
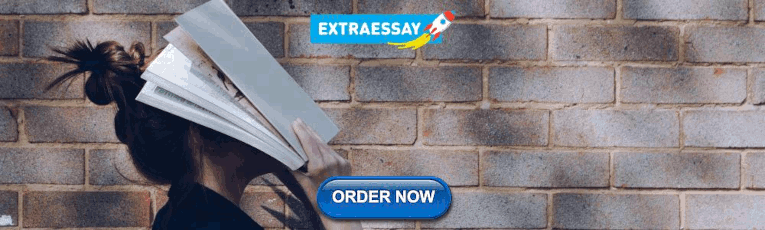
Research Topics & Ideas: Data Science
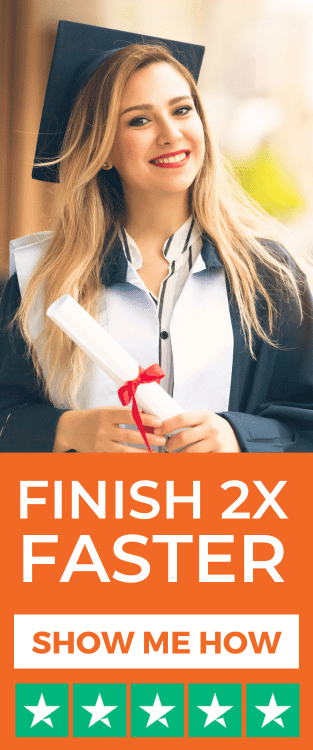
PS – This is just the start…
We know it’s exciting to run through a list of research topics, but please keep in mind that this list is just a starting point . These topic ideas provided here are intentionally broad and generic , so keep in mind that you will need to develop them further. Nevertheless, they should inspire some ideas for your project.
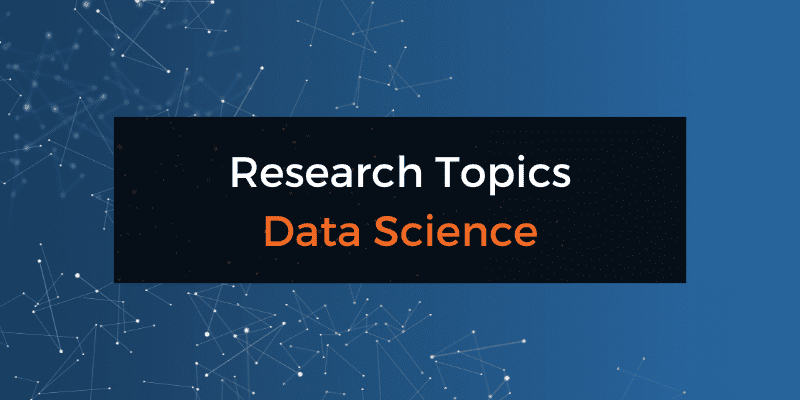
Data Science-Related Research Topics
- Developing machine learning models for real-time fraud detection in online transactions.
- The use of big data analytics in predicting and managing urban traffic flow.
- Investigating the effectiveness of data mining techniques in identifying early signs of mental health issues from social media usage.
- The application of predictive analytics in personalizing cancer treatment plans.
- Analyzing consumer behavior through big data to enhance retail marketing strategies.
- The role of data science in optimizing renewable energy generation from wind farms.
- Developing natural language processing algorithms for real-time news aggregation and summarization.
- The application of big data in monitoring and predicting epidemic outbreaks.
- Investigating the use of machine learning in automating credit scoring for microfinance.
- The role of data analytics in improving patient care in telemedicine.
- Developing AI-driven models for predictive maintenance in the manufacturing industry.
- The use of big data analytics in enhancing cybersecurity threat intelligence.
- Investigating the impact of sentiment analysis on brand reputation management.
- The application of data science in optimizing logistics and supply chain operations.
- Developing deep learning techniques for image recognition in medical diagnostics.
- The role of big data in analyzing climate change impacts on agricultural productivity.
- Investigating the use of data analytics in optimizing energy consumption in smart buildings.
- The application of machine learning in detecting plagiarism in academic works.
- Analyzing social media data for trends in political opinion and electoral predictions.
- The role of big data in enhancing sports performance analytics.
- Developing data-driven strategies for effective water resource management.
- The use of big data in improving customer experience in the banking sector.
- Investigating the application of data science in fraud detection in insurance claims.
- The role of predictive analytics in financial market risk assessment.
- Developing AI models for early detection of network vulnerabilities.
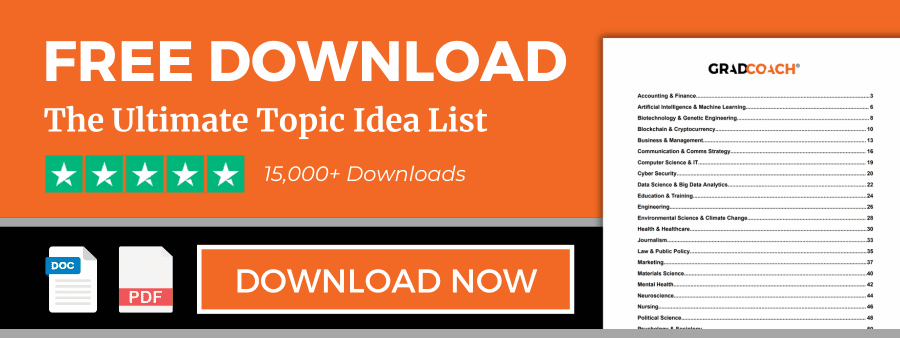
Data Science Research Ideas (Continued)
- The application of big data in public transportation systems for route optimization.
- Investigating the impact of big data analytics on e-commerce recommendation systems.
- The use of data mining techniques in understanding consumer preferences in the entertainment industry.
- Developing predictive models for real estate pricing and market trends.
- The role of big data in tracking and managing environmental pollution.
- Investigating the use of data analytics in improving airline operational efficiency.
- The application of machine learning in optimizing pharmaceutical drug discovery.
- Analyzing online customer reviews to inform product development in the tech industry.
- The role of data science in crime prediction and prevention strategies.
- Developing models for analyzing financial time series data for investment strategies.
- The use of big data in assessing the impact of educational policies on student performance.
- Investigating the effectiveness of data visualization techniques in business reporting.
- The application of data analytics in human resource management and talent acquisition.
- Developing algorithms for anomaly detection in network traffic data.
- The role of machine learning in enhancing personalized online learning experiences.
- Investigating the use of big data in urban planning and smart city development.
- The application of predictive analytics in weather forecasting and disaster management.
- Analyzing consumer data to drive innovations in the automotive industry.
- The role of data science in optimizing content delivery networks for streaming services.
- Developing machine learning models for automated text classification in legal documents.
- The use of big data in tracking global supply chain disruptions.
- Investigating the application of data analytics in personalized nutrition and fitness.
- The role of big data in enhancing the accuracy of geological surveying for natural resource exploration.
- Developing predictive models for customer churn in the telecommunications industry.
- The application of data science in optimizing advertisement placement and reach.
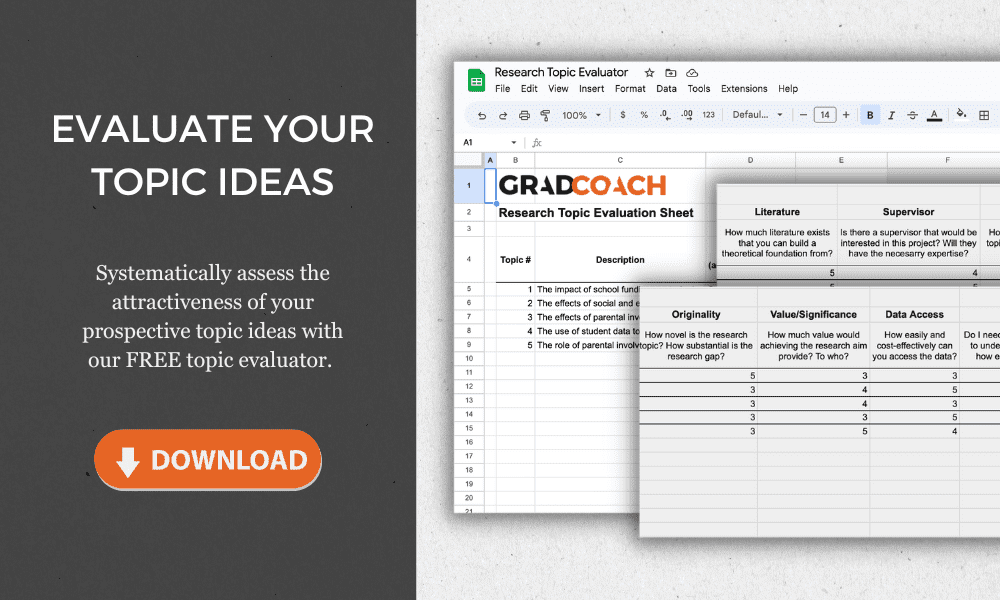
Recent Data Science-Related Studies
While the ideas we’ve presented above are a decent starting point for finding a research topic, they are fairly generic and non-specific. So, it helps to look at actual studies in the data science and analytics space to see how this all comes together in practice.
Below, we’ve included a selection of recent studies to help refine your thinking. These are actual studies, so they can provide some useful insight as to what a research topic looks like in practice.
- Data Science in Healthcare: COVID-19 and Beyond (Hulsen, 2022)
- Auto-ML Web-application for Automated Machine Learning Algorithm Training and evaluation (Mukherjee & Rao, 2022)
- Survey on Statistics and ML in Data Science and Effect in Businesses (Reddy et al., 2022)
- Visualization in Data Science VDS @ KDD 2022 (Plant et al., 2022)
- An Essay on How Data Science Can Strengthen Business (Santos, 2023)
- A Deep study of Data science related problems, application and machine learning algorithms utilized in Data science (Ranjani et al., 2022)
- You Teach WHAT in Your Data Science Course?!? (Posner & Kerby-Helm, 2022)
- Statistical Analysis for the Traffic Police Activity: Nashville, Tennessee, USA (Tufail & Gul, 2022)
- Data Management and Visual Information Processing in Financial Organization using Machine Learning (Balamurugan et al., 2022)
- A Proposal of an Interactive Web Application Tool QuickViz: To Automate Exploratory Data Analysis (Pitroda, 2022)
- Applications of Data Science in Respective Engineering Domains (Rasool & Chaudhary, 2022)
- Jupyter Notebooks for Introducing Data Science to Novice Users (Fruchart et al., 2022)
- Towards a Systematic Review of Data Science Programs: Themes, Courses, and Ethics (Nellore & Zimmer, 2022)
- Application of data science and bioinformatics in healthcare technologies (Veeranki & Varshney, 2022)
- TAPS Responsibility Matrix: A tool for responsible data science by design (Urovi et al., 2023)
- Data Detectives: A Data Science Program for Middle Grade Learners (Thompson & Irgens, 2022)
- MACHINE LEARNING FOR NON-MAJORS: A WHITE BOX APPROACH (Mike & Hazzan, 2022)
- COMPONENTS OF DATA SCIENCE AND ITS APPLICATIONS (Paul et al., 2022)
- Analysis on the Application of Data Science in Business Analytics (Wang, 2022)
As you can see, these research topics are a lot more focused than the generic topic ideas we presented earlier. So, for you to develop a high-quality research topic, you’ll need to get specific and laser-focused on a specific context with specific variables of interest. In the video below, we explore some other important things you’ll need to consider when crafting your research topic.
Get 1-On-1 Help
If you’re still unsure about how to find a quality research topic, check out our Private Coaching service, the perfect starting point for developing a unique, well-justified research topic.
Find The Perfect Research Topic
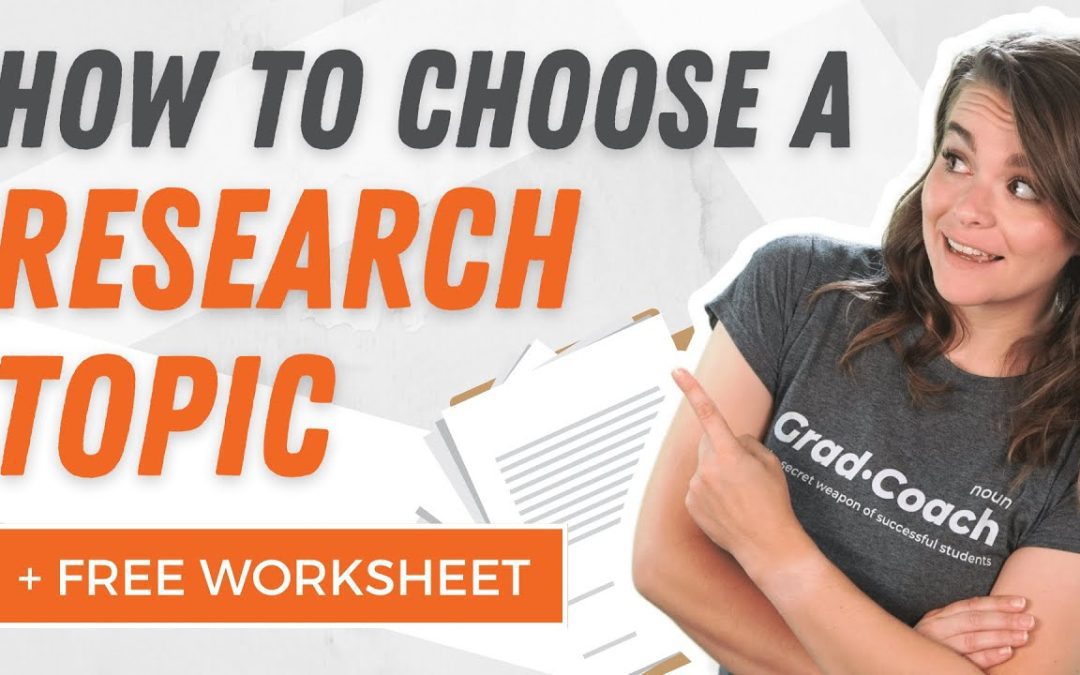
How To Choose A Research Topic: 5 Key Criteria
How To Choose A Research Topic Step-By-Step Tutorial With Examples + Free Topic...
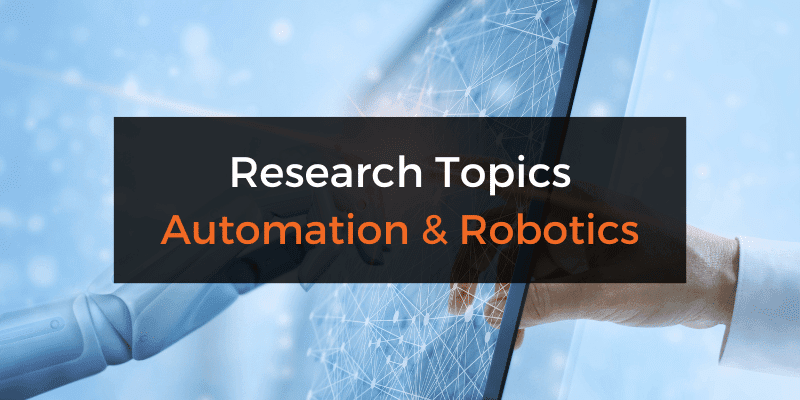
Research Topics & Ideas: Automation & Robotics
A comprehensive list of automation and robotics-related research topics. Includes free access to a webinar and research topic evaluator.
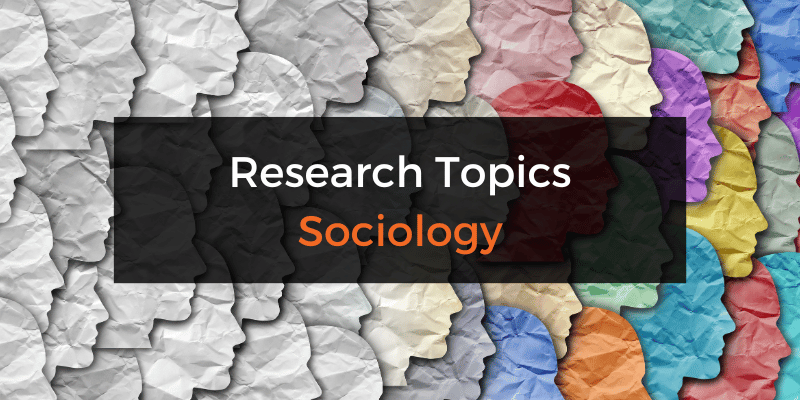
Research Topics & Ideas: Sociology
A comprehensive list of sociology-related research topics. Includes free access to a webinar and research topic evaluator.
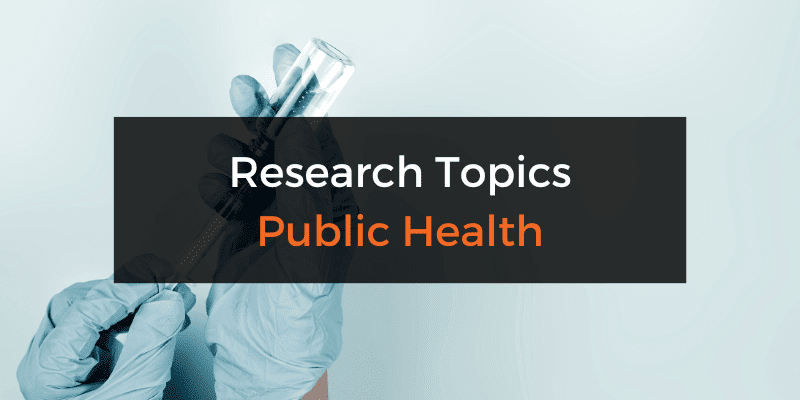
Research Topics & Ideas: Public Health & Epidemiology
A comprehensive list of public health-related research topics. Includes free access to a webinar and research topic evaluator.
