation, meaning that it cannot determine whether one variable causes changes in another variable. Instead, it only provides information about the relationship between the variables.
- Uses statistical analysis: Correlational research relies on statistical analysis to determine the strength and direction of the relationship between variables. This may include calculating correlation coefficients, regression analysis, or other statistical tests.
- Observes real-world phenomena : Correlational research is often used to observe real-world phenomena, such as the relationship between education and income or the relationship between stress and physical health.
- Can be conducted in a variety of fields : Correlational research can be conducted in a variety of fields, including psychology, sociology, education, and medicine.
- Can be conducted using different methods: Correlational research can be conducted using a variety of methods, including surveys, observational studies, and archival studies.
Advantages of Correlational Research
There are several advantages of using correlational research in a study:
- Allows for the exploration of relationships: Correlational research allows researchers to explore the relationships between variables in a natural setting without manipulating any variables. This can help identify possible relationships between variables that may not have been previously considered.
- Useful for predicting behavior: Correlational research can be useful for predicting future behavior. If a strong correlation is found between two variables, researchers can use this information to predict how changes in one variable may affect the other.
- Can be conducted in real-world settings: Correlational research can be conducted in real-world settings, which allows for the collection of data that is representative of real-world phenomena.
- Can be less expensive and time-consuming than experimental research: Correlational research is often less expensive and time-consuming than experimental research, as it does not involve manipulating variables or creating controlled conditions.
- Useful in identifying risk factors: Correlational research can be used to identify potential risk factors for negative outcomes. By identifying variables that are correlated with negative outcomes, researchers can develop interventions or policies to reduce the risk of negative outcomes.
- Useful in exploring new areas of research: Correlational research can be useful in exploring new areas of research, particularly when researchers are unsure of the direction of the relationship between variables. By conducting correlational research, researchers can identify potential areas for further investigation.
Limitation of Correlational Research
Correlational research also has several limitations that should be taken into account:
- Cannot establish causation: Correlational research cannot establish causation, meaning that it cannot determine whether one variable causes changes in another variable. This is because it is not possible to control all possible confounding variables that could affect the relationship between the variables being studied.
- Directionality problem: The directionality problem refers to the difficulty of determining which variable is influencing the other. For example, a correlation may exist between happiness and social support, but it is not clear whether social support causes happiness, or whether happy people are more likely to have social support.
- Third variable problem: The third variable problem refers to the possibility that a third variable, not included in the study, is responsible for the observed relationship between the two variables being studied.
- Limited generalizability: Correlational research is often limited in terms of its generalizability to other populations or settings. This is because the sample studied may not be representative of the larger population, or because the variables studied may behave differently in different contexts.
- Relies on self-reported data: Correlational research often relies on self-reported data, which can be subject to social desirability bias or other forms of response bias.
- Limited in explaining complex behaviors: Correlational research is limited in explaining complex behaviors that are influenced by multiple factors, such as personality traits, situational factors, and social context.
About the author
Muhammad Hassan
Researcher, Academic Writer, Web developer
You may also like

Survey Research – Types, Methods, Examples

One-to-One Interview – Methods and Guide

Transformative Design – Methods, Types, Guide

Exploratory Research – Types, Methods and...

Phenomenology – Methods, Examples and Guide

Research Methods – Types, Examples and Guide
6.2 Correlational Research
Learning objectives.
- Define correlational research and give several examples.
- Explain why a researcher might choose to conduct correlational research rather than experimental research or another type of non-experimental research.
- Interpret the strength and direction of different correlation coefficients.
- Explain why correlation does not imply causation.
What Is Correlational Research?
Correlational research is a type of non-experimental research in which the researcher measures two variables and assesses the statistical relationship (i.e., the correlation) between them with little or no effort to control extraneous variables. There are many reasons that researchers interested in statistical relationships between variables would choose to conduct a correlational study rather than an experiment. The first is that they do not believe that the statistical relationship is a causal one or are not interested in causal relationships. Recall two goals of science are to describe and to predict and the correlational research strategy allows researchers to achieve both of these goals. Specifically, this strategy can be used to describe the strength and direction of the relationship between two variables and if there is a relationship between the variables then the researchers can use scores on one variable to predict scores on the other (using a statistical technique called regression).
Another reason that researchers would choose to use a correlational study rather than an experiment is that the statistical relationship of interest is thought to be causal, but the researcher cannot manipulate the independent variable because it is impossible, impractical, or unethical. For example, while I might be interested in the relationship between the frequency people use cannabis and their memory abilities I cannot ethically manipulate the frequency that people use cannabis. As such, I must rely on the correlational research strategy; I must simply measure the frequency that people use cannabis and measure their memory abilities using a standardized test of memory and then determine whether the frequency people use cannabis use is statistically related to memory test performance.
Correlation is also used to establish the reliability and validity of measurements. For example, a researcher might evaluate the validity of a brief extraversion test by administering it to a large group of participants along with a longer extraversion test that has already been shown to be valid. This researcher might then check to see whether participants’ scores on the brief test are strongly correlated with their scores on the longer one. Neither test score is thought to cause the other, so there is no independent variable to manipulate. In fact, the terms independent variable and dependent variabl e do not apply to this kind of research.
Another strength of correlational research is that it is often higher in external validity than experimental research. Recall there is typically a trade-off between internal validity and external validity. As greater controls are added to experiments, internal validity is increased but often at the expense of external validity. In contrast, correlational studies typically have low internal validity because nothing is manipulated or control but they often have high external validity. Since nothing is manipulated or controlled by the experimenter the results are more likely to reflect relationships that exist in the real world.
Finally, extending upon this trade-off between internal and external validity, correlational research can help to provide converging evidence for a theory. If a theory is supported by a true experiment that is high in internal validity as well as by a correlational study that is high in external validity then the researchers can have more confidence in the validity of their theory. As a concrete example, correlational studies establishing that there is a relationship between watching violent television and aggressive behavior have been complemented by experimental studies confirming that the relationship is a causal one (Bushman & Huesmann, 2001) [1] . These converging results provide strong evidence that there is a real relationship (indeed a causal relationship) between watching violent television and aggressive behavior.
Data Collection in Correlational Research
Again, the defining feature of correlational research is that neither variable is manipulated. It does not matter how or where the variables are measured. A researcher could have participants come to a laboratory to complete a computerized backward digit span task and a computerized risky decision-making task and then assess the relationship between participants’ scores on the two tasks. Or a researcher could go to a shopping mall to ask people about their attitudes toward the environment and their shopping habits and then assess the relationship between these two variables. Both of these studies would be correlational because no independent variable is manipulated.
Correlations Between Quantitative Variables
Correlations between quantitative variables are often presented using scatterplots . Figure 6.3 shows some hypothetical data on the relationship between the amount of stress people are under and the number of physical symptoms they have. Each point in the scatterplot represents one person’s score on both variables. For example, the circled point in Figure 6.3 represents a person whose stress score was 10 and who had three physical symptoms. Taking all the points into account, one can see that people under more stress tend to have more physical symptoms. This is a good example of a positive relationship , in which higher scores on one variable tend to be associated with higher scores on the other. A negative relationship is one in which higher scores on one variable tend to be associated with lower scores on the other. There is a negative relationship between stress and immune system functioning, for example, because higher stress is associated with lower immune system functioning.

Figure 6.3 Scatterplot Showing a Hypothetical Positive Relationship Between Stress and Number of Physical Symptoms. The circled point represents a person whose stress score was 10 and who had three physical symptoms. Pearson’s r for these data is +.51.
The strength of a correlation between quantitative variables is typically measured using a statistic called Pearson’s Correlation Coefficient (or Pearson’s r ) . As Figure 6.4 shows, Pearson’s r ranges from −1.00 (the strongest possible negative relationship) to +1.00 (the strongest possible positive relationship). A value of 0 means there is no relationship between the two variables. When Pearson’s r is 0, the points on a scatterplot form a shapeless “cloud.” As its value moves toward −1.00 or +1.00, the points come closer and closer to falling on a single straight line. Correlation coefficients near ±.10 are considered small, values near ± .30 are considered medium, and values near ±.50 are considered large. Notice that the sign of Pearson’s r is unrelated to its strength. Pearson’s r values of +.30 and −.30, for example, are equally strong; it is just that one represents a moderate positive relationship and the other a moderate negative relationship. With the exception of reliability coefficients, most correlations that we find in Psychology are small or moderate in size. The website http://rpsychologist.com/d3/correlation/ , created by Kristoffer Magnusson, provides an excellent interactive visualization of correlations that permits you to adjust the strength and direction of a correlation while witnessing the corresponding changes to the scatterplot.

Figure 6.4 Range of Pearson’s r, From −1.00 (Strongest Possible Negative Relationship), Through 0 (No Relationship), to +1.00 (Strongest Possible Positive Relationship)
There are two common situations in which the value of Pearson’s r can be misleading. Pearson’s r is a good measure only for linear relationships, in which the points are best approximated by a straight line. It is not a good measure for nonlinear relationships, in which the points are better approximated by a curved line. Figure 6.5, for example, shows a hypothetical relationship between the amount of sleep people get per night and their level of depression. In this example, the line that best approximates the points is a curve—a kind of upside-down “U”—because people who get about eight hours of sleep tend to be the least depressed. Those who get too little sleep and those who get too much sleep tend to be more depressed. Even though Figure 6.5 shows a fairly strong relationship between depression and sleep, Pearson’s r would be close to zero because the points in the scatterplot are not well fit by a single straight line. This means that it is important to make a scatterplot and confirm that a relationship is approximately linear before using Pearson’s r . Nonlinear relationships are fairly common in psychology, but measuring their strength is beyond the scope of this book.

Figure 6.5 Hypothetical Nonlinear Relationship Between Sleep and Depression
The other common situations in which the value of Pearson’s r can be misleading is when one or both of the variables have a limited range in the sample relative to the population. This problem is referred to as restriction of range . Assume, for example, that there is a strong negative correlation between people’s age and their enjoyment of hip hop music as shown by the scatterplot in Figure 6.6. Pearson’s r here is −.77. However, if we were to collect data only from 18- to 24-year-olds—represented by the shaded area of Figure 6.6—then the relationship would seem to be quite weak. In fact, Pearson’s r for this restricted range of ages is 0. It is a good idea, therefore, to design studies to avoid restriction of range. For example, if age is one of your primary variables, then you can plan to collect data from people of a wide range of ages. Because restriction of range is not always anticipated or easily avoidable, however, it is good practice to examine your data for possible restriction of range and to interpret Pearson’s r in light of it. (There are also statistical methods to correct Pearson’s r for restriction of range, but they are beyond the scope of this book).

Figure 6.6 Hypothetical Data Showing How a Strong Overall Correlation Can Appear to Be Weak When One Variable Has a Restricted Range.The overall correlation here is −.77, but the correlation for the 18- to 24-year-olds (in the blue box) is 0.
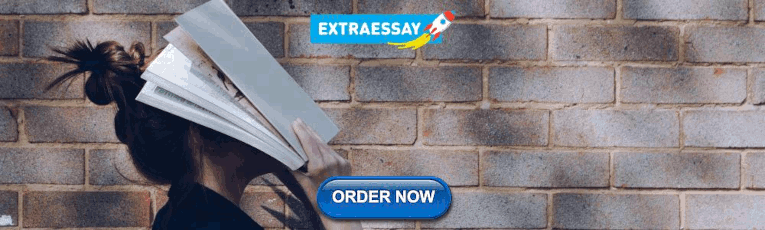
Correlation Does Not Imply Causation
You have probably heard repeatedly that “Correlation does not imply causation.” An amusing example of this comes from a 2012 study that showed a positive correlation (Pearson’s r = 0.79) between the per capita chocolate consumption of a nation and the number of Nobel prizes awarded to citizens of that nation [2] . It seems clear, however, that this does not mean that eating chocolate causes people to win Nobel prizes, and it would not make sense to try to increase the number of Nobel prizes won by recommending that parents feed their children more chocolate.
There are two reasons that correlation does not imply causation. The first is called the directionality problem . Two variables, X and Y , can be statistically related because X causes Y or because Y causes X . Consider, for example, a study showing that whether or not people exercise is statistically related to how happy they are—such that people who exercise are happier on average than people who do not. This statistical relationship is consistent with the idea that exercising causes happiness, but it is also consistent with the idea that happiness causes exercise. Perhaps being happy gives people more energy or leads them to seek opportunities to socialize with others by going to the gym. The second reason that correlation does not imply causation is called the third-variable problem . Two variables, X and Y , can be statistically related not because X causes Y , or because Y causes X , but because some third variable, Z , causes both X and Y . For example, the fact that nations that have won more Nobel prizes tend to have higher chocolate consumption probably reflects geography in that European countries tend to have higher rates of per capita chocolate consumption and invest more in education and technology (once again, per capita) than many other countries in the world. Similarly, the statistical relationship between exercise and happiness could mean that some third variable, such as physical health, causes both of the others. Being physically healthy could cause people to exercise and cause them to be happier. Correlations that are a result of a third-variable are often referred to as spurious correlations.
Some excellent and funny examples of spurious correlations can be found at http://www.tylervigen.com (Figure 6.7 provides one such example).