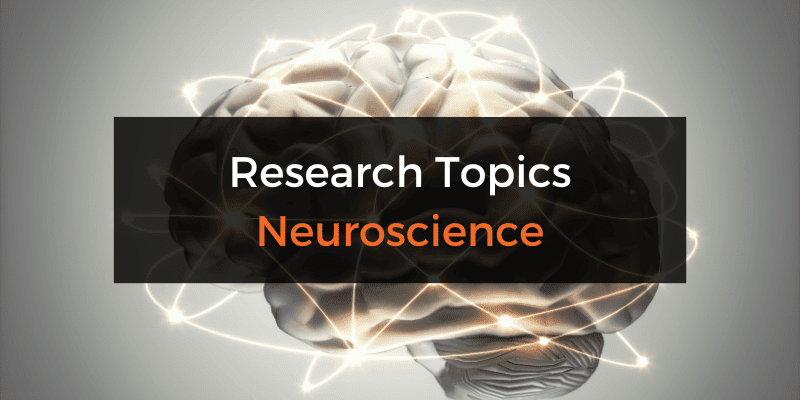
Research Topics & Ideas: Neuroscience
Research Topics & Ideas: Neuroscience 50 Topic Ideas To Kickstart Your Research...
📄 FREE TEMPLATES
Research Topic Ideation
Proposal Writing
Literature Review
Methodology & Analysis
Academic Writing
Referencing & Citing
Apps, Tools & Tricks
The Grad Coach Podcast
I have to submit dissertation. can I get any help
Submit a Comment Cancel reply
Your email address will not be published. Required fields are marked *
Save my name, email, and website in this browser for the next time I comment.
Submit Comment
- Print Friendly
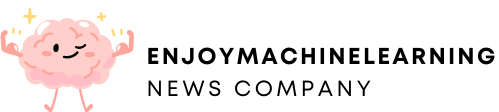
37 Research Topics In Data Science To Stay On Top Of
- February 22, 2024
As a data scientist, staying on top of the latest research in your field is essential.
The data science landscape changes rapidly, and new techniques and tools are constantly being developed.
To keep up with the competition, you need to be aware of the latest trends and topics in data science research.
In this article, we will provide an overview of 37 hot research topics in data science.
We will discuss each topic in detail, including its significance and potential applications.
These topics could be an idea for a thesis or simply topics you can research independently.
Stay tuned – this is one blog post you don’t want to miss!
37 Research Topics in Data Science
1.) predictive modeling.
Predictive modeling is a significant portion of data science and a topic you must be aware of.
Simply put, it is the process of using historical data to build models that can predict future outcomes.
Predictive modeling has many applications, from marketing and sales to financial forecasting and risk management.
As businesses increasingly rely on data to make decisions, predictive modeling is becoming more and more important.
While it can be complex, predictive modeling is a powerful tool that gives businesses a competitive advantage.
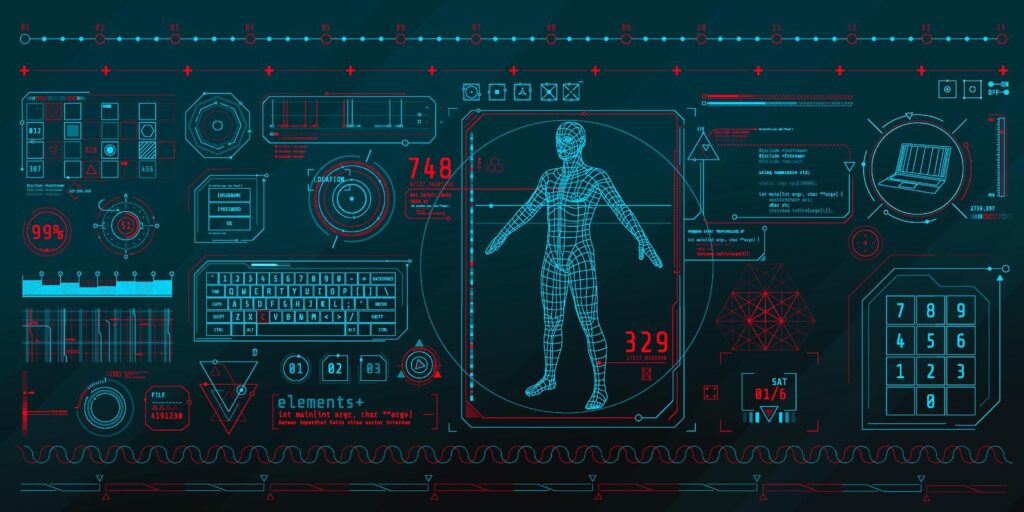
2.) Big Data Analytics
These days, it seems like everyone is talking about big data.
And with good reason – organizations of all sizes are sitting on mountains of data, and they’re increasingly turning to data scientists to help them make sense of it all.
But what exactly is big data? And what does it mean for data science?
Simply put, big data is a term used to describe datasets that are too large and complex for traditional data processing techniques.
Big data typically refers to datasets of a few terabytes or more.
But size isn’t the only defining characteristic – big data is also characterized by its high Velocity (the speed at which data is generated), Variety (the different types of data), and Volume (the amount of the information).
Given the enormity of big data, it’s not surprising that organizations are struggling to make sense of it all.
That’s where data science comes in.
Data scientists use various methods to wrangle big data, including distributed computing and other decentralized technologies.
With the help of data science, organizations are beginning to unlock the hidden value in their big data.
By harnessing the power of big data analytics, they can improve their decision-making, better understand their customers, and develop new products and services.
3.) Auto Machine Learning
Auto machine learning is a research topic in data science concerned with developing algorithms that can automatically learn from data without intervention.
This area of research is vital because it allows data scientists to automate the process of writing code for every dataset.
This allows us to focus on other tasks, such as model selection and validation.
Auto machine learning algorithms can learn from data in a hands-off way for the data scientist – while still providing incredible insights.
This makes them a valuable tool for data scientists who either don’t have the skills to do their own analysis or are struggling.
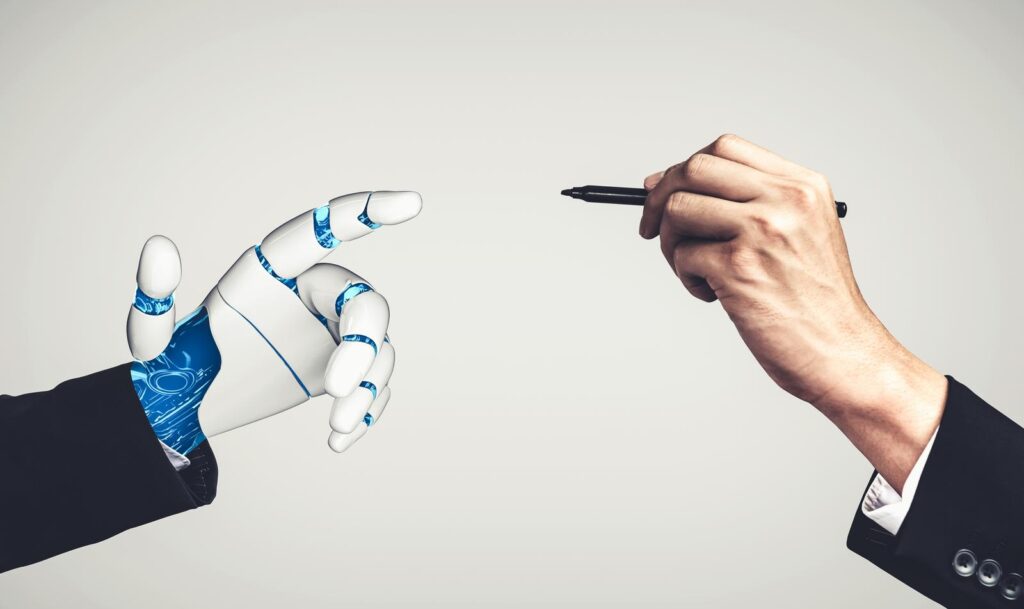
4.) Text Mining
Text mining is a research topic in data science that deals with text data extraction.
This area of research is important because it allows us to get as much information as possible from the vast amount of text data available today.
Text mining techniques can extract information from text data, such as keywords, sentiments, and relationships.
This information can be used for various purposes, such as model building and predictive analytics.
5.) Natural Language Processing
Natural language processing is a data science research topic that analyzes human language data.
This area of research is important because it allows us to understand and make sense of the vast amount of text data available today.
Natural language processing techniques can build predictive and interactive models from any language data.
Natural Language processing is pretty broad, and recent advances like GPT-3 have pushed this topic to the forefront.
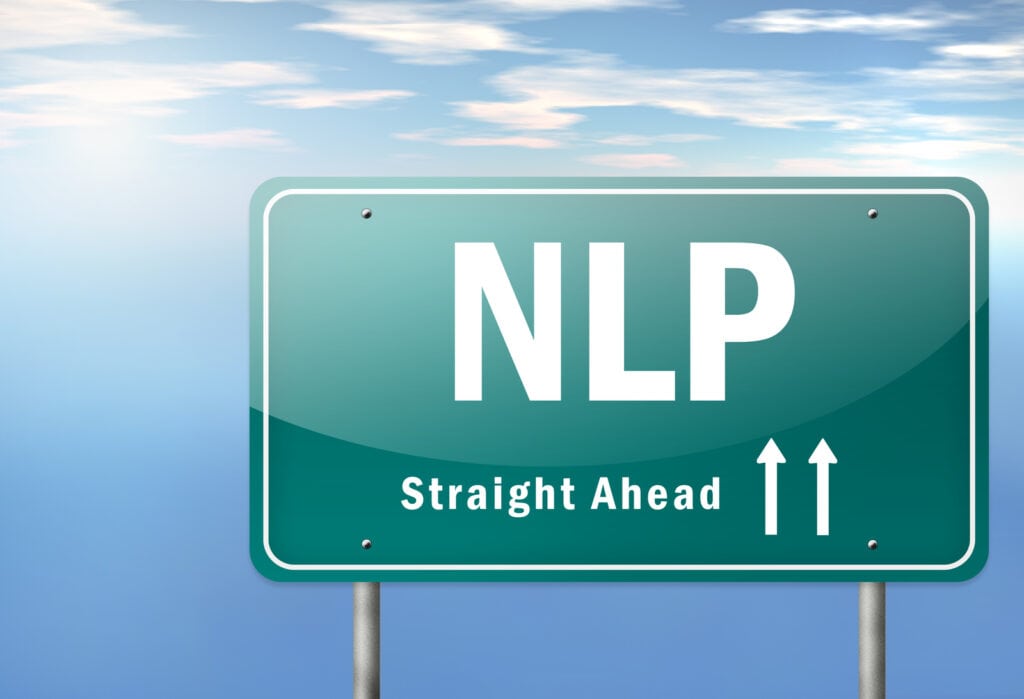
6.) Recommender Systems
Recommender systems are an exciting topic in data science because they allow us to make better products, services, and content recommendations.
Businesses can better understand their customers and their needs by using recommender systems.
This, in turn, allows them to develop better products and services that meet the needs of their customers.
Recommender systems are also used to recommend content to users.
This can be done on an individual level or at a group level.
Think about Netflix, for example, always knowing what you want to watch!
Recommender systems are a valuable tool for businesses and users alike.
7.) Deep Learning
Deep learning is a research topic in data science that deals with artificial neural networks.
These networks are composed of multiple layers, and each layer is formed from various nodes.
Deep learning networks can learn from data similarly to how humans learn, irrespective of the data distribution.
This makes them a valuable tool for data scientists looking to build models that can learn from data independently.
The deep learning network has become very popular in recent years because of its ability to achieve state-of-the-art results on various tasks.
There seems to be a new SOTA deep learning algorithm research paper on https://arxiv.org/ every single day!
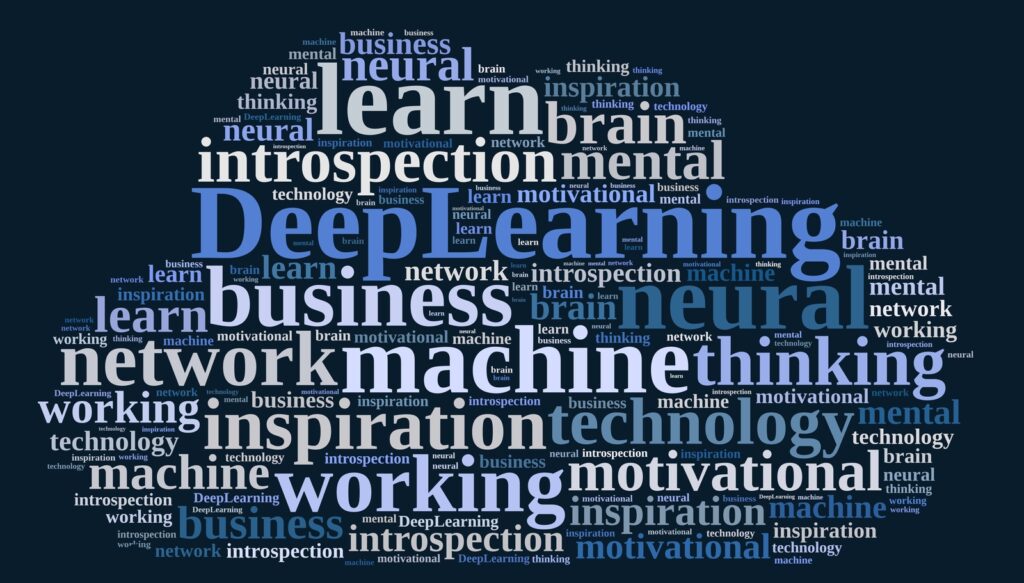
8.) Reinforcement Learning
Reinforcement learning is a research topic in data science that deals with algorithms that can learn on multiple levels from interactions with their environment.
This area of research is essential because it allows us to develop algorithms that can learn non-greedy approaches to decision-making, allowing businesses and companies to win in the long term compared to the short.
9.) Data Visualization
Data visualization is an excellent research topic in data science because it allows us to see our data in a way that is easy to understand.
Data visualization techniques can be used to create charts, graphs, and other visual representations of data.
This allows us to see the patterns and trends hidden in our data.
Data visualization is also used to communicate results to others.
This allows us to share our findings with others in a way that is easy to understand.
There are many ways to contribute to and learn about data visualization.
Some ways include attending conferences, reading papers, and contributing to open-source projects.
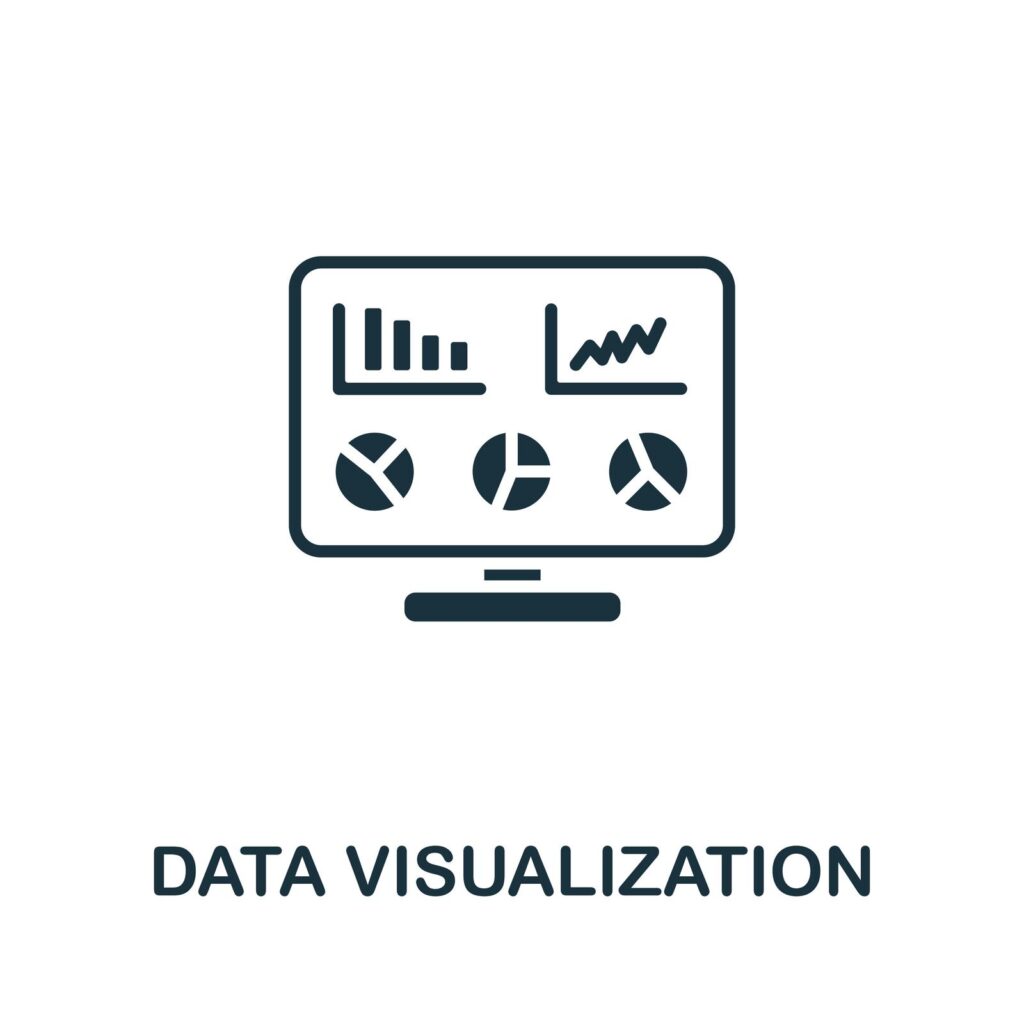
10.) Predictive Maintenance
Predictive maintenance is a hot topic in data science because it allows us to prevent failures before they happen.
This is done using data analytics to predict when a failure will occur.
This allows us to take corrective action before the failure actually happens.
While this sounds simple, avoiding false positives while keeping recall is challenging and an area wide open for advancement.
11.) Financial Analysis
Financial analysis is an older topic that has been around for a while but is still a great field where contributions can be felt.
Current researchers are focused on analyzing macroeconomic data to make better financial decisions.
This is done by analyzing the data to identify trends and patterns.
Financial analysts can use this information to make informed decisions about where to invest their money.
Financial analysis is also used to predict future economic trends.
This allows businesses and individuals to prepare for potential financial hardships and enable companies to be cash-heavy during good economic conditions.
Overall, financial analysis is a valuable tool for anyone looking to make better financial decisions.
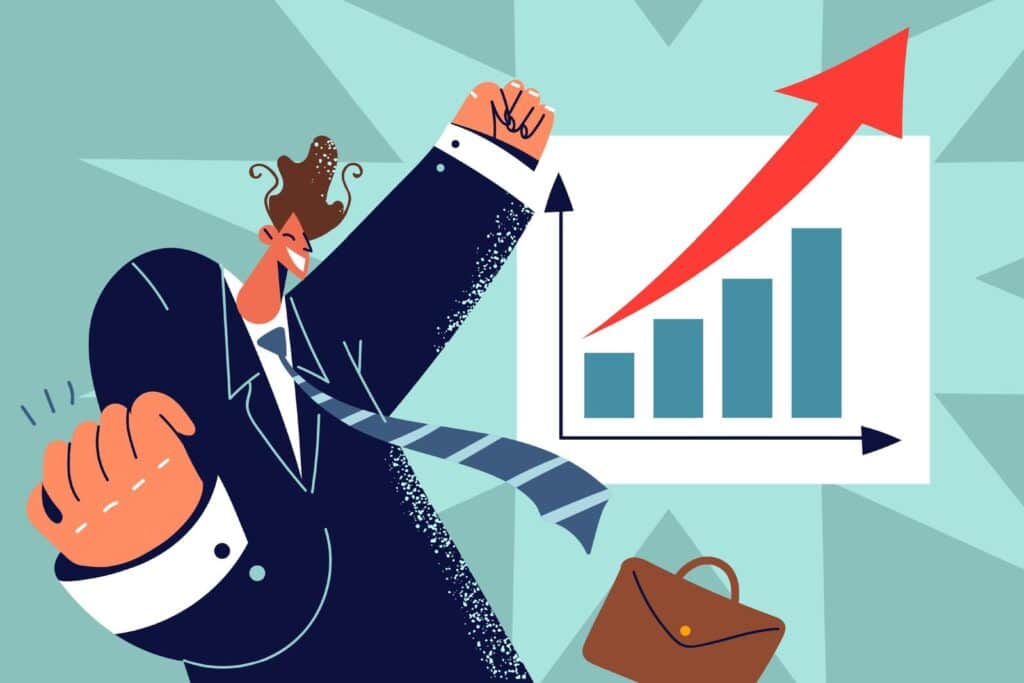
12.) Image Recognition
Image recognition is one of the hottest topics in data science because it allows us to identify objects in images.
This is done using artificial intelligence algorithms that can learn from data and understand what objects you’re looking for.
This allows us to build models that can accurately recognize objects in images and video.
This is a valuable tool for businesses and individuals who want to be able to identify objects in images.
Think about security, identification, routing, traffic, etc.
Image Recognition has gained a ton of momentum recently – for a good reason.
13.) Fraud Detection
Fraud detection is a great topic in data science because it allows us to identify fraudulent activity before it happens.
This is done by analyzing data to look for patterns and trends that may be associated with the fraud.
Once our machine learning model recognizes some of these patterns in real time, it immediately detects fraud.
This allows us to take corrective action before the fraud actually happens.
Fraud detection is a valuable tool for anyone who wants to protect themselves from potential fraudulent activity.
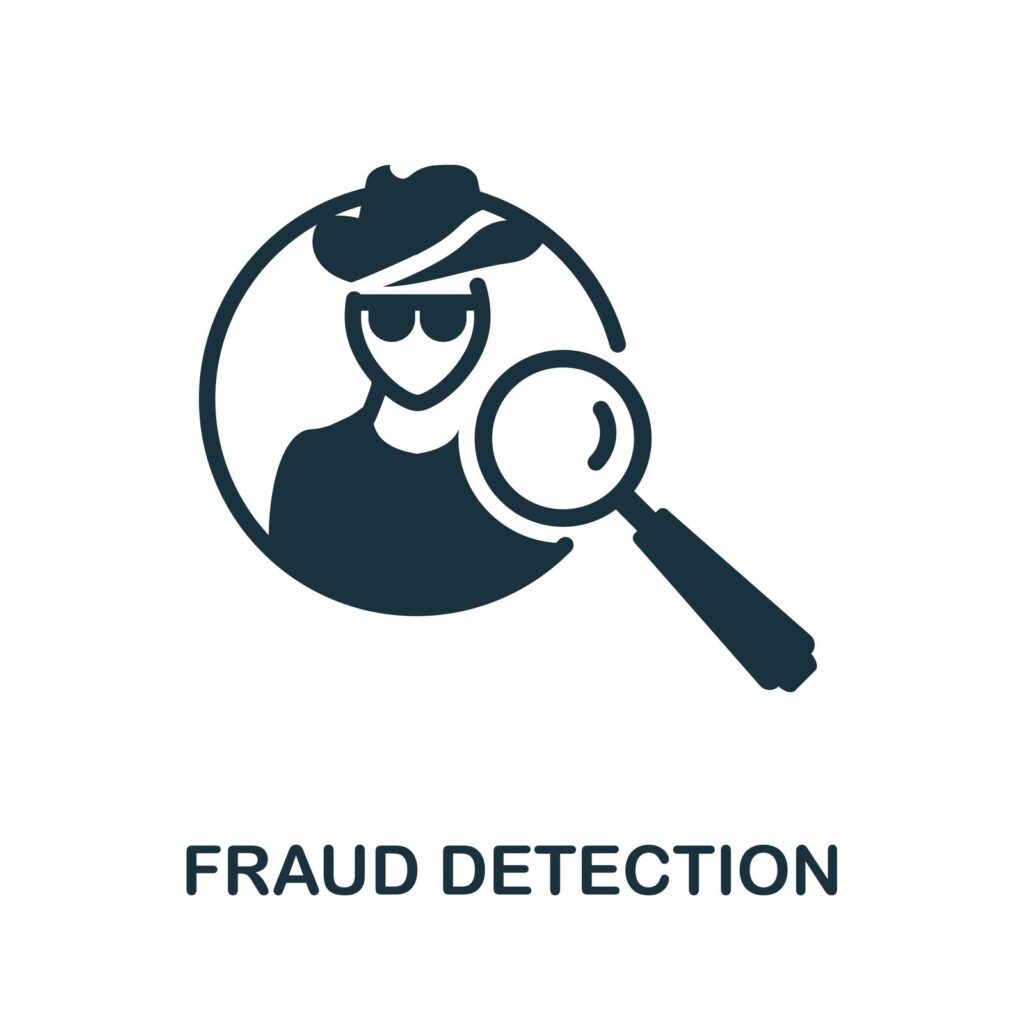
14.) Web Scraping
Web scraping is a controversial topic in data science because it allows us to collect data from the web, which is usually data you do not own.
This is done by extracting data from websites using scraping tools that are usually custom-programmed.
This allows us to collect data that would otherwise be inaccessible.
For obvious reasons, web scraping is a unique tool – giving you data your competitors would have no chance of getting.
I think there is an excellent opportunity to create new and innovative ways to make scraping accessible for everyone, not just those who understand Selenium and Beautiful Soup.
15.) Social Media Analysis
Social media analysis is not new; many people have already created exciting and innovative algorithms to study this.
However, it is still a great data science research topic because it allows us to understand how people interact on social media.
This is done by analyzing data from social media platforms to look for insights, bots, and recent societal trends.
Once we understand these practices, we can use this information to improve our marketing efforts.
For example, if we know that a particular demographic prefers a specific type of content, we can create more content that appeals to them.
Social media analysis is also used to understand how people interact with brands on social media.
This allows businesses to understand better what their customers want and need.
Overall, social media analysis is valuable for anyone who wants to improve their marketing efforts or understand how customers interact with brands.
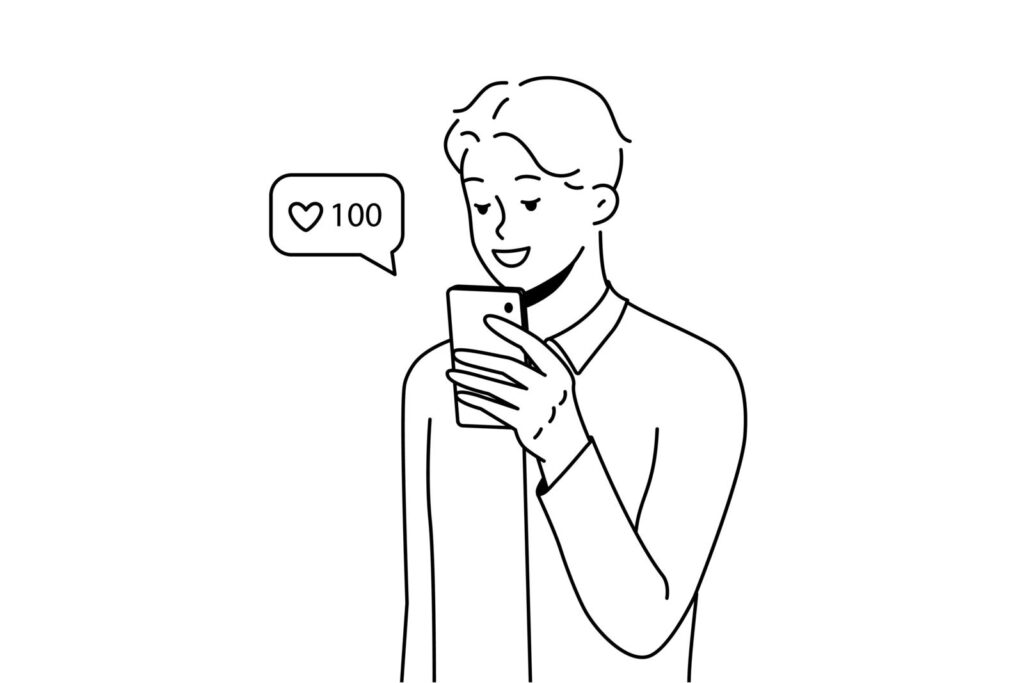
16.) GPU Computing
GPU computing is a fun new research topic in data science because it allows us to process data much faster than traditional CPUs .
Due to how GPUs are made, they’re incredibly proficient at intense matrix operations, outperforming traditional CPUs by very high margins.
While the computation is fast, the coding is still tricky.
There is an excellent research opportunity to bring these innovations to non-traditional modules, allowing data science to take advantage of GPU computing outside of deep learning.
17.) Quantum Computing
Quantum computing is a new research topic in data science and physics because it allows us to process data much faster than traditional computers.
It also opens the door to new types of data.
There are just some problems that can’t be solved utilizing outside of the classical computer.
For example, if you wanted to understand how a single atom moved around, a classical computer couldn’t handle this problem.
You’ll need to utilize a quantum computer to handle quantum mechanics problems.
This may be the “hottest” research topic on the planet right now, with some of the top researchers in computer science and physics worldwide working on it.
You could be too.
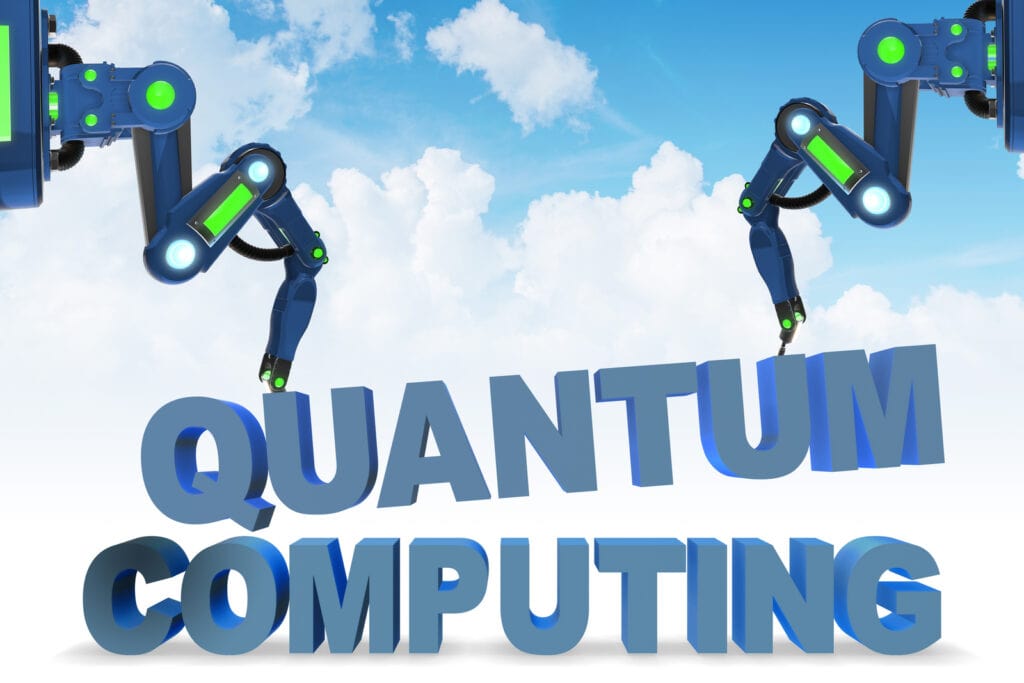
18.) Genomics
Genomics may be the only research topic that can compete with quantum computing regarding the “number of top researchers working on it.”
Genomics is a fantastic intersection of data science because it allows us to understand how genes work.
This is done by sequencing the DNA of different organisms to look for insights into our and other species.
Once we understand these patterns, we can use this information to improve our understanding of diseases and create new and innovative treatments for them.
Genomics is also used to study the evolution of different species.
Genomics is the future and a field begging for new and exciting research professionals to take it to the next step.
19.) Location-based services
Location-based services are an old and time-tested research topic in data science.
Since GPS and 4g cell phone reception became a thing, we’ve been trying to stay informed about how humans interact with their environment.
This is done by analyzing data from GPS tracking devices, cell phone towers, and Wi-Fi routers to look for insights into how humans interact.
Once we understand these practices, we can use this information to improve our geotargeting efforts, improve maps, find faster routes, and improve cohesion throughout a community.
Location-based services are used to understand the user, something every business could always use a little bit more of.
While a seemingly “stale” field, location-based services have seen a revival period with self-driving cars.
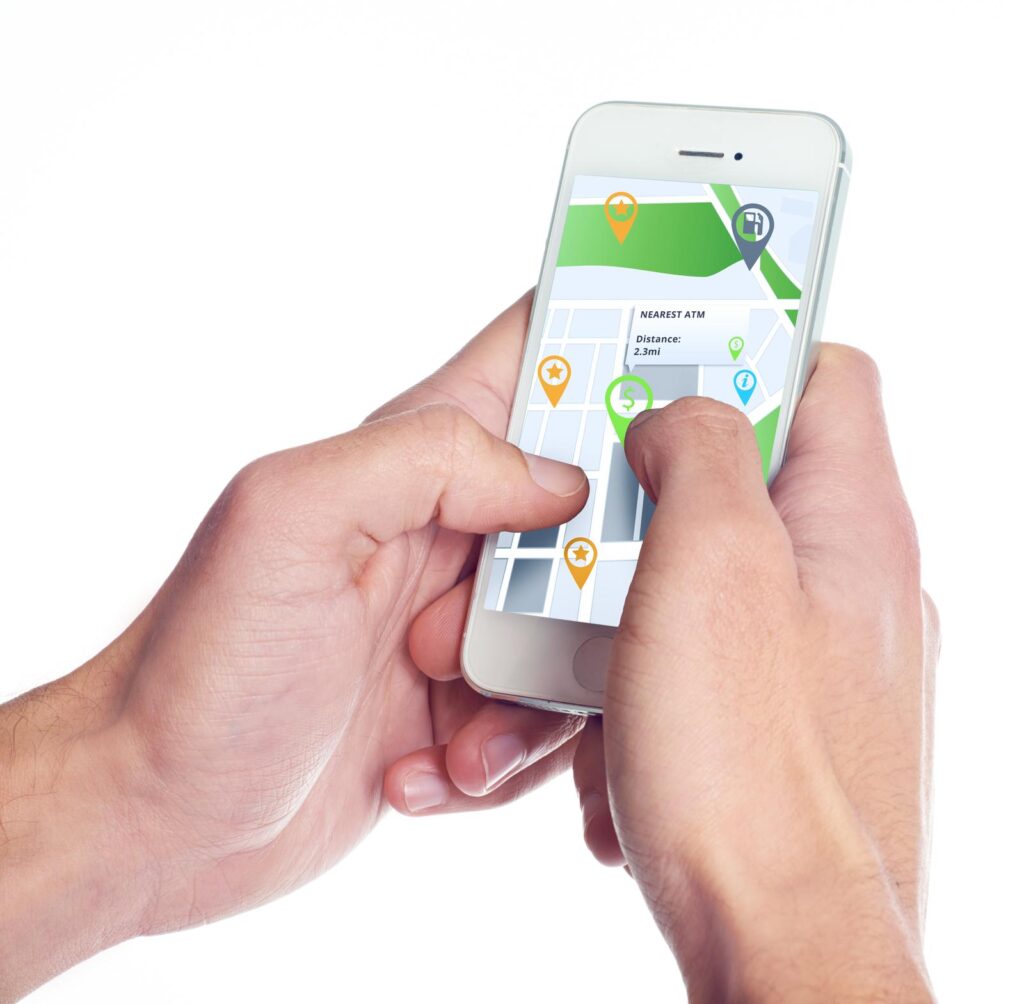
20.) Smart City Applications
Smart city applications are all the rage in data science research right now.
By harnessing the power of data, cities can become more efficient and sustainable.
But what exactly are smart city applications?
In short, they are systems that use data to improve city infrastructure and services.
This can include anything from traffic management and energy use to waste management and public safety.
Data is collected from various sources, including sensors, cameras, and social media.
It is then analyzed to identify tendencies and habits.
This information can make predictions about future needs and optimize city resources.
As more and more cities strive to become “smart,” the demand for data scientists with expertise in smart city applications is only growing.
21.) Internet Of Things (IoT)
The Internet of Things, or IoT, is exciting and new data science and sustainability research topic.
IoT is a network of physical objects embedded with sensors and connected to the internet.
These objects can include everything from alarm clocks to refrigerators; they’re all connected to the internet.
That means that they can share data with computers.
And that’s where data science comes in.
Data scientists are using IoT data to learn everything from how people use energy to how traffic flows through a city.
They’re also using IoT data to predict when an appliance will break down or when a road will be congested.
Really, the possibilities are endless.