“Lots of Candy Could Lead to Violence”
Although researchers in psychology know that correlation does not imply causation, many journalists do not. One website about correlation and causation, http://jonathan.mueller.faculty.noctrl.edu/100/correlation_or_causation.htm , links to dozens of media reports about real biomedical and psychological research. Many of the headlines suggest that a causal relationship has been demonstrated when a careful reading of the articles shows that it has not because of the directionality and third-variable problems.
One such article is about a study showing that children who ate candy every day were more likely than other children to be arrested for a violent offense later in life. But could candy really “lead to” violence, as the headline suggests? What alternative explanations can you think of for this statistical relationship? How could the headline be rewritten so that it is not misleading?
As you have learned by reading this book, there are various ways that researchers address the directionality and third-variable problems. The most effective is to conduct an experiment. For example, instead of simply measuring how much people exercise, a researcher could bring people into a laboratory and randomly assign half of them to run on a treadmill for 15 minutes and the rest to sit on a couch for 15 minutes. Although this seems like a minor change to the research design, it is extremely important. Now if the exercisers end up in more positive moods than those who did not exercise, it cannot be because their moods affected how much they exercised (because it was the researcher who determined how much they exercised). Likewise, it cannot be because some third variable (e.g., physical health) affected both how much they exercised and what mood they were in (because, again, it was the researcher who determined how much they exercised). Thus experiments eliminate the directionality and third-variable problems and allow researchers to draw firm conclusions about causal relationships.
Key Takeaways
- Correlational research involves measuring two variables and assessing the relationship between them, with no manipulation of an independent variable.
- Correlation does not imply causation. A statistical relationship between two variables, X and Y , does not necessarily mean that X causes Y . It is also possible that Y causes X , or that a third variable, Z , causes both X and Y .
- While correlational research cannot be used to establish causal relationships between variables, correlational research does allow researchers to achieve many other important objectives (establishing reliability and validity, providing converging evidence, describing relationships and making predictions)
- Correlation coefficients can range from -1 to +1. The sign indicates the direction of the relationship between the variables and the numerical value indicates the strength of the relationship.
- A cognitive psychologist compares the ability of people to recall words that they were instructed to “read” with their ability to recall words that they were instructed to “imagine.”
- A manager studies the correlation between new employees’ college grade point averages and their first-year performance reports.
- An automotive engineer installs different stick shifts in a new car prototype, each time asking several people to rate how comfortable the stick shift feels.
- A food scientist studies the relationship between the temperature inside people’s refrigerators and the amount of bacteria on their food.
- A social psychologist tells some research participants that they need to hurry over to the next building to complete a study. She tells others that they can take their time. Then she observes whether they stop to help a research assistant who is pretending to be hurt.
2. Practice: For each of the following statistical relationships, decide whether the directionality problem is present and think of at least one plausible third variable.
- People who eat more lobster tend to live longer.
- People who exercise more tend to weigh less.
- College students who drink more alcohol tend to have poorer grades.
- Bushman, B. J., & Huesmann, L. R. (2001). Effects of televised violence on aggression. In D. Singer & J. Singer (Eds.), Handbook of children and the media (pp. 223–254). Thousand Oaks, CA: Sage. ↵
- Messerli, F. H. (2012). Chocolate consumption, cognitive function, and Nobel laureates. New England Journal of Medicine, 367 , 1562-1564. ↵

Share This Book
- Increase Font Size

Want to create or adapt books like this? Learn more about how Pressbooks supports open publishing practices.
7.2 Correlational Research
Learning objectives.
- Define correlational research and give several examples.
- Explain why a researcher might choose to conduct correlational research rather than experimental research or another type of nonexperimental research.
What Is Correlational Research?
Correlational research is a type of nonexperimental research in which the researcher measures two variables and assesses the statistical relationship (i.e., the correlation) between them with little or no effort to control extraneous variables. There are essentially two reasons that researchers interested in statistical relationships between variables would choose to conduct a correlational study rather than an experiment. The first is that they do not believe that the statistical relationship is a causal one. For example, a researcher might evaluate the validity of a brief extraversion test by administering it to a large group of participants along with a longer extraversion test that has already been shown to be valid. This researcher might then check to see whether participants’ scores on the brief test are strongly correlated with their scores on the longer one. Neither test score is thought to cause the other, so there is no independent variable to manipulate. In fact, the terms independent variable and dependent variable do not apply to this kind of research.
The other reason that researchers would choose to use a correlational study rather than an experiment is that the statistical relationship of interest is thought to be causal, but the researcher cannot manipulate the independent variable because it is impossible, impractical, or unethical. For example, Allen Kanner and his colleagues thought that the number of “daily hassles” (e.g., rude salespeople, heavy traffic) that people experience affects the number of physical and psychological symptoms they have (Kanner, Coyne, Schaefer, & Lazarus, 1981). But because they could not manipulate the number of daily hassles their participants experienced, they had to settle for measuring the number of daily hassles—along with the number of symptoms—using self-report questionnaires. Although the strong positive relationship they found between these two variables is consistent with their idea that hassles cause symptoms, it is also consistent with the idea that symptoms cause hassles or that some third variable (e.g., neuroticism) causes both.
A common misconception among beginning researchers is that correlational research must involve two quantitative variables, such as scores on two extraversion tests or the number of hassles and number of symptoms people have experienced. However, the defining feature of correlational research is that the two variables are measured—neither one is manipulated—and this is true regardless of whether the variables are quantitative or categorical. Imagine, for example, that a researcher administers the Rosenberg Self-Esteem Scale to 50 American college students and 50 Japanese college students. Although this “feels” like a between-subjects experiment, it is a correlational study because the researcher did not manipulate the students’ nationalities. The same is true of the study by Cacioppo and Petty comparing college faculty and factory workers in terms of their need for cognition. It is a correlational study because the researchers did not manipulate the participants’ occupations.
Figure 7.2 “Results of a Hypothetical Study on Whether People Who Make Daily To-Do Lists Experience Less Stress Than People Who Do Not Make Such Lists” shows data from a hypothetical study on the relationship between whether people make a daily list of things to do (a “to-do list”) and stress. Notice that it is unclear whether this is an experiment or a correlational study because it is unclear whether the independent variable was manipulated. If the researcher randomly assigned some participants to make daily to-do lists and others not to, then it is an experiment. If the researcher simply asked participants whether they made daily to-do lists, then it is a correlational study. The distinction is important because if the study was an experiment, then it could be concluded that making the daily to-do lists reduced participants’ stress. But if it was a correlational study, it could only be concluded that these variables are statistically related. Perhaps being stressed has a negative effect on people’s ability to plan ahead (the directionality problem). Or perhaps people who are more conscientious are more likely to make to-do lists and less likely to be stressed (the third-variable problem). The crucial point is that what defines a study as experimental or correlational is not the variables being studied, nor whether the variables are quantitative or categorical, nor the type of graph or statistics used to analyze the data. It is how the study is conducted.
Figure 7.2 Results of a Hypothetical Study on Whether People Who Make Daily To-Do Lists Experience Less Stress Than People Who Do Not Make Such Lists

Data Collection in Correlational Research
Again, the defining feature of correlational research is that neither variable is manipulated. It does not matter how or where the variables are measured. A researcher could have participants come to a laboratory to complete a computerized backward digit span task and a computerized risky decision-making task and then assess the relationship between participants’ scores on the two tasks. Or a researcher could go to a shopping mall to ask people about their attitudes toward the environment and their shopping habits and then assess the relationship between these two variables. Both of these studies would be correlational because no independent variable is manipulated. However, because some approaches to data collection are strongly associated with correlational research, it makes sense to discuss them here. The two we will focus on are naturalistic observation and archival data. A third, survey research, is discussed in its own chapter.
Naturalistic Observation
Naturalistic observation is an approach to data collection that involves observing people’s behavior in the environment in which it typically occurs. Thus naturalistic observation is a type of field research (as opposed to a type of laboratory research). It could involve observing shoppers in a grocery store, children on a school playground, or psychiatric inpatients in their wards. Researchers engaged in naturalistic observation usually make their observations as unobtrusively as possible so that participants are often not aware that they are being studied. Ethically, this is considered to be acceptable if the participants remain anonymous and the behavior occurs in a public setting where people would not normally have an expectation of privacy. Grocery shoppers putting items into their shopping carts, for example, are engaged in public behavior that is easily observable by store employees and other shoppers. For this reason, most researchers would consider it ethically acceptable to observe them for a study. On the other hand, one of the arguments against the ethicality of the naturalistic observation of “bathroom behavior” discussed earlier in the book is that people have a reasonable expectation of privacy even in a public restroom and that this expectation was violated.
Researchers Robert Levine and Ara Norenzayan used naturalistic observation to study differences in the “pace of life” across countries (Levine & Norenzayan, 1999). One of their measures involved observing pedestrians in a large city to see how long it took them to walk 60 feet. They found that people in some countries walked reliably faster than people in other countries. For example, people in the United States and Japan covered 60 feet in about 12 seconds on average, while people in Brazil and Romania took close to 17 seconds.
Because naturalistic observation takes place in the complex and even chaotic “real world,” there are two closely related issues that researchers must deal with before collecting data. The first is sampling. When, where, and under what conditions will the observations be made, and who exactly will be observed? Levine and Norenzayan described their sampling process as follows:
Male and female walking speed over a distance of 60 feet was measured in at least two locations in main downtown areas in each city. Measurements were taken during main business hours on clear summer days. All locations were flat, unobstructed, had broad sidewalks, and were sufficiently uncrowded to allow pedestrians to move at potentially maximum speeds. To control for the effects of socializing, only pedestrians walking alone were used. Children, individuals with obvious physical handicaps, and window-shoppers were not timed. Thirty-five men and 35 women were timed in most cities. (p. 186)
Precise specification of the sampling process in this way makes data collection manageable for the observers, and it also provides some control over important extraneous variables. For example, by making their observations on clear summer days in all countries, Levine and Norenzayan controlled for effects of the weather on people’s walking speeds.
The second issue is measurement. What specific behaviors will be observed? In Levine and Norenzayan’s study, measurement was relatively straightforward. They simply measured out a 60-foot distance along a city sidewalk and then used a stopwatch to time participants as they walked over that distance. Often, however, the behaviors of interest are not so obvious or objective. For example, researchers Robert Kraut and Robert Johnston wanted to study bowlers’ reactions to their shots, both when they were facing the pins and then when they turned toward their companions (Kraut & Johnston, 1979). But what “reactions” should they observe? Based on previous research and their own pilot testing, Kraut and Johnston created a list of reactions that included “closed smile,” “open smile,” “laugh,” “neutral face,” “look down,” “look away,” and “face cover” (covering one’s face with one’s hands). The observers committed this list to memory and then practiced by coding the reactions of bowlers who had been videotaped. During the actual study, the observers spoke into an audio recorder, describing the reactions they observed. Among the most interesting results of this study was that bowlers rarely smiled while they still faced the pins. They were much more likely to smile after they turned toward their companions, suggesting that smiling is not purely an expression of happiness but also a form of social communication.
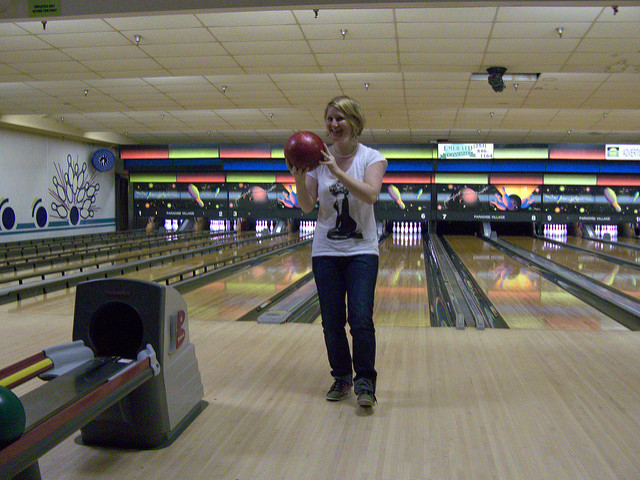
Naturalistic observation has revealed that bowlers tend to smile when they turn away from the pins and toward their companions, suggesting that smiling is not purely an expression of happiness but also a form of social communication.
sieneke toering – bowling big lebowski style – CC BY-NC-ND 2.0.
When the observations require a judgment on the part of the observers—as in Kraut and Johnston’s study—this process is often described as coding . Coding generally requires clearly defining a set of target behaviors. The observers then categorize participants individually in terms of which behavior they have engaged in and the number of times they engaged in each behavior. The observers might even record the duration of each behavior. The target behaviors must be defined in such a way that different observers code them in the same way. This is the issue of interrater reliability. Researchers are expected to demonstrate the interrater reliability of their coding procedure by having multiple raters code the same behaviors independently and then showing that the different observers are in close agreement. Kraut and Johnston, for example, video recorded a subset of their participants’ reactions and had two observers independently code them. The two observers showed that they agreed on the reactions that were exhibited 97% of the time, indicating good interrater reliability.
Archival Data
Another approach to correlational research is the use of archival data , which are data that have already been collected for some other purpose. An example is a study by Brett Pelham and his colleagues on “implicit egotism”—the tendency for people to prefer people, places, and things that are similar to themselves (Pelham, Carvallo, & Jones, 2005). In one study, they examined Social Security records to show that women with the names Virginia, Georgia, Louise, and Florence were especially likely to have moved to the states of Virginia, Georgia, Louisiana, and Florida, respectively.
As with naturalistic observation, measurement can be more or less straightforward when working with archival data. For example, counting the number of people named Virginia who live in various states based on Social Security records is relatively straightforward. But consider a study by Christopher Peterson and his colleagues on the relationship between optimism and health using data that had been collected many years before for a study on adult development (Peterson, Seligman, & Vaillant, 1988). In the 1940s, healthy male college students had completed an open-ended questionnaire about difficult wartime experiences. In the late 1980s, Peterson and his colleagues reviewed the men’s questionnaire responses to obtain a measure of explanatory style—their habitual ways of explaining bad events that happen to them. More pessimistic people tend to blame themselves and expect long-term negative consequences that affect many aspects of their lives, while more optimistic people tend to blame outside forces and expect limited negative consequences. To obtain a measure of explanatory style for each participant, the researchers used a procedure in which all negative events mentioned in the questionnaire responses, and any causal explanations for them, were identified and written on index cards. These were given to a separate group of raters who rated each explanation in terms of three separate dimensions of optimism-pessimism. These ratings were then averaged to produce an explanatory style score for each participant. The researchers then assessed the statistical relationship between the men’s explanatory style as college students and archival measures of their health at approximately 60 years of age. The primary result was that the more optimistic the men were as college students, the healthier they were as older men. Pearson’s r was +.25.
This is an example of content analysis —a family of systematic approaches to measurement using complex archival data. Just as naturalistic observation requires specifying the behaviors of interest and then noting them as they occur, content analysis requires specifying keywords, phrases, or ideas and then finding all occurrences of them in the data. These occurrences can then be counted, timed (e.g., the amount of time devoted to entertainment topics on the nightly news show), or analyzed in a variety of other ways.
Key Takeaways
- Correlational research involves measuring two variables and assessing the relationship between them, with no manipulation of an independent variable.
- Correlational research is not defined by where or how the data are collected. However, some approaches to data collection are strongly associated with correlational research. These include naturalistic observation (in which researchers observe people’s behavior in the context in which it normally occurs) and the use of archival data that were already collected for some other purpose.
Discussion: For each of the following, decide whether it is most likely that the study described is experimental or correlational and explain why.
- An educational researcher compares the academic performance of students from the “rich” side of town with that of students from the “poor” side of town.
- A cognitive psychologist compares the ability of people to recall words that they were instructed to “read” with their ability to recall words that they were instructed to “imagine.”
- A manager studies the correlation between new employees’ college grade point averages and their first-year performance reports.
- An automotive engineer installs different stick shifts in a new car prototype, each time asking several people to rate how comfortable the stick shift feels.
- A food scientist studies the relationship between the temperature inside people’s refrigerators and the amount of bacteria on their food.
- A social psychologist tells some research participants that they need to hurry over to the next building to complete a study. She tells others that they can take their time. Then she observes whether they stop to help a research assistant who is pretending to be hurt.
Kanner, A. D., Coyne, J. C., Schaefer, C., & Lazarus, R. S. (1981). Comparison of two modes of stress measurement: Daily hassles and uplifts versus major life events. Journal of Behavioral Medicine, 4 , 1–39.
Kraut, R. E., & Johnston, R. E. (1979). Social and emotional messages of smiling: An ethological approach. Journal of Personality and Social Psychology, 37 , 1539–1553.
Levine, R. V., & Norenzayan, A. (1999). The pace of life in 31 countries. Journal of Cross-Cultural Psychology, 30 , 178–205.
Pelham, B. W., Carvallo, M., & Jones, J. T. (2005). Implicit egotism. Current Directions in Psychological Science, 14 , 106–110.
Peterson, C., Seligman, M. E. P., & Vaillant, G. E. (1988). Pessimistic explanatory style is a risk factor for physical illness: A thirty-five year longitudinal study. Journal of Personality and Social Psychology, 55 , 23–27.
Research Methods in Psychology Copyright © 2016 by University of Minnesota is licensed under a Creative Commons Attribution-NonCommercial-ShareAlike 4.0 International License , except where otherwise noted.
- Skip to main content
- Skip to primary sidebar
- Skip to footer
- QuestionPro