With such a wide-open field, it’s easy to see why IoT is being researched by some of the top professionals in the world.
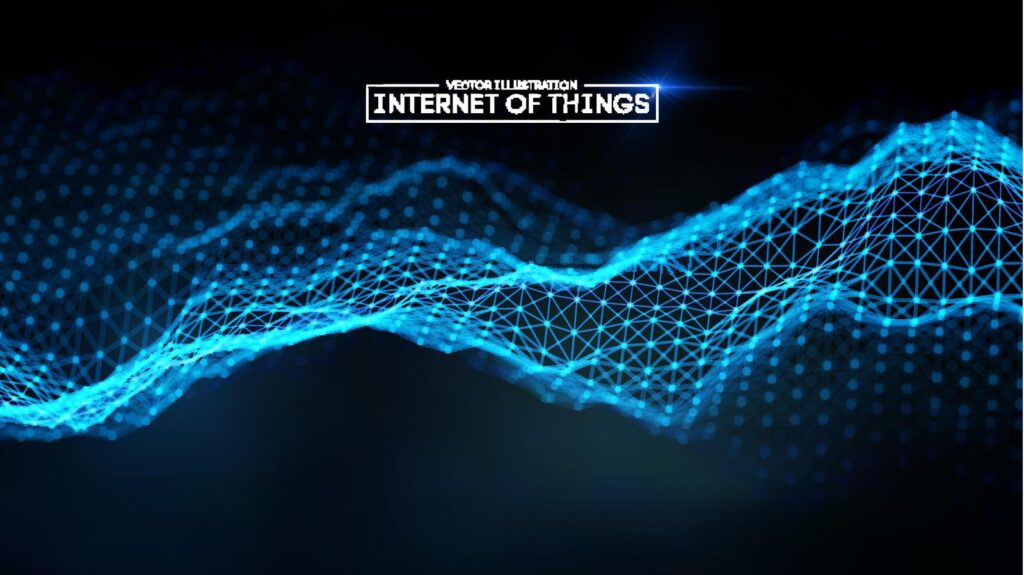
22.) Cybersecurity
Cybersecurity is a relatively new research topic in data science and in general, but it’s already garnering a lot of attention from businesses and organizations.
After all, with the increasing number of cyber attacks in recent years, it’s clear that we need to find better ways to protect our data.
While most of cybersecurity focuses on infrastructure, data scientists can leverage historical events to find potential exploits to protect their companies.
Sometimes, looking at a problem from a different angle helps, and that’s what data science brings to cybersecurity.
Also, data science can help to develop new security technologies and protocols.
As a result, cybersecurity is a crucial data science research area and one that will only become more important in the years to come.
23.) Blockchain
Blockchain is an incredible new research topic in data science for several reasons.
First, it is a distributed database technology that enables secure, transparent, and tamper-proof transactions.
Did someone say transmitting data?
This makes it an ideal platform for tracking data and transactions in various industries.
Second, blockchain is powered by cryptography, which not only makes it highly secure – but is a familiar foe for data scientists.
Finally, blockchain is still in its early stages of development, so there is much room for research and innovation.
As a result, blockchain is a great new research topic in data science that vows to revolutionize how we store, transmit and manage data.
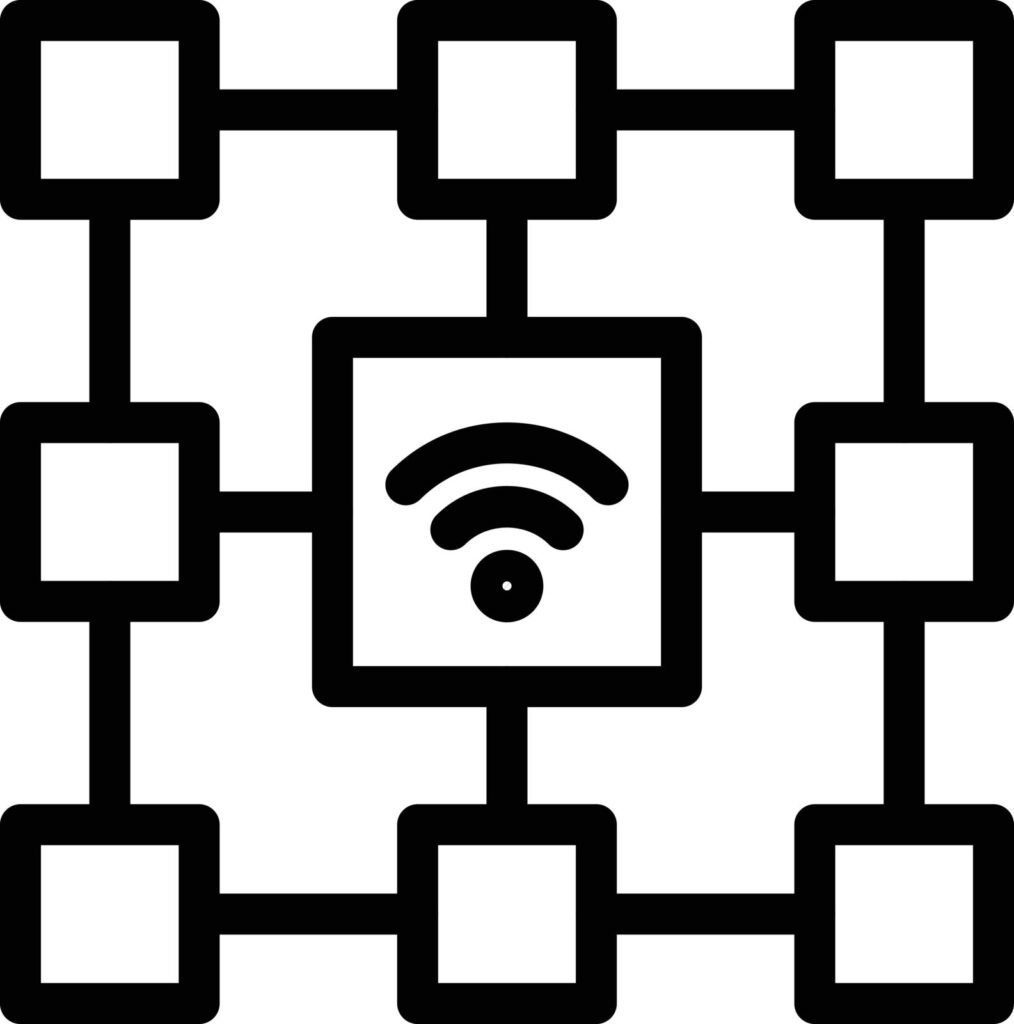
24.) Sustainability
Sustainability is a relatively new research topic in data science, but it is gaining traction quickly.
To keep up with this demand, The Wharton School of the University of Pennsylvania has started to offer an MBA in Sustainability .
This demand isn’t shocking, and some of the reasons include the following:
Sustainability is an important issue that is relevant to everyone.
Datasets on sustainability are constantly growing and changing, making it an exciting challenge for data scientists.
There hasn’t been a “set way” to approach sustainability from a data perspective, making it an excellent opportunity for interdisciplinary research.
As data science grows, sustainability will likely become an increasingly important research topic.
25.) Educational Data
Education has always been a great topic for research, and with the advent of big data, educational data has become an even richer source of information.
By studying educational data, researchers can gain insights into how students learn, what motivates them, and what barriers these students may face.
Besides, data science can be used to develop educational interventions tailored to individual students’ needs.
Imagine being the researcher that helps that high schooler pass mathematics; what an incredible feeling.
With the increasing availability of educational data, data science has enormous potential to improve the quality of education.
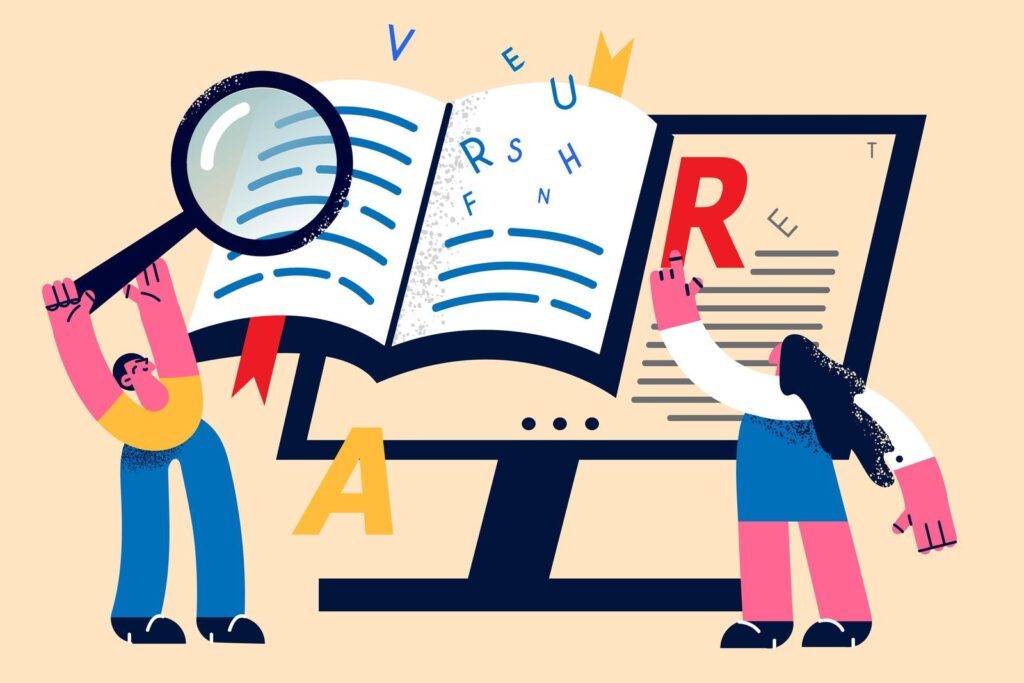
26.) Politics
As data science continues to evolve, so does the scope of its applications.
Originally used primarily for business intelligence and marketing, data science is now applied to various fields, including politics.
By analyzing large data sets, political scientists (data scientists with a cooler name) can gain valuable insights into voting patterns, campaign strategies, and more.
Further, data science can be used to forecast election results and understand the effects of political events on public opinion.
With the wealth of data available, there is no shortage of research opportunities in this field.
As data science evolves, so does our understanding of politics and its role in our world.
27.) Cloud Technologies
Cloud technologies are a great research topic.
It allows for the outsourcing and sharing of computer resources and applications all over the internet.
This lets organizations save money on hardware and maintenance costs while providing employees access to the latest and greatest software and applications.
I believe there is an argument that AWS could be the greatest and most technologically advanced business ever built (Yes, I know it’s only part of the company).
Besides, cloud technologies can help improve team members’ collaboration by allowing them to share files and work on projects together in real-time.
As more businesses adopt cloud technologies, data scientists must stay up-to-date on the latest trends in this area.
By researching cloud technologies, data scientists can help organizations to make the most of this new and exciting technology.
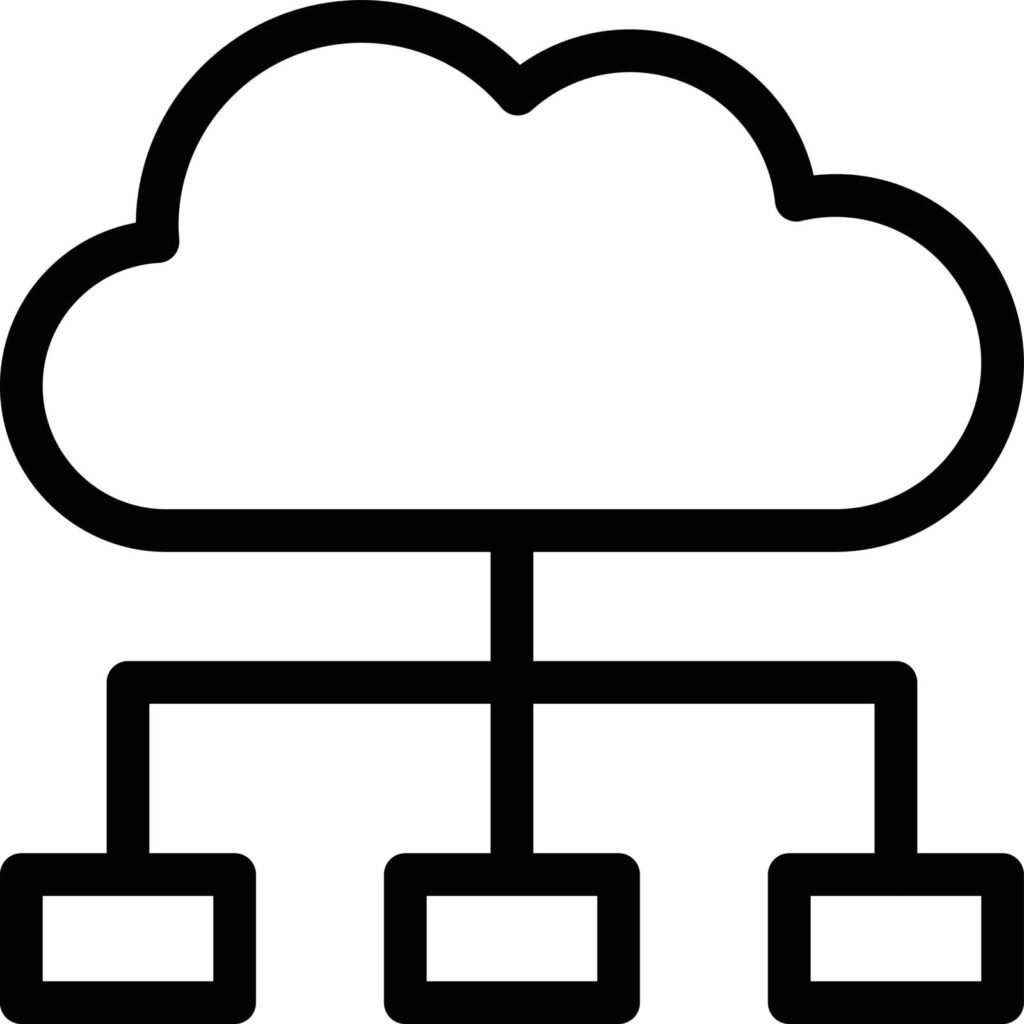
28.) Robotics
Robotics has recently become a household name, and it’s for a good reason.
First, robotics deals with controlling and planning physical systems, an inherently complex problem.
Second, robotics requires various sensors and actuators to interact with the world, making it an ideal application for machine learning techniques.
Finally, robotics is an interdisciplinary field that draws on various disciplines, such as computer science, mechanical engineering, and electrical engineering.
As a result, robotics is a rich source of research problems for data scientists.
29.) HealthCare
Healthcare is an industry that is ripe for data-driven innovation.
Hospitals, clinics, and health insurance companies generate a tremendous amount of data daily.
This data can be used to improve the quality of care and outcomes for patients.
This is perfect timing, as the healthcare industry is undergoing a significant shift towards value-based care, which means there is a greater need than ever for data-driven decision-making.
As a result, healthcare is an exciting new research topic for data scientists.
There are many different ways in which data can be used to improve healthcare, and there is a ton of room for newcomers to make discoveries.
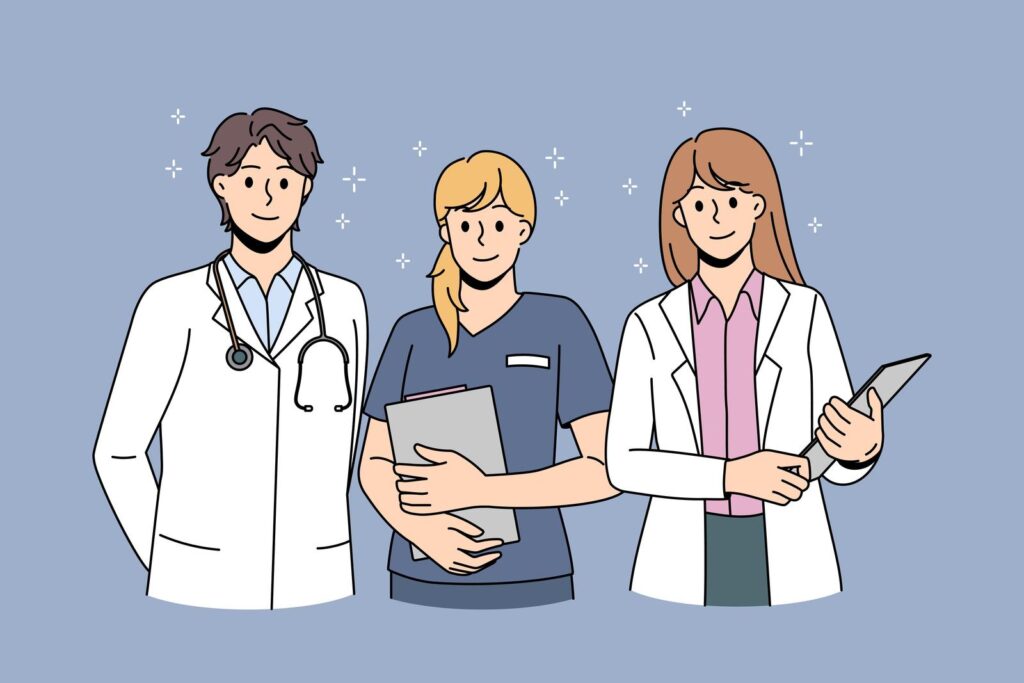
30.) Remote Work
There’s no doubt that remote work is on the rise.
In today’s global economy, more and more businesses are allowing their employees to work from home or anywhere else they can get a stable internet connection.
But what does this mean for data science? Well, for one thing, it opens up a whole new field of research.
For example, how does remote work impact employee productivity?
What are the best ways to manage and collaborate on data science projects when team members are spread across the globe?
And what are the cybersecurity risks associated with working remotely?
These are just a few of the questions that data scientists will be able to answer with further research.
So if you’re looking for a new topic to sink your teeth into, remote work in data science is a great option.
31.) Data-Driven Journalism
Data-driven journalism is an exciting new field of research that combines the best of both worlds: the rigor of data science with the creativity of journalism.
By applying data analytics to large datasets, journalists can uncover stories that would otherwise be hidden.
And telling these stories compellingly can help people better understand the world around them.
Data-driven journalism is still in its infancy, but it has already had a major impact on how news is reported.
In the future, it will only become more important as data becomes increasingly fluid among journalists.
It is an exciting new topic and research field for data scientists to explore.
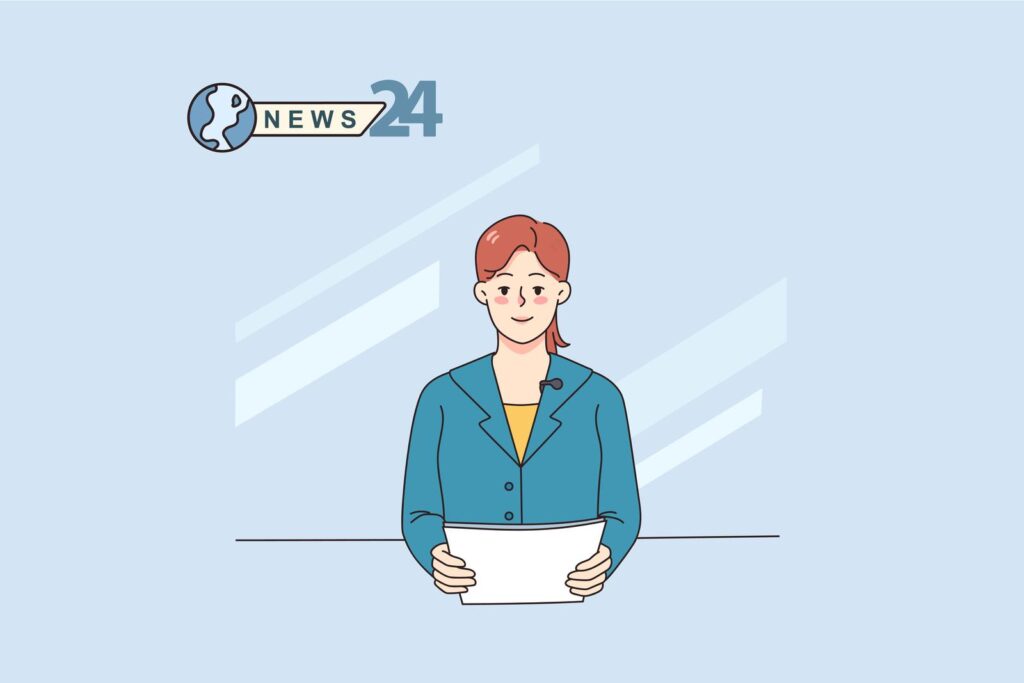
32.) Data Engineering
Data engineering is a staple in data science, focusing on efficiently managing data.
Data engineers are responsible for developing and maintaining the systems that collect, process, and store data.
In recent years, there has been an increasing demand for data engineers as the volume of data generated by businesses and organizations has grown exponentially.
Data engineers must be able to design and implement efficient data-processing pipelines and have the skills to optimize and troubleshoot existing systems.
If you are looking for a challenging research topic that would immediately impact you worldwide, then improving or innovating a new approach in data engineering would be a good start.
33.) Data Curation
Data curation has been a hot topic in the data science community for some time now.
Curating data involves organizing, managing, and preserving data so researchers can use it.
Data curation can help to ensure that data is accurate, reliable, and accessible.
It can also help to prevent research duplication and to facilitate the sharing of data between researchers.
Data curation is a vital part of data science. In recent years, there has been an increasing focus on data curation, as it has become clear that it is essential for ensuring data quality.
As a result, data curation is now a major research topic in data science.
There are numerous books and articles on the subject, and many universities offer courses on data curation.
Data curation is an integral part of data science and will only become more important in the future.
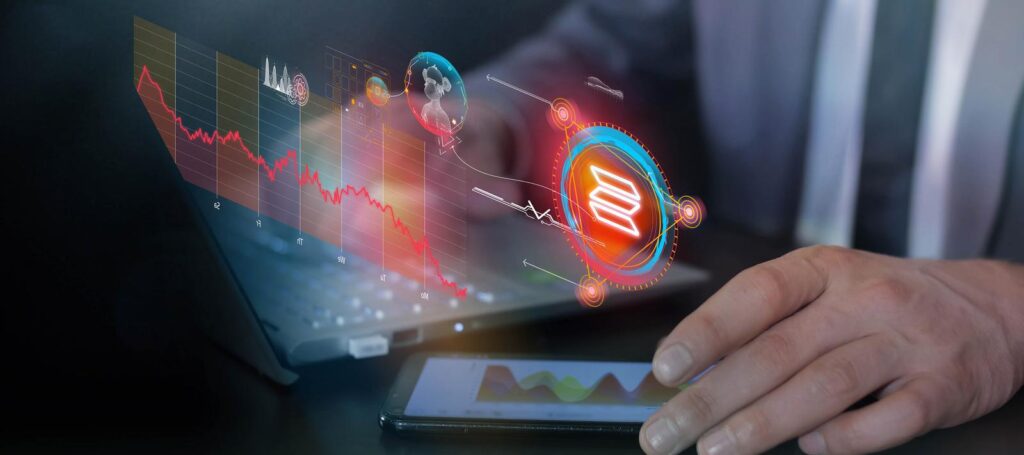
34.) Meta-Learning
Meta-learning is gaining a ton of steam in data science. It’s learning how to learn.
So, if you can learn how to learn, you can learn anything much faster.
Meta-learning is mainly used in deep learning, as applications outside of this are generally pretty hard.
In deep learning, many parameters need to be tuned for a good model, and there’s usually a lot of data.
You can save time and effort if you can automatically and quickly do this tuning.
In machine learning, meta-learning can improve models’ performance by sharing knowledge between different models.
For example, if you have a bunch of different models that all solve the same problem, then you can use meta-learning to share the knowledge between them to improve the cluster (groups) overall performance.
I don’t know how anyone looking for a research topic could stay away from this field; it’s what the Terminator warned us about!
35.) Data Warehousing
A data warehouse is a system used for data analysis and reporting.
It is a central data repository created by combining data from multiple sources.
Data warehouses are often used to store historical data, such as sales data, financial data, and customer data.
This data type can be used to create reports and perform statistical analysis.
Data warehouses also store data that the organization is not currently using.
This type of data can be used for future research projects.
Data warehousing is an incredible research topic in data science because it offers a variety of benefits.
Data warehouses help organizations to save time and money by reducing the need for manual data entry.
They also help to improve the accuracy of reports and provide a complete picture of the organization’s performance.
Data warehousing feels like one of the weakest parts of the Data Science Technology Stack; if you want a research topic that could have a monumental impact – data warehousing is an excellent place to look.
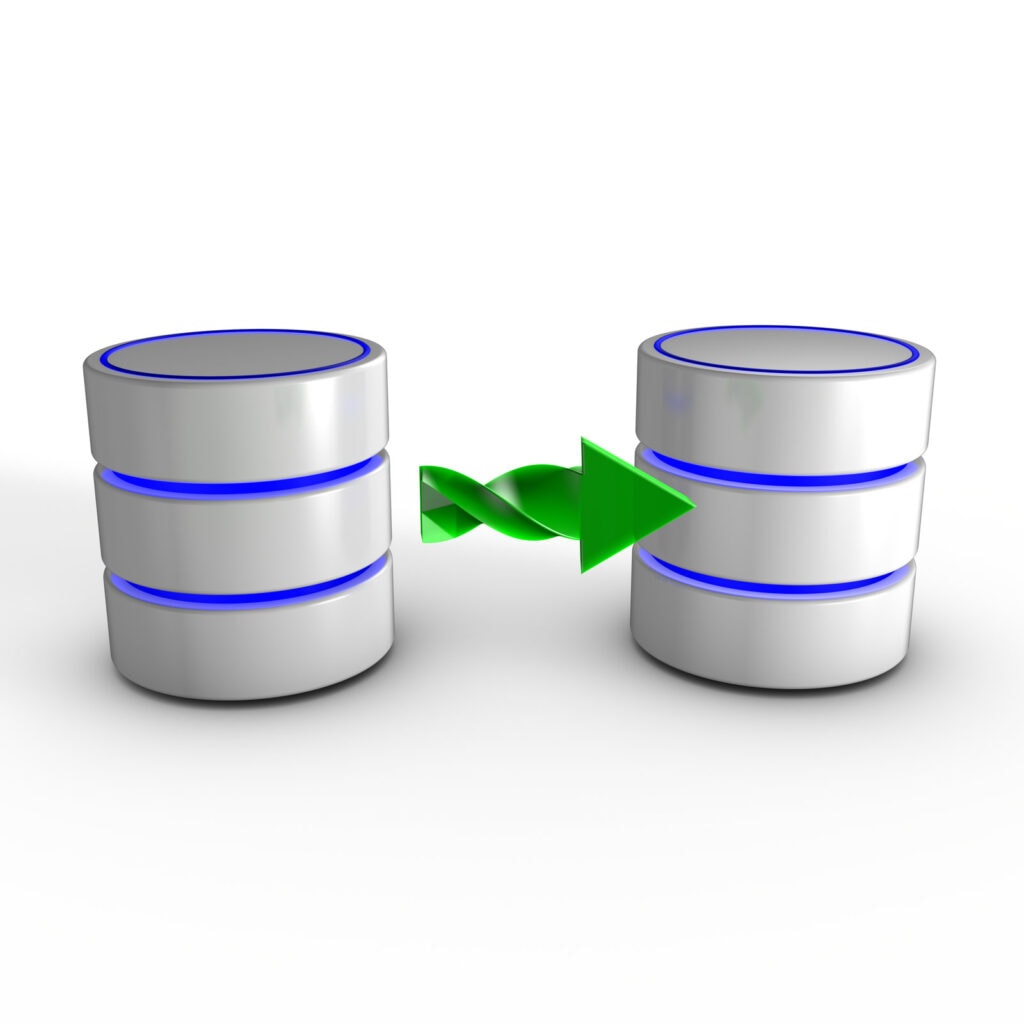
36.) Business Intelligence
Business intelligence aims to collect, process, and analyze data to help businesses make better decisions.
Business intelligence can improve marketing, sales, customer service, and operations.
It can also be used to identify new business opportunities and track competition.
BI is business and another tool in your company’s toolbox to continue dominating your area.
Data science is the perfect tool for business intelligence because it combines statistics, computer science, and machine learning.
Data scientists can use business intelligence to answer questions like, “What are our customers buying?” or “What are our competitors doing?” or “How can we increase sales?”
Business intelligence is a great way to improve your business’s bottom line and an excellent opportunity to dive deep into a well-respected research topic.
37.) Crowdsourcing
One of the newest areas of research in data science is crowdsourcing.
Crowdsourcing is a process of sourcing tasks or projects to a large group of people, typically via the internet.
This can be done for various purposes, such as gathering data, developing new algorithms, or even just for fun (think: online quizzes and surveys).
But what makes crowdsourcing so powerful is that it allows businesses and organizations to tap into a vast pool of talent and resources they wouldn’t otherwise have access to.
And with the rise of social media, it’s easier than ever to connect with potential crowdsource workers worldwide.
Imagine if you could effect that, finding innovative ways to improve how people work together.
That would have a huge effect.
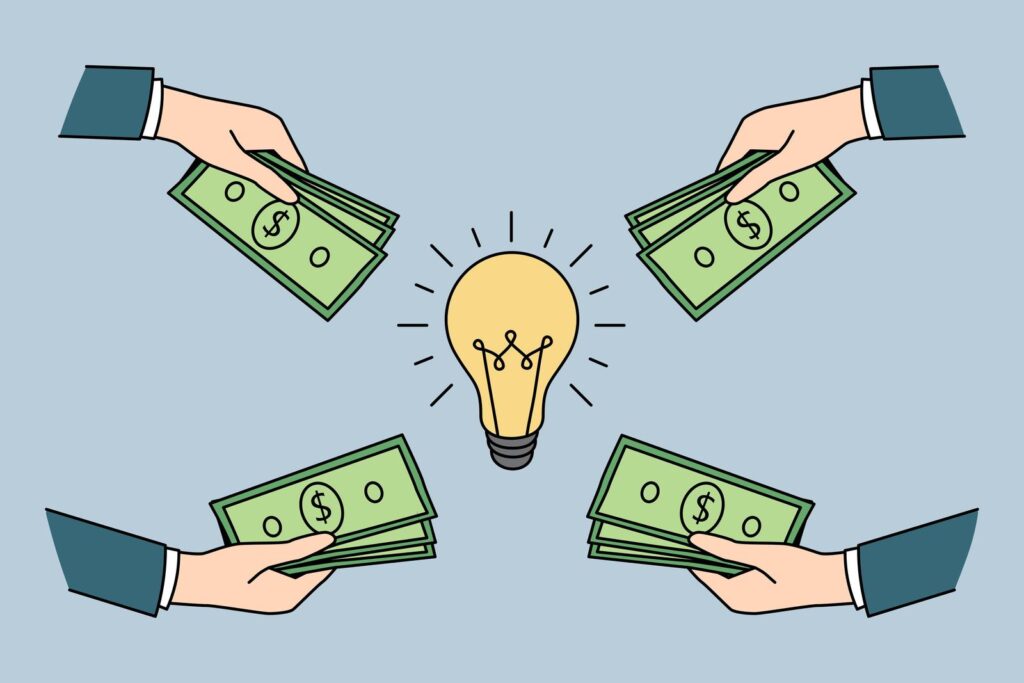
Final Thoughts, Are These Research Topics In Data Science For You?
Thirty-seven different research topics in data science are a lot to take in, but we hope you found a research topic that interests you.
If not, don’t worry – there are plenty of other great topics to explore.
The important thing is to get started with your research and find ways to apply what you learn to real-world problems.
We wish you the best of luck as you begin your data science journey!
Other Data Science Articles
We love talking about data science; here are a couple of our favorite articles:
- Why Are You Interested In Data Science?
- Recent Posts
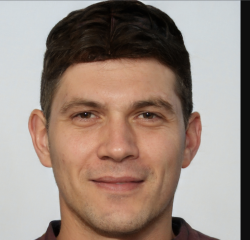
- Understanding Quality Gates in Software Testing [Unlock Secrets to Level Up Your Testing Game] - October 21, 2024
- Are Software Engineers Getting Hired? Expert Strategies Revealed [Boost Your Job Search!] - October 20, 2024
- How to Speed Up Tesla Software Update [Supercharge Your Update Process] - October 20, 2024
- Advertise with Us
.png)
- Cryptocurrencies
10 Best Research and Thesis Topic Ideas for Data Science in 2022
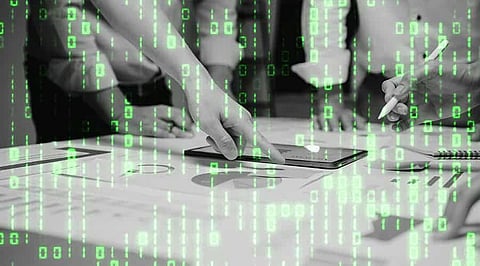
These research and thesis topics for data science will ensure more knowledge and skills for both students and scholars
As businesses seek to employ data to boost digital and industrial transformation, companies across the globe are looking for skilled and talented data professionals who can leverage the meaningful insights extracted from the data to enhance business productivity and help reach company objectives successfully. Recently, data science has turned into a lucrative career option. Nowadays, universities and institutes are offering various data science and big data courses to prepare students to achieve success in the tech industry. The best course of action to amplify the robustness of a resume is to participate or take up different data science projects. In this article, we have listed 10 such research and thesis topic ideas to take up as data science projects in 2022.
- Handling practical video analytics in a distributed cloud: With increased dependency on the internet, sharing videos has become a mode of data and information exchange. The role of the implementation of the Internet of Things (IoT), telecom infrastructure, and operators is huge in generating insights from video analytics. In this perspective, several questions need to be answered, like the efficiency of the existing analytics systems, the changes about to take place if real-time analytics are integrated, and others.
- Smart healthcare systems using big data analytics: Big data analytics plays a significant role in making healthcare more efficient, accessible, and cost-effective. Big data analytics enhances the operational efficiency of smart healthcare providers by providing real-time analytics. It enhances the capabilities of the intelligent systems by using short-span data-driven insights, but there are still distinct challenges that are yet to be addressed in this field.
- Identifying fake news using real-time analytics: The circulation of fake news has become a pressing issue in the modern era. The data gathered from social media networks might seem legit, but sometimes they are not. The sources that provide the data are unauthenticated most of the time, which makes it a crucial issue to be addressed.
- TOP 10 DATA SCIENCE JOB SKILLS THAT WILL BE ON HIGH DEMAND IN 2022
- TOP 10 DATA SCIENCE UNDERGRADUATE COURSES IN INDIA FOR 2022
- TOP DATA SCIENCE PROJECTS TO DO DURING YOUR OMICRON QUARANTINE
- Secure federated learning with real-world applications : Federated learning is a technique that trains an algorithm across multiple decentralized edge devices and servers. This technique can be adopted to build models locally, but if this technique can be deployed at scale or not, across multiple platforms with high-level security is still obscure.
- Big data analytics and its impact on marketing strategy : The advent of data science and big data analytics has entirely redefined the marketing industry. It has helped enterprises by offering valuable insights into their existing and future customers. But several issues like the existence of surplus data, integrating complex data into customers' journeys, and complete data privacy are some of the branches that are still untrodden and need immediate attention.
- Impact of big data on business decision-making: Present studies signify that big data has transformed the way managers and business leaders make critical decisions concerning the growth and development of the business. It allows them to access objective data and analyse the market environments, enabling companies to adapt rapidly and make decisions faster. Working on this topic will help students understand the present market and business conditions and help them analyse new solutions.
- Implementing big data to understand consumer behaviour : In understanding consumer behaviour, big data is used to analyse the data points depicting a consumer's journey after buying a product. Data gives a clearer picture in understanding specific scenarios. This topic will help understand the problems that businesses face in utilizing the insights and develop new strategies in the future to generate more ROI.
- Applications of big data to predict future demand and forecasting : Predictive analytics in data science has emerged as an integral part of decision-making and demand forecasting. Working on this topic will enable the students to determine the significance of the high-quality historical data analysis and the factors that drive higher demand in consumers.