- Solutions Industries Gaming Automotive Sports and events Education Government Travel & Hospitality Financial Services Healthcare Cannabis Technology Use Case NPS+ Communities Audience Contactless surveys Mobile LivePolls Member Experience GDPR Positive People Science 360 Feedback Surveys
- Resources Blog eBooks Survey Templates Case Studies Training Help center

Home Market Research
Correlational Research: What it is with Examples
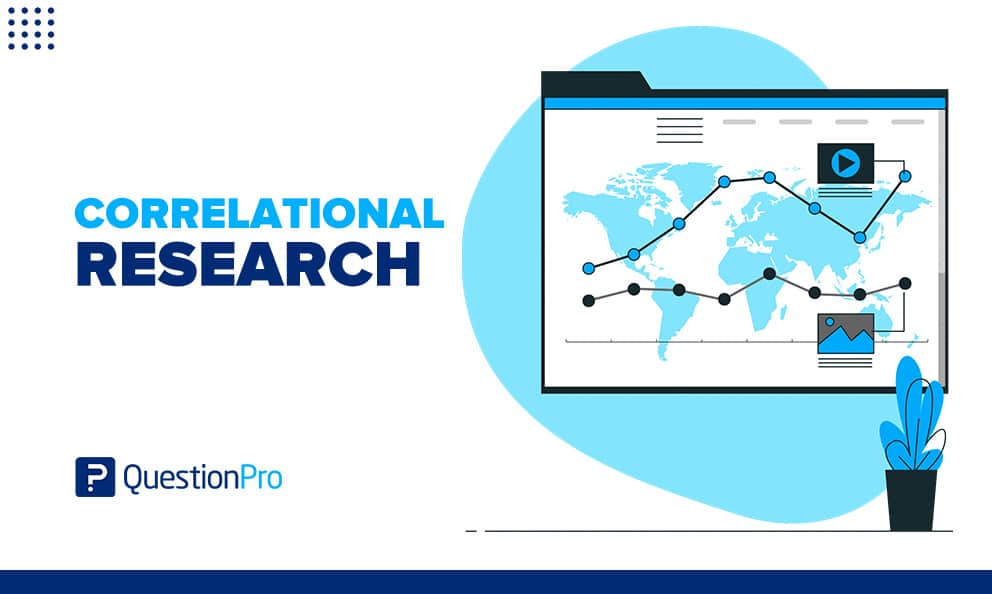
Our minds can do some brilliant things. For example, it can memorize the jingle of a pizza truck. The louder the jingle, the closer the pizza truck is to us. Who taught us that? Nobody! We relied on our understanding and came to a conclusion. We don’t stop there, do we? If there are multiple pizza trucks in the area and each one has a different jingle, we would memorize it all and relate the jingle to its pizza truck.
This is what correlational research precisely is, establishing a relationship between two variables, “jingle” and “distance of the truck” in this particular example. The correlational study looks for variables that seem to interact with each other. When you see one variable changing, you have a fair idea of how the other variable will change.
What is Correlational research?
Correlational research is a type of non-experimental research method in which a researcher measures two variables and understands and assesses the statistical relationship between them with no influence from any extraneous variable. In statistical analysis, distinguishing between categorical data and numerical data is essential, as categorical data involves distinct categories or labels, while numerical data consists of measurable quantities.
Correlational Research Example
The correlation coefficient shows the correlation between two variables (A correlation coefficient is a statistical measure that calculates the strength of the relationship between two variables), a value measured between -1 and +1. When the correlation coefficient is close to +1, there is a positive correlation between the two variables. If the value is relative to -1, there is a negative correlation between the two variables. When the value is close to zero, then there is no relationship between the two variables.
Let us take an example to understand correlational research.
Consider hypothetically, a researcher is studying a correlation between cancer and marriage. In this study, there are two variables: disease and marriage. Let us say marriage has a negative association with cancer. This means that married people are less likely to develop cancer.
However, this doesn’t necessarily mean that marriage directly avoids cancer. In correlational research, it is not possible to establish the fact, what causes what. It is a misconception that a correlational study involves two quantitative variables. However, the reality is two variables are measured, but neither is changed. This is true independent of whether the variables are quantitative or categorical.
Types of correlational research
Mainly three types of correlational research have been identified:
1. Positive correlation: A positive relationship between two variables is when an increase in one variable leads to a rise in the other variable. A decrease in one variable will see a reduction in the other variable. For example, the amount of money a person has might positively correlate with the number of cars the person owns.
2. Negative correlation: A negative correlation is quite literally the opposite of a positive relationship. If there is an increase in one variable, the second variable will show a decrease, and vice versa.
For example, being educated might negatively correlate with the crime rate when an increase in one variable leads to a decrease in another and vice versa. If a country’s education level is improved, it can lower crime rates. Please note that this doesn’t mean that lack of education leads to crimes. It only means that a lack of education and crime is believed to have a common reason – poverty.
3. No correlation: There is no correlation between the two variables in this third type . A change in one variable may not necessarily see a difference in the other variable. For example, being a millionaire and happiness are not correlated. An increase in money doesn’t lead to happiness.
Characteristics of correlational research
Correlational research has three main characteristics. They are:
- Non-experimental : The correlational study is non-experimental. It means that researchers need not manipulate variables with a scientific methodology to either agree or disagree with a hypothesis. The researcher only measures and observes the relationship between the variables without altering them or subjecting them to external conditioning.
- Backward-looking : Correlational research only looks back at historical data and observes events in the past. Researchers use it to measure and spot historical patterns between two variables. A correlational study may show a positive relationship between two variables, but this can change in the future.
- Dynamic : The patterns between two variables from correlational research are never constant and are always changing. Two variables having negative correlation research in the past can have a positive correlation relationship in the future due to various factors.
Data collection
The distinctive feature of correlational research is that the researcher can’t manipulate either of the variables involved. It doesn’t matter how or where the variables are measured. A researcher could observe participants in a closed environment or a public setting.
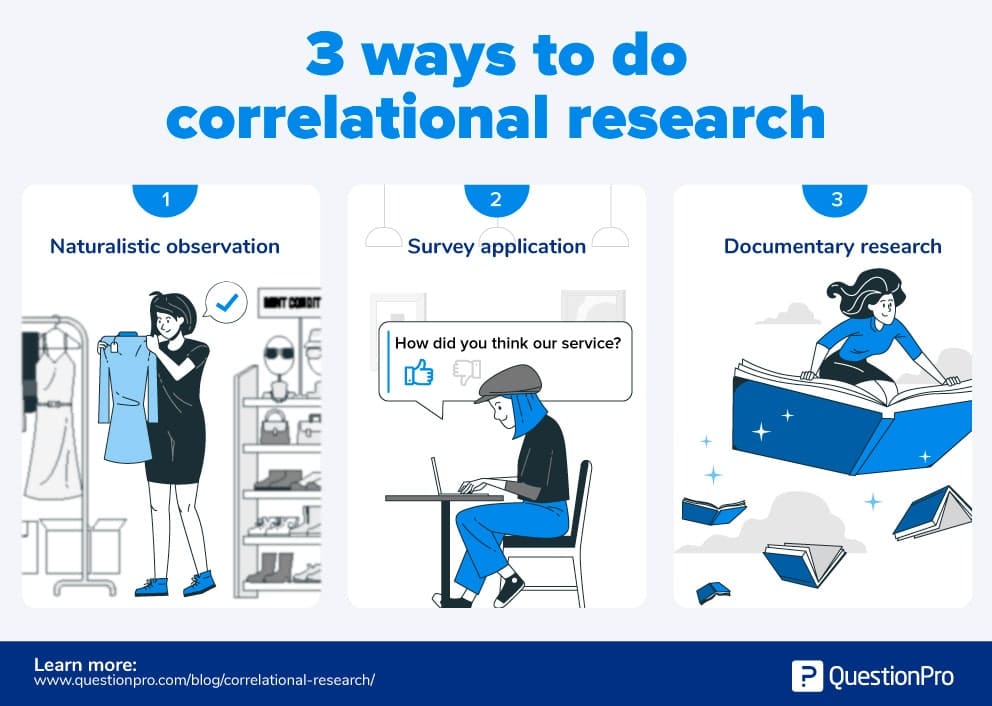
Researchers use two data collection methods to collect information in correlational research.
01. Naturalistic observation
Naturalistic observation is a way of data collection in which people’s behavioral targeting is observed in their natural environment, in which they typically exist. This method is a type of field research. It could mean a researcher might be observing people in a grocery store, at the cinema, playground, or in similar places.
Researchers who are usually involved in this type of data collection make observations as unobtrusively as possible so that the participants involved in the study are not aware that they are being observed else they might deviate from being their natural self.
Ethically this method is acceptable if the participants remain anonymous, and if the study is conducted in a public setting, a place where people would not normally expect complete privacy. As mentioned previously, taking an example of the grocery store where people can be observed while collecting an item from the aisle and putting in the shopping bags. This is ethically acceptable, which is why most researchers choose public settings for recording their observations. This data collection method could be both qualitative and quantitative . If you need to know more about qualitative data, you can explore our newly published blog, “ Examples of Qualitative Data in Education .”
02. Archival data
Another approach to correlational data is the use of archival data. Archival information is the data that has been previously collected by doing similar kinds of research . Archival data is usually made available through primary research .
In contrast to naturalistic observation, the information collected through archived data can be pretty straightforward. For example, counting the number of people named Richard in the various states of America based on social security records is relatively short.
Use the correlational research method to conduct a correlational study and measure the statistical relationship between two variables. Uncover the insights that matter the most. Use QuestionPro’s research platform to uncover complex insights that can propel your business to the forefront of your industry.
Research to make better decisions. Start a free trial today. No credit card required.
LEARN MORE FREE TRIAL
MORE LIKE THIS
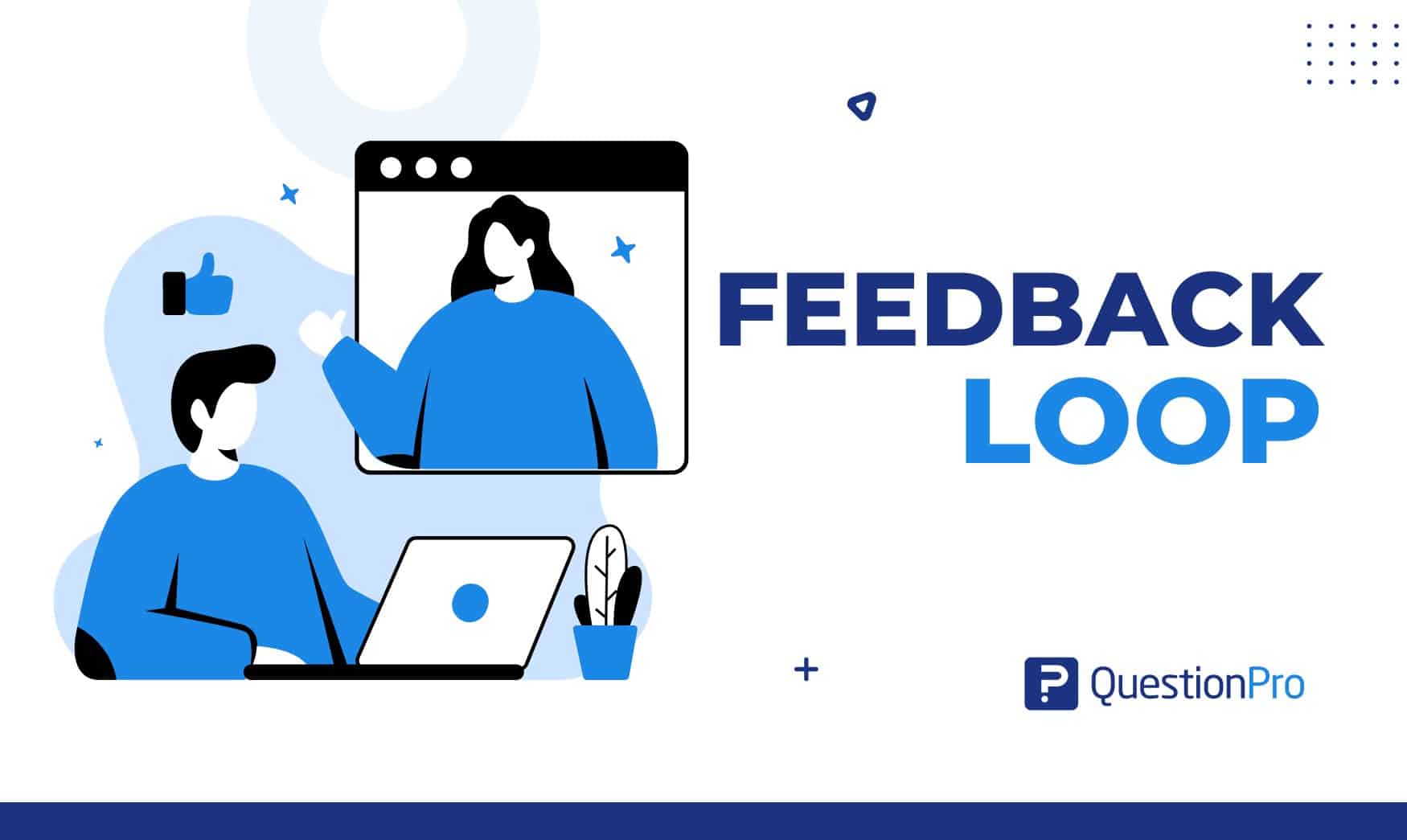
Feedback Loop: What It Is, Types & How It Works?
Jun 21, 2024
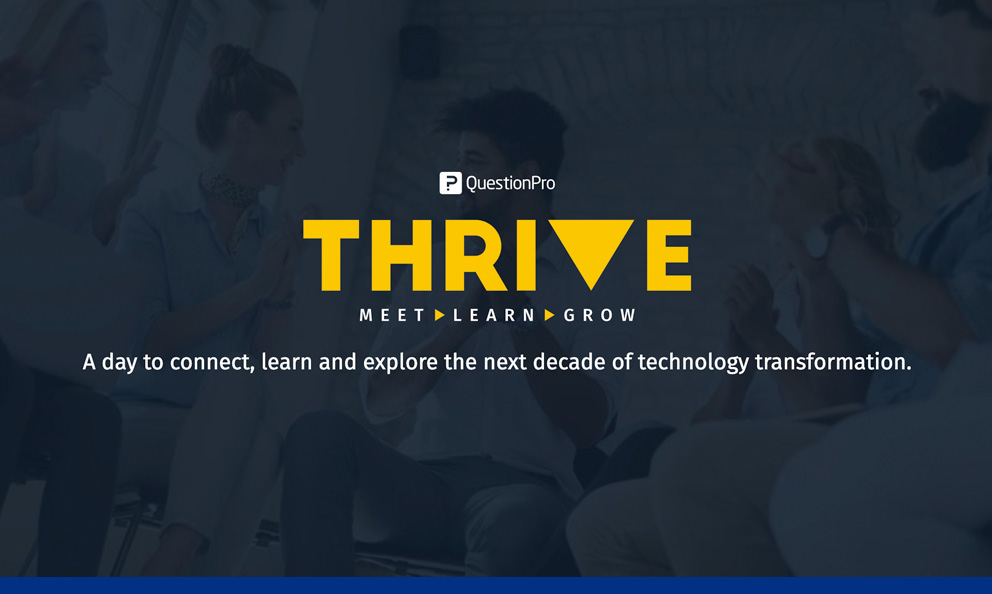
QuestionPro Thrive: A Space to Visualize & Share the Future of Technology
Jun 18, 2024
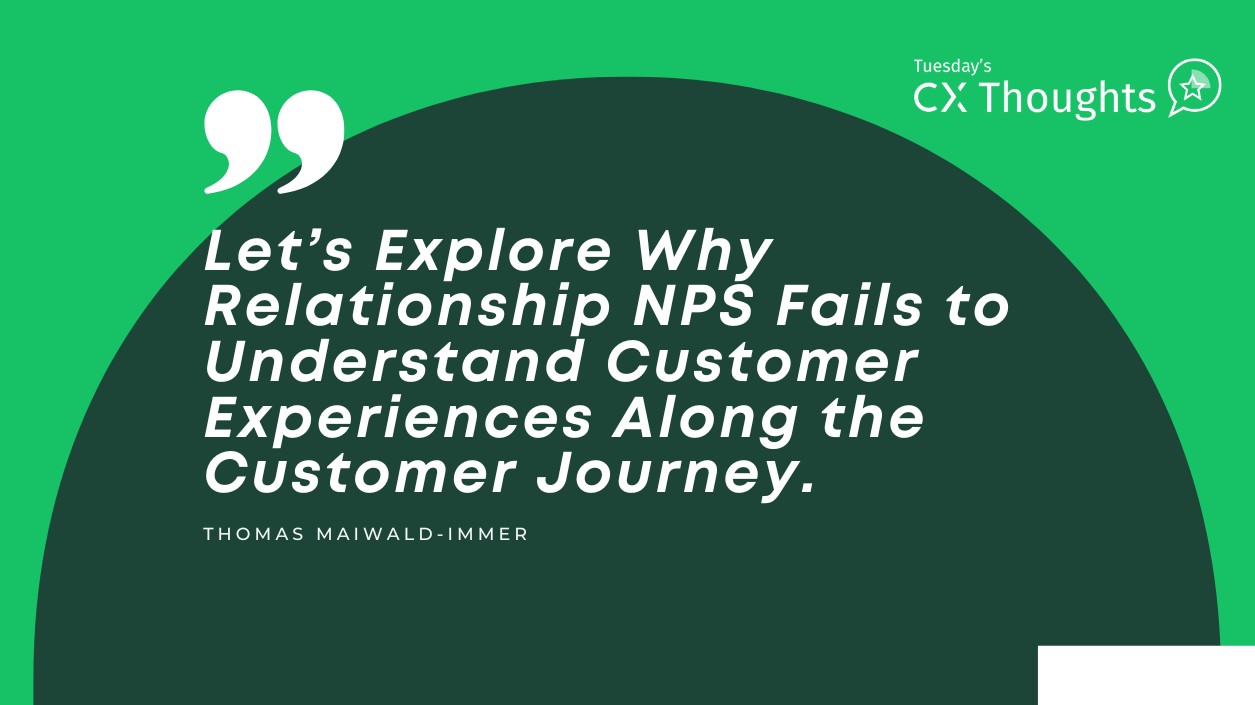
Relationship NPS Fails to Understand Customer Experiences — Tuesday CX
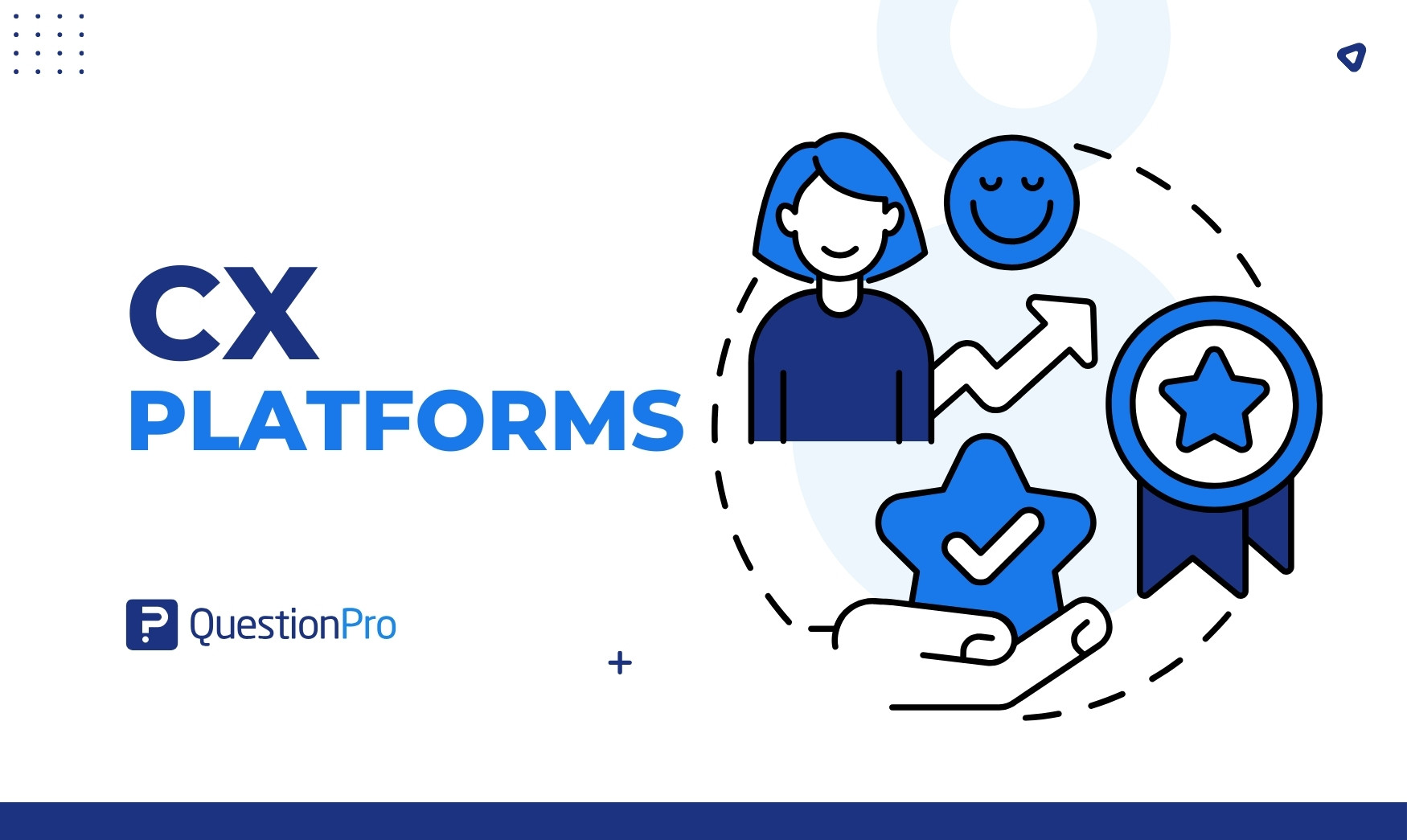
CX Platform: Top 13 CX Platforms to Drive Customer Success
Jun 17, 2024
Other categories
- Academic Research
- Artificial Intelligence
- Assessments
- Brand Awareness
- Case Studies
- Communities
- Consumer Insights
- Customer effort score
- Customer Engagement
- Customer Experience
- Customer Loyalty
- Customer Research
- Customer Satisfaction
- Employee Benefits
- Employee Engagement
- Employee Retention
- Friday Five
- General Data Protection Regulation
- Insights Hub
- Life@QuestionPro
- Market Research
- Mobile diaries
- Mobile Surveys
- New Features
- Online Communities
- Question Types
- Questionnaire
- QuestionPro Products
- Release Notes
- Research Tools and Apps
- Revenue at Risk
- Survey Templates
- Training Tips
- Tuesday CX Thoughts (TCXT)
- Uncategorized
- Video Learning Series
- What’s Coming Up
- Workforce Intelligence
- Bipolar Disorder
- Therapy Center
- When To See a Therapist
- Types of Therapy
- Best Online Therapy
- Best Couples Therapy
- Best Family Therapy
- Managing Stress
- Sleep and Dreaming
- Understanding Emotions
- Self-Improvement
- Healthy Relationships
- Student Resources
- Personality Types
- Guided Meditations
- Verywell Mind Insights
- 2024 Verywell Mind 25
- Mental Health in the Classroom
- Editorial Process
- Meet Our Review Board
- Crisis Support
Correlation Studies in Psychology Research
Determining the relationship between two or more variables.
Kendra Cherry, MS, is a psychosocial rehabilitation specialist, psychology educator, and author of the "Everything Psychology Book."
:max_bytes(150000):strip_icc():format(webp)/IMG_9791-89504ab694d54b66bbd72cb84ffb860e.jpg)
Emily is a board-certified science editor who has worked with top digital publishing brands like Voices for Biodiversity, Study.com, GoodTherapy, Vox, and Verywell.
:max_bytes(150000):strip_icc():format(webp)/Emily-Swaim-1000-0f3197de18f74329aeffb690a177160c.jpg)
Verywell / Brianna Gilmartin
- Characteristics
Potential Pitfalls
Frequently asked questions.
A correlational study is a type of research design that looks at the relationships between two or more variables. Correlational studies are non-experimental, which means that the experimenter does not manipulate or control any of the variables.
A correlation refers to a relationship between two variables. Correlations can be strong or weak and positive or negative. Sometimes, there is no correlation.