- The importance of data exploration over data analysis : Exploration enables a deeper understanding of the dataset, making it easier to navigate and use the data later. Intelligent analysts must understand and explore the differences between data exploration and analysis and use them according to specific needs to fulfill organizational requirements.
- Data science and software engineering : Software engineering and development are a major part of data science. Skilled data professionals should learn and explore the possibilities of the various technical and software skills for performing critical AI and big data tasks.
Join our WhatsApp Channel to get the latest news, exclusives and videos on WhatsApp
_____________
Disclaimer: Analytics Insight does not provide financial advice or guidance. Also note that the cryptocurrencies mentioned/listed on the website could potentially be scams, i.e. designed to induce you to invest financial resources that may be lost forever and not be recoverable once investments are made. You are responsible for conducting your own research (DYOR) before making any investments. Read more here.
Related Stories

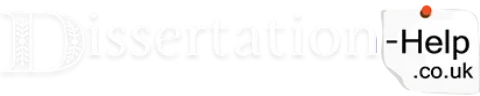
Click here to place an order for topic brief service to get instant approval from your professor.
99 Best Data Science Dissertation Topics
Table of Contents
What is a Data Science Dissertation?
A Data Science Dissertation is a research project where students explore the vast field of data science. This involves analyzing large sets of data, creating models, and finding patterns to solve problems or make decisions. In a data science dissertation, you might work on topics like machine learning, big data analytics, or predictive modeling. The goal is to contribute new insights or methods to the field of data science.
Why are Data Science Dissertation Topics Important?
Data science is one of the most in-demand fields today. Companies rely on data to make informed decisions, predict trends, and understand their customers better. By choosing a data science topic, you can explore real-world problems and provide solutions that can be applied in various industries like healthcare, finance, or technology. Your dissertation could help advance the field, making your research valuable and relevant.
Writing Tips for Data Science Dissertation
- Select a Relevant Topic: Pick a topic that is current and has a practical application. This will make your research more meaningful and impactful.
- Use Quality Data: Ensure you have access to high-quality and reliable data. Good data is crucial for accurate analysis and valid conclusions.
- Explain Your Methods Clearly: Data science can be complex, so clearly explain your methods and why you chose them. This helps others understand and replicate your work.
- Visualize Your Results: Use charts, graphs, and other visual tools to present your findings. This makes your dissertation easier to understand and more engaging.
List of Data Science Dissertation Topics
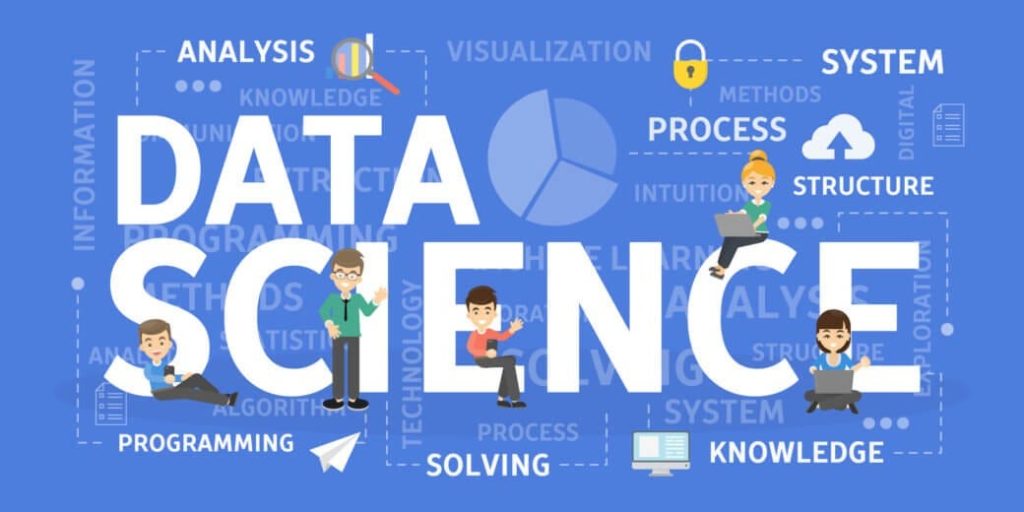
Machine Learning and Artificial Intelligence
- Enhancing Fraud Detection Systems using Deep Learning Algorithms
- Personalized Recommendation Systems: A Comparative Analysis of Machine Learning Approaches
- Predictive Modeling for Disease Diagnosis and Treatment
Big Data Analytics
- Optimizing Supply Chain Management through Big Data Analytics
- Sentiment Analysis on Social Media Data: Understanding Customer Perception
- Big Data-driven Strategies for Urban Planning and Development
Natural Language Processing (NLP)
- Automated Text Summarization Techniques: A Comparative Study
- Language Translation Models: Challenges and Opportunities
- Sentiment Analysis in Political Discourse: Uncovering Public Opinion
Data Mining and Knowledge Discovery
- Association Rule Mining for Market Basket Analysis
- Clustering Techniques for Customer Segmentation in E-commerce
- Predictive Analytics in Stock Market Forecasting
Health Informatics
- Predictive Modeling for Early Disease Detection
- Wearable Devices and Remote Patient Monitoring: A Data-driven Approach
- Data Privacy and Security in Healthcare Data Sharing Platforms
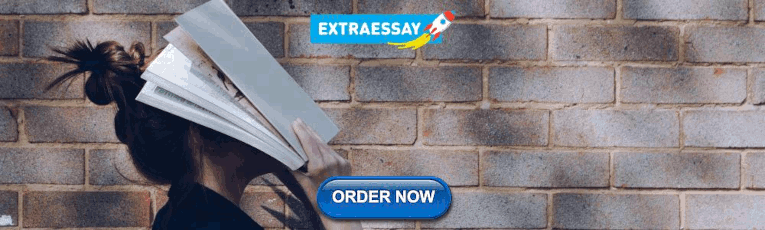
Business Intelligence and Analytics
- Data-driven Decision Making in Marketing Campaigns
- Customer Lifetime Value Prediction: A Machine Learning Approach
- Performance Analytics for Business Process Optimization
IoT and Sensor Data Analytics
- Smart Cities: Leveraging IoT Data for Urban Sustainability
- Predictive Maintenance in Industrial IoT: Anomaly Detection Techniques
- Environmental Monitoring using Sensor Networks: Challenges and Opportunities
Image and Video Analysis
- Object Detection and Recognition in Surveillance Videos
- Medical Image Analysis: Applications in Diagnosis and Treatment
- Deep Learning Approaches for Facial Recognition Systems
Social Network Analysis
- Influence Detection in Social Networks: A Graph-based Approach.
- Community Detection and Analysis in Online Social Platforms
- Fake News Detection using Social Network Analysis Techniques
Time Series Analysis
- Forecasting Demand in Retail: Time Series Models for Sales Prediction
- Financial Market Volatility Prediction using Time Series Analysis
- Energy Consumption Forecasting: A Comparative Study of Forecasting Models
Spatial Data Analysis
- Geographic Information Systems (GIS) for Urban Planning
- Spatial-Temporal Analysis of Crime Patterns: A Case Study
- Environmental Impact Assessment using Spatial Data Analysis Techniques
Bioinformatics
- Genomic Data Analysis: Towards Precision Medicine
- Protein Structure Prediction using Machine Learning Algorithms
- Computational Drug Discovery: Opportunities and Challenges
Data Privacy and Ethics
- Privacy-preserving Data Mining Techniques: Balancing Utility and Privacy
- Ethical Considerations in AI-driven Decision-Making Systems
- GDPR Compliance in Data-driven Businesses: Challenges and Solutions
Deep Learning Applications
- Deep Reinforcement Learning for Autonomous Vehicles
- Generative Adversarial Networks (GANs) for Synthetic Data Generation
- Deep Learning Models for Natural Language Understanding
Blockchain and Data Science
- Blockchain-enabled Data Sharing Platforms: Opportunities and Challenges
- Decentralized Data Marketplaces: A Paradigm Shift in Data Economy
- Security and Privacy in Blockchain-based Data Analytics
- Computer Science Research Topics (Approved Titles)
- Which topics are best for thesis in Computer Science?
- Information Systems Dissertation Topics Ideas
Writing a data science dissertation is an exciting opportunity to dive deep into a topic that interests you. Whether you’re exploring machine learning algorithms , data mining techniques, or the ethical implications of data usage, your research can make a significant impact. Choose a topic that aligns with your interests and has real-world relevance and remember to explain your methods and results clearly.
1. What are some common data science dissertation topics?
Common topics include machine learning applications, big data analytics, data visualization techniques, and the impact of AI on data processing.
2. How do I choose a data science dissertation topic?
Choose a topic that you find interesting, has enough data available, and is relevant to current trends in the field of data science.
3. What tools do I need for a data science dissertation?
You may need tools like Python, R, SQL, and data visualization software like Tableau or Power BI.
4. How long should my data science dissertation be?
The length varies, but most data science dissertations are around 80 to 120 pages. Check your institution’s guidelines for specific requirements.
Data Science Dissertation Topics Brief Service
Are you struggling to find the perfect Data Science Dissertation Topic tailored to your interests and expertise? Our customized topics brief service is designed to provide personalized guidance and support in selecting a dissertation topic that aligns with your academic goals. Fill the form below to get started on your journey towards academic excellence in data science.
WhatsApp and Get 35% off promo code now!
Luigi's guide to writing Master's theses (in Data Science)
Luigi Acerbi , University of Helsinki, Finland Last edited: 30 Aug 2024 ( added section on Thesis Review )
These recommendations are aimed primarily to my students from the Master's Programme in Data Science at the University of Helsinki, but many points are likely to apply to related programmes and other institutions. In fact, most of this guide generalizes to scientific academic writing in general (e.g., articles, PhD theses).
Disclaimer: There are loads of better materials elsewhere online; this page is mostly a collection of advice I realized I was repeating to multiple students, so I thought to put it in writing in a single place. Many of these points are not absolute rules, but my own sometimes-idiosyncratic opinions and personal recommendations: always double-check with your thesis advisor.
Before you start:
- Programme instructions: Read carefully all the instructions about Master's theses provided by the Programme, which you can find at this link (select your programme from the menu).
- Planning and deadlines: Writing the Master's thesis will take at the very minimum a full month, probably more, so plan accordingly. Be aware of the deadlines for submitting your thesis for review , and bring this up well in advance with your supervisor if you want to graduate at a given time. Generally, your examiners (two people who review and grade the thesis, see Thesis Review below) will need at least a month to read and review the thesis, and the second examiner (who is typically not directly involved in the thesis work) will need to be warned in advance. On top of that, there is the extra time needed to submit the thesis to the steering committee for approval. See the programme instructions above.
- Start writing before you start writing: Even before officially starting the writing period, write down what you do (e.g., in a scrap LaTeX document). Write partial results, derivations, notes, etc. - no need to be particularly organized at this stage. However, if you already have written down bits and pieces of your work, it will make your life much easier later.
- Look up other theses: Nothing better than learning by example. Since almost all University of Helsinki theses are published in the Helka database, it is easy to look at other theses. For example, this query will display all theses from the Master's programme in Data Science.
- Supervisor feedback: When writing the thesis it is wise to finish at least one or two chapters early on (e.g., Introduction and Background), and share them with your supervisor so that they can point out major issues before they are all over the thesis (citations, grammar, structure...). To make the most out of this step, the shared chapters should be in a decent shape — that is, as close as possible to how you would consider them "finished". This way, the supervisor can give real feedback on your writing — as opposed to commenting on a clearly unfinished and unpolished draft, which is not so useful. More generally, agree early on with your thesis supervisor how the feedback on the thesis will work.
- Meetings: The meetings are not for you to prove your supervisor that you have done something. Your supervisor is there to help you. The meetings are for your benefit. To make most of the meetings, always try to bring something to the meeting that you can discuss or get feedback from.
- Meeting notes: After each meeting with you supervisor, especially if you do not meet that often, it is a very good habit to write a brief recap as a bullet point list. Briefly summarize comments/clarifications about the work discussed during the metting, and list the action points about the things you need to work on next. Send the recap to your supervisor (via email or on Slack). This is a very good way to keep track of progress and potentially clarify misunderstandings.
General thesis:
- Layout: If you are in the hard or exact sciences, do not even think of writing your thesis in anything but LaTeX. Use the LaTeX template provided by the University of Helsinki (or your institution). If you are MSc Data Science student, you can find the template in the Moodle course Data Science MSc Thesis . For example, you can use Overleaf as an easy entry to LaTeX. The University of Helsinki has a premium license for Overleaf, accessible by signing in with your institution credentials.
- Length: While there is no set requirement for length, a typical Master's thesis will be between 40-60 pages. This is just a broad guideline; of course, nobody will complain if you solve the Riemann hypothesis in 10 pages. Longer theses are also possible, but think whether all the content is needed; if you really think that everything is essential, at least consider putting something in one or multiple Appendices.
- Structure: While titles can change, a typical thesis will have: a Summary/Abstract ; an Introduction chapter; a Preliminaries/Background chapter covering the literature review with background theory and tools used in your thesis (cover only what you need for your work, no need to write a full textbook or to show off knowledge of unrelated topics); likely a Methods chapter explaining more in detail what you actually did in the thesis (e.g., describe your model(s), your data, your theory); a Results chapter showing your method applied to the data; and a final Discussion chapter summarizing the thesis and conclusions. If needed, you could also have an Appendix for extra material. Of course, these are just generic guidelines - depending on your thesis work, you might have two chapters with results, or no methods chapter, etc.
- Consistency: The Master's thesis is a unified scholarly work so pay particular attention to consistency of notation, figures, tables, naming conventions, etc. across sections and chapters etc. (see below for more examples).
- Level of detail: Generally speaking, the thesis is about reporting what you did in a scientific way. Finding the right level of detail can be tricky, but try to be both informative and brief. You do not need to write every single detail - the thesis is not a diary of what you did. On the other hand you need to provide enough information so that the reader can figure out what you actually worked on and obtained.
- Target readership: The ideal target reader for the thesis is a peer Master's student, i.e. someone from your programme who may have taken a few different courses from you and ended up working on a completely different project for the thesis. So, when writing the thesis, think carefully what you can take for granted (e.g., you can safely assume that the reader knows what a real number is, what Numpy is, but also what K-means is), and what you may have to explain (e.g., you might have to at least write a paragraph or short section on what a Gaussian process is). As a rule of thumb, anything that you did not know before starting the thesis should be explained .
- Negative results: Most explorations and attempts in science do not work, and the same is true for data science and machine learning. Luckily, the Master's thesis is not a NeurIPS submission, so there can be plenty of merit in exploring and reporting "negative" results. It is totally fine and in fact quite normal to report negative results in a Master's thesis (i.e., things which did not work as planned), but try to keep a scientific approach. If the proposed method did not work, can you explain (with evidence) or at least hypothesize why it didn't work? Would you have a proposal on what could be done to fix it, had it there been more time?
- Text format: Ensure that function names are not written in italic, e.g. in LaTeX use "\exp" and "\log" and not "exp" and "log". Similarly, use "\text{}" as needed for textual elements that are not variables. For example, use $\hat{\theta}_\text{MAP}$ to denote the maximum-a-posteriori estimate, as opposed to $\hat{\theta}_{MAP}$. We do not want "MAP" to be in italic, since it is not a variable name.
- Notation: Even for relatively common notation, explain the notation you are using (unless it's truly basic). This is a must when there might be similar notations out there. For example, "$\mathcal{N}\left(x; \mu, \sigma^2\right)$ denotes the probability density function of a normal distribution with mean $\mu$ and variance $\sigma^2$."
- Basic presentation: Check that you have labelled all the axes, you have a legend if needed, the figure is readable (e.g., font size is large enough). Figures should look pretty.
- Captions: Figure captions should be brief but informative. Describe briefly what the axes are, what is being represented in the figure (or in each panel). For example, if there is a color map, what's the color map representing? Describe what the reader should look at and give a brief takeaway message if possible, i.e. why this figure is here? What is it showing of interest?
- Link from the text: Figures are somewhat independent from the text in that they should mostly read stand-alone. However, Figures should be always referred to from the text, ideally just before or just after the figure (e.g., "As shown in Figure 1, [...]").
- Consistency: Check for consistency across figures. For example, font size, color map, naming of axes, ordering of variables, etc. should be as consistent as possible for figures in the same work (here, in the same thesis). Additional consistency, if possible without harming presentation, is a bonus (e.g., consistent axis limits across figures).
- License: You are allowed to include figures which are in the public domain or for example with a CC-BY 4.0 license . Just be sure you are specifying somewhere (e.g, in the caption) the source and its license. In most cases, be mindful to modify the figure for your purposes, do not just copy-paste it (e.g., you might not need all the details from the original figure, or you might have to modify something to keep consistency with the rest of your thesis).
- Format: If you can, try to render figures as vector-based graphics such as pdf or svg, rather than as bitmap (png or jpg), to make them sharper and smaller in filesize.
- General comments: What written above for Figures generally applies to Tables too. Make sure that the tables are well-presented, readable, the caption is explanatory, the layout is consistent, etc.
- Figure or Table?: Think if the content of a table could be better conveyed using a figure (and vice versa: a very cluttered figure could become a neat table).
References:
- How many: The Master's thesis is a piece of scholarly work, so we expect to see appropriate citations to the literature (especially in the introductory and preliminary parts, but also later). Again, there is no set requirement for number of citations, but if your thesis cites less than ten articles / conference papers / books you could probably do a bit more of literature review, or be more mindful in citing the papers related to the methods you are using.
- Format: There are many different bibliography options to choose from in LaTeX. For a thesis , I recommend against the default number-only citation format, which is non-informative and hard to parse for humans (e.g., what's citation [31]?). Instead, use a "(Author(s), Year)" citation format, or alternatively the alphanumeric [authors' initials + year] format, e.g. "[ABC22]". One or the other might be more popular, depending on the community. If you use the author + year format, be sure to appropriately use \citep{} or \citet{} depending on the context.
- Bibliography check: Double and triple-check your formatted bibliography as generated by LaTeX. You will likely be using BibTex in LaTeX for your bibliography. Check that your .bib files are correct and that the references appear correctly in the bibliography. It is very easy that .bib entries taken from e.g. Google Scholar have missing parts (name of the journal, page number, even authors), or perhaps refer to an earlier arXiv preprint while the paper has been in the meantime published in a journal or conference. It is your job as a scholar to ensure the up-to-date validity and correctness of your bibliography. So be sure to read through the generated PDF to at least spot glaring omissions, and put effort in polishing the bibliography.