There are three possible outcomes of a correlation study: a positive correlation, a negative correlation, or no correlation. Researchers can present the results using a numerical value called the correlation coefficient, a measure of the correlation strength. It can range from –1.00 (negative) to +1.00 (positive). A correlation coefficient of 0 indicates no correlation.
- Positive correlations : Both variables increase or decrease at the same time. A correlation coefficient close to +1.00 indicates a strong positive correlation.
- Negative correlations : As the amount of one variable increases, the other decreases (and vice versa). A correlation coefficient close to -1.00 indicates a strong negative correlation.
- No correlation : There is no relationship between the two variables. A correlation coefficient of 0 indicates no correlation.
Characteristics of a Correlational Study
Correlational studies are often used in psychology, as well as other fields like medicine. Correlational research is a preliminary way to gather information about a topic. The method is also useful if researchers are unable to perform an experiment.
Researchers use correlations to see if a relationship between two or more variables exists, but the variables themselves are not under the control of the researchers.
While correlational research can demonstrate a relationship between variables, it cannot prove that changing one variable will change another. In other words, correlational studies cannot prove cause-and-effect relationships.
When you encounter research that refers to a "link" or an "association" between two things, they are most likely talking about a correlational study.
Types of Correlational Research
There are three types of correlational research: naturalistic observation, the survey method, and archival research. Each type has its own purpose, as well as its pros and cons.
Naturalistic Observation
The naturalistic observation method involves observing and recording variables of interest in a natural setting without interference or manipulation.
Can inspire ideas for further research
Option if lab experiment not available
Variables are viewed in natural setting
Can be time-consuming and expensive
Extraneous variables can't be controlled
No scientific control of variables
Subjects might behave differently if aware of being observed
This method is well-suited to studies where researchers want to see how variables behave in their natural setting or state. Inspiration can then be drawn from the observations to inform future avenues of research.
In some cases, it might be the only method available to researchers; for example, if lab experimentation would be precluded by access, resources, or ethics. It might be preferable to not being able to conduct research at all, but the method can be costly and usually takes a lot of time.
Naturalistic observation presents several challenges for researchers. For one, it does not allow them to control or influence the variables in any way nor can they change any possible external variables.
However, this does not mean that researchers will get reliable data from watching the variables, or that the information they gather will be free from bias.
For example, study subjects might act differently if they know that they are being watched. The researchers might not be aware that the behavior that they are observing is not necessarily the subject's natural state (i.e., how they would act if they did not know they were being watched).
Researchers also need to be aware of their biases, which can affect the observation and interpretation of a subject's behavior.
Surveys and questionnaires are some of the most common methods used for psychological research. The survey method involves having a random sample of participants complete a survey, test, or questionnaire related to the variables of interest. Random sampling is vital to the generalizability of a survey's results.
Cheap, easy, and fast
Can collect large amounts of data in a short amount of time
Results can be affected by poor survey questions
Results can be affected by unrepresentative sample
Outcomes can be affected by participants
If researchers need to gather a large amount of data in a short period of time, a survey is likely to be the fastest, easiest, and cheapest option.
It's also a flexible method because it lets researchers create data-gathering tools that will help ensure they get the information they need (survey responses) from all the sources they want to use (a random sample of participants taking the survey).
Survey data might be cost-efficient and easy to get, but it has its downsides. For one, the data is not always reliable—particularly if the survey questions are poorly written or the overall design or delivery is weak. Data is also affected by specific faults, such as unrepresented or underrepresented samples .
The use of surveys relies on participants to provide useful data. Researchers need to be aware of the specific factors related to the people taking the survey that will affect its outcome.
For example, some people might struggle to understand the questions. A person might answer a particular way to try to please the researchers or to try to control how the researchers perceive them (such as trying to make themselves "look better").
Sometimes, respondents might not even realize that their answers are incorrect or misleading because of mistaken memories .
Archival Research
Many areas of psychological research benefit from analyzing studies that were conducted long ago by other researchers, as well as reviewing historical records and case studies.
For example, in an experiment known as "The Irritable Heart ," researchers used digitalized records containing information on American Civil War veterans to learn more about post-traumatic stress disorder (PTSD).
Large amount of data
Can be less expensive
Researchers cannot change participant behavior
Can be unreliable
Information might be missing
No control over data collection methods
Using records, databases, and libraries that are publicly accessible or accessible through their institution can help researchers who might not have a lot of money to support their research efforts.
Free and low-cost resources are available to researchers at all levels through academic institutions, museums, and data repositories around the world.
Another potential benefit is that these sources often provide an enormous amount of data that was collected over a very long period of time, which can give researchers a way to view trends, relationships, and outcomes related to their research.
While the inability to change variables can be a disadvantage of some methods, it can be a benefit of archival research. That said, using historical records or information that was collected a long time ago also presents challenges. For one, important information might be missing or incomplete and some aspects of older studies might not be useful to researchers in a modern context.
A primary issue with archival research is reliability. When reviewing old research, little information might be available about who conducted the research, how a study was designed, who participated in the research, as well as how data was collected and interpreted.
Researchers can also be presented with ethical quandaries—for example, should modern researchers use data from studies that were conducted unethically or with questionable ethics?
You've probably heard the phrase, "correlation does not equal causation." This means that while correlational research can suggest that there is a relationship between two variables, it cannot prove that one variable will change another.
For example, researchers might perform a correlational study that suggests there is a relationship between academic success and a person's self-esteem. However, the study cannot show that academic success changes a person's self-esteem.
To determine why the relationship exists, researchers would need to consider and experiment with other variables, such as the subject's social relationships, cognitive abilities, personality, and socioeconomic status.
The difference between a correlational study and an experimental study involves the manipulation of variables. Researchers do not manipulate variables in a correlational study, but they do control and systematically vary the independent variables in an experimental study. Correlational studies allow researchers to detect the presence and strength of a relationship between variables, while experimental studies allow researchers to look for cause and effect relationships.
If the study involves the systematic manipulation of the levels of a variable, it is an experimental study. If researchers are measuring what is already present without actually changing the variables, then is a correlational study.
The variables in a correlational study are what the researcher measures. Once measured, researchers can then use statistical analysis to determine the existence, strength, and direction of the relationship. However, while correlational studies can say that variable X and variable Y have a relationship, it does not mean that X causes Y.
The goal of correlational research is often to look for relationships, describe these relationships, and then make predictions. Such research can also often serve as a jumping off point for future experimental research.
Heath W. Psychology Research Methods . Cambridge University Press; 2018:134-156.
Schneider FW. Applied Social Psychology . 2nd ed. SAGE; 2012:50-53.
Curtis EA, Comiskey C, Dempsey O. Importance and use of correlational research . Nurse Researcher . 2016;23(6):20-25. doi:10.7748/nr.2016.e1382
Carpenter S. Visualizing Psychology . 3rd ed. John Wiley & Sons; 2012:14-30.
Pizarro J, Silver RC, Prause J. Physical and mental health costs of traumatic war experiences among civil war veterans . Arch Gen Psychiatry . 2006;63(2):193. doi:10.1001/archpsyc.63.2.193
Post SG. The echo of Nuremberg: Nazi data and ethics . J Med Ethics . 1991;17(1):42-44. doi:10.1136/jme.17.1.42
Lau F. Chapter 12 Methods for Correlational Studies . In: Lau F, Kuziemsky C, eds. Handbook of eHealth Evaluation: An Evidence-based Approach . University of Victoria.
Akoglu H. User's guide to correlation coefficients . Turk J Emerg Med . 2018;18(3):91-93. doi:10.1016/j.tjem.2018.08.001
Price PC. Research Methods in Psychology . California State University.
By Kendra Cherry, MSEd Kendra Cherry, MS, is a psychosocial rehabilitation specialist, psychology educator, and author of the "Everything Psychology Book."
- Home
- UA Graduate and Undergraduate Research
- UA Theses and Dissertations
- Dissertations
Descriptive Correlational Study to Examine Variables and Relationships Proposed in Conceptual Framework of Virtual Transitioning Program Developed for Foreign Educated Nurses (FEN)

Degree Name
Degree level, degree program, degree grantor, collections.
entitlement
Show Statistical Information
Export search results
The export option will allow you to export the current search results of the entered query to a file. Different formats are available for download. To export the items, click on the button corresponding with the preferred download format.
By default, clicking on the export buttons will result in a download of the allowed maximum amount of items.
To select a subset of the search results, click "Selective Export" button and make a selection of the items you want to export. The amount of items that can be exported at once is similarly restricted as the full export.
After making a selection, click one of the export format buttons. The amount of items that will be exported is indicated in the bubble next to export format.
Designing and Carrying Out Correlational Studies Using Real-World Data
- First Online: 10 February 2022
Cite this chapter
- Charles P. Friedman 4 ,
- Jeremy C. Wyatt 5 &
- Joan S. Ash 6
Part of the book series: Health Informatics ((HI))
1494 Accesses
This chapter describes the benefits and challenges of carrying out studies of informatics resources using routine or real-world data. It describes how to carry out such studies in a professional manner using a trusted or virtual environment. It then examines the challenges of inferring causality from correlation, and offers strategies to overcome each of these.
This is a preview of subscription content, log in via an institution to check access.
Access this chapter
- Available as PDF
- Read on any device
- Instant download
- Own it forever
- Available as EPUB and PDF
- Compact, lightweight edition
- Dispatched in 3 to 5 business days
- Free shipping worldwide - see info
- Durable hardcover edition
Tax calculation will be finalised at checkout
Purchases are for personal use only
Institutional subscriptions
Assessment bias is also known as ascertainment bias.
Agiro A, Chen X, Eshete B, Sutphen R, Bourquardez Clark E, Burroughs CM, et al. Data linkages between patient-powered research networks and health plans: a foundation for collaborative research. J Am Med Inform Assoc 2019;26:594–602. Available from https://doi.org/10.1093/jamia/ocz012 . Accessed 2 July 2021.
Agniel D, Kohane IS, Weber GM. Biases in electronic health record data due to processes within the healthcare system: retrospective observational study. BMJ. 2018;361:k1479.
Article Google Scholar
Andersen B. Methodological errors in medical research. Oxford, UK: Blackwell Scientific Publications; 1990.
Google Scholar
Baker L. Hilarious graphs (and pirates) prove that correlation is not causation. Data Science Central. Issaquah, WA: Data Science Central, TechTarget; 2018. Available from https://www.datasciencecentral.com/profiles/blogs/hilarious-graphs-and-pirates-prove-that-correlation-is-not . Accessed 29 June 2021.
Benchimol EI, Smeeth L, Guttmann A, Harron K, Moher D, Petersen I, et al. RECORD Working Committee. The REporting of studies conducted using observational routinely-collected health data (RECORD) statement. PLoS Med 2015;12:e1001885. Available from https://doi.org/10.1371/journal.pmed.1001885 . Accessed 29 June 2021.
Britto MT, Fuller SC, Kaplan HC, Kotagal U, Lannon C, Margolis PA, et al. Using a network organisational architecture to support the development of learning healthcare systems. BMJ Qual Safety. 2018;27:937–46.
Byar DP. Why data bases should not replace randomized clinical trials. Biometrics. 1980;36:337–42.
Article CAS Google Scholar
Collins R, Bowman L, Landray M, Peto R. The magic of randomization versus the myth of real-world evidence. N Engl J Med. 2020;382:674–8.
Cooper GF, Bahar I, Becich MJ, Benos PV, Berg J, Espino JU, et al. Center for Causal Discovery Team. The center for causal discovery of biomedical knowledge from big data. J Am Med Inform Assoc 2015;22:1132–6. Available from https://doi.org/10.1093/jamia/ocv059 . Accessed 29 June 2021.
Davey Smith G. Capitalising on Mendelian randomization to assess the effects of treatments. James Lind Library Bull (JLL Bull) 2006;1:1. Available from https://www.jameslindlibrary.org/articles/capitalising-on-mendelian-randomization-to-assess-the-effects-of-treatments/ . Accessed 29 June 2021.
Deeny SR, Steventon A. Making sense of the shadows: priorities for creating a learning healthcare system based on routinely collected data. BMJ Qual Safety. 2015;24:505–15.
Equator Network, UK EQUATOR Centre. Results of Search for Reporting Guidelines: Observational Studies. Oxford, UK: Equator Network, UK EQUATOR Centre, Centre for Statistics in Medicine (CSM), NDORMS, University of Oxford; 2021. Available from https://www.equator-network.org/?post_type=eq_guidelines&eq_guidelines_study_design=observational-studies&eq_guidelines_clinical_specialty=0&eq_guidelines_report_section=0&s=+&eq_guidelines_study_design_sub_cat=0 . Accessed 29 June 2021.
Eriksson T, Maclure M, Kragstrup J. Consultation with the general practitioner triggered by advice from social network members. Scand J Prim Health Care. 2004;22:54–9.
Ford I, Murray H, Packard CJ, Shepherd J, Macfarlane PW, Cobbe SM. Long-term follow-up of the West of Scotland Coronary Prevention Study. N Eng J Med. 2000;357:1477–86.
Friedman CP, Wong AK, Blumenthal D. Achieving a nationwide learning health system. Sci Transl Med 2010;2:57cm29. Available from https://doi.org/10.1126/scitranslmed.3001456 . Accessed 29 June 2021.
Gray E, Marti J, Wyatt JC, Brewster DH, Hall PS, SATURNE Advisory Group. Chemotherapy effectiveness in trial-underrepresented groups with early breast cancer: a retrospective cohort study. PLoS Med. 2019;16:e1003006.
Grimes DA, Schulz KF. Bias and causal associations in observational research. Lancet. 2002;359:248–52.
Gymrek M, McGuire AL, Golan D, Halperin E, Erlich Y. Identifying personal genomes by surname inference. Science. 2013;339:321–4.
Hemkens LG, Contopoulos-Ioannidis DG, Ioannidis JP. Routinely collected data and comparative effectiveness evidence: promises and limitations. CMAJ 2016;188:158–164. Available from https://doi.org/10.1503/cmaj.150653 . Accessed 29 Jun 2021.
Hill AB. The environment and disease: association or causation? Proc R Soc Med. 1965;58:295–300.
CAS PubMed PubMed Central Google Scholar
Hilton Boon M, Craig P, Thomson H, Campbell M, Moore L. Regression discontinuity designs in health: a systematic review. Epidemiology. 2021;32:87–93.
Hundepool A, Domingo-Ferrer J, Franconi L, Giessing S, Lenz R, Longhurst J, et al. A CENtre of EXcellence for Statistical Disclosure Control Handbook on Statistical Disclosure Control Version 1.2 . Brussels, Belgium: CENtre of EXcellence for Statistical Disclosure Control (CENEX SDC), Portal on Collaboration in Research and Methodology for Official Statistics (CROS), ESSnet, European Commission; 2010.
Ioannidis JPA. Contradicted and initially stronger effects in highly cited clinical research. JAMA. 2005;294:218–28.
Julious SA, Mullee MA. Confounding and Simpson’s paradox. BMJ. 1994;309:1480–1.
Kahneman D. Thinking, fast and slow. New York: Macmillan; 2011.
Klein MS, Ross FV, Adams DL, Gilbert CM. Effect of online literature searching on length of stay and patient care costs. Acad Med. 1994;69(6):489–95.
König K, Pechmann A, Thiele S, Walter MC, Schorling D, Tassoni A, et al. De-duplicating patient records from three independent data sources reveals the incidence of rare neuromuscular disorders in Germany. Orphanet J Rare Dis. 2019;14:152.
Lawrence ND. Data readiness levels: turning data from palid to vivid. In: Lawrence ND, editors. inverseprobability.com : Neil Lawrence’s Homepage. Cambridge, UK: Neil D. Lawrence; 2017. Available from https://inverseprobability.com/2017/01/12/data-readiness-levels . Accessed 29 June 2021.
Maclure M. The case-crossover design: a method for studying transient effects on the risk of acute events. Am J Epidemiol. 1991;133:144–53.
Matthews GJ, Harel O. Data confidentiality: a review of methods for statistical disclosure limitation and methods for assessing privacy. Stat Surv. 2011;5:1–29.
O’Keefe CM, Rubin DB. Individual privacy versus public good: protecting confidentiality in health research. Stat Med. 2015;34:3081–103.
Ory HW. Association between oral contraceptives and myocardial infarction. A review. JAMA. 1977;237:2619–22.
Riaz H, Khan MS, Siddiqi TJ, Usman MS, Shah N, Goyal A, et al. Association between obesity and cardiovascular outcomes: a systematic review and meta-analysis of Mendelian randomization studies. JAMA Netw Open. 2018;1:e183788.
Rosenbaum PR, Rubin DB. The central role of the propensity score in observational studies for causal effects. Biometrika. 1983;70:41–55.
Seltzer CC, Bosse R, Garvey AJ. Mail survey response by smoking status. Am J Epidemiol. 1974;100:453–7.
Sherman RE, Anderson SA, Dal Pan GJ, Gray GW, Gross T, Hunter NL, et al. Real-world evidence - what is it and what can it tell us? N Engl J Med. 2016;375:2293–7.
Simpson EH. The interpretation of interaction in contingency tables. J R Stat Soc Ser B (Methodol). 1951;13:238–41.
Suissa S, Ernst P. Avoiding immortal time bias in observational studies. Eur Respir J. 2020;55:2000138.
Tsopra R, Wyatt JC, Beirne P, Rodger K, Callister M, Ghosh D, et al. Level of accuracy of diagnoses recorded in discharge summaries: a cohort study in three respiratory wards. J Eval Clin Pract. 2019;25:36–43.
Tufte E. The visual display of quantitative information. New York: Graphics Press; 1992.
United Kingdom National Health Service (NHS) Digital. Hospital Episode Statistics for Admitted Patient Care and Outpatient Data: Official Statistics Database. Leeds, UK: United Kingdom National Health Service (NHS) Digital, United Kingdom National Health Service (NHS); 2021. Available from https://digital.nhs.uk/data-and-information/publications/statistical/hospital-episode-statistics-for-admitted-patient-care-outpatient-and-accident-and-emergency-data . Accessed 29 June 2021.
Waruru A, Natukunda A, Nyagah LM, Kellogg TA, Zielinski-Gutierrez E, Waruiru W, et al. Where no universal health care identifier exists: comparison and determination of the utility of score-based persons matching algorithms using demographic data. JMIR Pub Health Surveill. 2018;4:e10436.
Yao XI, Wang X, Speicher PJ, Hwang ES, Cheng P, Harpole DH, et al. Reporting and guidelines in propensity score analysis: a systematic review of cancer and cancer surgical studies. J Natl Cancer Inst 2017;109:djw323. Available from https://doi.org/10.1093/jnci/djw323 . Accessed 29 June 2021.
Zhou Y, Matsouaka RA, Thomas L. Propensity score weighting under limited overlap and model misspecification. Stat Methods Med Res. 2020;29:3721–56.
Download references
Author information
Authors and affiliations.
Department of Learning Health Sciences, University of Michigan Medical School, Ann Arbor, MI, USA
Charles P. Friedman
Department of Primary Care, Population Sciences and Medical Education, School of Medicine, University of Southampton, Southampton, UK
Jeremy C. Wyatt
Department of Medical Informatics and Clinical Epidemiology, School of Medicine, Oregon Health & Science University, Portland, OR, USA