- Capitalization: As a subset of the above check, you want to capitalize words in article titles correctly (e.g., "Bayesian" should be capitalized, not "bayesian"). In a .bib entry, you can force BibTex to keep the capitalization by using curly brackets around letters. For example, you can write "title={{V}ariational {B}ayesian {M}onte {C}arlo}" to ensure proper capitalization.
- Reference management software: To keep track of references used during the thesis work and writing, it might be useful to use some reference management software (beyond a .bib file), such as Zotero or Paperpile .
Miscellanea:
- Footnotes: Unless stated otherwise, you can have footnotes in your thesis; which can be a good way to add side information without cluttering the main text. Use them sparsely and wisely.
- Spell-checking: Wherever you write your .tex files, you should find a way to run a spell-checker at least at the end, when you are polishing the text. For example, there should be one in Overleaf. A spell-checker should be able to catch the most obvious mistakes. Google's spell-checking (in doc and gmail, for example) is also very good. One notorious point to be aware of for some non-native English speakers, including myself, is the usage of articles (i.e., "the" or "a") which can be quite random, but modern spell checkers (at least the Google ones) are able to spot this and recommend when an article should be added or removed.
- Perspective: While important as the final step of your Master's studies, keep in mind this is a Master's thesis and not a doctoral dissertation, so make sure that the scope is appropriate, do not spend too many months on it. If you plan to continue with research, consider applying for a PhD position, which sometimes could build on top of your Master's work (if not directly, at least in terms of gained experience).
- Writing guides: Consider reading a book about scientific/academic writing (or at least some blogs/articles). There are also online courses available on the topic, such as on Edx and Coursera, which are often free if you only audit the course. As an example, read about "topic sentences" and see if they could help your writing process.
Thesis Review:
- These points are specific to the University of Helsinki.
- The thesis submission and review is performed in E-thesis . This is where you will submit your thesis when ready.
- Supervisor: The supervisor is the main instructor of the thesis, someone with a doctoral degree and employed by the Faculty of your Master's program (e.g., the Faculty of Science if you are in the Master's Programme in Data Science or Computer Science).
- Examiners: The examiners are two people who review and grade the thesis once it is submitted. The examiners need to be a University of Helsinki professor, associate or assistant professor, senior university lecturer, university lecturer or research director, together with a person who has at least a doctoral degree. The supervisor will almost always also be an examiner. Your supervisor (i.e., likely me if you are reading this) will find suitable examiners for your thesis, so this should not worry you.
Use of Large Language Models (LLMs):
- Master's in Data Science Guidelines: First of all, check the guidelines about AI usage provided for the Master's Programme in Data Science. In particular, " If you use a language model to produce the work you are returning, you must report in writing which model (e.g. ChatGPT, DeepL) you have used and in what way. This also applies to theses ." Disclose the usage in the Acknowledgments or some other appropriate section of the thesis.
- Beware of nonsense: It is your job to check that the output produced by the LLM is correct and meaningful. In particular, be aware of the tendency of many LLMs, when dealing with complex technical contexts, of spouting well-written word salads which really mean absolutely nothing. Another common problem is that LLMs can deal badly with technical words, for example substituting technical words with non-technical ones, with hilarious effects.
- Writing style: The writing style of some LLMs (ChatGPT in particular) is recognizable from miles away, objectively terrible, stereotyped, and banal. The sentence structure used by ChatGPT (at least at the time of writing) is over-repetitive, as well as its choice of wording ("leverage", "innovative", "tapestry", etc.). Moreover, ChatGPT has a tendency of overselling its content ("innovative", "groundbreaking", etc.). This is not generally true of all LLMs (and definitely is not a statement about their general writing capabilities; even GPT-3 could write better than this), but just a fact to be aware of and which might not be obvious if you have not spent many hours reading ChatGPT-generated content.
- Knowledge gathering: Writing aside, there are other areas where modern LLMs and AI tools can help during a Master's thesis. For example, in addition to Google Scholar and other academic repositories and good old-fashioned search, LLM-based services such as Perplexity and Elicit (and others) can be successfully used as additional tools to expand the way a researcher can explore the literature, find related work, and improve their understanding.
- Conclusions: In sum, I am in favor of using LLMs in writing especially to break the "blank page" and get things started, or occasionally to suggest how to write or rewrite a paragraph or sentence, but you need to remain in full control of the produced text. In particular, do not switch off your critical thinking and do not blindly accept what the LLM is proposing. We know what the extreme of that might look like .
Acknowledgments:
Thanks to Antti Honkela , Marlon Tobaben and Aki Rehn for useful comments and suggestions.

99+ Data Science Research Topics: A Path to Innovation
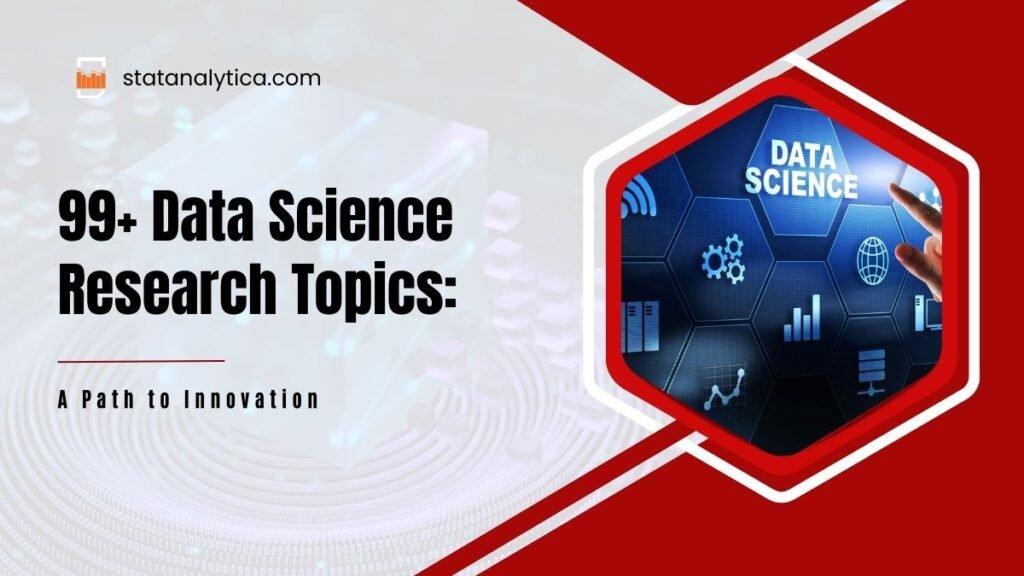
In today’s rapidly advancing digital age, data science research plays a pivotal role in driving innovation, solving complex problems, and shaping the future of technology. Choosing the right data science research topics is paramount to making a meaningful impact in this field.
In this blog, we will delve into the intricacies of selecting compelling data science research topics, explore a range of intriguing ideas, and discuss the methodologies to conduct meaningful research.
How to Choose Data Science Research Topics?
Table of Contents
Selecting the right research topic is the cornerstone of a successful data science endeavor. Several factors come into play when making this decision.
- First and foremost, personal interests and passion are essential. A genuine curiosity about a particular subject can fuel the dedication and enthusiasm needed for in-depth research.
- Current trends and challenges in data science provide valuable insights into areas that demand attention.
- Additionally, the availability of data and resources, as well as the potential impact and applications of the research, should be carefully considered.
: Tips & Tricks |
99+ Data Science Research Topics Ideas: Category Wise
Supervised machine learning.
- Predictive modeling for disease outbreak prediction.
- Credit scoring using machine learning for financial institutions.
- Sentiment analysis for stock market predictions.
- Recommender systems for personalized content recommendations.
- Customer churn prediction in e-commerce.
- Speech recognition for voice assistants.
- Handwriting recognition for digitization of historical documents.
- Facial recognition for security and surveillance.
- Time series forecasting for energy consumption.
- Object detection in autonomous vehicles.
Unsupervised Machine Learning
- Market basket analysis for retail optimization.
- Topic modeling for content recommendation.
- Clustering techniques for social network analysis.
- Anomaly detection in manufacturing processes.
- Customer segmentation for marketing strategies.
- Event detection in social media data.
- Network traffic anomaly detection for cybersecurity.
- Anomaly detection in healthcare data.
- Fraud detection in insurance claims.
- Outlier detection in environmental monitoring.
Natural Language Processing (NLP)
- Abstractive text summarization for news articles.
- Multilingual sentiment analysis for global brands.
- Named entity recognition for information extraction.
- Speech-to-text transcription for accessibility.
- Hate speech detection in social media.
- Aspect-based sentiment analysis for product reviews.
- Text classification for content moderation.
- Language translation for low-resource languages.
- Chatbot development for customer support.
- Emotion detection in text and speech.
Deep Learning
- Image super-resolution using convolutional neural networks.
- Reinforcement learning for game playing and robotics.
- Generative adversarial networks (GANs) for image generation.
- Transfer learning for domain adaptation in deep models.
- Deep learning for medical image analysis.
- Video analysis for action recognition.
- Natural language understanding with transformer models.
- Speech synthesis using deep neural networks.
- AI-powered creative art generation.
- Deep reinforcement learning for autonomous vehicles.
Big Data Analytics
- Real-time data processing for IoT sensor networks.
- Social media data analysis for marketing insights.
- Data-driven decision-making in supply chain management.
- Customer journey analysis for e-commerce.
- Predictive maintenance using sensor data.
- Stream processing for financial market data.
- Energy consumption optimization in smart grids.
- Data analytics for climate change mitigation.
- Smart city infrastructure optimization.
- Data analytics for personalized healthcare recommendations.
Data Ethics and Privacy
- Fairness and bias mitigation in AI algorithms.
- Ethical considerations in AI for criminal justice.
- Privacy-preserving data sharing techniques.
- Algorithmic transparency and interpretability.
- Data anonymization for privacy protection.
- AI ethics in healthcare decision support.
- Ethical considerations in facial recognition technology.
- Governance frameworks for AI and data use.
- Data protection in the age of IoT.
- Ensuring AI accountability and responsibility.
Reinforcement Learning
- Autonomous drone navigation for package delivery.
- Deep reinforcement learning for game AI.
- Optimal resource allocation in cloud computing.
- Reinforcement learning for personalized education.
- Dynamic pricing strategies using reinforcement learning.
- Robot control and manipulation with RL.
- Multi-agent reinforcement learning for traffic management.
- Reinforcement learning in healthcare for treatment plans.
- Learning to optimize supply chain logistics.
- Reinforcement learning for inventory management.
Computer Vision
- Video-based human activity recognition.
- 3D object detection and tracking.
- Visual question answering for image understanding.
- Scene understanding for autonomous robots.
- Facial emotion recognition in real-time.
- Image deblurring and restoration.
- Visual SLAM for augmented reality applications.
- Image forensics and deepfake detection.
- Object counting and density estimation.
- Medical image segmentation and diagnosis.
Time Series Analysis
- Time series forecasting for renewable energy generation.
- Stock price prediction using LSTM models.
- Climate data analysis for weather forecasting.
- Anomaly detection in industrial sensor data.
- Predictive maintenance for machinery.
- Time series analysis of social media trends.
- Human behavior modeling with time series data.
- Forecasting economic indicators.
- Time series analysis of health data for disease prediction.
- Traffic flow prediction and optimization.
Graph Analytics
- Social network analysis for influence prediction.
- Recommender systems with graph-based models.
- Community detection in complex networks.
- Fraud detection in financial networks.
- Disease spread modeling in epidemiology.
- Knowledge graph construction and querying.
- Link prediction in citation networks.
- Graph-based sentiment analysis in social media.
- Urban planning with transportation network analysis.
- Ontology alignment and data integration in semantic web.
What Is The Right Research Methodology?
- Alignment with Objectives: Ensure that the chosen research approach aligns with the specific objectives of your study. This will help you answer the research questions effectively.
- Data Collection Methods: Carefully plan and execute data collection methods. Consider using surveys, interviews, data mining, or a combination of these based on the nature of your research and the data availability.
- Data Analysis Techniques: Select appropriate data analysis techniques that suit the research questions. This may involve using statistical analysis for quantitative data, machine learning algorithms for predictive modeling, or deep learning models for complex pattern recognition, depending on the research context.
- Ethical Considerations: Prioritize ethical considerations in data science research. This includes obtaining informed consent from study participants and ensuring data anonymization to protect privacy. Ethical guidelines should be followed throughout the research process.
Choosing the right research methodology involves a thoughtful and purposeful selection of methods and techniques that best serve the objectives of your data science research.
How to Conduct Data Science Research?
Conducting data science research involves a systematic and structured approach to generate insights or develop solutions using data. Here are the key steps to conduct data science research:
- Define Research Objectives
Clearly define the goals and objectives of your research. What specific questions do you want to answer or problems do you want to solve?
- Literature Review
Conduct a thorough literature review to understand the current state of research in your chosen area. Identify gaps, challenges, and potential research opportunities.
- Data Collection
Gather the relevant data for your research. This may involve data from sources like databases, surveys, APIs, or even creating your datasets.
- Data Preprocessing
Clean and preprocess the data to ensure it is in a usable format. This includes handling missing values, outliers, and data transformations.
- Exploratory Data Analysis (EDA)
Perform EDA to gain a deeper understanding of the data. Visualizations, summary statistics, and data profiling can help identify patterns and insights.
- Hypothesis Formulation (if applicable)
If your research involves hypothesis testing, formulate clear hypotheses based on your data and objectives.
- Model Development
Choose the appropriate modeling techniques (e.g., machine learning, statistical models) based on your research objectives. Develop and train models as needed.
- Evaluation and Validation
Assess the performance and validity of your models or analytical methods. Use appropriate metrics to measure how well they achieve the research goals.
- Interpret Results
Analyze the results and interpret what they mean in the context of your research objectives. Visualizations and clear explanations are important.
- Iterate and Refine
If necessary, iterate on your data collection, preprocessing, and modeling steps to improve results. This process may involve adjusting parameters or trying different algorithms.
- Ethical Considerations
Ensure that your research complies with ethical guidelines, particularly concerning data privacy and informed consent.
- Documentation
Maintain comprehensive documentation of your research process, including data sources, methodologies, and results. This helps in reproducibility and transparency.
- Communication
Communicate your findings through reports, presentations, or academic papers. Clearly convey the significance of your research and its implications.
- Peer Review and Feedback
If applicable, seek peer review and feedback from experts in the field to validate your research and gain valuable insights.
- Publication and Sharing
Consider publishing your research in reputable journals or sharing it with the broader community through conferences, online platforms, or industry events.
- Continuous Learning
Stay updated with the latest developments in data science and related fields to refine your research skills and methodologies.
Conducting data science research is a dynamic and iterative process, and each step is essential for generating meaningful insights and contributing to the field. It’s important to approach your research with a critical and systematic mindset, ensuring that your work is rigorous and well-documented.
Challenges and Pitfalls of Data Science Research
Data science research, while promising and impactful, comes with its set of challenges. Common obstacles include data quality issues, lack of domain expertise, algorithmic biases, and ethical dilemmas.
Researchers must be aware of these challenges and devise strategies to overcome them. Collaboration with domain experts, thorough validation of algorithms, and adherence to ethical guidelines are some of the approaches to mitigate potential pitfalls.
Impact and Application
The impact of data science research topics extends far beyond the confines of laboratories and academic institutions. Research outcomes often find applications in real-world scenarios, revolutionizing industries and enhancing the quality of life.
Predictive models in healthcare improve patient care and treatment outcomes. Advanced fraud detection systems safeguard financial transactions. Natural language processing technologies power virtual assistants and language translation services, fostering global communication.
Real-time data processing in IoT applications drives smart cities and connected ecosystems. Ethical considerations and privacy-preserving techniques ensure responsible and respectful use of personal data, building trust between technology and society.
Embarking on a journey in data science research topics is an exciting and rewarding endeavor. By choosing the right research topics, conducting rigorous studies, and addressing challenges ethically and responsibly, researchers can contribute significantly to the ever-evolving field of data science.
As we explore the depths of machine learning, natural language processing, big data analytics, and ethical considerations, we pave the way for innovation, shape the future of technology, and make a positive impact on the world.