Joan S. Ash
You can also search for this author in PubMed Google Scholar
Corresponding author
Correspondence to Charles P. Friedman .
Answers to Self-Tests
Self-test 13.1.
Spurious correlation
The key observation of concern here is that the 3500 app users form only 1.4% of the overall population (3500/247,000) eligible to use the app. So, a study team needs to ask themselves what factors caused those relatively few individuals to use the app, and might these factors also have led to the observed weight loss ? Some possible factors could include:
Pressure from family and friends (and support) to lose weight
A forthcoming significant future event, e.g. a wedding or graduation
A health scare, e.g. a heart attack, suffered by the patients themselves or a close family member
Developing a new relationship that caused them to “turn over a new leaf” in their lives
Any of these factors are plausible explanations for why weight loss app users might start and continue to use the app, but are also reasons why that person might lose weight even without the app. So, use of the app might be a marker of the small proportion of people in the population who really intend to lose weight, and are being supported by others close to them to lose weight—rather than the cause. To investigate this possibility, a random subset of app users and non-users could be surveyed, asking them about any of the above factors. A qualitative investigation (using interviews or focus groups) of factors associated with success at weight loss (both promoters and inhibitors) would be useful to carry out before finalizing the survey questions to ensure that factors identified as important by responders are included.
Selection bias
Generally, consistent app users are younger than non-users and more likely to own and use a smartphone, which puts them in a income group than non-app users or people who do not own a smart phone. So, what we could be seeing here is bigger weight loss in the subset of people who own and use a smart phone compared to those who do not. This selection bias could be investigated by comparing data on the educational level and household income of app users and non-users, and adding these variable into a regression model to predict weight loss based on a number of factors that are known to be associated with success at losing weight. The person’s use or not of the smart phone app could then be added to this model to investigate if this new model explains more of the observed variation in weight loss, and if the coefficient for this variable is significant.
Information bias
If the person’s weight at the start and the end of the study period is recorded by the app in app users but is recorded informally (in a notebook, diary or vague remembrance of weight a year ago) in the non-users, this could lead to an information bias. Assume that the weight loss based on data recorded in the app is correct. However, it is possible that the weight loss for non-users is underestimated because the non-app users have forgotten or misplaced their weight recording from a year ago, and innocently substitute a figure that is closer to their current weight than it was in reality. If this is the case, the lack of an objective record in non-app users of their weight a year ago would tend to reduce their apparent weights loss, and thus make it appear less than the measured weight loss in app users. To eliminate this bias, we would need an objective record of the baseline weight for the entire population.
Confounding
It is possible that the app was provided to a subset of the population specifically selected by the health care delivery network as part of a risk management exercise to reduce their chances of developing a weight-related illness (such as diabetes, stroke, heart attacks). In addition, other actions may also have formed part of this program, for example regular contacts with a dietician, feedback on their weight and exercise regime compared to that of others in the program, or incentives such as a lower renewal fee if they were successful at reaching their weight loss goal at year end. All of these would be confounders in any study that claimed to measure the impact of the app on weight loss. A searching question directed back to the health care delivery network about potential reasons for app use would help the study team uncover whether the app was targeted to a specific population, and if the users also received other weight loss support.
Self-Test 13.2
The histogram shows how the percent online time increased dramatically in 2020, then reduced slightly in 2021 and 2022, but still remained higher than for the earlier years. Two of the added value scores (physiology and pathology) remained roughly the same, but the anatomy added value score appeared to drop in 2020 and the following years.
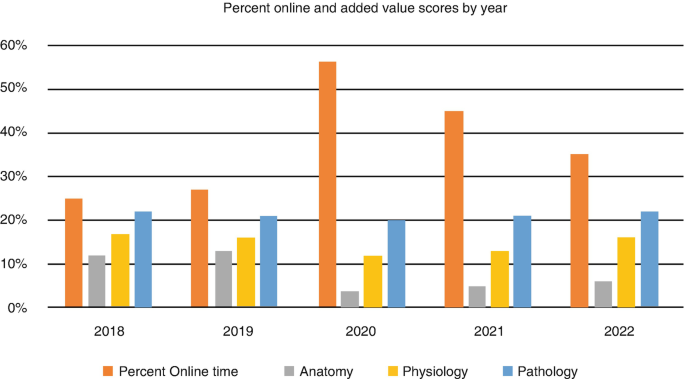
The X-Y plot of mean added value score against percent online time shows a negative correlation, with a slight drop in the mean score with increasing online time. This is confirmed by the regression line, showing all data points close to the line, and R squared of 0.97. From this analysis, one would be inclined to conclude that online learning does not promote student learning.
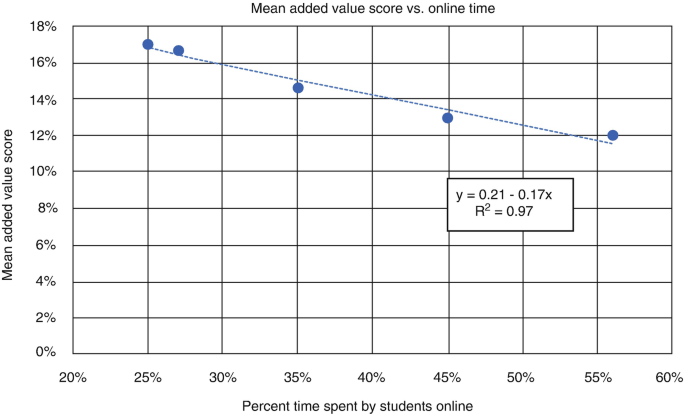
The histogram clearly indicates that the effects differ across the subjects, and the averaging across the subjects conceals this effect. It appears that the increased time spent online had little effect on the added value scores for two subjects, but was associated with a large drop in the anatomy program added value scores. This might be because anatomy is better taught face to face, or it might be another factor, such as a change in program admission criteria, in teaching staff or the materials used. For example, an increase in the baseline anatomy skills of the students in 2020 and onwards could explain the decrease in added value. Equally, the explanation for reduced anatomy added value scores may be an effect of the COVID pandemic which started in 2020, but this would be expected to affect all three subjects.
To investigate if the “online effect” is larger for anatomy than for the two other subjects, the slopes of the regression lines for anatomy alone versus the mean of all three subjects could be compared. The final graph below shows that the reduction in added value with online teaching is greater for anatomy with a regression coefficient of 0.29, almost double the coefficient of 0.17 for the mean across all three subjects.
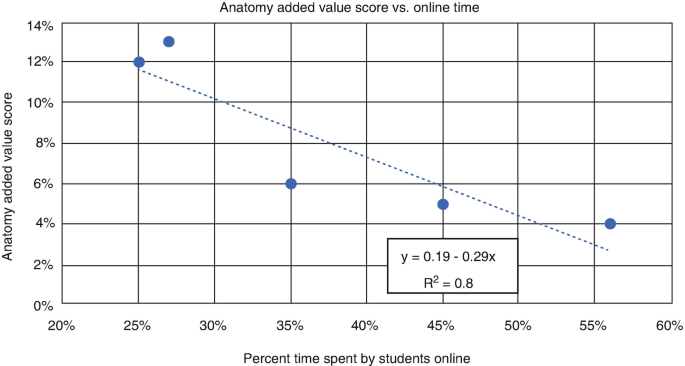
Further data that could be sought to understand the reason for the observed changes includes:
The numbers of students in the program each year, as some of these changes could simply be due to small numbers in certain years.
The actual entering scores of students for each subject and year, as interpretation of the added value score varies depending on the starting point.
Data about teaching staff numbers and skills and student participation rates and satisfaction year by year and across the three subjects, to help understand why online learning may have led to lower added value for anatomy, if this is true.
Further information about the types (and quality) of online learning experience offered across the three subjects. It is possible, for example, that the online offerings for anatomy differed from those in physiology and pathology.
Rights and permissions
Reprints and permissions
Copyright information
© 2022 Springer Nature Switzerland AG
About this chapter
Friedman, C.P., Wyatt, J.C., Ash, J.S. (2022). Designing and Carrying Out Correlational Studies Using Real-World Data. In: Evaluation Methods in Biomedical and Health Informatics. Health Informatics. Springer, Cham. https://doi.org/10.1007/978-3-030-86453-8_13
Download citation
DOI : https://doi.org/10.1007/978-3-030-86453-8_13
Published : 10 February 2022
Publisher Name : Springer, Cham
Print ISBN : 978-3-030-86452-1
Online ISBN : 978-3-030-86453-8
eBook Packages : Medicine Medicine (R0)
Share this chapter
Anyone you share the following link with will be able to read this content:
Sorry, a shareable link is not currently available for this article.
Provided by the Springer Nature SharedIt content-sharing initiative
- Publish with us
Policies and ethics
- Find a journal
- Track your research
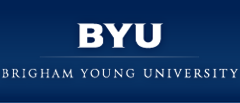
BYU ScholarsArchive
- < Previous
Home > Theses and Dissertations > 1607
Theses and Dissertations
A correlational study: the relationship between physical activity levels, physical self-worth, and global self-worth in high school physical education students.
Ashley Danelle Eyre , Brigham Young University - Provo Follow
The purpose of this study was to determine the relationship between physical activity levels, physical self-worth, and its sub-domains; (a) skill, (b) body attractiveness, (c) fitness and conditioning, and (d) strength, and overall global self-worth in high school students. One-Hundred and sixty-seven students (male = 66;female = 101) from the western United States, ages 13-18, participated in this study. Each participant completed a minimum of four days of physical activity (PA) data collection and completed the self-worth questionnaire. Pedometers were used to collect PA levels, calculating mean step counts, and the Children and Youth Physical Self-Perception Profile (CY-PSPP) was used to determine physical self-worth (PSW) and global self-worth (GSW) scores. Results showed a small but positive relationship between physical self-worth and PA level (r = 0.19, p < 0.01), with no relationship shown between PA level and GSW. Physical activity level was significantly correlated with skill and body attractiveness (p < .02). Quartile analyses of mean step count confirmed a significantly higher PSW in the top three quartiles compared to the bottom quartile. In summary, this study found that PA levels is positively associated with PSW and its two sub-domains of body attractiveness and skill, but PA levels did not show a significant relationship with GSW.
College and Department
Life Sciences; Exercise Sciences
http://lib.byu.edu/about/copyright/
BYU ScholarsArchive Citation
Eyre, Ashley Danelle, "A Correlational Study: The Relationship Between Physical Activity Levels, Physical Self-Worth, and Global Self-Worth in High School Physical Education Students" (2008). Theses and Dissertations . 1607. https://scholarsarchive.byu.edu/etd/1607
Date Submitted
Document type.
http://hdl.lib.byu.edu/1877/etd2740
physical activity, physical self-worth, global self-worth, physical self-perception, adolescents, physical education
Since July 28, 2014
Included in
Exercise Science Commons
Advanced Search
- Notify me via email or RSS
ScholarsArchive ISSN: 2572-4479
- Collections
- Disciplines
- Scholarly Communication
- Additional Collections
- Academic Research Blog
Author Corner
Hosted by the.
- Harold B. Lee Library
Home | About | FAQ | My Account | Accessibility Statement
Privacy Copyright
Search Icon
Events See all →
Dangerous waters.
Meyerson Hall, 210 S. 34th St.
June 2024 Wellness Walk