Related Posts
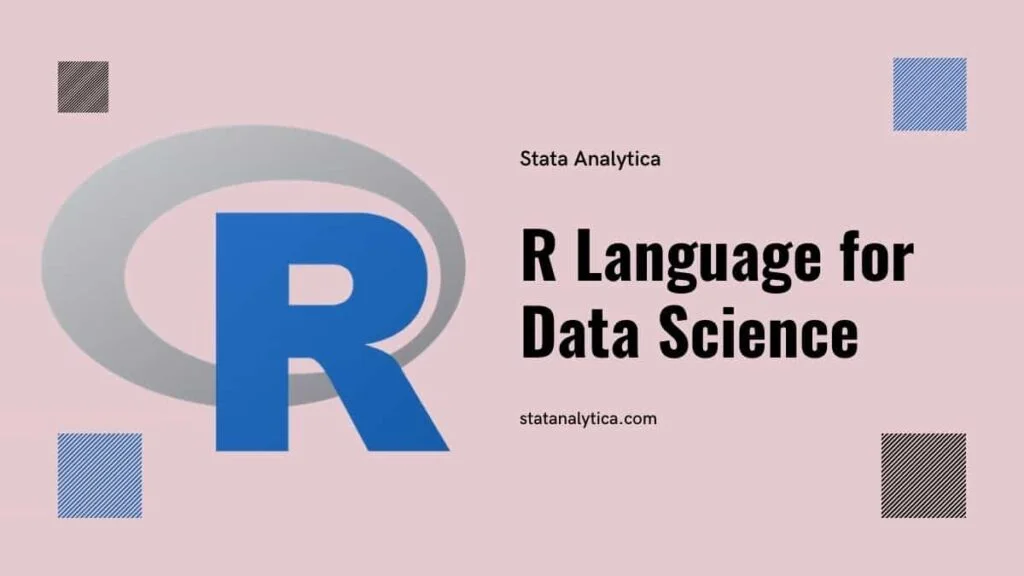
Top Reasons For Why Should You Use R for Data Science
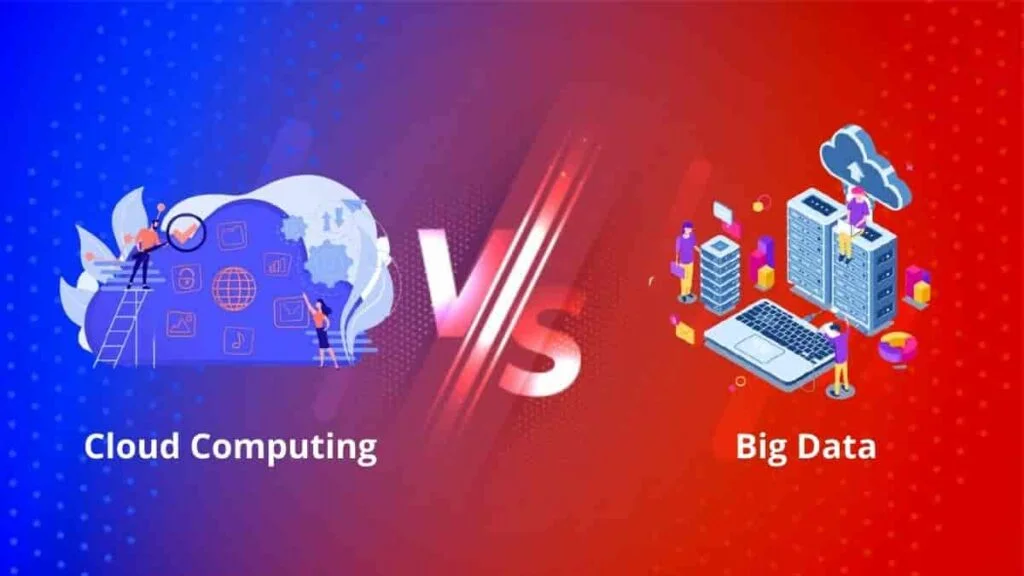
In Depth Difference b/w Big Data And Cloud Computing
Mon - Sat 9:00am - 12:00am
- Get a quote
List of Best Research and Thesis Topic Ideas for Data Science in 2022
In an era driven by digital and technological transformation, businesses actively seek skilled and talented data science potentials capable of leveraging data insights to enhance business productivity and achieve organizational objectives. In keeping with an increasing demand for data science professionals, universities offer various data science and big data courses to prepare students for the tech industry. Research projects are a crucial part of these programs and a well- executed data science project can make your CV appear more robust and compelling. A broad range of data science topics exist that offer exciting possibilities for research but choosing data science research topics can be a real challenge for students . After all, a good research project relies first and foremost on data analytics research topics that draw upon both mono-disciplinary and multi-disciplinary research to explore endless possibilities for real –world applications.
As one of the top-most masters and PhD online dissertation writing services , we are geared to assist students in the entire research process right from the initial conception to the final execution to ensure that you have a truly fulfilling and enriching research experience. These resources are also helpful for those students who are taking online classes .
By taking advantage of our best digital marketing research topics in data science you can be assured of producing an innovative research project that will impress your research professors and make a huge difference in attracting the right employers.
Get an Immediate Response
Discuss your requirments with our writers
Get 3 Customize Research Topic within 24 Hours
Data science thesis topics.
We have compiled a list of data science research topics for students studying data science that can be utilized in data science projects in 2022. our team of professional data experts have brought together master or MBA thesis topics in data science that cater to core areas driving the field of data science and big data that will relieve all your research anxieties and provide a solid grounding for an interesting research projects . The article will feature data science thesis ideas that can be immensely beneficial for students as they cover a broad research agenda for future data science . These ideas have been drawn from the 8 v’s of big data namely Volume, Value, Veracity, Visualization, Variety, Velocity, Viscosity, and Virility that provide interesting and challenging research areas for prospective researches in their masters or PhD thesis . Overall, the general big data research topics can be divided into distinct categories to facilitate the research topic selection process.
- Security and privacy issues
- Cloud Computing Platforms for Big Data Adoption and Analytics
- Real-time data analytics for processing of image , video and text
- Modeling uncertainty
How “The Research Guardian” Can Help You A lot!
Our top thesis writing experts are available 24/7 to assist you the right university projects. Whether its critical literature reviews to complete your PhD. or Master Levels thesis.
DATA SCIENCE PHD RESEARCH TOPICS
The article will also guide students engaged in doctoral research by introducing them to an outstanding list of data science thesis topics that can lead to major real-time applications of big data analytics in your research projects.
- Intelligent traffic control ; Gathering and monitoring traffic information using CCTV images.
- Asymmetric protected storage methodology over multi-cloud service providers in Big data.
- Leveraging disseminated data over big data analytics environment.
- Internet of Things.
- Large-scale data system and anomaly detection.
What makes us a unique research service for your research needs?
We offer all –round and superb research services that have a distinguished track record in helping students secure their desired grades in research projects in big data analytics and hence pave the way for a promising career ahead. These are the features that set us apart in the market for research services that effectively deal with all significant issues in your research for.
- Plagiarism –free ; We strictly adhere to a non-plagiarism policy in all our research work to provide you with well-written, original content with low similarity index to maximize chances of acceptance of your research submissions.
- Publication; We don’t just suggest PhD data science research topics but our PhD consultancy services take your research to the next level by ensuring its publication in well-reputed journals. A PhD thesis is indispensable for a PhD degree and with our premier best PhD thesis services that tackle all aspects of research writing and cater to essential requirements of journals , we will bring you closer to your dream of being a PhD in the field of data analytics.
- Research ethics: Solid research ethics lie at the core of our services where we actively seek to protect the privacy and confidentiality of the technical and personal information of our valued customers.
- Research experience: We take pride in our world –class team of computing industry professionals equipped with the expertise and experience to assist in choosing data science research topics and subsequent phases in research including findings solutions, code development and final manuscript writing.
- Business ethics: We are driven by a business philosophy that‘s wholly committed to achieving total customer satisfaction by providing constant online and offline support and timely submissions so that you can keep track of the progress of your research.
Now, we’ll proceed to cover specific research problems encompassing both data analytics research topics and big data thesis topics that have applications across multiple domains.
Get Help from Expert Thesis Writers!
TheresearchGuardian.com providing expert thesis assistance for university students at any sort of level. Our thesis writing service has been serving students since 2011.
Multi-modal Transfer Learning for Cross-Modal Information Retrieval
Aim and objectives.
The research aims to examine and explore the use of CMR approach in bringing about a flexible retrieval experience by combining data across different modalities to ensure abundant multimedia data.
- Develop methods to enable learning across different modalities in shared cross modal spaces comprising texts and images as well as consider the limitations of existing cross –modal retrieval algorithms.
- Investigate the presence and effects of bias in cross modal transfer learning and suggesting strategies for bias detection and mitigation.
- Develop a tool with query expansion and relevance feedback capabilities to facilitate search and retrieval of multi-modal data.
- Investigate the methods of multi modal learning and elaborate on the importance of multi-modal deep learning to provide a comprehensive learning experience.
The Role of Machine Learning in Facilitating the Implication of the Scientific Computing and Software Engineering
- Evaluate how machine learning leads to improvements in computational APA reference generator tools and thus aids in the implementation of scientific computing
- Evaluating the effectiveness of machine learning in solving complex problems and improving the efficiency of scientific computing and software engineering processes.
- Assessing the potential benefits and challenges of using machine learning in these fields, including factors such as cost, accuracy, and scalability.
- Examining the ethical and social implications of using machine learning in scientific computing and software engineering, such as issues related to bias, transparency, and accountability.
Trustworthy AI
The research aims to explore the crucial role of data science in advancing scientific goals and solving problems as well as the implications involved in use of AI systems especially with respect to ethical concerns.
- Investigate the value of digital infrastructures available through open data in aiding sharing and inter linking of data for enhanced global collaborative research efforts
- Provide explanations of the outcomes of a machine learning model for a meaningful interpretation to build trust among users about the reliability and authenticity of data
- Investigate how formal models can be used to verify and establish the efficacy of the results derived from probabilistic model.
- Review the concept of Trustworthy computing as a relevant framework for addressing the ethical concerns associated with AI systems.
The Implementation of Data Science and their impact on the management environment and sustainability
The aim of the research is to demonstrate how data science and analytics can be leveraged in achieving sustainable development.
- To examine the implementation of data science using data-driven decision-making tools
- To evaluate the impact of modern information technology on management environment and sustainability.
- To examine the use of data science in achieving more effective and efficient environment management
- Explore how data science and analytics can be used to achieve sustainability goals across three dimensions of economic, social and environmental.
Big data analytics in healthcare systems
The aim of the research is to examine the application of creating smart healthcare systems and how it can lead to more efficient, accessible and cost –effective health care.
- Identify the potential Areas or opportunities in big data to transform the healthcare system such as for diagnosis, treatment planning, or drug development.
- Assessing the potential benefits and challenges of using AI and deep learning in healthcare, including factors such as cost, efficiency, and accessibility
- Evaluating the effectiveness of AI and deep learning in improving patient outcomes, such as reducing morbidity and mortality rates, improving accuracy and speed of diagnoses, or reducing medical errors
- Examining the ethical and social implications of using AI and deep learning in healthcare, such as issues related to bias, privacy, and autonomy.
Large-Scale Data-Driven Financial Risk Assessment
The research aims to explore the possibility offered by big data in a consistent and real time assessment of financial risks.
- Investigate how the use of big data can help to identify and forecast risks that can harm a business.
- Categories the types of financial risks faced by companies.
- Describe the importance of financial risk management for companies in business terms.
- Train a machine learning model to classify transactions as fraudulent or genuine.
Scalable Architectures for Parallel Data Processing
Big data has exposed us to an ever –growing volume of data which cannot be handled through traditional data management and analysis systems. This has given rise to the use of scalable system architectures to efficiently process big data and exploit its true value. The research aims to analyses the current state of practice in scalable architectures and identify common patterns and techniques to design scalable architectures for parallel data processing.
- To design and implement a prototype scalable architecture for parallel data processing
- To evaluate the performance and scalability of the prototype architecture using benchmarks and real-world datasets
- To compare the prototype architecture with existing solutions and identify its strengths and weaknesses
- To evaluate the trade-offs and limitations of different scalable architectures for parallel data processing
- To provide recommendations for the use of the prototype architecture in different scenarios, such as batch processing, stream processing, and interactive querying
Robotic manipulation modelling
The aim of this research is to develop and validate a model-based control approach for robotic manipulation of small, precise objects.
- Develop a mathematical model of the robotic system that captures the dynamics of the manipulator and the grasped object.
- Design a control algorithm that uses the developed model to achieve stable and accurate grasping of the object.
- Test the proposed approach in simulation and validate the results through experiments with a physical robotic system.
- Evaluate the performance of the proposed approach in terms of stability, accuracy, and robustness to uncertainties and perturbations.
- Identify potential applications and areas for future work in the field of robotic manipulation for precision tasks.
Big data analytics and its impacts on marketing strategy
The aim of this research is to investigate the impact of big data analytics on marketing strategy and to identify best practices for leveraging this technology to inform decision-making.
- Review the literature on big data analytics and marketing strategy to identify key trends and challenges
- Conduct a case study analysis of companies that have successfully integrated big data analytics into their marketing strategies
- Identify the key factors that contribute to the effectiveness of big data analytics in marketing decision-making
- Develop a framework for integrating big data analytics into marketing strategy.
- Investigate the ethical implications of big data analytics in marketing and suggest best practices for responsible use of this technology.
Looking For Customize Thesis Topics?
Take a review of different varieties of thesis topics and samples from our website TheResearchGuardian.com on multiple subjects for every educational level.
Platforms for large scale data computing: big data analysis and acceptance
To investigate the performance and scalability of different large-scale data computing platforms.
- To compare the features and capabilities of different platforms and determine which is most suitable for a given use case.
- To identify best practices for using these platforms, including considerations for data management, security, and cost.
- To explore the potential for integrating these platforms with other technologies and tools for data analysis and visualization.
- To develop case studies or practical examples of how these platforms have been used to solve real-world data analysis challenges.
Distributed data clustering
Distributed data clustering can be a useful approach for analyzing and understanding complex datasets, as it allows for the identification of patterns and relationships that may not be immediately apparent.
To develop and evaluate new algorithms for distributed data clustering that is efficient and scalable.
- To compare the performance and accuracy of different distributed data clustering algorithms on a variety of datasets.
- To investigate the impact of different parameters and settings on the performance of distributed data clustering algorithms.
- To explore the potential for integrating distributed data clustering with other machine learning and data analysis techniques.
- To apply distributed data clustering to real-world problems and evaluate its effectiveness.
Analyzing and predicting urbanization patterns using GIS and data mining techniques".
The aim of this project is to use GIS and data mining techniques to analyze and predict urbanization patterns in a specific region.
- To collect and process relevant data on urbanization patterns, including population density, land use, and infrastructure development, using GIS tools.
- To apply data mining techniques, such as clustering and regression analysis, to identify trends and patterns in the data.
- To use the results of the data analysis to develop a predictive model for urbanization patterns in the region.
- To present the results of the analysis and the predictive model in a clear and visually appealing way, using GIS maps and other visualization techniques.
Use of big data and IOT in the media industry
Big data and the Internet of Things (IoT) are emerging technologies that are transforming the way that information is collected, analyzed, and disseminated in the media sector. The aim of the research is to understand how big data and IoT re used to dictate information flow in the media industry
- Identifying the key ways in which big data and IoT are being used in the media sector, such as for content creation, audience engagement, or advertising.
- Analyzing the benefits and challenges of using big data and IoT in the media industry, including factors such as cost, efficiency, and effectiveness.
- Examining the ethical and social implications of using big data and IoT in the media sector, including issues such as privacy, security, and bias.
- Determining the potential impact of big data and IoT on the media landscape and the role of traditional media in an increasingly digital world.
Exigency computer systems for meteorology and disaster prevention
The research aims to explore the role of exigency computer systems to detect weather and other hazards for disaster prevention and response
- Identifying the key components and features of exigency computer systems for meteorology and disaster prevention, such as data sources, analytics tools, and communication channels.
- Evaluating the effectiveness of exigency computer systems in providing accurate and timely information about weather and other hazards.
- Assessing the impact of exigency computer systems on the ability of decision makers to prepare for and respond to disasters.
- Examining the challenges and limitations of using exigency computer systems, such as the need for reliable data sources, the complexity of the systems, or the potential for human error.
Network security and cryptography
Overall, the goal of research is to improve our understanding of how to protect communication and information in the digital age, and to develop practical solutions for addressing the complex and evolving security challenges faced by individuals, organizations, and societies.
- Developing new algorithms and protocols for securing communication over networks, such as for data confidentiality, data integrity, and authentication
- Investigating the security of existing cryptographic primitives, such as encryption and hashing algorithms, and identifying vulnerabilities that could be exploited by attackers.
- Evaluating the effectiveness of different network security technologies and protocols, such as firewalls, intrusion detection systems, and virtual private networks (VPNs), in protecting against different types of attacks.
- Exploring the use of cryptography in emerging areas, such as cloud computing, the Internet of Things (IoT), and blockchain, and identifying the unique security challenges and opportunities presented by these domains.
- Investigating the trade-offs between security and other factors, such as performance, usability, and cost, and developing strategies for balancing these conflicting priorities.
Meet Our Professionals Ranging From Renowned Universities
Related topics.
- Sports Management Research Topics
- Special Education Research Topics
- Software Engineering Research Topics
- Primary Education Research Topics
- Microbiology Research Topics
- Luxury Brand Research Topics
- Cyber Security Research Topics
- Commercial Law Research Topics
- Change Management Research Topics
- Artificial intelligence Research Topics
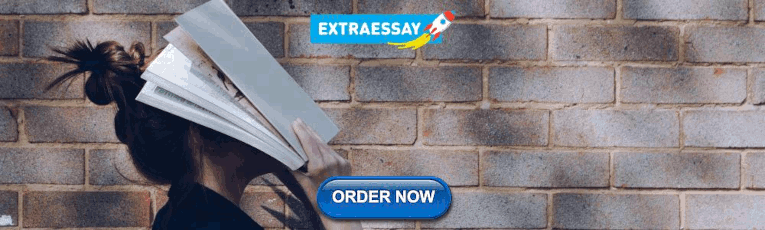
IMAGES
VIDEO
COMMENTS
A comprehensive list of data science and analytics-related research topics. Includes free access to a webinar and research topic evaluator.
37 Research Topics in Data Science. 1.) Predictive modeling is a significant portion of data science and a topic you must be aware of. Simply put, it is the process of using historical data to build models that can predict future outcomes.
In this article, we have listed 10 such research and thesis topic ideas to take up as data science projects in 2022. Handling practical video analytics in a distributed cloud: With increased dependency on the internet, sharing videos has become a mode of data and information exchange.
Explore unique Data Science dissertation topics. Learn the importance of data science research, get writing tips, and find dissertation ideas.
Explore cutting-edge data science research topics for master ’s degree. Dive into areas like machine learning, data visualization, predictive analytics, and natural...
Use the LaTeX template provided by the University of Helsinki (or your institution). If you are MSc Data Science student, you can find the template in the Moodle course Data Science MSc Thesis. For example, you can use Overleaf as an easy entry to LaTeX.
99+ Data Science Research Topics Ideas: Category Wise. Supervised Machine Learning. Predictive modeling for disease outbreak prediction. Credit scoring using machine learning for financial institutions. Sentiment analysis for stock market predictions. Recommender systems for personalized content recommendations.
Latest data science research topics are listed for Masters and PhD students for free. Beside this, get customized big data thesis topics from experts.
Choosing a Research Topic. Deciding on and clearly formulating an innovative, relevant and manageable research problem is in many cases the most challenging aspect of the entire process. Perhaps, you will need to write your thesis for a company or research initiative where you will get more guidance and directions from your supervisors.
Thesis topics for the master thesis Data Science and Business Analytics. Topic 1: Logistic regression for modern data structures. Promotor. Gerda Claeskens. Description. Logistic regression is widely used for binary classification.