Benjamin Franklin Statue
Wawa Welcome America Day

10:00 a.m. - 5:00 p.m.
Penn Museum, 3260 South St.
ICA Summer 2024 Opening Celebration
7:00 p.m. - 10:00 p.m.
Institute of Contemporary Art, 118 S. 36th St.
Arts, Humanities, & Social Sciences
New dissertation grants expand global research support
The newly established penn global dissertation grants program provides as much as $8,000 in funding to each of 11 ph.d. candidates to enhance global components in their research..
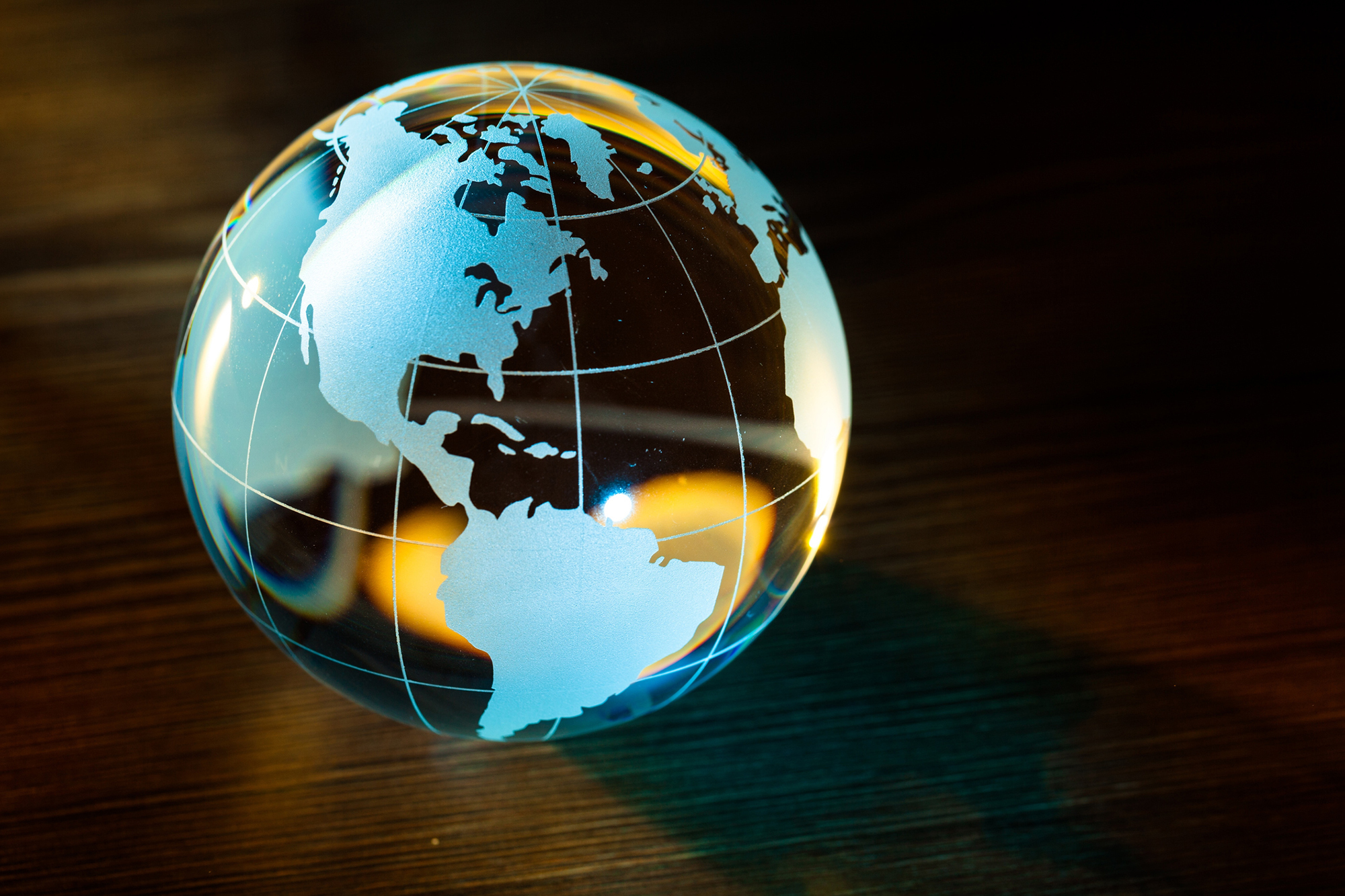
Penn Global has announced the first recipients of the newly established Penn Global Dissertation Grants program , providing as much as $8,000 each in funding to 11 Ph.D. students across four schools
With dissertations ranging from examinations of artificial intelligence and computational immunology in Vietnam to a look at the intersection of women, food, and freedom in the Dominican Republic, the recipients will use the funds to enhance global components in their dissertation research.
A main priority for Penn Global under its third strategic framework is to develop initiatives that support graduate and professional research with global dimensions. Introducing the Penn Global Dissertation Grants, which will be offered annually, augments global opportunities for graduate and professional students by expanding the scope of Penn Global’s research support.
“This program is the best example of how our strategic plan is responsive to the needs of the University community,” says Amy Gadsden , associate vice provost for global initiatives. “When Vice Provost for Global Initiatives Zeke Emanuel and I met with stakeholders across campus last year to further refine the strategic plan, a discussion emerged among faculty about the need to address a growing gap in support for graduate and professional students pursuing international research, particularly in the humanities and social sciences. It was clear that what we needed was a funding mechanism to support international dissertation research. We ran with this idea and, in close partnership with the Office of the Vice Provost for Education, established the Penn Global Dissertation Grants.”
“It was important for our office to get behind this initiative,” says Karen Detlefsen, Vice Provost for Education. “It met a real need to provide more University support for graduate work that has potential to extend well beyond the boundaries of any discipline. The research many of our students are doing in a global context is inspiring.”
This program is intended to harness the power of Penn’s graduate student community to enhance the University’s global engagement.
“Graduate students are on the cutting edge of research, but finding support for global inquiry can be difficult,” Gadsden says. “This program ensures that Penn’s graduates students can stay on the cutting edge of their fields and do deeply immersive work to advance new knowledge.”
The program offers two tracks for Ph.D. students seeking to incorporate global dimensions into their work: global exploration and global enhancement. Global exploration applicants are still in the development stages of their dissertation and will use this program’s resources to explore and engage global components for their dissertation research. Global enhancement applicants already include a core global focus to their dissertation and will use this program’s resources to broaden and deepen their research, ideally leveraging their dissertation toward future career opportunities in global leadership.
In the School of Arts & Sciences , recipients are Juan Arboleda, a history Ph.D. student whose research focuses on Brazil and Colombia; Adwaita Banerjee, an anthropology Ph.D. student whose research focuses on India; Tayeba Batool, an anthropology Ph.D. student whose research focuses on Pakistan; Nursyazwani Binte Jamaludin, an anthropology Ph.D. student whose research focuses on Myanmar; Jalen Chang, a history of art Ph.D. student whose research focuses on France, Oceania, and New Caledonia; Bonnie Maldonado, a Ph.D. student in Africana studies whose research focuses on the Dominican Republic; Taylor Prescott, a history Ph.D. student whose research focuses on Sierra Leone; and Alexandra Zborovsky a history Ph.D. student whose research focuses on Russia, Ukraine, the Netherlands, and Israel.
In the Perelman School of Medicine , the recipient is Van Truong, whose focus is on genomics and computational biology in Vietnam. In the Annenberg School for Communication , the recipient is Adetobi Moses, whose research focuses on Ghana. In the Weitzman School of Design, the recipient is Hui Tian, whose research focuses on China.
The Penn Global Dissertation Grant program will also offer a platform for these students to share their work and research experience with the broader Penn community. One stipulation of the program is developing individualized post-award commitment plans. The inaugural cohort of awardees is currently finalizing their own plans, which may include Penn undergraduate mentorship programs, panel discussions on their research topics and outcomes, and other forms of publishable multimedia.
Currently enrolled Penn Ph.D. students from all Penn Schools and disciplines, including the humanities, social sciences, and STEM fields, may apply. Additional program information is available at https://global.upenn.edu/global-initiatives/penn-global-dissertation-grants .
To Penn’s Class of 2024: ‘The world needs you’
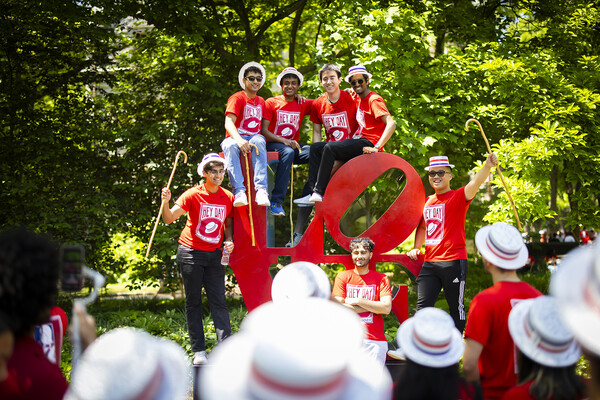
Campus & Community
Class of 2025 relishes time together at Hey Day
An iconic tradition at Penn, third-year students were promoted to senior status.
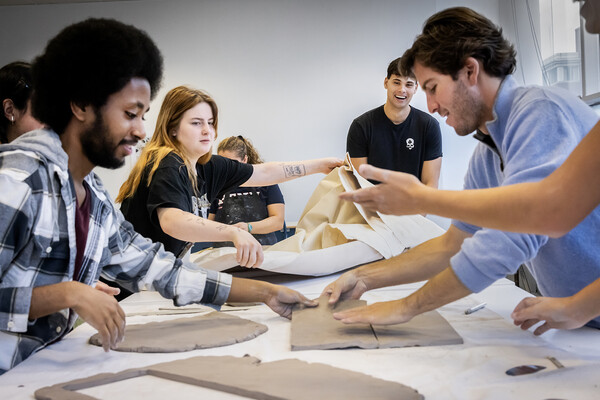
Picturing artistic pursuits
Hundreds of undergraduates take classes in the fine arts each semester, among them painting and drawing, ceramics and sculpture, printmaking and animation, photography and videography. The courses, through the School of Arts & Sciences and the Stuart Weitzman School of Design, give students the opportunity to immerse themselves in an art form in a collaborative way.
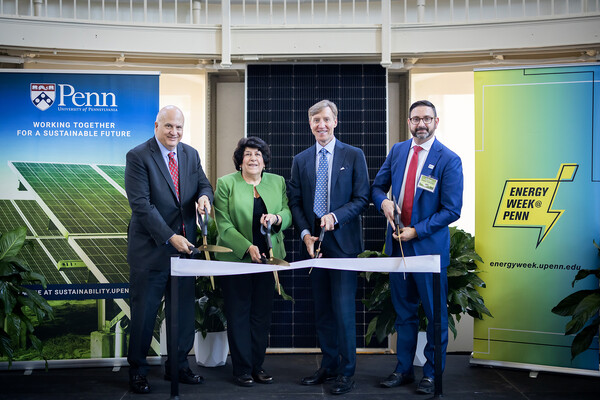
Penn celebrates operation and benefits of largest solar power project in Pennsylvania
Solar production has begun at the Great Cove I and II facilities in central Pennsylvania, the equivalent of powering 70% of the electricity demand from Penn’s academic campus and health system in the Philadelphia area.
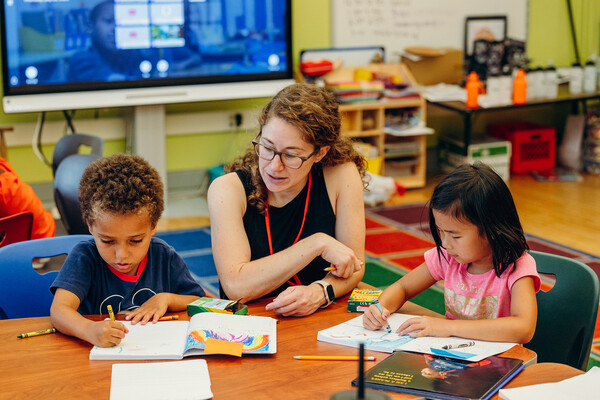
Education, Business, & Law
Investing in future teachers and educational leaders
The Empowerment Through Education Scholarship Program at Penn’s Graduate School of Education is helping to prepare and retain teachers and educational leaders.
Ohio State nav bar
Ohio state navigation bar.
- BuckeyeLink
- Search Ohio State
Haoyun Yang Dissertation Defense
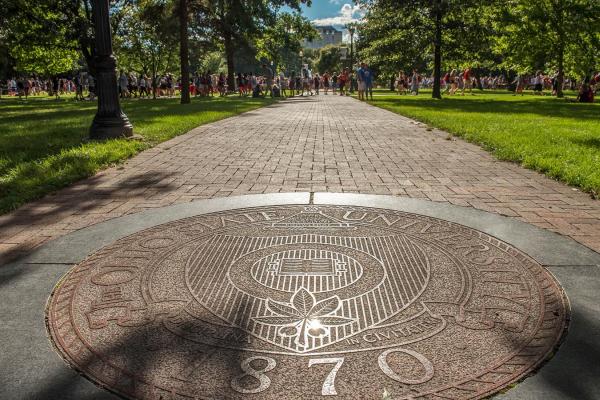
Help | Advanced Search
Astrophysics > Earth and Planetary Astrophysics
Title: a comparative simulation study of hot and ultra-hot jupiter atmospheres using different ground-based high-resolution spectrographs with cross-correlation spectroscopy.
Abstract: In the era of state-of-the-art space-borne telescopes, high-resolution ground-based observation has emerged as a crucial method for characterizing exoplanets, providing essential insights into their atmospheric compositions. In the optical and NIR regions, high-resolution spectroscopy has been powerful for hot Jupiters (HJ) and ultra-hot Jupiters (UHJ) during their primary transits, as it can probe molecules with better sensitivity. Here, we focus on a comparative simulation study of WASP-76 b (UHJ) and WASP-77 A b (HJ) for different number of transits, utilizing three ground-based spectrographs (GIANO-B (TNG), CARMENES (CAHA), and ANDES (E-ELT)) with varying instrumental parameters, spectral coverages, and resolutions. We aim to evaluate the feasibility of the upcoming ground-based European Extremely Large Telescope (E-ELT) in probing molecules from planet atmospheres and how it surpasses other ground-based observatories in terms of detectability. With the 1-D model, petitCODE, we have self-consistently simulated the atmospheric pressure-temperature profiles, which are subsequently integrated into the 1-D chemical kinetics model, VULCAN, to evolve the atmospheric chemistry. High-resolution spectra are obtained by performing line-by-line radiative transfer using petitRADTRANS. Finally, we use the resulting spectra to assess the detectability (sigma_det) of molecular bands, employing the ground-based noise simulator SPECTR. Utilizing cross-correlation spectroscopy, we have successfully demonstrated the robust consistency between our simulation study and real-time observations for both planets. ANDES excels overall in molecular detection due to its enhanced instrumental architecture, reinforcing E-ELT's importance for studying exoplanet atmospheres. Additionally, our theoretical simulations predict the detection of CO, NH3, and H2S on WASP-76 b atmosphere with a sigma_det> 3.
Comments: | Accepted for publication in the Astrophysical Journal; 25 pages (18 figures and 4 tables) |
Subjects: | Earth and Planetary Astrophysics (astro-ph.EP) |
Cite as: | [astro-ph.EP] |
(or [astro-ph.EP] for this version) | |
Focus to learn more arXiv-issued DOI via DataCite |
Submission history
Access paper:.
- HTML (experimental)
- Other Formats

References & Citations
- Google Scholar
- Semantic Scholar
BibTeX formatted citation

Bibliographic and Citation Tools
Code, data and media associated with this article, recommenders and search tools.
- Institution
arXivLabs: experimental projects with community collaborators
arXivLabs is a framework that allows collaborators to develop and share new arXiv features directly on our website.
Both individuals and organizations that work with arXivLabs have embraced and accepted our values of openness, community, excellence, and user data privacy. arXiv is committed to these values and only works with partners that adhere to them.
Have an idea for a project that will add value for arXiv's community? Learn more about arXivLabs .
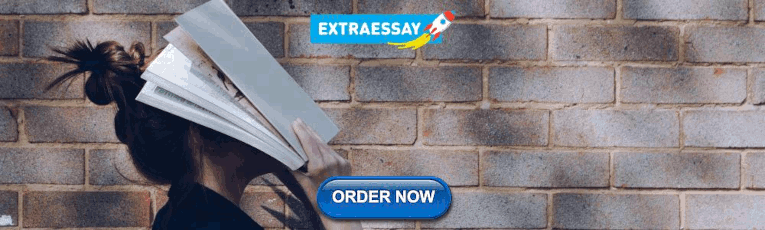
IMAGES
VIDEO
COMMENTS
The present study proposes to examine the relationship between parental age and the. academic success of their children. The study will examine children of parents from. different age groups, and through a variety of different measures examine if there is a link. between older parents and higher academic achievement.
A CORRELATIONAL STUDY ON THE RELATIONSHIP BETWEEN 2D:4D RATIO AND MOOD CHANGE . A Thesis . Presented . to the faculty of . California State University, Chico . In Partial Fulfillment . Of the Requirements for the Degree . Master of Arts . In . Psychology . Psychological Science Option . by . Donald Robert Rickgauer . Spring 2010
Correlational research is a type of study that explores how variables are related to each other. It can help you identify patterns, trends, and predictions in your data. In this guide, you will learn when and how to use correlational research, and what its advantages and limitations are. You will also find examples of correlational research questions and designs. If you want to know the ...
Boucaud, Akil A., "A Correlational Study Examining the Relationship Between Restorative Practices and School Climate in Selected Elementary Schools in a Large Mid-Atlantic Urban School District" (2017). ... Dissertation submitted to the Faculty of the College of Education in partial fulfillment of the requirements for the degree of
Revised on 5 December 2022. A correlational research design investigates relationships between variables without the researcher controlling or manipulating any of them. A correlation reflects the strength and/or direction of the relationship between two (or more) variables. The direction of a correlation can be either positive or negative.
A Quantitative Correlational Study between Transformational Leadership Behavior and Job Satisfaction among California Card Room Casino Employees Lydell H. Hall ... This Dissertation is brought to you for free and open access by UMass Global ScholarWorks. It has been accepted
Correlational research has many practical applications in various fields, including: Psychology: Correlational research is commonly used in psychology to explore the relationships between variables such as personality traits, behaviors, and mental health outcomes. For example, researchers may use correlational research to examine the ...
A Correlational Study Exploring the Possible Link Between Instructional Program Coherence and Student Achievement in North Carolina Middle Schools Dustin Johnson . A dissertation submitted to the faculty of the University of North Carolina at Chapel Hill in partial fulfillment of the requirements for the degree of Doctorate of Education in the
Correlational research is a type of non-experimental research in which the researcher measures two variables and assesses the statistical relationship (i.e., the correlation) between them with little or no effort to control extraneous variables. There are many reasons that researchers interested in statistical relationships between variables ...
What Is Correlational Research? Correlational research is a type of nonexperimental research in which the researcher measures two variables and assesses the statistical relationship (i.e., the correlation) between them with little or no effort to control extraneous variables. There are essentially two reasons that researchers interested in ...
This study examines teacher efficacy within the context of professional development to understand the relationship between teacher efficacy and teacher collaboration. Two theoretical frameworks framed this teacher efficacy study based on locus of control and social cognitive theory. A 29-item questionnaire was e-mailed to approximately 500 K-5
Mainly three types of correlational research have been identified: 1. Positive correlation:A positive relationship between two variables is when an increase in one variable leads to a rise in the other variable. A decrease in one variable will see a reduction in the other variable. For example, the amount of money a person has might positively ...
This dissertation was submitted by Danielle Simmons Banister under the direction of the persons listed below. It was submitted to the Gardner-Webb University School of ... A Correlational Study of Teacher Effectiveness: Evaluation Instrument and Value-Added Model. Banister, Danielle Simmons, 2015: Dissertation, Gardner-Webb University,
This Dissertation is brought to you for free and open access by the Walden Dissertations and Doctoral Studies Collection at ScholarWorks. It has been ... correlational study was to improve the current understanding of the relationships among project managers' project management experience, self-efficacy, and project success, for ...
Correlational studies, therefore, are distinct from true experimental designs where researchers randomly assign. participants to groups and provide appropriate control to hold all other variables ...
A correlational study is a type of research design that looks at the relationships between two or more variables. Correlational studies are non-experimental, which means that the experimenter does not manipulate or control any of the variables. A correlation refers to a relationship between two variables. Correlations can be strong or weak and ...
A descriptive correlational study was designed to study a convenience sample of FENs who met the following criteria: male or female nurse over the age of 18, born and completed their education in a foreign country, currently working full or part-time as a nurse for less than two years in the US.
The text, examples, and self-tests in this chapter will enable the reader to: 1. Explain the advantages and challenges of correlational studies using routinely collected data. 2. Given a description of correlational study, offer an alternative explanation to a direct causal relation between variables. 3.
The document is a proposal for doctoral research at the School of Advanced Studies of the University of Phoenix. The purpose of the quantitative correlational study is to profile the leadership of ...
A DESCRIPTIVE CORRELATIONAL STUDY EXAMINING THE RELATIONSHIP OF EMERGENCY DEPARTMENT CONTEXTUAL FACTORS AND TRANSFER INTERVAL TO AN INTERMEDIATE UNIT A thesis submitted in partial fulfillment of the requirements for the degree of Master of Science in Nursing By MARY KATHERINE TANKERSLEY B.S.N. Wright State University, 2003 2015
A Correlational Study of Manager-Employee Relationship, Employee Rewards, and Employee Engagement Hilda M. Concepcion ... This Dissertation is brought to you for free and open access by the Walden Dissertations and Doctoral Studies Collection at ScholarWorks. It has been accepted for inclusion in Walden Dissertations and Doctoral Studies by an
The purpose of this study was to determine the relationship between physical activity levels, physical self-worth, and its sub-domains; (a) skill, (b) body attractiveness, (c) fitness and conditioning, and (d) strength, and overall global self-worth in high school students. One-Hundred and sixty-seven students (male = 66;female = 101) from the western United States, ages 13-18, participated in ...
This study employed a correlational approach to analyze the answers of 143 psychology students from a particular institution in Metro Manila. According to the results of the Mental Health of the ...
Penn Global has announced the first recipients of the newly established Penn Global Dissertation Grants program, providing as much as $8,000 each in funding to 11 Ph.D. students across four schools. With dissertations ranging from examinations of artificial intelligence and computational immunology in Vietnam to a look at the intersection of women, food, and freedom in the Dominican Republic ...
Add to Calendar 2024-06-27 15:00:00 2024-06-27 16:00:00 Haoyun Yang Dissertation Defense Haoyun Yang, PhD Dissertation Defense Title: Structure and dynamics study of RNA and RNA binding proteins Location: Cunz Hall room 180 and on Zoom.Division: BiologicalAdvisor: Mark Foster 180 Cunz Hall and on Zoom Department of Chemistry and Biochemistry [email protected] America/New_York public
When the correlation coefficient value exceeds 0.8, strong correlation between factors exists, necessitating exclusion. The calculation results reveal a high absolute value of 0.93 for the Pearson correlation coefficient between slope and terrain roughness, likely due to the steep terrain characteristics of the study area.
Utilizing cross-correlation spectroscopy, we have successfully demonstrated the robust consistency between our simulation study and real-time observations for both planets. ANDES excels overall in molecular detection due to its enhanced instrumental architecture, reinforcing E-ELT's importance for studying exoplanet atmospheres. ...
The rock gardens had covered up to 21.1 square kilometers (8.1 square miles) and could have sustained up to 17,000 people, previous research suggested.That February 2013 finding bolstered the idea ...
A collaborative study between the University of Bristol and The Wave, released on June 15, 2024, has unveiled significant benefits of surfing on both physical health and mental well-being. The UK ...